Advertisement
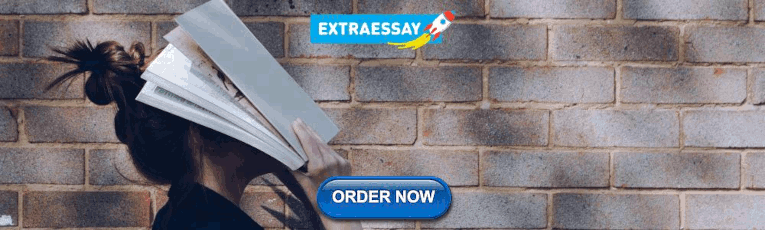
Critical Values Robust to P-hacking
- Cite Icon Cite
- Open the PDF for in another window
- Permissions
- Article contents
- Figures & tables
- Supplementary Data
- Peer Review
- Search Site
Adam McCloskey , Pascal Michaillat; Critical Values Robust to P-hacking. The Review of Economics and Statistics 2024; doi: https://doi.org/10.1162/rest_a_01456
Download citation file:
- Ris (Zotero)
- Reference Manager
P-hacking is prevalent in reality but absent from classical hypothesis-testing theory. We therefore build a model of hypothesis testing that accounts for p-hacking. From the model, we derive critical values such that, if they are used to determine significance, and if p-hacking adjusts to the new significance standards, spurious significant results do not occur more often than intended. Because of p-hacking, such robust critical values are larger than classical critical values. In the model calibrated to medical science, the robust critical value is the classical critical value for the same test statistic but with one fifth of the significance level.
Article PDF first page preview
Supplementary data, email alerts, related articles, affiliations.
- Online ISSN 1530-9142
- Print ISSN 0034-6535
A product of The MIT Press
Mit press direct.
- About MIT Press Direct
Information
- Accessibility
- For Authors
- For Customers
- For Librarians
- Direct to Open
- Open Access
- Media Inquiries
- Rights and Permissions
- For Advertisers
- About the MIT Press
- The MIT Press Reader
- MIT Press Blog
- Seasonal Catalogs
- MIT Press Home
- Give to the MIT Press
- Direct Service Desk
- Terms of Use
- Privacy Statement
- Crossref Member
- COUNTER Member
- The MIT Press colophon is registered in the U.S. Patent and Trademark Office
This Feature Is Available To Subscribers Only
Sign In or Create an Account

Want to create or adapt books like this? Learn more about how Pressbooks supports open publishing practices.
1.3 The Economists’ Tool Kit
Learning objectives.
- Explain how economists test hypotheses, develop economic theories, and use models in their analyses.
- Explain how the all-other-things unchanged (ceteris paribus) problem and the fallacy of false cause affect the testing of economic hypotheses and how economists try to overcome these problems.
- Distinguish between normative and positive statements.
Economics differs from other social sciences because of its emphasis on opportunity cost, the assumption of maximization in terms of one’s own self-interest, and the analysis of choices at the margin. But certainly much of the basic methodology of economics and many of its difficulties are common to every social science—indeed, to every science. This section explores the application of the scientific method to economics.
Researchers often examine relationships between variables. A variable is something whose value can change. By contrast, a constant is something whose value does not change. The speed at which a car is traveling is an example of a variable. The number of minutes in an hour is an example of a constant.
Research is generally conducted within a framework called the scientific method , a systematic set of procedures through which knowledge is created. In the scientific method, hypotheses are suggested and then tested. A hypothesis is an assertion of a relationship between two or more variables that could be proven to be false. A statement is not a hypothesis if no conceivable test could show it to be false. The statement “Plants like sunshine” is not a hypothesis; there is no way to test whether plants like sunshine or not, so it is impossible to prove the statement false. The statement “Increased solar radiation increases the rate of plant growth” is a hypothesis; experiments could be done to show the relationship between solar radiation and plant growth. If solar radiation were shown to be unrelated to plant growth or to retard plant growth, then the hypothesis would be demonstrated to be false.
If a test reveals that a particular hypothesis is false, then the hypothesis is rejected or modified. In the case of the hypothesis about solar radiation and plant growth, we would probably find that more sunlight increases plant growth over some range but that too much can actually retard plant growth. Such results would lead us to modify our hypothesis about the relationship between solar radiation and plant growth.
If the tests of a hypothesis yield results consistent with it, then further tests are conducted. A hypothesis that has not been rejected after widespread testing and that wins general acceptance is commonly called a theory . A theory that has been subjected to even more testing and that has won virtually universal acceptance becomes a law . We will examine two economic laws in the next two chapters.
Even a hypothesis that has achieved the status of a law cannot be proven true. There is always a possibility that someone may find a case that invalidates the hypothesis. That possibility means that nothing in economics, or in any other social science, or in any science, can ever be proven true. We can have great confidence in a particular proposition, but it is always a mistake to assert that it is “proven.”
Models in Economics
All scientific thought involves simplifications of reality. The real world is far too complex for the human mind—or the most powerful computer—to consider. Scientists use models instead. A model is a set of simplifying assumptions about some aspect of the real world. Models are always based on assumed conditions that are simpler than those of the real world, assumptions that are necessarily false. A model of the real world cannot be the real world.
We will encounter our first economic model in Chapter 35 “Appendix A: Graphs in Economics” . For that model, we will assume that an economy can produce only two goods. Then we will explore the model of demand and supply. One of the assumptions we will make there is that all the goods produced by firms in a particular market are identical. Of course, real economies and real markets are not that simple. Reality is never as simple as a model; one point of a model is to simplify the world to improve our understanding of it.
Economists often use graphs to represent economic models. The appendix to this chapter provides a quick, refresher course, if you think you need one, on understanding, building, and using graphs.
Models in economics also help us to generate hypotheses about the real world. In the next section, we will examine some of the problems we encounter in testing those hypotheses.
Testing Hypotheses in Economics
Here is a hypothesis suggested by the model of demand and supply: an increase in the price of gasoline will reduce the quantity of gasoline consumers demand. How might we test such a hypothesis?
Economists try to test hypotheses such as this one by observing actual behavior and using empirical (that is, real-world) data. The average retail price of gasoline in the United States rose from an average of $2.12 per gallon on May 22, 2005 to $2.88 per gallon on May 22, 2006. The number of gallons of gasoline consumed by U.S. motorists rose 0.3% during that period.
The small increase in the quantity of gasoline consumed by motorists as its price rose is inconsistent with the hypothesis that an increased price will lead to an reduction in the quantity demanded. Does that mean that we should dismiss the original hypothesis? On the contrary, we must be cautious in assessing this evidence. Several problems exist in interpreting any set of economic data. One problem is that several things may be changing at once; another is that the initial event may be unrelated to the event that follows. The next two sections examine these problems in detail.
The All-Other-Things-Unchanged Problem
The hypothesis that an increase in the price of gasoline produces a reduction in the quantity demanded by consumers carries with it the assumption that there are no other changes that might also affect consumer demand. A better statement of the hypothesis would be: An increase in the price of gasoline will reduce the quantity consumers demand, ceteris paribus. Ceteris paribus is a Latin phrase that means “all other things unchanged.”
But things changed between May 2005 and May 2006. Economic activity and incomes rose both in the United States and in many other countries, particularly China, and people with higher incomes are likely to buy more gasoline. Employment rose as well, and people with jobs use more gasoline as they drive to work. Population in the United States grew during the period. In short, many things happened during the period, all of which tended to increase the quantity of gasoline people purchased.
Our observation of the gasoline market between May 2005 and May 2006 did not offer a conclusive test of the hypothesis that an increase in the price of gasoline would lead to a reduction in the quantity demanded by consumers. Other things changed and affected gasoline consumption. Such problems are likely to affect any analysis of economic events. We cannot ask the world to stand still while we conduct experiments in economic phenomena. Economists employ a variety of statistical methods to allow them to isolate the impact of single events such as price changes, but they can never be certain that they have accurately isolated the impact of a single event in a world in which virtually everything is changing all the time.
In laboratory sciences such as chemistry and biology, it is relatively easy to conduct experiments in which only selected things change and all other factors are held constant. The economists’ laboratory is the real world; thus, economists do not generally have the luxury of conducting controlled experiments.
The Fallacy of False Cause
Hypotheses in economics typically specify a relationship in which a change in one variable causes another to change. We call the variable that responds to the change the dependent variable ; the variable that induces a change is called the independent variable . Sometimes the fact that two variables move together can suggest the false conclusion that one of the variables has acted as an independent variable that has caused the change we observe in the dependent variable.
Consider the following hypothesis: People wearing shorts cause warm weather. Certainly, we observe that more people wear shorts when the weather is warm. Presumably, though, it is the warm weather that causes people to wear shorts rather than the wearing of shorts that causes warm weather; it would be incorrect to infer from this that people cause warm weather by wearing shorts.
Reaching the incorrect conclusion that one event causes another because the two events tend to occur together is called the fallacy of false cause . The accompanying essay on baldness and heart disease suggests an example of this fallacy.
Because of the danger of the fallacy of false cause, economists use special statistical tests that are designed to determine whether changes in one thing actually do cause changes observed in another. Given the inability to perform controlled experiments, however, these tests do not always offer convincing evidence that persuades all economists that one thing does, in fact, cause changes in another.
In the case of gasoline prices and consumption between May 2005 and May 2006, there is good theoretical reason to believe the price increase should lead to a reduction in the quantity consumers demand. And economists have tested the hypothesis about price and the quantity demanded quite extensively. They have developed elaborate statistical tests aimed at ruling out problems of the fallacy of false cause. While we cannot prove that an increase in price will, ceteris paribus, lead to a reduction in the quantity consumers demand, we can have considerable confidence in the proposition.
Normative and Positive Statements
Two kinds of assertions in economics can be subjected to testing. We have already examined one, the hypothesis. Another testable assertion is a statement of fact, such as “It is raining outside” or “Microsoft is the largest producer of operating systems for personal computers in the world.” Like hypotheses, such assertions can be demonstrated to be false. Unlike hypotheses, they can also be shown to be correct. A statement of fact or a hypothesis is a positive statement .
Although people often disagree about positive statements, such disagreements can ultimately be resolved through investigation. There is another category of assertions, however, for which investigation can never resolve differences. A normative statement is one that makes a value judgment. Such a judgment is the opinion of the speaker; no one can “prove” that the statement is or is not correct. Here are some examples of normative statements in economics: “We ought to do more to help the poor.” “People in the United States should save more.” “Corporate profits are too high.” The statements are based on the values of the person who makes them. They cannot be proven false.
Because people have different values, normative statements often provoke disagreement. An economist whose values lead him or her to conclude that we should provide more help for the poor will disagree with one whose values lead to a conclusion that we should not. Because no test exists for these values, these two economists will continue to disagree, unless one persuades the other to adopt a different set of values. Many of the disagreements among economists are based on such differences in values and therefore are unlikely to be resolved.
Key Takeaways
- Economists try to employ the scientific method in their research.
- Scientists cannot prove a hypothesis to be true; they can only fail to prove it false.
- Economists, like other social scientists and scientists, use models to assist them in their analyses.
- Two problems inherent in tests of hypotheses in economics are the all-other-things-unchanged problem and the fallacy of false cause.
- Positive statements are factual and can be tested. Normative statements are value judgments that cannot be tested. Many of the disagreements among economists stem from differences in values.
Look again at the data in Table 1.1 “LSAT Scores and Undergraduate Majors” . Now consider the hypothesis: “Majoring in economics will result in a higher LSAT score.” Are the data given consistent with this hypothesis? Do the data prove that this hypothesis is correct? What fallacy might be involved in accepting the hypothesis?
Case in Point: Does Baldness Cause Heart Disease?
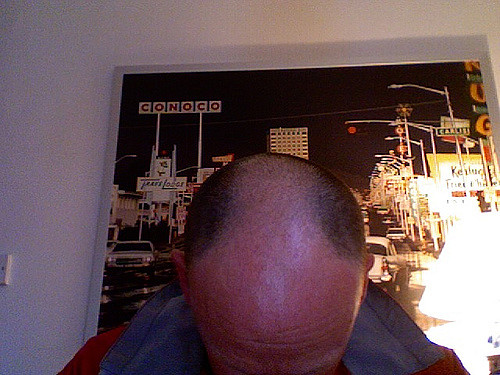
Mark Hunter – bald – CC BY-NC-ND 2.0.
A website called embarrassingproblems.com received the following email:
What did Dr. Margaret answer? Most importantly, she did not recommend that the questioner take drugs to treat his baldness, because doctors do not think that the baldness causes the heart disease. A more likely explanation for the association between baldness and heart disease is that both conditions are affected by an underlying factor. While noting that more research needs to be done, one hypothesis that Dr. Margaret offers is that higher testosterone levels might be triggering both the hair loss and the heart disease. The good news for people with early balding (which is really where the association with increased risk of heart disease has been observed) is that they have a signal that might lead them to be checked early on for heart disease.
Source: http://www.embarrassingproblems.com/problems/problempage230701.htm .
Answer to Try It! Problem
The data are consistent with the hypothesis, but it is never possible to prove that a hypothesis is correct. Accepting the hypothesis could involve the fallacy of false cause; students who major in economics may already have the analytical skills needed to do well on the exam.
Principles of Economics Copyright © 2016 by University of Minnesota is licensed under a Creative Commons Attribution-NonCommercial-ShareAlike 4.0 International License , except where otherwise noted.
ORIGINAL RESEARCH article
Integrating non-renewable energy consumption, geopolitical risks, economic development with the ecological intensity of well-being: evidence from quantile regression analysis provisionally accepted.
- 1 COMSATS University, Islamabad Campus, Pakistan
- 2 Medgar Evers College, United States
The final, formatted version of the article will be published soon.
This study delves into the intricate relationship between non-renewable energy sources, economic advancement, and the ecological footprint of well-being in Pakistan, spanning the years from 1980 to 2021. Employing the quantile regression model, we analyzed the co-integrating dynamics among the variables under scrutiny. Non-renewable energy sources were dissected into four distinct components-namely, gas, electricity, and oil consumption-facilitating a granular examination of their impacts. Our empirical investigations reveal that coal, gas, and electricity consumption exhibit a negative correlation with the ecological footprint of well-being. Conversely, coal consumption and overall energy consumption show a positive association with the ecological footprint of well-being.Additionally, the study underscores the detrimental impact of geopolitical risks on the ecological footprint of well-being. Our findings align with the Environmental Kuznets Curve (EKC) hypothesis, positing that environmental degradation initially surges with economic development, subsequently declining as a nation progresses economically. Consequently, our research advocates for Pakistan's imperative to prioritize the adoption of renewable energy sources as it traverses its developmental trajectory. This strategic pivot towards renewables, encompassing hydroelectric, wind, and solar energy, not only seeks to curtail environmental degradation but also endeavors to foster a cleaner and safer ecological milieu.
