Thank you for visiting nature.com. You are using a browser version with limited support for CSS. To obtain the best experience, we recommend you use a more up to date browser (or turn off compatibility mode in Internet Explorer). In the meantime, to ensure continued support, we are displaying the site without styles and JavaScript.
- View all journals
- Explore content
- About the journal
- Publish with us
- Sign up for alerts
- Review Article
- Published: 28 March 2024
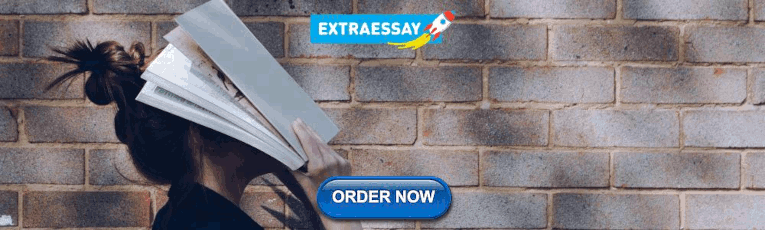
Genetic variation across and within individuals
- Zhi Yu ORCID: orcid.org/0000-0003-4810-3474 1 , 2 na1 ,
- Tim H. H. Coorens ORCID: orcid.org/0000-0002-5826-3554 1 na1 ,
- Md Mesbah Uddin ORCID: orcid.org/0000-0003-1846-0411 1 , 2 ,
- Kristin G. Ardlie 1 ,
- Niall Lennon 1 &
- Pradeep Natarajan ORCID: orcid.org/0000-0001-8402-7435 1 , 2 , 3
Nature Reviews Genetics ( 2024 ) Cite this article
5871 Accesses
91 Altmetric
Metrics details
- DNA sequencing
- Genetic variation
Germline variation and somatic mutation are intricately connected and together shape human traits and disease risks. Germline variants are present from conception, but they vary between individuals and accumulate over generations. By contrast, somatic mutations accumulate throughout life in a mosaic manner within an individual due to intrinsic and extrinsic sources of mutations and selection pressures acting on cells. Recent advancements, such as improved detection methods and increased resources for association studies, have drastically expanded our ability to investigate germline and somatic genetic variation and compare underlying mutational processes. A better understanding of the similarities and differences in the types, rates and patterns of germline and somatic variants, as well as their interplay, will help elucidate the mechanisms underlying their distinct yet interlinked roles in human health and biology.
This is a preview of subscription content, access via your institution
Access options
Access Nature and 54 other Nature Portfolio journals
Get Nature+, our best-value online-access subscription
24,99 € / 30 days
cancel any time
Subscribe to this journal
Receive 12 print issues and online access
176,64 € per year
only 14,72 € per issue
Buy this article
- Purchase on Springer Link
- Instant access to full article PDF
Prices may be subject to local taxes which are calculated during checkout
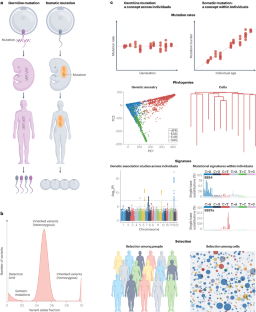
Similar content being viewed by others
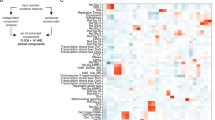
The impact of rare germline variants on human somatic mutation processes
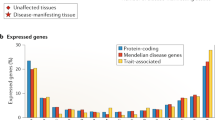
Mechanisms of tissue and cell-type specificity in heritable traits and diseases
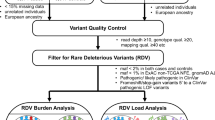
Germline rare deleterious variant load alters cancer risk, age of onset and tumor characteristics
Lynch, M. et al. Genetic drift, selection and the evolution of the mutation rate. Nat. Rev. Genet. 17 , 704–714 (2016).
Article CAS PubMed Google Scholar
Coorens, T. H. H. et al. Extensive phylogenies of human development inferred from somatic mutations. Nature 597 , 387–392 (2021). In this study, clones from many different normal tissues are sequenced, and phylogenetic trees of these normal cells are reconstructed, revealing embryonic lineages and somatic evolution.
Bizzotto, S. et al. Landmarks of human embryonic development inscribed in somatic mutations. Science 371 , 1249–1253 (2021).
Article CAS PubMed PubMed Central Google Scholar
Spencer Chapman, M. et al. Lineage tracing of human development through somatic mutations. Nature 595 , 85–90 (2021).
Fasching, L. et al. Early developmental asymmetries in cell lineage trees in living individuals. Science 371 , 1245–1248 (2021).
Park, S. et al. Clonal dynamics in early human embryogenesis inferred from somatic mutation. Nature 597 , 393–397 (2021).
Bates, G. P. History of genetic disease: the molecular genetics of Huntington disease — a history. Nat. Rev. Genet. 6 , 766–773 (2005).
Berberich, A. J. & Hegele, R. A. The complex molecular genetics of familial hypercholesterolaemia. Nat. Rev. Cardiol. 16 , 9–20 (2019).
Wooster, R. et al. Identification of the breast cancer susceptibility gene BRCA2 . Nature 378 , 789–792 (1995).
Miki, Y. et al. A strong candidate for the breast and ovarian cancer susceptibility gene BRCA1 . Science 266 , 66–71 (1994).
Martincorena, I. & Campbell, P. J. Somatic mutation in cancer and normal cells. Science 349 , 1483–1489 (2015).
Mustjoki, S. & Young, N. S. Somatic mutations in “benign” disease. N. Engl. J. Med. 384 , 2039–2052 (2021).
Miller, M. B. et al. Somatic genomic changes in single Alzheimer’s disease neurons. Nature 604 , 714–722 (2022).
Jaiswal, S. et al. Clonal hematopoiesis and risk of atherosclerotic cardiovascular disease. N. Engl. J. Med. 377 , 111–121 (2017).
Article PubMed PubMed Central Google Scholar
Wong, W. J. et al. Clonal haematopoiesis and risk of chronic liver disease. Nature 616 , 747–754 (2023).
Niroula, A. et al. Distinction of lymphoid and myeloid clonal hematopoiesis. Nat. Med. 27 , 1921–1927 (2021).
Silver, A. J., Bick, A. G. & Savona, M. R. Germline risk of clonal haematopoiesis. Nat. Rev. Genet. 22 , 603–617 (2021).
Robinson, P. S. et al. Increased somatic mutation burdens in normal human cells due to defective DNA polymerases. Nat. Genet. 53 , 1434–1442 (2021).
Lee, B. C. H. et al. Mutational landscape of normal epithelial cells in Lynch Syndrome patients. Nat. Commun. 13 , 2710 (2022).
Robinson, P. S. et al. Inherited MUTYH mutations cause elevated somatic mutation rates and distinctive mutational signatures in normal human cells. Nat. Commun. 13 , 3949 (2022).
Kazazian, H. H. Jr Mobile elements: drivers of genome evolution. Science 303 , 1626–1632 (2004).
Alexandrov, L. B. et al. The repertoire of mutational signatures in human cancer. Nature 578 , 94–101 (2020).
Haradhvala, N. J. et al. Mutational strand asymmetries in cancer genomes reveal mechanisms of DNA damage and repair. Cell 164 , 538–549 (2016).
Macintyre, G. et al. Copy number signatures and mutational processes in ovarian carcinoma. Nat. Genet. 50 , 1262–1270 (2018).
Li, Y. et al. Patterns of somatic structural variation in human cancer genomes. Nature 578 , 112–121 (2020).
Coorens, T. H. H. et al. Inherent mosaicism and extensive mutation of human placentas. Nature 592 , 80–85 (2021).
Moore, L. et al. The mutational landscape of human somatic and germline cells. Nature 597 , 381–386 (2021).
Blokzijl, F. et al. Tissue-specific mutation accumulation in human adult stem cells during life. Nature 538 , 260–264 (2016).
Lawson, A. R. J. et al. Extensive heterogeneity in somatic mutation and selection in the human bladder. Science 370 , 75–82 (2020). This study is one of the first to use organoid cultures of stem cells from different human tissues to study somatic mutations in normal cells by whole-genome sequencing.
Abascal, F. et al. Somatic mutation landscapes at single-molecule resolution. Nature 593 , 405–410 (2021).
Lee-Six, H. et al. The landscape of somatic mutation in normal colorectal epithelial cells. Nature 574 , 532–537 (2019).
Mitchell, E. et al. Clonal dynamics of haematopoiesis across the human lifespan. Nature 606 , 343–350 (2022).
Wang, Y. et al. APOBEC mutagenesis is a common process in normal human small intestine. Nat. Genet. 55 , 246–254 (2023).
Moore, L. et al. The mutational landscape of normal human endometrial epithelium. Nature 580 , 640–646 (2020).
Yoshida, K. et al. Tobacco smoking and somatic mutations in human bronchial epithelium. Nature 578 , 266–272 (2020).
Brunner, S. F. et al. Somatic mutations and clonal dynamics in healthy and cirrhotic human liver. Nature 574 , 538–542 (2019).
Martincorena, I. et al. Tumor evolution. High burden and pervasive positive selection of somatic mutations in normal human skin. Science 348 , 880–886 (2015). This study identifies large clonal expansions carrying driver mutations in normal skin.
Martincorena, I. et al. Somatic mutant clones colonize the human esophagus with age. Science 362 , 911–917 (2018).
Jaiswal, S. et al. Age-related clonal hematopoiesis associated with adverse outcomes. N. Engl. J. Med. 371 , 2488–2498 (2014). This is a landmark study demonstrating population-level associations of somatic mutation with both cancer and non-cancer health conditions.
Zekavat, S. M. et al. Hematopoietic mosaic chromosomal alterations increase the risk for diverse types of infection. Nat. Med. 27 , 1012–1024 (2021).
Colom, B. et al. Spatial competition shapes the dynamic mutational landscape of normal esophageal epithelium. Nat. Genet. 52 , 604–614 (2020).
Abby, E. et al. Notch1 mutations drive clonal expansion in normal esophageal epithelium but impair tumor growth. Nat. Genet. 55 , 232–245 (2023).
Ng, S. W. K. et al. Convergent somatic mutations in metabolism genes in chronic liver disease. Nature 598 , 473–478 (2021). This study identifies selection for recurrent somatic mutations as an adaptive mechanism to chronic liver disease.
Rahbari, R. et al. Timing, rates and spectra of human germline mutation. Nat. Genet. 48 , 126–133 (2016).
Jónsson, H. et al. Parental influence on human germline de novo mutations in 1,548 trios from Iceland. Nature 549 , 519–522 (2017).
Article PubMed Google Scholar
Kong, A. et al. Rate of de novo mutations and the importance of father’s age to disease risk. Nature 488 , 471–475 (2012).
Kaplanis, J. et al. Genetic and chemotherapeutic influences on germline hypermutation. Nature 605 , 503–508 (2022). This study, based on whole-genome data of over 20,000 families, identifies accelerated rates of de novo germline mutations and determines the likely causes of this hypermutation.
Maher, G. J. et al. Visualizing the origins of selfish de novo mutations in individual seminiferous tubules of human testes. Proc. Natl Acad. Sci. USA 113 , 2454–2459 (2016).
Goriely, A., McGrath, J. J., Hultman, C. M., Wilkie, A. O. M. & Malaspina, D. ‘Selfish spermatogonial selection’: a novel mechanism for the association between advanced paternal age and neurodevelopmental disorders. Am. J. Psychiatry 170 , 599–608 (2013).
Goriely, A., McVean, G. A. T., Röjmyr, M., Ingemarsson, B. & Wilkie, A. O. M. Evidence for selective advantage of pathogenic FGFR2 mutations in the male germ line. Science 301 , 643–646 (2003).
Pena, S. D. J. Advances of aneuploidy research in the maternal germline. Nat. Rev. Genet. 24 , 274 (2023).
Champion, K. J. et al. Germline mutation in BRAF codon 600 is compatible with human development: de novo p.V600G mutation identified in a patient with CFC syndrome. Clin. Genet. 79 , 468–474 (2011).
Sabeti, P. C. et al. Detecting recent positive selection in the human genome from haplotype structure. Nature 419 , 832–837 (2002).
Olson, N. D. et al. Variant calling and benchmarking in an era of complete human genome sequences. Nat. Rev. Genet. 24 , 464–483 (2023).
Jain, M. et al. Nanopore sequencing and assembly of a human genome with ultra-long reads. Nat. Biotechnol. 36 , 338–345 (2018).
Poplin, R. et al. A universal SNP and small-indel variant caller using deep neural networks. Nat. Biotechnol. 36 , 983–987 (2018).
Krusche, P. et al. Best practices for benchmarking germline small-variant calls in human genomes. Nat. Biotechnol. 37 , 555–560 (2019).
Grigoriadis, K. et al. CONIPHER: a computational framework for scalable phylogenetic reconstruction with error correction. Nat. Protoc. 19 , 159–183 (2024).
Luquette, L. J. et al. Single-cell genome sequencing of human neurons identifies somatic point mutation and indel enrichment in regulatory elements. Nat. Genet. 54 , 1564–1571 (2022).
Williams, N. et al. Life histories of myeloproliferative neoplasms inferred from phylogenies. Nature 602 , 162–168 (2022).
Ellis, P. et al. Reliable detection of somatic mutations in solid tissues by laser-capture microdissection and low-input DNA sequencing. Nat. Protoc. 16 , 841–871 (2021).
Bae, J. H. et al. Single duplex DNA sequencing with CODEC detects mutations with high sensitivity. Nat. Genet. 55 , 871–879 (2023).
Cibulskis, K. et al. Sensitive detection of somatic point mutations in impure and heterogeneous cancer samples. Nat. Biotechnol. 31 , 213–219 (2013).
Benjamin, D. et al. Calling somatic SNVs and indels with Mutect2. Preprint at bioRxiv 861054 https://doi.org/10.1101/861054 (2019).
Koboldt, D. C. et al. VarScan 2: somatic mutation and copy number alteration discovery in cancer by exome sequencing. Genome Res. 22 , 568–576 (2012).
Jones, D. et al. cgpCaVEManwrapper: simple execution of CaVEMan in order to detect somatic single nucleotide variants in NGS data. Curr. Protoc. Bioinformatics 56 , 15.10.1–15.10.18 (2016).
Yang, X. et al. Control-independent mosaic single nucleotide variant detection with DeepMosaic. Nat. Biotechnol. 41 , 870–877 (2023).
Zhou, W. et al. Global Biobank Meta-analysis Initiative: powering genetic discovery across human disease. Cell Genom. 2 , 100192 (2022).
Hofmeister, R. J., Ribeiro, D. M., Rubinacci, S. & Delaneau, O. Accurate rare variant phasing of whole-genome and whole-exome sequencing data in the UK Biobank. Nat. Genet. 55 , 1243–1249 (2023).
Rubinacci, S., Hofmeister, R. J., Sousa da Mota, B. & Delaneau, O. Imputation of low-coverage sequencing data from 150,119 UK Biobank genomes. Nat. Genet. 55 , 1088–1090 (2023).
Weiner, D. J. et al. Polygenic architecture of rare coding variation across 394,783 exomes. Nature 614 , 492–499 (2023).
Fiziev, P. P. et al. Rare penetrant mutations confer severe risk of common diseases. Science 380 , eabo1131 (2023).
Hujoel, M. L. A. et al. Influences of rare copy-number variation on human complex traits. Cell 185 , 4233–4248.e27 (2022).
Mukamel, R. E. et al. Protein-coding repeat polymorphisms strongly shape diverse human phenotypes. Science 373 , 1499–1505 (2021).
Li, Z. et al. A framework for detecting noncoding rare-variant associations of large-scale whole-genome sequencing studies. Nat. Methods 19 , 1599–1611 (2022).
Selvaraj, M. S. et al. Whole genome sequence analysis of blood lipid levels in >66,000 individuals. Nat. Commun. 13 , 5995 (2022).
Natarajan, P. et al. Deep-coverage whole genome sequences and blood lipids among 16,324 individuals. Nat. Commun. 9 , 3391 (2018).
Li, X. et al. Dynamic incorporation of multiple in silico functional annotations empowers rare variant association analysis of large whole-genome sequencing studies at scale. Nat. Genet. 52 , 969–983 (2020). This study introduces the STAAR series, which exemplifies multiple aspects of advancements in germline association studies: multi-ancestry study population, rare variants, multiple functional annotations and novel methods.
Tang, F. et al. mRNA-Seq whole-transcriptome analysis of a single cell. Nat. Methods 6 , 377–382 (2009).
Chen, K. H., Boettiger, A. N., Moffitt, J. R., Wang, S. & Zhuang, X. RNA imaging. Spatially resolved, highly multiplexed RNA profiling in single cells. Science 348 , aaa6090 (2015).
Buenrostro, J. D., Giresi, P. G., Zaba, L. C., Chang, H. Y. & Greenleaf, W. J. Transposition of native chromatin for fast and sensitive epigenomic profiling of open chromatin, DNA-binding proteins and nucleosome position. Nat. Methods 10 , 1213–1218 (2013).
Johnson, D. S., Mortazavi, A., Myers, R. M. & Wold, B. Genome-wide mapping of in vivo protein-DNA interactions. Science 316 , 1497–1502 (2007).
Nagano, T. et al. Single-cell Hi-C reveals cell-to-cell variability in chromosome structure. Nature 502 , 59–64 (2013).
Lieberman-Aiden, E. et al. Comprehensive mapping of long-range interactions reveals folding principles of the human genome. Science 326 , 289–293 (2009).
Ståhl, P. L. et al. Visualization and analysis of gene expression in tissue sections by spatial transcriptomics. Science 353 , 78–82 (2016).
Cong, L. et al. Multiplex genome engineering using CRISPR/Cas systems. Science 339 , 819–823 (2013).
Haniffa, M. et al. A roadmap for the human developmental cell atlas. Nature 597 , 196–205 (2021).
Nasser, J. et al. Genome-wide enhancer maps link risk variants to disease genes. Nature 593 , 238–243 (2021).
GTEx Consortium. The GTEx Consortium atlas of genetic regulatory effects across human tissues. Science 369 , 1318–1330 (2020).
Article Google Scholar
Zhang, M. J. et al. Polygenic enrichment distinguishes disease associations of individual cells in single-cell RNA-seq data. Nat. Genet. 54 , 1572–1580 (2022).
Weissbrod, O. et al. Functionally informed fine-mapping and polygenic localization of complex trait heritability. Nat. Genet. 52 , 1355–1363 (2020).
Wang, G., Sarkar, A., Carbonetto, P. & Stephens, M. A simple new approach to variable selection in regression, with application to genetic fine mapping. J. R. Stat. Soc. Series B 82 , 1273–1300 (2020).
Gazal, S. et al. Combining SNP-to-gene linking strategies to identify disease genes and assess disease omnigenicity. Nat. Genet. 54 , 827–836 (2022).
Saelens, W., Cannoodt, R., Todorov, H. & Saeys, Y. A comparison of single-cell trajectory inference methods. Nat. Biotechnol. 37 , 547–554 (2019).
Avsec, Ž. et al. Effective gene expression prediction from sequence by integrating long-range interactions. Nat. Methods 18 , 1196–1203 (2021). This study showcases how leveraging deep learning advancement can improve our understanding of genomic biology.
Li, X. et al. Powerful, scalable and resource-efficient meta-analysis of rare variant associations in large whole genome sequencing studies. Nat. Genet. 55 , 154–164 (2023).
Wu, M. C. et al. Rare-variant association testing for sequencing data with the sequence kernel association test. Am. J. Hum. Genet. 89 , 82–93 (2011).
Torkamani, A., Wineinger, N. E. & Topol, E. J. The personal and clinical utility of polygenic risk scores. Nat. Rev. Genet. 19 , 581–590 (2018).
Urbut, S. M., Wang, G., Carbonetto, P. & Stephens, M. Flexible statistical methods for estimating and testing effects in genomic studies with multiple conditions. Nat. Genet. 51 , 187–195 (2019).
Klarin, D. & Natarajan, P. Clinical utility of polygenic risk scores for coronary artery disease. Nat. Rev. Cardiol. 19 , 291–301 (2022).
Patel, A. P. et al. A multi-ancestry polygenic risk score improves risk prediction for coronary artery disease. Nat. Med. 29 , 1793–1803 (2023).
Weir, B. S., Anderson, A. D. & Hepler, A. B. Genetic relatedness analysis: modern data and new challenges. Nat. Rev. Genet. 7 , 771–780 (2006).
Slatkin, M. Linkage disequilibrium — understanding the evolutionary past and mapping the medical future. Nat. Rev. Genet. 9 , 477–485 (2008).
Lawson, D. J. et al. Is population structure in the genetic biobank era irrelevant, a challenge, or an opportunity? Hum. Genet. 139 , 23–41 (2020).
Jyoti, G., Dayal, M. S. & Arguello, A. Developmental genotype-tissue expression (dGTEx). National Human Genome Research Institute https://www.genome.gov/Funded-Programs-Projects/Developmental-Genotype-Tissue-Expression (2020).
Heyde, A. et al. Increased stem cell proliferation in atherosclerosis accelerates clonal hematopoiesis. Cell 184 , 1348–1361.e22 (2021).
Fuster, J. J. et al. Clonal hematopoiesis associated with TET2 deficiency accelerates atherosclerosis development in mice. Science 355 , 842–847 (2017).
Fabre, M. A. et al. The longitudinal dynamics and natural history of clonal haematopoiesis. Nature 606 , 335–342 (2022).
Macaulay, I. C. et al. G&T-seq: parallel sequencing of single-cell genomes and transcriptomes. Nat. Methods 12 , 519–522 (2015).
Muyas, F. et al. De novo detection of somatic mutations in high-throughput single-cell profiling data sets. Nat. Biotechnol . https://doi.org/10.1038/s41587-023-01863-z (2023).
Miles, L. A. et al. Single-cell mutation analysis of clonal evolution in myeloid malignancies. Nature 587 , 477–482 (2020).
Nam, A. S., Chaligne, R. & Landau, D. A. Integrating genetic and non-genetic determinants of cancer evolution by single-cell multi-omics. Nat. Rev. Genet. 22 , 3–18 (2021).
Uddin, M. D. M. et al. Clonal hematopoiesis of indeterminate potential, DNA methylation, and risk for coronary artery disease. Nat. Commun. 13 , 5350 (2022).
Izzo, F. et al. DNA methylation disruption reshapes the hematopoietic differentiation landscape. Nat. Genet. 52 , 378–387 (2020).
Gumuser, E. D. et al. Clonal hematopoiesis of indeterminate potential predicts adverse outcomes in patients with atherosclerotic cardiovascular disease. J. Am. Coll. Cardiol. 81 , 1996–2009 (2023).
Schratz, K. E. et al. Somatic reversion impacts myelodysplastic syndromes and acute myeloid leukemia evolution in the short telomere disorders. J. Clin. Investig. 131 , e147598 (2021).
Revy, P., Kannengiesser, C. & Fischer, A. Somatic genetic rescue in Mendelian haematopoietic diseases. Nat. Rev. Genet. 20 , 582–598 (2019).
Banda, K., Swisher, E. M., Wu, D., Pritchard, C. C. & Gadi, V. K. Somatic reversion of germline BRCA2 mutation confers resistance to poly(ADP-ribose) polymerase inhibitor therapy. JCO Precis. Oncol. 2 , 1–6 (2018).
Ashworth, A. Drug resistance caused by reversion mutation. Cancer Res. 68 , 10021–10023 (2008).
Sakai, W. et al. Secondary mutations as a mechanism of cisplatin resistance in BRCA2 -mutated cancers. Nature 451 , 1116–1120 (2008).
Saha, K. et al. The NIH somatic cell genome editing program. Nature 592 , 195–204 (2021).
Biswas, P. & Verma, R. S. Somatic mosaicism in inherited bone marrow failure and chromosomal instability syndrome. Genome Instab. Dis. 2 , 150–163 (2021).
Article CAS Google Scholar
Sebert, M. et al. Clonal hematopoiesis driven by chromosome 1q/MDM4 trisomy defines a canonical route toward leukemia in Fanconi anemia. Cell Stem Cell 30 , 153–170.e9 (2023).
Steinberg, G. D., Carter, B. S., Beaty, T. H., Childs, B. & Walsh, P. C. Family history and the risk of prostate cancer. Prostate 17 , 337–347 (1990).
DeBoy, E. A. et al. Familial clonal hematopoiesis in a long telomere syndrome. N. Engl. J. Med. 388 , 2422–2433 (2023).
McNally, E. J., Luncsford, P. J. & Armanios, M. Long telomeres and cancer risk: the price of cellular immortality. J. Clin. Investig. 129 , 3474–3481 (2019).
Franch-Expósito, S. et al. Associations between cancer predisposition mutations and clonal hematopoiesis in patients with solid tumors. JCO Precis. Oncol. 7 , e2300070 (2023).
Bick, A. G. et al. Inherited causes of clonal haematopoiesis in 97,691 whole genomes. Nature 586 , 763–768 (2020). This is a landmark study examining the germline genetic basis of one type of somatic mutation using population-level data.
Uddin, M. M. et al. Germline genomic and phenomic landscape of clonal hematopoiesis in 323,112 individuals. Preprint at medRxiv https://doi.org/10.1101/2022.07.29.22278015 (2022).
Kessler, M. D. et al. Common and rare variant associations with clonal haematopoiesis phenotypes. Nature 612 , 301–309 (2022).
Liu, A. et al. Population analyses of mosaic X chromosome loss identify genetic drivers and widespread signatures of cellular selection. Preprint at medRxiv https://doi.org/10.1101/2023.01.28.23285140 (2023).
Weinstock, J. S. et al. Aberrant activation of TCL1A promotes stem cell expansion in clonal haematopoiesis. Nature 616 , 755–763 (2023).
Bick, A. G. et al. Genetic interleukin 6 signaling deficiency attenuates cardiovascular risk in clonal hematopoiesis. Circulation 141 , 124–131 (2020).
Fidler, T. P. et al. The AIM2 inflammasome exacerbates atherosclerosis in clonal haematopoiesis. Nature 592 , 296–301 (2021).
Yu, Z. et al. Genetic modification of inflammation and clonal hematopoiesis-associated cardiovascular risk. J. Clin. Investig. 133 , e168597 (2023).
Hall, J. M. et al. Linkage of early-onset familial breast cancer to chromosome 17q21. Science 250 , 1684–1689 (1990).
Pareja, F. et al. Cancer-causative mutations occurring in early embryogenesis. Cancer Discov. 12 , 949–957 (2022).
Zhang, Y. D. et al. Assessment of polygenic architecture and risk prediction based on common variants across fourteen cancers. Nat. Commun. 11 , 3353 (2020).
Saha, R. et al. Heritability of endometriosis. Fertil. Steril. 104 , 947–952 (2015).
Anglesio, M. S. et al. Cancer-associated mutations in endometriosis without cancer. N. Engl. J. Med. 376 , 1835–1848 (2017).
Savola, P. et al. Somatic mutations in clonally expanded cytotoxic T lymphocytes in patients with newly diagnosed rheumatoid arthritis. Nat. Commun. 8 , 15869 (2017).
Magerus, A., Bercher-Brayer, C. & Rieux-Laucat, F. The genetic landscape of the FAS pathway deficiencies. Biomed. J. 44 , 388–399 (2021).
Bouzid, H. et al. Clonal hematopoiesis is associated with protection from Alzheimer’s disease. Nat. Med. 29 , 1662–1670 (2023).
Weeks, L. D. et al. Age-related diseases of inflammation in myelodysplastic syndrome and chronic myelomonocytic leukemia. Blood 139 , 1246–1250 (2022).
Weinstock, J. S. et al. The genetic determinants of recurrent somatic mutations in 43,693 blood genomes. Sci. Adv. 9 , eabm4945 (2023).
Office of the Commissioner. FDA approves first gene therapies to treat patients with sickle cell disease. U.S. Food and Drug Administration https://www.fda.gov/news-events/press-announcements/fda-approves-first-gene-therapies-treat-patients-sickle-cell-disease (2023).
Robertson, N. A. et al. Longitudinal dynamics of clonal hematopoiesis identifies gene-specific fitness effects. Nat. Med. 28 , 1439–1446 (2022).
National Institutes of Health. Somatic Mosaicism across Human Tissues (SMaHT). NIH https://commonfund.nih.gov/smaht (2021).
Hernan, M. A. & Robins, J. M. Causal Inference: What If 1st edn (Taylor & Francis Group, 2023).
Zeng, H. et al. Integrative in situ mapping of single-cell transcriptional states and tissue histopathology in a mouse model of Alzheimer’s disease. Nat. Neurosci. 26 , 430–446 (2023).
CAS PubMed Google Scholar
Zeng, H. et al. Spatially resolved single-cell translatomics at molecular resolution. Science 380 , eadd3067 (2023).
Genovese, G. et al. Clonal hematopoiesis and blood-cancer risk inferred from blood DNA sequence. N. Engl. J. Med. 371 , 2477–2487 (2014).
Yu, Z. et al. Polygenic risk scores for kidney function and their associations with circulating proteome, and incident kidney diseases. J. Am. Soc. Nephrol. 32 , 3161–3173 (2021).
Sondka, Z. et al. COSMIC: a curated database of somatic variants and clinical data for cancer. Nucleic Acids Res. 52 , D1210–D1217 (2024).
Bergström, A. et al. Insights into human genetic variation and population history from 929 diverse genomes. Science 367 , eaay5012 (2020).
Spielmann, M., Lupiáñez, D. G. & Mundlos, S. Structural variation in the 3D genome. Nat. Rev. Genet. 19 , 453–467 (2018).
Grandi, F. C., Modi, H., Kampman, L. & Corces, M. R. Chromatin accessibility profiling by ATAC-seq. Nat. Protoc. 17 , 1518–1552 (2022).
International Human Genome Sequencing Consortium. Finishing the euchromatic sequence of the human genome. Nature 431 , 931–945 (2004).
Lander, E. S. et al. Initial sequencing and analysis of the human genome. Nature 409 , 860–921 (2001).
Bycroft, C. et al. The UK Biobank resource with deep phenotyping and genomic data. Nature 562 , 203–209 (2018).
Taliun, D. et al. Sequencing of 53,831 diverse genomes from the NHLBI TOPMed Program. Nature 590 , 290–299 (2021).
Backman, J. D. et al. Exome sequencing and analysis of 454,787 UK Biobank participants. Nature 599 , 628–634 (2021).
Espina, V. et al. Laser-capture microdissection. Nat. Protoc. 1 , 586–603 (2006).
Lan, F., Demaree, B., Ahmed, N. & Abate, A. R. Single-cell genome sequencing at ultra-high-throughput with microfluidic droplet barcoding. Nat. Biotechnol. 35 , 640–646 (2017).
Gawad, C., Koh, W. & Quake, S. R. Single-cell genome sequencing: current state of the science. Nat. Rev. Genet. 17 , 175–188 (2016).
Emmert-Buck, M. R. et al. Laser capture microdissection. Science 274 , 998–1001 (1996).
Bonner, R. F. et al. Laser capture microdissection: molecular analysis of tissue. Science 278 , 1481–1483 (1997).
Navin, N. et al. Tumour evolution inferred by single-cell sequencing. Nature 472 , 90–94 (2011).
Payne, A. C. et al. In situ genome sequencing resolves DNA sequence and structure in intact biological samples. Science 371 , eaay3446 (2021).
Rao, A., Barkley, D., França, G. S. & Yanai, I. Exploring tissue architecture using spatial transcriptomics. Nature 596 , 211–220 (2021).
Jagadeesh, K. A. et al. Identifying disease-critical cell types and cellular processes by integrating single-cell RNA-sequencing and human genetics. Nat. Genet. 54 , 1479–1492 (2022).
Lomakin, A. et al. Spatial genomics maps the structure, nature and evolution of cancer clones. Nature 611 , 594–602 (2022).
Download references
Author information
These authors contributed equally: Zhi Yu, Tim H. H. Coorens.
Authors and Affiliations
Broad Institute of MIT and Harvard, Cambridge, MA, USA
Zhi Yu, Tim H. H. Coorens, Md Mesbah Uddin, Kristin G. Ardlie, Niall Lennon & Pradeep Natarajan
Cardiovascular Research Center and Center for Genomic Medicine, Massachusetts General Hospital, Boston, MA, USA
Zhi Yu, Md Mesbah Uddin & Pradeep Natarajan
Department of Medicine, Harvard Medical School, Boston, MA, USA
Pradeep Natarajan
You can also search for this author in PubMed Google Scholar
Contributions
T.H.H.C. and Y.Z. researched the literature. T.H.H.C., P.N. and Y.Z. contributed substantially to discussion of the content. T.H.H.C., M.M.U. and Y.Z. wrote the article. All authors reviewed and/or edited the manuscript before submission.
Corresponding author
Correspondence to Pradeep Natarajan .
Ethics declarations
Competing interests.
P.N. reports investigator-initiated grants from Amgen, Apple, Boston Scientific, Novartis and AstraZeneca; personal fees from Allelica, Apple, AstraZeneca, Blackstone Life Sciences, Foresite Labs, Genentech and Novartis; scientific board membership for Esperion Therapeutics, geneXwell and TenSixteen Bio; and spousal employment at Vertex, all unrelated to the present work. P.N. is a scientific co-founder of TenSixteen Bio, which is a company focused on clonal haematopoiesis but had no role in the present work. The other authors declare no competing interests.
Peer review
Peer review information.
Nature Reviews Genetics thanks Jan O. Korbel and the other, anonymous, reviewer(s) for their contribution to the peer review of this work.
Additional information
Publisher’s note Springer Nature remains neutral with regard to jurisdictional claims in published maps and institutional affiliations.
Related links
COSMIC: https://cancer.sanger.ac.uk/cosmic
Rights and permissions
Springer Nature or its licensor (e.g. a society or other partner) holds exclusive rights to this article under a publishing agreement with the author(s) or other rightsholder(s); author self-archiving of the accepted manuscript version of this article is solely governed by the terms of such publishing agreement and applicable law.
Reprints and permissions
About this article
Cite this article.
Yu, Z., Coorens, T.H.H., Uddin, M.M. et al. Genetic variation across and within individuals. Nat Rev Genet (2024). https://doi.org/10.1038/s41576-024-00709-x
Download citation
Accepted : 09 February 2024
Published : 28 March 2024
DOI : https://doi.org/10.1038/s41576-024-00709-x
Share this article
Anyone you share the following link with will be able to read this content:
Sorry, a shareable link is not currently available for this article.
Provided by the Springer Nature SharedIt content-sharing initiative
Quick links
- Explore articles by subject
- Guide to authors
- Editorial policies
Sign up for the Nature Briefing newsletter — what matters in science, free to your inbox daily.

ORIGINAL RESEARCH article
Genetic variation and the distribution of variant types in the horse.
- 1 Department of Veterinary Population Medicine, University of Minnesota, Minneapolis, MN, United States
- 2 Interval Bio LLC, Mountain View, CA, United States
- 3 Department of Veterinary and Biomedical Sciences, University of Minnesota, Minneapolis, MN, United States
Genetic variation is a key contributor to health and disease. Understanding the link between an individual’s genotype and the corresponding phenotype is a major goal of medical genetics. Whole genome sequencing (WGS) within and across populations enables highly efficient variant discovery and elucidation of the molecular nature of virtually all genetic variation. Here, we report the largest catalog of genetic variation for the horse, a species of importance as a model for human athletic and performance related traits, using WGS of 534 horses. We show the extent of agreement between two commonly used variant callers. In data from ten target breeds that represent major breed clusters in the domestic horse, we demonstrate the distribution of variants, their allele frequencies across breeds, and identify variants that are unique to a single breed. We investigate variants with no homozygotes that may be potential embryonic lethal variants, as well as variants present in all individuals that likely represent regions of the genome with errors, poor annotation or where the reference genome carries a variant. Finally, we show regions of the genome that have higher or lower levels of genetic variation compared to the genome average. This catalog can be used for variant prioritization for important equine diseases and traits, and to provide key information about regions of the genome where the assembly and/or annotation need to be improved.
1 Introduction
Genetic variation is a key contributor to health and disease, and understanding the link between an individual’s genotype and the corresponding phenotype is a major goal of genetic research ( Genomes Project et al., 2010 ). Whole genome sequencing (WGS) within and across populations enables highly efficient variant discovery and elucidation of the molecular nature of virtually all genetic variation, from single nucleotide polymorphisms (SNPs) to copy number variants (CNVs) and other large structural variants ( Sudmant et al., 2015 ). Large-scale studies of genetic variation in humans have dramatically improved our understanding of genetic variation across a species and within populations ( Genomes Project et al., 2012 ). Large-scale variant catalogs establish patterns of variation across the genome, including non-coding regions ( Mu et al., 2011 ), permitting elucidation of regional variability in mutation and recombination rates. Knowledge of the background genetic “noise” helps to decipher the link between genotype and phenotype—allowing filtering of likely neutral variants from potentially deleterious variants in genomic regions of interest or within biologic candidate genes.
The equine reference genome ( Wade et al., 2009 ) has provided a key basis for genetic investigations in horse populations ( Rebolledo-Mendez et al., 2015 ; Raudsepp et al., 2019 ). However, despite consistent progress in our understanding of genetic disease in the horse, disease-causing variants have been identified for less than 20% of the currently recognized genetic diseases (Online Mendelian Inheritance in Animals, OMIA). Similar to humans, many of the significant GWAS regions of interest found for equine traits have been in non-coding regions of the genome. The unknown function of these regions has been a barrier to pinpointing and confirming the causal variant, which is necessary to develop effective genetic tests or targeted treatments. A more complete account of “normal” genetic variation in the horse is critical to establishing the link between genotype and phenotype ( Yngvadottir et al., 2009 ; Genomes Project et al., 2010 ).
Here we provide the first large-scale catalog of genetic variation in the horse derived from WGS, with the intent of describing variant numbers, types, allele frequencies, and genomic location distribution across the general population and within and across major breeds.
2 Materials and Methods
2.1 samples.
Paired-end whole genome sequencing was performed on 534 horses using Illumina technology ( Supplementary Table S1 ). Forty-four different breeds predominantly from North America and Central Europe were selected ( Supplementary Table S2 ) based on availability of Illumina WGS from previous and ongoing studies ( Finno et al., 2015 ; McCoy et al., 2016 ; Norton et al., 2016 , 2019 ; Schultz 2016 ; Bellone et al., 2017 ; Schaefer et al., 2017 ), publicly available data from the Sequence Read Archive ( Leinonen et al., 2011 ), and with the aim of collecting a minimum of 15 individuals per breed for 10 target breeds (Arabian, Belgian, Clydesdale, Icelandic, Morgan, Quarter Horse, Shetland, Standardbred, Thoroughbred, and Welsh Pony) that represent major groups of worldwide equine genetic diversity ( Petersen et al., 2013 ).
2.2 Mapping and Variant Calling
Standard fastq quality control and trimming were performed using Fastqc 0.11.8 and Trimmomatic 0.38, respectively. Raw reads were aligned to the EquCab 3.0 reference horse assembly ( Kalbfleisch et al., 2018 ) and variants identified using a modified version of the genome analysis toolkit best practices ( Van der Auwera et al., 2013 ), modified to allow for joint variant calling by GATK haplotype caller ( McKenna et al., 2010 ) and BCFtools mpileup ( Li H. et al., 2009 ). In brief, reads were mapped to the EquCab 3.0 reference genome using Burrows-Wheeler Aligner (BWA) ( Li and Durbin 2009 ). PCR duplicates were detected and removed using Picard tools ( https://broadinstitute.github.io/picard/ ) version 2.18.27 and then indel realignment was performed using the Genome Analysis Toolkit (GATK) version 4.1.0.0 indel realigner and base-quality score recalibration was performed ( DePristo et al., 2011 ). Genome variant call format (genome VCF) files were produced for each individual horse, and then group variant calling was performed using GATK haplotype caller version 4.1.0.0 ( McKenna et al., 2010 ) and BCFtools mpileup version 1.9 ( Li H. et al., 2009 ) using default settings. Hard-filtering was performed using the GATK best practice guidelines ( Van der Auwera et al., 2013 ). To maximize the specificity of the variants, the intersection of the variants across both callers was obtained using GATK “SelectVariants” and was used for downstream analysis ( Van der Auwera et al., 2013 ).
2.3 Variant Analyses
Descriptive statistics for both variant callers and the intersection were created using BCFtools ( Li 2011 ). Missingness for each horse and each variant site was calculated using VCFtools. The transition to transversion (TsTv) and heterozygous to non-reference homozygous (hetNRhom) ratios were calculated for each variant caller, across the population and by breed using BCFtools ( Li 2011 ) and Python. Predicted functional effect for each variant in the intersect file was determined using SnpEff ( Cingolani et al., 2012b ) with a custom dictionary based on the RefSeq version of EquCab 3.0 ( Schaefer et al., 2017 ). High, moderate, and low impact variants were extracted using SnpSift ( Cingolani et al., 2012a ) and Python was used to manipulate VCF files. Python and BCFtools ( Li H. et al., 2009 ) were used to manipulate output files.
2.4 Breed Differences Between Variants
Variants that were considered rare (<3%) or common (>10%) were extracted from each breed VCF file using Python and BCFtools ( Li H. et al., 2009 ). Variants that were rare in one breed and common in another, rare/common or common/rare in the breed/population, or uniquely present in one breed were selected for investigation. BCFtools view was used to extract variants that were only present in homozygous states, or were present in all genotyped individuals. Python was to extract variant details.
2.5 Regions of the Genome With High or Low Genetic Variation
BCFtools stats was used on 10 kb regions across the genome. R was used to calculate the average genetic variation and to find regions with high (more than twice the average variation) and low (less than half the average variation) levels of genetic variation. BCFtools view was used to extract these regions from the intersect. Python was then used to extract variant details and additional analysis performed with R.
2.6 Statistical Analyses
A Kruskal Wallis test and linear regression were used to determine if there were breed differences. The nonlinear relationship between depth of coverage and the number of variants identified was determined using R. Due to the association between depth of coverage and the number of variants detected, estimated marginal means (EMMEANS) ( Lenth 2018 ) were calculated, with depth of coverage included in the regression models. T tests were used to compare variant types and allele frequencies between coding and non-coding variants, and to compare constraint metric scores between high and low variation regions. A chi-square test was used to compare the variant impact between the high and low variation regions. Confidence intervals (95%) were calculated for each breed. All statistical analyses were performed using R. Significance was set at p < 0.05.
3.1 Variant Discovery
WGS of 534 horses across 46 different breeds ( Supplementary Table S2 ), was performed on Illumina platforms ( Supplementary Table S1 ). This sample set included DNA from a minimum of 15 horses in each of 10 target breeds (Arabian, Belgian, Clydesdale, Icelandic Horse, Morgan, Quarter Horse, Shetland Pony, Standardbred, Thoroughbred, and Welsh Pony) that represent major breed clusters in the horse ( Petersen et al., 2013 ), which were sequenced to a target depth of coverage (DOC) of 10X. Raw reads were mapped, quality control was performed, and variants were identified using a modified version of the genome analysis toolkit best practices pipeline ( Van der Auwera et al., 2013 ) (see Section 2). 155,201,208,820 total reads from the 534 horses mapped uniquely to the EquCab 3.0 reference genome ( Kalbfleisch et al., 2018 ). Mean and median read length, uniquely mapped paired reads, and depth of coverage, and ranges for these values are provided in Table 1 .

TABLE 1 . Median, mean, and range of summary statistics of the mapping pipeline derived from WGS data from 534 horses.
GATK Haplotype Caller and BCFtools identified 42,900,494 and 33,395,275 variants, respectively. To increase specificity of the identified variants, the intersect of both variant callers [31,140,769 variants (29,038,030 SNPs and 2,102,379 indels)] was used for downstream analysis ( Table 2 ). On average, there were 2.27 variants (range 0.88–3.12) per kb of sequence. The mean number of heterozygous sites per individual per kb was 1.54 (range 0.56–2.63). There was a significant non-linear association between the number of variants identified and the depth of coverage [DOC (correlation estimate 0.62, p adjusted 0.009)]. The distribution of variants by DOC was similar for each breed ( Figure 1 ), therefore, estimated marginal means (EMMEANs) ( Lenth 2018 ) accounting for DOC were used for further analyses. The median (range) degree of missingness for each individual horse was 0.01 (0.001–0.58) and for each variant site was 0.026 (0.000–0.998) ( Figure 2 ). Missingness by individual was moderately negatively correlated with DOC: correlation −0.45, 95% confidence interval −0.511 to −0.38 and p < 0.001 ( Figure 2 ).

