
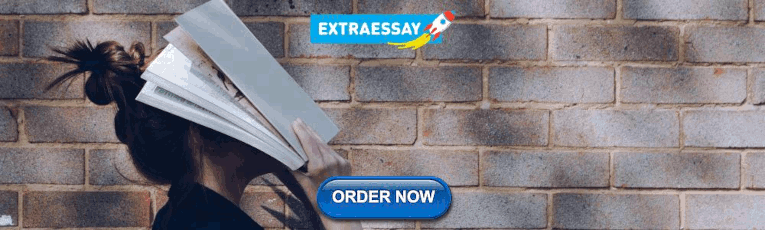
Chemistry Education Research and Practice
Exploring conceptual frameworks of models of atomic structures and periodic variations, chemical bonding, and molecular shape and polarity: a comparison of undergraduate general chemistry students with high and low levels of content knowledge.
* Corresponding authors
a Institute of Education, National Chiao Tung University, 1001 Ta-Hsueh Road, Hsinchu, Taiwan E-mail: [email protected]
b Science Education Centre, University of Missouri-Columbia, 321 Townsend Hall, Columbia, USA E-mail: [email protected]
The purpose of the study was to explore students’ conceptual frameworks of models of atomic structure and periodic variations, chemical bonding, and molecular shape and polarity, and how these conceptual frameworks influence their quality of explanations and ability to shift among chemical representations. This study employed a purposeful sampling technique and used three diagnostic instruments for conceptual understanding to determine the students’ level of content knowledge of the related concepts. Six student interviews were analyzed to portray students’ conceptual frameworks in high and low content knowledge (HCK and LCK, respectively) groups. The study’s major findings revealed that moving from a high toward a low level of content knowledge, the quality of students’ explanations declined, as did their ability to reconcile new information to their existing knowledge frameworks. Three essential concepts – models of atomic structure, effective core charge and principles of electrostatic force, and quantum mechanics descriptions – were identified that may explain students’ failure to learn the necessary aspects of molecular geometry and polarity. This study provides empirical evidence of how students’ content knowledge influences their understanding about molecular polarity. The findings have implications for college chemistry education with respect to teaching concepts about molecular polarity.
Article information
Download citation, permissions.

C. Wang and L. H. Barrow, Chem. Educ. Res. Pract. , 2013, 14 , 130 DOI: 10.1039/C2RP20116J
To request permission to reproduce material from this article, please go to the Copyright Clearance Center request page .
If you are an author contributing to an RSC publication, you do not need to request permission provided correct acknowledgement is given.
If you are the author of this article, you do not need to request permission to reproduce figures and diagrams provided correct acknowledgement is given. If you want to reproduce the whole article in a third-party publication (excluding your thesis/dissertation for which permission is not required) please go to the Copyright Clearance Center request page .
Read more about how to correctly acknowledge RSC content .
Social activity
Search articles by author, advertisements.
- Reference Manager
- Simple TEXT file
People also looked at
Editorial article, editorial: the future of nuclear structure: challenges and opportunities in the microscopic description of nuclei.
- 1 Istituto Nazionale di Fisica Nucleare, Sezione di Napoli, Napoli, Italy
- 2 Physics Department and McDonnell Center for the Space Sciences at Washington University in St. Louis, St. Louis, MO, United States
- 3 Department of Physics, University of Surrey, Guildford, United Kingdom
- 4 Dipartimento di Fisica, Universita Degli Studi di Milano, Milano, Italy
- 5 INFN, Sezione di Milano, Milano, Italy
Editorial on the Research Article The Future of Nuclear Structure: Challenges and Opportunities in the Microscopic Description of Nuclei
The past two decades have witnessed tremendous progress in the microscopic description of atomic nuclei. Within this approach, nuclei are described in terms of nucleons interacting via realistic two- and three-body forces, constrained to accurately reproduce a large body of data for few nucleons systems. The goal of the nuclear theory community is to gain an accurate and predictable understanding of how the properties of many-body systems, along with their dynamics and structure, emerge from internucleon correlations induced by the strong interaction.