Keywords: Non-renewable energy consumption, environmental kuznet hypothesis, Geopolitical risks, Economic Development, Pakistan
Received: 26 Feb 2024; Accepted: 10 May 2024.
Copyright: © 2024 Khurshid, Egbe and Akram. This is an open-access article distributed under the terms of the Creative Commons Attribution License (CC BY) . The use, distribution or reproduction in other forums is permitted, provided the original author(s) or licensor are credited and that the original publication in this journal is cited, in accordance with accepted academic practice. No use, distribution or reproduction is permitted which does not comply with these terms.
* Correspondence: Mx. Nabila Khurshid, COMSATS University, Islamabad Campus, Islamabad, Pakistan
People also looked at
What Is the Purpose of Economic Theory?
Mainstream economists believe that economic theory is valid when it “predicts” economic actions or trends. Austrian economists, however, say that the purpose of economic theory is to explain economic events.
Order a free copy of Murray Rothbard’s What Has Government Done to Our Money? at Mises.org/Money .
Original Article: What Is the Purpose of Economic Theory?
The Mises Institute is a non-profit organization that exists to promote teaching and research in the Austrian School of economics, individual freedom, honest history, and international peace, in the tradition of Ludwig von Mises and Murray N. Rothbard.
Non-political, non-partisan, and non-PC, we advocate a radical shift in the intellectual climate, away from statism and toward a private property order. We believe that our foundational ideas are of permanent value, and oppose all efforts at compromise, sellout, and amalgamation of these ideas with fashionable political, cultural, and social doctrines inimical to their spirit.
1.3 How Economists Use Theories and Models to Understand Economic Issues
Learning objectives.
By the end of this section, you will be able to:
- Interpret a circular flow diagram
- Explain the importance of economic theories and models
- Describe goods and services markets and labor markets
John Maynard Keynes (1883–1946), one of the greatest economists of the twentieth century, pointed out that economics is not just a subject area but also a way of thinking. Keynes ( Figure 1.6 ) famously wrote in the introduction to a fellow economist’s book: “[Economics] is a method rather than a doctrine, an apparatus of the mind, a technique of thinking, which helps its possessor to draw correct conclusions.” In other words, economics teaches you how to think, not what to think.
Watch this video about John Maynard Keynes and his influence on economics.
Economists see the world through a different lens than anthropologists, biologists, classicists, or practitioners of any other discipline. They analyze issues and problems using economic theories that are based on particular assumptions about human behavior. These assumptions tend to be different than the assumptions an anthropologist or psychologist might use. A theory is a simplified representation of how two or more variables interact with each other. The purpose of a theory is to take a complex, real-world issue and simplify it down to its essentials. If done well, this enables the analyst to understand the issue and any problems around it. A good theory is simple enough to understand, while complex enough to capture the key features of the object or situation you are studying.
Sometimes economists use the term model instead of theory. Strictly speaking, a theory is a more abstract representation, while a model is a more applied or empirical representation. We use models to test theories, but for this course we will use the terms interchangeably.
For example, an architect who is planning a major office building will often build a physical model that sits on a tabletop to show how the entire city block will look after the new building is constructed. Companies often build models of their new products, which are more rough and unfinished than the final product, but can still demonstrate how the new product will work.
A good model to start with in economics is the circular flow diagram ( Figure 1.7 ). It pictures the economy as consisting of two groups—households and firms—that interact in two markets: the goods and services market in which firms sell and households buy and the labor market in which households sell labor to business firms or other employees.
Firms produce and sell goods and services to households in the market for goods and services (or product market). Arrow “A” indicates this. Households pay for goods and services, which becomes the revenues to firms. Arrow “B” indicates this. Arrows A and B represent the two sides of the product market. Where do households obtain the income to buy goods and services? They provide the labor and other resources (e.g., land, capital, raw materials) firms need to produce goods and services in the market for inputs (or factors of production). Arrow “C” indicates this. In return, firms pay for the inputs (or resources) they use in the form of wages and other factor payments. Arrow “D” indicates this. Arrows “C” and “D” represent the two sides of the factor market.
Of course, in the real world, there are many different markets for goods and services and markets for many different types of labor. The circular flow diagram simplifies this to make the picture easier to grasp. In the diagram, firms produce goods and services, which they sell to households in return for revenues. The outer circle shows this, and represents the two sides of the product market (for example, the market for goods and services) in which households demand and firms supply. Households sell their labor as workers to firms in return for wages, salaries, and benefits. The inner circle shows this and represents the two sides of the labor market in which households supply and firms demand.
This version of the circular flow model is stripped down to the essentials, but it has enough features to explain how the product and labor markets work in the economy. We could easily add details to this basic model if we wanted to introduce more real-world elements, like financial markets, governments, and interactions with the rest of the globe (imports and exports).
Economists carry a set of theories in their heads like a carpenter carries around a toolkit. When they see an economic issue or problem, they go through the theories they know to see if they can find one that fits. Then they use the theory to derive insights about the issue or problem. Economists express theories as diagrams, graphs, or even as mathematical equations. (Do not worry. In this course, we will mostly use graphs.) Economists do not figure out the answer to the problem first and then draw the graph to illustrate. Rather, they use the graph of the theory to help them figure out the answer. Although at the introductory level, you can sometimes figure out the right answer without applying a model, if you keep studying economics, before too long you will run into issues and problems that you will need to graph to solve. We explain both micro and macroeconomics in terms of theories and models. The most well-known theories are probably those of supply and demand, but you will learn a number of others.
As an Amazon Associate we earn from qualifying purchases.
This book may not be used in the training of large language models or otherwise be ingested into large language models or generative AI offerings without OpenStax's permission.
Want to cite, share, or modify this book? This book uses the Creative Commons Attribution License and you must attribute OpenStax.
Access for free at https://openstax.org/books/principles-economics-3e/pages/1-introduction
- Authors: Steven A. Greenlaw, David Shapiro, Daniel MacDonald
- Publisher/website: OpenStax
- Book title: Principles of Economics 3e
- Publication date: Dec 14, 2022
- Location: Houston, Texas
- Book URL: https://openstax.org/books/principles-economics-3e/pages/1-introduction
- Section URL: https://openstax.org/books/principles-economics-3e/pages/1-3-how-economists-use-theories-and-models-to-understand-economic-issues
© Jan 23, 2024 OpenStax. Textbook content produced by OpenStax is licensed under a Creative Commons Attribution License . The OpenStax name, OpenStax logo, OpenStax book covers, OpenStax CNX name, and OpenStax CNX logo are not subject to the Creative Commons license and may not be reproduced without the prior and express written consent of Rice University.
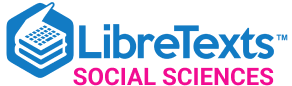
- school Campus Bookshelves
- menu_book Bookshelves
- perm_media Learning Objects
- login Login
- how_to_reg Request Instructor Account
- hub Instructor Commons
- Download Page (PDF)
- Download Full Book (PDF)
- Periodic Table
- Physics Constants
- Scientific Calculator
- Reference & Cite
- Tools expand_more
- Readability
selected template will load here
This action is not available.
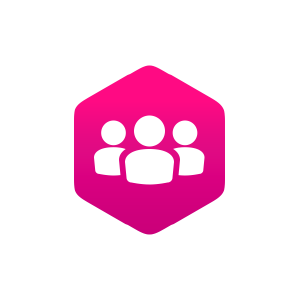
1.3: The Economists’ Tool Kit
- Last updated
- Save as PDF
- Page ID 109378

Learning Objective
- Explain how economists test hypotheses, develop economic theories, and use models in their analyses.
- Explain how the all-other-things unchanged (ceteris paribus) problem and the fallacy of false cause affect the testing of economic hypotheses and how economists try to overcome these problems.
- Distinguish between normative and positive statements.
Economics differs from other social sciences because of its emphasis on opportunity cost, the assumption of maximization in terms of one’s own self-interest, and the analysis of choices at the margin. But certainly much of the basic methodology of economics and many of its difficulties are common to every social science—indeed, to every science. This section explores the application of the scientific method to economics.
Researchers often examine relationships between variables. A variable is something whose value can change. By contrast, a constant is something whose value does not change. The speed at which a car is traveling is an example of a variable. The number of minutes in an hour is an example of a constant.
Research is generally conducted within a framework called the scientific method, a systematic set of procedures through which knowledge is created. In the scientific method, hypotheses are suggested and then tested. A hypothesis is an assertion of a relationship between two or more variables that could be proven to be false. A statement is not a hypothesis if no conceivable test could show it to be false. The statement “Plants like sunshine” is not a hypothesis; there is no way to test whether plants like sunshine or not, so it is impossible to prove the statement false. The statement “Increased solar radiation increases the rate of plant growth” is a hypothesis; experiments could be done to show the relationship between solar radiation and plant growth. If solar radiation were shown to be unrelated to plant growth or to retard plant growth, then the hypothesis would be demonstrated to be false.
If a test reveals that a particular hypothesis is false, then the hypothesis is rejected or modified. In the case of the hypothesis about solar radiation and plant growth, we would probably find that more sunlight increases plant growth over some range but that too much can actually retard plant growth. Such results would lead us to modify our hypothesis about the relationship between solar radiation and plant growth.
If the tests of a hypothesis yield results consistent with it, then further tests are conducted. A hypothesis that has not been rejected after widespread testing and that wins general acceptance is commonly called a theory. A theory that has been subjected to even more testing and that has won virtually universal acceptance becomes a law. We will examine two economic laws in the next two chapters.
Even a hypothesis that has achieved the status of a law cannot be proven true. There is always a possibility that someone may find a case that invalidates the hypothesis. That possibility means that nothing in economics, or in any other social science, or in any science, can ever be proven true. We can have great confidence in a particular proposition, but it is always a mistake to assert that it is “proven.”
Models in Economics
All scientific thought involves simplifications of reality. The real world is far too complex for the human mind—or the most powerful computer—to consider. Scientists use models instead. A model is a set of simplifying assumptions about some aspect of the real world. Models are always based on assumed conditions that are simpler than those of the real world, assumptions that are necessarily false. A model of the real world cannot be the real world.
We will encounter our first economic model in Chapter 35 “Appendix A: Graphs in Economics”. For that model, we will assume that an economy can produce only two goods. Then we will explore the model of demand and supply. One of the assumptions we will make there is that all the goods produced by firms in a particular market are identical. Of course, real economies and real markets are not that simple. Reality is never as simple as a model; one point of a model is to simplify the world to improve our understanding of it.
Economists often use graphs to represent economic models. The appendix to this chapter provides a quick, refresher course, if you think you need one, on understanding, building, and using graphs.
Models in economics also help us to generate hypotheses about the real world. In the next section, we will examine some of the problems we encounter in testing those hypotheses.
Testing Hypotheses in Economics
Here is a hypothesis suggested by the model of demand and supply: an increase in the price of gasoline will reduce the quantity of gasoline consumers demand. How might we test such a hypothesis?
Economists try to test hypotheses such as this one by observing actual behavior and using empirical (that is, real-world) data. The average retail price of gasoline in the United States rose from an average of $2.12 per gallon on May 22, 2005 to $2.88 per gallon on May 22, 2006. The number of gallons of gasoline consumed by U.S. motorists rose 0.3% during that period.
The small increase in the quantity of gasoline consumed by motorists as its price rose is inconsistent with the hypothesis that an increased price will lead to an reduction in the quantity demanded. Does that mean that we should dismiss the original hypothesis? On the contrary, we must be cautious in assessing this evidence. Several problems exist in interpreting any set of economic data. One problem is that several things may be changing at once; another is that the initial event may be unrelated to the event that follows. The next two sections examine these problems in detail.
The All-Other-Things-Unchanged Problem
The hypothesis that an increase in the price of gasoline produces a reduction in the quantity demanded by consumers carries with it the assumption that there are no other changes that might also affect consumer demand. A better statement of the hypothesis would be: An increase in the price of gasoline will reduce the quantity consumers demand, ceteris paribus. Ceteris paribus is a Latin phrase that means “all other things unchanged.”
But things changed between May 2005 and May 2006. Economic activity and incomes rose both in the United States and in many other countries, particularly China, and people with higher incomes are likely to buy more gasoline. Employment rose as well, and people with jobs use more gasoline as they drive to work. Population in the United States grew during the period. In short, many things happened during the period, all of which tended to increase the quantity of gasoline people purchased.
Our observation of the gasoline market between May 2005 and May 2006 did not offer a conclusive test of the hypothesis that an increase in the price of gasoline would lead to a reduction in the quantity demanded by consumers. Other things changed and affected gasoline consumption. Such problems are likely to affect any analysis of economic events. We cannot ask the world to stand still while we conduct experiments in economic phenomena. Economists employ a variety of statistical methods to allow them to isolate the impact of single events such as price changes, but they can never be certain that they have accurately isolated the impact of a single event in a world in which virtually everything is changing all the time.
In laboratory sciences such as chemistry and biology, it is relatively easy to conduct experiments in which only selected things change and all other factors are held constant. The economists’ laboratory is the real world; thus, economists do not generally have the luxury of conducting controlled experiments.
The Fallacy of False Cause
Hypotheses in economics typically specify a relationship in which a change in one variable causes another to change. We call the variable that responds to the change the dependent variable; the variable that induces a change is called the independent variable. Sometimes the fact that two variables move together can suggest the false conclusion that one of the variables has acted as an independent variable that has caused the change we observe in the dependent variable.
Consider the following hypothesis: People wearing shorts cause warm weather. Certainly, we observe that more people wear shorts when the weather is warm. Presumably, though, it is the warm weather that causes people to wear shorts rather than the wearing of shorts that causes warm weather; it would be incorrect to infer from this that people cause warm weather by wearing shorts.
Reaching the incorrect conclusion that one event causes another because the two events tend to occur together is called the fallacy of false cause. The accompanying essay on baldness and heart disease suggests an example of this fallacy.
Because of the danger of the fallacy of false cause, economists use special statistical tests that are designed to determine whether changes in one thing actually do cause changes observed in another. Given the inability to perform controlled experiments, however, these tests do not always offer convincing evidence that persuades all economists that one thing does, in fact, cause changes in another.
In the case of gasoline prices and consumption between May 2005 and May 2006, there is good theoretical reason to believe the price increase should lead to a reduction in the quantity consumers demand. And economists have tested the hypothesis about price and the quantity demanded quite extensively. They have developed elaborate statistical tests aimed at ruling out problems of the fallacy of false cause. While we cannot prove that an increase in price will, ceteris paribus, lead to a reduction in the quantity consumers demand, we can have considerable confidence in the proposition.