TABLE 2 . Number of variants, TsTv, and HetNRhom ratio from 534 WGS identified by each variant caller [GATK Haplotype Caller (HC) and BCFtools (BT), and the union and intersection of the variant callers].
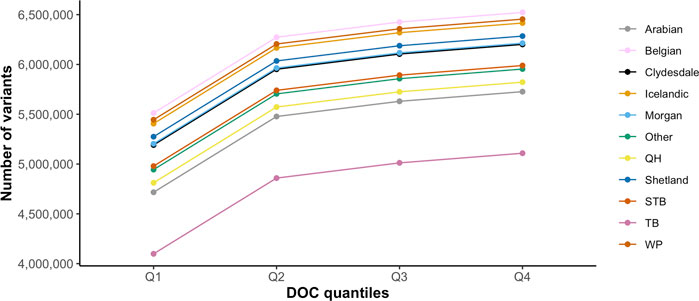
FIGURE 1 . Distribution of the average number of variants identified for each breed by DOC quantiles (Q1 = 1.43–7.14 X, Q2 = 7.15–9.16 X, Q3 = 9.17–14.6 X, Q4 = 14.7–46.7 X). The colored lines represent the 10 target horse breeds [Arabian, Belgian, Clydesdale, Icelandic horse, Morgan horse, Quarter Horse (QH), Shetland, Standardbred (STB), Thoroughbred (TB), and Welsh Pony (WP)] and the remaining horse breeds (Other).
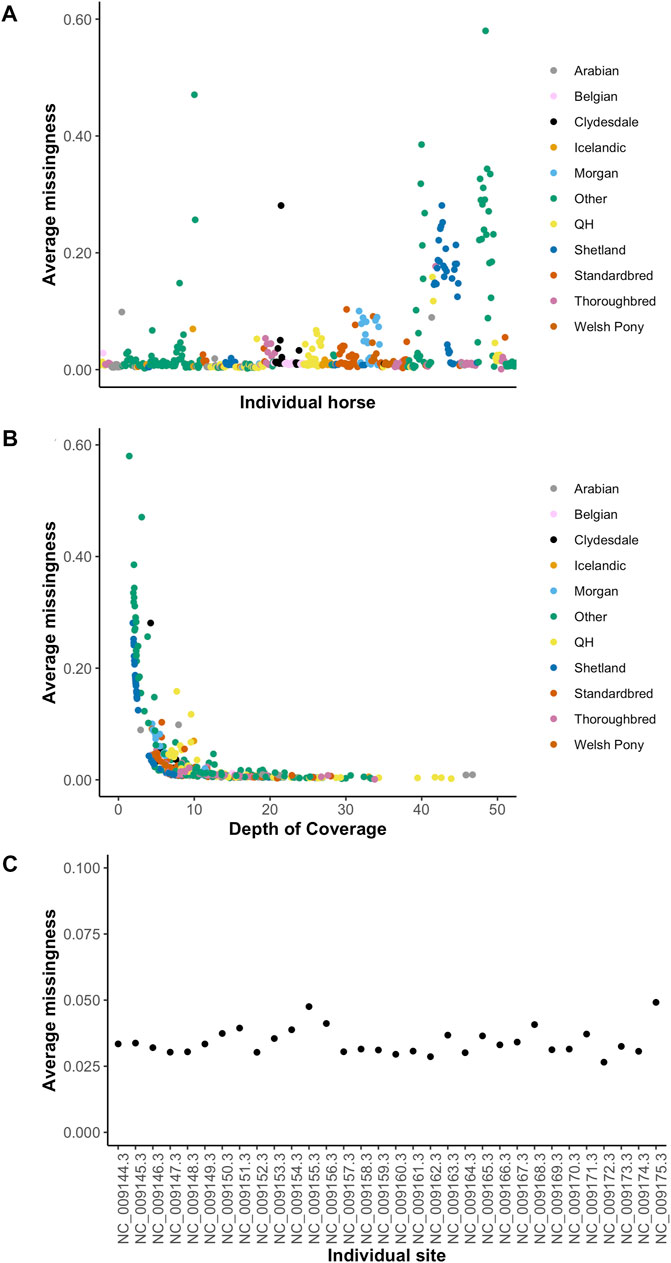
FIGURE 2 . Missingness by individual (A) , by depth of coverage (B) and by chromosome (C) . The 10 target breeds and other breeds are represented in colors shown in the figure legend in Figures 2A,B .
The transition to transversion (TsTv) ratio and the heterozygous to non-reference homozygous (hetNRhom) ratios from the variant callers intersect were 1.94 and 2.24, respectively ( Table 2 ). There were significant but marginal breed differences in the TsTv and hetNRhom ratios ( p < 0.001), with the highest TsTv ratio in Shetlands (1.95) and the lowest in Thoroughbreds (1.92), The same was true with the hetNRhom ratio, which was the highest in Thoroughbreds (3.21) and the lowest in Clydesdales (1.48) ( Table 3 ). The majority (57%) of variants had a minor allele frequency (MAF) < 5%, Figure 3 ), with the mean MAF being 13.2% (0.09–100%). In total, there were 2,481,075 (2,447,610 SNPs and 33,465 indels) singleton variants.
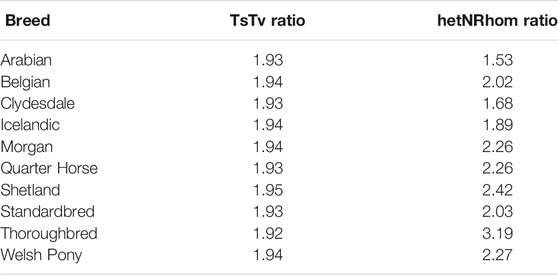
TABLE 3 . Estimated marginal mean of the transition to transversion (TsTv) and heterozygous to non-reference homozygous (hetNRhom) ratios accounting for depth of coverage.
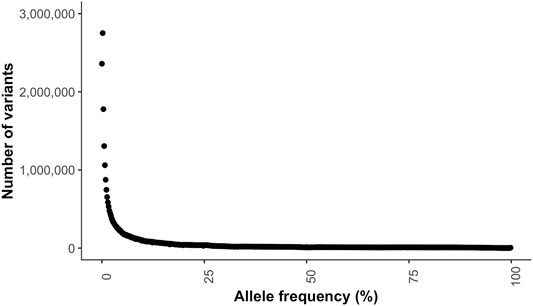
FIGURE 3 . Minor allele frequency distribution of the variants.
Each individual horse had on average 5,580,202 variants (5,099,978 SNPs and 480,224 indels), with on average 1,805,127 in homozygous and 3,775,075 in heterozygous states ( Table 4 ). There were also breed-specific differences in variant number and homozygous variant number per individual ( Table 4 ). The EMMEAN variants per individual, accounting for DOC, was lowest in Thoroughbreds (5,000,516) and highest in Belgians (6,100,544). The EMMEAN homozygous variants per individual, accounting for DOC, was also lowest in Thoroughbreds (1,225,441) and highest in Belgians (2,325,470).
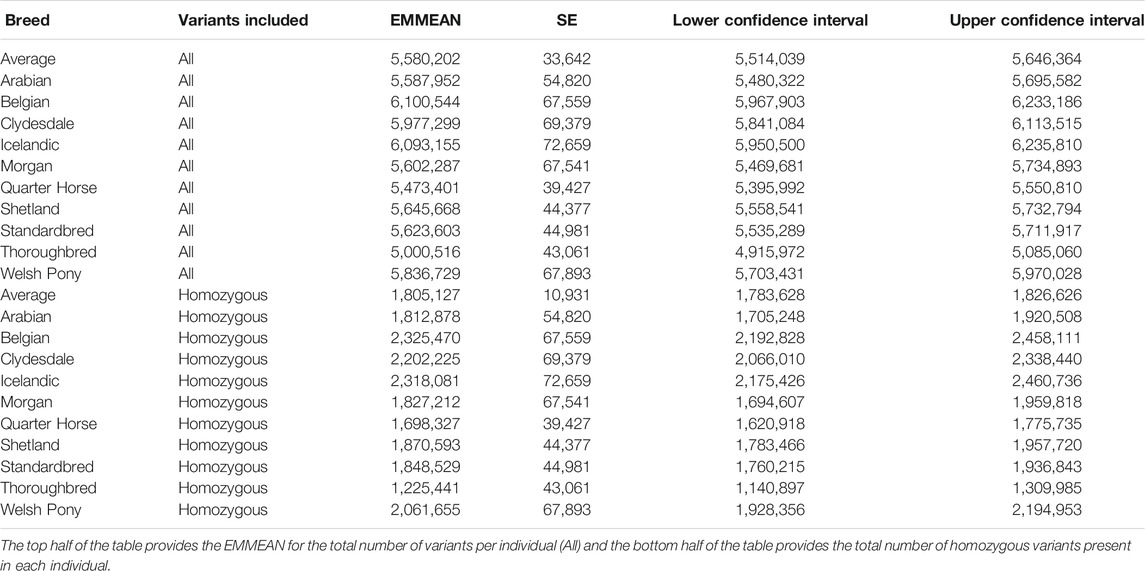
TABLE 4 . EMMEANs for number of variants within breeds accounting for DOC, standard error (SE), and 95% confidence intervals, with breed and DOC as predictor variables.
The EMMEAN variant number per breed was significantly correlated with one estimation of effective population size across breeds ( Petersen et al., 2013 ) ( p = 0.02, Pearson’s correlation = 0.83, 95% confidence interval 0.22–0.97), but not a more recent estimate of effective population size across breeds using a higher marker density ( Beeson et al., 2019 ) ( p = 0.54, Pearson’s correlation = 0.26, 95% confidence interval −0.54–0.82). The EMMEAN homozygous variant number per breed was not significantly correlated with either estimate ( Petersen et al., 2013 ; Beeson et al., 2019 ) of effective population size ( p = 0.07, Pearson’s correlation = 0.72, 95% confidence interval −0.08–0.95 and p = 1, Pearson’s correlation = −0.001, 95% confidence interval −0.70–0.70, respectively).
3.2 Variants Shared Across Breeds
In total, 27,719,724 variants (25,386,978 SNPs and 2,332,746 indels) were shared by at least two breeds (shared variants, Supplementary Table S3 ). Only 2% (637,610) of these variants were genic (within 5,000 bp of a gene). Genic variants included 8,427 high (4,934 SNPs and 3,493 indels), 199,243 moderate (195,630 SNPs and 3,613 indels), and 429,940 low (427,436 SNPs and 2,504 indels) impact variants with 6,697 predicted to cause loss of function (LOF). Genic variants were within or near 10,013 individual genes. The mean shared variant MAF was 0.07. The mean shared variants per breed was 13,802,105 (range 11,800,825 in Clydesdales to 15,724,634 in Quarter Horses).
3.3 Variants With Large Allele Frequency Discrepancies
10,633,492 variants (121,900 genic) were considered rare (MAF <3%) in one breed and common (MAF >10%) in at least one other breed ( Supplementary Table S4 ). Each breed had on average 1,216,800 variants that had large allele frequency discrepancies with another breed. The fewest number of variant discrepancies were between the Quarter Horse and Thoroughbred (728,459 variants) and the highest number of variant discrepancies were between the Thoroughbred and the Shetland (2,028,658 variants).
4,876,293 variants (190,653 genic) were rare (MAF <3%) in one breed and common (MAF >10%, mean MAF 0.15) in the remainder of the study population (excluding individuals of that breed) (breed-specific rare variants, Supplementary Table S4 ). Each genic variant was present in or close to at least one of 10,361 genes. The mean number of breed-specific rare variants was 475,458. The fewest number of variant discrepancies was between the Quarter Horse and the population (8,248 variants) and the highest number of variant discrepancies was between the Thoroughbred and the population (913,739).
3,563,454 variants (62,803 coding) were common (MAF >10%) in one breed and rare (MAF <3%, mean MAF 0.02) in the remaining population (excluding individuals of that breed) (breed-specific common variants, Supplementary Table S4 ). On average, each of these variants was present in 1.13 breeds (range 1–5) and each genic variant was shared by 1.11 breeds (range 1–4). Genic variants were present in or close to at least one of 10,163 genes. On average, each breed had 444,464 variants that were common in the breed and rare in the population. The fewest number of variant discrepancies was between the Thoroughbred and the population (115,241 variants) and the highest number of variant discrepancies was between the Icelandic horse and the population (745,623).
3.4 Variants With No Homozygotes Present
2,889 variants (2,586 SNPs, 303 indels) were only present in a heterozygous state, with no homozygotes identified. Twenty-six of these variants were present within 14 different genes. 12/14 of these genes are uncharacterized or equine specific transcripts or olfactory related genes. Six were predicted to be high impact (four were predicted to be LOF variants), 10 were predicted to be moderate impact, and 10 were predicted to be low impact variants ( Supplementary Table S5 ). The allele frequency was marginally but significantly different ( p = 0.003) between non-genic (0.498) and genic (0.499) variants.
3.5 Variants Present in all Horses
114,733 variants (103,414 SNPs, 11,319 indels) were present in all 534 horses. Of these variants, 1,426 were present in genic regions of 504 genes. These variants were predicted to have a high (170 variants), moderate (644 variants) and low (612 variants) impact on phenotype, with 145 predicted to be LOF variants. Of these variants, 9,756 (9,351 SNPs, 405 indels) were homozygous in all horses. Ninety-two of these were present in genic regions affecting 58 genes. Eight were predicted to be high impact (four were predicted to be LOF), 46 were predicted to be moderate impact, and 38 were predicted to be low impact variants.
3.6 Regions of the Genome With High or Low Genetic Variation
The variant caller intersect file was first split by chromosome and then into 10,000 bp windows (240,910 regions in total) to determine the average number of variants per 10 Kb region across the genome. Regions with more than two times or less than half of the average number of variants were classified as regions of high or low variability, respectively. Each 10 Kb region carried on average 122 variants (range 0–3,143 variants), consisting of 114 SNPs (range 0–3,075 SNPs) and 8 indels (range 0–121). There were 6,341 regions with more than double the mean variant number, including, on average 414 variants (396 SNPs and 18 indels) with a TsTv ratio of 1.76. There were 17,791 regions with less than half the average number of variants, including, on average 35 variants (32 SNPs and three indels) with a TsTv ratio of 1.59.
Highly variable regions contained 2,625,382 variants ( Supplementary Table S6 ) with a mean MAF of 17% (range 0.01–100%). The most common variant types in the high variability regions were intergenic (1,287,507) and intronic (574,441) ( Figure 4 ). The low variability regions contained 20,777 variants with a mean MAF of 11% (range 0.01–100%). The most common variant types in the low variation regions were intronic (306,569) and intergenic (182,937) variants ( Figure 4 ). The variant impact was significantly different between regions of high and low variation [ p < 0.001 ( Table 5 )].
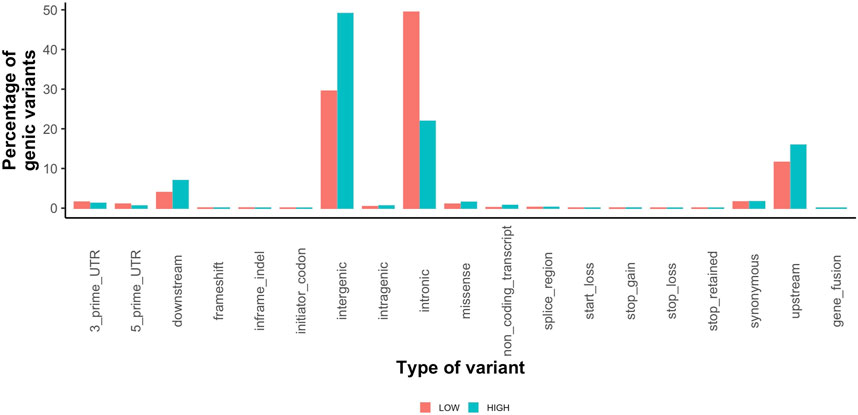
FIGURE 4 . Percentage of coding variants for each type of variant called by SnpEff for low variation regions (orange) and high variation regions (teal).

