PhD Thesis Defence
Exploiting the interplay between visual and textual data for scene interpretation.
I successfully defended my PhD the 08/10/2020 and got an excellent Cum Laude. In this page the thesis pdf, the presentation slides, and a video of the presentation are made available.
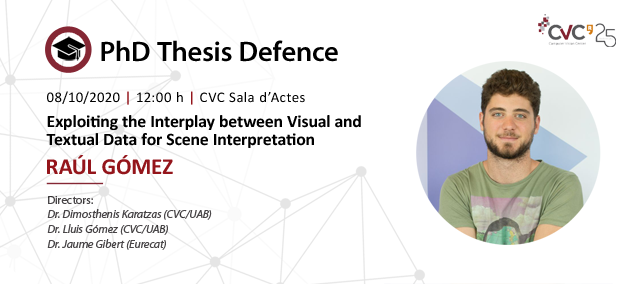
Thesis Slides
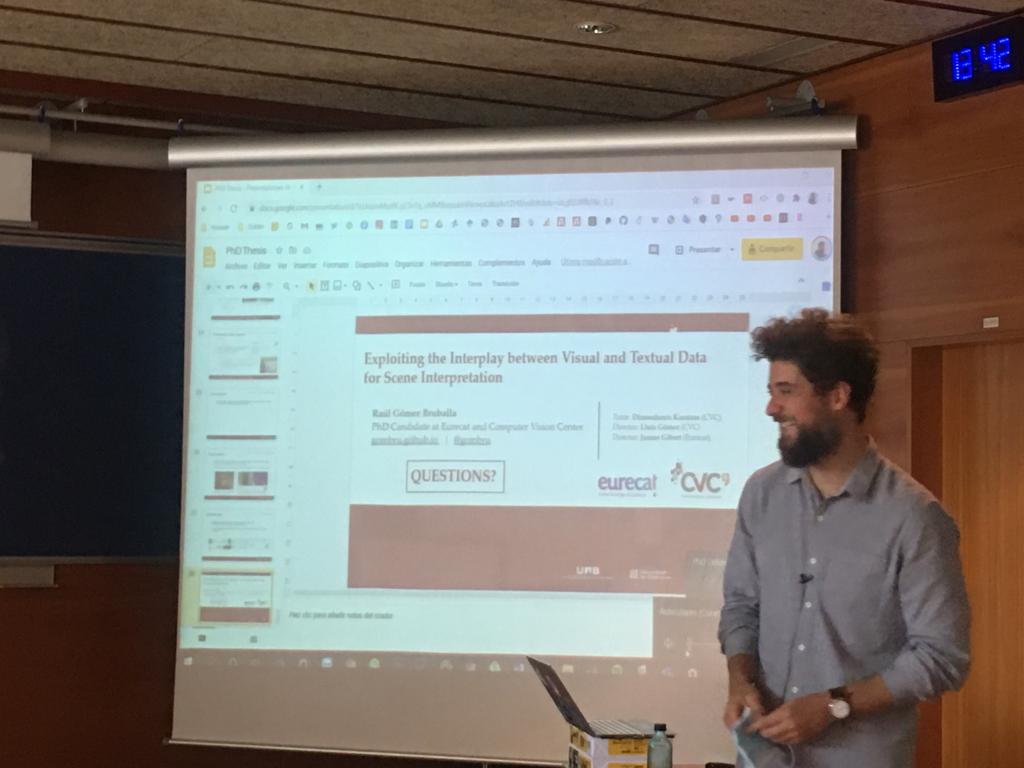
Dr. Josep Lladós (Centre de Visió per Computador, Universitat Autònoma de Barcelona)
Dr. Diane Larlus (Naver Labs Europe)
Dr. Francesc Moreno (Universitat Politècnica de Cataunya, Institut de Robòtica i Informàtica Industrial)
Thesis Director:
Dr. Dimosthenis Karatzas (Centre de Visió per Computador, Universitat Autònoma de Barcelona)
Thesis Co-Directors:
Dr. Lluis Gómez (Centre de Visió per Computador, Universitat Autònoma de Barcelona)
Dr. Jaume Gibert (Eurecat)
Machine learning experimentation under controlled scenarios and standard datasets is necessary to compare algorithms performance by evaluating all of them in the same setup. However, experimentation on how those algorithms perform on unconstrained data and applied tasks to solve real world problems is also a must to ascertain how that research can contribute to our society.
In this dissertation we experiment with the latest computer vision and natural language processing algorithms applying them to multimodal scene interpretation. Particularly, we research on how image and text understanding can be jointly exploited to address real world problems, focusing on learning from Social Media data.
We address several tasks that involve image and textual information, discuss their characteristics and offer our experimentation conclusions. First, we work on detection of scene text in images. Then, we work with Social Media posts, exploiting the captions associated to images as supervision to learn visual features, which we apply to multimodal semantic image retrieval. Subsequently, we work with geolocated Social Media images with associated tags, experimenting on how to use the tags as supervision, on location sensitive image retrieval and on exploiting location information for image tagging. Finally, we work on a specific classification problem of Social Media publications consisting on an image and a text: Multimodal hate speech classification.

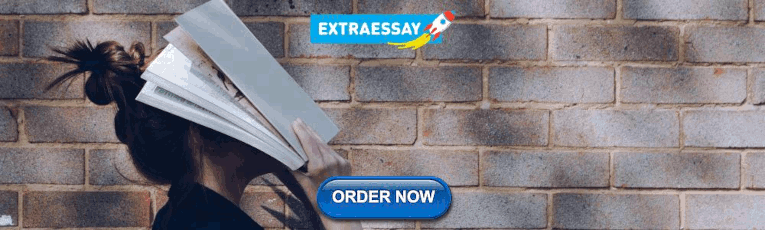
The Future of AI Research: 20 Thesis Ideas for Undergraduate Students in Machine Learning and Deep Learning for 2023!
A comprehensive guide for crafting an original and innovative thesis in the field of ai..
By Aarafat Islam on 2023-01-11
“The beauty of machine learning is that it can be applied to any problem you want to solve, as long as you can provide the computer with enough examples.” — Andrew Ng
This article provides a list of 20 potential thesis ideas for an undergraduate program in machine learning and deep learning in 2023. Each thesis idea includes an introduction , which presents a brief overview of the topic and the research objectives . The ideas provided are related to different areas of machine learning and deep learning, such as computer vision, natural language processing, robotics, finance, drug discovery, and more. The article also includes explanations, examples, and conclusions for each thesis idea, which can help guide the research and provide a clear understanding of the potential contributions and outcomes of the proposed research. The article also emphasized the importance of originality and the need for proper citation in order to avoid plagiarism.
1. Investigating the use of Generative Adversarial Networks (GANs) in medical imaging: A deep learning approach to improve the accuracy of medical diagnoses.
Introduction: Medical imaging is an important tool in the diagnosis and treatment of various medical conditions. However, accurately interpreting medical images can be challenging, especially for less experienced doctors. This thesis aims to explore the use of GANs in medical imaging, in order to improve the accuracy of medical diagnoses.
2. Exploring the use of deep learning in natural language generation (NLG): An analysis of the current state-of-the-art and future potential.
Introduction: Natural language generation is an important field in natural language processing (NLP) that deals with creating human-like text automatically. Deep learning has shown promising results in NLP tasks such as machine translation, sentiment analysis, and question-answering. This thesis aims to explore the use of deep learning in NLG and analyze the current state-of-the-art models, as well as potential future developments.
3. Development and evaluation of deep reinforcement learning (RL) for robotic navigation and control.
Introduction: Robotic navigation and control are challenging tasks, which require a high degree of intelligence and adaptability. Deep RL has shown promising results in various robotics tasks, such as robotic arm control, autonomous navigation, and manipulation. This thesis aims to develop and evaluate a deep RL-based approach for robotic navigation and control and evaluate its performance in various environments and tasks.
4. Investigating the use of deep learning for drug discovery and development.
Introduction: Drug discovery and development is a time-consuming and expensive process, which often involves high failure rates. Deep learning has been used to improve various tasks in bioinformatics and biotechnology, such as protein structure prediction and gene expression analysis. This thesis aims to investigate the use of deep learning for drug discovery and development and examine its potential to improve the efficiency and accuracy of the drug development process.
5. Comparison of deep learning and traditional machine learning methods for anomaly detection in time series data.
Introduction: Anomaly detection in time series data is a challenging task, which is important in various fields such as finance, healthcare, and manufacturing. Deep learning methods have been used to improve anomaly detection in time series data, while traditional machine learning methods have been widely used as well. This thesis aims to compare deep learning and traditional machine learning methods for anomaly detection in time series data and examine their respective strengths and weaknesses.
Photo by Joanna Kosinska on Unsplash
6. Use of deep transfer learning in speech recognition and synthesis.
Introduction: Speech recognition and synthesis are areas of natural language processing that focus on converting spoken language to text and vice versa. Transfer learning has been widely used in deep learning-based speech recognition and synthesis systems to improve their performance by reusing the features learned from other tasks. This thesis aims to investigate the use of transfer learning in speech recognition and synthesis and how it improves the performance of the system in comparison to traditional methods.
7. The use of deep learning for financial prediction.
Introduction: Financial prediction is a challenging task that requires a high degree of intelligence and adaptability, especially in the field of stock market prediction. Deep learning has shown promising results in various financial prediction tasks, such as stock price prediction and credit risk analysis. This thesis aims to investigate the use of deep learning for financial prediction and examine its potential to improve the accuracy of financial forecasting.
8. Investigating the use of deep learning for computer vision in agriculture.
Introduction: Computer vision has the potential to revolutionize the field of agriculture by improving crop monitoring, precision farming, and yield prediction. Deep learning has been used to improve various computer vision tasks, such as object detection, semantic segmentation, and image classification. This thesis aims to investigate the use of deep learning for computer vision in agriculture and examine its potential to improve the efficiency and accuracy of crop monitoring and precision farming.
9. Development and evaluation of deep learning models for generative design in engineering and architecture.
Introduction: Generative design is a powerful tool in engineering and architecture that can help optimize designs and reduce human error. Deep learning has been used to improve various generative design tasks, such as design optimization and form generation. This thesis aims to develop and evaluate deep learning models for generative design in engineering and architecture and examine their potential to improve the efficiency and accuracy of the design process.
10. Investigating the use of deep learning for natural language understanding.
Introduction: Natural language understanding is a complex task of natural language processing that involves extracting meaning from text. Deep learning has been used to improve various NLP tasks, such as machine translation, sentiment analysis, and question-answering. This thesis aims to investigate the use of deep learning for natural language understanding and examine its potential to improve the efficiency and accuracy of natural language understanding systems.
Photo by UX Indonesia on Unsplash
11. Comparing deep learning and traditional machine learning methods for image compression.
Introduction: Image compression is an important task in image processing and computer vision. It enables faster data transmission and storage of image files. Deep learning methods have been used to improve image compression, while traditional machine learning methods have been widely used as well. This thesis aims to compare deep learning and traditional machine learning methods for image compression and examine their respective strengths and weaknesses.
12. Using deep learning for sentiment analysis in social media.
Introduction: Sentiment analysis in social media is an important task that can help businesses and organizations understand their customers’ opinions and feedback. Deep learning has been used to improve sentiment analysis in social media, by training models on large datasets of social media text. This thesis aims to use deep learning for sentiment analysis in social media, and evaluate its performance against traditional machine learning methods.
13. Investigating the use of deep learning for image generation.
Introduction: Image generation is a task in computer vision that involves creating new images from scratch or modifying existing images. Deep learning has been used to improve various image generation tasks, such as super-resolution, style transfer, and face generation. This thesis aims to investigate the use of deep learning for image generation and examine its potential to improve the quality and diversity of generated images.
14. Development and evaluation of deep learning models for anomaly detection in cybersecurity.
Introduction: Anomaly detection in cybersecurity is an important task that can help detect and prevent cyber-attacks. Deep learning has been used to improve various anomaly detection tasks, such as intrusion detection and malware detection. This thesis aims to develop and evaluate deep learning models for anomaly detection in cybersecurity and examine their potential to improve the efficiency and accuracy of cybersecurity systems.
15. Investigating the use of deep learning for natural language summarization.
Introduction: Natural language summarization is an important task in natural language processing that involves creating a condensed version of a text that preserves its main meaning. Deep learning has been used to improve various natural language summarization tasks, such as document summarization and headline generation. This thesis aims to investigate the use of deep learning for natural language summarization and examine its potential to improve the efficiency and accuracy of natural language summarization systems.
Photo by Windows on Unsplash
16. Development and evaluation of deep learning models for facial expression recognition.
Introduction: Facial expression recognition is an important task in computer vision and has many practical applications, such as human-computer interaction, emotion recognition, and psychological studies. Deep learning has been used to improve facial expression recognition, by training models on large datasets of images. This thesis aims to develop and evaluate deep learning models for facial expression recognition and examine their performance against traditional machine learning methods.
17. Investigating the use of deep learning for generative models in music and audio.
Introduction: Music and audio synthesis is an important task in audio processing, which has many practical applications, such as music generation and speech synthesis. Deep learning has been used to improve generative models for music and audio, by training models on large datasets of audio data. This thesis aims to investigate the use of deep learning for generative models in music and audio and examine its potential to improve the quality and diversity of generated audio.
18. Study the comparison of deep learning models with traditional algorithms for anomaly detection in network traffic.
Introduction: Anomaly detection in network traffic is an important task that can help detect and prevent cyber-attacks. Deep learning models have been used for this task, and traditional methods such as clustering and rule-based systems are widely used as well. This thesis aims to compare deep learning models with traditional algorithms for anomaly detection in network traffic and analyze the trade-offs between the models in terms of accuracy and scalability.
19. Investigating the use of deep learning for improving recommender systems.
Introduction: Recommender systems are widely used in many applications such as online shopping, music streaming, and movie streaming. Deep learning has been used to improve the performance of recommender systems, by training models on large datasets of user-item interactions. This thesis aims to investigate the use of deep learning for improving recommender systems and compare its performance with traditional content-based and collaborative filtering approaches.
20. Development and evaluation of deep learning models for multi-modal data analysis.
Introduction: Multi-modal data analysis is the task of analyzing and understanding data from multiple sources such as text, images, and audio. Deep learning has been used to improve multi-modal data analysis, by training models on large datasets of multi-modal data. This thesis aims to develop and evaluate deep learning models for multi-modal data analysis and analyze their potential to improve performance in comparison to single-modal models.
I hope that this article has provided you with a useful guide for your thesis research in machine learning and deep learning. Remember to conduct a thorough literature review and to include proper citations in your work, as well as to be original in your research to avoid plagiarism. I wish you all the best of luck with your thesis and your research endeavors!
Continue Learning
Understanding the mechanics: how ai art generators produce unique artworks, 100 valuable chatgpt prompts to boost startups and businesses.
Spark your startups and businesses with these invaluable ChatGPT prompts.
ChatGPT-4 + Midjourney V5: A Heavenly Match for Image Gen
Analyzing the Capabilities of ChatGPT-4 and MidJourney V5
AI-Powered Chatbots: Design and Implementation Strategies
Generative ai breakthroughs: canada's role in generative ai advancements, how ai is altering our memories and perception of reality.