Normative and Positive Statements
Two kinds of assertions in economics can be subjected to testing. We have already examined one, the hypothesis. Another testable assertion is a statement of fact, such as “It is raining outside” or “Microsoft is the largest producer of operating systems for personal computers in the world.” Like hypotheses, such assertions can be demonstrated to be false. Unlike hypotheses, they can also be shown to be correct. A statement of fact or a hypothesis is a positive statement.
Although people often disagree about positive statements, such disagreements can ultimately be resolved through investigation. There is another category of assertions, however, for which investigation can never resolve differences. A normative statement is one that makes a value judgment. Such a judgment is the opinion of the speaker; no one can “prove” that the statement is or is not correct. Here are some examples of normative statements in economics: “We ought to do more to help the poor.” “People in the United States should save more.” “Corporate profits are too high.” The statements are based on the values of the person who makes them. They cannot be proven false.
Because people have different values, normative statements often provoke disagreement. An economist whose values lead him or her to conclude that we should provide more help for the poor will disagree with one whose values lead to a conclusion that we should not. Because no test exists for these values, these two economists will continue to disagree, unless one persuades the other to adopt a different set of values. Many of the disagreements among economists are based on such differences in values and therefore are unlikely to be resolved.
Key Takeaways
- Economists try to employ the scientific method in their research.
- Scientists cannot prove a hypothesis to be true; they can only fail to prove it false.
- Economists, like other social scientists and scientists, use models to assist them in their analyses.
- Two problems inherent in tests of hypotheses in economics are the all-other-things-unchanged problem and the fallacy of false cause.
- Positive statements are factual and can be tested. Normative statements are value judgments that cannot be tested. Many of the disagreements among economists stem from differences in values.
Look again at the data in Table 1.1 “LSAT Scores and Undergraduate Majors”. Now consider the hypothesis: “Majoring in economics will result in a higher LSAT score.” Are the data given consistent with this hypothesis? Do the data prove that this hypothesis is correct? What fallacy might be involved in accepting the hypothesis?
Case in Point: Does Baldness Cause Heart Disease?
A website called embarrassingproblems.com received the following email:
“Dear Dr. Margaret,
“I seem to be going bald. According to your website, this means I’m more likely to have a heart attack. If I take a drug to prevent hair loss, will it reduce my risk of a heart attack? ”
What did Dr. Margaret answer? Most importantly, she did not recommend that the questioner take drugs to treat his baldness, because doctors do not think that the baldness causes the heart disease. A more likely explanation for the association between baldness and heart disease is that both conditions are affected by an underlying factor. While noting that more research needs to be done, one hypothesis that Dr. Margaret offers is that higher testosterone levels might be triggering both the hair loss and the heart disease. The good news for people with early balding (which is really where the association with increased risk of heart disease has been observed) is that they have a signal that might lead them to be checked early on for heart disease.
Source: www.embarrassingproblems.com/problems/problempage230701.htm.
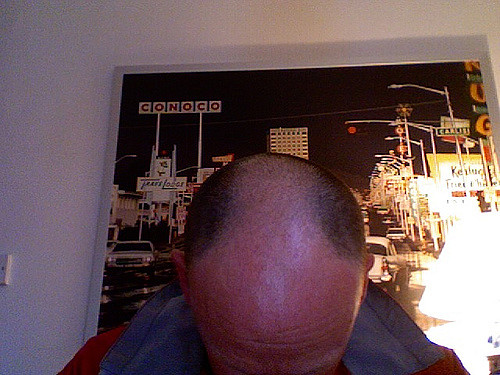
Answer to Try It! Problem
The data are consistent with the hypothesis, but it is never possible to prove that a hypothesis is correct. Accepting the hypothesis could involve the fallacy of false cause; students who major in economics may already have the analytical skills needed to do well on the exam.
Top 4 Types of Hypothesis in Consumption (With Diagram)
The following points highlight the top four types of Hypothesis in Consumption. The types of Hypothesis are: 1. The Post-Keynesian Developments 2. The Relative Income Hypothesis 3. The Life-Cycle Hypothesis 4. The Permanent Income Hypothesis.
Hypothesis Type # 1. The Post-Keynesian Developments:
Data collected and examined in the post-Second World War period (1945-) confirmed the Keynesian consumption function.
Time series data collected over long periods showed that the relation between income and consumption was different from what cross-section data revealed.
In the short run, there was a non-proportional relation between income and consumption. But in the long run the relation was proportional. By constructing new aggregate data on consumption and income from 1869 and examining the same, Simon Kuznets discovered that the ratio of consumption to income was fairly stable from decade to decade, despite large increases in income over the period he studied.
ADVERTISEMENTS:
This contradicted Keynes’ conjecture that the average propensity to consume would fall with increases in income. Kuznets’ findings indicated that the APC is fairly constant over long periods of time. This fact presented a puzzle which is illustrated in Fig. 17.10.
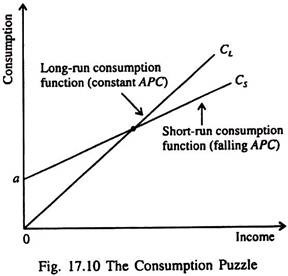
Studies of cross-section (household) data and short time series confirmed the Keynesian hypothesis — the relationship between consumption and income, as indicated by the consumption function C s in Fig. 17.10.
But studies of long time series found that APC did not vary systematically with income, as is shown by the long-run consumption function C L . The short-run consumption function has a falling APC, whereas the long-run consumption function has a constant APC.
Subsequent research on consumption attempted to explain how these two consumption functions could be consistent with each other.
Various attempts have been made to reconcile these conflicting evidences. In this context mention has to be made of James Duesenberry (who developed the relative income hypothesis), Ando, Brumberg and Modigliani (who developed the life cycle hypothesis of saving behaviour) and Milton Friedman who developed the permanent income hypothesis of consumption behaviour.
All these economists proposed explanations of these seemingly contradictory findings. These hypotheses may now be discussed one by one.
Hypothesis Type # 2. The Relative Income Hypothesis :
In 1949, James Duesenberry presented the relative income hypothesis. According to this hypothesis, saving (consumption) depends on relative income. The saving function is expressed as S t =f(Y t / Y p ), where Y t / Y p is the ratio of current income to some previous peak income. This is called relative income. Thus current consumption or saving is not a function-of current income but relative income.
Duensenberry pointed out that during depression when income falls consumption does not fall much. People try to protect their living standards either by reducing their past savings (or accumulated wealth) or by borrowing.
However as the economy gradually moves initially into the recovery and then in to the prosperity phase of the business cycle consumption does not rise even if income increases. People use a portion of their income either to restore the old saving rate or to repay their old debt.
Thus we see that there is a lack of symmetry in people’s consumption behaviour. People find it more difficult to reduce their consumption level than to raise it. This asymmetrical behaviour of consumers is known as the ratchet effect.
Thus if we observe a consumer’s short-run behaviour we find a non-proportional relation between income and consumption. Thus MPC is less than APC in the short run, as Keynes’s absolute income hypothesis has postulated. But if we study a consumer’s behaviour in the long run, i.e., over the entire business cycle we find a proportional relation between income and consumption. This means that in the long run MPC = APC.
Hypothesis Type # 3. The Life-Cycle Hypothesis :
In the late 1950s and early 1960s Franco Modigliani and his co-workers Albert Ando and Richard Brumberg related consumption expenditure to demography. Modigliani, in particular, emphasised that income varies systematically over peoples’ lives and that saving allows consumers to move income from early years of earning (when income is high) to later years after retirement when income is low.
This interpretation of household consumption behaviour forms the basis of his life-cycle hypothesis.
The life cycle hypothesis (henceforth LCH) represents an attempt to deal with the way in which consumers dispose off their income over time. In this hypothesis wealth is assigned a crucial role in consumption decision. Wealth includes not only property (houses, stocks, bonds, savings accounts, etc.) but also the value of future earnings.
Thus consumers visualise themselves as having a stock of initial wealth, a flow of income generated by that wealth over their lifetime and a target (which may be zero) as their end-of-life wealth. Consumption decisions are made with the whole series of financial flows in mind.
Thus, changes in wealth as reflected by unexpected changes in flow of earnings or unexpected movements in asset prices would have an impact on consumers’ spending decisions because they would enhance future earnings from property, labour or both. The theory has empirically testable implications for the relation between saving and age of a person as also for the role of wealth in influencing aggregate consumer spending.
The Hypothesis :
The main reason that an individual’s income varies is retirement. Since most people do not want their current living standard (as measured by consumption) to fall after retirement they save a portion of their income every year (over their entire service period). This motive for saving has an important implication for an individual’s consumption behaviour.
Suppose a representative consumer expects to live another T years, has wealth of W, and expects to earn income Y per year until he (she) retires R years from now. What should be the optimal level of consumption of the individual if he wishes to maintain a smooth level of consumption over his entire life?
The consumer’s lifetime endowments consist of initial wealth W and lifetime earnings RY. If we assume that the consumer divides his total wealth W + RY equally among the T years and wishes to consume smoothly over his lifetime then his annual consumption will be:
C = (W + RY)/T … (5)
This person’s consumption function can now be expressed as
C = (1/T)W + (R/T)Y
If all individuals plan their consumption in the same way then the aggregate consumption function is a replica of our representative consumer’s consumption function. To be more specific, aggregate consumption depends on both wealth and income. That is, the aggregate consumption function is
C = αW + βY …(6)
where the parameter α is the MPC out of wealth, and the parameter β is the MPC out of income.
Implications :
Fig. 17.11 shows the relationship between consumption and income in terms of the life cycle hypothesis. For any initial level of wealth w, the consumption function looks like the Keynesian function.
But the intercept αW which shows what would happen to consumption if income ever fell to zero, is not a constant, as is the term a in the Keynesian consumption function. Instead the intercept αW depends on the level of wealth. If W increases; the consumption line will shift upward parallely.
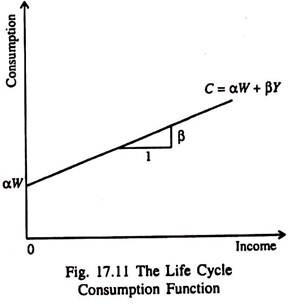
So one main prediction of the LCH is that consumption depends on wealth as well as income, as is shown by the intercept of the consumption function.
Solving the consumption puzzle:
The LCH can solve the consumption puzzle in a simple way.
According to this hypothesis, the APC is:
C/Y = α(W/Y) + β … (7)
Since wealth does not vary proportionately with income from person to person or from year to year, cross-section data (which show inter-individual differences in income and consumption over short periods) reveal that high income corresponds to a low APC. But in the long run, wealth and income grow together, resulting in a constant W/Y and a constant APC (as time-series show).
If wealth remains constant as in the short run the life cycle consumption function looks like the Keynesian consumption function, consumption function shifts upward as shown in Fig. 17.12. This prevents the APC from falling as income increases.
This means that the short-run consumption income relation (which takes wealth as constant) will not continue to hold in the long run when wealth increases. This is how the life cycle hypothesis (LCH) solves the consumption puzzle posed by Kuznets’ studies.

Other Predictions :
Another important prediction made by the LCH is that saving varies over a person’s lifetime. The LCH helps to link consumption and savings with the demographic considerations, especially with the age distribution of the population.
The MPC out of life-time income changes with age. If a person has no wealth at the beginning of his service life, then he will accumulate wealth over his working years and then run down his wealth after his retirement. Fig. 17.13 shows the consumer’s income, consumption and wealth over his adult life.
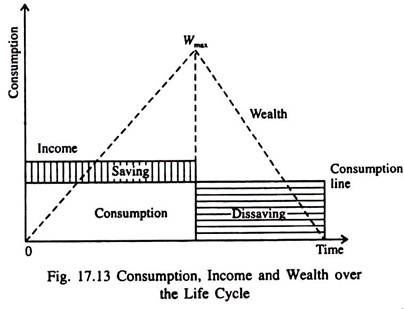
If a consumer smoothest consumption over his life (as indicated by the horizontal consumption line), he will save and accumulate wealth during his working years and then dissave and run down his wealth after retirement. In other words, since people want to smooth consumption over their lives, the young — who are working — save, while the old — who have retired — dissave.
In the long run the consumption-income ratio is very stable, but in the short run it fluctuates. The life cycle approach explains this by pointing out that people seek to maintain a smooth profile of consumption even if their lifetime income flow is uneven, and thus emphasises the role of wealth in the consumption function.
Theory and Evidence: Do Old People Dissave?
Some recent findings present a genuine problem for the LCH. Old people are found not to dissave as much as the hypothesis predicts. This means that the elderly do not reduce their wealth as fast as one would expect, if they were trying to smooth their consumption over their remaining years of life.
Two reasons explain why the old people do not dissave as much as the LCH predicts:
(i) Precautionary saving:
The old people are very much concerned about unpredictable expenses. So there is some precautionary motive for saving which originates from uncertainty. This uncertainty arises from the fact that old people often live longer than they expect. So they have to save more than what an average span of retirement would warrant.
Moreover uncertainty arises due to the fact that the medical expenses of old people increase faster than their age. So some sort of Malthusian spectre is found to be operating in this case. While an old person’s age increases at an arithmetical progression his medical expenses increase in geometrical progression due to accelerated depreciation of human body and the stronger possibility of illness.
The old people are likely to respond to this uncertainty by saving more in order to be able to overcome these contingencies.
Of course, there is an offsetting consideration here. Due to the spread of health and medical insurance in recent years old people can protect themselves against uncertainties about medical expenses at a low cost (i.e., just by paying a small premium).
Now-a-days various insurance plans are offered by both government and private agencies (such as Medisave, Mediclaim, Medicare, etc.). Of course, the premium rate increases with age. As a result the old people are required to increase their saving rate to fulfill their contractual obligations.
However, to protect against uncertainty regarding lifespan, old people can buy annuities from insurance companies. For a fixed fee, annuities offer a stream of income over the entire life span of the recipient.
(ii) Leaving bequests:
Old people do not dissave because they want to leave bequests to their children. The reason is that they care about them. But altruism is not really the reason that parents leave bequests. Parents often use the implicit threat of disinheritance to induce a desirable pattern of behaviour so that children and grandchildren take more care of them or be more attentive.
Thus LCH cannot fully explain consumption behaviour in the long run. No doubt providing for retirement is an important motive for saving, but other motives, such as precautionary saving and bequest, are no less important in determining people’s saving behaviour.
Another explanation, which differs in details but entirely shares the spirit of the life cycle approach is the permanent income hypothesis of consumption. The hypothesis, which is the brainchild of Milton Friedman, argues that people gear their consumption behaviour to their permanent or long term consumption opportunities, not to their current level of income.