TABLE 5 . Impact of variants identified in high and low variation regions.
The allele frequency of the variants in the low variability regions was significantly less than the allele frequency of variants in high variability regions ( p < 0.001, 95% confidence interval: 0.06–0.06). For the genes with haploinsufficiency scores available, the mean score was lower ( p < 0.001, 95% confidence interval: −0.15 to −0.10) in genes containing variants in the high variability regions (0.23) compared to genes containing variants in the low variability regions (0.33). The predicted loss of function tolerance score was not significantly different ( p = 0.13, 95% confidence interval: −0.07–0.01) between genes containing variants in the high variability regions (0.33) compared to genes containing variants in the low variability regions (0.37).
4 Discussion
This report comprises the first large-scale catalog of genetic variation developed for the horse, a species with potential as a translational model for many athletic phenotypes. We used WGS of 534 horses to determine overall genetic variation in the general equine population as well as 10 individual breeds, report variants with population and breed MAF discrepancies, identify variants with no homozygotes as well as variants that are present in all individuals, and in addition identify genomic regions with high or low genetic variation.
The significant association between the number of variants identified and the depth of coverage was unsurprising, as it has long been recognized that deeper coverage improves variant calling accuracy ( Li R. et al., 2009 ). The nonlinear association between depth of coverage and number of variants identified is particularly relevant to future genetic studies, as there is minimal to no gain in the numbers of variants detected by increasing depth of coverage over 10X.
We elected to use the intersect of two commonly used callers based on evidence from previous work showing that specificity of identified variants could be improved ( Field et al., 2015 ). This method identified 29,882,273 variants, which is higher than the 25,800,000 variants previously identified in a smaller cohort of 88 horses using only GATK haplotype caller ( Jagannathan et al., 2019b ). This difference is likely due to the increased number of horses and inclusion of more genetically distinct breeds in our study, increasing our ability to identify rare variants down to a MAF across breeds of 0.0009 compared with 0.0057 in the earlier study. Additionally, we use the intersect of two variant callers (GATK HaplotypeCaller and BCFtools) to improve specificity of variants identified in the previous publication ( Jagannathan et al., 2019b ). While this likely would have led to a reduced sensitivity, we were still able to identify an additional 4,082,273 variants.
The 1.54 heterozygous variants per kb of sequence is similar to that reported in cattle (1.44 per kb) ( Daetwyler et al., 2014 ), but is higher than reported in the Yoruba (1.03 per kb) and European human populations (0.68 per kb) ( Genomes Project et al., 2010 ). The cause of this higher variation in the horse is likely related to the heterogeneity of this population, which included 46 different breeds, compared to three cattle breeds ( Daetwyler et al., 2014 ) and only a single population in the human studies ( Genomes Project et al., 2010 ). Given the limited genetic diversity ( Petersen et al., 2013 ) of the horse compared with human populations this is still somewhat somewhat surprising. However, a recent study of effective population size in the horse suggests that several breeds have larger effective population sizes ( Beeson et al., 2019 ) than reported in human populations ( Tenesa et al., 2007 ), and we would therefore, expect to see increased heterozygosity. Another reason for the increased number of heterozygous variants in horses is likely to be related to errors in the reference genome which, unlike the human reference genome that is based on multiple individuals, is based on a single horse ( Kalbfleisch et al., 2018 ). In the original paper from the 1,000 human genomes consortium, it was concluded that a site where every individual was homozygous for an allele not present in the reference genome was a reference genome error. This accounted for ∼1 error per 30 kb of sequence. In this study, we identified 114,733 variants that were present in every individual in this population, which are presumed to be related to an error in the reference genome or a true rare variant that is present in the individual horse sequenced for the reference genome. The length of the RefSeq equine reference genome is 2,506.95 MB, therefore, we would expect to see ∼1.37 errors per 30 kb of sequence, which may partially explain the increased number of variants in horses compared with humans.
Unsurprisingly, the degree of missingness per individual was negatively correlated with depth of coverage. This was not a linear correlation however, and beyond 10X coverage, there was minimal improvement in the degree of missingness, suggesting that 10X coverage is a reasonable target for population scale sequencing projects. The average missingness per individual varied greatly. Most of the horses with missingness >0.20 were horses that were not included in the breed analysis owing to being in the other breed category. Six of the Shetland ponies had missingness >0.20 and these were ponies with a targeted depth of coverage of ∼ 6X. The degree of missingness across the 32 autosomes and X chromosomes also varied, with the highest degree of missingness on chromosomes 12 and X. This may be related to larger uncharacterized regions on the X chromosome compared to most autosomes.
The TsTv and hetNRhom ratios are frequently utilized for quality control of sequencing data ( Guo et al., 2013 ). The TsTv ratio here was similar (1.94) to reports of the expected TsTv ratio from genome sequencing data in humans of ∼2 ( Genomes Project et al., 2010 ), which is a good indicator of SNP quality ( Guo et al., 2013 ; Wang et al., 2015 ). The slight reduction in our study compared with human studies is likely related to decreased genetic diversity and smaller effective population sizes in horses ( Petersen et al., 2013 ) compared with humans, as well as a lower quality reference genome ( Wade et al., 2009 ). The TsTv ratio varies across the genome, but in humans does not vary based on ancestry ( Wang et al., 2015 ). In the 10 target breeds, we did find that the TsTv ratio varied significantly but marginally by ancestry. This may be related to the varying depth of sequence coverage, which was not uniform across breeds. The hetNRhom ratio (2.24) was higher than the expected value of 2 based on Hardy-Weinberg equilibrium ( Guo et al., 2013 ) and there were breed differences. This is consistent with human ( Wang et al., 2015 ) and canine ( Jagannathan et al., 2019a ) reports that the hetNRhom ratio varies by ancestry. In humans, the highest median hetNRhom ratio was 2.0 in African populations with the lowest ratio of 1.4 in Asian populations; none of the populations investigated had a median hetNRhom ratio >2.0 ( Wang et al., 2015 ). However, at least one dog breed had hetNRhom ratio of 3.3 in the catalog of canine genetic variation ( Jagannathan et al., 2019a ). This is likely related to the increased levels of inbreeding in horses compared with most human populations.
The variant totals differed by breed, which is consistent with reports in different cattle breeds ( Daetwyler et al., 2014 ) and regional human populations ( Genomes Project et al., 2010 ). Previously, this has been related to effective population size ( Daetwyler et al., 2014 ), and we did see a significant association ( p < 0.0001) between the number of variants identified in each of the 10 target breeds and a report of effective population size using 54K SNP array data ( Petersen et al., 2013 ). However, this association was not seen with a more recent estimate of effective population size that used imputed genome-wide SNP data ( Beeson et al., 2019 ). This may partially be related to different breeds studied, as the Beeson et al. (2019) paper did not include effective population size estimates for the Shetland and Clydesdale breeds. Additionally, we are not accounting for the degree of relatedness between the breeds studied and the reference genome. In this study, the Thoroughbred (which is the EquCab3 reference genome breed) has the fewest variants compared to the other breeds, consistent with both the reference genome being from a Thoroughbred and having the smallest effective population size (1,784) in Beeson et al. ( Beeson et al., 2019 ). However, the Quarter Horse, which has the largest population size (6,516) ( Beeson et al., 2019 ), but is more related to the Thoroughbred ( Petersen et al., 2013 ) than other breeds, has a number of variants that is closer to the median, consistent with its close relatedness to the Thoroughbred ( Petersen et al., 2013 ), but inconsistent with the large effective population size ( Beeson et al., 2019 ). This would suggest, that while the number of variants does vary by breed, as seen in different human regional populations, the relationship between the horse breed and the reference genome appears to have had an effect in this population.
A large number of variants were shared by at least one other breed, which is not surprising given the close relatedness of the breeds investigated ( Petersen et al., 2013 ). However, there were also multiple variants with large allele frequency discrepancies between breeds. We defined a minor allele frequency <3% as rare and >10% as common due to limitations in the number of horses in each breed investigated, rather than values used in most human studies (<0.5% for rare variants and >5% for common ( Bomba et al., 2017 )). With the 534 horses here our power to detect variants present at a minor allele frequency of 3 and 0.5% in the population is 1.00 and 0.93, respectively. However, it is important to note that for the breed analysis with the least number of horses in the Clydesdales (19) our power to detect these allele frequencies is only 0.44 and 0.01, respectively. To have a power greater than 0.8 to detect all rare variants <3% or <0.5% allele frequency within a breed we would need to sequence 55 and 325 horses within that breed, respectively. We therefore, had 80% power to detect the rare variants (minor allele frequency 3%) in Quarter Horses, Shetlands and Thoroughbreds. The number of variants with marked population discrepancies was quite high, with ∼35% of variants considered rare in one breed and common in another. The reason for large allele frequency differences between populations is thought to be related to genetic drift ( Hofer et al., 2009 ) in humans. However, given that different horse breeds have been selectively bred for different traits ( Avila et al., 2018 ), it is also likely that selection at least partially accounts for some of the variants with large allele frequency discrepancies between breeds. Only ∼5% of variants were unique to a single breed, with about 10% of these being in coding regions. The ∼5% of unique variants in horses is also lower than seen in cattle where ∼31% of variants are unique to a single breed ( Daetwyler et al., 2014 ).
Variants with no homozygotes were explored to determine if there were variants present in the general population that could be embryonic lethal in homozygous form. 2,888/2,889 of these variants were present at a minor allele frequency in the general population greater than would be expected for a homozygous lethal disease (MAF > 0.10). The one variant that was rare had a MAF of 0.03 and was an intergenic in frame deletion. Using the rules of Hardy-Weinberg equilibrium we would need to sequence 1,111 horses to identify just one homozygote, therefore using this dataset alone, we cannot determine if this variant is embryonic lethal. Given that 96% of the variants with no homozygotes have an allele frequency around 0.50, it is highly unlikely that these variants are embryonic lethal; rather it is possible that these regions are related to mapping errors due to the presence of paralogs or pseudogenes, or the presence of structural variants. This is supported by the fact that all but two of the genes containing these variants were uncharacterized or equine only transcripts or olfactory receptor genes.
Identifying regions of the genome with high or low variation in the general population is critically important for the investigation of possible disease-causing variants, as regions with high genetic variability are less likely to contain disease-causing variants for fully penetrant Mendelian diseases ( Karczewski et al., 2019 ). However, our analysis unexpectantly found that genes in high variability regions had lower haploinsufficiency scores, suggesting that damaging variants are less well tolerated ( Huang et al., 2010 ) than for genes present in the low variability regions. This is likely due to the large number of genes without haploinsufficiency scores (70% of high variation region genes and 23% of low variation region genes). 65% of genes in high and 18% of genes in low variation regions had the “LOC” designation that are genes unique to the horse or are only predicted to be equivalent to a human gene, and therefore would not be included in human databases of variant constraint. This would suggest that as expected, genes in the low variability regions are more similar to human genes than genes in the high variability regions.
Overall, this is the first large-scale catalog of genetic variation in the domestic horse and will be highly useful for evaluation of background genetic variation in any future genetic study. This catalog has paved the way for future investigation of the regions of the genome that are shared or have marked MAF discrepancies across breeds. Regions with marked discrepancies between breeds, or between a breed and the population, can then be interrogated to further look for signatures of selection both across breeds, as well as within breeds. Additionally, further investigation into which of the variants that are present in all individuals are related to poor genome annotation or instead are true variants in the reference genome is needed and will lead to improvement of the horse reference genome in the future. By improving knowledge of the poorly annotated regions of the horse genome we will be able to correct these for future versions of the reference genome. This will have benefits for future phenotype-causing variant identification studies. Given the relatively unique utility of the horse as a model for human athletic related traits ( Hill et al., 2010 ; Rooney et al., 2018 ) and diseases ( Ward et al., 2004 ; McCue et al., 2008 ; McIlwraith et al., 2012 ; McCoy et al., 2013 ; Norton et al., 2016 ), an improved ability to identify phenotype-causing variants in the horse may shed light on analogous genetic diseases in humans. This is especially true for complex phenotypes such as athleticism, osteoarthritis ( McIlwraith et al., 2012 ), and exertional rhabdomyolysis ( Norton et al., 2016 ) where the limited genetic diversity in the horse ( Petersen et al., 2013 ) may further accelerate our ability to identify the true phenotype-causing variants. This improvement in the reference genome combined with an improved understanding of the background genetic variation ( Yang et al., 2014 ; Farwell et al., 2015 ; Ellingford et al., 2016 ; Hartmannová et al., 2016 ; Känsäkoski et al., 2016 ; Kojima et al., 2016 ; Noll et al., 2016 ; Smedley et al., 2016 ; Dolzhenko et al., 2017 ; Eldomery et al., 2017 ; Schneider et al., 2017 ) in the horse should vastly increase the identification of phenotype-causing variants for important equine diseases.
Data Availability Statement
The variant datasets presented in this study can be found online at: https://www.ncbi.nlm.nih.gov/bioproject/PRJEB47918 .
Ethics Statement
Ethical review and approval was not required for this animal study because this study used samples previously collected by our lab and collaborators with institutional ethics review and approval and written consent from owners for the participation of their horses this study. The remainder of the samples were publicly available.
Author Contributions
SD-A was involved in the grant-writing, study design, data analysis, and manuscript preparation. MM and JM were responsible for the grant-writing and study design. They also supervised and provided expertise for the data analysis and manuscript preparation. RS, BG, and WC assisted with developing and running the mapping and variant calling pipelines. All authors approved the manuscript prior to submission to the journal.
This work was supported by: USDA NIFA-AFRI Project 2017-67015-26296: Tools to Link Phenotype to Genotype in the Horse, The American Quarter Horse Association, and a University of Minnesota Multistate grant. Salary support for SD-A was provided by an American College of Veterinary Internal Medicine Foundation fellowship, by a T32 Institutional Training Grant in Comparative Medicine and Pathology (5T320D010993-12), by the 2019 Elaine and Bertram Klein Development Award, and a Morris Animal Foundation Postdoctoral Research Fellowship (D20EQ-403).
Conflict of Interest
Authors BG and WC own and work for IntervalBio LLC, the computational company that was compensated to develop the mapping and variant calling pipeline.
The remaining authors declare that the research was conducted in the absence of any commercial or financial relationships that could be construed as a potential conflict of interest.
Publisher’s Note
All claims expressed in this article are solely those of the authors and do not necessarily represent those of their affiliated organizations, or those of the publisher, the editors and the reviewers. Any product that may be evaluated in this article, or claim that may be made by its manufacturer, is not guaranteed or endorsed by the publisher.
Acknowledgments
The authors acknowledge the Minnesota Supercomputing Institute (MSI) at the University of Minnesota for providing resources that contributed to the research results reported within this paper. URL: http://www.msi.umn.edu .
Supplementary Material
The Supplementary Material for this article can be found online at: https://www.frontiersin.org/articles/10.3389/fgene.2021.758366/full#supplementary-material
Auwera, G. A., Carneiro, M. O., Hartl, C., Poplin, R., Del Angel, G., Levy‐Moonshine, A., et al. (2013). From FastQ Data to High‐Confidence Variant Calls: The Genome Analysis Toolkit Best Practices Pipeline. Curr. Protoc. Bioinformatics 43, 111–1033. doi:10.1002/0471250953.bi1110s43
CrossRef Full Text | Google Scholar
Avila, F., Mickelson, J. R., Schaefer, R. J., and McCue, M. E. (2018). Genome-wide Signatures of Selection Reveal Genes Associated with Performance in American Quarter Horse Subpopulations. Front. Genet. 9. doi:10.3389/fgene.2018.00249
PubMed Abstract | CrossRef Full Text | Google Scholar
Beeson, S. K., Mickelson, J. R., and McCue, M. E. (2019). Exploration of fine-scale Recombination Rate Variation in the Domestic Horse. Genome Res. 29, 1744–1752. doi:10.1101/gr.243311.118
Bellone, R. R., Liu, J., Petersen, J. L., Mack, M., Singer-Berk, M., Drögemüller, C., et al. (2017). A Missense Mutation in Damage-specific DNA Binding Protein 2 Is a Genetic Risk Factor for Limbal Squamous Cell Carcinoma in Horses. Int. J. Cancer 141, 342–353. doi:10.1002/ijc.30744
Bomba, L., Walter, K., and Soranzo, N. (2017). The Impact of Rare and Low-Frequency Genetic Variants in Common Disease. Genome Biol. 18. doi:10.1186/s13059-017-1212-4
Cingolani, P., Patel, V. M., Coon, M., Nguyen, T., Land, S. J., Ruden, D. M., et al. (2012a). Using Drosophila melanogaster as a Model for Genotoxic Chemical Mutational Studies with a New Program, SnpSift. Front. Gene 3, 35. doi:10.3389/fgene.2012.00035
Cingolani, P., Platts, A., Wang, L. L., Coon, M., Nguyen, T., Wang, L., et al. (2012b). A Program for Annotating and Predicting the Effects of Single Nucleotide Polymorphisms, SnpEff. Fly 6, 80–92. doi:10.4161/fly.19695
Daetwyler, H. D., Capitan, A., Pausch, H., Stothard, P., van Binsbergen, R., Brøndum, R. F., et al. (2014). Whole-genome Sequencing of 234 Bulls Facilitates Mapping of Monogenic and Complex Traits in Cattle. Nat. Genet. 46, 858–865. doi:10.1038/ng.3034
DePristo, M. A., Banks, E., Poplin, R., Garimella, K. V., Maguire, J. R., Hartl, C., et al. (2011). A Framework for Variation Discovery and Genotyping Using Next-Generation DNA Sequencing Data. Nat. Genet. 43, 491–498. doi:10.1038/ng.806
Dolzhenko, E., van Vugt, J. J. F. A., Shaw, R. J., Bekritsky, M. A., Van Blitterswijk, M., Narzisi, G., et al. (2017). Detection of Long Repeat Expansions from PCR-free Whole-Genome Sequence Data. Genome Res. 27, 1895–1903. doi:10.1101/gr.225672.117
Eldomery, M. K., Coban-Akdemir, Z., Harel, T., Rosenfeld, J. A., Gambin, T., Stray-Pedersen, A., et al. (2017). Lessons Learned from Additional Research Analyses of Unsolved Clinical Exome Cases. Genome Med. 9. doi:10.1186/s13073-017-0412-6
Ellingford, J. M., Barton, S., Bhaskar, S., Williams, S. G., Sergouniotis, P. I., O'Sullivan, J., et al. (2016). Whole Genome Sequencing Increases Molecular Diagnostic Yield Compared with Current Diagnostic Testing for Inherited Retinal Disease. Ophthalmology 123, 1143–1150. doi:10.1016/j.ophtha.2016.01.009
Farwell, K. D., Shahmirzadi, L., El-Khechen, D., Powis, Z., Chao, E. C., Tippin Davis, B., et al. (2015). Enhanced Utility of Family-Centered Diagnostic Exome Sequencing with Inheritance Model-Based Analysis: Results from 500 Unselected Families with Undiagnosed Genetic Conditions. Genet. Med. 17, 578–586. doi:10.1038/gim.2014.154
Field, M. A., Cho, V., Andrews, T. D., and Goodnow, C. C. (2015). Reliably Detecting Clinically Important Variants Requires Both Combined Variant Calls and Optimized Filtering Strategies. PLoS ONE 10, e0143199. doi:10.1371/journal.pone.0143199
Finno, C. J., Stevens, C., Young, A., Affolter, V., Joshi, N. A., Ramsay, S., et al. (2015). SERPINB11 Frameshift Variant Associated with Novel Hoof Specific Phenotype in Connemara Ponies. Plos Genet. 11, e1005122. doi:10.1371/journal.pgen.1005122
Genomes Project, C., Abecasis, G. R., Altshuler, D., Auton, A., Brooks, L. D., Durbin, R. M., et al. (2010). A Map of Human Genome Variation from Population-Scale Sequencing. Nature 467, 1061–1073. doi:10.1038/nature09534
Genomes Project, C., Abecasis, G. R., Auton, A., Brooks, L. D., DePristo, M. A., Durbin, R. M., et al. (2012). An Integrated Map of Genetic Variation from 1,092 Human Genomes. Nature 491, 56–65. doi:10.1038/nature11632
Guo, Y., Ye, F., Sheng, Q., Clark, T., and Samuels, D. C. (2013). Three-stage Quality Control Strategies for DNA Re-sequencing Data. Brief. Bioinform. 15, 879–889. doi:10.1093/bib/bbt069
Hartmannová, H., Piherová, L., Tauchmannová, K., Kidd, K., Acott, P. D., Crocker, J. F. S., et al. (2016). Acadian Variant of Fanconi Syndrome Is Caused by Mitochondrial Respiratory Chain Complex I Deficiency Due to a Non-coding Mutation in Complex I Assembly Factor NDUFAF6. Hum. Mol. Genet. 25, 4062–4079. doi:10.1093/hmg/ddw245
Hill, E. W., McGivney, B. A., Gu, J., Whiston, R., and Machugh, D. E. (2010). A Genome-wide SNP-Association Study Confirms a Sequence Variant (g.66493737C>T) in the Equine Myostatin (MSTN) Gene as the Most Powerful Predictor of Optimum Racing Distance for Thoroughbred Racehorses. BMC genomics 11, 552. doi:10.1186/1471-2164-11-552
Hofer, T., Ray, N., Wegmann, D., and Excoffier, L. (2009). Large Allele Frequency Differences between Human continental Groups Are More Likely to Have Occurred by Drift during Range Expansions Than by Selection. Ann. Hum. Genet. 73, 95–108. doi:10.1111/j.1469-1809.2008.00489.x
Huang, N., Lee, I., Marcotte, E. M., and Hurles, M. E. (2010). Characterising and Predicting Haploinsufficiency in the Human Genome. Plos Genet. 6, e1001154. doi:10.1371/journal.pgen.1001154
Jagannathan, V., Drögemüller, C., Leeb, T., Aguirre, G., André, C., Bannasch, D., et al. (2019a). A Comprehensive Biomedical Variant Catalogue Based on Whole Genome Sequences of 582 Dogs and Eight Wolves. Anim. Genet. 50, 695–704. doi:10.1111/age.12834
Jagannathan, V., Gerber, V., Rieder, S., Tetens, J., Thaller, G., Drögemüller, C., et al. (2019b). Comprehensive Characterization of Horse Genome Variation by Whole-Genome Sequencing of 88 Horses. Anim. Genet. 50, 74–77. doi:10.1111/age.12753
Kalbfleisch, T. S., Rice, E. S., DePriest, M. S., Walenz, B. P., Hestand, M. S., Vermeesch, J. R., et al. (2018). EquCab3, an Updated Reference Genome for the Domestic Horse . bioRxiv. doi:10.1101/306928
Känsäkoski, J., Jääskeläinen, J., Jääskeläinen, T., Tommiska, J., Saarinen, L., Lehtonen, R., et al. (2016). Complete Androgen Insensitivity Syndrome Caused by a Deep Intronic Pseudoexon-Activating Mutation in the Androgen Receptor Gene. Sci. Rep. 6. doi:10.1038/srep32819
Karczewski, K. J., Francioli, L. C., Tiao, G., Cummings, B. B., Alföldi, J., Wang, Q., et al. (2019). Variation across 141,456 Human Exomes and Genomes Reveals the Spectrum of Loss-Of-Function Intolerance across Human Protein-Coding Genes. bioRxiv . doi:10.1101/531210
Kojima, K., Kawai, Y., Misawa, K., Mimori, T., and Nagasaki, M. (2016). STR-realigner: A Realignment Method for Short Tandem Repeat Regions. BMC Genomics 17. doi:10.1186/s12864-016-3294-x
Leinonen, R., Sugawara, H., and Shumway, M. (2011). The Sequence Read Archive. Nucleic Acids Res. 39, D19–D21. doi:10.1093/nar/gkq1019
Lenth, R. V. (2018). Emmeans: Estimated Marginal Means, Aka Least-Squares Means .
Google Scholar
Li, H. (2011). A Statistical Framework for SNP Calling, Mutation Discovery, Association Mapping and Population Genetical Parameter Estimation from Sequencing Data. Bioinformatics 27, 2987–2993. doi:10.1093/bioinformatics/btr509
Li, H., and Durbin, R. (2009). Fast and Accurate Short Read Alignment with Burrows-Wheeler Transform. Bioinformatics 25, 1754–1760. doi:10.1093/bioinformatics/btp324
Li, H., Handsaker, B., Wysoker, A., Fennell, T., Ruan, J., Homer, N., et al. (2009a). The Sequence Alignment/Map Format and SAMtools. Bioinformatics 25, 2078–2079. doi:10.1093/bioinformatics/btp352
Li, R., Li, Y., Fang, X., Yang, H., Wang, J., Kristiansen, K., et al. (2009b). SNP Detection for Massively Parallel Whole-Genome Resequencing. Genome Res. 19, 1124–1132. doi:10.1101/gr.088013.108
McCoy, A. M., Beeson, S. K., Splan, R. K., Lykkjen, S., Ralston, S. L., Mickelson, J. R., et al. (2016). Identification and Validation of Risk Loci for Osteochondrosis in Standardbreds. BMC genomics 17, 41–0162385. doi:10.1186/s12864-016-2385-z
McCoy, A. M., Toth, F., Dolvik, N. I., Ekman, S., Ellermann, J., Olstad, K., et al. (2013). Articular Osteochondrosis: A Comparison of Naturally-Occurring Human and Animal Disease, Osteoarthritis Cartilage 21, 1638–1647. doi:10.1016/j.joca.2013.08.011
McCue, M. E., Valberg, S. J., Miller, M. B., Wade, C., DiMauro, S., Akman, H. O., et al. (2008). Glycogen Synthase (GYS1) Mutation Causes a Novel Skeletal Muscle Glycogenosis. Genomics 91, 458–466. doi:10.1016/j.ygeno.2008.01.011
McIlwraith, C. W., Frisbie, D. D., and Kawcak, C. E. (2012). The Horse as a Model of Naturally Occurring Osteoarthritis. Bone Jt. Res. 1, 297–309. doi:10.1302/2046-3758.111.2000132
McKenna, A., Hanna, M., Banks, E., Sivachenko, A., Cibulskis, K., Kernytsky, A., et al. (2010). The Genome Analysis Toolkit: a MapReduce Framework for Analyzing Next-Generation DNA Sequencing Data. Genome Res. 20, 1297–1303. doi:10.1101/gr.107524.110
Mu, X. J., Lu, Z. J., Kong, Y., Lam, H. Y. K., and Gerstein, M. B. (2011). Analysis of Genomic Variation in Non-coding Elements Using Population-Scale Sequencing Data from the 1000 Genomes Project. Nucleic Acids Res. 39, 7058–7076. doi:10.1093/nar/gkr342
Noll, A. C., Miller, N. A., Smith, L. D., Yoo, B., Fiedler, S., Cooley, L. D., et al. (2016). Clinical Detection of Deletion Structural Variants in Whole-Genome Sequences. Npj Genomic Med. 1. doi:10.1038/npjgenmed.2016.26
Norton, E. M., Avila, F., Schultz, N. E., Mickelson, J. R., Geor, R. J., and McCue, M. E. (2019). Evaluation of an HMGA2 Variant for Pleiotropic Effects on Height and Metabolic Traits in Ponies. J. Vet. Intern. Med. 33, 942–952. doi:10.1111/jvim.15403
Norton, E. M., Mickelson, J. R., Binns, M. M., Blott, S. C., Caputo, P., Isgren, C. M., et al. (2016). Heritability of Recurrent Exertional Rhabdomyolysis in Standardbred and Thoroughbred Racehorses Derived from SNP Genotyping Data. Jhered 107, 537–543. doi:10.1093/jhered/esw042
Petersen, J. L., Mickelson, J. R., Cothran, E. G., Andersson, L. S., Axelsson, J., Bailey, E., et al. (2013). Genetic Diversity in the Modern Horse Illustrated from Genome-wide SNP Data. PloS one 8, e54997. doi:10.1371/journal.pone.0054997
Raudsepp, T., Finno, C. J., Bellone, R. R., and Petersen, J. L. (2019). Ten Years of the Horse Reference Genome: Insights into Equine Biology, Domestication and Population Dynamics in the post‐genome Era. Anim. Genet. 50, 569–597. doi:10.1111/age.12857
Rebolledo-Mendez, J., Hestand, M. S., Coleman, S. J., Zeng, Z., Orlando, L., MacLeod, J. N., et al. (2015). Comparison of the Equine Reference Sequence with its Sanger Source Data and New Illumina Reads. PLoS One 10, e0126852. doi:10.1371/journal.pone.0126852
Rooney, M. F., Hill, E. W., Kelly, V. P., and Porter, R. K. (2018). The “Speed Gene” Effect of Myostatin Arises in Thoroughbred Horses Due to a Promoter Proximal SINE Insertion. PLoS ONE 13, e0205664. doi:10.1371/journal.pone.0205664
Schaefer, R. J., Schubert, M., Bailey, E., Bannasch, D. L., Barrey, E., Bar-Gal, G. K., et al. (2017). Developing a 670k Genotyping Array to Tag ∼2M SNPs across 24 Horse Breeds. BMC Genomics 18, 565. doi:10.1186/s12864-017-3943-8
Schneider, V. A., Graves-Lindsay, T., Howe, K., Bouk, N., Chen, H.-C., Kitts, P. A., et al. (2017). Evaluation of GRCh38 and De Novo Haploid Genome Assemblies Demonstrates the Enduring Quality of the Reference Assembly. Genome Res. 27, 849–864. doi:10.1101/gr.213611.116
Schultz, N. (2016). Characterization of Equine Metabolic Syndrome and Mapping of Candidate Genetic Loci .
Smedley, D., Schubach, M., Jacobsen, J. O. B., Köhler, S., Zemojtel, T., Spielmann, M., et al. (2016). A Whole-Genome Analysis Framework for Effective Identification of Pathogenic Regulatory Variants in Mendelian Disease. Am. J. Hum. Genet. 99, 595–606. doi:10.1016/j.ajhg.2016.07.005
Sudmant, P. H., Rausch, T., Gardner, E. J., Handsaker, R. E., Abyzov, A., Huddleston, J., et al. (2015). An Integrated Map of Structural Variation in 2,504 Human Genomes. Nature 526, 75–81. doi:10.1038/nature15394
Tenesa, A., Navarro, P., Hayes, B. J., Duffy, D. L., Clarke, G. M., Goddard, M. E., et al. (2007). Recent Human Effective Population Size Estimated from Linkage Disequilibrium. Genome Res. 17, 520–526. doi:10.1101/gr.6023607
Wade, C. M., Giulotto, E., Sigurdsson, S., Zoli, M., Gnerre, S., Imsland, F., et al. (2009). Genome Sequence, Comparative Analysis, and Population Genetics of the Domestic Horse. Science 326, 865–867. doi:10.1126/science.1178158
Wang, J., Raskin, L., Samuels, D. C., Shyr, Y., and Guo, Y. (2015). Genome Measures Used for Quality Control Are Dependent on Gene Function and Ancestry. Bioinformatics 31, 318–323. doi:10.1093/bioinformatics/btu668
Ward, T., Valberg, S., Adelson, D., Abbey, C., Binns, M., and Mickelson, J. (2004). Glycogen Branching Enzyme (GBE1) Mutation Causing Equine Glycogen Storage Disease IV. Mamm. Genome 15, 570–577. doi:10.1007/s00335-004-2369-1
Yang, Y., Muzny, D. M., Xia, F., Niu, Z., Person, R., Ding, Y., et al. (2014). Molecular Findings Among Patients Referred for Clinical Whole-Exome Sequencing. Jama 312, 1870. doi:10.1001/jama.2014.14601
Yngvadottir, B., Xue, Y., Searle, S., Hunt, S., Delgado, M., Morrison, J., et al. (2009). A Genome-wide Survey of the Prevalence and Evolutionary Forces Acting on Human Nonsense SNPs. Am. J. Hum. Genet. 84, 224–234. doi:10.1016/j.ajhg.2009.01.008
Keywords: genetic variation, whole genome sequence, variant discovery, equine, breed differences, genetics
Citation: Durward-Akhurst SA, Schaefer RJ, Grantham B, Carey WK, Mickelson JR and McCue ME (2021) Genetic Variation and the Distribution of Variant Types in the Horse. Front. Genet. 12:758366. doi: 10.3389/fgene.2021.758366
Received: 16 August 2021; Accepted: 10 November 2021; Published: 02 December 2021.
Reviewed by:
Copyright © 2021 Durward-Akhurst, Schaefer, Grantham, Carey, Mickelson and McCue. This is an open-access article distributed under the terms of the Creative Commons Attribution License (CC BY). The use, distribution or reproduction in other forums is permitted, provided the original author(s) and the copyright owner(s) are credited and that the original publication in this journal is cited, in accordance with accepted academic practice. No use, distribution or reproduction is permitted which does not comply with these terms.
*Correspondence: S. A. Durward-Akhurst, [email protected]
Disclaimer: All claims expressed in this article are solely those of the authors and do not necessarily represent those of their affiliated organizations, or those of the publisher, the editors and the reviewers. Any product that may be evaluated in this article or claim that may be made by its manufacturer is not guaranteed or endorsed by the publisher.
March 28, 2024
Kombucha Tea-associated microbes remodel host metabolic pathways to suppress lipid accumulation
Image credit: pgen.1011003
Research Article
Genomic analyses of Symbiomonas scintillans show no evidence for endosymbiotic bacteria but does reveal the presence of giant viruses
A multi-gene tree showed the three SsV genome types branched within highly supported clades with each of BpV2, OlVs, and MpVs, respectively.
Image credit: pgen.1011218
Recently Published Articles
- Bacillus subtilis ">Two distinct regulatory systems control pulcherrimin biosynthesis in Bacillus subtilis
- iGWAS: Image-based genome-wide association of self-supervised deep phenotyping of retina fundus images
- Modelling daisy quorum drive: A short-term bridge across engineered fitness valleys
Current Issue April 2024
A natural bacterial pathogen of C . elegans uses a small RNA to induce transgenerational inheritance of learned avoidance
A mechanism of learning and remembering pathogen avoidance likely happens in the wild.
Image credit: pgen.1011178
Spoink , a LTR retrotransposon, invaded D. melanogaster populations in the 1990s
Evidence of Spoink retrotransposon's horizontal transfer into D. melanogaster populations post-1993, suggesting its origin from D.willistoni .
Image credit: pgen.1011201
Comparison of clinical geneticist and computer visual attention in assessing genetic conditions
Understanding AI, specifically Deep Learning, in facial diagnostics for genetic conditions can enhance the design and utilization of AI tools, facilitating more meaningful interactions between clinicians and AI technologies.
Image credit: pgen.1011168
Maintenance of proteostasis by Drosophila Rer1 is essential for competitive cell survival and Myc-driven overgrowth
Loss of Rer1 induces proteotoxic stress, leading to cell competition and elimination, while increased Rer1 levels provide cytoprotection and support Myc-driven overgrowth.
Image credit: pgen.1011171
Anthracyclines induce cardiotoxicity through a shared gene expression response signature
TOP2i induce thousands of shared gene expression changes in cardiomyocytes.
Image credit: pgen.1011164
CryptoCEN: A Co-Expression Network for Cryptococcus neoformans reveals novel proteins involved in DNA damage repair
Co-expression analysis of CryptoCEN network identifys 13 new DNA damage response genes.
Image credit: pgen.1011158
TRPS1 modulates chromatin accessibility to regulate estrogen receptor alpha (ER) binding and ER target gene expression in luminal breast cancer cells
TRPS1 orchestrates gene expression, estrogen signaling, and chromatin dynamics …
Image credit: pgen.1011159
IntroUNET: Identifying introgressed alleles via semantic segmentation
Deep learning algorithm accurately identifies introgressed alleles at the individual level, unveiling insights into the extent and fitness effects of introgression.
Image credit: pgen.1010657
New PLOS journals accepting submissions
Five new journals unified in addressing global health and environmental challenges are now ready to receive submissions: PLOS Climate , PLOS Sustainability and Transformation , PLOS Water , PLOS Digital Health , and PLOS Global Public Health
COVID-19 Collection
The COVID-19 Collection highlights all content published across the PLOS journals relating to the COVID-19 pandemic.
Submit your Lab and Study Protocols to PLOS ONE !
PLOS ONE is now accepting submissions of Lab Protocols, a peer-reviewed article collaboration with protocols.io, and Study Protocols, an article that credits the work done prior to producing and publishing results.
PLOS Reviewer Center
A collection of free training and resources for peer reviewers of PLOS journals—and for the peer review community more broadly—drawn from research and interviews with staff editors, editorial board members, and experienced reviewers.
Ten Simple Rules
PLOS Computational Biology 's "Ten Simple Rules" articles provide quick, concentrated guides for mastering some of the professional challenges research scientists face in their careers.
Welcome New Associate Editors!
PLOS Genetics welcomes several new Associate Editors to our board: Nicolas Bierne, Julie Simpson, Yun Li, Hongbin Ji, Hongbing Zhang, Bertrand Servin, & Benjamin Schwessinger
Expanding human variation at PLOS Genetics
The former Natural Variation section at PLOS Genetics relaunches as Human Genetic Variation and Disease. Read the editors' reasoning behind this change.
PLOS Genetics welcomes new Section Editors
Quanjiang Ji (ShanghaiTech University) joined the editorial board and Xiaofeng Zhu (Case Western Reserve University) was promoted as new Section Editors for the PLOS Genetics Methods section.
PLOS Genetics editors elected to National Academy of Sciences
Congratulations to Associate Editor Michael Lichten and Consulting Editor Nicole King, who are newly elected members of the National Academy of Sciences.
Harmit Malik receives Novitski Prize
Congratulations to Associate Editor Harmit Malik, who was awarded the Edward Novitski Prize by the Genetics Society of America for his work on genetic conflict. Harmit has also been elected as a new member of the American Academy of Arts & Sciences.
Publish with PLOS
- Submission Instructions
- Submit Your Manuscript
Connect with Us
- PLOS Genetics on Twitter
- PLOS on Facebook
Get new content from PLOS Genetics in your inbox
Thank you you have successfully subscribed to the plos genetics newsletter., sorry, an error occurred while sending your subscription. please try again later..
Population genetics: past, present, and future
- Published: 18 July 2020
- Volume 140 , pages 231–240, ( 2021 )
Cite this article
- Atsuko Okazaki 1 , 2 ,
- Satoru Yamazaki 3 ,
- Ituro Inoue 4 &
- Jurg Ott ORCID: orcid.org/0000-0002-6188-1388 2
6 Citations
6 Altmetric
Explore all metrics
We present selected topics of population genetics and molecular phylogeny. As several excellent review articles have been published and generally focus on European and American scientists, here, we emphasize contributions by Japanese researchers. Our review may also be seen as a belated 50-year celebration of Motoo Kimura’s early seminal paper on the molecular clock, published in 1968.
Similar content being viewed by others
Computational Tools for Population Genomics
Molecular Evolution: A Brief Introduction
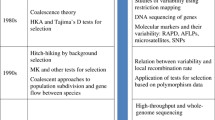
Population genetics from 1966 to 2016
Avoid common mistakes on your manuscript.
Introduction
In recent years, large amounts of DNA sequencing data have been generated in various projects such as 1000 Genomes (Genomes Project et al. 2010 , 2012 , 2015 ), the ALSPAC database (Fraser et al. 2013 ; Hameed et al. 2017 ), and Icelandic (Gudbjartsson et al. 2015 ), and Japanese populations (Nagasaki et al. 2015 ). Major achievements of these efforts have been as follows: (1) Larger genetic variation is observed within populations than between populations, and (2) each individual harbors large numbers of variants with low allele frequencies. These findings have long ago been predicted by population genetics and evolutionary studies. Therefore, it is instructive to look back at historic achievements in population genetics.
Excellent reviews of population genetics have been written (Chakraborty 2006 ; Charlesworth and Charlesworth 2017 ; Crow 1987 ; Crow and Kimura 1970 ) documenting the development of population genetics from early achievements by Mendel ( 1866 ), Hardy ( 1908 ), and Weinberg ( 1908 ) up to highly sophisticated theoretical developments, mostly by American, British, and Japanese scientists. Here, we review selected aspects of population genetics, genome evolution, and molecular phylogeny with an emphasis on contributions by Japanese researchers.
Historical aspects of population genetics and road to the neutral theory
Darwin’s theory of evolution through selection very well explains changes in time of heritable phenotypes. In the early 1900s, focusing on the evolution of genetic variants in the population, R. A. Fisher, S. Wright, and J. B. S. Haldane made fundamental theoretical contributions to population genetics (Provine 1971 ), Fisher in his 1922 paper (Fisher 1922 ), which was the first to introduce diffusion equations into population genetics, and Haldane in developing in 1927 (Haldane 1927 ) the approximation of change of numbers of copies of very rare mutants by branching processes. Wright ( 1938 ) developed the theory on the effects of genetic drift, that is, random changes in small populations. While his theory was supported only by a minority of scientists in an era when the molecular basis of genes had yet to be proven and the effects of genetic drift were underestimated, Wright’s theory made a great contribution to connecting Mendelian Genetics with the Darwinian theory of evolution.
More recently, it has become apparent that many molecular changes have no effects on phenotypes. Based on Wright’s drift hypothesis and Haldane’s approximation model of an advantageous mutation (Haldane 1927 ), Motoo Kimura ( 1964 ) then developed his neutral theory based on backward diffusion models, which showed the probability of fixation to zero of a variant in the population to be equal to 2 s ( N e / N ), where s is the selection coefficient, N the size of the breeding population, and N e the effective population size.
Mutations and selection are driving forces for evolution. Basically, mutations occur at random DNA bases. Harmful mutations tend to be eliminated within a short period of time and do not contribute to long-term evolution. This process is called negative or purifying selection as opposed to positive selection. Before Kimura ( 1964 ) proposed his neutral theory, there was little notion of neutral variation, although, at about the same time, Lewontin and Hubby ( 1966 ) considered the possibility of neutral mutation as a possible reason for a large amount of variation which they found in electrophoretic mobility. Still, natural selection was the mainstream hypothesis with the idea that advantageous variations in populations are the driving forces for evolution, and deleterious variations are removed in a rapid manner.
At the time, population genetics usually considered two alleles at each gene locus based on the assumption of genes being base pairs. On the other hand, Kimura and Crow ( 1964 ) assumed an infinite allele model (“neutral isoalleles”) and proposed that genetic variation in populations arises as to the balance between mutations and genetic drift. Comparing hemoglobin molecules between different organisms, Kimura ( 1968 ) postulated that amino-acid substitution rates are so high that they can only be explained by neutral mutations. In other words, mutation and random changes in a finite population can maintain considerable variation through random fixation of selectively neutral or nearly neutral mutants. In the light of current knowledge, however, Kimura’s reasoning appears somewhat flawed. For example, he argued that the “cost of natural selection” would be too high otherwise—more consideration has shown that no cost is imposed by beneficial mutations in the absence of environmental deterioration. He also used the total amount of DNA without distinguishing protein-coding regions and non-coding regions. Nonetheless, Kimura’s contributions to population genetics have been tremendous.
Together with the Darwinian selection hypothesis, the neutral theory is one of the two pillars of genome evolution. Thus, ‘survival of the luckiest, and not necessarily of the fittest’ may be a good explanation for the evolution of a great majority of genetic changes (Chakraborty 2006 ). Interestingly, Kimura ( 1969 ) also proposed the “infinite sites model”. In this model, if the mutation rate is low and the effective population size is small ( θ = 4 N e µ « 1), a mutant variant will always appear at a different site in the genome. If so, identity by state at the variant can be regarded as identity by descent, and in this respect, the infinite sites model represents one of the bases for genome-wide association studies using SNPs as genetic markers in unrelated individuals (Sella and Barton 2019 ).
The nearly neutral theory
The evolutionary rate, λ = fμ , in the neutral theory ( f is the proportion of neutral mutations among all mutations in a gene, μ is the mutation rate) disregards mutations favorable to survival and simply classifies other mutations into neutral ( f ) and deleterious (1 − f ) mutations. However, the extent of harmfulness measured by the selection coefficient, s , is a continuous quantity. Based on these ideas, Tomoko Ohta (Ohta 1973 , 1992 , 2002 ), who had built the foundation of the neutral theory with Motoo Kimura, proposed the “nearly neutral” theory, where slightly disadvantageous mutations (attenuated mutations) could persist in the population by chance if the population is small. Thus, according to her publications (Ohta 1973 , 1992 , 2002 ), a substantial fraction of changes is caused by random fixation of nearly neutral changes, a class that includes intermediates between neutral and advantageous, as well as between neutral and deleterious classes, although other population geneticists may disagree with this view (Kondrashov 1995 ; Nei 2005 ).
A difference from the neutral theory is that the nearly neutral theory allows for interactions between (1) genes having occurred through weak natural selection (or weak deleterious selection) and (2) genes without weak natural selections, and for the two types of genes to jointly contribute to evolution by opposing the action of genetic drift (Hurst 2009 ). In the nearly neutral theory, the effect of genetic drift is weakened, and slightly disadvantageous mutations are excluded from a population if the population is extremely large; if a population is small, then slightly disadvantageous mutations are kept (some are even fixed) by the effects of genetic drift. It seems that the structure of very large datasets such as 1000 Genomes or the Exome Sequencing Project 6500 can be explained by the nearly neutral theory, because there is increasing evidence that selection pressure in small populations such as mammals including humans is weaker compared to that in ancestral species, and slightly disadvantageous mutations have been accumulating in populations (Kosiol et al. 2008 ; Nelson et al. 2012 ; Nielsen et al. 2009 ; Tennessen et al. 2012 ).
Evolutionary rate of pseudogenes
In the second half of 1970, accumulated sequencing data confirmed the prediction by King and Jukes ( 1969 ) that mutation rates of synonymous variants are higher than those of non-synonymous variants, which supports the neutral theory. Kimura ( 1977 ) asserted that according to the neutral mutation-random drift hypothesis, most mutant substitutions detected among organisms should be the results of random fixation of selectively neutral or nearly neutral mutations. This conjecture was verified by the analysis of mutation rates of pseudogenes, that is, of genes with sequences similar to normal genes having lost their functions as they were duplicated to another location in the genome, and in the process, their transcription sequences were not preserved. Based on the neutral theory, Takashi Miyata calculated the replacement rates of non-synonymous variants and synonymous variants in nucleotide sequences of several pseudogenes, α and β globin, and compared them with those in their functional counterparts (Miyata and Hayashida 1981 ). Results showed that replacement rates were uniformly the same in different pseudogenes and almost equal to the mutation rate, with no other gene evolving at a faster rate. This observation clearly supported the neutral theory.
Junk DNA, a term publicized by Susumu Ohno ( 1972 ) but rarely used today (see below), contains inter-genic regions, most of which are SINEs ( S hort IN terspersed E lements) and LINEs ( L ong IN terspersed E lements). The term ‘junk DNA’ was mentioned by a few other authors in 1972 and even 9 years earlier in a paper little known to human geneticists (Ehret and De Haller 1963 ), but Ohno’s name tends to be most closely associated with this term.
Evolutionary rates of junk DNA are expected to be similar to those of synonymous mutations and pseudogenes. In mammals, most of the genome regions, likely well more than 90%, are predicted to be junk DNA. Therefore, evolutionary rates of whole genomes can be approximated as being those of junk DNA.
In 2012, the Encyclopedia of DNA elements (ENCODE) project (Consortium 2012 ) proved biochemical functions of 80% of the genome, especially outside of protein-coding regions, which was once considered junk DNA. The findings from the ENCODE project enable us to further explore the function of the human genome.
Genes and genomic duplication
In higher organisms, genomic duplication is known to be extremely important for evolution. Early on, Susumu Ohno proposed that evolution is caused by genomic duplication, which was a visionary idea at a time when large sequencing data were not yet available (Ohno 1970 ). It has been shown empirically and by theoretical considerations that the advantage of creating new copies of genomes (or individual genes) can result in higher fitness. An alternative model explaining genomic duplication is DDC ( D uplication D egeneration C omplementation) (Lynch and Conery 2000 ). In the DDC model, regulatory elements each controlling independent functions are duplicated and random null mutations in the regulatory elements through degeneration lead to sub-functionalization, where the regulatory elements complement each other to achieve the full ancestral repertoires. What is important in the process is that it does not require the help of positive selection, that is, functional diversification. In practice, it has been proposed that the selection of slightly disadvantageous mutations works with the expression level of each gene changing. Therefore, genetic duplication is predicted to proceed in a nearly neutral manner based on mutation pressure and genetic drift. In addition, “concerted evolution” in minisatellites used as markers for hyper-polymorphisms, and in other sequences such as rRNA genes can be explained well by Ohno’s theory (Hillis et al. 1991 ; Jeffreys et al. 1985 ).
Molecular phylogeny
Through evolution, currently, living organisms have descended from common ancestors. Systematic biology seeks to unravel relationships among organisms and to establish evolutionary trees. As every biology student knows, the classical approach to such discoveries is through painstaking analysis of morphological details. Depending on which of these phenotypes are considered most important, different relationships among organisms emerge.
Rather than relying on phenotypes that may or may not be heritable, molecular phylogeny relies on DNA sequences and their comparisons among organisms. Researchers with various backgrounds have made significant contributions to methods of creating phylogenetic trees and the evaluation of phylogenetic relationships. In this field, Joseph Felsenstein almost single-handedly established this field as a special branch of population genetics (Felsenstein 2004 ). For example, he introduced the maximum-likelihood method of establishing phylogenetic trees (Felsenstein 1978 ) (see below). One of his other contributions is the “Felsenstein Zone” (Huelsenbeck and Hillis 1993 ), which involves the phenomenon of “long-branch attraction”; that is, long branches will appear similar to each other and appear as sister taxa on a tree even though they do not share a common ancestry. The Zone is the set of trees on which long-branch attraction occurs. Such phenomena have been observed in many datasets and simulation analyses, and have led to the discovery of long-branch attraction, which leads to wrongly assuming phylogeny where none exists (Huelsenbeck and Hillis 1993 ). Furthermore, Felsenstein contributed greatly to molecular phylogeny by developing a program package, PHYLIP, combining various phylogenic tree estimation methods including DNAML. Thanks to his contributions, molecular phylogeny has become increasingly popular for empirical molecular evolutionists.
The development of molecular phylogeny may not seem to be related to disease gene discovery. However, it greatly contributes to such discoveries through interpretation of huge sequencing datasets obtained from the 1000 Genomes project and other projects. Generating a molecular phylogenetic tree for phylogenetic relationships between species led to the discovery of gene families (orthologs and paralogs). The coalescent theory, which examines the gene tree in a species by reversing the time, was also applied to reconstruct the demographic history of species of interest. In particular, regarding the coalescent theory, Tajima ( 1983 ) estimated nucleotide diversity based on the limited DNA polymorphic data, calculated the time of coalescence of genes sampled from a single population, and their theory applies to a few genes at the time of population splitting. Takahata and Nei ( 1985 ) further developed a coalescent theory from DNA sequencing data and theoretically showed that alleles with deep coalescences are relatively rare.
The neighbor-joining method
Many methods for creating (estimating) phylogenic trees have been developed. Historically, these methods can roughly be classified into two groups, distance matrix methods and character state methods. The former uses a distance matrix and estimates evolutionary distance such as the number of amino-acid substitutions or base substitutions based on all possible pairs of OTUs (Operational Taxonomic Units). This method was first applied to create phylogenic trees in the form of the UPGMA (Unweighted Pair Group Method with Arithmetic mean) method, where clusters of neighboring OTUs are created and connected in a stepwise fashion. The method is used not only for amino-acid or base-pair sequences but also in numerical taxonomy, which deals with expression analysis using microarray (Eisen et al. 1998 ) or trait-encoded information (Sokal and Michener 1958 ). However, since this method assumes constant evolutionary speed, it is problematic to apply to amino-acid or base-pair sequence data. To overcome this problem, distance methods were developed that did not assume a molecular clock (Fitch and Margoliash 1967 ). Masatoshi Nei and Naruya Saitou greatly improved upon this method and developed a much faster procedure (Saitou and Nei 1987 ). This method is one of the “star decomposition” methods that determine which, of a given pair of sequences, reduces length of the total tree most and combine neighboring nodes until all OTUs are included. In the neighbor-joining method, “neighbors” keep track of nodes on a tree rather than taxa or clusters of taxa. A modified distance matrix is obtained in which the separation between each pair of nodes is adjusted on the basis of their average divergence from all other nodes. The tree is constructed by joining the least-distant pair of nodes in this modified matrix. When two nodes are joined, their common ancestral node is added to the tree and the terminal nodes with their respective branches are removed from the tree. At each stage in the process, two terminal nodes are replaced by one new node. This iterative operation finds “neighbors” one after another, which creates the final phylogenetic tree. The neighbor-joining method is the most commonly used distance matrix method. Starting in 1971, Nei proposed that Nei’s distance be used for phylogenetic tree estimation, which was later incorporated into the neighbor-joining program package MEGA (Kumar et al. 1994 ; Saitou and Nei 1987 ).
The second group, character state methods, do not use a distance matrix and define characters (phenotypes) and use them for exploring tree topology. One of the examples of character state methods is the maximum-likelihood method discussed in the next section.
The maximum-likelihood method
Maximum likelihood (ML) was developed by Fisher ( 1922 ) as a method to estimate parameters in statistical models. It has several advantages over other methods, but tends to be more complicated to apply than simpler methods. In population genetics, Luigi Luca Cavalli-Sforza first applied the ML method to an approach for creating phylogenic trees based on allele frequencies (Cavalli-Sforza and Edwards 1967 ). The first use of maximum-likelihood inference of trees from molecular sequences was by Jerzy Neyman (Felsenstein 2001 ; Neyman 1971 ). Felsenstein proposed ML for creating phylogenic trees based on allele frequencies as continuous quantities (Felsenstein 1973a ), thus improving on the method previously proposed by Cavalli-Sforza, and introduced ML for estimating trees based on discrete datasets and the maximum parsimony criterion (Felsenstein 1973b ). Masami Hasegawa incorporated this approach into the MOLPHY program package and pioneered in the use of model selection methods such as AIC in comparing phylogenies (he was a member of Akaike’s institute) (Adachi and Hasegawa 1992 , 1996 ).
The ML method is the most efficient approach among all tree construction methods. For example, false-positive evidence of relationships of long branches (“long-branch attraction”) will not occur when trees are estimated by ML and the model of evolution is correct, although it can occur when the model is not correct. However, the ML method tends to be time-consuming and, for some large trees, may be impossible to apply.
Impact of variants on multifactorial disorders and missing heritability
Based on the material mentioned so far, we will now cover some topics on how progress in population genetics, genome evolution, and phylogenic studies can be applied to medical research.
Multifactorial disorders are assumed to occur through interactions between multiple genetic and environmental factors. Therefore, identifying disease susceptibility genes has been considered difficult, and detecting interactions with environmental factors even more so. Especially in the 1990s, such considerations were widespread, quite in contrast to the relative ease with which increased numbers of gene identifications for monogenic disorders have been achieved. However, there was a researcher to struggle with the solution for genetic causes of multifactorial disorders at that time. Ituro Inoue succeeded in narrowing down disease loci using linkage analysis with affected sib-pairs and constructing haplotypes of the angiotensinogen (AGT) gene using limited data (Inoue et al. 1997 ). Inoue assessed linkage disequilibrium (LD) at each site in the AGT gene and further demonstrated by in vitro functional assay that the combination between A (− 6) and T235 alleles affects the expression of the AGT gene. This study was visionary, since LD block structures had yet to be proved at that time.
After that, genome-wide association studies with large SNP data over the whole genome became available thanks to the HAPMAP project, SNP collections by Perlegen Science, LD block measurements, and construction of haplotype maps (HapMap 2005 ; Hinds et al. 2005 ). Although such genome-wide studies contributed to narrowing down locations of disease susceptibility genes, results are still insufficient for identifying many specific disease susceptibility genes, for example Moyamoya disease (Liu et al. 2011 ). A remaining challenge has been that identified susceptibility loci show only small odds ratios, and all susceptibility loci combined only explain up to 30% of most of the disease causes. These numbers are generally smaller than the heritability calculated in the previous twin studies, which is known as “missing heritability” (Manolio et al. 2009 ). Nowadays, however, methods for calculating SNP-based heritability have been developed (Yang et al. 2017 ) that come up with heritability estimates close to those obtained by classical segregation analysis, and part of the problem seems to be resolved.
Out-of-Africa hypothesis
Recent advances in sequencing technology have enabled the identification of whole genome structures at population levels. These successes have made it possible to compare current human genome sequences with ancient genomes such as Homo neanderthalensis or Denisova hominin , which greatly contributed to the understanding of the origin of Homo sapiens (Nielsen et al. 2017 ). Allan Wilson, along with Rebecca Cann and Mark Stoneking, first proposed the “out-of-Africa” hypothesis (Cann et al. 1987 ), which claims that Homo sapiens originated in Africa and then spread all over the world. They based their results on the analysis of mitochondrial DNA of various populations, which represented the first phylogenic tree of Homo sapiens . Work by Masatoshi Nei contributed to the out-of-Africa hypothesis: In the 1970s, Nei calculated heterozygosity for various protein isozymes and created phylogenic trees of Homo sapiens (Nei and Roychoudhury 1972 , 1974 ; Nielsen et al. 2017 ). An interesting finding based on this work is that genetic variation estimated by Nei’s distance or Wright’s F st is larger within populations than between populations (Lewontin 1972 ), which was later confirmed by the 1000 Genomes project. In other words, there are greater differences among individuals in a given population than between populations. However, this notion has also been challenged (Edwards 2003 ).
Relationship between recent explosive population growth and origin of deleterious variants
Numerous human genome sequence projects such as 1000 Genomes revealed that each individual harbors considerable numbers of private mutations. This fact had been proposed by Haldane in his “genetic load” theory, which predicted an association between the numbers of variants possessed over populations and survival rate (Haldane 1937 ). In his theory, he claimed that if we consider genetic load for the whole genome rather than a given locus, the fitness decrease by mutations is equal to the mutation rate, v , irrespective of the extent of selection. He also claimed that pathogenic mutations accumulate in the form of heterozygous variants unless such mutations are excluded as lethal homozygous mutations (Haldane 1937 ) (this theory is also known as the Haldane–Muller principle). The theory of genetic load was further elaborated upon by Kimura ( 1960 ); for neutral mutations, there is no load. Based on this background, for variants whose distributions differ among populations, estimating the age of each variant becomes possible, which is important for understanding the history of human evolution, as well as for developing novel methods for disease gene discovery. The mathematical theory of coalescence allowing haplotype and allele ages to be calculated was developed by John Kingman ( 2000 ), and Kimura and Ohta ( 1973 ) proposed a formula for determining allele age, − 2 x (1 − x )/log( x ). This formula represents the expected age of a neutral mutation of frequency x in a stationary population based on a diffusion process used in classical population genetics. Although there was a discussion regarding the restrictive assumption that the age distribution of a mutant allele with population frequency x should be the same as the distribution of the time to extinction of the allele, conditional on extinction, it made a great contribution to later calculations of allele age (Fu et al. 2013 ). Calculating allele age assuming the infinite many sites of model of mutation developed Kimura and Ohta formula, it showed that about three-quarters of all protein-coding SNV predicted to be deleterious across in the past 5000 years (Fu et al. 2013 ). This attempt provides important practical information that can be prioritized variants in disease gene discovery.
Inbreeding (mating between relatives) has so far not been discussed here as it does not lead to changes in allele frequencies. It does, however, lead to a decrease in heterozygotes and a corresponding increase in homozygotes. As is well known, at a bi-allelic locus with allele frequency p , the proportion of heterozygotes is given by 2 p (1 − p )(1 − F ), where F is the inbreeding coefficient. In many human populations, F tends to be rather small; for example, F = 0.00038 in the UK (Pattison 2016 ). An exception is offspring of first cousins ( F = 1/16). For rare deleterious recessive traits with disease allele frequency p , recessive offspring of first-cousin marriages occur with probability p 2 + p (1 − p ) F (Haldane and Moshinsky 1939 ). Through genetic linkage of such a trait with SNPs surrounding it, rare recessive traits tend to be located in long runs of homozygous SNPs (homozygosity mapping (Lander and Botstein 1987 )). More modern approaches have been developed, for example, based on the Hamming distance between chromosomes in affected and control individuals (Imai et al. 2015 ). This approach revealed a mutation, p.H96R in the BOLA3 gene, possibly having originated in a single Japanese founder individual (Imai et al. 2016 ).
Darwinian (evolutionary) medicine
From the viewpoint of Darwinian medicine (or evolutionary medicine), which is medicine based on evolution (Williams and Nesse 1991 ), we discuss a few aspects of how discovering variants can translate into medical care.
In the 1960s, Richard Lewontin discovered in Drosophila populations that heterozygosity is more often observed than expected (Lewontin and Hubby 1966 ). He interpreted this finding as advantageous fitness of heterozygosity compared to the homozygous state of the wild type or mutant (so-called over-dominance, or balancing selection) and emphasized its importance for survival. After the establishment of the neutral theory, as described below, the importance of balancing selection for some types of variants with high allele frequencies was rediscovered. Theoretical studies on natural selection also greatly progressed and “Tajima’s D”, developed by Fumio Tajima, is computed as the difference between two measures of genetic diversity: the mean number of pairwise differences and the number of segregating sites, each scaled so that they are expected to be the same in a neutrally evolving population of constant size. This is a unique contribution to statistical genetics by Japanese researchers in that this method can assess whether a given variant scattered over the whole genome is neutral or under selection pressure (Tajima 1989 ).
Analyzing genome sequences in several populations using the techniques of next-generation sequencing reveals some signals with positive selection pressure. One such example is infection-related diseases. Regarding the natural selection for resistance of a pathogen, this was revealed by next-generation sequencing to represent the strongest positive selection pressure in human evolution; that is, the well-known balancing signals on glycoproteins and positive selection signals on TLRs (Ferrer-Admetlla et al. 2008 ). Applying the history of evolution for various pathogens to disease susceptibility research will likely identify functional variants as well as intra-cellular mechanisms and treatment for various diseases. We believe that selection pressure for ancient pathogens will affect not only infectious and auto-immune diseases but also other traits. Recently, the association between life-style diseases and natural selection has become an attractive topic. Using 40 traits from the UK Biobank, functional low-frequency variants have been revealed to be under negative selection (Gazal et al. 2018 ). An alternative suggestion has been that positive selection acts on susceptibility loci for life-style diseases. An example is the thrifty gene hypothesis. At the dawn of the era of genomic medicine, the ancient history of human evolution is a powerful tool for understanding human biology leading to improving human health.
In this outline, we deliberately emphasized contributions to population genetics by Japanese researchers—in this field, Japanese scientists have arguably carried out comprehensive fundamental work. Thus, we feel justified in presenting this short review of population genetics from a Japanese point of view.
In terms of future developments in population genetics, we expect DNA sequencing to play an ever-increasing role. In an era where human genome sequence projects are underway around the world, established population genetics principles will be applied to reveal more detailed migration history, population history, and mechanisms of selection pressure, particularly in small ethnic populations (Antonio et al. 2019 ; Lipson et al. 2020 ).
Technological advances have changed the landscape of genetic screening (Ceyhan-Birsoy et al. 2019 ). Together with epidemiological and molecular genetics studies, population genetics approaches have demonstrated the association between disease mechanisms and mutations in populations. Cystic fibrosis is one such successful example (Bell et al. 2020 ). By identifying the relationship between specific mutations and a cystic fibrosis transmembrane conductance regulator (CFTR) defect, we can improve patient care including disease monitoring and treatment decisions. In the future, improvement of patient care in more diseases can be achieved by the combination of population genetics, epidemiological studies, and molecular genetics studies.
With the huge amount of genomic information currently available, it is challenging to link genotypes to phenotypes, predict regulatory functions, and classify mutant types. Therefore, new and innovative approaches are needed for further understanding of medical biology and connections to genetic disease. One approach is to collect previously reported SNV information and create a suitable mathematical model. As an example, a study by Davis et al. ( 2016 ) describes a biophysical metric of cardiomyocyte function, which accurately predicts human cardiac phenotypes.
Another approach is based on neural networks to automatically extract relevant features from input data (Zou et al. 2019 ). Since advances in sequencing technologies provide large amounts of data, it is realistic to utilize machine learning as a tool for analysis in the field of clinical healthcare and population genetics. Although deep learning has great potential, attempts to apply it to genomics have only just begun. For example, SpliceAI, a 32-layer deep neural network (DNN) was developed for predicting de novo mutations with predicted splice-altering consequences in patients with neurodevelopmental disorders, which paves the way for the application of deep learning on complex genetic variant prediction (Jaganathan et al. 2019 ). To identify pathogenic mutations in patients with rare diseases, a DNN model was developed combining common variants derived from human and six non-human primate species. The proposed model achieved an 88% accuracy and found 14 unreported candidate genes associated with intellectual disability (Sundaram et al. 2018 ).
Finally, epidemics and pandemics of viruses and their sequences provide rich sources of information. For example, population genetic analyses of 103 SARS-CoV-2 genomes indicated the presence of two major lineages, although the implications of these evolutionary changes remained unclear (Tang et al. 2020 ).
Adachi J, Hasegawa M (1992) MOLPHY, programs for molecular phylogenetics. I, PROTML, maximum likelihood inference of protein phylogeny. Computer science monographs, no. 27. Institute of Statistical Mathematics, Tokyo, pp 1–14
Adachi J, Hasegawa M (1996) MOLPHY version 2.3: Programs for molecular phylogenetics based on maximum likelihood. Computer science monographs, no. 28. Institute of Statistical Mathematics, Tokyo, pp 1–150
Antonio ML, Gao Z, Moots HM, Lucci M, Candilio F, Sawyer S, Oberreiter V, Calderon D, Devitofranceschi K, Aikens RC, Aneli S, Bartoli F, Bedini A, Cheronet O, Cotter DJ, Fernandes DM, Gasperetti G, Grifoni R, Guidi A, La Pastina F, Loreti E, Manacorda D, Matullo G, Morretta S, Nava A, Fiocchi Nicolai V, Nomi F, Pavolini C, Pentiricci M, Pergola P, Piranomonte M, Schmidt R, Spinola G, Sperduti A, Rubini M, Bondioli L, Coppa A, Pinhasi R, Pritchard JK (2019) Ancient Rome: a genetic crossroads of Europe and the Mediterranean. Science 366:708–714. https://doi.org/10.1126/science.aay6826
Article CAS PubMed PubMed Central Google Scholar
Bell SC, Mall MA, Gutierrez H, Macek M, Madge S, Davies JC, Burgel PR, Tullis E, Castanos C, Castellani C, Byrnes CA, Cathcart F, Chotirmall SH, Cosgriff R, Eichler I, Fajac I, Goss CH, Drevinek P, Farrell PM, Gravelle AM, Havermans T, Mayer-Hamblett N, Kashirskaya N, Kerem E, Mathew JL, McKone EF, Naehrlich L, Nasr SZ, Oates GR, O'Neill C, Pypops U, Raraigh KS, Rowe SM, Southern KW, Sivam S, Stephenson AL, Zampoli M, Ratjen F (2020) The future of cystic fibrosis care: a global perspective. Lancet Respir Med 8:65–124. https://doi.org/10.1016/S2213-2600(19)30337-6
Article CAS PubMed Google Scholar
Cann RL, Stoneking M, Wilson AC (1987) Mitochondrial DNA and human evolution. Nature 325:31–36
CAS PubMed Google Scholar
Cavalli-Sforza LL, Edwards AW (1967) Phylogenetic analysis. Models and estimation procedures. Am J Hum Genet 19:233–257
CAS PubMed PubMed Central Google Scholar
Ceyhan-Birsoy O, Murry JB, Machini K, Lebo MS, Yu TW, Fayer S, Genetti CA, Schwartz TS, Agrawal PB, Parad RB, Holm IA, McGuire AL, Green RC, Rehm HL, Beggs AH, BabySeq Project T (2019) Interpretation of Genomic Sequencing Results in Healthy and Ill Newborns: Results from the BabySeq Project. Am J Hum Genet 104:76–93. https://doi.org/10.1016/j.ajhg.2018.11.016
Chakraborty R (2006) Population Genetics: Historical Aspects. eLS. Wiley, Chichester, pp 1–3
Google Scholar
Charlesworth B, Charlesworth D (2017) Population genetics from 1966 to 2016. Heredity (Edinb) 118:2–9. https://doi.org/10.1038/hdy.2016.55
Article CAS Google Scholar
Consortium EP (2012) An integrated encyclopedia of DNA elements in the human genome. Nature 489:57–74. https://doi.org/10.1038/nature11247
Crow JF (1987) Population genetics history: a personal view. Annu Rev Genet 21:1–22. https://doi.org/10.1146/annurev.ge.21.120187.000245
Crow JF, Kimura M (1970) An introduction to population genetics theory. Harper & Row, New York
Davis J, Davis LC, Correll RN, Makarewich CA, Schwanekamp JA, Moussavi-Harami F, Wang D, York AJ, Wu H, Houser SR, Seidman CE, Seidman JG, Regnier M, Metzger JM, Wu JC, Molkentin JD (2016) A tension-based model distinguishes hypertrophic versus dilated cardiomyopathy. Cell 165:1147–1159. https://doi.org/10.1016/j.cell.2016.04.002
Edwards AW (2003) Human genetic diversity: Lewontin's fallacy. BioEssays 25:798–801. https://doi.org/10.1002/bies.10315
Ehret CF, De Haller G (1963) Origin, development and maturation of organelles and organelle systems of the cell surface in Paramecium. J Ultrastruct Res 23:1–42
Eisen MB, Spellman PT, Brown PO, Botstein D (1998) Cluster analysis and display of genome-wide expression patterns. Proc Natl Acad Sci USA 95:14863–14868. https://doi.org/10.1073/pnas.95.25.14863
Felsenstein J (1973a) Maximum-likelihood estimation of evolutionary trees from continuous characters. Am J Hum Genet 25:471–492
Felsenstein J (1973b) Maximum likelihood and minimum-steps methods for estimating evolutionary trees from data on discrete characters. Syst Biol 22:240–249
Felsenstein J (1978) The number of evolutionary trees. Syst Biol 27:27–33. https://doi.org/10.2307/2412810
Article Google Scholar
Felsenstein J (2001) Taking variation of evolutionary rates between sites into account in inferring phylogenies. J Mol Evol 53:447–455. https://doi.org/10.1007/s002390010234
Felsenstein J (2004) Inferring phylogenies. Sinauer Associates, Sunderland
Ferrer-Admetlla A, Bosch E, Sikora M, Marques-Bonet T, Ramirez-Soriano A, Muntasell A, Navarro A, Lazarus R, Calafell F, Bertranpetit J, Casals F (2008) Balancing selection is the main force shaping the evolution of innate immunity genes. J Immunol 181:1315–1322. https://doi.org/10.4049/jimmunol.181.2.1315
Fisher RA (1922) On the mathematical foundations of theoretical statistics. Phil Trans Roy Soc A202:309–368
Fitch WM, Margoliash E (1967) Construction of phylogenetic trees. Science 155:279–284. https://doi.org/10.1126/science.155.3760.279
Fraser A, Macdonald-Wallis C, Tilling K, Boyd A, Golding J, Davey Smith G, Henderson J, Macleod J, Molloy L, Ness A, Ring S, Nelson SM, Lawlor DA (2013) Cohort profile: the avon longitudinal study of parents and children: ALSPAC mothers cohort. Int J Epidemiol 42:97–110. https://doi.org/10.1093/ije/dys066
Article PubMed Google Scholar
Fu W, O'Connor TD, Jun G, Kang HM, Abecasis G, Leal SM, Gabriel S, Rieder MJ, Altshuler D, Shendure J, Nickerson DA, Bamshad MJ, Project NES, Akey JM (2013) Analysis of 6,515 exomes reveals the recent origin of most human protein-coding variants. Nature 493:216–220. https://doi.org/10.1038/nature11690
Gazal S, Loh P-R, Finucane HK, Ganna A, Schoech A, Sunyaev S, Price AL (2018) Functional architecture of low-frequency variants highlights strength of negative selection across coding and non-coding annotations. Nat Genet 50:1600–1607
Genomes Project C, Abecasis GR, Altshuler D, Auton A, Brooks LD, Durbin RM, Gibbs RA, Hurles ME, McVean GA (2010) A map of human genome variation from population-scale sequencing. Nature 467:1061–1073
Genomes Project C, Abecasis GR, Auton A, Brooks LD, DePristo MA, Durbin RM, Handsaker RE, Kang HM, Marth GT, McVean GA (2012) An integrated map of genetic variation from 1,092 human genomes. Nature 491:56–65
Genomes Project C, Auton A, Brooks LD, Durbin RM, Garrison EP, Kang HM, Korbel JO, Marchini JL, McCarthy S, McVean GA, Abecasis GR (2015) A global reference for human genetic variation. Nature 526:68–74. https://doi.org/10.1038/nature15393
Gudbjartsson DF, Helgason H, Gudjonsson SA, Zink F, Oddson A, Gylfason A, Besenbacher S, Magnusson G, Halldorsson BV, Hjartarson E, Sigurdsson GT, Stacey SN, Frigge ML, Holm H, Saemundsdottir J, Helgadottir HT, Johannsdottir H, Sigfusson G, Thorgeirsson G, Sverrisson JT, Gretarsdottir S, Walters GB, Rafnar T, Thjodleifsson B, Bjornsson ES, Olafsson S, Thorarinsdottir H, Steingrimsdottir T, Gudmundsdottir TS, Theodors A, Jonasson JG, Sigurdsson A, Bjornsdottir G, Jonsson JJ, Thorarensen O, Ludvigsson P, Gudbjartsson H, Eyjolfsson GI, Sigurdardottir O, Olafsson I, Arnar DO, Magnusson OT, Kong A, Masson G, Thorsteinsdottir U, Helgason A, Sulem P, Stefansson K (2015) Large-scale whole-genome sequencing of the Icelandic population. Nat Genet 47:435–444. https://doi.org/10.1038/ng.3247
Haldane J (1927) A mathematical theory of natural and artificial selection, Part V: selection and mutation. Math Proc Cambridge Philos Soc 23:838–844. https://doi.org/10.1017/S0305004100015644
Haldane JBS (1937) The effect of variation on fitness. Am Nat 71:337–349
Haldane JBS, Moshinsky P (1939) Inbreeding in mendelian populations with special reference to human cousin marriage. Ann Eugen 9:321–340
Hameed MA, Lingam R, Zammit S, Salvi G, Sullivan S, Lewis AJ (2017) Trajectories of early childhood developmental skills and early adolescent psychotic experiences: findings from the ALSPAC UK birth cohort. Front Psychol 8:2314. https://doi.org/10.3389/fpsyg.2017.02314
HapMap (2005) A haplotype map of the human genome. Nature 437:1299–1320
Hardy GH (1908) Mendelian proportions in a mixed population. Science 28:49–50
Hillis DM, Moritz C, Porter CA, Baker RJ (1991) Evidence for biased gene conversion in concerted evolution of ribosomal DNA. Science 251:308–310. https://doi.org/10.1126/science.1987647
Hinds DA, Stuve LL, Nilsen GB, Halperin E, Eskin E, Ballinger DG, Frazer KA, Cox DR (2005) Whole-genome patterns of common DNA variation in three human populations. Science 307:1072–1079. https://doi.org/10.1126/science.1105436
Huelsenbeck JP, Hillis DM (1993) Success of phylogenetic methods in the four-taxon case. Syst Biol 42:247–264. https://doi.org/10.1093/sysbio/42.3.247
Hurst LD (2009) Evolutionary genomics and the reach of selection. J Biol 8:12. https://doi.org/10.1186/jbiol113
Imai A, Nakaya A, Fahiminiya S, Tetreault M, Majewski J, Sakata Y, Takashima S, Lathrop M, Ott J (2015) Beyond homozygosity mapping: family-control analysis based on hamming distance for prioritizing variants in exome sequencing. Sci Rep 5:12028. https://doi.org/10.1038/srep12028
Article PubMed PubMed Central Google Scholar
Imai A, Kohda M, Nakaya A, Sakata Y, Murayama K, Ohtake A, Lathrop M, Okazaki Y, Ott J (2016) HDR: a statistical two-step approach successfully identifies disease genes in autosomal recessive families. J Hum Genet 61:959–963. https://doi.org/10.1038/jhg.2016.85
Inoue I, Nakajima T, Williams CS, Quackenbush J, Puryear R, Powers M, Cheng T, Ludwig EH, Sharma AM, Hata A, Jeunemaitre X, Lalouel JM (1997) A nucleotide substitution in the promoter of human angiotensinogen is associated with essential hypertension and affects basal transcription in vitro. J Clin Invest 99:1786–1797. https://doi.org/10.1172/JCI119343
Jaganathan K, Kyriazopoulou Panagiotopoulou S, McRae JF, Darbandi SF, Knowles D, Li YI, Kosmicki JA, Arbelaez J, Cui W, Schwartz GB, Chow ED, Kanterakis E, Gao H, Kia A, Batzoglou S, Sanders SJ, Farh KK (2019) Predicting splicing from primary sequence with deep learning. Cell 176(535–548):e24. https://doi.org/10.1016/j.cell.2018.12.015
Jeffreys AJ, Wilson V, Thein SL (1985) Hypervariable 'minisatellite' regions in human DNA. Nature 314:67–73. https://doi.org/10.1038/314067a0
Kimura M (1960) Optimum mutation rate and degree of dominance as determined by the principle of minimum genetic load. J Genet 57:21–34
Kimura M (1964) Diffusion models in population genetics. J Appl Probab 1:177–232
Kimura M (1968) Evolutionary rate at the molecular level. Nature 217:624–626
Kimura M (1969) The number of heterozygous nucleotide sites maintained in a finite population due to steady flux of mutations. Genetics 61:893–903
Kimura M (1977) Preponderance of synonymous changes as evidence for the neutral theory of molecular evolution. Nature 267:275–276
Kimura M, Crow JF (1964) The number of alleles that can be maintained in a finite population. Genetics 49:725–738
Kimura M, Ohta T (1973) The age of a neutral mutant persisting in a finite population. Genetics 75:199–212
King JL, Jukes TH (1969) Non-Darwinian evolution. Science 164:788–798
Kingman JF (2000) Origins of the coalescent. 1974–1982. Genetics 156:1461–1463
Kondrashov AS (1995) Contamination of the genome by very slightly deleterious mutations: why have we not died 100 times over? J Theor Biol 175:583–594. https://doi.org/10.1006/jtbi.1995.0167
Kosiol C, Vinar T, da Fonseca RR, Hubisz MJ, Bustamante CD, Nielsen R, Siepel A (2008) Patterns of positive selection in six Mammalian genomes. PLoS Genet 4:e1000144. https://doi.org/10.1371/journal.pgen.1000144
Kumar S, Tamura K, Nei M (1994) MEGA: molecular evolutionary genetics analysis software for microcomputers. Comput Appl Biosci 10:189–191
Lander ES, Botstein D (1987) Homozygosity mapping: a way to map human recessive traits with the DNA of inbred children. Science 236:1567–1570
Lewontin RC (1972) The apportionment of human diversity. In: Dobzhansky T, Hecht MK, Steere WC (eds) Evolutionary biology, vol 6. Appleton-Century-Crofts, New York, pp 381–398
Lewontin RC, Hubby JL (1966) A molecular approach to the study of genic heterozygosity in natural populations. II. Amount of variation and degree of heterozygosity in natural populations of Drosophila pseudoobscura. Genetics 54:595–609
Lipson M, Ribot I, Mallick S, Rohland N, Olalde I, Adamski N, Broomandkhoshbacht N, Lawson AM, Lopez S, Oppenheimer J, Stewardson K, Asombang RN, Bocherens H, Bradman N, Culleton BJ, Cornelissen E, Crevecoeur I, de Maret P, Fomine FLM, Lavachery P, Mindzie CM, Orban R, Sawchuk E, Semal P, Thomas MG, Van Neer W, Veeramah KR, Kennett DJ, Patterson N, Hellenthal G, Lalueza-Fox C, MacEachern S, Prendergast ME, Reich D (2020) Ancient West African foragers in the context of African population history. Nature 577:665–670. https://doi.org/10.1038/s41586-020-1929-1
Liu W, Morito D, Takashima S, Mineharu Y, Kobayashi H, Hitomi T, Hashikata H, Matsuura N, Yamazaki S, Toyoda A, Kikuta K, Takagi Y, Harada KH, Fujiyama A, Herzig R, Krischek B, Zou L, Kim JE, Kitakaze M, Miyamoto S, Nagata K, Hashimoto N, Koizumi A (2011) Identification of RNF213 as a susceptibility gene for moyamoya disease and its possible role in vascular development. PLoS ONE 6:e22542. https://doi.org/10.1371/journal.pone.0022542
Lynch M, Conery JS (2000) The evolutionary fate and consequences of duplicate genes. Science 290:1151–1155
Manolio TA, Collins FS, Cox NJ, Goldstein DB, Hindorff LA, Hunter DJ, McCarthy MI, Ramos EM, Cardon LR, Chakravarti A, Cho JH, Guttmacher AE, Kong A, Kruglyak L, Mardis E, Rotimi CN, Slatkin M, Valle D, Whittemore AS, Boehnke M, Clark AG, Eichler EE, Gibson G, Haines JL, Mackay TF, McCarroll SA, Visscher PM (2009) Finding the missing heritability of complex diseases. Nature 461:747–753
Mendel GJ (1866) Versuche über Pflanzen-Hybriden. Verh Naturforsch Ver Brünn 4:3–47
Miyata T, Hayashida H (1981) Extraordinarily high evolutionary rate of pseudogenes: evidence for the presence of selective pressure against changes between synonymous codons. Proc Natl Acad Sci USA 78:5739–5743. https://doi.org/10.1073/pnas.78.9.5739
Nagasaki M, Yasuda J, Katsuoka F, Nariai N, Kojima K, Kawai Y, Yamaguchi-Kabata Y, Yokozawa J, Danjoh I, Saito S, Sato Y, Mimori T, Tsuda K, Saito R, Pan X, Nishikawa S, Ito S, Kuroki Y, Tanabe O, Fuse N, Kuriyama S, Kiyomoto H, Hozawa A, Minegishi N, Douglas Engel J, Kinoshita K, Kure S, Yaegashi N, To MJRPP, Yamamoto M (2015) Rare variant discovery by deep whole-genome sequencing of 1,070 Japanese individuals. Nat Commun 6:8018. https://doi.org/10.1038/ncomms9018
Nei M (2005) Selectionism and neutralism in molecular evolution. Mol Biol Evol 22:2318–2342. https://doi.org/10.1093/molbev/msi242
Nei M, Roychoudhury AK (1972) Gene differences between Caucasian, Negro, and Japanese populations. Science 177:434–436
Nei M, Roychoudhury AK (1974) Genic variation within and between the three major races of man, Caucasoids, Negroids, and Mongoloids. Am J Hum Genet 26:421–443
Nelson MR, Wegmann D, Ehm MG, Kessner D, St Jean P, Verzilli C, Shen J, Tang Z, Bacanu SA, Fraser D, Warren L, Aponte J, Zawistowski M, Liu X, Zhang H, Zhang Y, Li J, Li Y, Li L, Woollard P, Topp S, Hall MD, Nangle K, Wang J, Abecasis G, Cardon LR, Zollner S, Whittaker JC, Chissoe SL, Novembre J, Mooser V (2012) An abundance of rare functional variants in 202 drug target genes sequenced in 14,002 people. Science 337:100–104. https://doi.org/10.1126/science.1217876
Neyman J (1971) Molecular studies of evolution: a source of novel statistical problems. In: Gupta SS, Yackel J (eds) Statistical decision theory and related topics. Academic Press, New York, pp 1–27
Nielsen R, Hubisz MJ, Hellmann I, Torgerson D, Andres AM, Albrechtsen A, Gutenkunst R, Adams MD, Cargill M, Boyko A, Indap A, Bustamante CD, Clark AG (2009) Darwinian and demographic forces affecting human protein coding genes. Genome Res 19:838–849. https://doi.org/10.1101/gr.088336.108
Nielsen R, Akey JM, Jakobsson M, Pritchard JK, Tishkoff S, Willerslev E (2017) Tracing the peopling of the world through genomics. Nature 541:302–310. https://doi.org/10.1038/nature21347
Ohno S (1970) Evolution by gene duplication. Springer, New York
Ohno S (1972) So much "junk" DNA in our genome. Brookhaven Symp Biol 23:366–370
Ohta T (1973) Slightly deleterious mutant substitutions in evolution. Nature 246:96–98
Ohta T (1992) The nearly neutral theory of molecular evolution. Annu Rev Ecol Syst 23:263–286
Ohta T (2002) Near-neutrality in evolution of genes and gene regulation. Proc Natl Acad Sci USA 99:16134–16137. https://doi.org/10.1073/pnas.252626899
Pattison JE (2016) An attempt to integrate previous localized estimates of human inbreeding for the whole of Britain. Hum Biol 88:264–274
PubMed Google Scholar
Provine WB (1971) The origins of theoretical population genetics. University of Chicago Press, Chicago
Saitou N, Nei M (1987) The neighbor-joining method: a new method for reconstructing phylogenetic trees. Mol Biol Evol 4:406–425. https://doi.org/10.1093/oxfordjournals.molbev.a040454
Sella G, Barton NH (2019) Thinking about the evolution of complex traits in the era of genome-wide association studies. Annu Rev Genomics Hum Genet 20:461–493. https://doi.org/10.1146/annurev-genom-083115-022316
Sokal RR, Michener CD (1958) A statistical method for evaluating systematic relationships. Univ Kansas Sci Bull 38:1409–1438
Sundaram L, Gao H, Padigepati SR, McRae JF, Li Y, Kosmicki JA, Fritzilas N, Hakenberg J, Dutta A, Shon J, Xu J, Batzoglou S, Li X, Farh KK (2018) Predicting the clinical impact of human mutation with deep neural networks. Nat Genet 50:1161–1170. https://doi.org/10.1038/s41588-018-0167-z
Tajima F (1983) Evolutionary relationship of DNA sequences in finite populations. Genetics 105:437–460
Tajima F (1989) Statistical method for testing the neutral mutation hypothesis by DNA polymorphism. Genetics 123:585–595
Takahata N, Nei M (1985) Gene genealogy and variance of interpopulational nucleotide differences. Genetics 110:325–344
Tang X, Wu C, Li X, Song Y, Yao X, Wu X, Duan Y, Zhang H, Wang Y, Qian Z, Cui J, Lu J (2020) On the origin and continuing evolution of SARS-CoV-2. Natl Sci Rev 7:1012–1023. https://doi.org/10.1093/nsr/nwaa036
Tennessen JA, Bigham AW, O'Connor TD, Fu W, Kenny EE, Gravel S, McGee S, Do R, Liu X, Jun G, Kang HM, Jordan D, Leal SM, Gabriel S, Rieder MJ, Abecasis G, Altshuler D, Nickerson DA, Boerwinkle E, Sunyaev S, Bustamante CD, Bamshad MJ, Akey JM, Broad GO, Seattle GO, Project NES (2012) Evolution and functional impact of rare coding variation from deep sequencing of human exomes. Science 337:64–69. https://doi.org/10.1126/science.1219240
Weinberg W (1908) Über den Nachweis der Vererbung beim Menschen. Jahreshefte des Vereins für vaterländische Naturkunde in Württemberg 64:369–382
Williams GC, Nesse RM (1991) The dawn of Darwinian medicine. Q Rev Biol 66:1–22. https://doi.org/10.1086/417048
Wright S (1938) Size of population and breeding structure in relation to evolution. Science 87:430–431
Yang J, Zeng J, Goddard ME, Wray NR, Visscher PM (2017) Concepts, estimation and interpretation of SNP-based heritability. Nat Genet 49:1304–1310. https://doi.org/10.1038/ng.3941
Zou J, Huss M, Abid A, Mohammadi P, Torkamani A, Telenti A (2019) A primer on deep learning in genomics. Nat Genet 51:12–18. https://doi.org/10.1038/s41588-018-0295-5
Download references
Acknowledgements
Helpful comments by Prof. Joseph Felsenstein on an earlier version of this manuscript are gratefully acknowledged. This work was supported by the Japan Society for the Promotion of Science (JSPS) KAKENHI, Grant numbers JP20K08497 and JP18K15863 (A. O.), and Grant number 19K09408 (S. Y.).
Author information
Authors and affiliations.
Intractable Disease Research Center, Juntendo University, Tokyo, Japan
Atsuko Okazaki
Laboratory of Statistical Genetics, Rockefeller University, 1230 York Avenue, New York, NY, 10065, USA
Atsuko Okazaki & Jurg Ott
Department of Molecular Pharmacology, National Cerebral and Cardiovascular Center, Osaka, Japan
Satoru Yamazaki
Division of the Human Genetics, National Institute of Genetics, Shizuoka, Japan
Ituro Inoue
You can also search for this author in PubMed Google Scholar
Corresponding author
Correspondence to Jurg Ott .
Ethics declarations
Conflict of interest.
The authors declare no conflict of interest.
Additional information
Publisher's note.
Springer Nature remains neutral with regard to jurisdictional claims in published maps and institutional affiliations.
Rights and permissions
Reprints and permissions
About this article
Okazaki, A., Yamazaki, S., Inoue, I. et al. Population genetics: past, present, and future. Hum Genet 140 , 231–240 (2021). https://doi.org/10.1007/s00439-020-02208-5
Download citation
Received : 12 May 2020
Accepted : 14 July 2020
Published : 18 July 2020
Issue Date : February 2021
DOI : https://doi.org/10.1007/s00439-020-02208-5
Share this article
Anyone you share the following link with will be able to read this content:
Sorry, a shareable link is not currently available for this article.
Provided by the Springer Nature SharedIt content-sharing initiative
- Find a journal
- Publish with us
- Track your research
- Research article
- Open access
- Published: 01 April 2022
The landscape of GWAS validation; systematic review identifying 309 validated non-coding variants across 130 human diseases
- Ammar J. Alsheikh ORCID: orcid.org/0000-0001-7125-0144 1 ,
- Sabrina Wollenhaupt 2 ,
- Emily A. King 1 ,
- Jonas Reeb 2 ,
- Sujana Ghosh 1 ,
- Lindsay R. Stolzenburg 1 ,
- Saleh Tamim 1 ,
- Jozef Lazar 1 ,
- J. Wade Davis 1 &
- Howard J. Jacob 1
BMC Medical Genomics volume 15 , Article number: 74 ( 2022 ) Cite this article
7072 Accesses
15 Citations
2 Altmetric
Metrics details
The remarkable growth of genome-wide association studies (GWAS) has created a critical need to experimentally validate the disease-associated variants, 90% of which involve non-coding variants.
To determine how the field is addressing this urgent need, we performed a comprehensive literature review identifying 36,676 articles. These were reduced to 1454 articles through a set of filters using natural language processing and ontology-based text-mining. This was followed by manual curation and cross-referencing against the GWAS catalog, yielding a final set of 286 articles.
We identified 309 experimentally validated non-coding GWAS variants, regulating 252 genes across 130 human disease traits. These variants covered a variety of regulatory mechanisms. Interestingly, 70% (215/309) acted through cis-regulatory elements, with the remaining through promoters (22%, 70/309) or non-coding RNAs (8%, 24/309). Several validation approaches were utilized in these studies, including gene expression (n = 272), transcription factor binding (n = 175), reporter assays (n = 171), in vivo models (n = 104), genome editing (n = 96) and chromatin interaction (n = 33).
Conclusions
This review of the literature is the first to systematically evaluate the status and the landscape of experimentation being used to validate non-coding GWAS-identified variants. Our results clearly underscore the multifaceted approach needed for experimental validation, have practical implications on variant prioritization and considerations of target gene nomination. While the field has a long way to go to validate the thousands of GWAS associations, we show that progress is being made and provide exemplars of validation studies covering a wide variety of mechanisms, target genes, and disease areas.
Peer Review reports
A central goal of genetics is to identify the genetic underpinnings of human diseases. Advancements in human genetics and its related fields and technologies over the past decades have had a remarkable impact on our understanding of human disease pathophysiology, diagnosis and management [ 1 ]. In Mendelian disorders and rare genetic diseases this often takes the form of a loss-of-function mutation or genomic abnormality driving the disease phenotype. There are more than 5,000 diseases that belong to this category accounted for in the Online Mendelian Inheritance in Man (OMIM) database [ 2 ]. For complex diseases, there are multiple genetic and environmental factors contributing to disease risk and the identification of genetic risk factors associated with complex diseases has been rapidly accelerating with the utilization of next generation sequencing and dense array genotyping technologies in genome-wide association studies (GWAS). In a GWAS, thousands of genetic variants are genotyped in individuals which are then used to identify statistical associations between variants at certain genomic loci and a particular phenotype [ 3 ]. Since the first reported GWAS association for age-related macular degeneration [ 4 ] the use of these studies have grown exponentially, with over 200,000 genetic variants associated with more than 3000 human traits reported [ 5 ]. The remarkable growth of GWAS has created a critical need to experimentally identify and validate the disease-associated variants [ 6 , 7 ]. This barrier has hindered the translation of GWAS findings to disease biology mechanisms and hence therapies. There are seemingly very few examples of GWAS-identified genetic loci at which the causal variant and molecular mechanisms driving the association have been experimentally determined, especially considering the sheer number of genotype–phenotype associations that have been reported passing the genome-wide significance threshold.
Dissecting GWAS loci to uncover the underlying biology is a complicated multi-step process. High linkage disequilibrium (LD) between many variants often necessitates utilizing statistical fine-mapping approaches and overlapping with functional genomic annotations for prioritization of variants before experimental validation [ 3 , 8 ]. For coding variants, the target gene is identified directly from the genomic location of the variant [ 9 ]. As protein-coding regions represent only a small percentage of the human genome, more than 90% of GWAS associated variants are annotated to be within non-coding parts of the genome [ 5 ]. Experimental identification and validation of non-coding variants involves additional level of complexity as compared to coding variants requiring the application of additional approaches [ 10 , 11 ]. Moreover, the functionality of regulatory elements is often cell-type specific, which necessitates studying the mechanism in disease-relevant cell types [ 12 ].
Experimental identification and validation are critical elements in translating GWAS findings. To date there has been limited study of the number of GWAS-identified loci that have been experimentally validated. A systematic literature review of 36,676 published articles identified 309 experimentally validated non-coding GWAS variants, regulating 252 genes across 130 human disease traits. This review of the literature is the first to systematically evaluate the status and the landscape of experimentation being used to validate non-coding GWAS-identified variants. We additionally curated key information from all included studies such as validated variant class, distance-to-target gene, and experimental validation methods. Our findings have value for future experimental validation studies, target gene prioritization and functional variant prediction. The approaches utilized to validate coding variants as well as current methods used to nominate candidate functional variants for functional studies are outside the scope of this manuscript and have been reviewed previously [ 8 , 9 ].
We conducted a systematic literature search and report it in compliance with the standards set forth by the 2020 PRISMA statement on the reporting of systematic reviews [ 13 ]. As a traditional keyword-based search approach would not enable us to thoroughly search for all relevant concepts and combinations, we leveraged natural language processing (NLP) and ontology-based text mining to ensure a systematic identification of relevant validation articles [ 14 , 15 ]. We defined the scope to include studies that perform validation of GWAS associated non-coding variants at least at a molecular level.
In order to build a comprehensive literature search strategy, we first identified 28 validation studies from recent reviews and published resources [ 6 , 7 , 16 ]. These index studies were evaluated to identify the optimal keywords and concepts that would be used in the systematic literature search. Figure 1 shows a flow diagram summarizing the systematic literature search approach that was employed. The systematic literature search was conducted using search and filter concepts identified by thorough manual and text mining-supported concept analysis of index articles. The initial broad search was based on four different sub-queries aimed at identifying any articles that might include experimental validation of GWAS variants. We included explicit mention of GWAS, non-coding, functional or causal variant as well as contextual mentions of non-coding concepts such as enhancers and promoters (Additional file 1 ). Queries were run on MEDLINE Full Index [ 17 ] (all MEDLINE content until February 19, 2021) using IQVIA/Linguamatics I2E KNIME nodes [ 18 ]. Concepts and various combinations were searched in title, abstract and meta-data (author keywords, Medical Subject Headings (MeSH) terms and substances) leveraging public standard life science ontologies (such as MeSH [ 19 ], NCI Thesaurus [ 20 ] or Entrez Gene [ 21 ], custom vocabularies and syntactical rules, grammatical pattern and linguistic entity classes allowing to build more generalized (comprehensive) queries, but at the same time more precise queries than standard key word search engines. The PMIDs identified by each query were combined and filtered for publication year ≥ 2007 (using “PubMed Publication Data (entrez)”). After removing duplicates, we arrived at 36,676 unique articles (Fig. 1 A). We built seven filters reflecting our key inclusion criteria to narrow down the search results: (1) filter for primary research articles and exclude other article types, (2) GWAS and/or association filter, (3) filter for any human disease, (4) filter for any human gene (RefSeq), (5) filter for explicit mention of “non-coding” or non-coding context (enhancers, intron, non-coding, microRNA, etc.), (6) filter for functional, causal, or regulatory variant or specific rsID, and (7) wet-lab experimental validation techniques (Fig. 1 B, Additional file 2 ). Filters were built using an in-house entity extraction and literature classification pipeline combining SciBite’s TERMite (TERM identification, tagging & extraction) API coupled with SciBite’s VOCabs [ 22 ] and IQVIA/Linguamatics I2E Software.
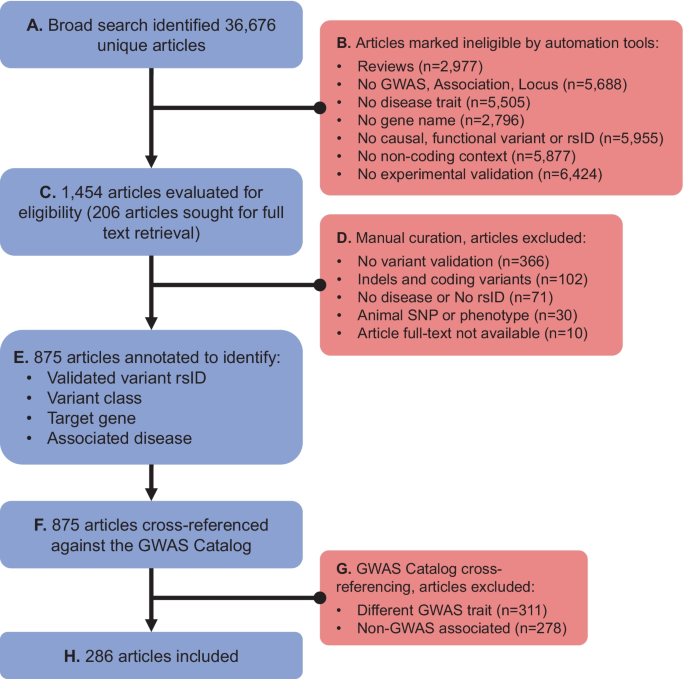
Systematic literature search and validation approach. Flow diagram demonstrating the systematic literature search strategy starting with A broad Medline search including all potentially related articles. The search included several concepts related to GWAS, non-coding contexts and other related terms detailed in Additional file 1 . B Using text-mining of article titles, abstracts and metadata, we built seven filters to narrow down the search results which excluded 35,222 articles. Exact search terms and their combinations used in the filters are provided in Additional file 2 . C 1454 articles of interest that passed all the filters were manually screened and evaluated for eligibility. D Through manual curation an additional set of 579 articles was excluded. E 875 eligible articles that passed manual curation were annotated to identify key information from each study. F These articles proceeded to cross-referencing against the GWAS Catalog to ensure that the validated variants and their reported associated disease trait match known GWAS associations. G Cross-referencing excluded 598 articles with poor GWAS trait matches or no variant match. H The final systematic review includes 286 articles. Reasons for exclusion at each stage are shown in red on the right side and described in more detail in the main text