Progress in the microscopic (or, ab initio ) theory has been quite notable and it has been supported by two major pillars: First, thanks to the advent of Effective Field Theories (EFTs), we can now systematically develop nuclear Hamiltonians that are rooted in the fundamental properties and symmetries of the underlying theory of QCD. Second, advances in computational resources and novel powerful algorithms allow us to solve 1) the many-nucleon problem efficiently, and 2) quantify the degree of reliability of theoretical calculations and predictions. In many cases, microscopic computations achieve an accuracy that is comparable or superior to the precision delivered by current EFT interactions. This sparked a renewed interest to further broaden the focus of ab initio theory and address open problems in nuclear physics.
While the status of the first pillar has been recently discussed by “The Long-Lasting Quest for Nuclear Interactions: The Past, the Present and the Future” Topical Review on this Journal, here we focus on the exciting new developments in microscopic theory. At present, ab initio computations of nuclear structure include up to medium-mass isotopes. The heaviest systems currently reached—with different degrees of accuracy—have mass number A ≈ 140 . These computational limits are constantly being pushed forward. At the same time, the community is expanding into new directions, in particular toward the study of electroweak observables and nuclear reactions, that nowadays require predictions with an accuracy never reached before for similar mass ranges.
In collecting the contributions for this Research Topic, we sought to gather contributions from authors who could summarize the current state-of-the-art microscopic calculations in Nuclear Theory, favoring a selected but broad view over an attempt to cover every application. All presented contributions stem from well-established methods in computational nuclear structure, and indicate recent theoretical advances and prospective outlooks, challenges and opportunities for Nuclear Theory. Most importantly, it is our hope that this collection will confer a big picture, including references to basic material, that will be valuable for young researches who intend to enter this exciting discipline.
The richness of applications in modern ab initio nuclear theory can be appreciated in Hergert ’s contribution that provides us with a general overview of the most successful microscopic many-body approaches currently in use. Traditionally, the refinement and sophistication of these computational tools has given fundamental support to advance the theories of nuclear forces. Quantum Monte Carlo (QMC) techniques allow to solve the many-body Schrödinger equation with high accuracy for light nuclei up to masses A ∼ 16–40. Gandolfi et al. discuss the use of QMC methods (namely, Variational, Green’s Function, and Auxiliary Diffusion Monte Carlo methods) in combination with local chiral interactions in coordinate space. QMC methods are used in lattice effective field theory, where the EFT Lagrangian is implemented in momentum space with nucleons and pions placed on a lattice. Lee discusses the basic features of this approach and its high potential for understanding clustering phenomena.
For heavier isotopes, ab initio theories can be pushed to masses A ∼ 140 provided that one retains only the relevant nuclear excitations, as it is done through all-orders resummations. Among these methods, the self-consistent Green’s function (SCGF) theory gives direct access to the spectral information probed by a wide range of experiments as reviewed in detail by Somà ’s contributions. Once in the region of the nuclear chart that corresponds to medium masses, open shell isotopes become the next challenge to be addressed by the theory. In fact, resolving the degeneracy in uncorrelated systems requires large scale configuration mixing. Coraggio and Itaco demonstrate how this can be handled by projecting the correlated many-body states into a shell model Hamiltonian, using the so-called “Q-box” formalism. A similar strategy is shared by other computational frameworks, such as coupled cluster and in-medium SRG, that are touched upon in the contribution by Hergert . A less conventional approach to open shells is to break SU(1) symmetry (in short, allowing for breaking particle number conservation). This is discussed by Somà within SCGFs and by Tichai et al. in the framework of many-body perturbation theory.
The remainder of this topical review focuses on selected open challenges in Nuclear Theory that require an ab initio approach. Two contributions show different aspect of studying infinite nucleon systems and the implications for astrophysical scenarios. Tews covers QMC calculations of the equation of state (EoS) of dense matter in neutron stars. With the recent observation of star mergers and the birth of multi-messenger astronomy, it has become of prime importance to understand the finite temperature properties of the EoS. Rios discusses this topic and how the structure of neutron matter depends on temperature, using SCGF theory.