An individual does not plan consumption within a period solely on the basis of income within the period; rather, consumption is planned in relation to income over a longer period. It is to this hypothesis that we turn now. We may now turn to Friedman’s permanent income hypothesis, which suggests an alternative explanation of long-run income-consumption relationship.
Hypothesis Type # 4. The Permanent Income Hypothesis :
Milton Friedman’s permanent income hypothesis (henceforth PIH) presented in 1957, complements Modigliani’s LCH. Both the hypotheses argue that consumption should not depend on current income alone.
But there is a difference of insight between the two hypotheses while the LCH emphasises that income follows a regular pattern over a person’s lifetime, the PIH emphasises that people experience random and temporary changes in their incomes from year to year.
The PIH, Friedman himself claims, ‘seems potentially more fruitful and in some measure more general” than the relative income hypothesis or the life-cycle hypothesis.
The idea of consumption spending that is geared to long-term average or permanent income is essentially the same as the life cycle theory. It raises two further questions. The first concerns the precise relationship between current consumption and permanent income. The second question is how to make the concept of present income operational, that is how to measure it.
The Basic Hypothesis :
According to Friedman the total measured income of an individual Y m has two components : permanent income Y p and transitory income Y t . That is, Y m – Y p + Y t .
Permanent income is that part of income which people expect to earn over their working life. Transitory income is that part of income which people do not expect to persist. In other words, while permanent income is average income, transitory income is the random deviation from that average.
Different forms of income have different degrees of persistence. While adequate investment in human capital (expenditure on training and education) provides a permanently higher income, good weather provides only transitorily higher income.
The PIH states that current consumption is not dependent solely on current disposable income but also on whether or not that income is expected to be permanent or transitory. The PIH argues that both income and consumption are split into two parts — permanent and transitory.
A person’s permanent income consists of such things as his long term earnings from employment (wages and salaries), retirement pensions and income derived from possessions of capital assets (interest and dividends).
The amount of a person’s permanent income will determine his permanent consumption plan, e.g., the size and quality of house he buys and, thus, his long term expenditure on mortgage repayments, etc.
Transitory income consists of short-term (temporary) overtime payments, bonuses and windfall gains from lotteries or stock appreciation and inheritances. Negative transitory income consists of short-term reduction in income arising from temporary unemployment and illness.
Transitory consumption such as additional holidays, clothes, etc. will depend upon his entire income. Long term consumption may also be related to changes in a person’s wealth, in particular the value of house over time. The economic significance of the PIH is that the short run level of consumption will be higher or lower than that indicated by the level of current disposable income.
According to Friedman consumption depends primarily on permanent income, because consumers use saving and borrowing to smooth consumption in response to transitory changes in income. The reason is that consumers spend their permanent income, but they save rather than spend most of their transitory income.
Since permanent income should be related to long run average income, this feature of the consumption function is clearly in line with the observed long run constancy of the consumption income ratio.
Let Y represent a consumer unit’s measured income for some time period, say, a year. This, according to Friedman, is the sum of two components : a permanent component (Y p ) and a transitory component (Y t ), or
Y = Y P + Y t …(8)
The permanent component reflects the effect of those factors that the unit regards as determining its capital value or wealth the non-human wealth it owns, the personal attributes of the earners in the unit, such as their training, ability, personality, the attributes of the economic activity of the earners, such as the occupation followed, the location of the economic activity, and so on.
The transitory component is to be interpreted as reflecting all ‘other’ factors, factors that are likely to be treated by the unit affected as ‘accident’ or ‘chance’ occurrences, for example, illness, a bad guess about when to buy or sell, windfall or chance gains from race or lotteries and so on. Permanent income is some sort of average.
Transitory income is a random variable. The difference between the two depends on how long the income persists. In other words, the distinction between the two is based on the degree of persistence. For example education gives an individual permanent income but luck — such as good weather — gives a farmer transitory income.
It may also be noted that permanent income cannot be zero or negative but transitory income can be.
Suppose a daily wage earner falls sick for a day or two and may not earn anything. So his transitory income is zero. Similarly if an individual sales a share in the stock exchange at a loss his transitory income is negative. Finally permanent income shows a steady trend but transitory income shows wide fluctuation(s).
Similarly, let C represent a consumer unit’s expenditures for some time period. It is also the sum of a permanent component (C p ) and a transitory component (C t ), so that
C = C p + C t … (9)
Some factors producing transitory components of consumption are: unusual sickness, a specifically favourable opportunity to purchase and the like. Permanent consumption is assumed to be the flow of utility services consumed by a group over a specific period.
The permanent income hypothesis is given by three simple equations (8), (9) and (10):
Y = Y p + Y t …(8)
C – C p + C t …(9)
C p = kY p , where k = f (r, W, u) …(10)
Here equation (6) defines a relation between permanent income and permanent consumption. Friedman specifies that the ratio between them is independent of the size of permanent income, but does depend on other variables in particular: (i) the rate of interest (r) or sets of rates of interest at which the consumer unit can borrow or lend; (ii) the relative importance of property and non-property income, symbolised by the ratio of non-human wealth to income (W) (iii) the factors symbolised by the random variable u determining the consumer unit’s tastes and preference for consumption versus additions to wealth. Equations (8) and (9) define the connection between the permanent components and the measured magnitudes.
Friedman assumes that the transistory components of income and consumption are uncorrelated with one another and with the corresponding permanent components, or
P ytyp = P ctcp = P ytct = 0 …(11)
where p stands for the correlation coefficient between the variables designated by the subscripts. The assumption that the third component in equation (11) — between the transitory components of income and consumption — is zero is indeed a strong assumption.
As Friedman says:
“The common notion that savings,…, are a ‘residue’ speaks strongly for the plausibility of the assumption. For this notion implies that consumption is determined by rather long-run considerations, so that any transitory changes in income lead primarily to additions to assets or to the use of previously accumulated balances rather than to corresponding changes in consumption.”
In Fig. 17.14 we consider the consumer units with a particular measured income, say which is above the mean measured income for the group as a whole — Y’. Given zero correlation between permanent and transitory components of income, the average permanent income of those units is less than Y 0 ; that is, the average transitory component is positive.
The average consumption of units with a measured income Y 0 is, therefore, equal to their average permanent consumption. In Friedman’s hypothesis this is k times their average permanent income.
If Y 0 were not only the measured income of these units but also their permanent income, their mean consumption would be Y 0 or Y 0 E. Since their mean permanent income is less than their measured income (i.e., the transitory component of income is positive), their average consumption, Y 0 F, is less than Y 0 E.
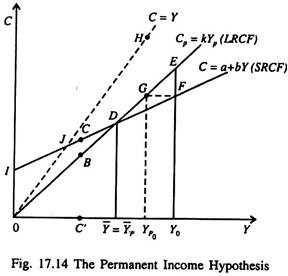
By the same logic, for consumer units with an income equal to the mean of the group as a whole, or Y, the average transitory component of income as well as of consumption is zero, so the ordinate of the regression line is equal to the ordinate of the line 0E which gives the relation between Y p and C p .
For units with an income below the mean, the average transitory component of income is negative, so average measured consumption (CC”) is greater than the ordinate of 0E (BC’). The regression line (C = a + bY), therefore, intersects 0E at D, is above it to the left of D, and below it to the right of D.
If k is less than unity, permanent consumption is always less than permanent income. But measured consumption is not necessarily less than measured income. The line OH is a 45° line along which C = Y.
The vertical distance between this line and IF is average measured savings. Point J is called the ‘break-even’ point at which average measured savings are zero. To the left of J, average measured savings are negative, to the right, positive; as measured income increases so does the ratio of average measured savings to measured income.
Friedman’s hypothesis thus yields a relation between measured consumption and measured income that reproduces the broadest features of the corresponding regressions that have been computed from observed data. The point is that consumption expenditures seem to be proportional to disposable income in the long run.
In the short run, on the other hand, the consumption-income ratio fluctuates considerably. In sum, current consumption is related to some long-run measure of income (e.g., permanent income) while short-run fluctuations in income tend primarily to affect the level of saving.
Estimating Permanent Income :
Dornbusch and Fischer have defined permanent income as “the steady rate of consumption a person could maintain for the rest of his or her life, given the present level of wealth and income earned now and in the future.”
One might estimate permanent income as being equal to last year’s income plus some fraction of the change in income from last year to this year:

- John A. List 1 ,
- Azeem M. Shaikh 1 &
- Yang Xu ORCID: orcid.org/0000-0001-5853-9461 1
8836 Accesses
235 Citations
58 Altmetric
Explore all metrics
The analysis of data from experiments in economics routinely involves testing multiple null hypotheses simultaneously. These different null hypotheses arise naturally in this setting for at least three different reasons: when there are multiple outcomes of interest and it is desired to determine on which of these outcomes a treatment has an effect; when the effect of a treatment may be heterogeneous in that it varies across subgroups defined by observed characteristics and it is desired to determine for which of these subgroups a treatment has an effect; and finally when there are multiple treatments of interest and it is desired to determine which treatments have an effect relative to either the control or relative to each of the other treatments. In this paper, we provide a bootstrap-based procedure for testing these null hypotheses simultaneously using experimental data in which simple random sampling is used to assign treatment status to units. Using the general results in Romano and Wolf (Ann Stat 38:598–633, 2010 ), we show under weak assumptions that our procedure (1) asymptotically controls the familywise error rate—the probability of one or more false rejections—and (2) is asymptotically balanced in that the marginal probability of rejecting any true null hypothesis is approximately equal in large samples. Importantly, by incorporating information about dependence ignored in classical multiple testing procedures, such as the Bonferroni and Holm corrections, our procedure has much greater ability to detect truly false null hypotheses. In the presence of multiple treatments, we additionally show how to exploit logical restrictions across null hypotheses to further improve power. We illustrate our methodology by revisiting the study by Karlan and List (Am Econ Rev 97(5):1774–1793, 2007 ) of why people give to charitable causes.
This is a preview of subscription content, log in via an institution to check access.
Access this article
Price includes VAT (Russian Federation)
Instant access to the full article PDF.
Rent this article via DeepDyve
Institutional subscriptions
Similar content being viewed by others
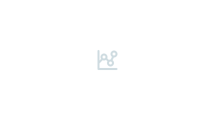
Symmetric experimental designs: conditions for equivalence of panel data estimators
Experiments and Econometrics
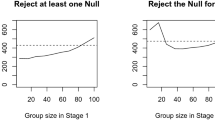
Improving the statistical power of economic experiments using adaptive designs
Anderson, M. (2008). Multiple inference and gender differences in the effects of early intervention: A re-evaluation of the abecedarian, perry preschool, and early training projects. Journal of the American Statistical Association , 103 (484), 1481–1495.
Article Google Scholar
Bettis, R. A. (2012). The search for asterisks: Compromised statistical tests and flawed theories. Strategic Management Journal , 33 (1), 108–113.
Bhattacharya, J., Shaikh, A. M., & Vytlacil, E. (2012). Treatment effect bounds: An application to swan-ganz catheterization. Journal of Econometrics , 168 (2), 223–243.
Bonferroni, C. E. (1935). Il calcolo delle assicurazioni su gruppi di teste . Rome: Tipografia del Senato.
Google Scholar
Bugni, F., Canay, I., & Shaikh, A. (2015). Inference under covariate-adaptive randomization. Technical report, cemmap working paper, Centre for Microdata Methods and Practice.
Camerer, C. F., Dreber, A., Forsell, E., Ho, T.-H., Huber, J., Johannesson, M., et al. (2016). Evaluating replicability of laboratory experiments in economics. Science , 351 (6280), 1433–1436.
Fink, G., McConnell, M., & Vollmer, S. (2014). Testing for heterogeneous treatment effects in experimental data: False discovery risks and correction procedures. Journal of Development Effectiveness , 6 (1), 44–57.
Flory, J. A., Gneezy, U., Leonard, K. L., & List, J. A. (2015a). Gender, age, and competition: The disappearing gap. Unpublished Manuscript.
Flory, J. A., Leibbrandt, A., & List, J. A. (2015b). Do competitive workplaces deter female workers? A large-scale natural field experiment on job-entry decisions. The Review of Economic Studies , 82 (1), 122–155.
Gneezy, U., Niederle, M., & Rustichini, A. (2003). Performance in competitive environments: Gender differences. The Quarterly Journal of Economics , 118 (3), 1049–1074.
Heckman, J., Moon, S. H., Pinto, R., Savelyev, P., & Yavitz, A. (2010). Analyzing social experiments as implemented: A reexamination of the evidence from the highscope perry preschool program. Quantitative Economics , 1 (1), 1–46.
Heckman, J. J., Pinto, R., Shaikh, A. M., & Yavitz, A. (2011). Inference with imperfect randomization: The case of the perry preschool program. National Bureau of Economic Research Working Paper w16935.
Holm, S. (1979). A simple sequentially rejective multiple test procedure. Scandinavian Journal of Statistics , 6 (2), 65–70.
Hossain, T., & List, J. A. (2012). The behavioralist visits the factory: Increasing productivity using simple framing manipulations. Management Science , 58 (12), 2151–2167.
Ioannidis, J. (2005). Why most published research findings are false. PLoS Med , 2 (8), e124.
Jennions, M. D., & Moller, A. P. (2002). Publication bias in ecology and evolution: An empirical assessment using the ‘trim and fill’ method. Biological Reviews of the Cambridge Philosophical Society , 77 (02), 211–222.
Karlan, D., & List, J. A. (2007). Does price matter in charitable giving? Evidence from a large-scale natural field experiment. The American Economic Review , 97 (5), 1774–1793.
Kling, J., Liebman, J., & Katz, L. (2007). Experimental analysis of neighborhood effects. Econometrica , 75 (1), 83–119.
Lee, S., & Shaikh, A. M. (2014). Multiple testing and heterogeneous treatment effects: Re-evaluating the effect of progresa on school enrollment. Journal of Applied Econometrics , 29 (4), 612–626.
Lehmann, E., & Romano, J. (2005). Generalizations of the familywise error rate. The Annals of Statistics , 33 (3), 1138–1154.
Lehmann, E. L., & Romano, J. P. (2006). Testing statistical hypotheses . Berlin: Springer.
Levitt, S. D., List, J. A., Neckermann, S., & Sadoff, S. (2012). The behavioralist goes to school: Leveraging behavioral economics to improve educational performance. National Bureau of Economic Research w18165.
List, J. A., & Samek, A. S. (2015). The behavioralist as nutritionist: Leveraging behavioral economics to improve child food choice and consumption. Journal of Health Economics , 39 , 135–146.