In total 1454 articles passed all filter criteria and were then manually reviewed by three curators (Fig. 1 C). All articles had to meet the following criteria to be considered for inclusion: (1) investigate variants associated with a human disease, (2) include experimental wet-lab molecular validation of one or more variants, (3) include putative validation of at least one non-coding variant, and (4) investigate single nucleotide polymorphisms (SNPs), excluding indels, purely coding, somatic, or rare variants. Abstracts and full texts were reviewed resulting in the exclusion of 579 articles (Fig. 1 D). Overall, this manual review identified 875 potentially relevant articles. All these articles were manually curated to confirm the rsID of the reportedly validated variants, variant class, the reported regulated gene, and the associated disease (Fig. 1 E).
We then used the information on the validated variant’s rsID and disease trait to cross validate our data with the GWAS Catalog [ 5 ] (accessed Mar 25, 2021) to confirm that each curated variant-disease association is reported in a GWAS (Fig. 1 F). Corresponding associations were identified through LD between the curated SNP and the reported GWAS Catalog SNP, and similarity between the reported GWAS trait and the traits extracted from the PubMed abstract as detailed below. Because the GWAS Catalog only reports the lead variant for each locus, and this variant is not necessarily identical to the causal variant for the association, we performed an LD expansion from each top SNP to identify additional possible causal variants. Broad ancestry as reported in the GWAS Catalog was mapped to a 1000 Genomes superpopulation following methods we described recently [ 23 ]. For each associated SNP in the GWAS Catalog, an LD expansion was performed to identify SNPs within 1 Mb with LD r 2 ≥ 0.5 in the corresponding 1000 Genomes super-population. A minor allele count threshold of 5 within the corresponding superpopulation was applied to reduce the impact of high variance LD estimates for rare variants. If it was not possible to map to a single superpopulation, LD expansion was performed using the full 1000 Genomes Phase 3 GRCh38 liftover to match the build used in the GWAS Catalog [ 24 ]. When the GWAS Catalog reported a specific risk allele, our LD expansion took this into account, such that for multiallelic SNPs we would only identify variants correlated with the reported allele. The choice of LD threshold is motivated by the goal to capture GWAS associations that could plausibly be explained by the cataloged variant and has been used elsewhere[ 25 ]. Using this methodology, it was possible to perform LD expansion for 91% of variants in the GWAS Catalog. GWAS Catalog variants for which an LD expansion was not possible were still included in the analysis but could only be matched to the reported variant rather than other possible causal variants.
GWAS Catalog Experimental Factor Ontology (EFO) terms and disease terms curated from the literature were mapped to the 2020 MeSH thesaurus vocabulary using the approach outlined previously [ 26 ]. To allow for inexact matches in MeSH terms (e.g., hypertension and systolic blood pressure), we use two similarity metrics: Lin-Resnik average similarity with a cutoff value of 0.75 [ 26 , 27 ] and odds ratio of MeSH term co-occurrence in the same PubMed article with a cutoff of 20 [ 23 ]. We count a match between an article identified in our systematic review and a GWAS study if any GWAS Catalog association satisfies the following criteria: (1) The reported variant in the GWAS Catalog has LD R 2 ≥ 0.5 to at least one curated variant, and (2) the reported trait in the GWAS Catalog has similarity to a main or manually curated disease from the PubMed abstract, meeting or exceeding the cutoff value. We excluded 347 SNPs in 311 articles from the analysis due to not being linked to a GWAS Catalog SNP. A further 292 SNPs contained within 278 articles were excluded due to a poor match between the reported GWAS trait and the trait reported in the abstract (Fig. 1 G). The final curated catalog includes 286 articles (Fig. 1 H) [ 28 , 29 , 30 , 31 , 32 , 33 , 34 , 35 , 36 , 37 , 38 , 39 , 40 , 41 , 42 , 43 , 44 , 45 , 46 , 47 , 48 , 49 , 50 , 51 , 52 , 53 , 54 , 55 , 56 , 57 , 58 , 59 , 60 , 61 , 62 , 63 , 64 , 65 , 66 , 67 , 68 , 69 , 70 , 71 , 72 , 73 , 74 , 75 , 76 , 77 , 78 , 79 , 80 , 81 , 82 , 83 , 84 , 85 , 86 , 87 , 88 , 89 , 90 , 91 , 92 , 93 , 94 , 95 , 96 , 97 , 98 , 99 , 100 , 101 , 102 , 103 , 104 , 105 , 106 , 107 , 108 , 109 , 110 , 111 , 112 , 113 , 114 , 115 , 116 , 117 , 118 , 119 , 120 , 121 , 122 , 123 , 124 , 125 , 126 , 127 , 128 , 129 , 130 , 131 , 132 , 133 , 134 , 135 , 136 , 137 , 138 , 139 , 140 , 141 , 142 , 143 , 144 , 145 , 146 , 147 , 148 , 149 , 150 , 151 , 152 , 153 , 154 , 155 , 156 , 157 , 158 , 159 , 160 , 161 , 162 , 163 , 164 , 165 , 166 , 167 , 168 , 169 , 170 , 171 , 172 , 173 , 174 , 175 , 176 , 177 , 178 , 179 , 180 , 181 , 182 , 183 , 184 , 185 , 186 , 187 , 188 , 189 , 190 , 191 , 192 , 193 , 194 , 195 , 196 , 197 , 198 , 199 , 200 , 201 , 202 , 203 , 204 , 205 , 206 , 207 , 208 , 209 , 210 , 211 , 212 , 213 , 214 , 215 , 216 , 217 , 218 , 219 , 220 , 221 , 222 , 223 , 224 , 225 , 226 , 227 , 228 , 229 , 230 , 231 , 232 , 233 , 234 , 235 , 236 , 237 , 238 , 239 , 240 , 241 , 242 , 243 , 244 , 245 , 246 , 247 , 248 , 249 , 250 , 251 , 252 , 253 , 254 , 255 , 256 , 257 , 258 , 259 , 260 , 261 , 262 , 263 , 264 , 265 , 266 , 267 , 268 , 269 , 270 , 271 , 272 , 273 , 274 , 275 , 276 , 277 , 278 , 279 , 280 , 281 , 282 , 283 , 284 , 285 , 286 , 287 , 288 , 289 , 290 , 291 , 292 , 293 , 294 , 295 , 296 , 297 , 298 , 299 , 300 , 301 , 302 , 303 , 304 , 305 , 306 , 307 , 308 , 309 , 310 , 311 , 312 , 313 ].
Curated catalog of 309 validated GWAS non-coding variants
Several prior studies have emphasized the importance of experimental validations to uncover the biological processes underlying the statistical GWAS associations [ 3 , 6 , 7 , 314 , 315 ]. The final list of 286 articles reports 309 experimentally validated functional non-coding variants regulating 252 genes across 130 human-diseases (Additional file 3 and Fig. 2 ). Additional File 3 includes several important aspects about the included articles and variants including PubMed identifiers (PMID), variant rsID, location, class, target gene as well as disease associations and experimental validation approaches. We examined several characteristics of the validated non-coding variants in relation to GWAS catalog studies and variants. Between 2007 and 2020 there is a steady increase in the number of validation articles over time up to the 286 we report here. In contrast, the total number of published GWAS articles is 4342 versus 286 validation articles for non-coding variants (Fig. 3 A). Next, we evaluated the relationship between disease heritability explained by common SNPs and the ratio of validated variants to the total number of lead-GWAS variants. We mapped disease associations for all variants to the higher order disease categories in the MeSH terms tree structure. For heritability estimates, we considered liability scale h 2 for UK Biobank phenotypes estimated using LD Score Regression[ 316 , 317 ] which (1) mapped to a MeSH disease (2) were considered high or medium confidence and averaged the heritability across higher level MeSH to get average heritability per disease category. Using this approach, we find a statistically significant ( p = 0.01; correlation coefficient 0.51) positive relationship between mean heritability and the ratio of validated/lead GWAS variants per disease category (Fig. 3 B). Examination of individual validated variants showed the majority of validated variants are in strong LD with and in close proximity to the GWAS variant (Fig. 3 C, D ). Allele frequencies of validated variants have slightly skewed distribution with fewer validated variants having lower allele frequencies (Fig. 3 E). Comparing the location of experimentally validated non-coding GWAS variants to GWAS lead variants, we found that validated variants are about equally likely to be located within a protein-coding gene (58% for functional variants versus 55% for GWAS lead variants). However, they are much more likely to be within 10 kb of a gene boundary (20% versus 11%) and much less likely to be more than 100 kb from the nearest gene (7% versus 16%) (Fig. 3 F). Overall, these findings quantify the persistent need for more experimental validation studies to bridge the gap between association and biology. These findings also suggest that focusing experimental validation efforts to variants in close proximity and strong LD to the lead GWAS variant would lead to the identification of a causal variant in the majority of genetic loci.
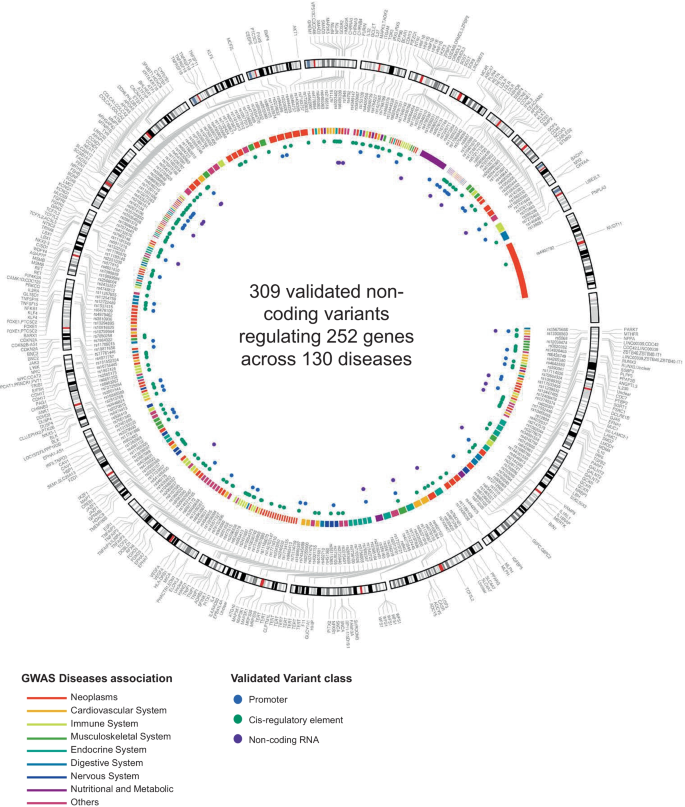
Map of 309 validated GWAS non-coding variants. The Circos plot displays the 309 experimentally validated variants studied within the 286 included articles. The outer most layer (i) shows the validated variants’ 252 target genes, (ii) the chromosomal map, (iii) the location of validated variants marked by their rsIDs, (iv) using higher order ontology mapping, we display inner links between variants associated with diseases in the same category. Disease systems that contain ten or more validated variants are displayed while those contain less than ten validated variants are grouped in “Others” category, and (v) the manually annotated validated variant class. Additional File 3 contains all variant details and annotations
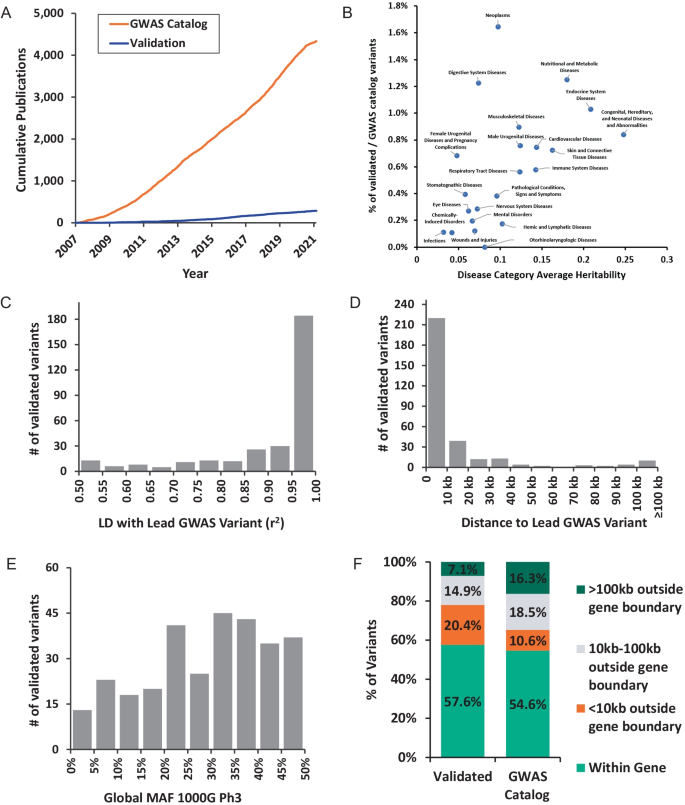
Functional validation remains the bottleneck of GWAS follow-up. A Comparison of the number of published studies in the GWAS catalog and non-coding variant validation studies over time. B Relationship between the ratio of validated non-coding variants to the total GWAS variants and disease category mean heritability. C Linkage disequilibrium between reported variant in GWAS Catalog and validated variants. D Distance between validated variant and GWAS Catalog-reported variant. E Global minor allele frequency (MAF) of validated variants in 1000 genomes phase 3. F Location of experimentally validated non-coding GWAS variants in relation to all protein-coding genes compared to GWAS lead variants
Validated variants regulate 252 target genes through a variety of mechanisms
Non-coding genetic variants can exert their effect on target genes through a variety of mechanisms [ 318 , 319 , 320 ]. We divided variants into three broad categories based on their mechanism of regulation: cis-regulatory element (CRE) variants, promoter variants and variants acting through non-coding RNAs (Fig. 4 A). Promoter variants were grouped separately from other CREs because they are functionally distinct and in addition the methods utilized for their validation are different from other CREs. Below we highlight several exemplar studies validating variants across all these mechanisms and many diseases. Interestingly, the majority of non-coding variants identified in our catalog regulate genes through CREs (n = 215). These include variants in enhancers such as rs4420550- MAPK3-TAOK2 in schizophrenia [ 168 ], rs11236797- LRRC32 in inflammatory bowel disease [ 40 ], and rs9349379- EDN1 in vascular diseases [ 49 ]. Some variants exerted their effect through silencers such as rs12038474- CDC42 in endometriosis [ 130 ], rs2494737- AKT1 in endometrial carcinoma [ 37 ] and rs9508032- FLT1 in acute respiratory distress syndrome[ 267 ]. Additionally, rs12936231- GSDMB-ORMDL3-ZPBP2 seems to function through an insulator in an asthma and autoimmune disease risk locus [ 71 ].
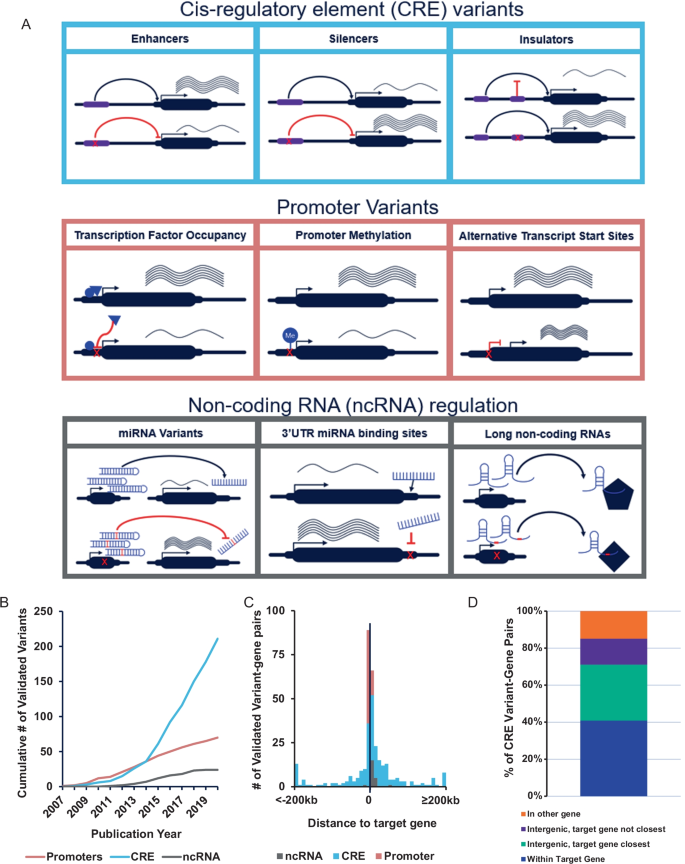
Non-coding variants regulate 252 target genes through diverse mechanisms. A Illustration of some of the diverse mechanisms of regulation within each variant category. Examples of each mechanism from included studies are discussed in the text. B Cumulative number of validated variants grouped by non-coding variant categories over time. C We used Encode’s Biomart and hg38 to calculate the distance (in kb) between validated variants and their target gene’s closest transcription start site (TSS). Graph plots the number of variant- gene pairs grouped by variant class. Variants more than 200 kb away are plotted at 200 kb. D Distribution of CRE variants relative to their target gene. CRE = Cis-Regulatory Element, ncRNA = non-coding RNA
Variants in gene promoters can alter transcription factor binding and promoter activity. For example, rs1887428- JAK2 in inflammatory bowel disease [ 256 ], rs11789015- BARX1 in esophageal adenocarcinoma [ 88 ], rs4065275- ORMDL3 and rs8076131- ORMDL3 in asthma, [ 248 ] and rs11603334- ARAP1 in type 2 diabetes mellitus [ 34 ]. DNA methylation is an important epigenetic mechanism of gene regulation and increased DNA methylation at gene promoters can repress gene transcription [ 321 , 322 ]. We identified several validated variants that appear to alter promoter methylation including rs780093- NRBP1 in gout [ 127 ], rs143383- GDF5 in osteoarthritis [ 119 ], and rs35705950- MUC5B in idiopathic pulmonary fibrosis [ 258 ]. Alternatively, variants could alter promoter and transcription start site usage. Examples for these mechanisms in our catalog include rs922483 -BLK in systemic lupus erythematosus [ 302 ] and rs10465885- GJA5 in atrial fibrillation [ 32 ].
The third broad category by which variants from our catalog exert their regulatory effect is through non-coding RNAs [ 323 ]. microRNAs are a major and well-studied class of regulatory small non-coding RNAs. Variants in microRNAs are known to impact disease biology through post-transcriptional regulation of their target genes, primarily via 3’ untranslated region (UTR) binding [ 324 , 325 , 326 ]. GWAS variants located within microRNAs can alter their biogenesis, expression levels and/or target specificity, while variants located in target genes are capable of altering microRNA binding sites [ 326 ]. Examples of validated variants within microRNAs included in this catalog are miR-196a2 variant rs11614913 regulating SFMBT1 and HOXC8 in metabolic syndrome [ 277 ], and miR-4513 variant rs2168518 regulating GOSR2 in cardiometabolic diseases [ 51 ]. Given that microRNAs typically target hundreds to thousands of genes, it is very difficult to confidently assign target genes that are mediating the effect of a microRNA variant. On the other hand, studying variants located within mircoRNA-binding sites of target genes may yield more success in assigning underlying mechanisms [ 326 , 327 ]. There are numerous examples of such variants reported in this catalog, such as rs5068 altering regulation of NPPA by miR-425 in hypertension [ 96 ], rs1058205 altering regulation of KLK3 by miR-3162-5p and rs1010 altering regulation of VAMP8 by miR-370 in prostate cancer [ 54 ], and rs372883 altering BACH1 regulation by miR-1257 in pancreatic ductal adenocarcinoma [ 174 ]. Another important class of non-coding RNAs is long non-coding RNAs that are recognized to play an important role in biology and disease [ 328 , 329 ]. Some examples of long non-coding RNA variants in this catalog include rs6983267 in CCAT2 regulating cancer metabolism through allele-specific binding of CPSF7 [ 76 ] and rs2147578 in LAMC2-1 modulating microRNA binding to it in colorectal cancer [ 43 ]. We examined the distribution of these three broad categories of validated variants across publication dates. We observed a steady increase in the validation of promoter variants (n = 70) and variants acting through non-coding RNAs (n = 24) since 2007, but a sharp increase in the number of studies validating CRE variants around 2015. This trend persisted through 2020 to reach a total of 215 variants representing 70% of this catalog (Fig. 4 B). We also characterized the distance between each validated variant and its target gene’s closest transcription start site according to variant category. As expected, promoter variants clustered immediately upstream or downstream of their target’s transcription start site. CRE variants were more widely distributed, but nevertheless, 157 (66%) of these fell within 50 kb from their target gene TSS. A notable example of a distally acting enhancer variant > 50 kb, is the obesity FTO locus variant rs1421085 regulating IRX3 and IRX5, which are 500 kb and 1,163 kb away respectively [ 147 ]. Since the majority of variants acting through non-coding RNAs identified in our catalog were located within 3’ UTRs, this group of variants tended to cluster within 100 kb downstream of gene transcript start sites (Fig. 4 C). The dataset gave us the opportunity to examine the relationship between CRE variants and their target genes (n = 235 CRE variant-target gene pairs). Plotting the distribution of CRE variants based on their location relative to the target gene indicated that 41% of CRE variants are located within their target gene, and an additional 30% are intergenic and their target gene is the closest gene to the variant. 14% of CRE variants were intergenic and their target gene is not the closest gene, and the remaining 15% are located within a different gene than their target gene. (Fig. 4 D). These results are interesting and provide greater support for consideration of same gene and nearby genes as candidate targets for CREs. These findings are also in agreement with recent empirical data [ 330 , 331 ].
Next, using text mining, we extracted and analyzed the experimental methods that were used in each study to validate variants. We broadly classified them under six broad categories covering different types of established validation techniques and related terms: (1) gene expression, including eQTL and molecular assessment of target gene expression and allele specific regulation (n = 272 articles), (2) reporter assays, including luciferase and massively parallel reporter assays (n = 171 articles), (3) transcription factor binding, including chromatin immunoprecipitation and electrophoretic mobility shift assays (n = 175 articles), (4) in vivo or animal models (n = 104 articles), (5) genome editing, including CRISPR and TALEN (n = 96 articles), and (6) chromatin interaction, including chromosome conformation capture (n = 33 articles) [ 11 ]. We examined the number of these approaches that were utilized by the included studies and found that 189 (66%) of all articles utilized three or more approaches (Fig. 5 ). These results demonstrate the multifaceted approach needed for validation of non-coding variants [ 11 ].
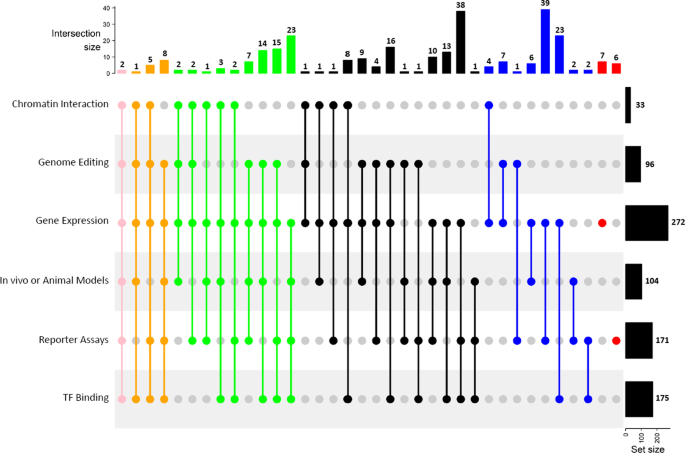
Studies utilize multiple avenues in validating non-coding variants. Using text-mining of abstracts and metadata, we examined the utilization of different avenues for non-coding variant validation across 286 included articles. The six broad categories were gene expression, reporter assays, transcription factor binding, in vivo or animal models, genome editing, and chromatin interaction. The intersection size denotes the number of articles that have the combination of validation categories below it. The color denotes the number of avenues used; pink – 6, orange—5, green—4, black—3, blue—2, red—1. The upset plot shows the overlap of the variant validation avenues and the number of articles. The Set size bars on the right reflect the total number of studies that used/employed each of the categories
GWAS have seen a remarkable growth in the past decade. The impact of GWAS on human healthcare is severely limited by the bottle neck of experimental validation of disease-associated variants. Here, we report the first systematic approach to curate all experimental validation studies of non-coding GWAS variants. While there is general recognition that experimental validation of GWAS are seriously lacking [ 7 ], this systematic assessment of (1) the number of published experimentally validated non-coding variants is quantified, (2) cataloged, and (3) methods used in identified studies analyzed.
Using a comprehensive approach, we employed natural-language processing-based text mining, manual curation and GWAS catalog cross validation. We have curated 286 validation studies that include 309 putatively validated variants regulating 252 genes across 130 diseases. We then evaluated several important characteristics of the identified variants and their relation to GWAS lead variants. The ratio of validated non-coding variants to total GWAS lead variants showed a positive correlation to the mean heritability of disease groups. This relationship could indicate greater success in validating variants in diseases with higher heritability perhaps because of greater individual contribution of these variants to the overall disease susceptibility. This could also potentially represent a greater interest of scientists to pursue validation of variants in more heritable diseases and with larger effect sizes, thus leading to greater proportion of variants being validated. However, we do not have enough data to directly address this possibility. We also evaluated the relationship in LD and distance between validated variants and GWAS lead variants. We find that ~ 70% of validated variants fall within 10 kb and r 2 ≥ 0.9 with the lead GWAS variant. On one hand, this could reflect underlying genetics that most validated variants are in strong LD with lead GWAS variants and suggests that more productive research should be limited to SNPs in high LD and closer distance to lead GWAS variants. On the other hand, the status quo might be reflective of prior limits in search space already considered by scientists who performed validation studies, however we do not have data to support this possibility[ 8 ].
Next, we annotated variants into broad classes based on the mechanisms by which these non-coding variants acted. This identified several interesting patterns, such as an increase in the number of variants functioning through cis-regulatory elements over time. One explanation for this increase could be the growing awareness of the importance of these regulatory elements in human biology and disease which has led to the initiation of large projects aimed at identification, annotation and prioritization of non-coding regulatory elements [ 10 , 320 , 332 ]. Additionally, several SNP-enrichment analyses have demonstrated that GWAS variants are significantly enriched in active regulatory regions [ 314 ]. We expect this trend to continue with publications by larger consortia and projects that investigate regulatory elements in different life stages, tissues and biological conditions [ 332 ]. Interestingly, the majority of cis-regulatory element variants that we found appeared to act through transcriptional enhancers. This dominance of enhancer variants over other regulatory elements might be a result of enhancer elements having more clearly defined functions and biochemical markers (i.e., histone modification signatures) [ 333 , 334 ]. This highlights the potential for increased discovery of GWAS variants acting through silencers and insulators as our understanding of their distinct biochemical signatures is refined and assayed in disease relevant cell types [ 333 , 335 ].
Our comprehensive search and filter strategy enabled us to identify validated variants across a large number of complex human diseases and those that act through a myriad of mechanisms. Nevertheless, the systematic search was limited to the MEDLINE database. Relevant articles published in journals not indexed in this standard database for biomedical literature will be missing in our data set [ 336 , 337 ]. For quality control and to identify limitations of our search and filter approach, we analyzed the recall of our index studies throughout the entire process (Fig. 1 A–H). It is important to highlight that broadening the initial search to include non-coding contexts and association/locus instead of limiting to explicit mentions of non-coding and GWAS terms ensured identification of relevant studies that we had otherwise missed. A significant number of index articles did not explicitly mention these terms [ 48 , 78 , 134 , 143 , 147 , 171 , 178 , 210 , 230 , 256 , 302 ]. Our final broad search covered 27 out of the 28 index studies which demonstrates good search coverage. Through an iterative process, we narrowed down these results, trying to maximize the recall of index studies while maintaining a manageable number of articles for manual review. We are aware that the implemented stringent criteria bias the search to exclude true validation articles that did not mention any disease, protein or specific experimental validation terms [ 338 , 339 , 340 , 341 , 342 , 343 , 344 , 345 ]. Additionally, the tagging of the articles and normalization of concepts for filtering relies on accurate named entity recognition (NER) and ontologies. Even when using highly curated, enriched vocabularies and state-of-the-art NER routines, recall rates of at maximum 80–95% are assumed (depending on entity type). Overall, a total of 19 index studies passed all filtering stages and were included in the final catalog. Finally, the data of our curated catalog is mainly based on the publications’ abstract information. Only in cases where information was missing or unclear in the abstract did we gather data from the full text. Therefore, it is possible that information gathered from the final set of articles may be incomplete. This would have affected the experimental validation techniques analysis in particular, which was based only on abstract mining.
Construction of the catalog using controlled vocabularies for diseases, variants, genes, variant classes, and functional follow up methods is aimed to facilitate use in bioinformatics follow up analyses. We expect this resource to be useful in evaluating the performance of computational fine mapping and target prioritization methods. Quantifying the performance of these methods on real datasets has previously been hindered by a lack of true positive examples. A large dataset of true positive examples would allow researchers to computationally identify features associated with functional variation. Recent efforts to compile such true positive datasets and use them to train target prioritization methods have come with concerns about bias towards coding variation [ 16 ] or are aimed at a specific trait subset such as molecular phenotypes [ 346 ] or immune disease [ 347 ]. We expect this catalog to contribute a large number of much needed examples of functional noncoding variants in human disease and the genes on which they act. Despite this important contribution, bias towards nearby genes and variants to the top GWAS SNP is still a concern for our catalog due to the limited number of variants and genes evaluated in the cataloged studies. To generate an unbiased training set for computational methods, an ideal functional study following up on a GWAS association would consider all credible causal SNPs and their nearby genes, but studies in our catalog typically consider a more limited set of genes and SNPs. For example, eQTL variants may be shared among multiple transcripts [ 348 ], and in this scenario functional studies considering only a single gene could be misleading about the causal gene.
This review is the first to systematically evaluate the status and the landscape of experimentation being used to validate non-coding GWAS-identified variants. Our results clearly underscore the multifaceted approach needed for experimental validation. The findings of validated variants relationship to lead GWAS variants as well as to their target genes provide practical insights for future validation studies. Finally, we aim for the catalog to be a useful resource aiding in the development of prediction tools by providing a truth set of experimentally validated variants. Collectively this contributes to the overall effort to bridge the gap between genetic association and function in complex diseases.
Availability of data and materials
The data supporting the conclusions of this article is included within the article (and its additional files).
Abbreviations
Cis-regulatory element
Genome-Wide Association Study
Linkage disequilibrium
Medical subject headings
Preferred reporting items for systematic reviews and meta-analyses
Single nucleotide polymorphism
Collins FS, Doudna JA, Lander ES, Rotimi CN. Human molecular genetics and genomics—Important advances and exciting possibilities. N Engl J Med. 2021;384:1–4.
Article CAS PubMed Google Scholar
OMIM - Online Mendelian Inheritance in Man. https://www.omim.org/ . 2021 [cited 2021 Apr 11]; Available from: https://www.omim.org/
Tam V, Patel N, Turcotte M, Bossé Y, Paré G, Meyre D. Benefits and limitations of genome-wide association studies. Nat Rev Genet. 2019;20:467–84.
Klein RJ, Zeiss C, Chew EY, Tsai J-Y, Sackler RS, Haynes C, et al. Complement factor H polymorphism in age-related macular degeneration. Science. 2005;308:385–9.
Article CAS PubMed PubMed Central Google Scholar
Buniello A, MacArthur JAL, Cerezo M, Harris LW, Hayhurst J, Malangone C, et al. The NHGRI-EBI GWAS Catalog of published genome-wide association studies, targeted arrays and summary statistics 2019. Nucleic Acids Res. 2019;47:D1005–12.
Visscher PM, Wray NR, Zhang Q, Sklar P, McCarthy MI, Brown MA, et al. 10 Years of GWAS discovery: biology, function, and translation. Am J Human Genet. 2017;101:5–22.
Article CAS Google Scholar
Gallagher MD, Chen-Plotkin AS. The Post-GWAS Era: From Association to Function. Am J Hum Genet. 2018;102:717–30.
Schaid DJ, Chen W, Larson NB. From genome-wide associations to candidate causal variants by statistical fine-mapping. Nat Rev Genet. 2018;19:491–504.
Cai M, Ran D, Zhang X. Advances in identifying coding variants of common complex diseases. J Bio-X Res. 2019;2:153–8.
Google Scholar
Tak YG, Farnham PJ. Making sense of GWAS: using epigenomics and genome engineering to understand the functional relevance of SNPs in non-coding regions of the human genome. Epigenetics Chromatin. 2015;8:57.
Article PubMed PubMed Central Google Scholar
Rao S, Yao Y, Bauer DE. Editing GWAS: experimental approaches to dissect and exploit disease-associated genetic variation. Genome Med. 2021;13:41.
Liu B, Montgomery SB. Identifying causal variants and genes using functional genomics in specialized cell types and contexts. Hum Genet. 2020;139:95–102.
Article PubMed Google Scholar
Page MJ, McKenzie JE, Bossuyt PM, Boutron I, Hoffmann TC, Mulrow CD, et al. The PRISMA 2020 statement: an updated guideline for reporting systematic reviews. BMJ: Br Med J Publ Group. 2021;372:71.
Article Google Scholar
Chang M, Chang M, Reed JZ, Milward D, Xu JJ, Cornell WD. Developing timely insights into comparative effectiveness research with a text-mining pipeline. Drug Discov Today. 2016;21:473–80.
McEntire R, Szalkowski D, Butler J, Kuo MS, Chang M, Chang M, et al. Application of an automated natural language processing (NLP) workflow to enable federated search of external biomedical content in drug discovery and development. Drug Discov Today. 2016;21:826–35.
Ghoussaini M, Mountjoy E, Carmona M, Peat G, Schmidt EM, Hercules A, et al. Open Targets Genetics: systematic identification of trait-associated genes using large-scale genetics and functional genomics. Nucleic Acids Res. 2021;49:D1311–20.
MEDLINE. http://wayback.archive-it.org/org-350/20180312141554/https://www.nlm.nih.gov/pubs/factsheets/medline.html . 2021 [cited 2021 Jun 15]; Available from: http://wayback.archive-it.org/org-350/20180312141554/https://www.nlm.nih.gov/pubs/factsheets/medline.html
Linguamatics. https://www.linguamatics.com/ . 2021 [cited 2021 Jun 15]; Available from: https://www.linguamatics.com/
Medical Subject Headings - Home Page [Internet]. U.S. National Library of Medicine; [cited 2021 Jun 15]. Available from: https://www.nlm.nih.gov/mesh/meshhome.html
NCI Thesaurus. https://ncit.nci.nih.gov/ncitbrowser/ . 2021;
NCBI Gene Database. https://www.ncbi.nlm.nih.gov/gene/ . 2021 [cited 2021 Jun 15]; Available from: https://www.ncbi.nlm.nih.gov/gene/
TERMite - SciBite. https://www.scibite.com/platform/termite/ . SciBite [Internet]. 2021 [cited 2021 Jun 15]; Available from: https://www.scibite.com/platform/termite/
King EA, Dunbar F, Davis JW, Degner JF. Estimating colocalization probability from limited summary statistics. BMC Bioinform. 2021;22:254.
Auton A, Abecasis GR, Altshuler DM, Durbin RM, Abecasis GR, Bentley DR, et al. A global reference for human genetic variation. Nature. 2015;526:68–74.
Farh KK-H, Marson A, Zhu J, Kleinewietfeld M, Housley WJ, Beik S, et al. Genetic and epigenetic fine mapping of causal autoimmune disease variants. Nature. 2015;518:337–43.
King EA, Davis JW, Degner JF. Are drug targets with genetic support twice as likely to be approved? Revised estimates of the impact of genetic support for drug mechanisms on the probability of drug approval. PLoS Genet. 2019;15:e1008489.
Nelson MR, Tipney H, Painter JL, Shen J, Nicoletti P, Shen Y, et al. The support of human genetic evidence for approved drug indications. Nat Genet. 2015;47:856–60.
Almontashiri NAM, Antoine D, Zhou X, Vilmundarson RO, Zhang SX, Hao KN, et al. 9p21.3 coronary artery disease risk variants disrupt TEAD transcription factor-dependent transforming growth factor β regulation of p16 expression in human aortic smooth muscle cells. Circulation. 2015;132:1969–78.
Yu C-Y, Han J-X, Zhang J, Jiang P, Shen C, Guo F, et al. A 16q22.1 variant confers susceptibility to colorectal cancer as a distal regulator of ZFP90. Oncogene. 2020;39:1347–60.
Piao X, Yahagi N, Takeuchi Y, Aita Y, Murayama Y, Sawada Y, et al. A candidate functional SNP rs7074440 in TCF7L2 alters gene expression through C-FOS in hepatocytes. FEBS Lett England. 2018;592:422–33.
Kretschmer A, Möller G, Lee H, Laumen H, von Toerne C, Schramm K, et al. A common atopy-associated variant in the Th2 cytokine locus control region impacts transcriptional regulation and alters SMAD3 and SP1 binding. Allergy Denmark. 2014;69:632–42.
Wirka RC, Gore S, Van Wagoner DR, Arking DE, Lubitz SA, Lunetta KL, et al. A common connexin-40 gene promoter variant affects connexin-40 expression in human atria and is associated with atrial fibrillation. Circ Arrhythm Electrophysiol. 2011;4:87–93.
Lattka E, Eggers S, Moeller G, Heim K, Weber M, Mehta D, et al. A common FADS2 promoter polymorphism increases promoter activity and facilitates binding of transcription factor ELK1. J Lipid Res. 2010;51:182–91.
Kulzer JR, Stitzel ML, Morken MA, Huyghe JR, Fuchsberger C, Kuusisto J, et al. A common functional regulatory variant at a type 2 diabetes locus upregulates ARAP1 expression in the pancreatic beta cell. Am J Hum Genet. 2014;94:186–97.
Choi J, Xu M, Makowski MM, Zhang T, Law MH, Kovacs MA, et al. A common intronic variant of PARP1 confers melanoma risk and mediates melanocyte growth via regulation of MITF. Nat Genet United States. 2017;49:1326–35.
Kycia I, Wolford BN, Huyghe JR, Fuchsberger C, Vadlamudi S, Kursawe R, et al. A common Type 2 diabetes risk variant potentiates activity of an evolutionarily conserved islet stretch enhancer and increases C2CD4A and C2CD4B expression. Am J Hum Genet. 2018;102:620–35.
Painter JN, Kaufmann S, O’Mara TA, Hillman KM, Sivakumaran H, Darabi H, et al. A common variant at the 14q32 endometrial cancer risk locus activates AKT1 through YY1 binding. Am J Hum Genet. 2016;98:1159–69.
Guo X, Lin W, Bao J, Cai Q, Pan X, Bai M, et al. A Comprehensive cis-eQTL analysis revealed target genes in breast cancer susceptibility loci identified in genome-wide association studies. Am J Hum Genet. 2018;102:890–903.
Gallagher MD, Posavi M, Huang P, Unger TL, Berlyand Y, Gruenewald AL, et al. A dementia-associated risk variant near TMEM106B alters chromatin architecture and gene expression. Am J Hum Genet. 2017;101:643–63.
Nasrallah R, Imianowski CJ, Bossini-Castillo L, Grant FM, Dogan M, Placek L, et al. A distal enhancer at risk locus 11q13.5 promotes suppression of colitis by T(reg) cells. Nature. 2020;583:447–52.
Díaz-Jiménez D, Núñez L, De la Fuente M, Dubois-Camacho K, Sepúlveda H, Montecino M, et al. A functional IL1RL1 variant regulates corticosteroid-induced sST2 expression in ulcerative colitis. Sci Rep. 2017;7:10180.
Shou W, Wang Y, Xie F, Wang B, Yang L, Wu H, et al. A functional polymorphism affecting the APOA5 gene expression is causally associated with plasma triglyceride levels conferring coronary atherosclerosis risk in Han Chinese Population. Biochim Biophys Acta Netherlands. 2014;1842:2147–54.
Gong J, Tian J, Lou J, Ke J, Li L, Li J, et al. A functional polymorphism in lnc-LAMC2-1:1 confers risk of colorectal cancer by affecting miRNA binding. Carcinogenesis England. 2016;37:443–51.
Saeki N, Saito A, Choi IJ, Matsuo K, Ohnami S, Totsuka H, et al. A functional single nucleotide polymorphism in mucin 1, at chromosome 1q22, determines susceptibility to diffuse-type gastric cancer. Gastroenterol USA. 2011;140:892–902.
Ogura Y, Kou I, Miura S, Takahashi A, Xu L, Takeda K, et al. A functional SNP in BNC2 is associated with adolescent idiopathic scoliosis. Am J Hum Genet. 2015;97:337–42.
Ye J, Tucker NR, Weng L-C, Clauss S, Lubitz SA, Ellinor PT. A functional variant associated with atrial fibrillation regulates PITX2c expression through TFAP2a. Am J Hum Genet. 2016;99:1281–91.
Akamatsu S, Takata R, Ashikawa K, Hosono N, Kamatani N, Fujioka T, et al. A functional variant in NKX3.1 associated with prostate cancer susceptibility down-regulates NKX3.1 expression. Hum Mol Genet. 2010;19:4265–72.
Ali MW, Patro CPK, Zhu JJ, Dampier CH, Plummer SJ, Kuscu C, et al. A functional variant on 20q13.33 related to glioma risk alters enhancer activity and modulates expression of multiple genes. Hum Mutat. 2021;42:77–88.
Gupta RM, Hadaya J, Trehan A, Zekavat SM, Roselli C, Klarin D, et al. A genetic variant associated with five vascular diseases is a distal regulator of endothelin-1 gene expression. Cell. 2017;170:522-533.e15.
De Castro-Orós I, Pérez-López J, Mateo-Gallego R, Rebollar S, Ledesma M, León M, et al. A genetic variant in the LDLR promoter is responsible for part of the LDL-cholesterol variability in primary hypercholesterolemia. BMC Med Genomics. 2014;7:17.
Ghanbari M, de Vries PS, de Looper H, Peters MJ, Schurmann C, Yaghootkar H, et al. A genetic variant in the seed region of miR-4513 shows pleiotropic effects on lipid and glucose homeostasis, blood pressure, and coronary artery disease. Hum Mutat USA. 2014;35:1524–31.
Stegeman S, Moya L, Selth LA, Spurdle AB, Clements JA, Batra J. A genetic variant of MDM4 influences regulation by multiple microRNAs in prostate cancer. Endocr Relat Cancer England. 2015;22:265–76.
Schaefer AS, Richter GM, Nothnagel M, Manke T, Dommisch H, Jacobs G, et al. A genome-wide association study identifies GLT6D1 as a susceptibility locus for periodontitis. Hum Mol Genet England. 2010;19:553–62.
Stegeman S, Amankwah E, Klein K, O’Mara TA, Kim D, Lin H-Y, et al. A large-scale analysis of genetic variants within putative miRNA binding sites in prostate cancer. Cancer Discov. 2015;5:368–79.
Kahali B, Chen Y, Feitosa MF, Bielak LF, O’Connell JR, Musani SK, et al. A noncoding variant near PPP1R3B promotes liver glycogen storage and MetS, but protects against myocardial infarction. J Clin Endocrinol Metab. 2021;106:372–87.
Yan R, Lai S, Yang Y, Shi H, Cai Z, Sorrentino V, et al. A novel type 2 diabetes risk allele increases the promoter activity of the muscle-specific small ankyrin 1 gene. Sci Rep. 2016;6:25105.
Rodriguez BAT, Bhan A, Beswick A, Elwood PC, Niiranen TJ, Salomaa V, et al. A platelet function modulator of thrombin activation is causally linked to cardiovascular disease and affects PAR4 receptor signaling. Am J Hum Genet. 2020;107:211–21.
Hing B, Davidson S, Lear M, Breen G, Quinn J, McGuffin P, et al. A polymorphism associated with depressive disorders differentially regulates brain derived neurotrophic factor promoter IV activity. Biol Psychiatry. 2012;71:618–26.
Schieck M, Sharma V, Michel S, Toncheva AA, Worth L, Potaczek DP, et al. A polymorphism in the TH 2 locus control region is associated with changes in DNA methylation and gene expression. Allergy Denmark. 2014;69:1171–80.
Huang Q, Whitington T, Gao P, Lindberg JF, Yang Y, Sun J, et al. A prostate cancer susceptibility allele at 6q22 increases RFX6 expression by modulating HOXB13 chromatin binding. Nat Genet United States. 2014;46:126–35.
Chang J, Tian J, Yang Y, Zhong R, Li J, Zhai K, et al. A Rare Missense variant in TCF7L2 associates with colorectal cancer risk by interacting with a GWAS-identified regulatory variant in the MYC enhancer. Cancer Res United States. 2018;78:5164–72.
CAS Google Scholar
Walavalkar K, Saravanan B, Singh AK, Jayani RS, Nair A, Farooq U, et al. A rare variant of African ancestry activates 8q24 lncRNA hub by modulating cancer associated enhancer. Nat Commun. 2020;11:3598.
Sinnott-Armstrong N, Sousa IS, Laber S, Rendina-Ruedy E, Nitter Dankel SE, Ferreira T, et al. A regulatory variant at 3q21.1 confers an increased pleiotropic risk for hyperglycemia and altered bone mineral density. Cell Metab. 2021;33:615-628.e13.
Chinnaswamy S, Chatterjee S, Boopathi R, Mukherjee S, Bhattacharjee S, Kundu TK. A single nucleotide polymorphism associated with hepatitis C virus infections located in the distal region of the IL28B promoter influences NF-κB-mediated gene transcription. PLoS ONE. 2013;8:e75495.
Lidral AC, Liu H, Bullard SA, Bonde G, Machida J, Visel A, et al. A single nucleotide polymorphism associated with isolated cleft lip and palate, thyroid cancer and hypothyroidism alters the activity of an oral epithelium and thyroid enhancer near FOXE1. Hum Mol Genet. 2015;24:3895–907.
Dos Santos C, Bougnères P, Fradin D. A single-nucleotide polymorphism in a methylatable Foxa2 binding site of the G6PC2 promoter is associated with insulin secretion in vivo and increased promoter activity in vitro. Diabetes. 2009;58:489–92.
Roman TS, Cannon ME, Vadlamudi S, Buchkovich ML, Wolford BN, Welch RP, et al. A Type 2 diabetes-associated functional regulatory variant in a pancreatic islet enhancer at the ADCY5 locus. Diabetes. 2017;66:2521–30.
Hiramoto M, Udagawa H, Ishibashi N, Takahashi E, Kaburagi Y, Miyazawa K, et al. A type 2 diabetes-associated SNP in KCNQ1 (rs163184) modulates the binding activity of the locus for Sp3 and Lsd1/Kdm1a, potentially affecting CDKN1C expression. Int J Mol Med. 2018;41:717–28.
CAS PubMed Google Scholar
Justice CM, Kim J, Kim S-D, Kim K, Yagnik G, Cuellar A, et al. A variant associated with sagittal nonsyndromic craniosynostosis alters the regulatory function of a non-coding element. Am J Med Genet A. 2017;173:2893–7.
Jee SH, Sull JW, Lee J-E, Shin C, Park J, Kimm H, et al. Adiponectin concentrations: a genome-wide association study. Am J Hum Genet. 2010;87:545–52.
Verlaan DJ, Berlivet S, Hunninghake GM, Madore A-M, Larivière M, Moussette S, et al. Allele-specific chromatin remodeling in the ZPBP2/GSDMB/ORMDL3 locus associated with the risk of asthma and autoimmune disease. Am J Hum Genet. 2009;85:377–93.
Li X-X, Peng T, Gao J, Feng J-G, Wu D-D, Yang T, et al. Allele-specific expression identified rs2509956 as a novel long-distance cis-regulatory SNP for SCGB1A1, an important gene for multiple pulmonary diseases. Am J Physiol Lung Cell Mol Physiol. 2019;317:L456–63.
Palstra R-J, de Crignis E, Röling MD, van Staveren T, Kan TW, van Ijcken W, et al. Allele-specific long-distance regulation dictates IL-32 isoform switching and mediates susceptibility to HIV-1. Sci Adv. 2018;4:e1701729.
Benaglio P, D’Antonio-Chronowska A, Ma W, Yang F, Young Greenwald WW, Donovan MKR, et al. Allele-specific NKX2-5 binding underlies multiple genetic associations with human electrocardiographic traits. Nat Genet. 2019;51:1506–17.
Lee H, Qian K, von Toerne C, Hoerburger L, Claussnitzer M, Hoffmann C, et al. Allele-specific quantitative proteomics unravels molecular mechanisms modulated by cis-regulatory PPARG locus variation. Nucleic Acids Res. 2017;45:3266–79.
Redis RS, Vela LE, Lu W, Ferreira de Oliveira J, Ivan C, Rodriguez-Aguayo C, et al. Allele-specific reprogramming of cancer metabolism by the long non-coding RNA CCAT2. Mol Cell. 2016;61:520–34.
Richards TJ, Park C, Chen Y, Gibson KF, Di Peter Y, Pardo A, et al. Allele-specific transactivation of matrix metalloproteinase 7 by FOXA2 and correlation with plasma levels in idiopathic pulmonary fibrosis. Am J Physiol Lung Cell Mol Physiol. 2012;302:L746-754.
Fogarty MP, Panhuis TM, Vadlamudi S, Buchkovich ML, Mohlke KL. Allele-specific transcriptional activity at type 2 diabetes-associated single nucleotide polymorphisms in regions of pancreatic islet open chromatin at the JAZF1 locus. Diabetes. 2013;62:1756–62.
Nakaoka H, Gurumurthy A, Hayano T, Ahmadloo S, Omer WH, Yoshihara K, et al. Allelic imbalance in regulation of ANRIL through chromatin interaction at 9p21 endometriosis risk locus. PLoS Genet. 2016;12:e1005893.
Pittman AM, Naranjo S, Jalava SE, Twiss P, Ma Y, Olver B, et al. Allelic variation at the 8q23.3 colorectal cancer risk locus functions as a cis-acting regulator of EIF3H. PLoS Genet. 2010;6:e1001126.
Barrie ES, Lee S-H, Frater JT, Kataki M, Scharre DW, Sadee W. Alpha-synuclein mRNA isoform formation and translation affected by polymorphism in the human SNCA 3’UTR. Mol Genet Genomic Med. 2018;6:565–74.
Article CAS PubMed Central Google Scholar
Gallego X, Cox RJ, Laughlin JR, Stitzel JA, Ehringer MA. Alternative CHRNB4 3’-UTRs mediate the allelic effects of SNP rs1948 on gene expression. PLoS ONE. 2013;8:e63699.
Wasserman NF, Aneas I, Nobrega MA. An 8q24 gene desert variant associated with prostate cancer risk confers differential in vivo activity to a MYC enhancer. Genome Res. 2010;20:1191–7.
Thynn HN, Chen X-F, Hu W-X, Duan Y-Y, Zhu D-L, Chen H, et al. An allele-specific functional SNP associated with two systemic autoimmune diseases modulates IRF5 expression by long-range chromatin loop formation. J Invest Dermatol United States. 2020;140:348-360.e11.
Roberts AR, Vecellio M, Chen L, Ridley A, Cortes A, Knight JC, et al. An ankylosing spondylitis-associated genetic variant in the IL23R-IL12RB2 intergenic region modulates enhancer activity and is associated with increased Th1-cell differentiation. Ann Rheum Dis. 2016;75:2150–6.
Caussy C, Charrière S, Marçais C, Di Filippo M, Sassolas A, Delay M, et al. An APOA5 3’ UTR variant associated with plasma triglycerides triggers APOA5 downregulation by creating a functional miR-485-5p binding site. Am J Hum Genet. 2014;94:129–34.
Wang S, Wen F, Wiley GB, Kinter MT, Gaffney PM. An enhancer element harboring variants associated with systemic lupus erythematosus engages the TNFAIP3 promoter to influence A20 expression. PLoS Genet. 2013;9:e1003750.
Yan C, Ji Y, Huang T, Yu F, Gao Y, Gu Y, et al. An esophageal adenocarcinoma susceptibility locus at 9q22 also confers risk to esophageal squamous cell carcinoma by regulating the function of BARX1. Cancer Lett Ireland. 2018;421:103–11.
Savic D, Bell GI, Nobrega MA. An in vivo cis-regulatory screen at the type 2 diabetes associated TCF7L2 locus identifies multiple tissue-specific enhancers. PLoS ONE. 2012;7:e36501.
Zhao H, Yang W, Qiu R, Li J, Xin Q, Wang X, et al. An intronic variant associated with systemic lupus erythematosus changes the binding affinity of Yinyang1 to downregulate WDFY4. Genes Immun England. 2012;13:536–42.
Chen X-F, Zhu D-L, Yang M, Hu W-X, Duan Y-Y, Lu B-J, et al. An osteoporosis risk SNP at 1p36.12 acts as an allele-specific enhancer to modulate LINC00339 expression via long-range loop formation. Am J Hum Genet. 2018;102:776–93.
Liu H, Duncan K, Helverson A, Kumari P, Mumm C, Xiao Y, et al. Analysis of zebrafish periderm enhancers facilitates identification of a regulatory variant near human KRT8/18. Elife. 2020;9.
Park JH, Chang HS, Park C-S, Jang A-S, Park BL, Rhim TY, et al. Association analysis of CD40 polymorphisms with asthma and the level of serum total IgE. Am J Respir Crit Care Med. 2007;175:775–82.
Zhao Z, Fan Q, Zhou P, Ye H, Cai L, Lu Y. Association of alpha A-crystallin polymorphisms with susceptibility to nuclear age-related cataract in a Han Chinese population. BMC Ophthalmol. 2017;17:133.
De T, Alarcon C, Hernandez W, Liko I, Cavallari LH, Duarte JD, et al. Association of genetic variants with warfarin-associated bleeding among patients of African descent. JAMA. 2018;320:1670–7.
Arora P, Wu C, Khan AM, Bloch DB, Davis-Dusenbery BN, Ghorbani A, et al. Atrial natriuretic peptide is negatively regulated by microRNA-425. J Clin Invest. 2013;123:3378–82.
Gao P, Xia J-H, Sipeky C, Dong X-M, Zhang Q, Yang Y, et al. Biology and clinical implications of the 19q13 aggressive prostate cancer susceptibility locus. Cell. 2018;174:576-589.e18.
Bai X, Mangum KD, Dee RA, Stouffer GA, Lee CR, Oni-Orisan A, et al. Blood pressure-associated polymorphism controls ARHGAP42 expression via serum response factor DNA binding. J Clin Invest. 2017;127:670–80.
de Smith AJ, Walsh KM, Francis SS, Zhang C, Hansen HM, Smirnov I, et al. BMI1 enhancer polymorphism underlies chromosome 10p12.31 association with childhood acute lymphoblastic leukemia. Int J Cancer. 2018;143:2647–58.
Cowper-Sal lari R, Zhang X, Wright JB, Bailey SD, Cole MD, Eeckhoute J, et al. Breast cancer risk-associated SNPs modulate the affinity of chromatin for FOXA1 and alter gene expression. Nat Genet. 2012;44:1191–8.
Shah MY, Ferracin M, Pileczki V, Chen B, Redis R, Fabris L, et al. Cancer-associated rs6983267 SNP and its accompanying long noncoding RNA CCAT2 induce myeloid malignancies via unique SNP-specific RNA mutations. Genome Res. 2018;28:432–47.
Glubb DM, Shi W, Beesley J, Fachal L, Pritchard J-L, McCue K, et al. Candidate Causal Variants at the 8p12 Breast Cancer Risk Locus Regulate DUSP4. Cancers (Basel). 2020;12.
McGovern A, Schoenfelder S, Martin P, Massey J, Duffus K, Plant D, et al. Capture Hi-C identifies a novel causal gene, IL20RA, in the pan-autoimmune genetic susceptibility region 6q23. Genome Biol. 2016;17:212.
Ahluwalia TS, Troelsen JT, Balslev-Harder M, Bork-Jensen J, Thuesen BH, Cerqueira C, et al. Carriers of a VEGFA enhancer polymorphism selectively binding CHOP/DDIT3 are predisposed to increased circulating levels of thyroid-stimulating hormone. J Med Genet England. 2017;54:166–75.
Spisák S, Lawrenson K, Fu Y, Csabai I, Cottman RT, Seo J-H, et al. CAUSEL: an epigenome- and genome-editing pipeline for establishing function of noncoding GWAS variants. Nat Med. 2015;21:1357–63.
Mehta ZB, Fine N, Pullen TJ, Cane MC, Hu M, Chabosseau P, et al. Changes in the expression of the type 2 diabetes-associated gene VPS13C in the β-cell are associated with glucose intolerance in humans and mice. Am J Physiol Endocrinol Metab. 2016;311:E488-507.
Prokop JW, Yeo NC, Ottmann C, Chhetri SB, Florus KL, Ross EJ, et al. Characterization of coding/noncoding variants for SHROOM3 in patients with CKD. J Am Soc Nephrol. 2018;29:1525–35.
Xia Q, Deliard S, Yuan C-X, Johnson ME, Grant SFA. Characterization of the transcriptional machinery bound across the widely presumed type 2 diabetes causal variant, rs7903146, within TCF7L2. Eur J Hum Genet. 2015;23:103–9.
Comiskey DFJ, He H, Liyanarachchi S, Sheikh MS, Hendrickson IV, Yu L, et al. Characterizing the function of EPB41L4A in the predisposition to papillary thyroid carcinoma. Sci Rep. 2020;10:19984.
Du M, Tillmans L, Gao J, Gao P, Yuan T, Dittmar RL, et al. Chromatin interactions and candidate genes at ten prostate cancer risk loci. Sci Rep. 2016;6:23202.
Matoba N, Liang D, Sun H, Aygün N, McAfee JC, Davis JE, et al. Common genetic risk variants identified in the SPARK cohort support DDHD2 as a candidate risk gene for autism. Transl Psychiatry. 2020;10:265.
Hiramoto M, Udagawa H, Watanabe A, Miyazawa K, Ishibashi N, Kawaguchi M, et al. Comparative analysis of type 2 diabetes-associated SNP alleles identifies allele-specific DNA-binding proteins for the KCNQ1 locus. Int J Mol Med Greece. 2015;36:222–30.
Hazelett DJ, Rhie SK, Gaddis M, Yan C, Lakeland DL, Coetzee SG, et al. Comprehensive functional annotation of 77 prostate cancer risk loci. PLoS Genet. 2014;10:e1004102.
Cheng M, Huang X, Zhang M, Huang Q. Computational and functional analyses of T2D GWAS SNPs for transcription factor binding. Biochem Biophys Res Commun United States. 2020;523:658–65.
Ye W, Wang Y, Mei B, Hou S, Liu X, Wu G, et al. Computational and functional characterization of four SNPs in the SOST locus associated with osteoporosis. Bone United States. 2018;108:132–44.
Clifton-Bligh RJ, Nguyen TV, Au A, Bullock M, Cameron I, Cumming R, et al. Contribution of a common variant in the promoter of the 1-α-hydroxylase gene (CYP27B1) to fracture risk in the elderly. Calcif Tissue Int. 2011;88:109–16.
Miller CL, Haas U, Diaz R, Leeper NJ, Kundu RK, Patlolla B, et al. Coronary heart disease-associated variation in TCF21 disrupts a miR-224 binding site and miRNA-mediated regulation. PLoS Genet. 2014;10:e1004263.
Gee F, Rushton MD, Loughlin J, Reynard LN. Correlation of the osteoarthritis susceptibility variants that map to chromosome 20q13 with an expression quantitative trait locus operating on NCOA3 and with functional variation at the polymorphism rs116855380. Arthritis Rheumatol. 2015;67:2923–32.
Reynard LN, Bui C, Syddall CM, Loughlin J. CpG methylation regulates allelic expression of GDF5 by modulating binding of SP1 and SP3 repressor proteins to the osteoarthritis susceptibility SNP rs143383. Hum Genet. 2014;133:1059–73.
Wu J, Yang S, Yu D, Gao W, Liu X, Zhang K, et al. CRISPR/cas9 mediated knockout of an intergenic variant rs6927172 identified IL-20RA as a new risk gene for multiple autoimmune diseases. Genes Immun England. 2019;20:103–11.
Deng Y, Zhao J, Sakurai D, Sestak AL, Osadchiy V, Langefeld CD, et al. Decreased SMG7 expression associates with lupus-risk variants and elevated antinuclear antibody production. Ann Rheum Dis. 2016;75:2007–13.
Vezzoli G, Terranegra A, Aloia A, Arcidiacono T, Milanesi L, Mosca E, et al. Decreased transcriptional activity of calcium-sensing receptor gene promoter 1 is associated with calcium nephrolithiasis. J Clin Endocrinol Metab. 2013;98:3839–47.
Ryu J, Lee C. Differential promoter activity by nucleotide substitution at a type 2 diabetes genome-wide association study signal upstream of the wolframin gene. J Diabetes Australia. 2016;8:253–9.
Smith JG, Felix JF, Morrison AC, Kalogeropoulos A, Trompet S, Wilk JB, et al. Discovery of genetic variation on chromosome 5q22 associated with mortality in heart failure. PLoS Genet. 2016;12:e1006034.
Miller CL, Anderson DR, Kundu RK, Raiesdana A, Nürnberg ST, Diaz R, et al. Disease-related growth factor and embryonic signaling pathways modulate an enhancer of TCF21 expression at the 6q23.2 coronary heart disease locus. PLoS Genet. 2013;9:e1003652.
Rahimov F, Marazita ML, Visel A, Cooper ME, Hitchler MJ, Rubini M, et al. Disruption of an AP-2alpha binding site in an IRF6 enhancer is associated with cleft lip. Nat Genet. 2008;40:1341–7.
Zhu Z, Meng W, Liu P, Zhu X, Liu Y, Zou H. DNA hypomethylation of a transcription factor binding site within the promoter of a gout risk gene NRBP1 upregulates its expression by inhibition of TFAP2A binding. Clin Epigenetics. 2017;9:99.
Wang X, Srivastava Y, Jankowski A, Malik V, Wei Y, Del Rosario RC, et al. DNA-mediated dimerization on a compact sequence signature controls enhancer engagement and regulation by FOXA1. Nucleic Acids Res. 2018;46:5470–86.
Kim BS, Park S-M, Uhm TG, Kang JH, Park J-S, Jang A-S, et al. Effect of single nucleotide polymorphisms within the interleukin-4 promoter on aspirin intolerance in asthmatics and interleukin-4 promoter activity. Pharmacogenet Genomics United States. 2010;20:748–58.
Powell JE, Fung JN, Shakhbazov K, Sapkota Y, Cloonan N, Hemani G, et al. Endometriosis risk alleles at 1p36.12 act through inverse regulation of CDC42 and LINC00339. Hum Mol Genet. 2016;25:5046–58.
Gant VU, Junco JJ, Terrell M, Rashid R, Rabin KR. Enhancer polymorphisms at the IKZF1 susceptibility locus for acute lymphoblastic leukemia impact B-cell proliferation and differentiation in both Down syndrome and non-Down syndrome genetic backgrounds. PLoS ONE. 2021;16:e0244863.
Sio YY, Matta SA, Ng YT, Chew FT. Epistasis between phenylethanolamine N-methyltransferase and β2-adrenergic receptor influences extracellular epinephrine level and associates with the susceptibility to allergic asthma. Clin Exp Allergy England. 2020;50:352–63.
Vecellio M, Cortes A, Roberts AR, Ellis J, Cohen CJ, Knight JC, et al. Evidence for a second ankylosing spondylitis-associated RUNX3 regulatory polymorphism. RMD Open. 2018;4:e000628.
Ghoussaini M, Edwards SL, Michailidou K, Nord S, Cowper-Sal Lari R, Desai K, et al. Evidence that breast cancer risk at the 2q35 locus is mediated through IGFBP5 regulation. Nat Commun. 2014;4:4999.
Shepherd C, Skelton AJ, Rushton MD, Reynard LN, Loughlin J. Expression analysis of the osteoarthritis genetic susceptibility locus mapping to an intron of the MCF2L gene and marked by the polymorphism rs11842874. BMC Med Genet. 2015;16:108.
Surgucheva I, Surguchov A. Expression of caveolin in trabecular meshwork cells and its possible implication in pathogenesis of primary open angle glaucoma. Mol Vis. 2011;17:2878–88.
CAS PubMed PubMed Central Google Scholar
Lou H, Yeager M, Li H, Bosquet JG, Hayes RB, Orr N, et al. Fine mapping and functional analysis of a common variant in MSMB on chromosome 10q11.2 associated with prostate cancer susceptibility. Proc Natl Acad Sci USA. 2009;106:7933–8.
Chang B-L, Cramer SD, Wiklund F, Isaacs SD, Stevens VL, Sun J, et al. Fine mapping association study and functional analysis implicate a SNP in MSMB at 10q11 as a causal variant for prostate cancer risk. Hum Mol Genet. 2009;18:1368–75.
Westra H-J, Martínez-Bonet M, Onengut-Gumuscu S, Lee A, Luo Y, Teslovich N, et al. Fine-mapping and functional studies highlight potential causal variants for rheumatoid arthritis and type 1 diabetes. Nat Genet. 2018;50:1366–74.
Orr N, Dudbridge F, Dryden N, Maguire S, Novo D, Perrakis E, et al. Fine-mapping identifies two additional breast cancer susceptibility loci at 9q31.2. Hum Mol Genet. 2015;24:2966–84.
Painter JN, O’Mara TA, Batra J, Cheng T, Lose FA, Dennis J, et al. Fine-mapping of the HNF1B multicancer locus identifies candidate variants that mediate endometrial cancer risk. Hum Mol Genet. 2015;24:1478–92.
Pan Y, Tian R, Lee C, Bao G, Gibson G. Fine-mapping within eQTL credible intervals by expression CROP-seq. Biol Methods Protoc. 2020;5:bpaa008.
Glubb DM, Maranian MJ, Michailidou K, Pooley KA, Meyer KB, Kar S, et al. Fine-scale mapping of the 5q11.2 breast cancer locus reveals at least three independent risk variants regulating MAP3K1. Am J Hum Genet. 2015;96:5–20.
Meyer KB, O’Reilly M, Michailidou K, Carlebur S, Edwards SL, French JD, et al. Fine-scale mapping of the FGFR2 breast cancer risk locus: putative functional variants differentially bind FOXA1 and E2F1. Am J Hum Genet. 2013;93:1046–60.
Cheng TH, Thompson DJ, O’Mara TA, Painter JN, Glubb DM, Flach S, et al. Five endometrial cancer risk loci identified through genome-wide association analysis. Nat Genet. 2016;48:667–74.
Bohaczuk SC, Thackray VG, Shen J, Skowronska-Krawczyk D, Mellon PL. FSHB Transcription is Regulated by a Novel 5’ Distal Enhancer With a Fertility-Associated Single Nucleotide Polymorphism. Endocrinology. 2021;162.
Claussnitzer M, Dankel SN, Kim K-H, Quon G, Meuleman W, Haugen C, et al. FTO obesity variant circuitry and adipocyte browning in humans. N Engl J Med. 2015;373:895–907.
Buckley MA, Woods NT, Tyrer JP, Mendoza-Fandiño G, Lawrenson K, Hazelett DJ, et al. Functional analysis and fine mapping of the 9p22.2 ovarian cancer susceptibility locus. Cancer Res. 2019;79:467–81.
Boardman-Pretty F, Smith AJP, Cooper J, Palmen J, Folkersen L, Hamsten A, et al. Functional analysis of a carotid intima-media thickness locus implicates BCAR1 and suggests a causal variant. Circ Cardiovasc Genet United States. 2015;8:696–706.
Turner AW, Martinuk A, Silva A, Lau P, Nikpay M, Eriksson P, et al. Functional analysis of a novel genome-wide association study signal in SMAD3 that confers protection from coronary artery disease. Arterioscler Thromb Vasc Biol United States. 2016;36:972–83.
Hamdi Y, Leclerc M, Dumont M, Dubois S, Tranchant M, Reimnitz G, et al. Functional analysis of promoter variants in genes involved in sex steroid action, DNA repair and cell cycle control. Genes (Basel). 2019;10.
Pang DX, Smith AJP, Humphries SE. Functional analysis of TCF7L2 genetic variants associated with type 2 diabetes. Nutr Metab Cardiovasc Dis. 2013;23:550–6.
Baskin R, Woods NT, Mendoza-Fandiño G, Forsyth P, Egan KM, Monteiro ANA. Functional analysis of the 11q23.3 glioma susceptibility locus implicates PHLDB1 and DDX6 in glioma susceptibility. Sci Rep. 2015;5:17367.
Egli RJ, Southam L, Wilkins JM, Lorenzen I, Pombo-Suarez M, Gonzalez A, et al. Functional analysis of the osteoarthritis susceptibility-associated GDF5 regulatory polymorphism. Arthritis Rheum. 2009;60:2055–64.
Douvris A, Soubeyrand S, Naing T, Martinuk A, Nikpay M, Williams A, et al. Functional analysis of the TRIB1 associated locus linked to plasma triglycerides and coronary artery disease. J Am Heart Assoc. 2014;3:e000884.
Zhang Y, Kuipers AL, Yerges-Armstrong LM, Nestlerode CS, Jin Z, Wheeler VW, et al. Functional and association analysis of frizzled 1 (FZD1) promoter haplotypes with femoral neck geometry. Bone. 2010;46:1131–7.
Fang J, Jia J, Makowski M, Xu M, Wang Z, Zhang T, et al. Functional characterization of a multi-cancer risk locus on chr5p15.33 reveals regulation of TERT by ZNF148. Nat Commun. 2017;8:15034.
Eckart N, Song Q, Yang R, Wang R, Zhu H, McCallion AS, et al. Functional characterization of schizophrenia-associated variation in CACNA1C. PLoS ONE. 2016;11:e0157086.
Flora AV, Zambrano CA, Gallego X, Miyamoto JH, Johnson KA, Cowan KA, et al. Functional characterization of SNPs in CHRNA3/B4 intergenic region associated with drug behaviors. Brain Res. 2013;1529:1–15.
Bigot P, Colli LM, Machiela MJ, Jessop L, Myers TA, Carrouget J, et al. Functional characterization of the 12p12.1 renal cancer-susceptibility locus implicates BHLHE41. Nat Commun. 2016;7:12098.
Roca-Ayats N, Martínez-Gil N, Cozar M, Gerousi M, Garcia-Giralt N, Ovejero D, et al. Functional characterization of the C7ORF76 genomic region, a prominent GWAS signal for osteoporosis in 7q21.3. Bone. 2019;123:39–47.
Kessler T, Wobst J, Wolf B, Eckhold J, Vilne B, Hollstein R, et al. Functional characterization of the GUCY1A3 coronary artery disease risk locus. Circulation. 2017;136:476–89.
Maloney B, Ge Y-W, Petersen RC, Hardy J, Rogers JT, Pérez-Tur J, et al. Functional characterization of three single-nucleotide polymorphisms present in the human APOE promoter sequence: Differential effects in neuronal cells and on DNA-protein interactions. Am J Med Genet B Neuropsychiatr Genet. 2010;153B:185–201.
Helbig S, Wockner L, Bouendeu A, Hille-Betz U, McCue K, French JD, et al. Functional dissection of breast cancer risk-associated TERT promoter variants. Oncotarget. 2017;8:67203–17.
Ge M, Shi M, An C, Yang W, Nie X, Zhang J, et al. Functional evaluation of TERT-CLPTM1L genetic variants associated with susceptibility of papillary thyroid carcinoma. Sci Rep. 2016;6:26037.
Elsby LM, Orozco G, Denton J, Worthington J, Ray DW, Donn RP. Functional evaluation of TNFAIP3 (A20) in rheumatoid arthritis. Clin Exp Rheumatol. 2010;28:708–14.
Vecellio M, Chen L, Cohen CJ, Cortes A, Li Y, Bonham S, et al. Functional genomic analysis of a RUNX3 polymorphism associated with ankylosing spondylitis. Arthritis Rheumatol United States. 2021;73:980–90.
Chang H, Cai X, Li H-J, Liu W-P, Zhao L-J, Zhang C-Y, et al. Functional genomics identify a regulatory risk variation rs4420550 in the 16p11.2 Schizophrenia-Associated Locus. Biol Psychiatry United States. 2021;89:246–55.
Guo L, Yamashita H, Kou I, Takimoto A, Meguro-Horike M, Horike S, et al. Functional Investigation of a Non-coding Variant Associated with Adolescent Idiopathic Scoliosis in Zebrafish: Elevated Expression of the Ladybird Homeobox Gene Causes Body Axis Deformation. PLoS Genet. 2016;12:e1005802.
Kong M, Kim Y, Lee C. Functional investigation of a venous thromboembolism GWAS signal in a promoter region of coagulation factor XI gene. Mol Biol Rep Netherlands. 2014;41:2015–9.
Lawrenson K, Kar S, McCue K, Kuchenbaeker K, Michailidou K, Tyrer J, et al. Functional mechanisms underlying pleiotropic risk alleles at the 19p13.1 breast-ovarian cancer susceptibility locus. Nat Commun. 2016;7:12675.
Pérez-Razo JC, Cano-Martínez LJ, Vargas Alarcón G, Canizales-Quinteros S, Martínez-Rodríguez N, Canto P, et al. Functional polymorphism rs13306560 of the MTHFR gene is associated with essential hypertension in a Mexican-Mestizo Population. Circ Cardiovasc Genet United States. 2015;8:603–9.
Nanda V, Wang T, Pjanic M, Liu B, Nguyen T, Matic LP, et al. Functional regulatory mechanism of smooth muscle cell-restricted LMOD1 coronary artery disease locus. PLoS Genet. 2018;14:e1007755.
Huang X, Zheng J, Li J, Che X, Tan W, Tan W, et al. Functional role of BTB and CNC Homology 1 gene in pancreatic cancer and its association with survival in patients treated with gemcitabine. Theranostics. 2018;8:3366–79.
Ustiugova AS, Korneev KV, Kuprash DV, Afanasyeva AMA. Functional SNPs in the Human Autoimmunity-Associated Locus 17q12–21. Genes (Basel). 2019;10.
Klein JC, Keith A, Rice SJ, Shepherd C, Agarwal V, Loughlin J, et al. Functional testing of thousands of osteoarthritis-associated variants for regulatory activity. Nat Commun. 2019;10:2434.
Yu W, Zhang K, Wang Z, Zhang J, Chen T, Jin L. Functional variant in the promoter region of IL-27 alters gene transcription and confers a risk for ulcerative colitis in northern Chinese Han. Hum Immunol United States. 2017;78:287–93.
French JD, Ghoussaini M, Edwards SL, Meyer KB, Michailidou K, Ahmed S, et al. Functional variants at the 11q13 risk locus for breast cancer regulate cyclin D1 expression through long-range enhancers. Am J Hum Genet. 2013;92:489–503.
Andiappan AK, Sio YY, Lee B, Suri BK, Matta SA, Lum J, et al. Functional variants of 17q12-21 are associated with allergic asthma but not allergic rhinitis. J Allergy Clin Immunol United States. 2016;137:758-766.e3.
Li Y, Nie Y, Cao J, Tu S, Lin Y, Du Y, et al. G-A variant in miR-200c binding site of EFNA1 alters susceptibility to gastric cancer. Mol Carcinog United States. 2014;53:219–29.
Gaulton KJ, Ferreira T, Lee Y, Raimondo A, Mägi R, Reschen ME, et al. Genetic fine mapping and genomic annotation defines causal mechanisms at type 2 diabetes susceptibility loci. Nat Genet. 2015;47:1415–25.
Liu S, Wu N, Zuo Y, Zhou Y, Liu J, Liu Z, et al. Genetic Polymorphism of LBX1 Is Associated With Adolescent Idiopathic Scoliosis in Northern Chinese Han Population. Spine (Phila Pa 1976). United States; 2017;42:1125–9.
Oldridge DA, Wood AC, Weichert-Leahey N, Crimmins I, Sussman R, Winter C, et al. Genetic predisposition to neuroblastoma mediated by a LMO1 super-enhancer polymorphism. Nature. 2015;528:418–21.
Cavalli M, Pan G, Nord H, Wallén Arzt E, Wallerman O, Wadelius C. Genetic prevention of hepatitis C virus-induced liver fibrosis by allele-specific downregulation of MERTK. Hepatol Res Netherlands. 2017;47:826–30.
Krause MD, Huang R-T, Wu D, Shentu T-P, Harrison DL, Whalen MB, et al. Genetic variant at coronary artery disease and ischemic stroke locus 1p32.2 regulates endothelial responses to hemodynamics. Proc Natl Acad Sci USA. 2018;115:E11349–58.
Soderquest K, Hertweck A, Giambartolomei C, Henderson S, Mohamed R, Goldberg R, et al. Genetic variants alter T-bet binding and gene expression in mucosal inflammatory disease. PLoS Genet. 2017;13:e1006587.
Wu C, Hu Z, Yu D, Huang L, Jin G, Liang J, et al. Genetic variants on chromosome 15q25 associated with lung cancer risk in Chinese populations. Cancer Res United States. 2009;69:5065–72.
Bernstein DI, Lummus ZL, Kesavalu B, Yao J, Kottyan L, Miller D, et al. Genetic variants with gene regulatory effects are associated with diisocyanate-induced asthma. J Allergy Clin Immunol United States. 2018;142:959–69.
Bamji-Mirza M, Li Y, Najem D, Liu QY, Walker D, Lue L-F, et al. Genetic Variations in ABCA7 Can Increase Secreted Levels of Amyloid-β40 and Amyloid-β42 Peptides and ABCA7 Transcription in Cell Culture Models. J Alzheimers Dis Netherlands. 2016;53:875–92.
Keller M, Gebhardt C, Huth S, Schleinitz D, Heyne H, Scholz M, et al. Genetically programmed changes in transcription of the novel progranulin regulator. J Mol Med (Berl). 2020;98:1139–48.
Hou S, Du L, Lei B, Pang CP, Zhang M, Zhuang W, et al. Genome-wide association analysis of Vogt-Koyanagi-Harada syndrome identifies two new susceptibility loci at 1p31.2 and 10q21.3. Nat Genet. 2014;46:1007–11.
Kawamura R, Tabara Y, Tsukada A, Igase M, Ohashi J, Yamada R, et al. Genome-wide association study of plasma resistin levels identified rs1423096 and rs10401670 as possible functional variants in the Japanese population. Physiol Genomics United States. 2016;48:874–81.
Stitzel ML, Sethupathy P, Pearson DS, Chines PS, Song L, Erdos MR, et al. Global epigenomic analysis of primary human pancreatic islets provides insights into type 2 diabetes susceptibility loci. Cell Metab. 2010;12:443–55.
Kalita CA, Brown CD, Freiman A, Isherwood J, Wen X, Pique-Regi R, et al. High-throughput characterization of genetic effects on DNA-protein binding and gene transcription. Genome Res. 2018;28:1701–8.
Zhou Y, Oskolkov N, Shcherbina L, Ratti J, Kock K-H, Su J, et al. HMGB1 binds to the rs7903146 locus in TCF7L2 in human pancreatic islets. Mol Cell Endocrinol Ireland. 2016;430:138–45.
Ross-Adams H, Ball S, Lawrenson K, Halim S, Russell R, Wells C, et al. HNF1B variants associate with promoter methylation and regulate gene networks activated in prostate and ovarian cancer. Oncotarget. 2016;7:74734–46.
Smith EN, D’Antonio-Chronowska A, Greenwald WW, Borja V, Aguiar LR, Pogue R, et al. Human iPSC-derived retinal pigment epithelium: a model system for prioritizing and functionally characterizing causal variants at AMD risk loci. Stem Cell Reports. 2019;12:1342–53.
Hitomi Y, Kawashima M, Aiba Y, Nishida N, Matsuhashi M, Okazaki H, et al. Human primary biliary cirrhosis-susceptible allele of rs4979462 enhances TNFSF15 expression by binding NF-1. Hum Genet Germany. 2015;134:737–47.
López Rodríguez M, Kaminska D, Lappalainen K, Pihlajamäki J, Kaikkonen MU, Laakso M. Identification and characterization of a FOXA2-regulated transcriptional enhancer at a type 2 diabetes intronic locus that controls GCKR expression in liver cells. Genome Med. 2017;9:63.
Biancolella M, Fortini BK, Tring S, Plummer SJ, Mendoza-Fandino GA, Hartiala J, et al. Identification and characterization of functional risk variants for colorectal cancer mapping to chromosome 11q23.1. Hum Mol Genet. 2014;23:2198–209.
Flachsbart F, Dose J, Gentschew L, Geismann C, Caliebe A, Knecht C, et al. Identification and characterization of two functional variants in the human longevity gene FOXO3. Nat Commun. 2017;8:2063.
Spracklen CN, Shi J, Vadlamudi S, Wu Y, Zou M, Raulerson CK, et al. Identification and functional analysis of glycemic trait loci in the China Health and Nutrition Survey. PLoS Genet. 2018;14:e1007275.
Liu L, Pei Y-F, Liu T-L, Hu W-Z, Yang X-L, Li S-C, et al. Identification of a 1p21 independent functional variant for abdominal obesity. Int J Obes (Lond). 2019;43:2480–90.
Zhou X, Baron RM, Hardin M, Cho MH, Zielinski J, Hawrylkiewicz I, et al. Identification of a chronic obstructive pulmonary disease genetic determinant that regulates HHIP. Hum Mol Genet. 2012;21:1325–35.
Boulling A, Masson E, Zou W-B, Paliwal S, Wu H, Issarapu P, et al. Identification of a functional enhancer variant within the chronic pancreatitis-associated SPINK1 c.101A>G (p.Asn34Ser)-containing haplotype. Hum Mutat. 2017;38:1014–24.
Ke J, Tian J, Li J, Gong Y, Yang Y, Zhu Y, et al. Identification of a functional polymorphism affecting microRNA binding in the susceptibility locus 1q25.3 for colorectal cancer. Mol Carcinog. 2017;56:2014–21.
Alcina A, Fedetz M, Fernández O, Saiz A, Izquierdo G, Lucas M, et al. Identification of a functional variant in the KIF5A-CYP27B1-METTL1-FAM119B locus associated with multiple sclerosis. J Med Genet. 2013;50:25–33.
Lo PHY, Urabe Y, Kumar V, Tanikawa C, Koike K, Kato N, et al. Identification of a functional variant in the MICA promoter which regulates MICA expression and increases HCV-related hepatocellular carcinoma risk. PLoS ONE. 2013;8:e61279.
Ke J, Lou J, Chen X, Li J, Liu C, Gong Y, et al. Identification of a potential regulatory variant for colorectal cancer risk mapping to chromosome 5q31.1: A Post-GWAS Study. PLoS ONE. 2015;10:e0138478.
Fogarty MP, Cannon ME, Vadlamudi S, Gaulton KJ, Mohlke KL. Identification of a regulatory variant that binds FOXA1 and FOXA2 at the CDC123/CAMK1D type 2 diabetes GWAS locus. PLoS Genet. 2014;10:e1004633.
Parker MM, Hao Y, Guo F, Pham B, Chase R, Platig J, et al. Identification of an emphysema-associated genetic variant near TGFB2 with regulatory effects in lung fibroblasts. Elife. 2019;8.
Ryoo H, Kong M, Kim Y, Lee C. Identification of functional nucleotide and haplotype variants in the promoter of the CEBPE gene. J Hum Genet England. 2013;58:600–3.
van Ouwerkerk AF, Bosada FM, Liu J, Zhang J, van Duijvenboden K, Chaffin M, et al. Identification of functional variant enhancers associated with atrial fibrillation. Circ Res United States. 2020;127:229–43.
Castaldi PJ, Guo F, Qiao D, Du F, Naing ZZC, Li Y, et al. Identification of functional variants in the FAM13A chronic obstructive pulmonary disease genome-wide Association Study Locus by Massively Parallel Reporter Assays. Am J Respir Crit Care Med. 2019;199:52–61.
Bai W-Y, Wang L, Ying Z-M, Hu B, Xu L, Zhang G-Q, et al. Identification of PIEZO1 polymorphisms for human bone mineral density. Bone. 2020;133:115247.
Fairoozy RH, White J, Palmen J, Kalea AZ, Humphries SE. Identification of the functional variant(s) that explain the low-density lipoprotein receptor (LDLR) GWAS SNP rs6511720 association with lower LDL-C and risk of CHD. PLoS ONE. 2016;11:e0167676.
Guo X, Lin W, Wen W, Huyghe J, Bien S, Cai Q, et al. Identifying novel susceptibility genes for colorectal cancer risk from a transcriptome-wide association study of 125,478 subjects. Gastroenterology. 2021;160:1164-1178.e6.
Amlie-Wolf A, Tang M, Way J, Dombroski B, Jiang M, Vrettos N, et al. Inferring the molecular mechanisms of noncoding Alzheimer’s disease-associated genetic variants. J Alzheimers Dis. 2019;72:301–18.
Hamadou I, Garritano S, Romanel A, Naimi D, Hammada T, Demichelis F. Inherited variant in NFκB-1 promoter is associated with increased risk of IBD in an Algerian population and modulates SOX9 binding. Cancer Rep (Hoboken). 2020;3:e1240.
Pan DZ, Garske KM, Alvarez M, Bhagat YV, Boocock J, Nikkola E, et al. Integration of human adipocyte chromosomal interactions with adipose gene expression prioritizes obesity-related genes from GWAS. Nat Commun. 2018;9:1512.
Zhang X, Cowper-Sal lari R, Bailey SD, Moore JH, Lupien M. Integrative functional genomics identifies an enhancer looping to the SOX9 gene disrupted by the 17q24.3 prostate cancer risk locus. Genome Res. 2012;22:1437–46.
Miller CL, Pjanic M, Wang T, Nguyen T, Cohain A, Lee JD, et al. Integrative functional genomics identifies regulatory mechanisms at coronary artery disease loci. Nat Commun. 2016;7:12092.
Zhang Y, Manjunath M, Zhang S, Chasman D, Roy S, Song JS. Integrative genomic analysis predicts causative Cis-regulatory mechanisms of the Breast Cancer-Associated Genetic Variant rs4415084. Cancer Res. 2018;78:1579–91.
Berlivet S, Moussette S, Ouimet M, Verlaan DJ, Koka V, Al Tuwaijri A, et al. Interaction between genetic and epigenetic variation defines gene expression patterns at the asthma-associated locus 17q12-q21 in lymphoblastoid cell lines. Hum Genet. 2012;131:1161–71.
Wang X, Raghavan A, Peters DT, Pashos EE, Rader DJ, Musunuru K. Interrogation of the atherosclerosis-associated SORT1 (Sortilin 1) locus with primary human hepatocytes, induced pluripotent stem cell-hepatocytes, and locus-humanized mice. Arterioscler Thromb Vasc Biol. 2018;38:76–82.
Hammaker D, Whitaker JW, Maeshima K, Boyle DL, Ekwall A-KH, Wang W, et al. LBH Gene Transcription Regulation by the Interplay of an Enhancer Risk Allele and DNA Methylation in Rheumatoid Arthritis. Arthritis Rheumatol. 2016;68:2637–45.
Reschen ME, Gaulton KJ, Lin D, Soilleux EJ, Morris AJ, Smyth SS, et al. Lipid-induced epigenomic changes in human macrophages identify a coronary artery disease-associated variant that regulates PPAP2B Expression through Altered C/EBP-beta binding. PLoS Genet. 2015;11:e1005061.
Zhang Y, Chen X-F, Li J, He F, Li X, Guo Y. lncRNA Neat1 stimulates osteoclastogenesis via sponging miR-7. J Bone Miner Res. 2020;35:1772–81.
Mei B, Wang Y, Ye W, Huang H, Zhou Q, Chen Y, et al. LncRNA ZBTB40-IT1 modulated by osteoporosis GWAS risk SNPs suppresses osteogenesis. Hum Genet. 2019;138:151–66.
Vicente CT, Edwards SL, Hillman KM, Kaufmann S, Mitchell H, Bain L, et al. Long-range modulation of PAG1 expression by 8q21 allergy risk variants. Am J Hum Genet. 2015;97:329–36.
Cavalli M, Pan G, Nord H, Wadelius C. Looking beyond GWAS: allele-specific transcription factor binding drives the association of GALNT2 to HDL-C plasma levels. Lipids Health Dis. 2016;15:18.
Lu X, Zoller EE, Weirauch MT, Wu Z, Namjou B, Williams AH, et al. Lupus risk variant increases pSTAT1 binding and decreases ETS1 expression. Am J Hum Genet. 2015;96:731–9.
Choi J, Zhang T, Vu A, Ablain J, Makowski MM, Colli LM, et al. Massively parallel reporter assays of melanoma risk variants identify MX2 as a gene promoting melanoma. Nat Commun. 2020;11:2718.
Elek Z, Németh N, Nagy G, Németh H, Somogyi A, Hosszufalusi N, et al. Micro-RNA binding site polymorphisms in the WFS1 gene are risk factors of diabetes mellitus. PLoS ONE. 2015;10:e0139519.
Rong H, Gu S, Zhang G, Kang L, Yang M, Zhang J, et al. MiR-2964a-5p binding site SNP regulates ATM expression contributing to age-related cataract risk. Oncotarget. 2017;8:84945–57.
Elek Z, Dénes R, Prokop S, Somogyi A, Yowanto H, Luo J, et al. Multicapillary gel electrophoresis based analysis of genetic variants in the WFS1 gene. Electrophoresis. 2016;37:2313–21.
Zhu D-L, Chen X-F, Hu W-X, Dong S-S, Lu B-J, Rong Y, et al. Multiple functional variants at 13q14 risk locus for osteoporosis regulate RANKL expression through long-range super-enhancer. J Bone Miner Res. 2018;33:1335–46.
He H, Li W, Liyanarachchi S, Srinivas M, Wang Y, Akagi K, et al. Multiple functional variants in long-range enhancer elements contribute to the risk of SNP rs965513 in thyroid cancer. Proc Natl Acad Sci U S A. 2015;112:6128–33.
Roman TS, Marvelle AF, Fogarty MP, Vadlamudi S, Gonzalez AJ, Buchkovich ML, et al. Multiple hepatic regulatory variants at the GALNT2 GWAS locus associated with high-density lipoprotein cholesterol. Am J Hum Genet. 2015;97:801–15.
Bojesen SE, Pooley KA, Johnatty SE, Beesley J, Michailidou K, Tyrer JP, et al. Multiple independent variants at the TERT locus are associated with telomere length and risks of breast and ovarian cancer. Nat Genet. 2013;45:371–84, 384e1–2.
Beaudoin M, Gupta RM, Won H-H, Lo KS, Do R, Henderson CA, et al. Myocardial infarction-associated SNP at 6p24 interferes with MEF2 binding and associates with PHACTR1 expression levels in human coronary arteries. Arterioscler Thromb Vasc Biol. 2015;35:1472–9.
John G, Hegarty JP, Yu W, Berg A, Pastor DM, Kelly AA, et al. NKX2-3 variant rs11190140 is associated with IBD and alters binding of NFAT. Mol Genet Metab United States. 2011;104:174–9.
Bailey SD, Desai K, Kron KJ, Mazrooei P, Sinnott-Armstrong NA, Treloar AE, et al. Noncoding somatic and inherited single-nucleotide variants converge to promote ESR1 expression in breast cancer. Nat Genet. 2016;48:1260–6.
Gorbatenko A, Olesen CW, Loebl N, Sigurdsson HH, Bianchi C, Pedraz-Cuesta E, et al. Oncogenic p95HER2 regulates Na+-HCO3- cotransporter NBCn1 mRNA stability in breast cancer cells via 3’UTR-dependent processes. Biochem J England. 2016;473:4027–44.
Wang Y, Ye W, Liu Y, Mei B, Liu X, Huang Q. Osteoporosis genome-wide association study variant c.3781 C>A is regulated by a novel anti-osteogenic factor miR-345–5p. Hum Mutat. 2020;41:709–18.
Zheng J, Huang X, Tan W, Yu D, Du Z, Chang J, et al. Pancreatic cancer risk variant in LINC00673 creates a miR-1231 binding site and interferes with PTPN11 degradation. Nat Genet. 2016;48:747–57.
Soldner F, Stelzer Y, Shivalila CS, Abraham BJ, Latourelle JC, Barrasa MI, et al. Parkinson-associated risk variant in distal enhancer of α-synuclein modulates target gene expression. Nature. 2016;533:95–9.
Schedel M, Michel S, Gaertner VD, Toncheva AA, Depner M, Binia A, et al. Polymorphisms related to ORMDL3 are associated with asthma susceptibility, alterations in transcriptional regulation of ORMDL3, and changes in TH2 cytokine levels. J Allergy Clin Immunol. 2015;136:893-903.e14.
Yang C, Stueve TR, Yan C, Rhie SK, Mullen DJ, Luo J, et al. Positional integration of lung adenocarcinoma susceptibility loci with primary human alveolar epithelial cell epigenomes. Epigenomics. 2018;10:1167–87.
Oldoni F, Palmen J, Giambartolomei C, Howard P, Drenos F, Plagnol V, et al. Post-GWAS methodologies for localisation of functional non-coding variants: ANGPTL3. Atherosclerosis. 2016;246:193–201.
Sakurai D, Zhao J, Deng Y, Kelly JA, Brown EE, Harley JB, et al. Preferential binding to Elk-1 by SLE-associated IL10 risk allele upregulates IL10 expression. PLoS Genet. 2013;9:e1003870.
Padhy B, Hayat B, Nanda GG, Mohanty PP, Alone DP. Pseudoexfoliation and Alzheimer’s associated CLU risk variant, rs2279590, lies within an enhancer element and regulates CLU, EPHX2 and PTK2B gene expression. Hum Mol Genet. 2017;26:4519–29.
Bu H, Narisu N, Schlick B, Rainer J, Manke T, Schäfer G, et al. Putative prostate cancer risk SNP in an androgen receptor-binding site of the melanophilin gene illustrates enrichment of risk snps in androgen receptor target sites. Hum Mutat. 2016;37:52–64.
Jones SA, Cantsilieris S, Fan H, Cheng Q, Russ BE, Tucker EJ, et al. Rare variants in non-coding regulatory regions of the genome that affect gene expression in systemic lupus erythematosus. Sci Rep. 2019;9:15433.
Richard AC, Peters JE, Savinykh N, Lee JC, Hawley ET, Meylan F, et al. Reduced monocyte and macrophage TNFSF15/TL1A expression is associated with susceptibility to inflammatory bowel disease. PLoS Genet. 2018;14:e1007458.
Cardinale CJ, March ME, Lin X, Liu Y, Spruce LA, Bradfield JP, et al. Regulation of Janus kinase 2 by an inflammatory bowel disease causal non-coding single nucleotide polymorphism. J Crohns Colitis England. 2020;14:646–53.
Qin L, Tiwari AK, Zai CC, Freeman N, Zhai D, Liu F, et al. Regulation of melanocortin-4-receptor (MC4R) expression by SNP rs17066842 is dependent on glucose concentration. Eur Neuropsychopharmacol Netherlands. 2020;37:39–48.
Helling BA, Gerber AN, Kadiyala V, Sasse SK, Pedersen BS, Sparks L, et al. Regulation of MUC5B expression in idiopathic pulmonary fibrosis. Am J Respir Cell Mol Biol. 2017;57:91–9.
Reinisalo M, Putula J, Mannermaa E, Urtti A, Honkakoski P. Regulation of the human tyrosinase gene in retinal pigment epithelium cells: the significance of transcription factor orthodenticle homeobox 2 and its polymorphic binding site. Mol Vis. 2012;18:38–54.
Du M, Zheng R, Ma G, Chu H, Lu J, Li S, et al. Remote modulation of lncRNA GCLET by risk variant at 16p13 underlying genetic susceptibility to gastric cancer. Sci Adv. 2020;6:eaay5525.
Pasula S, Tessneer KL, Fu Y, Gopalakrishnan J, Pelikan RC, Kelly JA, et al. Role of systemic lupus erythematosus risk variants with opposing functional effects as a driver of hypomorphic expression of TNIP1 and other genes within a three-dimensional chromatin network. Arthritis Rheumatol. 2020;72:780–90.
Yang Y-C, Fu W-P, Zhang J, Zhong L, Cai S-X, Sun C. rs401681 and rs402710 confer lung cancer susceptibility by regulating TERT expression instead of CLPTM1L in East Asian populations. Carcinogenesis England. 2018;39:1216–21.
Pan G, Cavalli M, Carlsson B, Skrtic S, Kumar C, Wadelius C. rs953413 Regulates polyunsaturated fatty acid metabolism by modulating ELOVL2 expression. iScience. 2020;23:100808.
Nanda GG, Kumar MV, Pradhan L, Padhy B, Sundaray S, Das S, et al. rs4246215 is targeted by hsa-miR1236 to regulate FEN1 expression but is not associated with Fuchs’ endothelial corneal dystrophy. PLoS ONE. 2018;13:e0204278.
Hauberg ME, Holm-Nielsen MH, Mattheisen M, Askou AL, Grove J, Børglum AD, et al. Schizophrenia risk variants affecting microRNA function and site-specific regulation of NT5C2 by miR-206. Eur Neuropsychopharmacol. 2016;26:1522–6.
Hou Y, Liang W, Zhang J, Li Q, Ou H, Wang Z, et al. Schizophrenia-associated rs4702 G allele-specific downregulation of FURIN expression by miR-338-3p reduces BDNF production. Schizophr Res. 2018;199:176–80.
Guillen-Guio B, Lorenzo-Salazar JM, Ma S-F, Hou P-C, Hernandez-Beeftink T, Corrales A, et al. Sepsis-associated acute respiratory distress syndrome in individuals of European ancestry: a genome-wide association study. Lancet Respir Med. 2020;8:258–66.
Xiao F, Zhang P, Wang Y, Tian Y, James M, Huang C-C, et al. Single-nucleotide polymorphism rs13426236 contributes to an increased prostate cancer risk via regulating MLPH splicing variant 4. Mol Carcinog. 2020;59:45–55.
Hou G, Harley ITW, Lu X, Zhou T, Xu N, Yao C, et al. SLE non-coding genetic risk variant determines the epigenetic dysfunction of an immune cell specific enhancer that controls disease-critical microRNA expression. Nat Commun. 2021;12:135.
Fortini BK, Tring S, Devall MA, Ali MW, Plummer SJ, Casey G. SNPs associated with colorectal cancer at 15q13.3 affect risk enhancers that modulate GREM1 gene expression. Hum Mutat. 2021;42:237–45.
Liu S, Liu Y, Zhang Q, Wu J, Liang J, Yu S, et al. Systematic identification of regulatory variants associated with cancer risk. Genome Biol. 2017;18:194.
Kong X, Sawalha AH. Takayasu arteritis risk locus in IL6 represses the anti-inflammatory gene GPNMB through chromatin looping and recruiting MEF2-HDAC complex. Ann Rheum Dis. 2019;78:1388–97.
Wang S, Wen F, Tessneer KL, Gaffney PM. TALEN-mediated enhancer knockout influences TNFAIP3 gene expression and mimics a molecular phenotype associated with systemic lupus erythematosus. Genes Immun. 2016;17:165–70.
Wei R, Cao L, Pu H, Wang H, Zheng Y, Niu X, et al. TERT Polymorphism rs2736100-C is associated with EGFR mutation-positive non-small cell lung cancer. Clin Cancer Res. 2015;21:5173–80.
Sheng X, Tong N, Tao G, Luo D, Wang M, Fang Y, et al. TERT polymorphisms modify the risk of acute lymphoblastic leukemia in Chinese children. Carcinogenesis England. 2013;34:228–35.
Lubbe SJ, Pittman AM, Olver B, Lloyd A, Vijayakrishnan J, Naranjo S, et al. The 14q22.2 colorectal cancer variant rs4444235 shows cis-acting regulation of BMP4. Oncogene. 2012;31:3777–84.
Ghanbari M, Sedaghat S, de Looper HWJ, Hofman A, Erkeland SJ, Franco OH, et al. The association of common polymorphisms in miR-196a2 with waist to hip ratio and miR-1908 with serum lipid and glucose. Obesity (Silver Spring); 2015;23:495–503.
Prestel M, Prell-Schicker C, Webb T, Malik R, Lindner B, Ziesch N, et al. The atherosclerosis risk variant rs2107595 mediates allele-specific transcriptional regulation of HDAC9 via E2F3 and Rb1. Stroke United States. 2019;50:2651–60.
Tuupanen S, Turunen M, Lehtonen R, Hallikas O, Vanharanta S, Kivioja T, et al. The common colorectal cancer predisposition SNP rs6983267 at chromosome 8q24 confers potential to enhanced Wnt signaling. Nat Genet. 2009;41:885–90.
Matthews SM, Eshelman MA, Berg AS, Koltun WA, Yochum GS. The Crohn’s disease associated SNP rs6651252 impacts MYC gene expression in human colonic epithelial cells. PLoS ONE. 2019;14:e0212850.
Li D, Zhu G, Lou S, Ma L, Zhang C, Pan Y, et al. The functional variant of NTN1 contributes to the risk of nonsyndromic cleft lip with or without cleft palate. Eur J Hum Genet. 2020;28:453–60.
Vecellio M, Roberts AR, Cohen CJ, Cortes A, Knight JC, Bowness P, et al. The genetic association of RUNX3 with ankylosing spondylitis can be explained by allele-specific effects on IRF4 recruitment that alter gene expression. Ann Rheum Dis. 2016;75:1534–40.
Deng Y, Li P, Liu W, Pu R, Yang F, Song J, et al. The genetic polymorphism down-regulating HLA-DRB1 enhancer activity facilitates HBV persistence, evolution and hepatocarcinogenesis in the Chinese Han population. J Viral Hepat England. 2020;27:1150–61.
Yang S, Gao Y, Liu G, Li J, Shi K, Du B, et al. The human ATF1 rs11169571 polymorphism increases essential hypertension risk through modifying miRNA binding. FEBS Lett England. 2015;589:2087–93.
Li C, Yu Q, Han L, Wang C, Chu N, Liu S. The hURAT1 rs559946 polymorphism and the incidence of gout in Han Chinese men. Scand J Rheumatol. 2014;43:35–42.
Wang L, Li H, Yang B, Guo L, Han X, Li L, et al. The hypertension risk variant Rs820430 functions as an enhancer of SLC4A7. Am J Hypertens. 2017;30:202–8.
Syddall CM, Reynard LN, Young DA, Loughlin J. The identification of trans-acting factors that regulate the expression of GDF5 via the osteoarthritis susceptibility SNP rs143383. PLoS Genet. 2013;9:e1003557.
Zhou L, Fu G, Wei J, Shi J, Pan W, Ren Y, et al. The identification of two regulatory ESCC susceptibility genetic variants in the TERT-CLPTM1L loci. Oncotarget. 2016;7:5495–506.
Shao L, Zuo X, Yang Y, Zhang Y, Yang N, Shen B, et al. The inherited variations of a p53-responsive enhancer in 13q12.12 confer lung cancer risk by attenuating TNFRSF19 expression. Genome Biol. 2019;20:103.
Tuo XM, Zhu DL, Chen XF, Rong Y, Guo Y, Yang TL. The osteoporosis susceptible SNP rs4325274 remotely regulates the SOX6 gene through enhancers. Yi Chuan China. 2020;42:889–97.
Richardson K, Louie-Gao Q, Arnett DK, Parnell LD, Lai C-Q, Davalos A, et al. The PLIN4 variant rs8887 modulates obesity related phenotypes in humans through creation of a novel miR-522 seed site. PLoS ONE. 2011;6:e17944.
Jendrzejewski J, He H, Radomska HS, Li W, Tomsic J, Liyanarachchi S, et al. The polymorphism rs944289 predisposes to papillary thyroid carcinoma through a large intergenic noncoding RNA gene of tumor suppressor type. Proc Natl Acad Sci USA. 2012;109:8646–51.
Kong HK, Yoon S, Park JH. The regulatory mechanism of the LY6K gene expression in human breast cancer cells. J Biol Chem. 2012;287:38889–900.
Wang Y, He H, Liyanarachchi S, Genutis LK, Li W, Yu L, et al. The role of SMAD3 in the genetic predisposition to papillary thyroid carcinoma. Genet Med. 2018;20:927–35.
Afanasyeva MA, Putlyaeva LV, Demin DE, Kulakovskiy IV, Vorontsov IE, Fridman MV, et al. The single nucleotide variant rs12722489 determines differential estrogen receptor binding and enhancer properties of an IL2RA intronic region. PLoS ONE. 2017;12:e0172681.
Xia Q, Chesi A, Manduchi E, Johnston BT, Lu S, Leonard ME, et al. The type 2 diabetes presumed causal variant within TCF7L2 resides in an element that controls the expression of ACSL5. Diabetologia Germany. 2016;59:2360–8.
Mellado-Gil JM, Fuente-Martín E, Lorenzo PI, Cobo-Vuilleumier N, López-Noriega L, Martín-Montalvo A, et al. The type 2 diabetes-associated HMG20A gene is mandatory for islet beta cell functional maturity. Cell Death Dis. 2018;9:279.
Kamens HM, Miyamoto J, Powers MS, Ro K, Soto M, Cox R, et al. The β3 subunit of the nicotinic acetylcholine receptor: modulation of gene expression and nicotine consumption. Neuropharmacology. 2015;99:639–49.
Pattison JM, Posternak V, Cole MD. Transcription factor KLF5 binds a cyclin E1 polymorphic intronic enhancer to confer increased bladder cancer risk. Mol Cancer Res. 2016;14:1078–86.
Ding C, Zhang C, Kopp R, Kuney L, Meng Q, Wang L, et al. Transcription factor POU3F2 regulates TRIM8 expression contributing to cellular functions implicated in schizophrenia. Mol Psychiatry. 2020;
Liu W, Anstee QM, Wang X, Gawrieh S, Gamazon ER, Athinarayanan S, et al. Transcriptional regulation of PNPLA3 and its impact on susceptibility to nonalcoholic fatty liver Disease (NAFLD) in humans. Aging (Albany NY). 2016;9:26–40.
Guthridge JM, Lu R, Sun H, Sun C, Wiley GB, Dominguez N, et al. Two functional lupus-associated BLK promoter variants control cell-type- and developmental-stage-specific transcription. Am J Hum Genet. 2014;94:586–98.
Liu L, Yang X-L, Zhang H, Zhang Z-J, Wei X-T, Feng G-J, et al. Two novel pleiotropic loci associated with osteoporosis and abdominal obesity. Hum Genet. 2020;139:1023–35.
Lewis MJ, Vyse S, Shields AM, Boeltz S, Gordon PA, Spector TD, et al. UBE2L3 polymorphism amplifies NF-κB activation and promotes plasma cell development, linking linear ubiquitination to multiple autoimmune diseases. Am J Hum Genet. 2015;96:221–34.
Dryden NH, Broome LR, Dudbridge F, Johnson N, Orr N, Schoenfelder S, et al. Unbiased analysis of potential targets of breast cancer susceptibility loci by Capture Hi-C. Genome Res. 2014;24:1854–68.
Wright JB, Brown SJ, Cole MD. Upregulation of c-MYC in cis through a large chromatin loop linked to a cancer risk-associated single-nucleotide polymorphism in colorectal cancer cells. Mol Cell Biol. 2010;30:1411–20.
Smith AJP, Howard P, Shah S, Eriksson P, Stender S, Giambartolomei C, et al. Use of allele-specific FAIRE to determine functional regulatory polymorphism using large-scale genotyping arrays. PLoS Genet. 2012;8:e1002908.
Wang X, Hayes JE, Xu X, Gao X, Mehta D, Lilja HG, et al. Validation of prostate cancer risk variants rs10993994 and rs7098889 by CRISPR/Cas9 mediated genome editing. Gene. 2021;768:145265.
Sribudiani Y, Metzger M, Osinga J, Rey A, Burns AJ, Thapar N, et al. Variants in RET associated with Hirschsprung’s disease affect binding of transcription factors and gene expression. Gastroenterology. 2011;140:572-582.e2.
Vincentz JW, Firulli BA, Toolan KP, Arking DE, Sotoodehnia N, Wan J, et al. Variation in a left ventricle-specific Hand1 enhancer impairs GATA transcription factor binding and disrupts conduction system development and function. Circ Res. 2019;125:575–89.
Shirts BH, Howard MT, Hasstedt SJ, Nanjee MN, Knight S, Carlquist JF, et al. Vitamin D dependent effects of APOA5 polymorphisms on HDL cholesterol. Atherosclerosis. 2012;222:167–74.
Chen G, Ribeiro CMP, Sun L, Okuda K, Kato T, Gilmore RC, et al. XBP1S regulates MUC5B in a promoter variant-dependent pathway in idiopathic pulmonary fibrosis airway epithelia. Am J Respir Crit Care Med. 2019;200:220–34.
Mizuta I, Takafuji K, Ando Y, Satake W, Kanagawa M, Kobayashi K, et al. YY1 binds to α-synuclein 3’-flanking region SNP and stimulates antisense noncoding RNA expression. J Hum Genet England. 2013;58:711–9.
Cano-Gamez E, Trynka G. From GWAS to Function: Using Functional Genomics to Identify the Mechanisms Underlying Complex Diseases. Front Genet [Internet]. 2020 [cited 2020 Jun 8];11. Available from: https://www.ncbi.nlm.nih.gov/pmc/articles/PMC7237642/
Edwards SL, Beesley J, French JD, Dunning AM. Beyond GWASs: illuminating the dark road from association to function. Am J Human Genet. 2013;93:779–97.
Bulik-Sullivan BK, Loh P-R, Finucane H, Ripke S, Yang J, Patterson N, et al. LD score regression distinguishes confounding from polygenicity in genome-wide association studies. Nat Genet. 2015;47:291–5.
Heritability of >4,000 traits & disorders in UK Biobank [Internet]. [cited 2022 Feb 4]. Available from: https://nealelab.github.io/UKBB_ldsc/
Perenthaler E, Yousefi S, Niggl E, Barakat TS. Beyond the Exome: The Non-coding Genome and Enhancers in Neurodevelopmental Disorders and Malformations of Cortical Development. Front Cell Neurosci [Internet]. Frontiers; 2019 [cited 2021 Jun 10];13. Available from: https://doi.org/10.3389/fncel.2019.00352/full
French JD, Edwards SL. The role of noncoding variants in heritable disease. Trends Genet. 2020;36:880–91.
Rojano E, Seoane P, Ranea JAG, Perkins JR. Regulatory variants: from detection to predicting impact. Brief Bioinform. 2019;20:1639–54.
Moore LD, Le T, Fan G. DNA methylation and its basic function. Neuropsychopharmacology. 2013;38:23–38.
Lowdon RF, Jang HS, Wang T. Evolution of epigenetic regulation in vertebrate genomes. Trends Genet. 2016;32:269–83.
Zhang P, Wu W, Chen Q, Chen M. Non-Coding RNAs and their Integrated Networks. J Integr Bioinform [Internet]. 2019 [cited 2021 May 31];16. Available from: https://www.ncbi.nlm.nih.gov/pmc/articles/PMC6798851/
Cammaerts S, Strazisar M, De Rijk P, Del Favero J. Genetic variants in microRNA genes: impact on microRNA expression, function, and disease. Front Genet. 2015;6:186.
Felekkis K, Touvana E, Stefanou C, Deltas C. microRNAs: a newly described class of encoded molecules that play a role in health and disease. Hippokratia. 2010;14:236–40.
Steri M, Idda ML, Whalen MB, Orrù V. Genetic variants in mRNA untranslated regions. Wiley Interdiscip Rev RNA. 2018;9:e1474.
A M, M G, Jf C, R B. SNPs in microRNA target sites and their potential role in human disease. Open biology [Internet]. Open Biol; 2017 [cited 2021 May 31];7. Available from: https://pubmed.ncbi.nlm.nih.gov/28381629/
Statello L, Guo C-J, Chen L-L, Huarte M. Gene regulation by long non-coding RNAs and its biological functions. Nature Rev Mol Cell Biol. 2021;22:96–118.
Giral H, Landmesser U, Kratzer A. Into the Wild: GWAS Exploration of Non-coding RNAs. Front Cardiovasc Med [Internet]. Frontiers; 2018 [cited 2021 Jun 10];5. Available from: https://doi.org/10.3389/fcvm.2018.00181/full
Gasperini M, Hill AJ, McFaline-Figueroa JL, Martin B, Kim S, Zhang MD, et al. A genome-wide framework for mapping gene regulation via cellular genetic screens. Cell. 2019;176:377-390.e19.
Schraivogel D, Gschwind AR, Milbank JH, Leonce DR, Jakob P, Mathur L, et al. Targeted Perturb-seq enables genome-scale genetic screens in single cells. Nat Methods [Internet]. 2020 [cited 2020 Jun 2]; Available from: http://www.nature.com/articles/s41592-020-0837-5
Boix CA, James BT, Park YP, Meuleman W, Kellis M. Regulatory genomic circuitry of human disease loci by integrative epigenomics. Nature. 2021;590:300–7.
Doni Jayavelu N, Jajodia A, Mishra A, Hawkins RD. Candidate silencer elements for the human and mouse genomes. Nat Commun [Internet]. 2020 [cited 2020 Apr 21];11. Available from: https://www.ncbi.nlm.nih.gov/pmc/articles/PMC7044160/
Gasperini M, Tome JM, Shendure J. Towards a comprehensive catalogue of validated and target-linked human enhancers. Nat Rev Genet. 2020;21:292–310.
Pang B, Snyder MP. Systematic identification of silencers in human cells. Nat Genet. 2020;52:254–63.
Bramer WM, de Jonge GB, Rethlefsen ML, Mast F, Kleijnen J. A systematic approach to searching: an efficient and complete method to develop literature searches. J Med Libr Assoc. 2018;106:531–41.
Bramer WM, Giustini D, Kramer BMR. Comparing the coverage, recall, and precision of searches for 120 systematic reviews in Embase, MEDLINE, and Google Scholar: a prospective study. Syst Rev. 2016;5:39.
Wang X, Tucker NR, Rizki G, Mills R, Krijger PH, de Wit E, et al. Discovery and validation of sub-threshold genome-wide association study loci using epigenomic signatures. Elife. 2016;5.
Stadhouders R, Aktuna S, Thongjuea S, Aghajanirefah A, Pourfarzad F, van Ijcken W, et al. HBS1L-MYB intergenic variants modulate fetal hemoglobin via long-range MYB enhancers. J Clin Invest. 2014;124:1699–710.
Pashos EE, Park Y, Wang X, Raghavan A, Yang W, Abbey D, et al. Large, diverse population cohorts of hiPSCs and derived hepatocyte-like cells reveal functional genetic variation at blood lipid-associated loci. Cell Stem Cell. 2017;20:558-570.e10.
Visser M, Kayser M, Palstra R-J. HERC2 rs12913832 modulates human pigmentation by attenuating chromatin-loop formation between a long-range enhancer and the OCA2 promoter. Genome Res. 2012;22:446–55.
Musunuru K, Strong A, Frank-Kamenetsky M, Lee NE, Ahfeldt T, Sachs KV, et al. From noncoding variant to phenotype via SORT1 at the 1p13 cholesterol locus. Nature. 2010;466:714–9.
Guo H, Ahmed M, Zhang F, Yao CQ, Li S, Liang Y, et al. Modulation of long noncoding RNAs by risk SNPs underlying genetic predispositions to prostate cancer. Nat Genet. 2016;48:1142–50.
Ghoussaini M, French JD, Michailidou K, Nord S, Beesley J, Canisus S, et al. Evidence that the 5p12 variant rs10941679 confers susceptibility to estrogen-receptor-positive breast cancer through FGF10 and MRPS30 regulation. Am J Hum Genet. 2016;99:903–11.
Viñuela A, Varshney A, van de Bunt M, Prasad RB, Asplund O, Bennett A, et al. Genetic variant effects on gene expression in human pancreatic islets and their implications for T2D. Nat Commun. 2020;11:4912.
Stacey D, Fauman EB, Ziemek D, Sun BB, Harshfield EL, Wood AM, et al. ProGeM: a framework for the prioritization of candidate causal genes at molecular quantitative trait loci. Nucleic Acids Res. 2019;47:e3.
Fang H, ULTRA-DD Consortium, De Wolf H, Knezevic B, Burnham KL, Osgood J, et al. A genetics-led approach defines the drug target landscape of 30 immune-related traits. Nat Genet. 2019;51:1082–91.
Lukowski SW, Lloyd-Jones LR, Holloway A, Kirsten H, Hemani G, Yang J, et al. Genetic correlations reveal the shared genetic architecture of transcription in human peripheral blood. Nat Commun. 2017;8:483.
Download references
Acknowledgements
The authors would like to thank Rainer Winnenburg of AbbVie for his assistance with higher order disease mapping and Mark Reppell of AbbVie for helpful advice pertaining to the GWAS Catalog portion of the analysis and for suggesting heritability datasets.
The design, study conduct, and financial support for this research were provided by AbbVie. AbbVie participated in the interpretation of data, review, and approval of the publication.
Author information
Authors and affiliations.
Genomics Research Center, AbbVie Inc, North Chicago, Illinois, 60064, USA
Ammar J. Alsheikh, Emily A. King, Sujana Ghosh, Lindsay R. Stolzenburg, Saleh Tamim, Jozef Lazar, J. Wade Davis & Howard J. Jacob
Information Research, AbbVie Deutschland GmbH & Co. KG, 67061, Knollstrasse, Ludwigshafen, Germany
Sabrina Wollenhaupt & Jonas Reeb
You can also search for this author in PubMed Google Scholar
Contributions
AA, SW, JL designed the study. SW, JR collected the data. AA, SW, EK, JR, SG, ST, LS analyzed the results. AA, SW, EK, JR, SG, ST, LS, JL, JWD and HJ interpreted results. AA, SW, EK wrote the manuscript. JL, JWD and HJ supervised the project. All authors read and approved the final manuscript.
Corresponding author
Correspondence to Ammar J. Alsheikh .
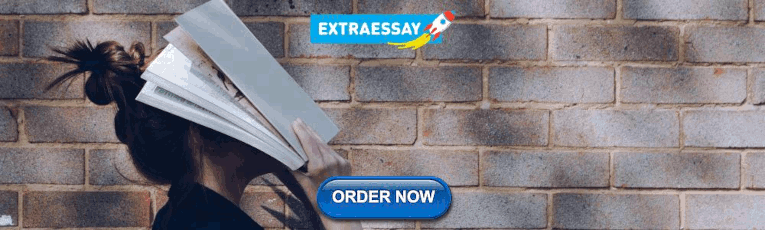
Ethics declarations
Ethics approval and consent to participate.
Not applicable.
Consent for publication
Competing interests.
AA, SW, EK, JR, SG, ST and HJ are employees of AbbVie. LS, JWD and JL were employees of AbbVie at the time of the study.
Additional information
Publisher's note.
Springer Nature remains neutral with regard to jurisdictional claims in published maps and institutional affiliations.
Supplementary Information
Additional file 1.
contains exact search terms and criteria used for creating the initial broad literature search.
Additional file 2
contains exact terms and phrases used to setup the seven filters that were used to narrow down the broad search results.
Additional file 3
contains all the validated variants and their details. The file is formatted to include separate rows for unique PMID-variant-gene triples, therefore variants that regulate multiple genes and variants that have been validated in more than one publication have more than one row in the file.
Rights and permissions
Open Access This article is licensed under a Creative Commons Attribution 4.0 International License, which permits use, sharing, adaptation, distribution and reproduction in any medium or format, as long as you give appropriate credit to the original author(s) and the source, provide a link to the Creative Commons licence, and indicate if changes were made. The images or other third party material in this article are included in the article's Creative Commons licence, unless indicated otherwise in a credit line to the material. If material is not included in the article's Creative Commons licence and your intended use is not permitted by statutory regulation or exceeds the permitted use, you will need to obtain permission directly from the copyright holder. To view a copy of this licence, visit http://creativecommons.org/licenses/by/4.0/ . The Creative Commons Public Domain Dedication waiver ( http://creativecommons.org/publicdomain/zero/1.0/ ) applies to the data made available in this article, unless otherwise stated in a credit line to the data.
Reprints and permissions
About this article
Cite this article.
Alsheikh, A.J., Wollenhaupt, S., King, E.A. et al. The landscape of GWAS validation; systematic review identifying 309 validated non-coding variants across 130 human diseases. BMC Med Genomics 15 , 74 (2022). https://doi.org/10.1186/s12920-022-01216-w
Download citation
Received : 13 October 2021
Accepted : 17 March 2022
Published : 01 April 2022
DOI : https://doi.org/10.1186/s12920-022-01216-w
Share this article
Anyone you share the following link with will be able to read this content:
Sorry, a shareable link is not currently available for this article.
Provided by the Springer Nature SharedIt content-sharing initiative
- Experimental validation
- Functional variant
- Systematic review
BMC Medical Genomics
ISSN: 1755-8794
- Submission enquiries: [email protected]
- General enquiries: [email protected]