Advertisement
Deep Learning: A Comprehensive Overview on Techniques, Taxonomy, Applications and Research Directions
- Review Article
- Published: 18 August 2021
- Volume 2 , article number 420 , ( 2021 )
Cite this article
- Iqbal H. Sarker ORCID: orcid.org/0000-0003-1740-5517 1 , 2
253k Accesses
986 Citations
24 Altmetric
Explore all metrics
Deep learning (DL), a branch of machine learning (ML) and artificial intelligence (AI) is nowadays considered as a core technology of today’s Fourth Industrial Revolution (4IR or Industry 4.0). Due to its learning capabilities from data, DL technology originated from artificial neural network (ANN), has become a hot topic in the context of computing, and is widely applied in various application areas like healthcare, visual recognition, text analytics, cybersecurity, and many more. However, building an appropriate DL model is a challenging task, due to the dynamic nature and variations in real-world problems and data. Moreover, the lack of core understanding turns DL methods into black-box machines that hamper development at the standard level. This article presents a structured and comprehensive view on DL techniques including a taxonomy considering various types of real-world tasks like supervised or unsupervised. In our taxonomy, we take into account deep networks for supervised or discriminative learning , unsupervised or generative learning as well as hybrid learning and relevant others. We also summarize real-world application areas where deep learning techniques can be used. Finally, we point out ten potential aspects for future generation DL modeling with research directions . Overall, this article aims to draw a big picture on DL modeling that can be used as a reference guide for both academia and industry professionals.
Similar content being viewed by others
Deep Learning: History and State-of-the-Arts
Machine Learning and Deep Learning: A Comparative Review
Future and discussions, explore related subjects.
- Artificial Intelligence
Avoid common mistakes on your manuscript.
Introduction
In the late 1980s, neural networks became a prevalent topic in the area of Machine Learning (ML) as well as Artificial Intelligence (AI), due to the invention of various efficient learning methods and network structures [ 52 ]. Multilayer perceptron networks trained by “Backpropagation” type algorithms, self-organizing maps, and radial basis function networks were such innovative methods [ 26 , 36 , 37 ]. While neural networks are successfully used in many applications, the interest in researching this topic decreased later on. After that, in 2006, “Deep Learning” (DL) was introduced by Hinton et al. [ 41 ], which was based on the concept of artificial neural network (ANN). Deep learning became a prominent topic after that, resulting in a rebirth in neural network research, hence, some times referred to as “new-generation neural networks”. This is because deep networks, when properly trained, have produced significant success in a variety of classification and regression challenges [ 52 ].
Nowadays, DL technology is considered as one of the hot topics within the area of machine learning, artificial intelligence as well as data science and analytics, due to its learning capabilities from the given data. Many corporations including Google, Microsoft, Nokia, etc., study it actively as it can provide significant results in different classification and regression problems and datasets [ 52 ]. In terms of working domain, DL is considered as a subset of ML and AI, and thus DL can be seen as an AI function that mimics the human brain’s processing of data. The worldwide popularity of “Deep learning” is increasing day by day, which is shown in our earlier paper [ 96 ] based on the historical data collected from Google trends [ 33 ]. Deep learning differs from standard machine learning in terms of efficiency as the volume of data increases, discussed briefly in Section “ Why Deep Learning in Today's Research and Applications? ”. DL technology uses multiple layers to represent the abstractions of data to build computational models. While deep learning takes a long time to train a model due to a large number of parameters, it takes a short amount of time to run during testing as compared to other machine learning algorithms [ 127 ].
While today’s Fourth Industrial Revolution (4IR or Industry 4.0) is typically focusing on technology-driven “automation, smart and intelligent systems”, DL technology, which is originated from ANN, has become one of the core technologies to achieve the goal [ 103 , 114 ]. A typical neural network is mainly composed of many simple, connected processing elements or processors called neurons, each of which generates a series of real-valued activations for the target outcome. Figure 1 shows a schematic representation of the mathematical model of an artificial neuron, i.e., processing element, highlighting input ( \(X_i\) ), weight ( w ), bias ( b ), summation function ( \(\sum\) ), activation function ( f ) and corresponding output signal ( y ). Neural network-based DL technology is now widely applied in many fields and research areas such as healthcare, sentiment analysis, natural language processing, visual recognition, business intelligence, cybersecurity, and many more that have been summarized in the latter part of this paper.
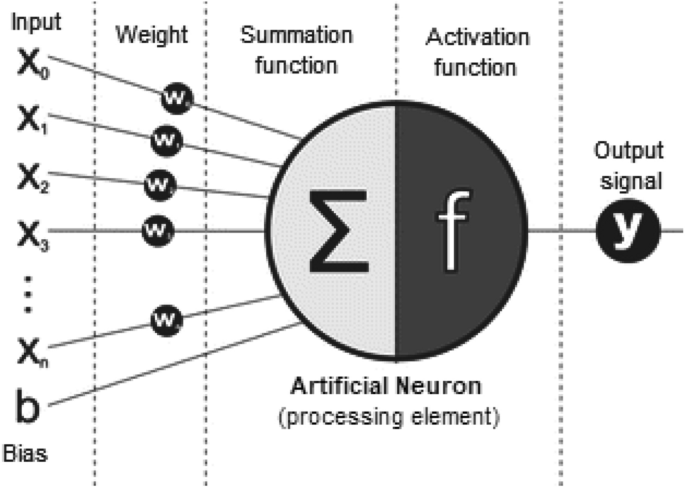
Schematic representation of the mathematical model of an artificial neuron (processing element), highlighting input ( \(X_i\) ), weight ( w ), bias ( b ), summation function ( \(\sum\) ), activation function ( f ) and output signal ( y )
Although DL models are successfully applied in various application areas, mentioned above, building an appropriate model of deep learning is a challenging task, due to the dynamic nature and variations of real-world problems and data. Moreover, DL models are typically considered as “black-box” machines that hamper the standard development of deep learning research and applications. Thus for clear understanding, in this paper, we present a structured and comprehensive view on DL techniques considering the variations in real-world problems and tasks. To achieve our goal, we briefly discuss various DL techniques and present a taxonomy by taking into account three major categories: (i) deep networks for supervised or discriminative learning that is utilized to provide a discriminative function in supervised deep learning or classification applications; (ii) deep networks for unsupervised or generative learning that are used to characterize the high-order correlation properties or features for pattern analysis or synthesis, thus can be used as preprocessing for the supervised algorithm; and (ii) deep networks for hybrid learning that is an integration of both supervised and unsupervised model and relevant others. We take into account such categories based on the nature and learning capabilities of different DL techniques and how they are used to solve problems in real-world applications [ 97 ]. Moreover, identifying key research issues and prospects including effective data representation, new algorithm design, data-driven hyper-parameter learning, and model optimization, integrating domain knowledge, adapting resource-constrained devices, etc. is one of the key targets of this study, which can lead to “Future Generation DL-Modeling”. Thus the goal of this paper is set to assist those in academia and industry as a reference guide, who want to research and develop data-driven smart and intelligent systems based on DL techniques.
The overall contribution of this paper is summarized as follows:
This article focuses on different aspects of deep learning modeling, i.e., the learning capabilities of DL techniques in different dimensions such as supervised or unsupervised tasks, to function in an automated and intelligent manner, which can play as a core technology of today’s Fourth Industrial Revolution (Industry 4.0).
We explore a variety of prominent DL techniques and present a taxonomy by taking into account the variations in deep learning tasks and how they are used for different purposes. In our taxonomy, we divide the techniques into three major categories such as deep networks for supervised or discriminative learning, unsupervised or generative learning, as well as deep networks for hybrid learning, and relevant others.
We have summarized several potential real-world application areas of deep learning, to assist developers as well as researchers in broadening their perspectives on DL techniques. Different categories of DL techniques highlighted in our taxonomy can be used to solve various issues accordingly.
Finally, we point out and discuss ten potential aspects with research directions for future generation DL modeling in terms of conducting future research and system development.
This paper is organized as follows. Section “ Why Deep Learning in Today's Research and Applications? ” motivates why deep learning is important to build data-driven intelligent systems. In Section“ Deep Learning Techniques and Applications ”, we present our DL taxonomy by taking into account the variations of deep learning tasks and how they are used in solving real-world issues and briefly discuss the techniques with summarizing the potential application areas. In Section “ Research Directions and Future Aspects ”, we discuss various research issues of deep learning-based modeling and highlight the promising topics for future research within the scope of our study. Finally, Section “ Concluding Remarks ” concludes this paper.
Why Deep Learning in Today’s Research and Applications?
The main focus of today’s Fourth Industrial Revolution (Industry 4.0) is typically technology-driven automation, smart and intelligent systems, in various application areas including smart healthcare, business intelligence, smart cities, cybersecurity intelligence, and many more [ 95 ]. Deep learning approaches have grown dramatically in terms of performance in a wide range of applications considering security technologies, particularly, as an excellent solution for uncovering complex architecture in high-dimensional data. Thus, DL techniques can play a key role in building intelligent data-driven systems according to today’s needs, because of their excellent learning capabilities from historical data. Consequently, DL can change the world as well as humans’ everyday life through its automation power and learning from experience. DL technology is therefore relevant to artificial intelligence [ 103 ], machine learning [ 97 ] and data science with advanced analytics [ 95 ] that are well-known areas in computer science, particularly, today’s intelligent computing. In the following, we first discuss regarding the position of deep learning in AI, or how DL technology is related to these areas of computing.
The Position of Deep Learning in AI
Nowadays, artificial intelligence (AI), machine learning (ML), and deep learning (DL) are three popular terms that are sometimes used interchangeably to describe systems or software that behaves intelligently. In Fig. 2 , we illustrate the position of deep Learning, comparing with machine learning and artificial intelligence. According to Fig. 2 , DL is a part of ML as well as a part of the broad area AI. In general, AI incorporates human behavior and intelligence to machines or systems [ 103 ], while ML is the method to learn from data or experience [ 97 ], which automates analytical model building. DL also represents learning methods from data where the computation is done through multi-layer neural networks and processing. The term “Deep” in the deep learning methodology refers to the concept of multiple levels or stages through which data is processed for building a data-driven model.
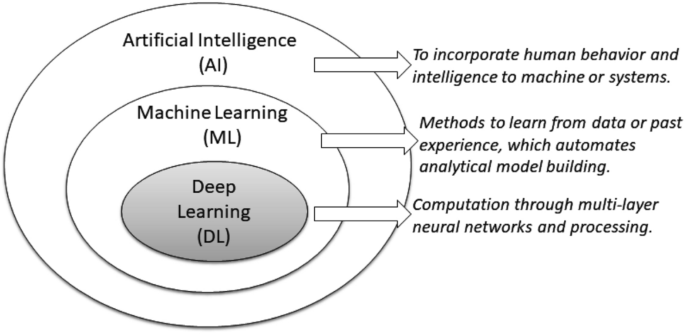
An illustration of the position of deep learning (DL), comparing with machine learning (ML) and artificial intelligence (AI)
Thus, DL can be considered as one of the core technology of AI, a frontier for artificial intelligence, which can be used for building intelligent systems and automation. More importantly, it pushes AI to a new level, termed “Smarter AI”. As DL are capable of learning from data, there is a strong relation of deep learning with “Data Science” [ 95 ] as well. Typically, data science represents the entire process of finding meaning or insights in data in a particular problem domain, where DL methods can play a key role for advanced analytics and intelligent decision-making [ 104 , 106 ]. Overall, we can conclude that DL technology is capable to change the current world, particularly, in terms of a powerful computational engine and contribute to technology-driven automation, smart and intelligent systems accordingly, and meets the goal of Industry 4.0.
Understanding Various Forms of Data
As DL models learn from data, an in-depth understanding and representation of data are important to build a data-driven intelligent system in a particular application area. In the real world, data can be in various forms, which typically can be represented as below for deep learning modeling:
Sequential Data Sequential data is any kind of data where the order matters, i,e., a set of sequences. It needs to explicitly account for the sequential nature of input data while building the model. Text streams, audio fragments, video clips, time-series data, are some examples of sequential data.
Image or 2D Data A digital image is made up of a matrix, which is a rectangular array of numbers, symbols, or expressions arranged in rows and columns in a 2D array of numbers. Matrix, pixels, voxels, and bit depth are the four essential characteristics or fundamental parameters of a digital image.
Tabular Data A tabular dataset consists primarily of rows and columns. Thus tabular datasets contain data in a columnar format as in a database table. Each column (field) must have a name and each column may only contain data of the defined type. Overall, it is a logical and systematic arrangement of data in the form of rows and columns that are based on data properties or features. Deep learning models can learn efficiently on tabular data and allow us to build data-driven intelligent systems.
The above-discussed data forms are common in the real-world application areas of deep learning. Different categories of DL techniques perform differently depending on the nature and characteristics of data, discussed briefly in Section “ Deep Learning Techniques and Applications ” with a taxonomy presentation. However, in many real-world application areas, the standard machine learning techniques, particularly, logic-rule or tree-based techniques [ 93 , 101 ] perform significantly depending on the application nature. Figure 3 also shows the performance comparison of DL and ML modeling considering the amount of data. In the following, we highlight several cases, where deep learning is useful to solve real-world problems, according to our main focus in this paper.
DL Properties and Dependencies
A DL model typically follows the same processing stages as machine learning modeling. In Fig. 4 , we have shown a deep learning workflow to solve real-world problems, which consists of three processing steps, such as data understanding and preprocessing, DL model building, and training, and validation and interpretation. However, unlike the ML modeling [ 98 , 108 ], feature extraction in the DL model is automated rather than manual. K-nearest neighbor, support vector machines, decision tree, random forest, naive Bayes, linear regression, association rules, k-means clustering, are some examples of machine learning techniques that are commonly used in various application areas [ 97 ]. On the other hand, the DL model includes convolution neural network, recurrent neural network, autoencoder, deep belief network, and many more, discussed briefly with their potential application areas in Section 3 . In the following, we discuss the key properties and dependencies of DL techniques, that are needed to take into account before started working on DL modeling for real-world applications.
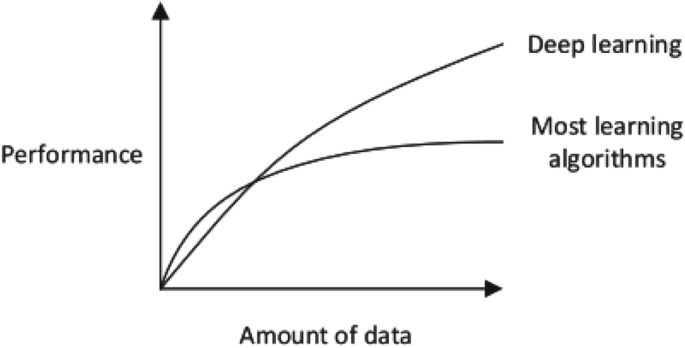
An illustration of the performance comparison between deep learning (DL) and other machine learning (ML) algorithms, where DL modeling from large amounts of data can increase the performance
Data Dependencies Deep learning is typically dependent on a large amount of data to build a data-driven model for a particular problem domain. The reason is that when the data volume is small, deep learning algorithms often perform poorly [ 64 ]. In such circumstances, however, the performance of the standard machine-learning algorithms will be improved if the specified rules are used [ 64 , 107 ].
Hardware Dependencies The DL algorithms require large computational operations while training a model with large datasets. As the larger the computations, the more the advantage of a GPU over a CPU, the GPU is mostly used to optimize the operations efficiently. Thus, to work properly with the deep learning training, GPU hardware is necessary. Therefore, DL relies more on high-performance machines with GPUs than standard machine learning methods [ 19 , 127 ].