Machado, C., Shaikh, A., Vytlacil, E., & Lunch, C. (2013). Instrumental variables, and the sign of the average treatment effect. Unpublished Manuscript, Getulio Vargas Foundation, University of Chicago, and New York University. [2049].
Maniadis, Z., Tufano, F., & List, J. A. (2014). One swallow doesn’t make a summer: New evidence on anchoring effects. The American Economic Review , 104 (1), 277–290.
Niederle, M., & Vesterlund, L. (2007). Do women shy away from competition? Do men compete too much? The Quarterly Journal of Economics , 122 (3), 1067–1101.
Nosek, B. A., Spies, J. R., & Motyl, M. (2012). Scientific utopia ii. Restructuring incentives and practices to promote truth over publishability. Perspectives on Psychological Science , 7 (6), 615–631.
Romano, J. P., & Shaikh, A. M. (2006a). On stepdown control of the false discovery proportion. In Lecture Notes-Monograph Series (pp. 33–50).
Romano, J. P., & Shaikh, A. M. (2006b). Stepup procedures for control of generalizations of the familywise error rate. The Annals of Statistics , 34 , 1850–1873.
Romano, J. P., & Shaikh, A. M. (2012). On the uniform asymptotic validity of subsampling and the bootstrap. The Annals of Statistics , 40 (6), 2798–2822.
Romano, J. P., Shaikh, A. M., & Wolf, M. (2008a). Control of the false discovery rate under dependence using the bootstrap and subsampling. Test , 17 (3), 417–442.
Romano, J. P., Shaikh, A. M., & Wolf, M. (2008b). Formalized data snooping based on generalized error rates. Econometric Theory , 24 (02), 404–447.
Romano, J. P., & Wolf, M. (2005). Stepwise multiple testing as formalized data snooping. Econometrica , 73 (4), 1237–1282.
Romano, J. P., & Wolf, M. (2010). Balanced control of generalized error rates. The Annals of Statistics , 38 , 598–633.
Sutter, M., & Glätzle-Rützler, D. (2014). Gender differences in the willingness to compete emerge early in life and persist. Management Science , 61 (10), 2339–23354.
Westfall, P. H., & Young, S. S. (1993). Resampling-based multiple testing: Examples and methods for p value adjustment (Vol. 279). New York: Wiley.
Download references
Acknowledgements
We would like to thank Joseph P. Romano for helpful comments on this paper. We also thank Joseph Seidel for his excellent research assistance. The research of the second author was supported by National Science Foundation Grants DMS-1308260, SES-1227091, and SES-1530661.
Author information
Authors and affiliations.
Department of Economics, University of Chicago, 5757 S University Ave, Chicago, IL, 60637, USA
John A. List, Azeem M. Shaikh & Yang Xu
You can also search for this author in PubMed Google Scholar
Corresponding author
Correspondence to Yang Xu .
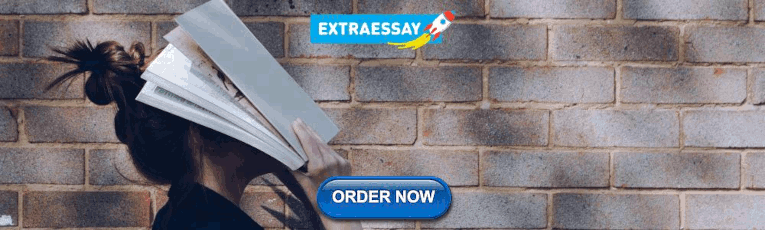
Additional information
Documentation of our procedures and our Stata and Matlab code can be found at https://github.com/seidelj/mht .
1.1 Proof of Theorem 3.1
First note that under Assumption 2.1 , \(Q\in \omega _{s}\) if and only if \(P\in {\tilde{\omega }}_{s}\) , where
The proof of this result now follows by verifying the conditions of Corollary 5.1 in Romano and Wolf ( 2010 ). In particular, we verify Assumptions B.1–B.4 in Romano and Wolf ( 2010 ).
In order to verify Assumption B.1 in Romano and Wolf ( 2010 ), let
and note that
with \(A_{n,i}(P)\) equal to the \(2|{\mathcal {S}}|\) -dimensional vector formed by stacking vertically for \(s\in {\mathcal {S}}\) the terms
and \(B_{n}\) is the \(2|{\mathcal {S}}|\) -dimensional vector formed by stacking vertically for \(s\in {\mathcal {S}}\) the terms
and \(f:{\mathbf {R}}^{2|{\mathcal {S}}|}\times {\mathbf {R}}^{2|{\mathcal {S}}|}\rightarrow {\mathbf {R}}^{2|{\mathcal {S}}|}\) is the function of \(A_{n}(P)\) and \(B_{n}\) whose s th argument for \(s\in {\mathcal {S}}\) is given by the inner product of the s th pair of terms in \(A_{n}(P)\) and the s th pair of terms in \(B_{n}\) , i.e., the inner product of ( 10 ) and ( 11 ). The weak law of large numbers and central limit theorem imply that
where B ( P ) is the \(2|{\mathcal {S}}|\) -dimensional vector formed by stacking vertically for \(s\in {\mathcal {S}}\) the terms
Next, note that \(E_{P}[A_{n,i}(P)]=0\) . Assumption 2.3 and the central limit theorem therefore imply that
for an appropriate choice of \(V_{A}(P)\) . In particular, the diagonal elements of \(V_{A}(P)\) are of the form
The continuous mapping theorem thus implies that
for an appropriate variance matrix V ( P ). In particular, the s th diagonal element of V ( P ) is given by
In order to verify Assumptions B.2–B.3 in Romano and Wolf ( 2010 ), it suffices to note that ( 12 ) is strictly greater than zero under our assumptions. Note that it is not required that V ( P ) be non-singular for these assumptions to be satisfied.
In order to verify Assumption B.4 in Romano and Wolf ( 2010 ), we first argue that
under \(P_{n}\) for an appropriate sequence of distributions \(P_{n}\) for \((Y_{i},D_{i},Z_{i})\) . To this end, assume that
\(P_{n}{\mathop {\rightarrow }\limits ^{d}}P\) .
\({\tilde{\mu }}_{k|d,z}(P_{n})\rightarrow {\tilde{\mu }}_{k|d,z}(P)\) .
\(B_{n}{\mathop {\rightarrow }\limits ^{P_{n}}}B(P)\) .
\(\text {Var}_{P_{n}}[A_{n,i}(P_{n})]\rightarrow \text {Var}_{P}[A_{n,i}(P)]\) .
Under (a) and (b), it follows that \(A_{n,i}(P_{n}){\mathop {\rightarrow }\limits ^{d}}A_{n,i}(P)\) under \(P_{n}\) . By arguing as in Theorem 15.4.3 in Lehmann and Romano ( 2006 ) and using (d), it follows from the Lindeberg–Feller central limit theorem that
under \(P_{n}\) . It thus follows from (c) and the continuous mapping theorem that ( 13 ) holds under \(P_{n}\) . Assumption B.4 in Romano and Wolf ( 2010 ) now follows simply by nothing that the Glivenko-Cantelli theorem, strong law of large numbers and continuous mapping theorem ensure that \({\hat{P}}_{n}\) satisfies (a)–(d) with probability one under P .
Rights and permissions
Reprints and permissions
About this article
List, J.A., Shaikh, A.M. & Xu, Y. Multiple hypothesis testing in experimental economics. Exp Econ 22 , 773–793 (2019). https://doi.org/10.1007/s10683-018-09597-5
Download citation
Received : 09 October 2017
Revised : 10 November 2018
Accepted : 19 November 2018
Published : 29 January 2019
Issue Date : December 2019
DOI : https://doi.org/10.1007/s10683-018-09597-5
Share this article
Anyone you share the following link with will be able to read this content:
Sorry, a shareable link is not currently available for this article.
Provided by the Springer Nature SharedIt content-sharing initiative
- Experiments
- Multiple hypothesis testing
- Multiple treatments
- Multiple outcomes
- Multiple subgroups
- Randomized controlled trial
JEL Classification
- Find a journal
- Publish with us
- Track your research
- Search Search Please fill out this field.
- Efficient Market Hypothesis (EMH)
- How It Works
Special Considerations
Efficient market hypothesis (emh): definition and critique.
:max_bytes(150000):strip_icc():format(webp)/lucas_downey2-5bfc261fc9e77c00262f627c.jpg)
Gordon Scott has been an active investor and technical analyst or 20+ years. He is a Chartered Market Technician (CMT).
:max_bytes(150000):strip_icc():format(webp)/gordonscottphoto-5bfc26c446e0fb00265b0ed4.jpg)
What Is the Efficient Market Hypothesis (EMH)?
The efficient market hypothesis (EMH), alternatively known as the efficient market theory, is a hypothesis that states that share prices reflect all available information and consistent alpha generation is impossible.
According to the EMH, stocks always trade at their fair value on exchanges, making it impossible for investors to purchase undervalued stocks or sell stocks for inflated prices. Therefore, it should be impossible to outperform the overall market through expert stock selection or market timing , and the only way an investor can obtain higher returns is by purchasing riskier investments.
Key Takeaways
- The efficient market hypothesis (EMH) or theory states that share prices reflect all information.
- The EMH hypothesizes that stocks trade at their fair market value on exchanges.
- Proponents of EMH posit that investors benefit from investing in a low-cost, passive portfolio.
- Opponents of EMH believe that it is possible to beat the market and that stocks can deviate from their fair market values.
Investopedia / Theresa Chiechi
Understanding the Efficient Market Hypothesis (EMH)
Although it is a cornerstone of modern financial theory, the EMH is highly controversial and often disputed. Believers argue it is pointless to search for undervalued stocks or to try to predict trends in the market through either fundamental or technical analysis .
Theoretically, neither technical nor fundamental analysis can produce risk-adjusted excess returns (alpha) consistently, and only inside information can result in outsized risk-adjusted returns.
$599,090.00
The February 9, 2024 share price of the most expensive stock in the world: Berkshire Hathaway Inc. Class A (BRK.A).
While academics point to a large body of evidence in support of EMH, an equal amount of dissension also exists. For example, investors such as Warren Buffett have consistently beaten the market over long periods, which by definition is impossible according to the EMH.
Detractors of the EMH also point to events such as the 1987 stock market crash, when the Dow Jones Industrial Average (DJIA) fell by over 20% in a single day, and asset bubbles as evidence that stock prices can seriously deviate from their fair values.
The assumption that markets are efficient is a cornerstone of modern financial economics—one that has come under question in practice.
Proponents of the Efficient Market Hypothesis conclude that, because of the randomness of the market, investors could do better by investing in a low-cost, passive portfolio.
Data compiled by Morningstar Inc., in its June 2019 Active/Passive Barometer study, supports the EMH. Morningstar compared active managers’ returns in all categories against a composite made of related index funds and exchange-traded funds (ETFs) . The study found that over a 10 year period beginning June 2009, only 23% of active managers were able to outperform their passive peers. Better success rates were found in foreign equity funds and bond funds. Lower success rates were found in US large-cap funds. In general, investors have fared better by investing in low-cost index funds or ETFs.
While a percentage of active managers do outperform passive funds at some point, the challenge for investors is being able to identify which ones will do so over the long term. Less than 25 percent of the top-performing active managers can consistently outperform their passive manager counterparts over time.
What Does It Mean for Markets to Be Efficient?
Market efficiency refers to how well prices reflect all available information. The efficient markets hypothesis (EMH) argues that markets are efficient, leaving no room to make excess profits by investing since everything is already fairly and accurately priced. This implies that there is little hope of beating the market, although you can match market returns through passive index investing.
Has the Efficient Markets Hypothesis Any Validity?
The validity of the EMH has been questioned on both theoretical and empirical grounds. There are investors who have beaten the market, such as Warren Buffett , whose investment strategy focused on undervalued stocks made billions and set an example for numerous followers. There are portfolio managers who have better track records than others, and there are investment houses with more renowned research analysis than others. EMH proponents, however, argue that those who outperform the market do so not out of skill but out of luck, due to the laws of probability: at any given time in a market with a large number of actors, some will outperform the mean, while others will underperform .
Can Markets Be Inefficient?
There are certainly some markets that are less efficient than others. An inefficient market is one in which an asset's prices do not accurately reflect its true value, which may occur for several reasons. Market inefficiencies may exist due to information asymmetries, a lack of buyers and sellers (i.e. low liquidity), high transaction costs or delays, market psychology, and human emotion, among other reasons. Inefficiencies often lead to deadweight losses. In reality, most markets do display some level of inefficiencies, and in the extreme case, an inefficient market can be an example of a market failure.
Accepting the EMH in its purest (strong) form may be difficult as it states that all information in a market, whether public or private, is accounted for in a stock's price. However, modifications of EMH exist to reflect the degree to which it can be applied to markets:
- Semi-strong efficiency: This form of EMH implies all public (but not non-public) information is calculated into a stock's current share price. Neither fundamental nor technical analysis can be used to achieve superior gains.
- Weak efficiency: This type of EMH claims that all past prices of a stock are reflected in today's stock price. Therefore, technical analysis cannot be used to predict and beat the market.
What Can Make a Market More Efficient?
The more participants are engaged in a market, the more efficient it will become as more people compete and bring more and different types of information to bear on the price. As markets become more active and liquid, arbitrageurs will also emerge, profiting by correcting small inefficiencies whenever they might arise and quickly restoring efficiency.
The Library of Economics and Liberty. " Efficient Capital Markets ."
Yahoo Finance. " Berkshire Hathaway Inc. (BRK-A) ."
Federal Reserve History. " Stock Market Crash of 1987 ."
Morningstar. " Active Funds vs. Passive Funds: Which Fund Types Had Increased Success Rates? "
:max_bytes(150000):strip_icc():format(webp)/128013432-5bfc2b96c9e77c0026b4fb00.jpg)
- Terms of Service
- Editorial Policy
- Privacy Policy
- Your Privacy Choices
Thank you for visiting nature.com. You are using a browser version with limited support for CSS. To obtain the best experience, we recommend you use a more up to date browser (or turn off compatibility mode in Internet Explorer). In the meantime, to ensure continued support, we are displaying the site without styles and JavaScript.