In the quest for physics beyond the Standard Model, Nuclear Theory, and in particular accurate calculations of neutrino-nucleus interactions at all energy scaler, plays a crucial role. This is carefully analyzed by Rocco ’s contribution that address this challenge with emphasis on impacts to neutrino oscillations experimental programs. The last contribution of this Topical Review addresses one of the hardest open challenges in the interpretation of experimental data: the lack of a truly first-principles theory that can describe consistently both structure and reaction processes. Rotureau highlights recent steps in deriving an ab inito optical potential using the coupled cluster method (that, together with SCGF, is one of the two possible approaches to this problem).
We are really grateful to all the scientists participating in this project and hope that the reader will enjoy this Topical Review.
Author Contributions
All authors listed have made a substantial, direct, and intellectual contribution to the work and approved it for publication.
Conflict of Interest
The authors declare that the research was conducted in the absence of any commercial or financial relationships that could be construed as a potential conflict of interest.
The handling editor declared a past co-authorship with one of the authors SP.
Keywords: Ab initio (calculations), nuclear theory, nuclear reactions, effective field theories, many-body physics, nuclear structure
Citation: Coraggio L, Pastore S and Barbieri C (2021) Editorial: The Future of Nuclear Structure: Challenges and Opportunities in the Microscopic Description of Nuclei. Front. Phys. 8 :626976. doi: 10.3389/fphy.2020.626976
Received: 07 November 2020; Accepted: 20 November 2020; Published: 05 February 2021.
Edited and Reviewed by:
Copyright © 2021 Coraggio, Pastore and Barbieri. This is an open-access article distributed under the terms of the Creative Commons Attribution License (CC BY). The use, distribution or reproduction in other forums is permitted, provided the original author(s) and the copyright owner(s) are credited and that the original publication in this journal is cited, in accordance with accepted academic practice. No use, distribution or reproduction is permitted which does not comply with these terms.
*Correspondence: Luigi Coraggio, [email protected] ; Saori Pastore, [email protected] ; Carlo Barbieri, [email protected]
This article is part of the Research Topic
The Future of Nuclear Structure: Challenges and Opportunities in the Microscopic Description of Nuclei
Historical Teaching of Atomic and Molecular Structure
- First Online: 30 December 2013
Cite this chapter
- José Antonio Chamizo 2 &
- Andoni Garritz 2
6382 Accesses
1 Citations
1 Altmetric
Besides the presentation and conclusions, the chapter is divided into two equally important sections. The first one describes the modern development of atomic and molecular structure, emphasising some of the philosophical problems that have been taken, and those that have to be faced in its understanding. The second discusses the alternative conceptions and difficulties of students of different educational levels and also the different approaches to its historical or philosophical teaching. Finally, we recognise the necessity for science teachers to assume a specific historical-philosophical position.
This is a preview of subscription content, log in via an institution to check access.
Access this chapter
- Available as PDF
- Read on any device
- Instant download
- Own it forever
- Available as EPUB and PDF
- Compact, lightweight edition
- Dispatched in 3 to 5 business days
- Free shipping worldwide - see info
- Durable hardcover edition
Tax calculation will be finalised at checkout
Purchases are for personal use only
Institutional subscriptions
See also Moreno-Ramírez et al. ( 2010 ).
In German he says ‘Die Energie eines Resonators ändert sich durch Absorption und Emision sprungweise, und zwar ein ganzzahliges Vielfache von (R/N)βν’ (Einstein 1906 , p. 202).
See, for example, Lee et al. ( 1993 ), Novick and Nussbaum ( 1978 , 1981 ), Nussbaum ( 1985 ), Valanides ( 2000 ), and Wightman et al. ( 1987 ).
As can be seen in Birk and Kurtz ( 1999 ), Boo ( 1998 ), Furió and Calatayud ( 1996 ), Griffiths and Preston ( 1992 ), Hund ( 1977 ), Kutzelnigg ( 1984 ), Magnasco ( 2004 ), Özmen ( 2004 ), and Sutcliffe ( 1996 ).
For example, Coll and Treagust ( 2002 ), Niaz ( 2001 ), and Peterson et al. ( 1989 ).
Such as in Coll and Treagust ( 2003a ) and De Posada ( 1997 , 1999 ).
See, for example, Butts and Smith ( 1987 ), Coll and Treagust ( 2003b ), and Taber ( 1994 , 1997 ).