Feature Engineering Process Feature engineering is the process of extracting features (characteristics, properties, and attributes) from raw data using domain knowledge. A fundamental distinction between DL and other machine-learning techniques is the attempt to extract high-level characteristics directly from data [ 22 , 97 ]. Thus, DL decreases the time and effort required to construct a feature extractor for each problem.
Model Training and Execution time In general, training a deep learning algorithm takes a long time due to a large number of parameters in the DL algorithm; thus, the model training process takes longer. For instance, the DL models can take more than one week to complete a training session, whereas training with ML algorithms takes relatively little time, only seconds to hours [ 107 , 127 ]. During testing, deep learning algorithms take extremely little time to run [ 127 ], when compared to certain machine learning methods.
Black-box Perception and Interpretability Interpretability is an important factor when comparing DL with ML. It’s difficult to explain how a deep learning result was obtained, i.e., “black-box”. On the other hand, the machine-learning algorithms, particularly, rule-based machine learning techniques [ 97 ] provide explicit logic rules (IF-THEN) for making decisions that are easily interpretable for humans. For instance, in our earlier works, we have presented several machines learning rule-based techniques [ 100 , 102 , 105 ], where the extracted rules are human-understandable and easier to interpret, update or delete according to the target applications.
The most significant distinction between deep learning and regular machine learning is how well it performs when data grows exponentially. An illustration of the performance comparison between DL and standard ML algorithms has been shown in Fig. 3 , where DL modeling can increase the performance with the amount of data. Thus, DL modeling is extremely useful when dealing with a large amount of data because of its capacity to process vast amounts of features to build an effective data-driven model. In terms of developing and training DL models, it relies on parallelized matrix and tensor operations as well as computing gradients and optimization. Several, DL libraries and resources [ 30 ] such as PyTorch [ 82 ] (with a high-level API called Lightning) and TensorFlow [ 1 ] (which also offers Keras as a high-level API) offers these core utilities including many pre-trained models, as well as many other necessary functions for implementation and DL model building.
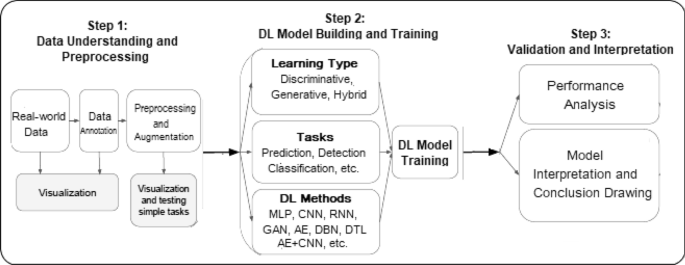
A typical DL workflow to solve real-world problems, which consists of three sequential stages (i) data understanding and preprocessing (ii) DL model building and training (iii) validation and interpretation
Deep Learning Techniques and Applications
In this section, we go through the various types of deep neural network techniques, which typically consider several layers of information-processing stages in hierarchical structures to learn. A typical deep neural network contains multiple hidden layers including input and output layers. Figure 5 shows a general structure of a deep neural network ( \(hidden \; layer=N\) and N \(\ge\) 2) comparing with a shallow network ( \(hidden \; layer=1\) ). We also present our taxonomy on DL techniques based on how they are used to solve various problems, in this section. However, before exploring the details of the DL techniques, it’s useful to review various types of learning tasks such as (i) Supervised: a task-driven approach that uses labeled training data, (ii) Unsupervised: a data-driven process that analyzes unlabeled datasets, (iii) Semi-supervised: a hybridization of both the supervised and unsupervised methods, and (iv) Reinforcement: an environment driven approach, discussed briefly in our earlier paper [ 97 ]. Thus, to present our taxonomy, we divide DL techniques broadly into three major categories: (i) deep networks for supervised or discriminative learning; (ii) deep networks for unsupervised or generative learning; and (ii) deep networks for hybrid learning combing both and relevant others, as shown in Fig. 6 . In the following, we briefly discuss each of these techniques that can be used to solve real-world problems in various application areas according to their learning capabilities.
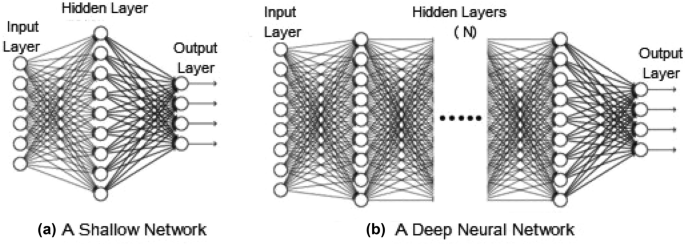
A general architecture of a a shallow network with one hidden layer and b a deep neural network with multiple hidden layers
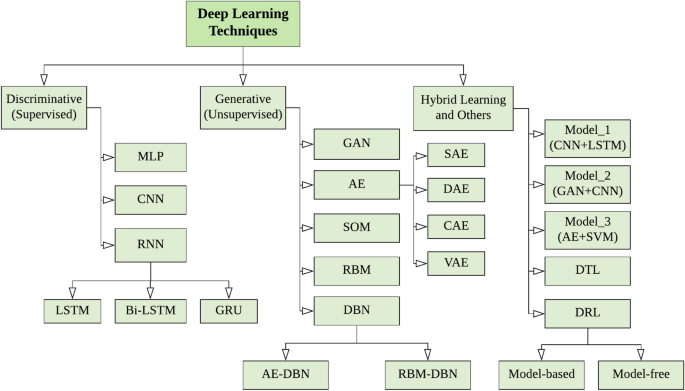
A taxonomy of DL techniques, broadly divided into three major categories (i) deep networks for supervised or discriminative learning, (ii) deep networks for unsupervised or generative learning, and (ii) deep networks for hybrid learning and relevant others
Deep Networks for Supervised or Discriminative Learning
This category of DL techniques is utilized to provide a discriminative function in supervised or classification applications. Discriminative deep architectures are typically designed to give discriminative power for pattern classification by describing the posterior distributions of classes conditioned on visible data [ 21 ]. Discriminative architectures mainly include Multi-Layer Perceptron (MLP), Convolutional Neural Networks (CNN or ConvNet), Recurrent Neural Networks (RNN), along with their variants. In the following, we briefly discuss these techniques.
Multi-layer Perceptron (MLP)
Multi-layer Perceptron (MLP), a supervised learning approach [ 83 ], is a type of feedforward artificial neural network (ANN). It is also known as the foundation architecture of deep neural networks (DNN) or deep learning. A typical MLP is a fully connected network that consists of an input layer that receives input data, an output layer that makes a decision or prediction about the input signal, and one or more hidden layers between these two that are considered as the network’s computational engine [ 36 , 103 ]. The output of an MLP network is determined using a variety of activation functions, also known as transfer functions, such as ReLU (Rectified Linear Unit), Tanh, Sigmoid, and Softmax [ 83 , 96 ]. To train MLP employs the most extensively used algorithm “Backpropagation” [ 36 ], a supervised learning technique, which is also known as the most basic building block of a neural network. During the training process, various optimization approaches such as Stochastic Gradient Descent (SGD), Limited Memory BFGS (L-BFGS), and Adaptive Moment Estimation (Adam) are applied. MLP requires tuning of several hyperparameters such as the number of hidden layers, neurons, and iterations, which could make solving a complicated model computationally expensive. However, through partial fit, MLP offers the advantage of learning non-linear models in real-time or online [ 83 ].
Convolutional Neural Network (CNN or ConvNet)
The Convolutional Neural Network (CNN or ConvNet) [ 65 ] is a popular discriminative deep learning architecture that learns directly from the input without the need for human feature extraction. Figure 7 shows an example of a CNN including multiple convolutions and pooling layers. As a result, the CNN enhances the design of traditional ANN like regularized MLP networks. Each layer in CNN takes into account optimum parameters for a meaningful output as well as reduces model complexity. CNN also uses a ‘dropout’ [ 30 ] that can deal with the problem of over-fitting, which may occur in a traditional network.
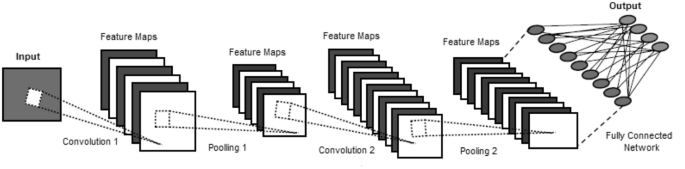
An example of a convolutional neural network (CNN or ConvNet) including multiple convolution and pooling layers
CNNs are specifically intended to deal with a variety of 2D shapes and are thus widely employed in visual recognition, medical image analysis, image segmentation, natural language processing, and many more [ 65 , 96 ]. The capability of automatically discovering essential features from the input without the need for human intervention makes it more powerful than a traditional network. Several variants of CNN are exist in the area that includes visual geometry group (VGG) [ 38 ], AlexNet [ 62 ], Xception [ 17 ], Inception [ 116 ], ResNet [ 39 ], etc. that can be used in various application domains according to their learning capabilities.
Recurrent Neural Network (RNN) and its Variants
A Recurrent Neural Network (RNN) is another popular neural network, which employs sequential or time-series data and feeds the output from the previous step as input to the current stage [ 27 , 74 ]. Like feedforward and CNN, recurrent networks learn from training input, however, distinguish by their “memory”, which allows them to impact current input and output through using information from previous inputs. Unlike typical DNN, which assumes that inputs and outputs are independent of one another, the output of RNN is reliant on prior elements within the sequence. However, standard recurrent networks have the issue of vanishing gradients, which makes learning long data sequences challenging. In the following, we discuss several popular variants of the recurrent network that minimizes the issues and perform well in many real-world application domains.
Long short-term memory (LSTM) This is a popular form of RNN architecture that uses special units to deal with the vanishing gradient problem, which was introduced by Hochreiter et al. [ 42 ]. A memory cell in an LSTM unit can store data for long periods and the flow of information into and out of the cell is managed by three gates. For instance, the ‘Forget Gate’ determines what information from the previous state cell will be memorized and what information will be removed that is no longer useful, while the ‘Input Gate’ determines which information should enter the cell state and the ‘Output Gate’ determines and controls the outputs. As it solves the issues of training a recurrent network, the LSTM network is considered one of the most successful RNN.
Bidirectional RNN/LSTM Bidirectional RNNs connect two hidden layers that run in opposite directions to a single output, allowing them to accept data from both the past and future. Bidirectional RNNs, unlike traditional recurrent networks, are trained to predict both positive and negative time directions at the same time. A Bidirectional LSTM, often known as a BiLSTM, is an extension of the standard LSTM that can increase model performance on sequence classification issues [ 113 ]. It is a sequence processing model comprising of two LSTMs: one takes the input forward and the other takes it backward. Bidirectional LSTM in particular is a popular choice in natural language processing tasks.
Gated recurrent units (GRUs) A Gated Recurrent Unit (GRU) is another popular variant of the recurrent network that uses gating methods to control and manage information flow between cells in the neural network, introduced by Cho et al. [ 16 ]. The GRU is like an LSTM, however, has fewer parameters, as it has a reset gate and an update gate but lacks the output gate, as shown in Fig. 8 . Thus, the key difference between a GRU and an LSTM is that a GRU has two gates (reset and update gates) whereas an LSTM has three gates (namely input, output and forget gates). The GRU’s structure enables it to capture dependencies from large sequences of data in an adaptive manner, without discarding information from earlier parts of the sequence. Thus GRU is a slightly more streamlined variant that often offers comparable performance and is significantly faster to compute [ 18 ]. Although GRUs have been shown to exhibit better performance on certain smaller and less frequent datasets [ 18 , 34 ], both variants of RNN have proven their effectiveness while producing the outcome.
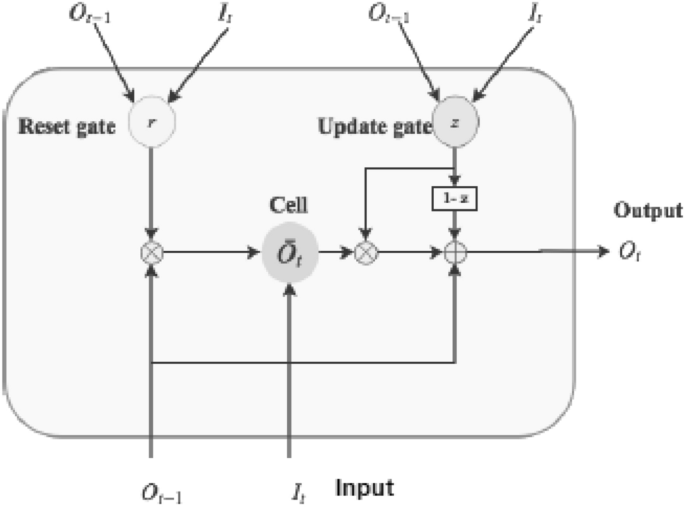
Basic structure of a gated recurrent unit (GRU) cell consisting of reset and update gates
Overall, the basic property of a recurrent network is that it has at least one feedback connection, which enables activations to loop. This allows the networks to do temporal processing and sequence learning, such as sequence recognition or reproduction, temporal association or prediction, etc. Following are some popular application areas of recurrent networks such as prediction problems, machine translation, natural language processing, text summarization, speech recognition, and many more.
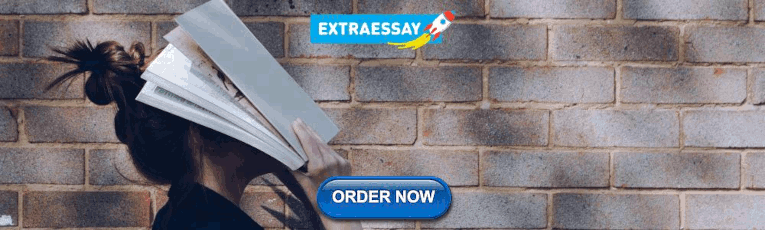
Deep Networks for Generative or Unsupervised Learning
This category of DL techniques is typically used to characterize the high-order correlation properties or features for pattern analysis or synthesis, as well as the joint statistical distributions of the visible data and their associated classes [ 21 ]. The key idea of generative deep architectures is that during the learning process, precise supervisory information such as target class labels is not of concern. As a result, the methods under this category are essentially applied for unsupervised learning as the methods are typically used for feature learning or data generating and representation [ 20 , 21 ]. Thus generative modeling can be used as preprocessing for the supervised learning tasks as well, which ensures the discriminative model accuracy. Commonly used deep neural network techniques for unsupervised or generative learning are Generative Adversarial Network (GAN), Autoencoder (AE), Restricted Boltzmann Machine (RBM), Self-Organizing Map (SOM), and Deep Belief Network (DBN) along with their variants.
Generative Adversarial Network (GAN)
A Generative Adversarial Network (GAN), designed by Ian Goodfellow [ 32 ], is a type of neural network architecture for generative modeling to create new plausible samples on demand. It involves automatically discovering and learning regularities or patterns in input data so that the model may be used to generate or output new examples from the original dataset. As shown in Fig. 9 , GANs are composed of two neural networks, a generator G that creates new data having properties similar to the original data, and a discriminator D that predicts the likelihood of a subsequent sample being drawn from actual data rather than data provided by the generator. Thus in GAN modeling, both the generator and discriminator are trained to compete with each other. While the generator tries to fool and confuse the discriminator by creating more realistic data, the discriminator tries to distinguish the genuine data from the fake data generated by G .