- View all journals
- Explore content
- About the journal
- Publish with us
- Sign up for alerts
- Perspective
- Published: 02 December 2019
The ergodicity problem in economics
- Ole Peters ORCID: orcid.org/0000-0002-1246-8570 1
Nature Physics volume 15 , pages 1216–1221 ( 2019 ) Cite this article
276k Accesses
81 Citations
1134 Altmetric
Metrics details
- Applied mathematics
- Statistical physics, thermodynamics and nonlinear dynamics
Matters Arising to this article was published on 02 December 2020
An Author Correction to this article was published on 06 December 2019
This article has been updated
The ergodic hypothesis is a key analytical device of equilibrium statistical mechanics. It underlies the assumption that the time average and the expectation value of an observable are the same. Where it is valid, dynamical descriptions can often be replaced with much simpler probabilistic ones — time is essentially eliminated from the models. The conditions for validity are restrictive, even more so for non-equilibrium systems. Economics typically deals with systems far from equilibrium — specifically with models of growth. It may therefore come as a surprise to learn that the prevailing formulations of economic theory — expected utility theory and its descendants — make an indiscriminate assumption of ergodicity. This is largely because foundational concepts to do with risk and randomness originated in seventeenth-century economics, predating by some 200 years the concept of ergodicity, which arose in nineteenth-century physics. In this Perspective, I argue that by carefully addressing the question of ergodicity, many puzzles besetting the current economic formalism are resolved in a natural and empirically testable way.
You have full access to this article via your institution.
Similar content being viewed by others
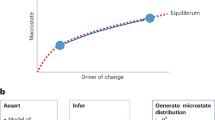
Entropy, irreversibility and inference at the foundations of statistical physics
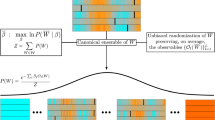
Maximum entropy approach to multivariate time series randomization
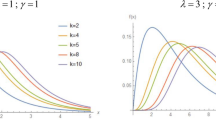
Truncated Weibull–exponential distribution: methods and applications
Ergodic theory is a forbiddingly technical branch of mathematics. Luckily, for the purpose of this discussion, we will need virtually none of the technicalities. We will call an observable ergodic if its time average equals its expectation value, that is, if it satisfies Birkhoff’s equation
Here, f is determined by the system’s state ω . On the left-hand side, the state in turn depends on time t . On the right-hand side, a timeless P ( ω ) assigns weights to ω . If equation ( 1 ) holds we can avoid integrating over time (up to the divergent averaging time, T , on the left), and instead integrate over the space of all states, Ω (on the right). In our case P ( ω ) is given as the distribution of a stochastic process. In systems with transient behaviour, that may require defining P ( ω ) as the t → ∞ limit of a time dependent density function P ( ω ; t ).
Famously, ergodicity is assumed in equilibrium statistical mechanics, which successfully describes the thermodynamic behaviour of gases. However, in a wider context, many observables don’t satisfy equation ( 1 ). And it turns out a surprising reframing of economic theory follows directly from asking the core ergodicity question: is the time average of an observable equal to its expectation value?
At a crucial place in the foundations of economics, it is assumed that the answer is always yes — a pernicious error. To make economic decisions, I often want to know how fast my personal fortune grows under different scenarios. This requires determining what happens over time in some model of wealth. But by wrongly assuming ergodicity, wealth is often replaced with its expectation value before growth is computed. Because wealth is not ergodic, nonsensical predictions arise. After all, the expectation value effectively averages over an ensemble of copies of myself that cannot be accessed.
This key error is patched up with psychological arguments about human behaviour. The consequences are numerous, but over the centuries their root cause has become invisible in the growing formalism. Observed behaviour deviates starkly from model predictions. Paired with a firm belief in its models, this has led to a narrative of human irrationality in large parts of economics. Scientifically, this deserves some reflection: the models were exonerated by declaring the object of study irrational.
I stumbled on this error about a decade ago, and with my collaborators at the London Mathematical Laboratory and the Santa Fe Institute I have identified a number of long-standing puzzles or paradoxes in economics that derive from it. If we pay close attention to the ergodicity problem, natural solutions emerge. We therefore have reason to be optimistic about the future of economic theory.
This Perspective is structured as follows. I will first sketch the conceptual basis of mainstream economic theory: discounted expected utility. I will then develop our conceptually different approach, based on addressing the ergodicity problem, and establish its relationship with the mainstream model by pointing out a mapping. Finally, I will report on a recent laboratory experiment that pits the two approaches against one another: where do their predictions differ? And which model fares better empirically?
A simple gamble
In economics, a gamble is a random variable, ∆ x , representing possible changes in wealth, x . In the discrete case, that’s a set of pairs of possible wealth changes and corresponding probabilities {(∆ x i , p i )}.
For example, a gamble can model the following situation: toss a coin, and for heads you win 50% of your current wealth, for tails you lose 40%. Mathematically, we can represent this as (Fig. 1a ):
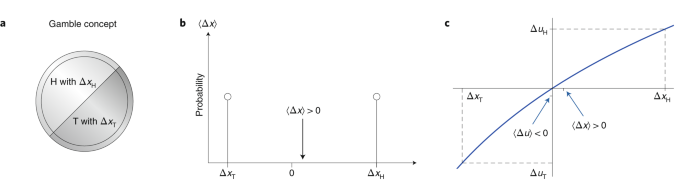
a , Events (here H and T) are associated with probabilities p H and p T , and with dollar wealth changes Δ x H and Δ x T . b , The oldest formal evaluation of a gamble computes the expected wealth change 〈∆ x 〉. c , Expected utility theory evaluates gambles by the expected change in a (nonlinear) utility function of wealth, 〈∆ u 〉, here u ( x ) = ln x shown for the coin-toss example. Note that all the concepts are formally atemporal. Only magnitudes and probabilities enter into the analysis.
The word ‘gamble’ conjures up images of smoke-filled casinos and roulette wheels. But here the term refers to a universal concept in economics: formally, any decision we make is modelled as a gamble, be it choosing the kindergarten for your child or deciding on matters of taxation. We never quite know the consequences in advance, and economically this is often expressed as a random wealth change.
The original treatment
The development of probability theory was motivated by gambling problems, with early formal attempts in Cardano’s wonderful (and unpublishably sinful) sixteenth-century book De ludo aleae 1 . The actual starting point is the famous exchange of letters between Fermat and Pascal in 1654 2 . Their correspondence established the expectation value as a key object in the theory of randomness.
Pascal and Fermat were not looking for gambling advice; they were solving a moral problem: namely how to assess people’s hopes and expectations in a fair way. Nonetheless, a few years later, the following rule of thumb had become a well-established behavioural model: given the choice between two gambles, we pick the greater expected wealth change, 〈∆ x 〉. This model predicts that people would generally accept the gamble in equation ( 2 ), in which case 〈∆ x 〉 = +0.05 x (Fig. 1b ), whereas the alternative (not accepting) would yield 〈∆ x 〉 = $0.
St. Petersburg paradox
But is this model realistic? Would you accept the gamble and risk losing at the toss of a coin 40% of your house, car and life savings?
A similar objection was raised in 1713 by Nicolas Bernoulli 3 . He proposed a hypothetical gamble whose expectation value was divergent: ∆ x was power-law distributed with a non-existent first moment. But this terminology hadn’t been developed yet, and N. Bernoulli said laconically we would find something “curious” if we tried to compute the expectation value 〈 ∆x 〉.
What people eventually deemed indeed curious was the following: if we had to pay a fee, F , to play this gamble, what should it be? The expected wealth model tells us that we would pay any finite fee, but that went against intuition. Even though ∆ x had a heavy right tail, the probabilities of very large gains were still vanishing, and no one was willing (hypothetically) to pay much for a negligible chance to win a large amount. The failure of the expected wealth model to describe actual human behaviour is known as the St. Petersburg paradox, and is treated in many textbooks on economics and probability theory. It is one of the puzzles that go away when we switch to the new formalism 4 .
Utility theory
By 1713, it was clear that there’s more than expected wealth changes to financial decisions under uncertainty, and in 1738 Daniel Bernoulli updated the prevailing theory 5 : when people decide whether to take part in a gamble, they don’t consider the expected changes in wealth, x , but the expected changes in the usefulness of wealth, u ( x ).
Specifically, D. Bernoulli surmised that the usefulness, or utility, of an extra dollar is roughly inversely proportional to how many dollars one already has. This leads to the differential equation d u = 1/ x d x with solution u ( x ) = ln x (calculus had just been invented). But he mentioned that the square-root function would also work. In general, a monotonically increasing u ( x ) reflects a preference for more wealth over less, and concave u ( x ) reflects a dislike for risk. Thus, the utility function encodes the psychology of a particular individual. We might write u brave ( x ) = x and u scared ( x ) = ln x .
D. Bernoulli’s model became known as ‘expected utility theory’. It produces different preferences than the expected wealth model if the utility function is nonlinear, as shown in Fig. 1c .
Intriguingly, D. Bernoulli’s paper contains an error (see ref. 4 ) that continues to haunt the formalism today: in one place his computations actually only work for linear u ( x ), which would defeat the purpose of introducing u ( x ) in the first place. But we will not take D. Bernoulli literally and instead interpret his writings as Laplace 6 and von Neumann and Morgenstern 7 did: each person i has an idiosyncratic utility function u i ( x ) and intuitively computes 〈∆ u i ( x )〉. If that’s positive we accept the gamble, if it’s negative we reject it (assuming rejection results in no wealth change).
In the coin-toss example described by equation ( 2 ), the expected change in D. Bernoulli’s logarithmic utility is 〈∆ln x 〉 ≈ −0.05. A person whose psychology is well described by u scared therefore won’t accept the gamble.
Discounting
Utility theory considers a static probability space, without an explicit treatment of time. For instance, D. Bernoulli and his followers did not discuss the rate of change of utilities but only magnitudes of changes.
Time is dealt with quite separately, namely through a process referred to as discounting. Originally, discounting assigned a present value to payments to be received in the future. It is often justified with a no-arbitrage argument: a payment received sooner, at a time t , is worth more than the same payment received later, at t + ∆ t , if it can be profitably invested for the duration ∆ t .
With references to interest in the Bible (for example, Deuteronomy 23:19), the practice of temporal discounting is thus much older than the notion of utility. Today the two concepts coexist but without much clarity regarding their respective domains: based on the no-arbitrage argument one would discount cash, but since 1937 it has been common to discount utility instead — not even utility of cash but of consumption of cash or even more general resources 8 .
The no-arbitrage argument ties discounting to available investment options. But in an ambitious attempt at generality, discounting nowadays is often phrased in terms of another subjective function, d (∆ t ): some of us are impatient and discount strongly with a fast-decaying d (∆ t ); others are more patient. The functional form of d (∆ t ), supposedly, is another part of our psychology — it can be hyperbolic or exponential or whatever else fits the data 9 .
A modern treatment asking the ergodicity question
Let’s step back, and take a completely fresh look at the problem.
First, we consider financial decisions without uncertainty, which is very similar to the original idea of discounting. In the second step, we generalize by introducing noise. Placing considerations of time and ergodicity centre stage, we will arrive at a clear interpretation both of discounting and of utility theory, without appealing to subjective psychology or indeed other forms of personalization.
Financial decisions without uncertainty
A gamble without uncertainty is just a payment. A trivial model would be: we accept positive payments and reject negative ones. But what if we have to choose between two payments, or payment streams, at different times?
In this case, one consideration must be some form of a growth rate. For instance, I may choose between a job that offers $12,000 per year, and another that offers $2,000 per month. Let’s say the jobs are identical in all other respects: I would then choose the one that pays $2,000 per month — not because $2,000 is the greater payment (it isn’t), but because the payments correspond to a higher (additive) growth rate of my wealth. I would maximize
Alternatively, I may have a choice between two savings accounts. One pays 4% per year, the other 1% per month — again, it’s the growth rate I would optimize: in this case the exponential growth rate
Since ∆ t divides a difference in a generally nonlinear function of wealth, time now enters with a clear meaning but in potentially quite complicated ways — linearly (called hyperbolic in economics) as in equation ( 3 ) or exponentially as in equation ( 4 ).
Additive earnings and multiplicative returns on investments are the two most common processes that change our wealth, but we could think of other growth processes whose growth rates would have different functional forms. For example, the growth rate for the sigmoidal growth curves, in biology, of body mass versus time, has a different functional form 10 . For an arbitrary growth process x ( t ), the general growth rate is
where v ( x ) is a monotonically increasing function chosen such that g does not change in time. Additive and multiplicative growth correspond to v a ( x ) = x and v e ( x ) = ln x . Generalizing, v ( x ) is the inverse of the process x ( t ) at unit rate, denoted
For financial processes, fitting more general functions often results in an interpolation between linear and logarithmic, maybe in a square-root function, or a similar small tweak.
Ergodic observables
Real-life financial decisions usually come with a degree of uncertainty. We let the model reflect this by introducing noise. But how?
To perturb the process in a consistent way, we remind ourselves that what’s constant about the process in the absence of noise is the growth rate. If we perturb that with a constant-amplitude noise, the scale of the perturbation will be time independent in v -space, and in that sense adapted to the dynamics. That’s easily done by writing equation ( 5 ) in differential form, replacing the function g by its (constant) value, γ , say, rearranging and adding the noise (here represented by a Wiener term d W with amplitude σ )
The process itself is found by integrating equation ( 7 ) and solving for x . For our two key examples, this produces Brownian motion (with v a = x ) and geometric Brownian motion (with v e = ln x ).
The growth rates for these processes are no longer constant because they are noisy. But the lack of constancy is due to nothing other than the noise. Using the nomenclature introduced in equation ( 1 ), the relevant growth rates are ergodic observables of their respective processes. By design, their (time or ensemble) averages tell us what tends to happen over time.
This is not the case for wealth itself, and it exposes the expected wealth model as physically naive. The expected wealth change simply does not reflect what happens over time (unless the wealth dynamic is additive; Fig. 2 ). The initial correction — expected utility theory — overlooked the physical problem and jumped to psychological arguments, which are hard to constrain and often circular.
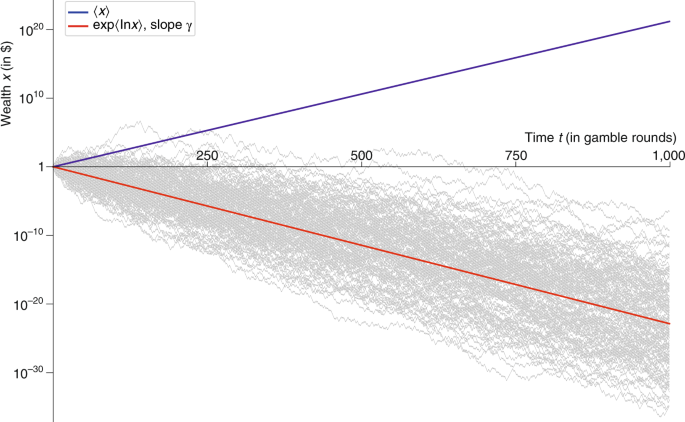
The example gamble is given in equation ( 2 ). The expectation value, 〈 x 〉, (blue line) is the average over an infinite ensemble, but it doesn’t reflect what happens over time. The ergodic growth rate for the process (slope of the red line) tells us what happens to a typical individual trajectory. 150 trajectories are shown, each consists of 1,000 repetitions.