Such as Dobson et al. ( 2000 ), Petri and Niedderer ( 1998 ), Shiland ( 1995 , 1997 ), and Tsaparlis and Papaphotis ( 2002 , 2009 ).
For example, Hadzidaki et al. ( 2000 ), Johnston et al. ( 1998 ), Kalkanis et al. ( 2003 ), Michelini et al. ( 2000 ), Paoloni ( 1982 ), and Wittmann et al. ( 2002 ).
As can be seen in Ardac ( 2002 ), Melrose and Scerri ( 1996 ), Niaz and Fernández ( 2008 ), and Scerri ( 1991 ).
For example, Cervellati and Perugini ( 1981 ), Conceicao and Koscinski ( 2003 ), Ogilvie ( 1994 ), Scerri ( 2000a ), Taber ( 2002a , b ; 2005 ), and Tsaparlis ( 1997a ).
For example, Buchwald and Warwick ( 2001 ), Giunta ( 2010 ), Marinacci ( 1995 ), Nye ( 1993 ), Snow ( 1981 ), and Toulmin and Goodfield ( 1962 ).
Achinstein, P. (2001). Who really discovered the electron? In Buchwald J.Z. & Warwick A. (eds.) Histories of the Electron. The Birth of Microphysics , (Chapter 13 pp. 403–424), Cambridge, Massachusetts: The MIT Press.
Google Scholar
Adúriz-Bravo A. (2012) A ‘Semantic’ View of Scientific Models for Science Education, Science & Education , Online First, 17 January.
Anderson, P. W. (1972). More Is Different, Science , 177(4047), 393–396. Aug. 4.
Arabatzis, T. (2001). The Zeeman Effect and the Discovery of the Electron? In Buchwald J.Z. & Warwick A. (eds.) Histories of the Electron. The Birth of Microphysics , (Chapter 5 pp. 171–193), Cambridge, Massachusetts: The MIT Press.
Ardac, D. (2002). Solving quantum number problems: An examination of novice performance in terms of conceptual based requirements, Journal of Chemical Education , 79(4), 510–3.
Atkins, P., de Paula, J., & Friedman, R. (2008). Quanta, Matter and Change: A Molecular Approach to Physical Chemistry , Oxford: Oxford University Press.
Ayar, M., & Yalvac, B. (2010). A sociological standpoint to authentic scientific practices and its role in school science teaching, Ahi Evran Uni. Kirsehir Journal of Education (KEFAD) 11, 113–127.
Baggott, J. (2011). The Quantum Story. A History in 40 Moments . Oxford: Oxford University Press.
Bensaude-Vincent, B. (1999). Atomism and Positivism: A legend about French Chemistry, Annals of Science , 56, 81–94.
Bent, H. A. (1984). Should orbitals be X-rated in beginning chemistry courses? Journal of Chemical Education , 61(5), 421–423.
Birk, J., & Kurtz, M. (1999). Effect of experience on retention and elimination of misconceptions about molecular structure and bonding, Journal of Chemical Education , 76(1), 124–128.
Bishop, D. M. (1973). Group theory and chemistry , Oxford, UK: Clarendon Press.
Boo, H. K. (1998). Students’ Understandings of Chemical Bonds and the Energetics of Chemical Reactions, Journal of Research in Science Teaching , 35(5), 569–581.
Branch, G.E.K. (1984). Gilbert Newton Lewis, 1875–1946, Journal of Chemical Education , 61(1), 18–21.
Bucat, R., & Mocerino, M. (2009). Learning at the Sub-micro Level: Structural Representations, in Gilbert, J. K. & Treagust, D. (Eds.) Multiple Representations in Chemical Education , (Chapter 1, pp. 11–29), Secaucus, NJ, USA: Springer.
Buchwald, J. Z. & Warwick, A. (ed) (2001). Histories of the electron. The Birth of microphysics , Cambridge Massachusetts: The MIT Press.
Butts, B., & Smith, R. (1987). HSC chemistry students’ understanding of the structure and properties of molecular and ionic compounds, Research in Science Education , 17, 192–201.