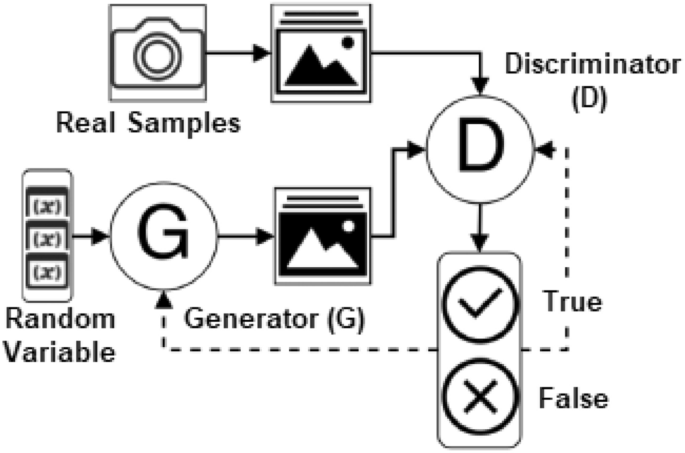
Schematic structure of a standard generative adversarial network (GAN)
Generally, GAN network deployment is designed for unsupervised learning tasks, but it has also proven to be a better solution for semi-supervised and reinforcement learning as well depending on the task [ 3 ]. GANs are also used in state-of-the-art transfer learning research to enforce the alignment of the latent feature space [ 66 ]. Inverse models, such as Bidirectional GAN (BiGAN) [ 25 ] can also learn a mapping from data to the latent space, similar to how the standard GAN model learns a mapping from a latent space to the data distribution. The potential application areas of GAN networks are healthcare, image analysis, data augmentation, video generation, voice generation, pandemics, traffic control, cybersecurity, and many more, which are increasing rapidly. Overall, GANs have established themselves as a comprehensive domain of independent data expansion and as a solution to problems requiring a generative solution.
Auto-Encoder (AE) and Its Variants
An auto-encoder (AE) [ 31 ] is a popular unsupervised learning technique in which neural networks are used to learn representations. Typically, auto-encoders are used to work with high-dimensional data, and dimensionality reduction explains how a set of data is represented. Encoder, code, and decoder are the three parts of an autoencoder. The encoder compresses the input and generates the code, which the decoder subsequently uses to reconstruct the input. The AEs have recently been used to learn generative data models [ 69 ]. The auto-encoder is widely used in many unsupervised learning tasks, e.g., dimensionality reduction, feature extraction, efficient coding, generative modeling, denoising, anomaly or outlier detection, etc. [ 31 , 132 ]. Principal component analysis (PCA) [ 99 ], which is also used to reduce the dimensionality of huge data sets, is essentially similar to a single-layered AE with a linear activation function. Regularized autoencoders such as sparse, denoising, and contractive are useful for learning representations for later classification tasks [ 119 ], while variational autoencoders can be used as generative models [ 56 ], discussed below.
Sparse Autoencoder (SAE) A sparse autoencoder [ 73 ] has a sparsity penalty on the coding layer as a part of its training requirement. SAEs may have more hidden units than inputs, but only a small number of hidden units are permitted to be active at the same time, resulting in a sparse model. Figure 10 shows a schematic structure of a sparse autoencoder with several active units in the hidden layer. This model is thus obliged to respond to the unique statistical features of the training data following its constraints.
Denoising Autoencoder (DAE) A denoising autoencoder is a variant on the basic autoencoder that attempts to improve representation (to extract useful features) by altering the reconstruction criterion, and thus reduces the risk of learning the identity function [ 31 , 119 ]. In other words, it receives a corrupted data point as input and is trained to recover the original undistorted input as its output through minimizing the average reconstruction error over the training data, i.e, cleaning the corrupted input, or denoising. Thus, in the context of computing, DAEs can be considered as very powerful filters that can be utilized for automatic pre-processing. A denoising autoencoder, for example, could be used to automatically pre-process an image, thereby boosting its quality for recognition accuracy.
Contractive Autoencoder (CAE) The idea behind a contractive autoencoder, proposed by Rifai et al. [ 90 ], is to make the autoencoders robust of small changes in the training dataset. In its objective function, a CAE includes an explicit regularizer that forces the model to learn an encoding that is robust to small changes in input values. As a result, the learned representation’s sensitivity to the training input is reduced. While DAEs encourage the robustness of reconstruction as discussed above, CAEs encourage the robustness of representation.
Variational Autoencoder (VAE) A variational autoencoder [ 55 ] has a fundamentally unique property that distinguishes it from the classical autoencoder discussed above, which makes this so effective for generative modeling. VAEs, unlike the traditional autoencoders which map the input onto a latent vector, map the input data into the parameters of a probability distribution, such as the mean and variance of a Gaussian distribution. A VAE assumes that the source data has an underlying probability distribution and then tries to discover the distribution’s parameters. Although this approach was initially designed for unsupervised learning, its use has been demonstrated in other domains such as semi-supervised learning [ 128 ] and supervised learning [ 51 ].
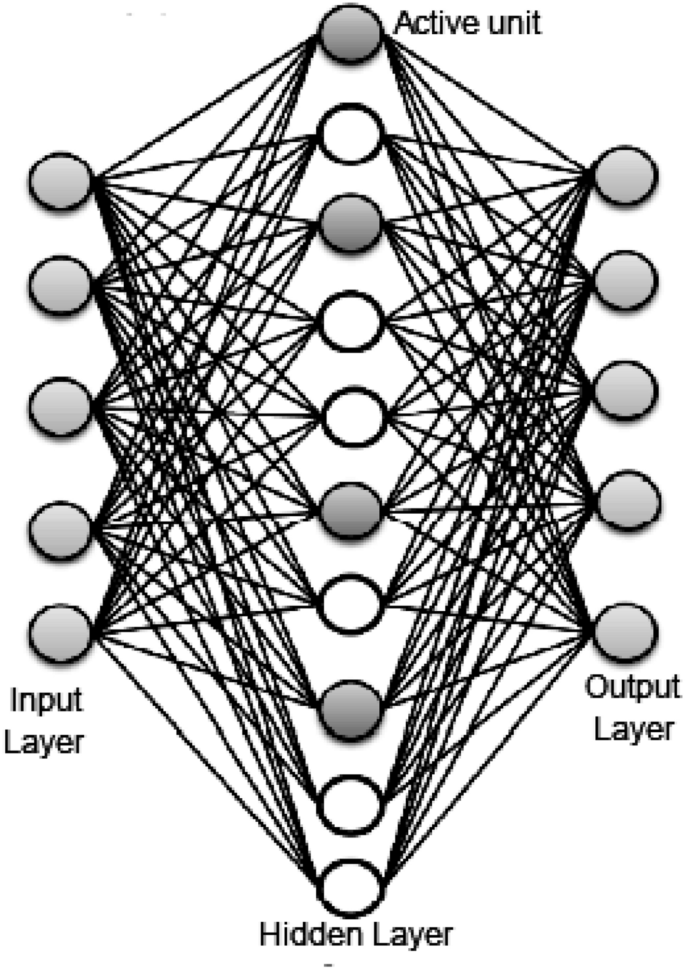
Schematic structure of a sparse autoencoder (SAE) with several active units (filled circle) in the hidden layer
Although, the earlier concept of AE was typically for dimensionality reduction or feature learning mentioned above, recently, AEs have been brought to the forefront of generative modeling, even the generative adversarial network is one of the popular methods in the area. The AEs have been effectively employed in a variety of domains, including healthcare, computer vision, speech recognition, cybersecurity, natural language processing, and many more. Overall, we can conclude that auto-encoder and its variants can play a significant role as unsupervised feature learning with neural network architecture.
Kohonen Map or Self-Organizing Map (SOM)
A Self-Organizing Map (SOM) or Kohonen Map [ 59 ] is another form of unsupervised learning technique for creating a low-dimensional (usually two-dimensional) representation of a higher-dimensional data set while maintaining the topological structure of the data. SOM is also known as a neural network-based dimensionality reduction algorithm that is commonly used for clustering [ 118 ]. A SOM adapts to the topological form of a dataset by repeatedly moving its neurons closer to the data points, allowing us to visualize enormous datasets and find probable clusters. The first layer of a SOM is the input layer, and the second layer is the output layer or feature map. Unlike other neural networks that use error-correction learning, such as backpropagation with gradient descent [ 36 ], SOMs employ competitive learning, which uses a neighborhood function to retain the input space’s topological features. SOM is widely utilized in a variety of applications, including pattern identification, health or medical diagnosis, anomaly detection, and virus or worm attack detection [ 60 , 87 ]. The primary benefit of employing a SOM is that this can make high-dimensional data easier to visualize and analyze to understand the patterns. The reduction of dimensionality and grid clustering makes it easy to observe similarities in the data. As a result, SOMs can play a vital role in developing a data-driven effective model for a particular problem domain, depending on the data characteristics.
Restricted Boltzmann Machine (RBM)
A Restricted Boltzmann Machine (RBM) [ 75 ] is also a generative stochastic neural network capable of learning a probability distribution across its inputs. Boltzmann machines typically consist of visible and hidden nodes and each node is connected to every other node, which helps us understand irregularities by learning how the system works in normal circumstances. RBMs are a subset of Boltzmann machines that have a limit on the number of connections between the visible and hidden layers [ 77 ]. This restriction permits training algorithms like the gradient-based contrastive divergence algorithm to be more efficient than those for Boltzmann machines in general [ 41 ]. RBMs have found applications in dimensionality reduction, classification, regression, collaborative filtering, feature learning, topic modeling, and many others. In the area of deep learning modeling, they can be trained either supervised or unsupervised, depending on the task. Overall, the RBMs can recognize patterns in data automatically and develop probabilistic or stochastic models, which are utilized for feature selection or extraction, as well as forming a deep belief network.
Deep Belief Network (DBN)
A Deep Belief Network (DBN) [ 40 ] is a multi-layer generative graphical model of stacking several individual unsupervised networks such as AEs or RBMs, that use each network’s hidden layer as the input for the next layer, i.e, connected sequentially. Thus, we can divide a DBN into (i) AE-DBN which is known as stacked AE, and (ii) RBM-DBN that is known as stacked RBM, where AE-DBN is composed of autoencoders and RBM-DBN is composed of restricted Boltzmann machines, discussed earlier. The ultimate goal is to develop a faster-unsupervised training technique for each sub-network that depends on contrastive divergence [ 41 ]. DBN can capture a hierarchical representation of input data based on its deep structure. The primary idea behind DBN is to train unsupervised feed-forward neural networks with unlabeled data before fine-tuning the network with labeled input. One of the most important advantages of DBN, as opposed to typical shallow learning networks, is that it permits the detection of deep patterns, which allows for reasoning abilities and the capture of the deep difference between normal and erroneous data [ 89 ]. A continuous DBN is simply an extension of a standard DBN that allows a continuous range of decimals instead of binary data. Overall, the DBN model can play a key role in a wide range of high-dimensional data applications due to its strong feature extraction and classification capabilities and become one of the significant topics in the field of neural networks.
In summary, the generative learning techniques discussed above typically allow us to generate a new representation of data through exploratory analysis. As a result, these deep generative networks can be utilized as preprocessing for supervised or discriminative learning tasks, as well as ensuring model accuracy, where unsupervised representation learning can allow for improved classifier generalization.
Deep Networks for Hybrid Learning and Other Approaches
In addition to the above-discussed deep learning categories, hybrid deep networks and several other approaches such as deep transfer learning (DTL) and deep reinforcement learning (DRL) are popular, which are discussed in the following.
Hybrid Deep Neural Networks
Generative models are adaptable, with the capacity to learn from both labeled and unlabeled data. Discriminative models, on the other hand, are unable to learn from unlabeled data yet outperform their generative counterparts in supervised tasks. A framework for training both deep generative and discriminative models simultaneously can enjoy the benefits of both models, which motivates hybrid networks.
Hybrid deep learning models are typically composed of multiple (two or more) deep basic learning models, where the basic model is a discriminative or generative deep learning model discussed earlier. Based on the integration of different basic generative or discriminative models, the below three categories of hybrid deep learning models might be useful for solving real-world problems. These are as follows:
Hybrid \(Model\_1\) : An integration of different generative or discriminative models to extract more meaningful and robust features. Examples could be CNN+LSTM, AE+GAN, and so on.
Hybrid \(Model\_2\) : An integration of generative model followed by a discriminative model. Examples could be DBN+MLP, GAN+CNN, AE+CNN, and so on.
Hybrid \(Model\_3\) : An integration of generative or discriminative model followed by a non-deep learning classifier. Examples could be AE+SVM, CNN+SVM, and so on.
Thus, in a broad sense, we can conclude that hybrid models can be either classification-focused or non-classification depending on the target use. However, most of the hybrid learning-related studies in the area of deep learning are classification-focused or supervised learning tasks, summarized in Table 1 . The unsupervised generative models with meaningful representations are employed to enhance the discriminative models. The generative models with useful representation can provide more informative and low-dimensional features for discrimination, and they can also enable to enhance the training data quality and quantity, providing additional information for classification.
Deep Transfer Learning (DTL)
Transfer Learning is a technique for effectively using previously learned model knowledge to solve a new task with minimum training or fine-tuning. In comparison to typical machine learning techniques [ 97 ], DL takes a large amount of training data. As a result, the need for a substantial volume of labeled data is a significant barrier to address some essential domain-specific tasks, particularly, in the medical sector, where creating large-scale, high-quality annotated medical or health datasets is both difficult and costly. Furthermore, the standard DL model demands a lot of computational resources, such as a GPU-enabled server, even though researchers are working hard to improve it. As a result, Deep Transfer Learning (DTL), a DL-based transfer learning method, might be helpful to address this issue. Figure 11 shows a general structure of the transfer learning process, where knowledge from the pre-trained model is transferred into a new DL model. It’s especially popular in deep learning right now since it allows to train deep neural networks with very little data [ 126 ].
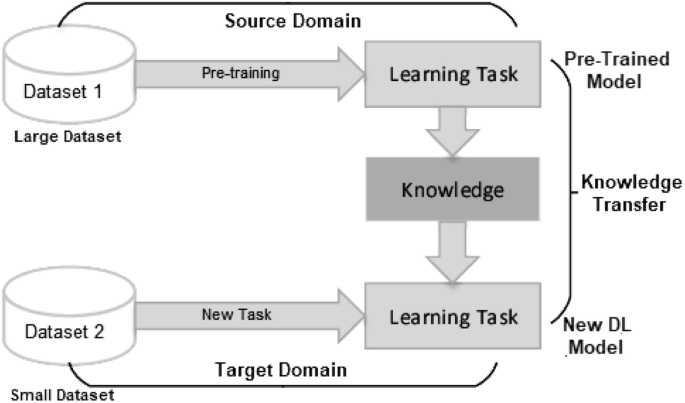
A general structure of transfer learning process, where knowledge from pre-trained model is transferred into new DL model
Transfer learning is a two-stage approach for training a DL model that consists of a pre-training step and a fine-tuning step in which the model is trained on the target task. Since deep neural networks have gained popularity in a variety of fields, a large number of DTL methods have been presented, making it crucial to categorize and summarize them. Based on the techniques used in the literature, DTL can be classified into four categories [ 117 ]. These are (i) instances-based deep transfer learning that utilizes instances in source domain by appropriate weight, (ii) mapping-based deep transfer learning that maps instances from two domains into a new data space with better similarity, (iii) network-based deep transfer learning that reuses the partial of network pre-trained in the source domain, and (iv) adversarial based deep transfer learning that uses adversarial technology to find transferable features that both suitable for two domains. Due to its high effectiveness and practicality, adversarial-based deep transfer learning has exploded in popularity in recent years. Transfer learning can also be classified into inductive, transductive, and unsupervised transfer learning depending on the circumstances between the source and target domains and activities [ 81 ]. While most current research focuses on supervised learning, how deep neural networks can transfer knowledge in unsupervised or semi-supervised learning may gain further interest in the future. DTL techniques are useful in a variety of fields including natural language processing, sentiment classification, visual recognition, speech recognition, spam filtering, and relevant others.