- News Releases
Researchers show genetic variant common among Black Americans contributes to large cardiovascular disease burden
Brigham and Women's Hospital
Researchers at Brigham and Women’s Hospital and Duke University showed that a genetic variant, present in 3-4% of self-identified Black individuals in the U.S., increases the risk for both heart failure and death and contributes to significant decreases in longevity at the population level
A genetic variant carried by 3-4 percent of self-identified Black Americans increases the risk for heart failure and death, contributing to a significant decrease in longevity at the population level, according to a new study led by researchers at Brigham and Women’s Hospital , a founding member of the Mass General Brigham healthcare system, and Duke University School of Medicine . The new research shows that individuals who carry the V142I transthyretin variant are at significantly increased risk for heart failure beginning in their 60s, with an increased risk for death beginning in their 70s. Further, the researchers showed that carriers on average died 2 to 2.5 years earlier than expected. With nearly half a million Black Americans carriers over age 50, the researchers estimate that approximately a million years of life will be lost due to this variant among currently living Black individuals who are in mid-to-late life. Results are published in JAMA .
“We believe these data will inform clinicians and patients regarding risk when these genetic findings are known, either through family screening, medical, or even commercial genetic testing,” said senior author Scott D. Solomon, MD , the Edward D. Frohlich Distinguished Chair, Professor of Medicine at Brigham and Women’s Hospital and Harvard Medical School. “There are now several potential new therapies for cardiac amyloidosis, and understanding the magnitude of this risk, at the individual and societal level, will help determine which patients might be best suited for novel therapies.”
The V142I variant causes transthyretin, a protein in the blood, to misfold leading to deposits of abnormal amyloid protein in the heart and other parts of the body. In the heart, these deposits cause the muscle to become thick and stiffened, a condition known as cardiac amyloidosis, which can ultimately lead to heart failure. Recently, several therapies have been developed to treat cardiac amyloidosis, including therapies that: prevent the protein from misfolding, reduce the amount of protein, remove the protein, and even a gene-editing therapy that is currently undergoing clinical trials. A better understanding of the epidemiology of V142I and cardiac amyloidosis would help physicians connect patients with the appropriate treatment at the appropriate age, the researchers say.
Although the association between the V142I variant and heart failure has been previously described, precise estimates of how the variant increases risk were unclear until now. Considering approximately 48 million Americans self-identify as Black, 1.5 million across the lifespan are estimated to carry this variant. However, since effects of the variant aren’t typically seen until after age 50, the researchers focused on the risk among Black Americans in mid-to-late life.
To uncover these details, the researchers pooled data from self-reported Black participants in four NIH-funded studies in the United States (ARIC, MESA, REGARDS and Women’s Health Initiative). Altogether, the team examined data from 23,338 self-reported Black individuals, 754 (3.23 percent) of whom carried the V142I genetic variant.
They showed that V142I increased the risk for heart failure hospitalization by age 63 and the risk of death by age 72. The variant’s contribution to heart failure risk increased substantially with age but was not itself increased by other known risk factors such as diabetes and hypertension. The team also showed that female and male carriers of the variant were equally at risk, contrary to some previous studies showing that men were more affected. This suggests that women are likely underdiagnosed with the condition. The researchers estimated that individual carriers with the V142I variant live 2-2.5 years less than expected.
“Since 3-4 percent of self-identified Black individuals in the United States carry this variant, a significant number are at elevated risk for developing cardiac amyloidosis, being hospitalized for heart failure, and dying several years earlier than expected,” said first author Senthil Selvaraj, MD, an advanced heart failure physician-scientist at Duke University School of Medicine . “With our improved understanding of the risks with the variant, future efforts to increase disease awareness and ultimately connect carriers with the disease to effective therapies will be important.”
In future studies, the researchers plan to investigate why some, but not all, carriers of the V142I variant develop cardiac amyloidosis. They are also actively involved in developing and testing therapies for the disease, including the gene therapy mentioned above.
“One of the areas that will be really important going forward will be whether we can actually prevent the onset of the disease if we identify these patients earlier,” said Solomon.
Authorship: Additional Brigham authors include Brian Claggett, and JoAnn E. Manson. Other authors include Robert J. Mentz, Svati H. Shah, Michel G. Khouri, Ani W. Manichaikul, Sadiya S. Khan, Stephen S. Rich, Thomas H. Mosley, Emily B. Levitan, Pankaj Arora, Parag Goyal, Bernhard Haring, Charles B. Eaton, Richard K. Cheng, Gretchen L. Wells, and Marianna Fontana.
Disclosures: Selvaraj receives research support from the National Heart, Lung, and Blood Institute (K23HL161348), Doris Duke Charitable Foundation (#2020061), American Heart Association (#935275), the Mandel Foundation, Duke Heart Center Leadership Council, the Institute for Translational Medicine and Therapeutics, and Foundation for Sarcoidosis Research. He has participated in advisory boards for AstraZeneca. Solomon has received research grants from Alexion, Alnylam, AstraZeneca, Bellerophon, Bayer, BMS, Cytokinetics, Eidos, Gossamer, GSK, Ionis, Lilly, MyoKardia, NIH/NHLBI, Novartis, NovoNordisk, Respicardia, Sanofi Pasteur, Theracos, US2.AI and has consulted for Abbott, Action, Akros, Alexion, Alnylam, Amgen, Arena, AstraZeneca, Bayer, Boeringer-Ingelheim, BMS, Cardior, Cardurion, Corvia, Cytokinetics, Daiichi-Sankyo, GSK, Lilly, Merck, Myokardia, Novartis, Roche, Theracos, Quantum Genomics, Cardurion, Janssen, Cardiac Dimensions, Tenaya, Sanofi-Pasteur, Dinaqor, Tremeau, CellProThera, Moderna, American Regent, Sarepta, Lexicon, Anacardio, Akros, Valo. Additional author disclosures can be found in the paper. Funding: Funding for the cohorts was provided by the National Institutes of Health.
Paper cited: Selvaraj, S et al. “Cardiovascular Burden of the V1421 Transthyretin Variant” JAMA DOI: 10.1001/jama.2024.4467
10.1001/jama.2024.4467
Article Title
Selvaraj, S et al. “Cardiovascular Burden of the V1421 Transthyretin Variant” JAMA DOI: 10.1001/jama.2024.4467
Article Publication Date
12-May-2024
Disclaimer: AAAS and EurekAlert! are not responsible for the accuracy of news releases posted to EurekAlert! by contributing institutions or for the use of any information through the EurekAlert system.
- Share full article
Advertisement
Supported by
Study Suggests Genetics as a Cause, Not Just a Risk, for Some Alzheimer’s
People with two copies of the gene variant APOE4 are almost certain to get Alzheimer’s, say researchers, who proposed a framework under which such patients could be diagnosed years before symptoms.