Campbell, J. A. (1962). Chemical Education Material Study . Berkeley, CA, USA: Lawrence Hall of Science.
Cervellati, R. & Perugini, D. (1981). The understanding of the atomic orbital concept by Italian high school students, Journal of Chemical Education , 58(7), 568–9.
Chalmers, A. (1998). Retracing the Ancient Steps to atomic theory, Science & Education , 7(1), 69–84.
Chamizo, J.A. (1992). El maestro de lo infinitamente pequeño . John Dalton [The master of the infinitely small. John Dalton], México: Conaculta-Pangea.
Chamizo, J.A. (2001) El curriculum oculto en la enseñanza de la química, Educación Química , 12(4), 194–198.
Chamizo, J. A. (2007). Teaching modern chemistry through ‘historical recurrent teaching models’, Science & Education , 16(2), 197–216.
Chamizo, J.A. (2011). A new definition of Models and Modelling for chemistry Teaching, Science & Education OnLine First 01 November, special issue on [Philosophical Considerations in Teaching of Chemistry] edited by Sibel Erduran.
Chamizo, J.A. (2012). Heuristic Diagrams as a Tool to teach History of Science, Science & Education , 21(5), 745–762. OnLine First 23th August, 2011.
Christie, M. & Christie, J. R. (2000). ‘Laws’ and ‘Theories’ in Chemistry Do not Obey The rules in Bhushan N. & Rosenfeld S. (ed) Of Minds and Molecules. New Philosophical Perspectives on Chemistry , New York: Oxford University Press.
Coll, R. K., & Treagust, D. F. (2002). Exploring tertiary students’ understanding of covalent bonding, Research in Science and Technological Education , 20, 241–267.
Coll, R. K., & Treagust, D. F. (2003a). Learners’ mental models of metallic bonding: A cross-age study, Science Education , 87(5), 685–707.
Coll, R. K., & Treagust, D. F. (2003b). Investigation of secondary school, undergraduate, and graduate learners’ mental models of ionic bonding, Journal of Research in Science Teaching , 40(5), 464–486.
Conceicao, J., & Koscinski, J. T. (2003). Exploring Atomic and Molecular Orbital in Freshman Chemistry using Computational Chemistry, The Chemical Educator , 8, 378–382.
Cotton, F. A. (1963). Chemical Applications of Group Theory , New York: John Wiley & Sons.
Cruz, D., Chamizo, J. A. y Garritz, A. (1986). Estructura atómica. Un enfoque químico [Atomic structure. A chemical approach], Wilmington, DE, USA: Addison Wesley Iberoamericana.
De Posada, J. M. (1997). Conceptions of high school students concerning the internal structure of metals and their electric conduction: structure and evolution, Science Education , 81(4), 445–467.
De Posada, J. M. (1999). The presentation of metallic bonding in high school science textbooks during three decades: science educational reforms and substantive changes of tendencies, Science Education , 83, 423–447.
Develaki, M. (2007). ‘The Model-Based view of Scientific Theories and the structuring of school science, Science & Education , 16(7–8), 725–749.
Didis, N. & SakirErkoc, S. (2009). ‘History of Science for Science Courses: “Spin” Example from Physics, Latin American Journal of Physics Education , 3, 9–12.
Dirac, P.A.M. (1929). Quantum Mechanics of Many-Electron Systems, Proceedings of the Royal Society (London) A123, 714–733.
Dobson, K., Lawrence, I., & Britton, P. (2000). The A to B of quantum physics, Physics Education , 35, 400–5.
Doyle M. (ed) (1993). Historical Science Experiments on File, Facts on File , New York.
Duschl, R. A. (1994). Research on the History and Philosophy of Science, in Gabel D. (Ed.) Handbook of Research on Science Teaching and Learning , (pp. 443–465) New York: MacMillan.
Early, J. E. (2004). Would Introductory Chemistry Courses work better with a new Philosophical basis? Foundations of Chemistry , 6, 137–160.
Echeverria, J. Introducción a la Metodología de la Ciencia , [Introduction to Science’s Methodology] Madrid: Cátedra, 2003.