Deep Reinforcement Learning (DRL)
Reinforcement learning takes a different approach to solving the sequential decision-making problem than other approaches we have discussed so far. The concepts of an environment and an agent are often introduced first in reinforcement learning. The agent can perform a series of actions in the environment, each of which has an impact on the environment’s state and can result in possible rewards (feedback) - “positive” for good sequences of actions that result in a “good” state, and “negative” for bad sequences of actions that result in a “bad” state. The purpose of reinforcement learning is to learn good action sequences through interaction with the environment, typically referred to as a policy.
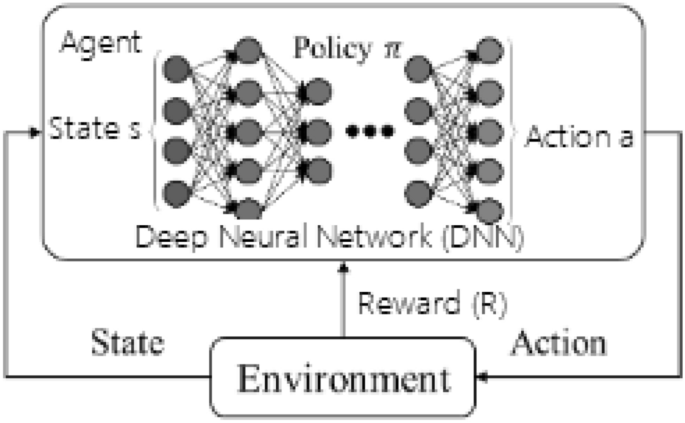
Schematic structure of deep reinforcement learning (DRL) highlighting a deep neural network
Deep reinforcement learning (DRL or deep RL) [ 9 ] integrates neural networks with a reinforcement learning architecture to allow the agents to learn the appropriate actions in a virtual environment, as shown in Fig. 12 . In the area of reinforcement learning, model-based RL is based on learning a transition model that enables for modeling of the environment without interacting with it directly, whereas model-free RL methods learn directly from interactions with the environment. Q-learning is a popular model-free RL technique for determining the best action-selection policy for any (finite) Markov Decision Process (MDP) [ 86 , 97 ]. MDP is a mathematical framework for modeling decisions based on state, action, and rewards [ 86 ]. In addition, Deep Q-Networks, Double DQN, Bi-directional Learning, Monte Carlo Control, etc. are used in the area [ 50 , 97 ]. In DRL methods it incorporates DL models, e.g. Deep Neural Networks (DNN), based on MDP principle [ 71 ], as policy and/or value function approximators. CNN for example can be used as a component of RL agents to learn directly from raw, high-dimensional visual inputs. In the real world, DRL-based solutions can be used in several application areas including robotics, video games, natural language processing, computer vision, and relevant others.
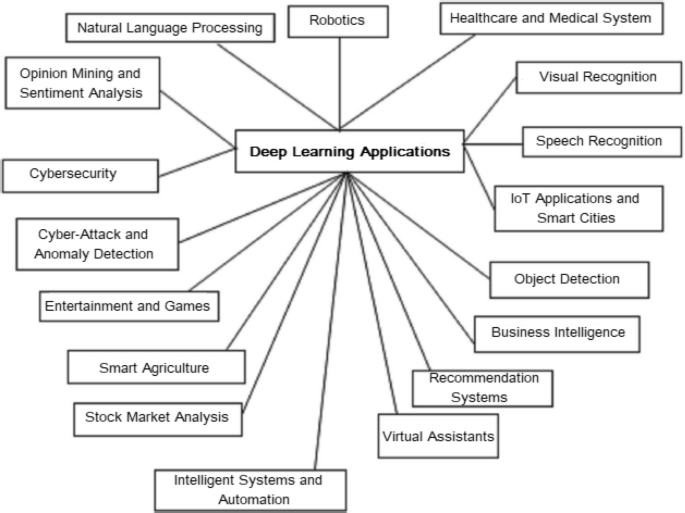
Several potential real-world application areas of deep learning
Deep Learning Application Summary
During the past few years, deep learning has been successfully applied to numerous problems in many application areas. These include natural language processing, sentiment analysis, cybersecurity, business, virtual assistants, visual recognition, healthcare, robotics, and many more. In Fig. 13 , we have summarized several potential real-world application areas of deep learning. Various deep learning techniques according to our presented taxonomy in Fig. 6 that includes discriminative learning, generative learning, as well as hybrid models, discussed earlier, are employed in these application areas. In Table 1 , we have also summarized various deep learning tasks and techniques that are used to solve the relevant tasks in several real-world applications areas. Overall, from Fig. 13 and Table 1 , we can conclude that the future prospects of deep learning modeling in real-world application areas are huge and there are lots of scopes to work. In the next section, we also summarize the research issues in deep learning modeling and point out the potential aspects for future generation DL modeling.
Research Directions and Future Aspects
While existing methods have established a solid foundation for deep learning systems and research, this section outlines the below ten potential future research directions based on our study.
Automation in Data Annotation According to the existing literature, discussed in Section 3 , most of the deep learning models are trained through publicly available datasets that are annotated. However, to build a system for a new problem domain or recent data-driven system, raw data from relevant sources are needed to collect. Thus, data annotation, e.g., categorization, tagging, or labeling of a large amount of raw data, is important for building discriminative deep learning models or supervised tasks, which is challenging. A technique with the capability of automatic and dynamic data annotation, rather than manual annotation or hiring annotators, particularly, for large datasets, could be more effective for supervised learning as well as minimizing human effort. Therefore, a more in-depth investigation of data collection and annotation methods, or designing an unsupervised learning-based solution could be one of the primary research directions in the area of deep learning modeling.
Data Preparation for Ensuring Data Quality As discussed earlier throughout the paper, the deep learning algorithms highly impact data quality, and availability for training, and consequently on the resultant model for a particular problem domain. Thus, deep learning models may become worthless or yield decreased accuracy if the data is bad, such as data sparsity, non-representative, poor-quality, ambiguous values, noise, data imbalance, irrelevant features, data inconsistency, insufficient quantity, and so on for training. Consequently, such issues in data can lead to poor processing and inaccurate findings, which is a major problem while discovering insights from data. Thus deep learning models also need to adapt to such rising issues in data, to capture approximated information from observations. Therefore, effective data pre-processing techniques are needed to design according to the nature of the data problem and characteristics, to handling such emerging challenges, which could be another research direction in the area.
Black-box Perception and Proper DL/ML Algorithm Selection In general, it’s difficult to explain how a deep learning result is obtained or how they get the ultimate decisions for a particular model. Although DL models achieve significant performance while learning from large datasets, as discussed in Section 2 , this “black-box” perception of DL modeling typically represents weak statistical interpretability that could be a major issue in the area. On the other hand, ML algorithms, particularly, rule-based machine learning techniques provide explicit logic rules (IF-THEN) for making decisions that are easier to interpret, update or delete according to the target applications [ 97 , 100 , 105 ]. If the wrong learning algorithm is chosen, unanticipated results may occur, resulting in a loss of effort as well as the model’s efficacy and accuracy. Thus by taking into account the performance, complexity, model accuracy, and applicability, selecting an appropriate model for the target application is challenging, and in-depth analysis is needed for better understanding and decision making.
Deep Networks for Supervised or Discriminative Learning: According to our designed taxonomy of deep learning techniques, as shown in Fig. 6 , discriminative architectures mainly include MLP, CNN, and RNN, along with their variants that are applied widely in various application domains. However, designing new techniques or their variants of such discriminative techniques by taking into account model optimization, accuracy, and applicability, according to the target real-world application and the nature of the data, could be a novel contribution, which can also be considered as a major future aspect in the area of supervised or discriminative learning.
Deep Networks for Unsupervised or Generative Learning As discussed in Section 3 , unsupervised learning or generative deep learning modeling is one of the major tasks in the area, as it allows us to characterize the high-order correlation properties or features in data, or generating a new representation of data through exploratory analysis. Moreover, unlike supervised learning [ 97 ], it does not require labeled data due to its capability to derive insights directly from the data as well as data-driven decision making. Consequently, it thus can be used as preprocessing for supervised learning or discriminative modeling as well as semi-supervised learning tasks, which ensure learning accuracy and model efficiency. According to our designed taxonomy of deep learning techniques, as shown in Fig. 6 , generative techniques mainly include GAN, AE, SOM, RBM, DBN, and their variants. Thus, designing new techniques or their variants for an effective data modeling or representation according to the target real-world application could be a novel contribution, which can also be considered as a major future aspect in the area of unsupervised or generative learning.
Hybrid/Ensemble Modeling and Uncertainty Handling According to our designed taxonomy of DL techniques, as shown in Fig 6 , this is considered as another major category in deep learning tasks. As hybrid modeling enjoys the benefits of both generative and discriminative learning, an effective hybridization can outperform others in terms of performance as well as uncertainty handling in high-risk applications. In Section 3 , we have summarized various types of hybridization, e.g., AE+CNN/SVM. Since a group of neural networks is trained with distinct parameters or with separate sub-sampling training datasets, hybridization or ensembles of such techniques, i.e., DL with DL/ML, can play a key role in the area. Thus designing effective blended discriminative and generative models accordingly rather than naive method, could be an important research opportunity to solve various real-world issues including semi-supervised learning tasks and model uncertainty.
Dynamism in Selecting Threshold/ Hyper-parameters Values, and Network Structures with Computational Efficiency In general, the relationship among performance, model complexity, and computational requirements is a key issue in deep learning modeling and applications. A combination of algorithmic advancements with improved accuracy as well as maintaining computational efficiency, i.e., achieving the maximum throughput while consuming the least amount of resources, without significant information loss, can lead to a breakthrough in the effectiveness of deep learning modeling in future real-world applications. The concept of incremental approaches or recency-based learning [ 100 ] might be effective in several cases depending on the nature of target applications. Moreover, assuming the network structures with a static number of nodes and layers, hyper-parameters values or threshold settings, or selecting them by the trial-and-error process may not be effective in many cases, as it can be changed due to the changes in data. Thus, a data-driven approach to select them dynamically could be more effective while building a deep learning model in terms of both performance and real-world applicability. Such type of data-driven automation can lead to future generation deep learning modeling with additional intelligence, which could be a significant future aspect in the area as well as an important research direction to contribute.
Lightweight Deep Learning Modeling for Next-Generation Smart Devices and Applications: In recent years, the Internet of Things (IoT) consisting of billions of intelligent and communicating things and mobile communications technologies have become popular to detect and gather human and environmental information (e.g. geo-information, weather data, bio-data, human behaviors, and so on) for a variety of intelligent services and applications. Every day, these ubiquitous smart things or devices generate large amounts of data, requiring rapid data processing on a variety of smart mobile devices [ 72 ]. Deep learning technologies can be incorporate to discover underlying properties and to effectively handle such large amounts of sensor data for a variety of IoT applications including health monitoring and disease analysis, smart cities, traffic flow prediction, and monitoring, smart transportation, manufacture inspection, fault assessment, smart industry or Industry 4.0, and many more. Although deep learning techniques discussed in Section 3 are considered as powerful tools for processing big data, lightweight modeling is important for resource-constrained devices, due to their high computational cost and considerable memory overhead. Thus several techniques such as optimization, simplification, compression, pruning, generalization, important feature extraction, etc. might be helpful in several cases. Therefore, constructing the lightweight deep learning techniques based on a baseline network architecture to adapt the DL model for next-generation mobile, IoT, or resource-constrained devices and applications, could be considered as a significant future aspect in the area.
Incorporating Domain Knowledge into Deep Learning Modeling Domain knowledge, as opposed to general knowledge or domain-independent knowledge, is knowledge of a specific, specialized topic or field. For instance, in terms of natural language processing, the properties of the English language typically differ from other languages like Bengali, Arabic, French, etc. Thus integrating domain-based constraints into the deep learning model could produce better results for such particular purpose. For instance, a task-specific feature extractor considering domain knowledge in smart manufacturing for fault diagnosis can resolve the issues in traditional deep-learning-based methods [ 28 ]. Similarly, domain knowledge in medical image analysis [ 58 ], financial sentiment analysis [ 49 ], cybersecurity analytics [ 94 , 103 ] as well as conceptual data model in which semantic information, (i.e., meaningful for a system, rather than merely correlational) [ 45 , 121 , 131 ] is included, can play a vital role in the area. Transfer learning could be an effective way to get started on a new challenge with domain knowledge. Moreover, contextual information such as spatial, temporal, social, environmental contexts [ 92 , 104 , 108 ] can also play an important role to incorporate context-aware computing with domain knowledge for smart decision making as well as building adaptive and intelligent context-aware systems. Therefore understanding domain knowledge and effectively incorporating them into the deep learning model could be another research direction.
Designing General Deep Learning Framework for Target Application Domains One promising research direction for deep learning-based solutions is to develop a general framework that can handle data diversity, dimensions, stimulation types, etc. The general framework would require two key capabilities: the attention mechanism that focuses on the most valuable parts of input signals, and the ability to capture latent feature that enables the framework to capture the distinctive and informative features. Attention models have been a popular research topic because of their intuition, versatility, and interpretability, and employed in various application areas like computer vision, natural language processing, text or image classification, sentiment analysis, recommender systems, user profiling, etc [ 13 , 80 ]. Attention mechanism can be implemented based on learning algorithms such as reinforcement learning that is capable of finding the most useful part through a policy search [ 133 , 134 ]. Similarly, CNN can be integrated with suitable attention mechanisms to form a general classification framework, where CNN can be used as a feature learning tool for capturing features in various levels and ranges. Thus, designing a general deep learning framework considering attention as well as a latent feature for target application domains could be another area to contribute.
To summarize, deep learning is a fairly open topic to which academics can contribute by developing new methods or improving existing methods to handle the above-mentioned concerns and tackle real-world problems in a variety of application areas. This can also help the researchers conduct a thorough analysis of the application’s hidden and unexpected challenges to produce more reliable and realistic outcomes. Overall, we can conclude that addressing the above-mentioned issues and contributing to proposing effective and efficient techniques could lead to “Future Generation DL” modeling as well as more intelligent and automated applications.
Concluding Remarks
In this article, we have presented a structured and comprehensive view of deep learning technology, which is considered a core part of artificial intelligence as well as data science. It starts with a history of artificial neural networks and moves to recent deep learning techniques and breakthroughs in different applications. Then, the key algorithms in this area, as well as deep neural network modeling in various dimensions are explored. For this, we have also presented a taxonomy considering the variations of deep learning tasks and how they are used for different purposes. In our comprehensive study, we have taken into account not only the deep networks for supervised or discriminative learning but also the deep networks for unsupervised or generative learning, and hybrid learning that can be used to solve a variety of real-world issues according to the nature of problems.