By Pam Belluck
Scientists are proposing a new way of understanding the genetics of Alzheimer’s that would mean that up to a fifth of patients would be considered to have a genetically caused form of the disease.
Currently, the vast majority of Alzheimer’s cases do not have a clearly identified cause. The new designation, proposed in a study published Monday, could broaden the scope of efforts to develop treatments, including gene therapy, and affect the design of clinical trials.
It could also mean that hundreds of thousands of people in the United States alone could, if they chose, receive a diagnosis of Alzheimer’s before developing any symptoms of cognitive decline, although there currently are no treatments for people at that stage.
The new classification would make this type of Alzheimer’s one of the most common genetic disorders in the world, medical experts said.
“This reconceptualization that we’re proposing affects not a small minority of people,” said Dr. Juan Fortea, an author of the study and the director of the Sant Pau Memory Unit in Barcelona, Spain. “Sometimes we say that we don’t know the cause of Alzheimer’s disease,” but, he said, this would mean that about 15 to 20 percent of cases “can be tracked back to a cause, and the cause is in the genes.”
The idea involves a gene variant called APOE4. Scientists have long known that inheriting one copy of the variant increases the risk of developing Alzheimer’s, and that people with two copies, inherited from each parent, have vastly increased risk.
The new study , published in the journal Nature Medicine, analyzed data from over 500 people with two copies of APOE4, a significantly larger pool than in previous studies. The researchers found that almost all of those patients developed the biological pathology of Alzheimer’s, and the authors say that two copies of APOE4 should now be considered a cause of Alzheimer’s — not simply a risk factor.
The patients also developed Alzheimer’s pathology relatively young, the study found. By age 55, over 95 percent had biological markers associated with the disease. By 65, almost all had abnormal levels of a protein called amyloid that forms plaques in the brain, a hallmark of Alzheimer’s. And many started developing symptoms of cognitive decline at age 65, younger than most people without the APOE4 variant.
“The critical thing is that these individuals are often symptomatic 10 years earlier than other forms of Alzheimer’s disease,” said Dr. Reisa Sperling, a neurologist at Mass General Brigham in Boston and an author of the study.
She added, “By the time they are picked up and clinically diagnosed, because they’re often younger, they have more pathology.”
People with two copies, known as APOE4 homozygotes, make up 2 to 3 percent of the general population, but are an estimated 15 to 20 percent of people with Alzheimer’s dementia, experts said. People with one copy make up about 15 to 25 percent of the general population, and about 50 percent of Alzheimer’s dementia patients.
The most common variant is called APOE3, which seems to have a neutral effect on Alzheimer’s risk. About 75 percent of the general population has one copy of APOE3, and more than half of the general population has two copies.
Alzheimer’s experts not involved in the study said classifying the two-copy condition as genetically determined Alzheimer’s could have significant implications, including encouraging drug development beyond the field’s recent major focus on treatments that target and reduce amyloid.
Dr. Samuel Gandy, an Alzheimer’s researcher at Mount Sinai in New York, who was not involved in the study, said that patients with two copies of APOE4 faced much higher safety risks from anti-amyloid drugs.
When the Food and Drug Administration approved the anti-amyloid drug Leqembi last year, it required a black-box warning on the label saying that the medication can cause “serious and life-threatening events” such as swelling and bleeding in the brain, especially for people with two copies of APOE4. Some treatment centers decided not to offer Leqembi, an intravenous infusion, to such patients.
Dr. Gandy and other experts said that classifying these patients as having a distinct genetic form of Alzheimer’s would galvanize interest in developing drugs that are safe and effective for them and add urgency to current efforts to prevent cognitive decline in people who do not yet have symptoms.
“Rather than say we have nothing for you, let’s look for a trial,” Dr. Gandy said, adding that such patients should be included in trials at younger ages, given how early their pathology starts.
Besides trying to develop drugs, some researchers are exploring gene editing to transform APOE4 into a variant called APOE2, which appears to protect against Alzheimer’s. Another gene-therapy approach being studied involves injecting APOE2 into patients’ brains.
The new study had some limitations, including a lack of diversity that might make the findings less generalizable. Most patients in the study had European ancestry. While two copies of APOE4 also greatly increase Alzheimer’s risk in other ethnicities, the risk levels differ, said Dr. Michael Greicius, a neurologist at Stanford University School of Medicine who was not involved in the research.
“One important argument against their interpretation is that the risk of Alzheimer’s disease in APOE4 homozygotes varies substantially across different genetic ancestries,” said Dr. Greicius, who cowrote a study that found that white people with two copies of APOE4 had 13 times the risk of white people with two copies of APOE3, while Black people with two copies of APOE4 had 6.5 times the risk of Black people with two copies of APOE3.
“This has critical implications when counseling patients about their ancestry-informed genetic risk for Alzheimer’s disease,” he said, “and it also speaks to some yet-to-be-discovered genetics and biology that presumably drive this massive difference in risk.”
Under the current genetic understanding of Alzheimer’s, less than 2 percent of cases are considered genetically caused. Some of those patients inherited a mutation in one of three genes and can develop symptoms as early as their 30s or 40s. Others are people with Down syndrome, who have three copies of a chromosome containing a protein that often leads to what is called Down syndrome-associated Alzheimer’s disease .
Dr. Sperling said the genetic alterations in those cases are believed to fuel buildup of amyloid, while APOE4 is believed to interfere with clearing amyloid buildup.
Under the researchers’ proposal, having one copy of APOE4 would continue to be considered a risk factor, not enough to cause Alzheimer’s, Dr. Fortea said. It is unusual for diseases to follow that genetic pattern, called “semidominance,” with two copies of a variant causing the disease, but one copy only increasing risk, experts said.
The new recommendation will prompt questions about whether people should get tested to determine if they have the APOE4 variant.
Dr. Greicius said that until there were treatments for people with two copies of APOE4 or trials of therapies to prevent them from developing dementia, “My recommendation is if you don’t have symptoms, you should definitely not figure out your APOE status.”
He added, “It will only cause grief at this point.”
Finding ways to help these patients cannot come soon enough, Dr. Sperling said, adding, “These individuals are desperate, they’ve seen it in both of their parents often and really need therapies.”
Pam Belluck is a health and science reporter, covering a range of subjects, including reproductive health, long Covid, brain science, neurological disorders, mental health and genetics. More about Pam Belluck
The Fight Against Alzheimer’s Disease
Alzheimer’s is the most common form of dementia, but much remains unknown about this daunting disease..
How is Alzheimer’s diagnosed? What causes Alzheimer’s? We answered some common questions .
A study suggests that genetics can be a cause of Alzheimer’s , not just a risk, raising the prospect of diagnosis years before symptoms appear.
Determining whether someone has Alzheimer’s usually requires an extended diagnostic process . But new criteria could lead to a diagnosis on the basis of a simple blood test .
The F.D.A. has given full approval to the Alzheimer’s drug Leqembi. Here is what to know about i t.
Alzheimer’s can make communicating difficult. We asked experts for tips on how to talk to someone with the disease .