Eggen, P.O., Kvittingen, L., Lykknes, A., & Wittje, R. (2012). Reconstructing Iconic Experiments on Electrochemistry: Experiences from a History of Science Course. Science & Education , 21, 179–189.
Einstein, A. (1906). Zur Theorie der Lichterzeugung und Lichtabsorption, Annals of Physics , 325, 199–206.
Einstein, A. (1909). Zum gegenwärtigen Stand des Strahlungsproblems, Phys. Zeitschr. 10, 185–193.
Einstein, A. (1926; 1944; 1948). Letters to Max Born; The Born-Einstein Letters, translated by Irene Born , New York: Walker and Company, 1971. Taken from the URL http://www.spaceandmotion.com/quantum-theory-albert-einstein-quotes.htm
Erduran, S., & Scerri, E. (2002). ‘The nature of chemical knowledge and chemical education’, in Gilbert J.K. et al. (eds.) Chemical Education: Towards Research-based Practice , Kluwer, Dordrecht.
Erduran, S. (2005). Applying the Philosophical Concept of Reduction to the Chemistry of Water: Implications for Chemical Education, Science & Education , 14: 161–171.
Feldman, B. (2001). The Nobel Prize: A History of Genius, Controversy, and Prestige , New York, USA: Arcade Publishing, Reed Business Information, Inc.
Feynman, R. (1985). The Strange Theory of Light and Matter . London: Penguin.
Furió, C. & Calatayud, M. L. (1996). Difficulties with the Geometry and Polarity of Molecules. Beyond Misconceptions, Journal of Chemical Education , 73(1), 36–41.
Gagliardi, R. (1988) Cómo utilizar la historia de las ciencias en la enseñanza de las ciencias, [How to use history of sciences in the teaching of sciences], Enseñanza de las Ciencias , 6, 291–296.
Garritz, A. (2013). Teaching the Philosophical Interpretations of Quantum Mechanics and Quantum Chemistry through Controversies. Accepted for publication in the special issue on [Philosophical Considerations in Teaching of Chemistry] edited by Sibel Erduran, Science & Education , 22(7), 1787–1808.
Gault, C. (1991) History of science, individual development and science teaching, Research in Science Education , 21, 133–140.
Gell-Mann, M. (1994). The Quark and the Jaguar: adventures in the simple and the complex , New York, USA: Freeman.
Giere, R. N. (1999). Science without laws , Chicago, USA: University of Chicago Press.
Gilbert, J. K. (2006). On the Nature of “Context” in Chemical Education, International Journal of Science Education , 28(9), 957–976.
Gillespie, R. J. (1991). What is wrong with the general chemistry course? Journal of Chemical Education , 68(3), 192–4.
Giunta, C. (2010). Atoms in Chemistry: From Dalton’s predecessors to Complex Atoms and Beyond , American Chemical Society-Oxford University Press, Washington.
Griffiths, A. K., & Preston, K. R. (1992). Grade-12 students’ misconceptions relating to fundamental characteristics of atoms and molecules, Journal of Research in Science Teaching , 29, 611–628.
Grosslight, L., Unger, C., Jay, E., & Smith, C. (1991). Understanding models and their use in scienceconceptions of middle and high school students and experts. Journal of Research in Science Teaching , 28, 799–822.
Hacking, I. (1983). Representing and Intervening , Cambridge, UK: Cambridge University Press.
Hadzidaki, P., Kalkanis, G. & Stavrou, D. (2000). Quantum mechanics: A systemic component of the modern physics paradigm, Physics Education , 35, 386–392.
Hargittai, M. & Hargittai, I. (2009). Group Symmetry through the Eyes of a Chemist , 3rd edition, Dordrecht, The Netherlands: Springer.
Harré, R. (2004). Modelling: Gateway to the Unknown , Amsterdam: Elsevier.
Harris, D. C. & Bertolucci, M. D. (1978). Symmetry and spectroscopy. An introduction to vibrational and electronic spectroscopy , New York: Dover.
Harrison, A. G., & Treagust, D. F. (1996). Secondary students’ mental models of atoms and molecules: Implications for teaching science, Science Education , 80, 509–534.
Hawkes, S. J. (1992). Why should they know that? Journal of Chemical Education , 69(3), 178–181.