Growth rate optimization is now sometimes called ‘ergodicity economics’. This doesn’t mean that ergodicity is assumed — quite the opposite: it refers to doing economics by asking explicitly whether something is ergodic, which is often not the case. As we have seen, ergodicity economics is a perspective that arises from constructing ergodic observables for non-ergodic (growth) processes.
Both expected utility theory and ergodicity economics introduce nonlinear transformations of wealth, and the equations that appear in the two frameworks can be very similar. More precisely, the mapping is this: the appropriate growth rate for a given process is formally identical to the rate of change of a specific utility function
The time average of this growth rate is identical to the rate of change of the specific expected utility function — because of ergodicity.
Despite the mapping, conceptually the two approaches couldn’t be more different, and ergodicity economics stays closer to physical reality.
Expected utility theory is a loose end of the mapping because the only constraints on the utility function it provides are loose references to psychology. While some view this as a way to ensure generality, my second criticism is more severe and I’m unable to resolve it: in maximizing the expectation value — an ensemble average over all possible outcomes of the gamble — expected utility theory implicitly assumes that individuals can interact with copies of themselves, effectively in parallel universes (the other members of the ensemble). An expectation value of a non-ergodic observable physically corresponds to pooling and sharing among many entities. That may reflect what happens in a specially designed large collective, but it doesn’t reflect the situation of an individual decision-maker.
Expected utility theory computes what happens to a loosely specified model of my psychology averaged across a multiverse. But I do not live spread out across a multiverse, let alone harvest the average psychological consequences of the actions of my multiverse clones.
Ergodicity economics, in contrast, computes what will happen to my physical wealth as time goes by, without appeal to an intangible psychology or a multiverse. We all live through time and suffer the physical consequences (and psychological consequences, for that matter) of the actions of our younger selves.
With ergodicity economics, the psychological insight that some people are systematically more cautious than others attains a physical interpretation. Perhaps people aren’t so different, but their circumstances are. Someone maximizing growth in an additive process would appear to be brave: v a = u brave , whereas the same person doing the same thing but for a multiplicative process would appear to be scared: v e = u scared . Note also the scale dependence of these statements: the same ∆ x (in dollars) corresponds to large logarithmic wealth changes for a poor person and to small logarithmic changes for a rich person — the latter are linearizable, and the rich person looks brave.
It also makes historical sense: in the early days of probability theory there was a firm belief that things should be expressed in terms of expectation values. For that to make any sense in the context of individuals making financial decisions, an ergodic observable had to be created. Expected utility theory — unknowingly, because ergodicity hadn’t been invented — did just that. But because of the lack of conceptual clarity, the entire field of economics drifted in a direction that places too much emphasis on psychology.
A discriminating experiment
This mapping is fascinating: careful thinking leads to almost identical mathematical expressions, whether we use the tools of 1738 or those of today. This is in spite of there being completely different concepts and languages. Does the difference between concepts enable an experiment that has discriminating power between expected utility theory and ergodicity economics?
I was sceptical about this possibility, but a group of neuroscientists from Copenhagen, led by Oliver Hulme, appears to have made very promising progress in this regard.
They followed very closely the discussion put forward in ref. 4 , where we had worked out in detail the correspondences between linear utility and additive dynamics; and between logarithmic utility and multiplicative dynamics. These correspondences provide the basis for the experiment: what if the dynamics of wealth could be controlled? With two artificial environments — one additive, the other one multiplicative — do people adjust their behaviour to be growth optimal in each?
A positive result — people changing behaviour in response to the dynamics — would corroborate ergodicity economics and falsify expected utility theory (insofar as experiments falsify models). If people don’t change behaviour, one would conclude that dynamic effects (at least in this experiment) are not important, and personality differences may dominate.
The experiment is described in detail in ref. 11 . Here I will only outline the setup. In the additive environment people were given a starting wealth of about $150 and then each made 312 choices between additive gambles, with fixed dollar amounts at stake, for example between tossing a coin for winning $40 or losing $30; and tossing a coin for winning $30 or losing $20. In contrast, in the multiplicative environment, the same people were also given about $150, and then made 312 choices between multiplicative gambles, with fixed proportions of wealth at stake, for example between tossing a coin for a 100% gain in wealth or a 70% loss; and tossing a coin for a 30% gain or a 20% loss.
The choices of the participants were consequential: a single decision could lead to winning or losing several hundred real dollars.
The choices observed in the two environments were used to fit a utility function of the form
The parameter η interpolates between linear, η = 0, and logarithmic, η = 1, functions, and it controls the concavity of u ( x ; η ) — larger values correspond to stronger concavity.
The Copenhagen group fed the observations into a Bayesian hierarchical model 12 , the output of which is a posterior distribution for η . Roughly, for each person in each environment this tells us how likely it is that the subject was optimizing expected changes in equation ( 9 ) with different values for η , a result shown in Fig. 3 .
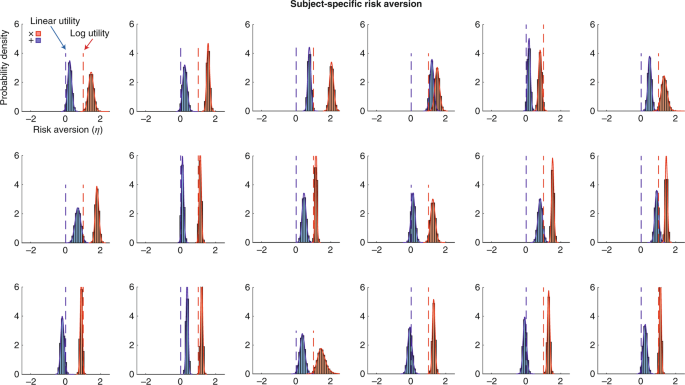
Each set of axes represents one individual, blue for the additive environment, red for multiplicative. Dashed lines are the null-model predictions of 0 and 1. All tested individuals changed behaviour noticeably in response to the wealth dynamic, in all cases the multiplicative environment led to the shift to the right predicted by ergodicity economics. Not all individuals are the same, but an overall pattern is clearly seen. Data reproduced from ref. 11 .
Expected utility theory predicts that people are insensitive to changes in the dynamics. People may have wildly different utility functions, which would be reflected in wildly different best-fit values of η , but the dynamic setting should make no difference. Utility functions are supposedly psychological or even neurological properties. They indicate personality types — risk seekers and scaredy cats.
Ergodicity economics predicts something quite different. First, it predicts that the dynamic setting significantly changes the best-fit ‘utility function’, which is really the ergodicity mapping in the relevant ergodic growth rate. The effective utility function will be different for one and the same individual under additive dynamics and under multiplicative dynamics.
The direction of the change should go towards greater ‘risk aversion’ for multiplicative dynamics — the ergodicity mapping is more concave there. The magnitude of the change in η should be about 1. And finally, if we take seriously the absolute null models of additive and multiplicative dynamics, the distributions should be centred near 0 for the additive setting and near 1 for the multiplicative setting.
Given the limitations of the experiment — for instance, people only had a one-hour training phase to get used to a given environment — these predictions don’t look so bad. Of course, the 11,232 individual choices summarized in Fig. 3 may be happenstance, or the experiment may be flawed in a way we don’t yet understand. So we might put it this way: the strong focus on psychology and lack of consideration for dynamics, prevalent in expected utility theory, corresponds to the belief that the difference between the red and blue curves is spurious.
This may be a good place to acknowledge further heroes of the story. That the geometric mean exp〈ln x 〉 is less than the arithmetic mean 〈 x 〉 was known to Euclid ( Elements , Book V, Proposition 25), and it is a special case of Jensen’s inequality 13 of 1906. Its connection to gambling and investment problems was noted by Whitworth 14 in 1870, is implied by Itô’s work 15 of 1944, and is well known among gamblers as Kelly’s criterion 16 of 1956. Our modest contribution is to frame these observations as a question of ergodicity, which we have found to be a fruitful perspective. It enforces physical realism by precluding interactions among members of a statistical ensemble, it enables us to consider dynamics other than additive and multiplicative (corresponding to linear and logarithmic utility functions), and it naturally leads to treatments of problems whose solutions are less readily visible in previous framings of the issue.
The present situation is both dispiriting and uplifting. It is dispiriting because economics is firmly stuck in the wrong conceptual space. Because the core mistake is 350 years old, the corresponding mindset is now firmly institutionalized.
However, it is also uplifting and scientifically exciting because of the many opportunities that have just opened up. The situation is similar to pre-standard model particle physics (except, with a copy of ref. 17 in the back pocket): each behavioural pattern that follows from growth rate maximization has its own narrative and vocabulary. Take discounting as an example: thousands of studies investigate subjective perceptions of the value of a dollar in the future. When expressed mathematically, the heart of this narrative becomes a story about growth rates. One has to relabel and rearrange some terms in the relevant equation, but eventually the ergodic growth rate is recovered as the fundamental concept that explains the phenomenon 18 . The same is true for expected utility theory 19 .
Similarly, we’ve learned a lot about market stability and have found a natural resolution of the equity premium puzzle 20 or — as Ken Arrow used to call it — the volatility puzzle. Growth rate optimization predicts a relationship between how fast something grows and how volatile it is. This relationship holds not only for the stock market indexes we have checked but even for bitcoin. It can be used for fraud detection: the relationship doesn’t hold for Bernie Madoff’s fraudulent fund, for example. It also suggests a protocol for setting central-bank interest rates 21 .
Perhaps the most significant change lies in the nature of the model human that arises from our conceptual reframing. Homo economicus has been criticized, perhaps most succinctly for being short-termist. Given that time is so poorly represented in mainstream economics, this should come as no surprise. Our Homo economicus , or Homo ergodicus ? — the new guy — is really rather nice. He cares about others, understands that cooperation leads to better results, and is patient and kind 22 . Nor do we have to assume huge individual differences in psychology or skill to explain the huge observed differences in wealth: a trivial null model — though one that doesn’t blindly assume ergodicity — predicts the robust features of the wealth distribution 23 , 24 , 25 . A well-known measure of inequality 26 turned out to be the time-integrated difference between ensemble and time-average growth rates in geometric Brownian motion 27 .
The model I have presented here — optimizing time-average growth rates — is a null model, and it has all the shortcomings that null models have. The improvement is clear when we compare ours to the prevailing null model of optimizing expected time-integrated discounted utility. Rather than adding correcting components to that conceptually flawed null model, we remove the conceptual flaw. The use of a null model of any kind, in my view, is a form of caution: of this complex system I only know a few simple aspects with the degree of certainty that makes it promising to incorporate them in a formal model. Adding further details would require careful checks against overfitting.
We have reason to hope for a future economic science that is more parsimonious, conceptually clearer and less subjective. It will resemble reality more closely and be better aligned with our moral intuitions.
Change history
06 december 2019.
An amendment to this paper has been published and can be accessed via a link at the top of the paper.
Ore, O. Cardano the Gambling Scholar (Princeton Univ. Press, 1953).
Devlin, K. The Unfinished Game (Basic Books, 2008).
Montmort, P. R. Essay d’analyse sur les jeux de hazard 2nd edn (Jacque Quillau, 1713; reprinted by American Mathematical Society, 2006).
Peters, O. & Gell-Mann, M. Evaluating gambles using dynamics. Chaos 26 , 23103 (2016).
Article MathSciNet Google Scholar
Bernoulli, D. Exposition of a new theory on the measurement of risk. Econometrica 22 , 23–36 (1954).
Laplace, P. S. Théorie analytique des probabilités 2nd edn (Courcier, 1814).
von Neumann, J. & Morgenstern, O. Theory of Games and Economic Behavior (Princeton Univ. Press, 1944).
Samuelson, P. A. A note on measurement of utility. Rev. Econ. Stud. 4 , 155–161 (1937).
Article Google Scholar
Frederick, S., Loewenstein, G. & O’Donoghue, T. Time discounting and time preferences: a critical review. J. Econ. Lit. 40 , 351–401 (2002).
West, G. B., Brown, J. H. & Enquist, B. J. A general model for ontogenetic growth. Nature 413 , 628–631 (2001).
Article ADS Google Scholar
Meder, D. et al. Ergodicity-breaking reveals time optimal economic behavior in humans. Preprint at https://arxiv.org/abs/1906.04652 (2019).
Lee, M. D. & Wagenmakers, E.-J. Bayesian Cognitive Modeling (Cambridge Univ. Press, 2013).
Jensen, J. L. W. V. Sur les fonctions convexes et les inégalités entre les valeurs moyennes . Acta Math. 30 , 175–193 (1906).
Whitworth, W. A. Choice and Chance 2nd edn (D. Bell and Co., 1870)
Itô, K. Stochastic integral. Proc. Imp. Acad. 20 , 519–524 (1944).
Kelly, J. L. A new interpretation of information rate. Bell Syst. Tech. J. 35 , 917–926 (1956).
Gell-Mann, M. A schematic model of baryons and mesons. Phys. Lett. 8 , 214–215 (1964).
Adamou, A., Berman, Y., Mavroyiannis, D. & Peters, O. Microfoundations of discounting. Preprint at https://arxiv.org/abs/1910.02137 (2019).
Peters, O. & Adamou, A. The time interpretation of expected utility theory. Preprint at https://arxiv.org/abs/1801.03680 (2018).
Peters, O. & Adamou, A. Leverage efficiency. Preprint at http://arXiv.org/abs/1101.4548 (2011).
Peters, O. & Adamou, A. Lecture notes. Ergodicity Economics https://ergodicityeconomics.com/lecture-notes/ (2018).
Peters, O. & Adamou, A. An evolutionary advantage of cooperation. Preprint at http://arxiv.org/abs/1506.03414 (2018).
Berman, Y., Peters, O. & Adamou, A. An empirical test of the ergodic hypothesis: wealth distributions in the United States. SSRN https://ssrn.com/abstract=2794830 (2017).
Marsili, M., Maslov, S. & Zhang, Y.-C. Dynamical optimization theory of a diversified portfolio. Physica A 253 , 403–418 (1998).
Bouchaud, J.-P. & Mézard, M. Wealth condensation in a simple model of economy. Physica A 282 , 536–545 (2000).
Theil, H. Economics and Information Theory (North-Holland Publishing Company, 1967).
Adamou, A. & Peters, O. Dynamics of inequality. Significance 13 , 32–35 (2016).
Download references
Author information
Authors and affiliations.
London Mathematical Laboratory, London, UK
You can also search for this author in PubMed Google Scholar
Corresponding author
Correspondence to Ole Peters .