An official website of the United States government
The .gov means it’s official. Federal government websites often end in .gov or .mil. Before sharing sensitive information, make sure you’re on a federal government site.
The site is secure. The https:// ensures that you are connecting to the official website and that any information you provide is encrypted and transmitted securely.
- Publications
- Account settings
Preview improvements coming to the PMC website in October 2024. Learn More or Try it out now .
- Advanced Search
- Journal List
- Elsevier - PMC COVID-19 Collection

SARS-CoV-2 Mutations and their Viral Variants
Begum cosar.
a Başkent University, Faculty of Science and Letters, Department of Molecular Biology and Genetics, Ankara, Turkey
Zeynep Yagmur Karagulleoglu
b Yıldız Technical University, Faculty of Arts and Science, Department of Molecular Biology and Genetics, İstanbul, Turkey
Ahmet Turan Ince
c Sivas Cumhuriyet University, Faculty of Medicine, Sivas, Turkey
Dilruba Beyza Uncuoglu
d Ankara University, Graduate School of Natural and Applied Sciences, Department of Biology, Ankara, Turkey
Gizem Tuncer
e Hacettepe University, Graduate School of Science and Engineering, General Biology Program, Ankara, Turkey
f HücreCELL Biotechnology Development and Commerce, Inc., Ankara, Turkey
Bugrahan Regaip Kilinc
g Kastamonu University, School of Engineering and Architecture, Department of Genetics and Bioengineering, Kastamonu, Turkey
h Kastamonu University, School of Engineering and Architecture, Department of Biomedical Engineering, Kastamonu, Turkey
Yunus Emre Ozkan
i Gebze Technical University, Faculty of Science, Department of Molecular Biology and Genetics, Kocaeli, Turkey
Hikmet Ceyda Ozkoc
j Akdeniz University, Faculty of Medicine, Department of Medical Pharmacology, Antalya, Turkey
Ibrahim Naki Demir
k Akdeniz University, Faculty of Medicine, Antalya, Turkey
Feyzanur Karagoz
Said yasin simsek, bunyamin yasar.
l Alanya Alaaddin Keykubat University, Department of Molecular Medicine, Antalya, Turkey
Mehmetcan Pala
m Sivas Cumhuriyet University, Faculty of Science, Department of Molecular Biology and Genetics, Sivas, Turkey
Aysegul Demir
n Üsküdar University, Faculty of Engineering and Natural Sciences, Department of Molecular Biology and Genetics, İstanbul, Turkey
Irem Naz Atak
o Ankara University, Faculty of Science, Department of Biology, Ankara, Turkey
Aysegul Hanife Mendi
p Gazi University, Faculty of Dentistry, Department of Basic Sciences, Division of Medical Microbiology, Ankara, Turkey
Vahdi Umut Bengi
q Gülhane Training and Research Hospital, Faculty of Dentistry, Department of Periodontology, Ankara, Turkey
Guldane Cengiz Seval
r Ankara University, School of Medicine Department of Hematology, Cebeci, Ankara, Turkey
Evrim Gunes Altuntas
s Ankara University, Biotechnology Institute, Ankara, Turkey
Pelin Kilic
t Ankara University, Stem Cell Institute, Ankara, Turkey
Devrim Demir-Dora
u Akdeniz University, Faculty of Medicine, Department of Medical Pharmacology, Antalya, Turkey
v Akdeniz University, Health Sciences Institute, Department of Gene and Cell Therapy, Antalya, Turkey
w Akdeniz University, Health Sciences Institute, Department of Medical Biotechnology, Antalya, Turkey
Mutations in the severe acute respiratory syndrome coronavirus 2 (SARS-CoV-2) occur spontaneously during replication. Thousands of mutations have accumulated and continue to since the emergence of the virus. As novel mutations continue appearing at the scene, naturally, new variants are increasingly observed.
Since the first occurrence of the SARS-CoV-2 infection, a wide variety of drug compounds affecting the binding sites of the virus have begun to be studied. As the drug and vaccine trials are continuing, it is of utmost importance to take into consideration the SARS-CoV-2 mutations and their respective frequencies since these data could lead the way to multi-drug combinations. The lack of effective therapeutic and preventive strategies against human coronaviruses (hCoVs) necessitates research that is of interest to the clinical applications.
The reason why the mutations in glycoprotein S lead to vaccine escape is related to the location of the mutation and the affinity of the protein. At the same time, it can be said that variations should occur in areas such as the receptor-binding domain (RBD), and vaccines and antiviral drugs should be formulated by targeting more than one viral protein.
In this review, a literature survey in the scope of the increasing SARS-CoV-2 mutations and the viral variations is conducted. In the light of current knowledge, the various disguises of the mutant SARS-CoV-2 forms and their apparent differences from the original strain are examined as they could possibly aid in finding the most appropriate therapeutic approaches.
1. Background information
Mutations in the severe acute respiratory syndrome coronavirus 2 (SARS-CoV-2) occur spontaneously during replication. Thousands of cumulative mutations have occurred since the emergence of the virus [ 1 ]. As novel mutations continue to emerge, naturally, new mutants are increasingly observed. Most of the mutations that occur in the SARS-CoV-2 genome have no notable effect on the spread and the virulence of the virus, and hence on the course of the disease [ 2 ]. The greatest concern about such emerging mutations is a risky change that could lead to an increase in the severity of the infection or a failure on the effects of vaccines currently being developed. This is mainly because the viral signals may escape the immune protection which originate from a preceding infection or vaccination [ 3 ]. The first occurrence of any mutation is difficult to correlate with the continuity of the alterations. Understanding the significance of the alterations may be possible through experimental studies, by showing a link between the mutation in question and a subtle change in viral biology. However, testing the effect of thousands of mutants takes considerable time and effort.
As the case with other CoVs, the SARS-CoV-2 genome contains at least 23 open reading frames (ORFs) [ 4 ]. The SARS-CoV-2 genome contains ORFs that are responsible for the production of non-structural proteins (Nsps) [ 5 ]. ORFs encode at least 4 main structural proteins: the spike (S), membrane (M), envelope (E), and nucleocapsid (N) proteins [ 6 ]. Among these, the most notable mutations are those in the gene encoding the S protein, which is associated with viral entry into the cells. There are currently around 4000 mutations in the S protein gene. There are a few mutations in the region called the receptor-binding motifs (RBMs) of the S protein, the region responsible for viral entry through its interaction with the human angiotensin-converting enzyme 2 (hACE2) receptor on the host cells [ 7 ].
In our review, we conducted a literature survey under the scope of the exponentially increasing SARS-CoV-2 mutations and the numerous viral variations as the outcome. In the light of current knowledge, we aim to elaborate SARS-CoV-2′s ever changing disguises into novel mutant forms in various locations around the world, to analyze what features of such upcoming mutants differ from its original manifestation, and to emphasize the apparent discrepancies, which may be able to, in return, possibly aid in finding solutions for developing novel therapeutic approaches.
2. An overview of SARS-CoV-2
CoVs are a group of infectious pathogens that cause a wide range of clinical conditions such as respiratory, enteric, hepatic and neurological diseases. Highly pathogenic human CoVs belong to the Coronaviridae family. CoVs are divided into four genera: alpha-CoV, beta-CoV, gamma-CoV and delta-CoV. As well-known today, SARS-CoV-2 is an RNA coronavirus responsible for the coronavirus disease 2019 (COVID-19) outbreak. Proven to be the novel pathogen of COVID-19, SARS-CoV-2 belongs to the beta-CoV genus, a linear, single-stranded RNA genome of approximately 30 kb, and the Sarbecovirus sub-gene, as seen in Table 1 [ 14 ].
Comparison of SARS-CoV, MERS-CoV and other human coronaviruses (hCoVs) by species, genome, genome length and percentage (%) similarity to the SARS-CoV-2 genome.
CoVs are enveloped viruses with positive sense RNA genomes with a single cistern of approximately 26−32 kb, which have the largest known genome size for an RNA virus. Seven CoVs – i.e., GC-V-229E, Human CoV-NL63 (hCoV-NL63), human CoV-OC43 (hCoV-OC43), human CoV-HKU1 (hCoV-HKU1), SARS-CoV, Middle East respiratory syndrome CoV (MERS-CoV), SARS-CoV-2 – have infected humans to date [ 15 ] ( Table 1 ). The estimated mutation rates of CoVs are moderate or high compared to other single-stranded positive-sense RNA (+ssRNA) viruses. The antigenic surface of SARS-CoV-2 is quite different compared to other CoVs. Both the SARS-CoV-2 and the SARS infections have many common features. Both cause respiratory diseases. They are transmitted from animals to humans as an intermediate host. Both airborne and can be transmitted via respiratory fluids, which are fine droplets released during respiration from an infected person [ 16 ]. People with the SARS-CoV-2 infection tend to transmit more rapidly than those with the SARS infection ( Table 2 ).
Percentage (%) of sequential similarity of SARS-CoV, MERS-CoV, HCoV-HKU1 and HCoV-OC43 proteins with SARS-CoV-2 proteins.
SARS-CoV had emerged as a major cause of severe lower respiratory tract infection in humans in 2002. In some studies conducted at that time, new strains and the possibility of future outbreaks were mentioned [ 22 , 23 ]. The severe and sudden symptoms resulting in atypical pneumonia with dry cough and persistent high fever in severe cases of the acute respiratory virus have revealed the importance of CoVs as potentially lethal human pathogens, and the identification of several zoonotic reservoirs has reappeared.
SARS-CoV-2 is the seventh CoV known to infect humans [ 24 ]. The world experienced its first international health emergency in the 21st century with the disease called SARS, in 2003. SARS had first started in China and soon spread to Asia, North America and Europe, causing 800 deaths in approximately 30 countries. Similarly, cases of pneumonia of unknown etiology were reported on December 31, 2019 in Wuhan, Hubei Province, China. It was identified on January 7, 2020, that the disease agent was an unprecedented CoV (2019-nCoV) in humans.
2.1. SARS-CoV-2 structural properties and the replication cycle
SARS-CoV-2 has typical features among the CoV family, belongs to the beta-CoV 2b group and is an enveloped +ssRNA virus [ 25 ]. SARS-CoV-2 encodes the basic structural proteins of S, M, E and N, as seen in Fig. 1 . Also as observed in Table 1 , the SARS-CoV, MERS-CoV, hCoV-HKU1 and hCoV-OC43 proteins have sequencing similarities with SARS-CoV-2 proteins [ 26 ].

The to-date defined surface protein structure of of SARS-CoV-2 (+ssRNA: single-stranded positive-sense RNA).
+ssRNA viruses, a large group that includes human pathogens such as SARS-CoV, replicate in the cytoplasm of the infected host cells. Replication complexes are generally associated with modified host cell membranes [ 27 ]. The SARS-CoV replication is driven by the membrane-bound viral enzyme complex. This complex is often linked to modified intracellular membranes. CoVs and other members of the Nidovirus family have a polycistronic genome, and use a variety of transcriptional and (post-) translational mechanisms to regulate their expression [ 28 , 29 ]. Post-translational modifications are covalent modifications of proteins after they are translated by ribosomes. It identifies new functional groups such as phosphate and carbohydrates, expands the chemical repertoire of 20 standard amino acids through post-translational modifications, and plays important roles in regulating the folding, stability, enzymatic activity, subcellular localization and interaction of a protein with other proteins [ 30 ]. Viruses that maintain compulsory cell life receive support from the protein synthesis mechanisms of the host cells after respiration. For this reason, after the polypeptides are synthesized, they modify protein functions by creating covalent modifications [ 31 ]. The gene encoding the replicase/transcriptase (this gene is commonly referred to as "replicase"), contains nearly two-thirds of the CoV genome, the largest known RNA genome to date. The replicase gene consists of ORFs 1a and 1b. ORF1b is expressed by a ribosomal frameshift near the 3′-terminal of the ORF1a. Thus, the SARS-CoV genome translation yields two polyproteins (pp1a and pp1ab) that are auto-proteolytically cleaved into 16 Nsps by proteases found in Nsp3 and Nsp5 [ 32 , 33 ].
2.2. Entry into the cell
The default gateway, the cellular receptor, or SARS-CoV-2 is angiotensin-converting enzyme 2 (ACE2) [ 34 , 35 ]. Both SARS-CoV-2 and SARS-CoV use hACE2 as the input receptor and human proteases as input activators. The S protein, the leading viral surface protein, mediates the entry of SARS-CoV-2 into the cell. To fulfill the function of SARS-CoV-2, the receptor binds to hACE2 via the receptor-binding domain (RBD) and is proteolytically activated by human proteases. It is thought-provoking that the recombinant hACE2 (rhACE2) significantly reduces viral utilization in human cell-derived organoids [ 36 ], possibly serving as a decoy for virus binding.
Normally, ACE2 acts in regulating blood pressure. However when the CoV binds to ACE2, a series of chemical changes occur, that effectively inter-connect the membranes around the cell and the virus, allowing the RNA of the virus to enter the cell. To enter the host cells, CoVs first bind to a cell surface receptor for viral attachment, then penetrate into the endosomes, and eventually join the viral and lysosomal membranes [ 37 , 38 ].
Protease activators have also been studied for SARS-CoV-2 entry at the receptor level. Both the transmembrane protease serine 2 (TMPRSS2) and lysosomal proteases are important for SARS-CoV-2 entry [ 39 , 40 ]. A successful viral entry requires proteolytic processing of the viral coat glycoprotein S, which is able to be carried out by TMPRSS2. Both camostat and the camostat-related agent nafamostat [ 41 ] block SARS-CoV-2 replication in human cells which express TMPRSS2. CoVs use the endo-lysosomal pathway to enter the cell before reproducing.
The CoV life cycle includes several potentially targetable steps: i) endocytic entry into host cells (via ACE2 and TMPRSS2), ii) RNA replication and transcription (helicase-containing transcription), and RNA-dependent RNA polymerase (RdRp) activation, translation and proteolytic processing of viral proteins, and iii) viron assembly and release of new viruses through exocytic systems [ 42 ] ( Fig. 2 ).

Cell entry of SARS-CoV-2, replication cycle and synthesis of viral components. 1: SARS-CoV-2 binds via the S glycoprotein to the ACE-2 receptor expressed in the host cell. 2. SARS-CoV-2 enters the cell with clathrin-coated pits. 3. The clathrin structures are separated from the main structure. 4. Endosome fusion (with dynein) takes place to release the viral RNA genome. 5. The dynein units are separated from the structure and the endosome begins to open. 6. The opening of the endosome and release of the viral RNA genome. The viral RNA genome is synthesized using host ribosomes, viral polymerase. 7. Genomic and subgenomic RNA synthesis takes place in the synthesis of viral proteins. Then, with the help of ribosomes, viral RNAs are transmitted and viral proteins are synthesized. 8. Viral components come together to form the endosomal structure, then to make up for SARS-CoV-2.
3. Mutations in the spike (S) protein
The entry of SARS-CoV-2 into the cell takes place through the S protein [ 43 ], which has an important role in viral infection and pathogenesis [ 44 ]. The S protein consists of two subdomains: i) S1, and ii) S2. The S1 protein consists of an N-terminal domain (NTD) and a C-terminal domain (CTD) ( Fig. 3 ). These two domains act as RBD and can bind various sugars and proteins [ 45 ]. S1 recognizes and binds to hACE2 receptors. S2 facilitates fusion through conformational changes [ 46 , 47 ]. While the S1 domain varies even among a single CoV species, the S2 domain is the most reserved region of the S protein.