Deep learning, unlike traditional machine learning and data mining algorithms, can produce extremely high-level data representations from enormous amounts of raw data. As a result, it has provided an excellent solution to a variety of real-world problems. A successful deep learning technique must possess the relevant data-driven modeling depending on the characteristics of raw data. The sophisticated learning algorithms then need to be trained through the collected data and knowledge related to the target application before the system can assist with intelligent decision-making. Deep learning has shown to be useful in a wide range of applications and research areas such as healthcare, sentiment analysis, visual recognition, business intelligence, cybersecurity, and many more that are summarized in the paper.
Finally, we have summarized and discussed the challenges faced and the potential research directions, and future aspects in the area. Although deep learning is considered a black-box solution for many applications due to its poor reasoning and interpretability, addressing the challenges or future aspects that are identified could lead to future generation deep learning modeling and smarter systems. This can also help the researchers for in-depth analysis to produce more reliable and realistic outcomes. Overall, we believe that our study on neural networks and deep learning-based advanced analytics points in a promising path and can be utilized as a reference guide for future research and implementations in relevant application domains by both academic and industry professionals.
Abadi M, Barham P, Chen J, Chen Z, Davis A, Dean J, Devin Ma, Ghemawat S, Irving G, Isard M, et al. Tensorflow: a system for large-scale machine learning. In: 12th { USENIX } Symposium on operating systems design and implementation ({ OSDI } 16), 2016; p. 265–283.
Abdel-Basset M, Hawash H, Chakrabortty RK, Ryan M. Energy-net: a deep learning approach for smart energy management in iot-based smart cities. IEEE Internet of Things J. 2021.
Aggarwal A, Mittal M, Battineni G. Generative adversarial network: an overview of theory and applications. Int J Inf Manag Data Insights. 2021; p. 100004.
Al-Qatf M, Lasheng Y, Al-Habib M, Al-Sabahi K. Deep learning approach combining sparse autoencoder with svm for network intrusion detection. IEEE Access. 2018;6:52843–56.
Article Google Scholar
Ale L, Sheta A, Li L, Wang Y, Zhang N. Deep learning based plant disease detection for smart agriculture. In: 2019 IEEE Globecom Workshops (GC Wkshps), 2019; p. 1–6. IEEE.
Amarbayasgalan T, Lee JY, Kim KR, Ryu KH. Deep autoencoder based neural networks for coronary heart disease risk prediction. In: Heterogeneous data management, polystores, and analytics for healthcare. Springer; 2019. p. 237–48.
Anuradha J, et al. Big data based stock trend prediction using deep cnn with reinforcement-lstm model. Int J Syst Assur Eng Manag. 2021; p. 1–11.
Aqib M, Mehmood R, Albeshri A, Alzahrani A. Disaster management in smart cities by forecasting traffic plan using deep learning and gpus. In: International Conference on smart cities, infrastructure, technologies and applications. Springer; 2017. p. 139–54.
Arulkumaran K, Deisenroth MP, Brundage M, Bharath AA. Deep reinforcement learning: a brief survey. IEEE Signal Process Mag. 2017;34(6):26–38.
Aslan MF, Unlersen MF, Sabanci K, Durdu A. Cnn-based transfer learning-bilstm network: a novel approach for covid-19 infection detection. Appl Soft Comput. 2021;98:106912.
Bu F, Wang X. A smart agriculture iot system based on deep reinforcement learning. Futur Gener Comput Syst. 2019;99:500–7.
Chang W-J, Chen L-B, Hsu C-H, Lin C-P, Yang T-C. A deep learning-based intelligent medicine recognition system for chronic patients. IEEE Access. 2019;7:44441–58.
Chaudhari S, Mithal V, Polatkan Gu, Ramanath R. An attentive survey of attention models. arXiv preprint arXiv:1904.02874, 2019.
Chaudhuri N, Gupta G, Vamsi V, Bose I. On the platform but will they buy? predicting customers’ purchase behavior using deep learning. Decis Support Syst. 2021; p. 113622.
Chen D, Wawrzynski P, Lv Z. Cyber security in smart cities: a review of deep learning-based applications and case studies. Sustain Cities Soc. 2020; p. 102655.
Cho K, Van MB, Gulcehre C, Bahdanau D, Bougares F, Schwenk H, Bengio Y. Learning phrase representations using rnn encoder-decoder for statistical machine translation. arXiv preprint arXiv:1406.1078, 2014.
Chollet F. Xception: Deep learning with depthwise separable convolutions. In: Proceedings of the IEEE Conference on computer vision and pattern recognition, 2017; p. 1251–258.
Chung J, Gulcehre C, Cho KH, Bengio Y. Empirical evaluation of gated recurrent neural networks on sequence modeling. arXiv preprint arXiv:1412.3555, 2014.
Coelho IM, Coelho VN, da Eduardo J, Luz S, Ochi LS, Guimarães FG, Rios E. A gpu deep learning metaheuristic based model for time series forecasting. Appl Energy. 2017;201:412–8.
Da'u A, Salim N. Recommendation system based on deep learning methods: a systematic review and new directions. Artif Intel Rev. 2020;53(4):2709–48.
Deng L. A tutorial survey of architectures, algorithms, and applications for deep learning. APSIPA Trans Signal Inf Process. 2014; p. 3.
Deng L, Dong Yu. Deep learning: methods and applications. Found Trends Signal Process. 2014;7(3–4):197–387.
Article MathSciNet MATH Google Scholar
Deng S, Li R, Jin Y, He H. Cnn-based feature cross and classifier for loan default prediction. In: 2020 International Conference on image, video processing and artificial intelligence, volume 11584, page 115841K. International Society for Optics and Photonics, 2020.
Dhyani M, Kumar R. An intelligent chatbot using deep learning with bidirectional rnn and attention model. Mater Today Proc. 2021;34:817–24.
Donahue J, Krähenbühl P, Darrell T. Adversarial feature learning. arXiv preprint arXiv:1605.09782, 2016.
Du K-L, Swamy MNS. Neural networks and statistical learning. Berlin: Springer Science & Business Media; 2013.
MATH Google Scholar
Dupond S. A thorough review on the current advance of neural network structures. Annu Rev Control. 2019;14:200–30.
Google Scholar
Feng J, Yao Y, Lu S, Liu Y. Domain knowledge-based deep-broad learning framework for fault diagnosis. IEEE Trans Ind Electron. 2020;68(4):3454–64.
Garg S, Kaur K, Kumar N, Rodrigues JJPC. Hybrid deep-learning-based anomaly detection scheme for suspicious flow detection in sdn: a social multimedia perspective. IEEE Trans Multimed. 2019;21(3):566–78.
Géron A. Hands-on machine learning with Scikit-Learn, Keras. In: and TensorFlow: concepts, tools, and techniques to build intelligent systems. O’Reilly Media; 2019.
Goodfellow I, Bengio Y, Courville A, Bengio Y. Deep learning, vol. 1. Cambridge: MIT Press; 2016.
Goodfellow I, Pouget-Abadie J, Mirza M, Xu B, Warde-Farley D, Ozair S, Courville A, Bengio Y. Generative adversarial nets. In: Advances in neural information processing systems. 2014; p. 2672–680.
Google trends. 2021. https://trends.google.com/trends/ .
Gruber N, Jockisch A. Are gru cells more specific and lstm cells more sensitive in motive classification of text? Front Artif Intell. 2020;3:40.
Gu B, Ge R, Chen Y, Luo L, Coatrieux G. Automatic and robust object detection in x-ray baggage inspection using deep convolutional neural networks. IEEE Trans Ind Electron. 2020.
Han J, Pei J, Kamber M. Data mining: concepts and techniques. Amsterdam: Elsevier; 2011.
Haykin S. Neural networks and learning machines, 3/E. London: Pearson Education; 2010.
He K, Zhang X, Ren S, Sun J. Spatial pyramid pooling in deep convolutional networks for visual recognition. IEEE Trans Pattern Anal Mach Intell. 2015;37(9):1904–16.
He K, Zhang X, Ren S, Sun J. Deep residual learning for image recognition. In: Proceedings of the IEEE Conference on computer vision and pattern recognition, 2016; p. 770–78.
Hinton GE. Deep belief networks. Scholarpedia. 2009;4(5):5947.
Hinton GE, Osindero S, Teh Y-W. A fast learning algorithm for deep belief nets. Neural Comput. 2006;18(7):1527–54.
Hochreiter S, Schmidhuber J. Long short-term memory. Neural Comput. 1997;9(8):1735–80.
Huang C-J, Kuo P-H. A deep cnn-lstm model for particulate matter (pm2. 5) forecasting in smart cities. Sensors. 2018;18(7):2220.
Huang H-H, Fukuda M, Nishida T. Toward rnn based micro non-verbal behavior generation for virtual listener agents. In: International Conference on human-computer interaction, 2019; p. 53–63. Springer.
Hulsebos M, Hu K, Bakker M, Zgraggen E, Satyanarayan A, Kraska T, Demiralp Ça, Hidalgo C. Sherlock: a deep learning approach to semantic data type detection. In: Proceedings of the 25th ACM SIGKDD International Conference on knowledge discovery & data mining, 2019; p. 1500–508.
Imamverdiyev Y, Abdullayeva F. Deep learning method for denial of service attack detection based on restricted Boltzmann machine. Big Data. 2018;6(2):159–69.
Islam MZ, Islam MM, Asraf A. A combined deep cnn-lstm network for the detection of novel coronavirus (covid-19) using x-ray images. Inf Med Unlock. 2020;20:100412.
Ismail WN, Hassan MM, Alsalamah HA, Fortino G. Cnn-based health model for regular health factors analysis in internet-of-medical things environment. IEEE. Access. 2020;8:52541–9.
Jangid H, Singhal S, Shah RR, Zimmermann R. Aspect-based financial sentiment analysis using deep learning. In: Companion Proceedings of the The Web Conference 2018, 2018; p. 1961–966.
Kaelbling LP, Littman ML, Moore AW. Reinforcement learning: a survey. J Artif Intell Res. 1996;4:237–85.
Kameoka H, Li L, Inoue S, Makino S. Supervised determined source separation with multichannel variational autoencoder. Neural Comput. 2019;31(9):1891–914.
Karhunen J, Raiko T, Cho KH. Unsupervised deep learning: a short review. In: Advances in independent component analysis and learning machines. 2015; p. 125–42.
Kawde P, Verma GK. Deep belief network based affect recognition from physiological signals. In: 2017 4th IEEE Uttar Pradesh Section International Conference on electrical, computer and electronics (UPCON), 2017; p. 587–92. IEEE.
Kim J-Y, Seok-Jun B, Cho S-B. Zero-day malware detection using transferred generative adversarial networks based on deep autoencoders. Inf Sci. 2018;460:83–102.
Kingma DP, Welling M. Auto-encoding variational bayes. arXiv preprint arXiv:1312.6114, 2013.
Kingma DP, Welling M. An introduction to variational autoencoders. arXiv preprint arXiv:1906.02691, 2019.
Kiran PKR, Bhasker B. Dnnrec: a novel deep learning based hybrid recommender system. Expert Syst Appl. 2020.
Kloenne M, Niehaus S, Lampe L, Merola A, Reinelt J, Roeder I, Scherf N. Domain-specific cues improve robustness of deep learning-based segmentation of ct volumes. Sci Rep. 2020;10(1):1–9.
Kohonen T. The self-organizing map. Proc IEEE. 1990;78(9):1464–80.
Kohonen T. Essentials of the self-organizing map. Neural Netw. 2013;37:52–65.
Kök İ, Şimşek MU, Özdemir S. A deep learning model for air quality prediction in smart cities. In: 2017 IEEE International Conference on Big Data (Big Data), 2017; p. 1983–990. IEEE.
Krizhevsky A, Sutskever I, Hinton GE. Imagenet classification with deep convolutional neural networks. In: Advances in neural information processing systems. 2012; p. 1097–105.
Latif S, Rana R, Younis S, Qadir J, Epps J. Transfer learning for improving speech emotion classification accuracy. arXiv preprint arXiv:1801.06353, 2018.
LeCun Y, Bengio Y, Hinton G. Deep learning. Nature. 2015;521(7553):436–44.
LeCun Y, Bottou L, Bengio Y, Haffner P. Gradient-based learning applied to document recognition. Proc IEEE. 1998;86(11):2278–324.
Li B, François-Lavet V, Doan T, Pineau J. Domain adversarial reinforcement learning. arXiv preprint arXiv:2102.07097, 2021.
Li T-HS, Kuo P-H, Tsai T-N, Luan P-C. Cnn and lstm based facial expression analysis model for a humanoid robot. IEEE Access. 2019;7:93998–4011.
Liu C, Cao Y, Luo Y, Chen G, Vokkarane V, Yunsheng M, Chen S, Hou P. A new deep learning-based food recognition system for dietary assessment on an edge computing service infrastructure. IEEE Trans Serv Comput. 2017;11(2):249–61.
Liu W, Wang Z, Liu X, Zeng N, Liu Y, Alsaadi FE. A survey of deep neural network architectures and their applications. Neurocomputing. 2017;234:11–26.
López AU, Mateo F, Navío-Marco J, Martínez-Martínez JM, Gómez-Sanchís J, Vila-Francés J, Serrano-López AJ. Analysis of computer user behavior, security incidents and fraud using self-organizing maps. Comput Secur. 2019;83:38–51.
Lopez-Martin M, Carro B, Sanchez-Esguevillas A. Application of deep reinforcement learning to intrusion detection for supervised problems. Expert Syst Appl. 2020;141:112963.
Ma X, Yao T, Menglan H, Dong Y, Liu W, Wang F, Liu J. A survey on deep learning empowered iot applications. IEEE Access. 2019;7:181721–32.
Makhzani A, Frey B. K-sparse autoencoders. arXiv preprint arXiv:1312.5663, 2013.
Mandic D, Chambers J. Recurrent neural networks for prediction: learning algorithms, architectures and stability. Hoboken: Wiley; 2001.
Book Google Scholar
Marlin B, Swersky K, Chen B, Freitas N. Inductive principles for restricted boltzmann machine learning. In: Proceedings of the Thirteenth International Conference on artificial intelligence and statistics, p. 509–16. JMLR Workshop and Conference Proceedings, 2010.
Masud M, Muhammad G, Alhumyani H, Alshamrani SS, Cheikhrouhou O, Ibrahim S, Hossain MS. Deep learning-based intelligent face recognition in iot-cloud environment. Comput Commun. 2020;152:215–22.
Memisevic R, Hinton GE. Learning to represent spatial transformations with factored higher-order boltzmann machines. Neural Comput. 2010;22(6):1473–92.
Article MATH Google Scholar
Minaee S, Azimi E, Abdolrashidi AA. Deep-sentiment: sentiment analysis using ensemble of cnn and bi-lstm models. arXiv preprint arXiv:1904.04206, 2019.
Naeem M, Paragliola G, Coronato A. A reinforcement learning and deep learning based intelligent system for the support of impaired patients in home treatment. Expert Syst Appl. 2021;168:114285.
Niu Z, Zhong G, Hui Yu. A review on the attention mechanism of deep learning. Neurocomputing. 2021;452:48–62.
Pan SJ, Yang Q. A survey on transfer learning. IEEE Trans Knowl Data Eng. 2009;22(10):1345–59.