Ethics declarations
Competing interests.
The author declares no competing interests.
Additional information
Publisher’s note Springer Nature remains neutral with regard to jurisdictional claims in published maps and institutional affiliations.
Rights and permissions
Reprints and permissions
About this article
Cite this article.
Peters, O. The ergodicity problem in economics. Nat. Phys. 15 , 1216–1221 (2019). https://doi.org/10.1038/s41567-019-0732-0
Download citation
Received : 29 September 2018
Accepted : 28 October 2019
Published : 02 December 2019
Issue Date : December 2019
DOI : https://doi.org/10.1038/s41567-019-0732-0
Share this article
Anyone you share the following link with will be able to read this content:
Sorry, a shareable link is not currently available for this article.
Provided by the Springer Nature SharedIt content-sharing initiative
This article is cited by
Creative evolution in economics.
- Abigail Devereaux
- Roger Koppl
- Stuart Kauffman
Journal of Evolutionary Economics (2024)
Real-space observation of ergodicity transitions in artificial spin ice
- Michael Saccone
- Francesco Caravelli
- Alan Farhan
Nature Communications (2023)
Flexibility for intergenerational justice in climate resilience decision-making: an application on sea-level rise in the Netherlands
- Jose D. Teodoro
Sustainability Science (2023)
Robust portfolio optimization for banking foundations: a CVaR approach for asset allocation with mandatory constraints
- Maria Cristina Arcuri
- Gino Gandolfi
- Fabrizio Laurini
Central European Journal of Operations Research (2023)
Uncertainty quantification and exploration–exploitation trade-off in humans
- Antonio Candelieri
- Andrea Ponti
- Francesco Archetti
Journal of Ambient Intelligence and Humanized Computing (2023)
Quick links
- Explore articles by subject
- Guide to authors
- Editorial policies
Sign up for the Nature Briefing: AI and Robotics newsletter — what matters in AI and robotics research, free to your inbox weekly.


Life-Cycle Hypothesis
Definition: The Life-cycle hypothesis was developed by Franco Modigliani in 1957. The theory states that individuals seek to smooth consumption over the course of a lifetime – borrowing in times of low-income and saving during periods of high income.
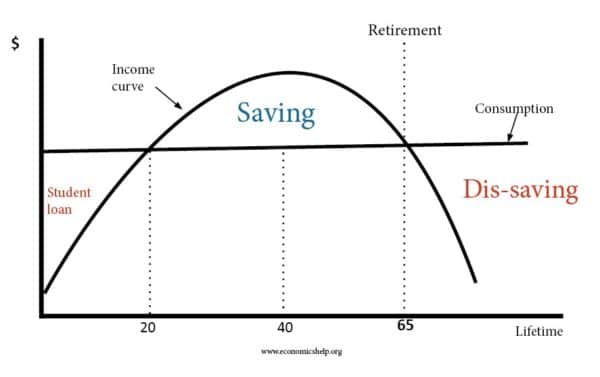
- As a student, it is rational to borrow to fund education.
- Then during your working life, you pay off student loans and begin saving for your retirement.
- This saving during working life enables you to maintain similar levels of income during your retirement.
It suggests wealth will build up in working age, but then fall in retirement.
Wealth in the Life-Cycle Hypothesis
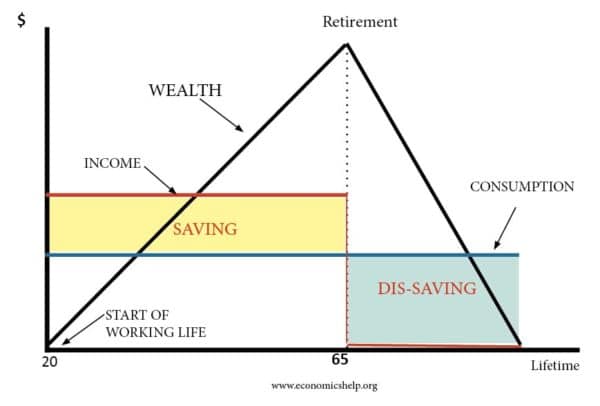
The theory states consumption will be a function of wealth, expected lifetime earnings and the number of years until retirement.
Consumption will depend on
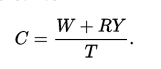
- C= consumption
- R = Years until retirement. Remaining years of work
- T= Remaining years of life
It suggests for the whole economy consumption will be a function of both wealth and income.
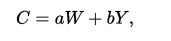
Prior to life-cycle theories, it was assumed that consumption was a function of income. For example, the Keynesian consumption function saw a more direct link between income and spending.
However, this failed to account for how consumption may vary depending on the position in life-cycle.
Motivation for life-cycle consumption patterns
- Diminishing marginal utility of income. If income is high during working life, there is a diminishing marginal utility of spending extra money at that particular time.
- Harder to work and earn money, in old age. Life Cycle enables people to work hard and spend less.
Does the Life-cycle theory happen in reality?
Mervyn King suggests life-cycle consumption patterns can be found in approx 75% of the population. However, 20-25% don’t plan in the long term. (NBER paper on economics of saving )
Reasons for why people don’t smooth consumption over a lifetime.
- Present focus bias – People can find it hard to value income a long time in the future
- Inertia and status quo bias . Planning for retirement requires effort, forward thinking and knowledge of financial instruments such as pensions. People may prefer to procrastinate – even though they know they should save more – and so saving gets put off.
Criticisms of Life Cycle Theory
- It assumes people run down wealth in old age, but often this doesn’t happen as people would like to pass on inherited wealth to children. Also, there can be an attachment to wealth and an unwillingness to run it down. See: Prospect theory and the endowment effect.
- It assumes people are rational and forward planning. Behavioural economics suggests many people have motivations to avoid planning.
- People may lack the self-control to reduce spending now and save more for future.
- Life-cycle is easier for people on high incomes. They are more likely to have financial knowledge, also they have the ‘luxury’ of being able to save. People on low-incomes, with high credit card debts, may feel there is no disposable income to save.
- Leisure. Rather than smoothing out consumption, individuals may prefer to smooth out leisure – working fewer hours during working age, and continuing to work part-time in retirement.
- Government means-tested benefits for old-age people may provide an incentive not to save because lower savings will lead to more social security payments.
Other theories
- Permanent income hypothesis of Milton Friedman – This states people only spend more when they see it as an increase in permanent income.
- Ricardian Equivalence – consumers may see tax cuts as only a temporary rise in income so will not alter spending.
- Autonomous consumption – In Keynesian consumption function, the level of consumption that is independent of income.
- Marginal propensity to consume – how much of extra income is spent.
15 thoughts on “Life-Cycle Hypothesis”
Thanks for the reminder of the theory… Am a moi university Economic student in Nairobi Kenya.
Thanks for the most summarised note ever. it will help me with presentation. Gulu university. JALON
prof premraj pushpakaran writes — 2018 marks the 100th birth year of Franco Modigliani!!!
Thanks for the analysis on the hypothesis
Nice piece of work for economist. Been applicable in my presentation at Kyambogo university Uganda
This piece of paper is very important as far as consumption is concerned…
This piece is the best I have seen so far, this is a great work
Thanks for this work.it Will help me in my presentation at metropolitan international University
Very coincise and well articulated. This work reconnects me with themechanics of consumption theories. I appreaciate for a job well done.
Very nice and comprensive information. It will help me in my exams at university of jos Nigeria, studying economics
thank u for the summarized notes,it will help me in my exam at Kibabii university
Great job. Thanks for this masterpiece.
Good job. Thanks for this masterpiece. It reconnects me with the consumption theories.
A good summarised piece of work on life cycle hypothesis, it will help me in my group presentation. Kenyatta University economics student.
Comments are closed.
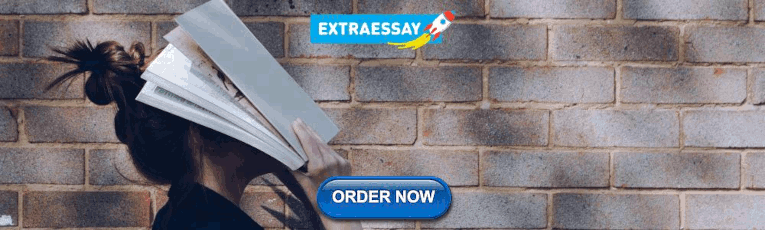
IMAGES
VIDEO
COMMENTS
Abstract. P-hacking is prevalent in reality but absent from classical hypothesis-testing theory. We therefore build a model of hypothesis testing that accounts for p-hacking. From the model, we derive critical values such that, if they are used to determine significance, and if p-hacking adjusts to the new significance standards, spurious significant results do not occur more often than ...
Economists, like other social scientists and scientists, use models to assist them in their analyses. Two problems inherent in tests of hypotheses in economics are the all-other-things-unchanged problem and the fallacy of false cause. Positive statements are factual and can be tested.
Econometrics is the application of statistical and mathematical theories in economics for the purpose of testing hypotheses and forecasting future trends. It takes economic models, tests them ...
This study delves into the intricate relationship between non-renewable energy sources, economic advancement, and the ecological footprint of well-being in Pakistan, spanning the years from 1980 to 2021. Employing the quantile regression model, we analyzed the co-integrating dynamics among the variables under scrutiny. Non-renewable energy sources were dissected into four distinct components ...
The Mises Institute is a non-profit organization that exists to promote teaching and research in the Austrian School of economics, individual freedom, honest history, and international peace, in the tradition of Ludwig von Mises and Murray N. Rothbard.
A hypothesis is an educated guess or a guess based on evidence and research. We formulate an economic question, create a hypothesis about this question, and test to accept or reject that ...
John Maynard Keynes (1883-1946), one of the greatest economists of the twentieth century, pointed out that economics is not just a subject area but also a way of thinking. Keynes famously wrote in the introduction to a fellow economist's book: "[Economics] is a method rather than a doctrine, an apparatus of the mind, a technique of thinking, which helps its possessor to draw correct ...
Life-Cycle Hypothesis (LCH): The Life-Cycle Hypothesis (LCH) is an economic theory that pertains to the spending and saving habits of people over the course of a lifetime. The concept was ...
Hypothesis Testing in Econometrics Joseph P. Romano,1 Azeem M. Shaikh,2 and Michael Wolf3 1Departments of Economics and Statistics, Stanford University, Stanford, California 94305; email: [email protected] 2Department of Economics, University of Chicago, Chicago, Illinois 60637 3Institute for Empirical Research in Economics, University of Zu¨rich, CH-8006 Zu¨rich, Switzerland
In the scientific method, hypotheses are suggested and then tested. A hypothesis is an assertion of a relationship between two or more variables that could be proven to be false. A statement is not a hypothesis if no conceivable test could show it to be false. ... Testing Hypotheses in Economics. Here is a hypothesis suggested by the model of ...
The Hypotheses to be Tested. Formal statement of the null and alternative hypotheses. H 0: >= 5,000 against. H 1: < 5,000. u a ways contains the '=' sign. This is a one tailed test, since the rejection region occupies only one side of the distribution. the alternative hypothesis suggests that the true distribution is to the left of the null ...
Hypotheses Testing in Econometrics. This course is part of Econometrics for Economists and Finance Practitioners Specialization. Taught in English. 21 languages available. Some content may not be translated. Instructor: Dr Leone Leonida. Enroll for Free. Starts May 8. Financial aid available.
Testing Restrictions on Parameters. For those who believe that economic hypotheses have to be confirmed by empirical observations, hypothesis testing is an important subject in economics. As a classical example, when an economic relation is represented by a linear regression model: $$ Y= X\beta +\upvarepsilon $$. (1)
The following points highlight the top four types of Hypothesis in Consumption. The types of Hypothesis are: 1. The Post-Keynesian Developments 2. The Relative Income Hypothesis 3. The Life-Cycle Hypothesis 4. The Permanent Income Hypothesis. Hypothesis Type # 1. The Post-Keynesian Developments: Data collected and examined in the post-Second World War period (1945-) confirmed the Keynesian ...
Hypothesis Testing in Econometrics. This article reviews important concepts and methods that are useful for hypothesis testing. First, we discuss the Neyman-Pearson framework. Various approaches to optimality are presented, including finite-sample and large-sample optimality. Then, we summarize some of the most important methods, as well as ...
The hypothesis that per capita output converges across economies over time represents one of the oldest controversies in economics. This essay surveys the history and development of the hypothesis, focusing particularly on its vast literature since the mid-1980s. A summary of empirical analyses, econometric issues, and various tests of the convergence hypothesis are also presented. Moreover ...
We have thus arrived at quantity. a definite hypothesis, and many economists have tried to make it a basis for a general theory of economics. f X dx+Y dy+Z dz We can make an estimate of the generality of such. a system. In the first place, it is essentially competi- The general condition for three variables, in order.
Department of Economics University of Chicago [email protected] Michael Wolf Institute for Empirical Research in Economics University of Zurich [email protected] September 2009 Abstract This paper reviews important concepts and methods that are useful for hypothesis test-ing. First, we discuss the Neyman-Pearson framework. Various approaches ...
The analysis of data from experiments in economics routinely involves testing multiple null hypotheses simultaneously. These different null hypotheses arise naturally in this setting for at least three different reasons: when there are multiple outcomes of interest and it is desired to determine on which of these outcomes a treatment has an effect; when the effect of a treatment may be ...
Aspirin Count Theory: A market theory that states stock prices and aspirin production are inversely related. The Aspirin count theory is a lagging indicator and actually hasn't been formally ...
The ergodic hypothesis is a key analytical device of equilibrium statistical mechanics. It underlies the assumption that the time average and the expectation value of an observable are the same.
24 May 2019 by Tejvan Pettinger. Definition: The Life-cycle hypothesis was developed by Franco Modigliani in 1957. The theory states that individuals seek to smooth consumption over the course of a lifetime - borrowing in times of low-income and saving during periods of high income. The graph shows individuals save from the age of 20 to 65.
Developing a hypothesis (with example) Step 1. Ask a question. Writing a hypothesis begins with a research question that you want to answer. The question should be focused, specific, and researchable within the constraints of your project. Example: Research question.
In economics, the absolute income hypothesis concerns how a consumer divides their disposable income between consumption and saving. [1] It is part of the theory of consumption proposed by economist John Maynard Keynes. The hypothesis was subject to further research in the 1960s and 70s, most notably by American economist James Tobin (1918-2002).
Economics. The idea of convergence in economics (also sometimes known as the catch-up effect) is the hypothesis that poorer economies ' per capita incomes will tend to grow at faster rates than richer economies. In the Solow-Swan growth model, economic growth is driven by the accumulation of physical capital until this optimum level of capital ...