The structure of the SARS-CoV-2 spike (S) protein. (RBD: receptor binding domain; NDT: N-terminal domain; FP: fusion protein; T.A.: transmembrane anchor and I.T.: intracelluar tail).
The S protein found in the SARS-CoV-2 genome is of great importance ACE2 receptor binding and membrane fusion of the virus, and running scientific studies on therapeutic approaches and on the formation of immune response. Therefore, mutations that occur in the S protein, especially the RBD in the S gene, should be thoroughly examined.
There are currently around 4000 mutations in the S protein gene. The well-known mutations are listed in Table 3 .
The molecular location and geographical distribution of mutations in the S gene region.
3.1. Mutations in the receptor-binding domain (RBD) of SARS-CoV-2
The S protein RBD is defined as the critical determinant of viral tropism and infectivity. Therefore, more attention should be paid to whether mutations in the RBD of circulating SARS-CoV-2 strains alter the receptor-binding affinity and cause these strains to be more contagious. RBD mutation analysis provides information about the changes in SARS-CoV-2. The RBD CoV genome in the S protein is the most variable part [ 48 ]. Six RBD amino acids are critical for binding to ACE2 receptors and determining the seven major sequences of the SARS-CoV-like virus. While analyses suggest that SARS-CoV-2 can bind human ACE2 with high-affinity, computational analyses reveal that the interaction is not so ideal and that the RBD sequence differs from those shown to be optimal for receptor binding in SARS-CoV [ 49 ]. Thus, the high-affinity binding of the SARS-CoV-2 S protein to human ACE2 is most likely the result of natural selection on an hACE2 or human-like ACE2, which allows for another emerging optimal solution for binding [ 50 ]. This is strong evidence that SARS-CoV-2 is not a product of targeted manipulation. There are 725 present non-degenerate mutations in the SARS-CoV-2 S protein. Among such, 89 mutations involved in the binding of the SARS-CoV-2 S protein and ACE2 which occurrs in the RBD. Moreover, 52 of the 89 mutations are on the CRBM, the RBD region that is in direct contact with ACE2. Many mutations on RBD such as N439 K, L452R, T478I and E484D are noted to have significant free energy changes. Mutations in the RBM take up 58 % (52 of 89) of all mutations on the RBD, potentially increasing the complexity of antiviral drug and vaccine development. This overall analysis suggests that mutations in the RBD enhance the binding of the S protein and ACE2, leading to the more infectious SARS-CoV-2 [ 2 ]. Based on the up-to-date literature survey performed in this study, we retrieved 28 different S protein variants. Out of these variants, 12 belong to the RBD region, only.
3.2. Important mutations in the RBD and other domains of the S protein
3.2.1. d614g.
The D614G (Asp614-to-Gly)) mutation was first detected in Germany and China in late January 2020 [ 55 ]. It has become a worldwide mutant thereafter [ 56 ]. D614G was determined as the most prominent sequence variation with a rate of 56 % in experiments performed on experimental animals with the SARS-CoV-2 virus isolated in Anatolia [ 57 ]. It was formed by replacing the natural form of Asp614 with Gly in the S protein [ 58 ]. The D614G strain was accompanied by two different mutations. The first was a silent cytosine thymine (CT) mutation in the Nsp3 gene at position 3.037 and the second is a CT mutation of amino acid change at position 14.409 (RdRp P323 L), resulting in an RdRp [ 51 ]. The D614G mutation increased transduction in many cell types, including lung, liver, and colon cells. It is also more resistant to proteolytic cleavage. Accordingly, it is 4–9 times more contagious [ 52 ], however not an escape mutation [ 59 ].
3.2.2. S943P
The S943P mutation was the first to occur in the S protein in Belgium. In Belgium, 23 S943P mutations were found in 284 SARS-CoV-2 S sequences, but not among the remainder of the 6,063 S sequences sampled worldwide from outside of Belgium. As a result, the AGT (S) → CCT (P) mutation emerged [ 60 ]. The S943P mutation is a result of recombination of different viruses in an infected host and has evolved significantly [ 61 , 62 ].
3.2.3. V483a
The V483a mutation was first seen in North America [ 63 ]. V483a occurred in the S1 domain RBM of the S protein found in the virus genome [ 64 ]. This mutation occurs when the hydrophobic alanine replaces the hydrophobic valine, an important amino acid residue in the RBM region of glycoprotein S at position 483, and is caused by the transition from thymine (uracil) to cytosine at the genome position 23010 [ 65 ]. Since the V483a mutation site is not in direct contact with the ACE2 receptor [ 66 ], no significant change was observed without binding to the ACE2 receptor [ 62 ]. The RNA replication rate in the resulting mutant strain causes the virus to mutate in the host, resulting in the mutant strain to have strong drug resistance.
3.2.4. E484K
The E484K mutation, which was first observed in South Africa, is a rapid spread mutation found in the variants of South Africa (B.1.351) [ 67 ] and Brazil (B.1.1.28) [ 68 ]. This mutation in the S protein suggests that the virus is further developing and may become resistant to vaccines [ 69 ].
3.2.5. COH.20G/501Y
The COH.20G/501Y variant has a 20G backbone and was identified in Columbus independent of the 20G variant available in Ohio [ 70 ]. The S N501Y mutation, located within the RBD, is of particular concern for two reasons: i) its increased affinity to ACE2 [ 71 , 72 ], and ii) that it may impact association of receptor binding neutralizing antibodies including those in the Regeneron cocktail [ 71 , 73 ].
3.2.6. L452R
The L452R mutant was first detected in Denmark in March 2020. In California, the mutant prominently spread in Los Angeles. This mutation was found in 45 % of the existing samples in California [ 74 ]. This mutation weakened antibody neutralization and increased the virus's ability to infect [ 75 ].
3.2.7. Q677
The Q677 mutation was first noticed in New Mexico and Louisiana. In some strains, its 677th amino acid glutamine (Q) has been converted to proline (P). This variant is known as Q677P. In other strains, the same amino acid has transformed into histidine (H). This variant is also named Q677H [ 75 ]. This mutation has enabled SARS-CoV-2 to enter the human cells more easily due to its Q location [ 76 ].
3.2.8. P681H
The P681H mutation has been observed worldwide as of December 31, 2020 [ 77 ]. P681H results from a loss of proline and a gain of histidine containing imidazole. It also has mutations that result in cysteine residues. This potentially causes breakdown of the disulfide bridges in and around the RBD [ 77 ]. It is not thought to be associated with increased infection or spread, yet studies are ongoing [ 78 ].
3.2.9. E484Q
The E484Q mutation is caused by the change between glutamic acid (El) and glutamine (Q) at position 484. It causes an increase in ACE2 affinity in the B.1.617 double mutation strain seen in India [ 79 , 80 ].
3.2.10. K417
The K417 spike protein has been observed in several strains, mainly P.1 and B.1.351. This mutation is manifested as K417 N in the B.1.351 strain and as K417 T in the P.1 strain [ 80 , 81 ].
3.2.11. S477G/N
The S477 residue has the highest number of mutations in the RBD. It occurs as a result of amino acid changes at position 477. An increased binding affinity for hACE2 is observed with S477G and S477N, the two most frequently demonstrated mutations of S477 [ 82 ].
4. Some SARS-COV-2 variants recently associated with rapid spread
RNA viruses, one of which is SARS-CoV-2, are defined by a high mutation rate, one million times higher than their host. Viral mutagenic ability depends on several factors, including the quality of viral enzymes that replicate nucleic acids like RdRp. The mutation rate drives viral evolution and genome variability, thus allowing viruses to escape host immunity and hence develop drug resistance [ 83 ].
A number of SARS-CoV-2 variants have emerged worldwide since the COVID-19 outbreak. The fastest-spreading variants recently detected in UK, South Africa and Brazil have been the focus of attention ( Fig. 4 ). Scientists suspect that variants have the potential to affect certain mutation patterns, their infectivity, virulence and/or their ability to escape from parts of the immune system. Second, it could render vaccine-induced or naturally immune humans vulnerable to re-infection with the new variants to SARS-CoV-2, and such possible effects are still under investigation.

Countries with the fastest-spreading variants. B.1.1.7: Denmark, United States of America, France, Spain, Belgium, Netherlands, Italy, Switzerland, Ireland, Turkey, Israel, Portugal, Austria, Sweden, Australia, Finland, Germany, Norway, Nigeria, Slovakia, Ghana, India, Singapore, New Zealand, Jordan, Canada, Romania, Luxembourg, South Korea, Brazil, United Arab Emirates, Iceland, Poland, Czech Republic, Sri Lanka, Northern Macedonia, Saint Lucia, Aruba, Hong Kong, Thailand, Montenegro, Mexico, Ecuador, Bosnia and Herzegovina, Hungary, Latvia, Slovenia, Greece, Guadeloupe, Jamaica, Barbados, Kosovo, Bangladesh, Gambia, Cayman Islands, Republic of Serbia, Malaysia, Democratic Republic of the Congo, Taiwan, Pakistan, Peru, Iran, Argentina, Mayotte, Curaçao, Oman, Senegal, Kuwait, Dominican Republic, Trinidad and Tobago, South Africa, B.1.351: Mayotte, United Kingdom, Belgium, France, Netherlands, Switzerland, Mozambique, Botswana, Zambia, New Zealand, Australia, Austria, Denmark, United States of America, Turkey, Germany, Ireland, Israel, Kenya, Finland, Sweden, United Arab Emirates, Ghana, South Korea, Thailand, Spain, Canada, Portugal, Luxembourg, Singapore, Democratic Republic of the Congo, Italy, Norway, Panama, Bangladesh, P.1: Brazil, Switzerland, Colombia, Italy, Belgium, Japan, France, United States of America, Netherlands, French Guiana, Spain, South Korea, Mexico, Faroe Islands, Peru, B.1.525: Denmark, United Kingdom, Nigeria, United States of America, France, Canada, Ghana, Australia, Netherlands, Jordan, Singapore, Finland, Mayotte, Belgium, Spain. More than one mutant type is seen at once in the blackened countries or regions.
4.1. B.1.1.7, 20I/501Y.V1, VOC202012/01
The B.1.1.7 variant was first seen in UK and began to spread rapidly. After a short time, it was seen in particularly India, the Netherlands, Switzerland, France, Brazil, Finland, Belgium, Mexico, Bangladesh, Turkey, China (Bejing and Wuhan), South Korea, 62 European countries, Asia and UK [ 84 ]. The B.1.1.7 strain N5014, P681H, H69-V70 and Y144/145 have significant mutations in the deletion processes. The reason for this rapid spread is due to the N501Y mutation increasing the receptor binding affinity. The variant also has a deletion at positions 69 and 70 of the S protein [ 85 ]. Furthermore, the B.1.1.7 variant appears to have a 30 % higher mortality rate along with other variants of SARS-CoV-2 [ 86 ].
4.2. B.1.351, 20C/501Y.V2
The B.1.351 variant originated in South Africa. B.1.351 contains 9 S mutations in addition to those of D614G, including a cluster of mutations (e.g., 242-244del & R246I) in NTD, three mutations (K417N, E484K, & N501Y) in RBD, and one mutation (A701V) near the furin cleavage site [ 87 ]. There is a growing concern that these new variants could impair the efficacy of current monoclonal antibody (mAb) therapies or vaccines. This is mainly because many of the mutations reside in the antigenic supersite in NTD16,17 or in the ACE2-binding site (also known as the RBM) which is a major target of potent virus-neutralizing antibodies [ 88 ].
One of Brazil's detected variants of SARS-CoV-2 is the P.1 variant, a descendant of B.1.1.28. This a highly diverse variable, which includes the E484K, K417T and N501Y mutations, was identified in 42 % of the positive individuals [ 68 ]. Viruses that show co-mutations with the P.1 variant cause concern that they may carry a more infectious risk [ 89 ]. As a matter of fact, the inclusion of a common mutation allows it to be contaminated similar to the South African variant as well as to create more re-emerging risks.
This variant was first coined in the US in November 2020. It contains the mutations T95I, D253 G, L5F, S477N, E484K, D614G, A701V [ 90 ], spreads rapidly, and neutralization has been observed to be reduced in patients harboring this mutation [ 91 ].
4.5. B.1.525
The B.1.525 variant, which was first determined in December 2020 and identified in many countries, especially Denmark, is similar to the E484K, Q677H, F888L variants. In addition, B.1.525 is similar to the highly transferable variant B.1.1.7, which also occurs in UK, in that it includes the mutations S:69-70 and S:144 of B.1.1.7 (501Y.V1) [ 92 ]. However, further research is necessary to assess whether B.1.525 causes more contagiousness and more severe outcomes.
4.6. B.1.526
B.1.526 was first identified in New York [ 93 ]. This variant contains the mutations L5F, T95I, D253G, E484K, D614G and A701V [ 94 ]. This variant is thought to spread especially in countries with high seroprevalence. It poses a threat on therapeutic approaches because it harbors previously unseen S protein mutations. Moreover, inoculated plasma is shown to negatively affect the neutralization titer [ 95 ].
4.7. B.1.427/B.1.429
The variant B.1.427/B.1.429 first appeared in California. It spread rapidly in 25 countries in the US and onward [ 96 ]. The emergence of this mutation was triggered by the acquisition of the L452R mutation, which is markedly resistant to mAbs [ 97 , 98 ]. More research is needed to determine whether this variant, known as CAL20C, is more contagious than other forms of the virus.
4.8. B.1.617
Currently available in eight countries, the B.1.617 mutation was first seen in India in October 2020 [ 99 ]. It is the first strain where the E484Q and L425R mutations were first seen together. The effect of these mutations individually on SARS-CoV-2 is well known; however, the combined effect of these mutations still remains unknown [ 100 ].
4.9. B.1.1.298
First defined in June 2020 in a mink farm in Denmark [ 96 ], although it shows similar variations with the B.1.1.7 mutation, B.1.1.298 also contains the Y453F, I692V and M1229I mutations. Although it is reported as an escape mutation, it is seen in fewer people compared to other variants in the current situation, however it is a variant with a high mutation potential [ 101 ]. This variant has also been recently reported to cause a 4-fold increase in hACE2 affinity [ 102 ].
The P.3 variant occurs in South Africa, Brazil and the United Kingdom. It has also been reported recently in the Philippines [ 103 ]. Includes E484K, N501Y and P681H S mutations found in rapidly spreading variants such as B.1.351, P.1 and B.1.1.7 variants [ 104 , 105 ]. It is thought that it may have important effects with ACE2 receptor affinity and neutralizing antibodies in studies [ 106 ].
4.11. Lambda (C.37)
The lambda (C.37) variant, first seen in Peru in August 2020, was identified by the World Health Organization in June 2021 [107,108]. Later, it was seen in 26 countries, especially in America, Europe and Oceania [ 109 ]. C.37 variant B.1.1.7, B.1.351. and P.1 variants as a result of a deletion in the ORF1A gene [ 110 ]. It also harbors mutations Δ246-252, G75V, T76I, L452Q, F490S, D614G and T859N in the S protein. It spreads rapidly with a high prevalence [ 108 ]. This variant shows increased infectivity and immune evasion from antibodies [ 109 ] ( Table 4 ).
Comparison of the fastest-spreading variants.
5. Emergence and observation of CoV viral variants by country
Characterization of the genetic variants of SARS-CoV-2 is crucial for tracking and evaluating its spread across countries. Table 5 shows the variants of SARS-CoV-2 by country, and the changes and effects on the virus. The genomic variability of SARS-CoV-2 samples scattered around the world may be under geographically specific etiological influences. Continuous monitoring of mutations will also be crucial in tracking the movement of the virus between individuals and across geographic areas.
Coronavirus (CoV) mutations and effects by country. ( BCSIR: Bangladesh Council of Scientific and Industrial Research, NILMRC: National Institute of Laboratory Medicine and Referral Center).
After February 2020, it was observed that the viral genomes presented distinct point mutations were clearly discernible in different geographic regions. Three distinct repetitive mutations were detected in Europe and North America. The number and occurrence and the median value of virus point mutations recorded in Asia have increased over time [ 83 ]. It has been determined that the RdRp mutation at position 14408 in European viral genomes is associated with a larger number of point mutations compared to viral genomes from Asia.
Two clinical isolates from India were sequenced. Sequence analysis was performed on S protein of Indian isolates according to Chinese Wuhan isolates. Point mutations were identified in Indian isolates. One of the two isolates was found to harbor a mutation in the RBM at position 407. It has been determined that arginine (a positively charged amino acid) is replaced by isoleucine (hydrophobic amino acid) in this region. With this, a secondary change in the structure of the protein in the region has been demonstrated, and this could potentially alter the receptor binding of the virus [ 109 ].
However, given the small sample size, it is difficult to determine whether D614G is the dominant species in these countries. A recent report supports the high prevalence of D614G in Europe [ 121 ].
Three variants (H49Y, T573I and D614G) found in the Mexican population show multiple sequence alignments of SARS-CoV-2 S proteins. These variants are away from the RBD of the S protein. G614 is neutralized by a polyclonal antibody similar to D614. To date, this variant has become the dominant form, replacing the wild type (WT) according to the mutation levels in the world presented in the Nextstrain database.The H49Y variant is produced with the C/T change at the 21.707 positions. The properties of H/Y residues vary from positive to neutral charge, causing a reduction in total free energy, while D614G-substituted mutants exhibit stabilizing structure, suggesting a prevalent role in S protein evolution. Although these are minute changes due to the chemical nature of the substitution, they are expected to take place at the structural level [ 54 ].
Several common gene mutations have been observed in between the SARS-CoV-2 sequences in China. These mutations are common across countries and follow standard roles. Highlights are T4402C, G5062T, C8782T, C17373T, C20692T, T28144C, C29095T and G29868C. The T4402C mutation causing a silent mutation was recorded in the ORF1a/b gene segment. This mutation is frequently associated with the C8782T, G5062T and T28144C mutations. Similar T4402C and G5062T point mutations were observed in both, isolated in the South Korean strain [ 114 ], C8782T was the dominant mutation reported worldwide in the SARS-CoV-2 gene mutation [ 114 , 115 ]. This mutation is always associated with the ORF8 gene segment T28144C [ 117 ], coexisting with a missense point mutation. The C17373T silent mutation, which was noticed in Singapore and the US, was also observed in Wuhan [ 1 ]. C20692T was restricted to Wuhan and is present with the G29868C gene mutation of the 3′-terminal loop. The C29095T mutation of the gene coding the N protein has also been reported in the US [ 114 , 116 ].
In terms of mutation variants in the genes coding the structural proteins, typical to the European isolates, several additional mutations have been identified, including a synonym mutation in the gene M (C26750T), characteristic to the Russian isolates [ 122 ]. The double mutation, R203K and G204R, in the gene coding the N protein that had previously appeared in Europe began to spread, and quickly became dominant in Russia. The results show that the viral genome of most of the Russian isolates has evolved with the accumulation of new mutations associated with increased viral transmission. Generation of 20A seems to be one of the most common, showing the European origin of Russian isolates. This is based on mutational and phylogenetic analyses of the SARS-CoV-2 genomes isolated in Russia in March-April 2020. However, in Russia, unlike in Western Europe, the triple mutation - G28881A, G28882A and G28883C - which results in double substitution of R203K and G204R in the N protein, has spread and become the dominant form. Thus, by the end of April 2020, the double mutated R203K and G204R genome abundance was over 69.5 % and 32.6 % in Russia and in Europe, respectively [ 117 ].
In the US, the number of genomes belonging to the same subclass identified by the R203K and G204R mutations was even lower, accounting for 13.3 %. The observed variant was likely to to have emerged in Russia in early March 2020. Further spread of the variant was accompanied by the formation of new subtypes with accumulation of the characteristic mutations in the gene M (C26750T) or ORF1b (M1499I or G17964T), following subsequent divergence due to new single (mostly synonymous) mutations in the ORF1ab gene. The rapid spread of the variant with double mutations R203K and G204R in gene N may be indicative of its adaptability and ability to increase the transmission rate rather than modulate the virulence [ 117 ].
The sequencing of three SARS-CoV-2 genomes were reported in Bangladesh. Evidence reveals the first signs in Bangladesh in May-June 2020, followed by constant human-to-human transmission, thus leading to sampled infections. Compared to hCoV-19/Wuhan/WIV04/2019 for the BCSIR-NILMRC-006 strain, eight mutations were found, including Nsp2_G339S, N_R203K, N_G204R, Nsp3_Q172R, S_D614G, Nsp2_I120F, Nsp12_P323L. Six mutations were found in BCSIR-NILMRC-007, S_D614G, N_R203K, N_G204R, Nsp12_K59N, Nsp2_I120F and Nsp12_P323L. Genomic mutations S_D614G, N_R203K, N_G204R, NSP2_I120F, Nsp12_P323L, and Nsp3_P822S were observed in BCSIR-NILMRC-008. A unique mutation, Nsp2_V480I, was observed in the BCSIR-NILMRC-006 genome sequence compared to the genome sequences found in GISAID CoVsurver (GISAID Initiative_CoVsurver_files) [ 98 ].
According to mutation analysis, 59 of the 80 isolates from Turkey in the S protein 23.403A > G (D614G) signed contained the mutation, and this clearly manifested itself to be a frequent mutation (73 %). Most samples with the D614G mutation were strongly associated with two other mutations in the ORF1ab region (3037 C > T and 14.408C > T). These co-occurring mutations have recently been identified as being characteristic to one of the major SARS-CoV-2 variants occurring in Europe. It is assumed that the 14,408C > T (P4715 L) and 3037 C > T (F106 F) variants in ORF1ab occur at high frequency and are associated, resulting in mutations in RdRP/Nsp12 and Nsp3 gene. RdRP/Nsp12 is a key component of the replication/transcription mechanism, and therefore the leucine mutation at position 4715 of RdRP/Nsp12 could potentially affect its function. Moreover, the proline to leucine mutation has been consistently observed as a common mutation in Europe (51.6 %) and North America (58.1 %). C3037T, A23403G and C14408T are the most common mutations found in the isolates from Turkey (73 %) [ 112 ].
The three-dimensional crystaline structure of the s2m RNA element of the SARS-CoV-2 indicates that the mutated guanosine 19 in Australian isolates is critical in tertiary contacts to form an RNA base quartet containing two adjacent G-C pairs (G19, C20, G28 and C31). Since s2m plays an important role in viral RNA to replace host protein synthesis, it is assumed that the degradation of s2m can significantly alter viral viability or infectivity. The s2m sequence of CoVs is highly conserved, and spontaneous changes in this motif are likely due to recombination as mutation is not expected. Due to the high frequency of recombination events occurring in CoVs, RNA recombination can either improve the adaptation process to its new host, such as to humans, or cause unpredictable changes in virulence during infection [ 123 ].
The single amino acid mutation was observed in the virus’s main proteinase (M pro ) of the SARS-CoV-2 Vietnam isolate, R60C, and in the RdRp of the SARS-CoV-2 Indian isolate, A408 V. In silico findings have revelaed that both strains showed 2 mutations to reduce the stability of the protein. Molecular Dynamics (MD) simulation studies on M pro also confirmed that point mutation affects the stability of proteins and binding of the inhibitor. In silico studies found that the M pro catalytic active amino was found to be surrounded by a strand (142-145, 175-200), short helix (40-43, 46-50) and beta leaf regions (25-27, 164-167). The R60C mutant is found in the helix adjacent to the short helix (H2) forming the catalytic channel. A loss of conserved ionic interaction between arginine amide nitrogen and the carboxylic oxygen atom of aspartic acid at position 48 of the catalytic channel was observed [ 118 ].
In UK, the first variant to be investigated in December 2020 was named VUI-202012/01. According to a recent study, this variant is progressing faster than the other existing variants. Cases have been detected in approximately 60 different local government districts. Due to the S protein, changes in the binding properties to host ACE2 receptors can cause the SARS-CoV-2 virus to become more rapid in its spread among humans. The R-value for this variant is thought to be increased by 0.4, or 70 %. According to the data obtained so far, there is no evidence that this variant has a higher probability of causing serious illness or a higher mortality rate [ 119 ].
South Africa was the most severely affected region in Africa, with more than 56,000 extreme natural deaths (about 950 per million population) by December 2020. Three mutations of this new strain (K417 N, E484 K and N501Y) are in the key regions of the RBD. Two, E484 K and N501Y, are within the RBM, which is the main functional motif that interfaces with the hACE2 receptor. The N501Y mutation was recently identified in a new strain (B.1.1.7) in UK and there is some preliminary evidence that this may be more contagious. The E484K mutation is so rare that it is present in <0.02 % of sequences from outside of South Africa. E484 resides in the RBM and interacts with the K31 interaction hotspot residue of hACE2. This is the most striking difference in the RBD-hACE2 complex between SARS-CoV-2 and SARS-CoV, and benefits SARS-CoV-2′s improved binding affinity to hACE2. While all the effects of this new lineage in South Africa have yet to be determined, these findings highlight the importance of coordinated molecular surveillance systems around the world [ 120 ].
6. What the future holds
Since the SARS-CoV-2 virus first emerged, a wide variety of drug compounds affecting the binding sites of the virus have been being studied. Drug trials and vaccine studies are continuing. However, considering the frequency of mutation of the SARS-CoV-2 virus in all drug and vaccine studies, it is necessary to try multiple therapeutic combinations in different mutation types and to compare such studies, preventing possible pathways before the virus mutates. The lack of effective therapeutic and preventive strategies against hCoVs necessitates drug and treatment research. It has previously been shown that designing a broad-spectrum inhibitor in a conservative target is a viable method for developing anti-CoV therapeutics, given the high rates of mutation and recombination observed in viral replication.
The SARS-CoV-2/B.1.1.7 variant has been detected in the US and more than 30 countries, predominantly in England. The B.1.1.7 variant, which exhibits rapid growth and transmission, has the potential to affect healthcare, pandemic management and prevention. However, B.1.1.7, which is transmitted more efficiently than other SARS-CoV-2 variants, has been suggested to be a no neutralization escape variant for existing vaccines and infection. In addition, mAbs specific to the RBD showed full activity against the variant. However, all this shows that the development of SARS-CoV-2 and the emergence of new variants which serve for the immune system escape mechanism are becoming more likely. All this information indicates that our fight against SARS-CoV-2 may still continue in the next 10 years. Large-scale studies on different mutant types in various geographic regions around the world are not yet in the desired intensity. Conducting related studies in increased numbers will pave the way for the efficacy of therapeutic approaches to be developed for the virus in question. Different therapeutic approaches against SARS-CoV-2 have been shown according to different types of CoVs (SARS-CoV, MERS-CoV, etc.), which are similar to SARS-CoV-2, in terms of the location and effectiveness of variation.
If different types of viruses have different serological characteristics, a different vaccine for each subtype will be more effective in preventing COVID-19. Epidemiological studies should be conducted in different countries to understand the pathogenicity course of these subtypes.
The reason why the mutations in glycoprotein S lead to vaccine escape is related to the location of the mutation and the affinity of the protein. However, more evidence is necessary to better understand whether the variants will respond to the vaccines. It probably suggests a situation where we would have to give more than one vaccine, of which the options will possibly vary over time. At the same time, it can be said that variations should be mostly occuring in areas such as the RBD, and vaccines and antiviral drugs should be formulated by targeting more than one viral protein. With the current vaccine developments, antibodies are produced against many regions in the S protein. A single change is unlikely to make the vaccine less effective. However, this can happen as more mutations emerge over time.
Laboratory experiments will be necessary to understand if and how the genomic changes in SARS-CoV-2 may or may not be linked to increases in cases. Nevertheless, many studies have suggested that the new strain does not cause a more severe illness. We must practice active surveillance to detect changes in SARS-CoV-2 as they occur.
7. Discussion
It has been reported that 7 CoVs, including SARS-CoV-2, infect humans in the CoV family with a +ssRNA genome of approximately 30 kb. The rest are SARS-CoV, MERS-CoV, hCoV-NL63, hCoV-229E, hCoV-HKU1 and hCoV-OC43. When the percentage (%) similarity in the sequencing of SARS-CoV, MERS-CoV, hCoV-HKU1 and hCoV-OC43 proteins with SARS-CoV-2 proteins is examined, it is understood that the strain with the highest similarity to SARS-CoV-2 is SARS-CoV.
The S glycoprotein RBD is a critical determinant for viral tropism and infectivity. Mutations in this region will change the affinity of the RBD and show the different infective consequences of the strains. The fact that the most variable region of the CoV family is the RBD causes different strains to emerge and such strains already show different infective profiles. The binding of the SARS-CoV-2 S protein with a high affinity to the ACE-2 receptor is a result of natural selection.
The excess of SARS-CoV-2 S mutations poses a great difficulty in the SARS-CoV-2 targeted therapy and vaccination processes. Mutations, which are one of the largest obstacles in the development of antiviral drug and vaccine formulations, have a crucial role in the preparation, administration and follow-up of vaccines and antiviral drugs.
RNA viruses that exhibit a higher mutation rate than what the host allows them, may escape host immunity and develop drug resistance. This mutation rate drives viral evolution and genome change. Clearly distinguishable mutations of viral genomes have emerged in different geographies. The presence of such mutations is supported by clinical findings. The D614G, S943P and V483a mutations, viral protein mutants, and the emergence of viral strains due to block mutation, play an important role in CoV evolution. Recombination contributes significantly to the viral evolution in the current pandemic. Since viruses mutate during replication, the effect of the antibody concentration produced prior to infection can also be lost. A single amino acid change associated with the mutation rate is effective in the emergence of a new variant with the same epitope. Also, the increase or decrease of hydrogen bonds in receptor interactions is associated with changes in affinity.
The presence of the SARS-CoV-2 strains can be attributed to the heterogeneity of the COVID-19 cases in different regions. Analysis with genomic sequencing has shown that SARS-CoV-2 has transformed into a less contagious strain that affects a number of COVID-19 cases in different regions. The time when different SARS-CoV-2 strains become dominant in a country or a region may indicate the time it will need to overcome the peak of COVID-19 cases. Prospective epidemiological studies of the strains should be conducted to confirm these assumptions. To modulate virus pathogenicity, potential drugs targeting that site can be designed depending on the localization of a given mutation.
Contributors’ statement
All authors contributed to the study conception and design, while Pelin KILIC additionally conducted the overall supervision of the review. Material preparation, data collection and analysis were performed by Begum COSAR, Zeynep Yagmur KARAGULLEOGLU, Sinan UNAL, Ahmet Turan INCE, Dilruba Beyza UNCUOGLU, Gizem TUNCER, Bugrahan Regaip KILINC, Yunus Emre OZKAN, Hikmet Ceyda OZKOC, Ibrahim Naki DEMIR, Ali EKER, Feyzanur KARAGOZ, Said Yasin SIMSEK, Bunyamin YASAR, Mehmetcan PALA, Aysegul DEMIR, Irem Naz ATAK, Aysegul Hanife MENDI, Vahdi Umut BENGI, Guldane CENGIZ SEVAL, Evrim GUNES ALTUNTAS, Devrim DEMIR-DORA and Pelin KILIC, and all authors commented on previous versions of the manuscript. All authors read and approved the final manuscript.
Funding statement
The authors declare no specific funding for this work
Compliance with ethical standards
Not applicable.
Consent to participate
Consent for publication, declaration of competing interest.
The authors declare there are no competing interests.
Acknowledgements
The preparation of this review was supported by the Scientific and Technological Research Council of Turkey (TÜBİTAK), Project # 18AG020 and TÜBİTAK Intern Researchers (STAR) Program #2247-C.

Dr. Devrim Demir Dora has graduated from Hacettepe University Faculty of Pharmacy in 1995 and got her Msc and PhD degree from Hacettepe University Graduate School of Health Sciences Pharmaceutical Biotechnology Program. She has worked as a Research Assistant at Hacettepe University Faculty of Pharmacy, Pharmaceutical Biotechnology Department from 1996 to 2005. Between 2005 and 2007, she has worked at Turkish Ministry of Health, General Directorate of Pharmaceuticals and Pharmacy, Quality Control Department, and she has participated in GMP Inspection team especially for the biotechnology derived products. She has worked as an Assistant Professor between 2007 and 2010 at Ege University Faculty of Pharmacy Department of Pharmaceutical Biotechnology and since 2010 at Akdeniz University Faculty of Medicine Department of Medical Pharmacology, Medical Biotechnology and Gene and Cell Therapy. Besides her academic duties, Dr. Demir Dora has been worked as ‘Advisory Board Member’ for approval of biotechnological/biosimilar medicinal products since 2009 at Turkish Medicines and Medical Devices Agency. Her areas of interest are recombinant protein production, regulation of biotechnological and biosimilar products, development of biopharmaceuticals, nanotechnology, advanced therapy medicinal products, gene therapy medicinal products, development of non viral nucleic acid delivery systems for gene therapy, cancer therapy, bacterial transformation, quorum sensing mechanism and genetic competence. She has ‘Bacterial Transformation Kit’ patent and three patent applications about non-viral gene delivery system for treatment of breast cancer and pseudomonas infection.
share this!
May 13, 2024
This article has been reviewed according to Science X's editorial process and policies . Editors have highlighted the following attributes while ensuring the content's credibility:
fact-checked
peer-reviewed publication
trusted source
Genetic analyses reveal new viruses on the horizon
by German Cancer Research Center
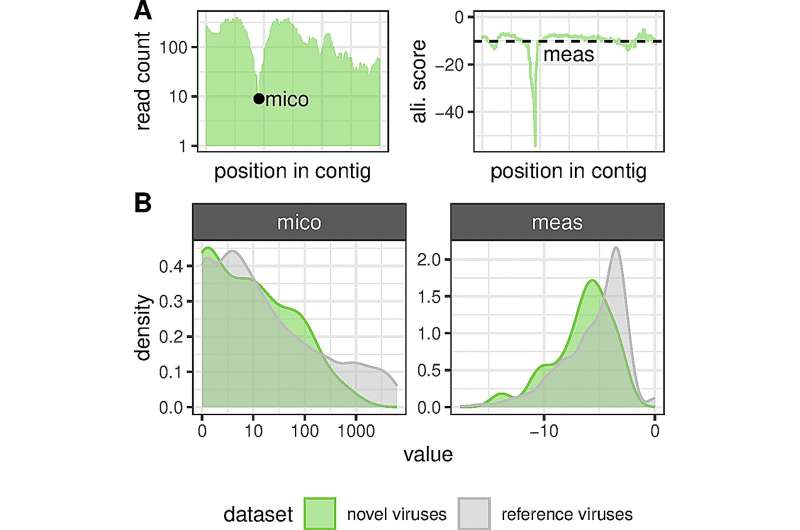
Suddenly they appear, and like the SARS-CoV-2 coronavirus, can trigger major epidemics: Viruses that nobody had on their radar. They are not really new, but they have changed genetically. In particular, the exchange of genetic material between different virus species can lead to the sudden emergence of threatening pathogens with significantly altered characteristics.
This is suggested by current genetic analyses carried out by an international team of researchers. Virologists from the German Cancer Research Center (DKFZ) were in charge of the large-scale study, published in the journal PLOS Pathogens .
"Using a new computer-assisted analysis method, we discovered 40 previously unknown nidoviruses in various vertebrates from fish to rodents, including 13 coronaviruses," reports DKFZ group leader Stefan Seitz. With the help of high-performance computers, the research team, which also includes Chris Lauber's working group from the Helmholtz Center for Infection Research in Hanover, has sifted through almost 300,000 data sets . According to virologist Seitz, the fact that we can now analyze such huge amounts of data at once opens up completely new perspectives.
Virus research is still in its relative infancy. Only a fraction of all viruses occurring in nature are known, especially those that cause diseases in humans, domestic animals and crops. The new method therefore promises a quantum leap in knowledge with regard to the natural virus reservoir. Stefan Seitz and his colleagues sent genetic data from vertebrates stored in scientific databases through their high-performance computers with new questions. They searched for virus-infected animals in order to obtain and study viral genetic material on a large scale. The main focus was on so-called nidoviruses, which include the coronavirus family.
Nidoviruses, whose genetic material consists of RNA (ribonucleic acid), are widespread in vertebrates. This species-rich group of viruses has some common characteristics that distinguish them from all other RNA viruses and document their relationship. Otherwise, however, nidoviruses are very different from each other, i.e., in terms of the size of their genome.
One discovery is particularly interesting with regard to the emergence of new viruses: In host animals that are simultaneously infected with different viruses, a recombination of viral genes can occur during virus replication.
"Apparently, the nidoviruses we discovered in fish frequently exchange genetic material between different virus species, even across family boundaries," says Seitz. And when distant relatives "crossbreed," this can lead to the emergence of viruses with completely new properties. According to Seitz, such evolutionary leaps can affect the aggressiveness and dangerousness of the viruses, but also their attachment to certain host animals.
"A genetic exchange, as we have found in fish viruses, will probably also occur in mammalian viruses," explains Seitz. Bats, which—like shrews—are often infected with a large number of different viruses, are considered a true melting pot. The SARS-CoV-2 coronavirus probably also developed in bats and jumped from there to humans.
After gene exchange between nidoviruses, the spike protein with which the viruses dock onto their host cells often changes. Chris Lauber, first author of the study, was able to show this by means of family tree analyses. Modifying this anchor molecule can significantly change the properties of the viruses to their advantage—by increasing their infectiousness or enabling them to switch hosts.
A change of host, especially from animals to humans, can greatly facilitate the spread of the virus, as the corona pandemic has emphatically demonstrated. Viral "game-changers" can suddenly appear at any time, becoming a massive threat, and—if push comes to shove—triggering a pandemic. The starting point can be a single double-infected host animal.
The new high-performance computer process could help to prevent the spread of new viruses. It enables a systematic search for virus variants that are potentially dangerous for humans, explains Seitz.
The DKFZ researcher sees another important possible application with regard to his special field of research, virus-associated carcinogenesis: "I could imagine that we could use the new High Performance Computing (HPC) to systematically examine cancer patients or immunocompromised people for viruses. We know that cancer can be triggered by viruses, the best-known example being human papillomaviruses. But we are probably only seeing the tip of the iceberg so far. The HPC method offers the opportunity to track down viruses that, previously undetected, nestle in the human organism and increase the risk of malignant tumors."
Journal information: PLoS Pathogens
Provided by German Cancer Research Center
Explore further
Feedback to editors

A devastating fire 2,200 years ago preserved a moment of life and war in Iron Age Spain, down to a single gold earring
2 hours ago

Airborne technology brings new hope to map shallow aquifers in Earth's most arid deserts
8 hours ago

First-generation medical students face unique challenges and need more targeted support, say researchers
9 hours ago

Thermoelectric materials approach boosts band convergence to avoid time-consuming trial-and-error approach

Ion swap dramatically improves performance of CO₂-defeating catalyst
10 hours ago

Military rank affects medical care, offering societal insights: Study

Mystery CRISPR unlocked: A new ally against antibiotic resistance?
11 hours ago

Researchers develop a detector for continuously monitoring toxic gases
12 hours ago

Sea otter study finds tool use allows access to larger prey, reduces tooth damage

Accelerated discovery research unveils 21 novel materials for advanced organic solid-state laser technology
Relevant physicsforums posts, and now, here comes covid-19 version ba.2, ba.4, ba.5,....
3 hours ago
Is it usual for vaccine injection site to hurt again during infection?
13 hours ago
A Brief Biography of Dr Virgina Apgar, creator of the baby APGAR test
May 12, 2024
Who chooses official designations for individual dolphins, such as FB15, F153, F286?
May 9, 2024
The Cass Report (UK)
May 1, 2024
Is 5 milliamps at 240 volts dangerous?
Apr 29, 2024
More from Biology and Medical
Related Stories

Cross-species virus transmission found in several species of small furry animals
Sep 25, 2023

Genome study shows humans pass more viruses to animals than we catch from them
Mar 25, 2024

Virus ancestry could help predict next pandemic
Feb 5, 2024

Bats in Switzerland harbor diverse viruses, some potentially zoonotic
Jun 16, 2021

Q&A: Pork, pathogens and progress—a close look at PRRSV research
Feb 16, 2024

Ancient origins of viruses discovered
Apr 4, 2018
Recommended for you

Protein prediction technology yields accurate results to efficiently find the best drug candidate for many conditions

Researchers discover new family of bacteria with high pharmaceutical potential
17 hours ago

Study highlights pathoblockers as a future alternative to antibiotics
14 hours ago

Research identifies mechanism behind drug resistance in malaria parasite
Let us know if there is a problem with our content.
Use this form if you have come across a typo, inaccuracy or would like to send an edit request for the content on this page. For general inquiries, please use our contact form . For general feedback, use the public comments section below (please adhere to guidelines ).
Please select the most appropriate category to facilitate processing of your request
Thank you for taking time to provide your feedback to the editors.
Your feedback is important to us. However, we do not guarantee individual replies due to the high volume of messages.
E-mail the story
Your email address is used only to let the recipient know who sent the email. Neither your address nor the recipient's address will be used for any other purpose. The information you enter will appear in your e-mail message and is not retained by Phys.org in any form.
Newsletter sign up
Get weekly and/or daily updates delivered to your inbox. You can unsubscribe at any time and we'll never share your details to third parties.
More information Privacy policy
Donate and enjoy an ad-free experience
We keep our content available to everyone. Consider supporting Science X's mission by getting a premium account.
E-mail newsletter
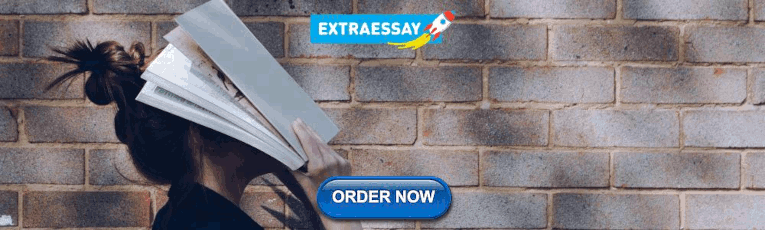
IMAGES
VIDEO
COMMENTS
Advancements in genetic research from 1977 to 2023 have enabled high-resolution variant identification, large-scale DNA sequencing, cell-type-specific regulation understanding and breakthroughs in ...
The discovery of mutations associated with human genetic disease is an exercise in comparative genomics (see Glossary). Although there are many different strategies and approaches, the central premise is that affected persons harbor a significant excess of pathogenic DNA variants as compared with a group of unaffected persons (controls) that is ...
Genomic research has evolved from seeking to understand the fundamentals of the human genetic code to examining the ways in which this code varies among people, and then applying this knowledge to ...
Genome sequences from diverse human groups are needed to understand the structure of genetic variation in our species and the history of, and relationships between, different populations. We present 929 high-coverage genome sequences from 54 diverse human populations, 26 of which are physically phased using linked-read sequencing.
Additional large-scale variations include balanced inversions (average of 18 Mb) and complex, difficult-to-resolve alterations. Collectively, ~1% of an individual's genome will differ from the human reference sequence. When comparing across a generation, fewer than 100 new genetic variants are typically detected in the euchromatic portion of ...
1 Introduction. Genetic variation is a key contributor to health and disease, and understanding the link between an individual's genotype and the corresponding phenotype is a major goal of genetic research (Genomes Project et al., 2010).Whole genome sequencing (WGS) within and across populations enables highly efficient variant discovery and elucidation of the molecular nature of virtually ...
1. Genetic variations. History of studies in genetic variations is not so long. The first DNA-based genetic variation was reported by Kan and Dozy in 1978. 1) They found a polymorphism of DNA sequence adjacent to human β-globin gene. Until the PCR (polymerase chain reaction) method was established, DNA polymorphisms were detected by a combination of DNA restriction enzymes and Southern ...
The genetic diversity found in the 520 known nonhuman primate species is the result of ongoing natural experiments on genetic variation that have been running uninterrupted for millions of years. Today, more than 60% of primate species on Earth are threatened with extinction in the next decade as a result of man-made factors . We must decide ...
Modern DNA sequencing technologies enable geneticists to rapidly identify genetic variation among many human genomes. However, isolating the minority of variants underlying disease remains an important, yet formidable challenge for medical genetics. We have developed GEMINI (GEnome MINIng), a flexible software package for exploring all forms of human genetic variation. Unlike existing tools ...
Science. For the past 20 years, the human reference genome (GRCh38) has served as the bedrock of human genetics and genomics ( 1 - 3 ). One of the central applications of the human reference genome, and of reference genomes in general, has been to serve as a substrate for clinical, comparative, and population genomic analyses.
Comparison of clinical geneticist and computer visual attention in assessing genetic conditions. Understanding AI, specifically Deep Learning, in facial diagnostics for genetic conditions can enhance the design and utilization of AI tools, facilitating more meaningful interactions between clinicians and AI technologies. Image credit: pgen.1011168.
research can be required in order to identify the variants underlying both mendelian and complex genetic traits (see video, available at NEJM.org). For example, X-linked color blindness is a well ...
Darwin's theory of evolution through selection very well explains changes in time of heritable phenotypes. In the early 1900s, focusing on the evolution of genetic variants in the population, R. A. Fisher, S. Wright, and J. B. S. Haldane made fundamental theoretical contributions to population genetics (Provine 1971), Fisher in his 1922 paper (Fisher 1922), which was the first to introduce ...
The remarkable growth of genome-wide association studies (GWAS) has created a critical need to experimentally validate the disease-associated variants, 90% of which involve non-coding variants. To determine how the field is addressing this urgent need, we performed a comprehensive literature review identifying 36,676 articles. These were reduced to 1454 articles through a set of filters using ...
In the early 1900s, focusing on the evolution of genetic variants in the population, R. A. Fisher, S. Wright, and J. B. S. Haldane made fundamental theoretical contributions to population genetics (Provine 1971), Fisher in his 1922 paper (Fisher 1922), which was the first to introduce diffusion equations into population genetics, and Haldane in ...
We used information on traditional risk factors and 10 common genetic variants associated with breast cancer in 5590 case subjects and 5998 control subjects, 50 to 79 years of age, from four U.S ...
New analyses show that trait variability links evolution across vastly different timescales
The new research shows that individuals who carry the V142I transthyretin variant are at significantly increased risk for heart failure beginning in their 60s, with an increased risk for death ...
May 6, 2024 Updated 12:19 p.m. ET. Scientists are proposing a new way of understanding the genetics of Alzheimer's that would mean that up to a fifth of patients would be considered to have a ...
Characterization of the genetic variants of SARS-CoV-2 is crucial for tracking and evaluating its spread across countries. ... She has worked as a Research Assistant at Hacettepe University Faculty of Pharmacy, Pharmaceutical Biotechnology Department from 1996 to 2005. ... Choe H., Farzan M. JBC Papers in Press; 2003. A 193-amino-acid Fragment ...
Virologists from the German Cancer Research Center (DKFZ) were in charge of the large-scale study, published in the journal PLOS Pathogens. "Using a new computer-assisted analysis method, we ...