Paszke A, Gross S, Massa F, Lerer A, Bradbury J, Chanan G, Killeen T, Lin Z, Gimelshein N, Antiga L, et al. Pytorch: An imperative style, high-performance deep learning library. Adv Neural Inf Process Syst. 2019;32:8026–37.
Pedregosa F, Varoquaux G, Gramfort A, Michel V, Thirion B, Grisel O, Blondel M, Prettenhofer P, Weiss R, Dubourg V, et al. Scikit-learn: machine learning in python. J Mach Learn Res. 2011;12:2825–30.
MathSciNet MATH Google Scholar
Pi Y, Nath ND, Behzadan AH. Convolutional neural networks for object detection in aerial imagery for disaster response and recovery. Adv Eng Inf. 2020;43:101009.
Piccialli F, Giampaolo F, Prezioso E, Crisci D, Cuomo S. Predictive analytics for smart parking: A deep learning approach in forecasting of iot data. ACM Trans Internet Technol (TOIT). 2021;21(3):1–21.
Puterman ML. Markov decision processes: discrete stochastic dynamic programming. Hoboken: Wiley; 2014.
Qu X, Lin Y, Kai G, Linru M, Meng S, Mingxing K, Mu L, editors. A survey on the development of self-organizing maps for unsupervised intrusion detection. Mob Netw Appl. 2019; p. 1–22.
Rahman MW, Tashfia SS, Islam R, Hasan MM, Sultan SI, Mia S, Rahman MM. The architectural design of smart blind assistant using iot with deep learning paradigm. Internet of Things. 2021;13:100344.
Ren J, Green M, Huang X. From traditional to deep learning: fault diagnosis for autonomous vehicles. In: Learning control. Elsevier. 2021; p. 205–19.
Rifai S, Vincent P, Muller X, Glorot X, Bengio Y. Contractive auto-encoders: Explicit invariance during feature extraction. In: Icml, 2011.
Rosa RL, Schwartz GM, Ruggiero WV, Rodríguez DZ. A knowledge-based recommendation system that includes sentiment analysis and deep learning. IEEE Trans Ind Inf. 2018;15(4):2124–35.
Sarker IH. Context-aware rule learning from smartphone data: survey, challenges and future directions. J Big Data. 2019;6(1):1–25.
Article MathSciNet Google Scholar
Sarker IH. A machine learning based robust prediction model for real-life mobile phone data. Internet of Things. 2019;5:180–93.
Sarker IH. Cyberlearning: effectiveness analysis of machine learning security modeling to detect cyber-anomalies and multi-attacks. Internet of Things. 2021;14:100393.
Sarker IH. Data science and analytics: an overview from data-driven smart computing, decision-making and applications perspective. SN Comput Sci. 2021.
Sarker IH. Deep cybersecurity: a comprehensive overview from neural network and deep learning perspective. SN Computer. Science. 2021;2(3):1–16.
MathSciNet Google Scholar
Sarker IH. Machine learning: Algorithms, real-world applications and research directions. SN Computer. Science. 2021;2(3):1–21.
Sarker IH, Abushark YB, Alsolami F, Khan AI. Intrudtree: a machine learning based cyber security intrusion detection model. Symmetry. 2020;12(5):754.
Sarker IH, Abushark YB, Khan AI. Contextpca: Predicting context-aware smartphone apps usage based on machine learning techniques. Symmetry. 2020;12(4):499.
Sarker IH, Colman A, Han J. Recencyminer: mining recency-based personalized behavior from contextual smartphone data. J Big Data. 2019;6(1):1–21.
Sarker IH, Colman A, Han J, Khan AI, Abushark YB, Salah K. Behavdt: a behavioral decision tree learning to build user-centric context-aware predictive model. Mob Netw Appl. 2020;25(3):1151–61.
Sarker IH, Colman A, Kabir MA, Han J. Individualized time-series segmentation for mining mobile phone user behavior. Comput J. 2018;61(3):349–68.
Sarker IH, Furhad MH, Nowrozy R. Ai-driven cybersecurity: an overview, security intelligence modeling and research directions. SN Computer. Science. 2021;2(3):1–18.
Sarker IH, Hoque MM, Uddin MK. Mobile data science and intelligent apps: concepts, ai-based modeling and research directions. Mob Netw Appl. 2021;26(1):285–303.
Sarker IH, Kayes ASM. Abc-ruleminer: User behavioral rule-based machine learning method for context-aware intelligent services. J Netw Comput Appl. 2020;168:102762.
Sarker IH, Kayes ASM, Badsha S, Alqahtani H, Watters P, Ng A. Cybersecurity data science: an overview from machine learning perspective. J Big data. 2020;7(1):1–29.
Sarker IH, Kayes ASM, Watters P. Effectiveness analysis of machine learning classification models for predicting personalized context-aware smartphone usage. J Big Data. 2019;6(1):1–28.
Sarker IH, Salah K. Appspred: predicting context-aware smartphone apps using random forest learning. Internet of Things. 2019;8:100106.
Satt A, Rozenberg S, Hoory R. Efficient emotion recognition from speech using deep learning on spectrograms. In: Interspeec, 2017; p. 1089–1093.
Sevakula RK, Singh V, Verma NK, Kumar C, Cui Y. Transfer learning for molecular cancer classification using deep neural networks. IEEE/ACM Trans Comput Biol Bioinf. 2018;16(6):2089–100.
Sujay Narumanchi H, Ananya Pramod Kompalli Shankar A, Devashish CK. Deep learning based large scale visual recommendation and search for e-commerce. arXiv preprint arXiv:1703.02344, 2017.
Shao X, Kim CS. Multi-step short-term power consumption forecasting using multi-channel lstm with time location considering customer behavior. IEEE Access. 2020;8:125263–73.
Siami-Namini S, Tavakoli N, Namin AS. The performance of lstm and bilstm in forecasting time series. In: 2019 IEEE International Conference on Big Data (Big Data), 2019; p. 3285–292. IEEE.
Ślusarczyk B. Industry 4.0: are we ready? Pol J Manag Stud. 2018; p. 17
Sumathi P, Subramanian R, Karthikeyan VV, Karthik S. Soil monitoring and evaluation system using edl-asqe: enhanced deep learning model for ioi smart agriculture network. Int J Commun Syst. 2021; p. e4859.
Szegedy C, Liu W, Jia Y, Sermanet P, Reed S, Anguelov D, Erhan D, Vanhoucke V, Rabinovich A. Going deeper with convolutions. In: Proceedings of the IEEE Conference on computer vision and pattern recognition, 2015; p. 1–9.
Tan C, Sun F, Kong T, Zhang W, Yang C, Liu C. A survey on deep transfer learning. In: International Conference on artificial neural networks, 2018; p. 270–279. Springer.
Vesanto J, Alhoniemi E. Clustering of the self-organizing map. IEEE Trans Neural Netw. 2000;11(3):586–600.
Vincent P, Larochelle H, Lajoie I, Bengio Y, Manzagol P-A, Bottou L. Stacked denoising autoencoders: Learning useful representations in a deep network with a local denoising criterion. J Mach Learn Res. 2010;11(12).
Wang J, Liang-Chih Yu, Robert Lai K, Zhang X. Tree-structured regional cnn-lstm model for dimensional sentiment analysis. IEEE/ACM Trans Audio Speech Lang Process. 2019;28:581–91.
Wang S, Wan J, Li D, Liu C. Knowledge reasoning with semantic data for real-time data processing in smart factory. Sensors. 2018;18(2):471.
Wang W, Zhao M, Wang J. Effective android malware detection with a hybrid model based on deep autoencoder and convolutional neural network. J Ambient Intell Humaniz Comput. 2019;10(8):3035–43.
Wang X, Liu J, Qiu T, Chaoxu M, Chen C, Zhou P. A real-time collision prediction mechanism with deep learning for intelligent transportation system. IEEE Trans Veh Technol. 2020;69(9):9497–508.
Wang Y, Huang M, Zhu X, Zhao L. Attention-based lstm for aspect-level sentiment classification. In: Proceedings of the 2016 Conference on empirical methods in natural language processing, 2016; p. 606–615.
Wei P, Li Y, Zhang Z, Tao H, Li Z, Liu D. An optimization method for intrusion detection classification model based on deep belief network. IEEE Access. 2019;7:87593–605.
Weiss K, Khoshgoftaar TM, Wang DD. A survey of transfer learning. J Big data. 2016;3(1):9.
Xin Y, Kong L, Liu Z, Chen Y, Li Y, Zhu H, Gao M, Hou H, Wang C. Machine learning and deep learning methods for cybersecurity. Ieee access. 2018;6:35365–81.
Xu W, Sun H, Deng C, Tan Y. Variational autoencoder for semi-supervised text classification. In: Thirty-First AAAI Conference on artificial intelligence, 2017.
Xue Q, Chuah MC. New attacks on rnn based healthcare learning system and their detections. Smart Health. 2018;9:144–57.
Yousefi-Azar M, Hamey L. Text summarization using unsupervised deep learning. Expert Syst Appl. 2017;68:93–105.
Yuan X, Shi J, Gu L. A review of deep learning methods for semantic segmentation of remote sensing imagery. Expert Syst Appl. 2020;p. 114417.
Zhang G, Liu Y, Jin X. A survey of autoencoder-based recommender systems. Front Comput Sci. 2020;14(2):430–50.
Zhang X, Yao L, Huang C, Wang S, Tan M, Long Gu, Wang C. Multi-modality sensor data classification with selective attention. arXiv preprint arXiv:1804.05493, 2018.
Zhang X, Yao L, Wang X, Monaghan J, Mcalpine D, Zhang Y. A survey on deep learning based brain computer interface: recent advances and new frontiers. arXiv preprint arXiv:1905.04149, 2019; p. 66.
Zhang Y, Zhang P, Yan Y. Attention-based lstm with multi-task learning for distant speech recognition. In: Interspeech, 2017; p. 3857–861.
Download references
Author information
Authors and affiliations.
Swinburne University of Technology, Melbourne, VIC, 3122, Australia
Iqbal H. Sarker
Chittagong University of Engineering & Technology, Chittagong, 4349, Bangladesh
You can also search for this author in PubMed Google Scholar
Corresponding author
Correspondence to Iqbal H. Sarker .
Ethics declarations
Conflict of interest.
The author declares no conflict of interest.
Additional information
Publisher's note.
Springer Nature remains neutral with regard to jurisdictional claims in published maps and institutional affiliations.
This article is part of the topical collection “Advances in Computational Approaches for Artificial Intelligence, Image Processing, IoT and Cloud Applications” guest edited by Bhanu Prakash K. N. and M. Shivakumar.
Rights and permissions
Reprints and permissions
About this article
Sarker, I.H. Deep Learning: A Comprehensive Overview on Techniques, Taxonomy, Applications and Research Directions. SN COMPUT. SCI. 2 , 420 (2021). https://doi.org/10.1007/s42979-021-00815-1
Download citation
Received : 29 May 2021
Accepted : 07 August 2021
Published : 18 August 2021
DOI : https://doi.org/10.1007/s42979-021-00815-1
Share this article
Anyone you share the following link with will be able to read this content:
Sorry, a shareable link is not currently available for this article.
Provided by the Springer Nature SharedIt content-sharing initiative
- Deep learning
- Artificial neural network
- Artificial intelligence
- Discriminative learning
- Generative learning
- Hybrid learning
- Intelligent systems
- Find a journal
- Publish with us
- Track your research
Navigation Menu
Search code, repositories, users, issues, pull requests..., provide feedback.
We read every piece of feedback, and take your input very seriously.
Saved searches
Use saved searches to filter your results more quickly.
To see all available qualifiers, see our documentation .
- Notifications You must be signed in to change notification settings
Presentation for Master's Thesis - Deep Reinforcement Learning for Robotic Grasping from Octrees
AndrejOrsula/master_thesis_presentation
Folders and files, repository files navigation.
This repository contains LaTeX (Beamer) setup for the presentation of my Master's Thesis conducted at Aalborg University, Denmark. Master's Thesis can be found under AndrejOrsula/master_thesis . If you are interested in the source code, please see AndrejOrsula/drl_grasping .
Compiled PDF: presentation.pdf
The PDF contains embedded videos that might not be supported on your browser's default viewer. If that is the case, please try a different viewer. Alternatively, some of the recordings can also be viewed under YouTube playlist .
Disclaimer (LaTeX Template)
Latex template is adapted from jkjaer/aauLatexTemplates and modified to my preferences.
DAVID STUTZ
Phd defense slides and lessons learned.
In July this year I finally defended my PhD which mainly focused on (adversarial) robustness and uncertainty estimation in deep learning. In my case, the defense consisted of a (public) 30 minute talk about my work, followed by questions from the thesis committee and audience. In this article, I want to share the slides and some lessons learned in preparing for my defense.
My PhD defense marks the successdful end of a four year journey in machine learning and computer vision research. While defense vary widely across institutions and universities, I had to prepare a 30 minute talk that is followed by questions from the committee and audience. In addition to the difficulty of squeezing several papers into a 30 minute polished talk, there was also a significant delay between submitting and defending my PhD — roughly 4 months. While I can't share the final thesis yet, you can find my defense slides below, followed by some lessons learned in preparing for my defense.
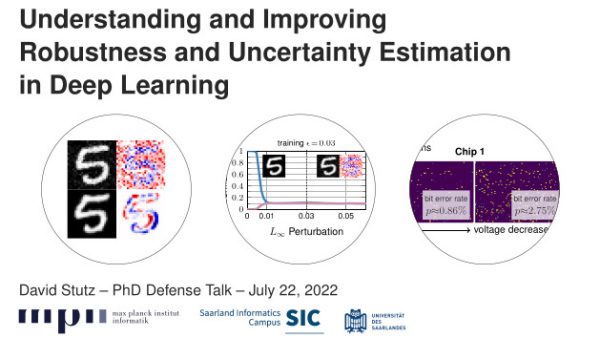
The slides include some GIFs, so with 27MB the download might take a second. Also, make sure to view them in a PDF viewer supporting animations:
Lessons Learned
Preparing for my defense took significantly longer than I initially estimated. I thought it could not be too hard to put together slides of all my projects, given that I already gave a few talks and had slides for most of my papers ready. However, preparing a polished talk that highlights achievements, giving a good high-level intuition while at the same time demonstrating technical knowledge, was incredibly difficult and took multiple iterations. Besides, I wanted to talk to steer the committee's questions to some extent and make sure I will stick to the 30 minute limit. Here are some lessons I learned surrounding the preparation:
- Start preparing early enough: Even though I thought the preparation to take less time, I took some long weekends roughly one and a half months before the defense to start preparing the slides. Looking back, this was a great decision as I ended up working on my slides until the last couple of days before the defense.
- Schedule a practice talk: In my case, a practice talk really made a huge difference. Initially, I included too much content in my slides and it was good to get objective feedback on what to focus on, where more details and time is required and which topics can be covered with less details. I had the practice talk two weeks before the defense and I really used these two weeks to improve my presentation.
- Practice: Before the practice talk, I practiced the talk and timed it to the minute. This really came in handy in the end because it reduced stress as I knew exactly how to start or get back into the talk on each slide. During the talk this was also helpful as interruptions didn't break the flow of my presentation.
- Backup slides: Even though I did not end up using most of my backup slides, preparing them helped me to think about potential questions that the committee might ask.
- Test the setup: A day before the defense, I tested the technical setup. As my defense was hybrid, there was a lot that could go wrong — the virtual meeting room, the microphone etc. It really reduced stress to have it tested once before the defense. Some things that are important to keep in mind is that the setup should also take into account discussion and questions of the committee/audience which are sometimes difficult to handle in a hybrid setting.
Regarding the content of the talk, I found the following to work well — and I found a similar scheme can be found in many other defenses, as well:
- Fuse motivation with the outline: I ended up putting a lot of effort into a good motivation of my talk that also happened to reflect the outline of my talk. This really helped to tell a consistent high-level story throughout the talk.
- Highligh achievements: I feel that it was important to highlight the papers that I have published over the years — even those not included in the talk.
- Technical detail: For two projects, I decided to go into technical detail. While I did not have many technical questions, I got the impression that this was expected by the commitee. Of course, it was tricky to decide which projects to highlight in detail as this usually means that all other projects can only be covered on a higher level.
- Ignore some projects: In order to go into technical detail for few projects, I decided to not talk about three of my papers. I just mentioned this line of work in my conclusion and the introduction. Initially, I found it difficult to let go of these parts, but it helped to improve the focus of the talk.
- Have some slides on future work: An easy way to steer the discussion/questions after the defense talk are future work topics. Also, I found that these slides can be used to end the talk on a positive note by expressing your excitement about future research — note that I had these slides after the conclusion.
SEARCH THEBLOG
- 2024 —
- 2023 —
- 2022 —
- 2021 —
- 2020 —
- 2019 —
- 2018 —
- 2017 —
- 2016 —
- 2015 —
- 2014 —
- 2013 —
- ADVERSARIAL MACHINE LEARNING
- ARTIFICIAL INTELLIGENCE
- COMPRESSED SENSING
- COMPUTER GRAPHICS
- COMPUTER SCIENCE
- COMPUTER VISION
- DATA MINING
- DEEP LEARNING
- DNN ACCELERATORS
- GAME THEORY
- GENERATIVE AI
- IMAGE PROCESSING
- MACHINE LEARNING
- MATHEMATICS
- MEDIA COVERAGE
- MEDICAL IMAGE PROCESSING
- NATURAL LANGUAGE PROCESSING
- NUMERICAL ANALYSIS
- OPTIMIZATION
- PUBLICATION
- RASPBERRY PI
- SECURITY AND PRIVACY
- SOCIAL NETWORKS
- SOFTWARE ENGINEERING
- TWITTER BOOTSTRAP
- UNCERTAINTY ESTIMATION
- WEB SECURITY
Newly Launched - AI Presentation Maker

Researched by Consultants from Top-Tier Management Companies
AI PPT Maker
Powerpoint Templates
PPT Bundles
Kpi Dashboard
Professional
Business Plans
Swot Analysis
Gantt Chart
Business Proposal
Marketing Plan
Project Management
Business Case
Business Model
Cyber Security
Business PPT
Digital Marketing
Digital Transformation
Human Resources
Product Management
Artificial Intelligence
Company Profile
Acknowledgement PPT
PPT Presentation
Reports Brochures
One Page Pitch
Interview PPT
All Categories
Must-Have Deep Learning PPT Templates with Examples and Samples

Yajur Sharma

"Artificial Intelligence, deep learning, machine learning — whatever you're doing, if you don't understand it — learn it. Because otherwise, you're going to be a dinosaur within 3 years."
- Mark Cuban (an American entrepreneur, investor, author, and television personality)
The exponential expansion of Artificial Intelligence (AI) has brought about a new age where ideas such as deep learning and machine learning aren't just advantageous—they’re imperative to stay relevant in an increasingly digital world.
Deep learning is a groundbreaking approach to artificial intelligence, revolutionizing our thoughts. Taking inspiration from the intricate neural networks of the human brain, deep learning algorithms have the remarkable ability to learn from data independently without explicit instructions. These algorithms can solve previously daunting tasks by recognizing complex patterns and extracting detailed representations. From recognizing images and speech to understanding language, the potential of deep learning knows no bounds.
A defining characteristic of deep learning lies in its heavy dependence on copious amounts of data. The phrase " data is the new oil " holds profound significance in this realm, as the efficacy of deep learning systems is closely tied to the sheer quantity and caliber of data they are exposed to during training. Employing methods like supervised learning, unsupervised learning, and reinforcement learning, deep learning models continually fine-tune their parameters, honing their capacity to generalize and provide precise forecasts.
Are you ready to take your machine-learning presentations to the next level? Click on this link for the Top 10 Machine Learning Templates and learn how they can make complex algorithms accessible yet understandable!
Shaping the Future of Intelligence
The uses of deep learning are both far-reaching and impactful. In the field of healthcare, deep learning is revolutionizing the way diseases are identified through medical imagery, forecasting patient prognosis, and customizing treatment methods. In finance, these powerful algorithms are instrumental in flagging fraud, powering algorithmic trading, and evaluating risk. The transportation industry also benefits from deep learning technology, with its ability to support self-driving vehicles, streamline navigation, and elevate safety measures. Finally, in the entertainment world, deep learning allows for individualized recommendations, creates new content, and enhances immersive virtual experiences.
SlideTeam has created the best Deep-Learning PPT Templates that offer several benefits for professionals and educators alike. They provide a head-start by offering pre-designed layouts. This saves valuable time and effort in creating presentations from scratch. Additionally, our templates are completely customizable and editable, allowing you to tailor them to your needs and requirements.
Whether you're conducting a training session on mastering the fundamentals of deep learning or delivering a presentation on the nuances between artificial intelligence, machine learning, and deep learning, our templates offer the flexibility to adapt content seamlessly.
Let’s Explore!
Template 1: Deep Learning Mastering the Fundamentals Training
Investing in deep learning training gives businesses the boost they need to utilize AI's potential in opening up new creative and efficient pathways in today's quickly changing technological world. This training slide gives in-depth knowledge about the importance and work of deep learning. It also compares deep learning and machine learning (ML) and covers deep learning functions, Sigmoid Activation Functions, Hyperbolic Tangent Functions, Loss Functions, Optimizer Functions, etc. It dives into the process of deep learning, highlighting its advantages, limitations, and applications. The deck has additional slides on About Us, Vision, Mission, Goal, 30-60-90 Days Plan, Timeline, and roadmap. Lastly, organizations may benefit by using advanced algorithms to analyze large datasets, make wise decisions, and maintain an advantage in competitive marketplaces by grasping the principles of deep learning. Download this today!

CLICK HERE TO DOWNLOAD
Template 2: Artificial Intelligence Machine Learning Deep Learning
Artificial Intelligence goes beyond simply creating machines capable of human-like Intelligence. Within this broad concept, Machine Learning is a specialized area that involves teaching computers to learn from data and enhance their abilities over time. This PowerPoint Template bundle in 99 slides displays the disparity between AI, ML, and deep learning through its informative design. It concerns training neural networks with multiple layers to identify complex patterns and gain deeper insights from massive sets of information, leading to advanced problem-solving and decision-making capabilities. This template also highlights the areas that can benefit from AI, like supply chain, customer experience, human resources, fraud detection, research, etc. Highlighting the booming rate of AI business and its future revenue forecast are also mentioned in this informative bundle. These technologies help businesses by streamlining operations, automating jobs, and providing valuable information that enhances decision-making and increases income.

CLICK HERE TO DOWNLOAD
Template 3: Machine Learning Vs Deep Learning PowerPoint Template
Machine Learning usually uses basic algorithms that depend on feature engineering and extraction to make predictions. On the other hand, deep learning utilizes complex neural networks with multiple layers, often without the requirement for explicit feature engineering. In addition to that, to attain optimal performance, machine learning models frequently need significant preprocessing of the data together with created features. However, because Deep Learning models can learn directly from unprocessed data, they are better able to handle unstructured data, like text, audio, and pictures. This PowerPoint Slide showcases the transformation in Machine learning and Deep learning. Elements like custom hardware, transfer learning, etc., are included. In addition to that, multiple slides in this informative bundle display how machine learning or deep learning benefits businesses involved in various sectors such as finance, medical, retail, automotive, etc. Further, it includes the workflow of machine learning and deep learning. Grab this professionally created bundle today!

Discovering Tomorrow's Solutions Today with Deep Learning
Deep learning is a game changer in the world of technology, paving the way for endless possibilities and discoveries in various industries. By having the capacity to uncover intricate patterns from massive sets of data independently, deep learning has reshaped our approach to artificial intelligence. This has sparked advancements in crucial sectors like healthcare, finance, transportation, and entertainment. To fully tap into the power of deep learning and minimize potential drawbacks, it is essential to foster cooperation, transparency, and responsible implementation.
From revolutionizing industries to augmenting everyday tasks, deep learning has a multitude of far-reaching and significant uses. With visually engaging graphics, charts, and diagrams, our templates enhance audience engagement and comprehension. Moreover, they ensure consistency in branding and messaging across presentations, reinforcing professionalism and credibility. So, leverage our deep learning PowerPoint templates to streamline your presentation creation process and effectively communicate complex concepts with clarity and impact.
Related posts:
- Must-Have Artificial Intelligence Industry Report Samples with Templates and Examples
- 5 Steps to Implement Artificial Intelligence In Your Business – Best Templates Included
- Virtual Reality is the Future of Business (And These 15 PowerPoint Templates Will Show You Why)
- [Updated 2023]: Top 10 Data Science Templates To Enable Better Decision-Making
Liked this blog? Please recommend us

Top 7 Consulting Agreement Templates for Professional Relationships!

Top 7 Relationship Mapping Templates with Samples and Examples
This form is protected by reCAPTCHA - the Google Privacy Policy and Terms of Service apply.


Disclaimer/Complaints regulations
If you believe that digital publication of certain material infringes any of your rights or (privacy) interests, please let the Library know, stating your reasons. In case of a legitimate complaint, the Library will make the material inaccessible and/or remove it from the website. Please Ask the Library , or send a letter to: Library of the University of Amsterdam, Secretariat, Singel 425, 1012 WP Amsterdam, The Netherlands. You will be contacted as soon as possible.
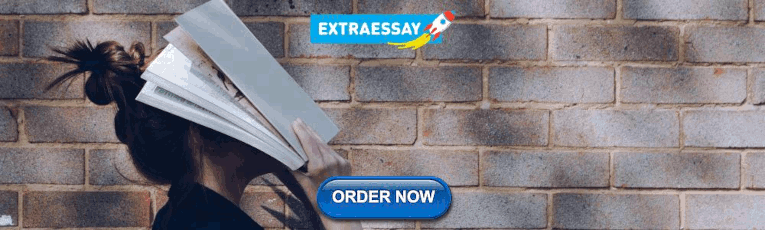
IMAGES
VIDEO
COMMENTS
deep learning. Her ambition and foresight ignited my passion for bridging the research in deep learning and hardware. Sitting on the same floor with Fei-Fei and her students spawned many researchspark. IsincerelythankFei-Fei'sstudentsAndrejKarpathy,YukeZhu,JustinJohnson,
1 Introduction to Deep Learning Mustafa Mustafa NERSC @mustafa240m Data Seminars, NERSC March 2019, Berkeley Lab 2 @mustafa240m Deep Learning is powering many recent technologies 3 @mustafa240m Deep Learning is powered by Deep Neural Networks 4 @mustafa240m Neural Networks history goes back to the 50s Fig. credit Efstratios Gavves, Intro. DL 5 ...
Irfan Aziz: Deep Learning: An Overview of Convolutional Neural Network M.Sc Thesis Tampere University Master Degree Programme in Computational Big Data Analytics April 2020 In the last two decades, deep learning, an area of machine learning has made exponential progress and breakthroughs.
Go 1.0 was released in March 2012 [22]. The focus of this thesis is to integrate GPU computation with the Go language for the purpose of developing deep learning models. This chapter includes a review of some of the packages that were developed for GPU computation with Go, the applications that use them, and other deep learning frameworks. 2.1 ...
Chapter1 Introduction: TheImportanceofKnowingWhat WeDon'tKnow IntheBayesianmachinelearning communityweworkwithprobabilisticmodelsand uncertainty ...
Exploiting the Interplay between Visual and Textual Data for Scene Interpretation. I successfully defended my PhD the 08/10/2020 and got an excellent Cum Laude. In this page the thesis pdf, the presentation slides, and a video of the presentation are made available. Thesis Slides. Photos:
The new model family introduced in this thesis is summarized under the term Recursive Deep Learning. The models in this family are variations and extensions of unsupervised and supervised recursive neural networks (RNNs) which generalize deep and feature learning ideas to hierarchical structures. The RNN models of this thesis
This thesis considers deep learning theories of brain function, and in particular biologically plausible deep learning. The idea is to treat a standard deep network as a high-level model of a neural circuit (e.g., the visual stream), adding biological constraints to some clearly artificial features. Two big questions are possible. First,
Using Deep Learning Master's thesis in Biomedical Engineering Linus Lagergren and Carl Rosengren Department of Electrical Engineering CHALMERS UNIVERSITY OF TECHNOLOGY Gothenburg, Sweden 2020 ... Using deep neural networks to perform semantic segmentation of brain tumors in multi-modalMagneticResonanceImages
Deep learning models have been used for this task, and traditional methods such as clustering and rule-based systems are widely used as well. This thesis aims to compare deep learning models with traditional algorithms for anomaly detection in network traffic and analyze the trade-offs between the models in terms of accuracy and scalability. 19.
Deep learning (DL), a branch of machine learning (ML) and artificial intelligence (AI) is nowadays considered as a core technology of today's Fourth Industrial Revolution (4IR or Industry 4.0). Due to its learning capabilities from data, DL technology originated from artificial neural network (ANN), has become a hot topic in the context of computing, and is widely applied in various ...
Presentation for Master's Thesis - Deep Reinforcement Learning for Robotic Grasping from Octrees This repository contains LaTeX (Beamer) setup for the presentation of my Master's Thesis conducted at Aalborg University, Denmark.
ARTICLE. PhD Defense Slides and Lessons Learned. In July this year I finally defended my PhD which mainly focused on (adversarial) robustness and uncertainty estimation in deep learning. In my case, the defense consisted of a (public) 30 minute talk about my work, followed by questions from the thesis committee and audience.
Chapter 6. Overall Conclusions. • In this thesis, the capabilities and limitations of deep learning techniques for predicting visual. saliency on s till images we re studied. • In Chapter 2, a ...
Finally, in the entertainment world, deep learning allows for individualized recommendations, creates new content, and enhances immersive virtual experiences. SlideTeam has created the best Deep-Learning PPT Templates that offer several benefits for professionals and educators alike. They provide a head-start by offering pre-designed layouts.
A deep-learning architecture is a mul tilayer stack of simple mod- ules, all (or most) of which are subject to learning, and man y of which compute non-linea r input-outpu t mappings.
Deep learning with graph-structured representations. 23 April 2020. 164. 9789463758512. PhD thesis. Faculty of Science (FNWI) Informatics Institute (IVI) In this thesis, we propose novel approaches to machine learning with structured data. Our proposed methods are largely based on the theme of structuring the representations and computations of ...
Thesis Description. The thesis aims to develop and implement AI-driven algorithms for target identification in passive radar systems by analyzing micro-Doppler signatures. This involves several key objectives: Data Collection: Gather simulated and real-world micro-Doppler signatures from various target types under different conditions.