Heilbron, J. L. & Kuhn, T. S. (1969). The Genesis of the Bohr Atom, Historical Studies in the Physical Sciences . 1(3–4), 211–290.
Herrestein-Smith, B. (1981). Narrative Versions, Narrative Theories. In W. Mitchel (Ed.), On Narrative , (pp 209–232) Chicago: University of Chicago Press.
Hoffmann, R. (1998) Qualitative thinking in the age of modern computational chemistry-or what Liones Salem knows, Journal of Molecular Structure , 424: 1–6
Hohenberg, P. & Kohn, W. (1964). Inhomogeneous electron gas, Physical Review , 136, B864–71.
Holbrow, C. H., Amato, J. C., Galvez, E. J. & Lloyd, J. N. (1995). Modernizing Introductory Physics, American Journal of Physics , 63, 1078–1090.
Hund, F. (1977). Early History of the Quantum Mechanical Treatment of the Chemical Bond, Angewandte Chemie , International Edition in English, 16, 87–91.
Husbands, C. (2003). What is history teaching? Language, ideas and meaning in learning about the past. Buckingham: Open University Press.
Izquierdo, M. & Adúriz, A. (2009). Physical construction of the chemical atom: Is it Convenient to go All the Way Back? Science & Education , 18(3–4), 443–455.
Izquierdo, M. (2010). La transformación del átomo químico en una partícula física ¿se puede realizar el proceso inverso? In Chamizo J.A. (ed) Historia y Filosofía de la Química [History and philosophy of chemistry], (pp 195–209) México: Siglo XXI-UNAM.
Jensen, W. B. (1980). The Lewis acid–base concepts , New York, Wiley.
Jensen, W. B. (1998). Logic, History, and the Chemistry Textbook. I. Does Chemistry Have a Logical Structure? Journal of Chemical Education , 75(6), 679–687; II. Can We Unmuddle the Chemistry Textbook? 75(7), 817–828; III. One Chemical Revolution or Three? 75(8), 961–969.
Jensen, W.B (ed) (2002). Mendeleev on the Periodic Law. Selected Writings, 1869–1905 , New York, Dover.
Jensen, W.B. (2010). Four Centuries of Atomic Theory in Giunta C. (ed) Atoms in Chemistry: From Dalton’s predecessors to Complex Atoms and Beyond , American Chemical Society-Oxford University Press, Washington.
Jensen, W. P., Palenik, G. J., & Suh, I. (2003). The History of Molecular Structure Determination Viewed through the Nobel Prizes, Journal of Chemical Education , 80(7), 753–761.
Johnston, I. D., Crawford, K., & Fletcher, P. R. (1998). Student difficulties in learning quantum mechanics, International Journal of Science Education , 20(5), 427–446.
Justi, R., & Gilbert, J. (2000). History and philosophy of science through models: some challenges in the case of ‘the atom, International Journal of Science Education , 22(9), 993–1009.
Kalkanis, G., Hadzidaki, P., & Stavrou, D. (2003). An instructional model for a radical conceptual change towards quantum mechanics concepts, Science Education , 87, 257–280.
Karakostas, V. & Hadzidaki, P. (2005). Realism vs. Constructivism in Contemporary Physics: The Impact of the Debate on the Understanding of Quantum Theory and its Instructional Process, Science & Education , 14(7–8), 607–629.
Kauffman, G. B. & Kauffman, L. M. (1996). An Interview with Linus Pauling, Journal of Chemical Education , 73(1), 29–32.
Kauffman, G. B. (1999). From Triads to Catalysis: Johann Wolfgang Döbereiner (1780–1849) on the 150th Anniversary of His Death, The Chemical Educator , 4, 186–197.
Kauffman, G. B. (2004). Sir William Ramsay: Noble Gas Pioneer. On the 100th Anniversary of His Nobel Prize, The Chemical Educator , 9, 378–383.
Kauffman, G. B. (2006). Radioactivity and Isotopes: A Retrospective View of Frederick Soddy (1877.1956) on the 50th Anniversary of His Death, The Chemical Educator , 11, 289–297.
Kauffman, G. B. (2010). The 150th Anniversary of the First International Congress of Chemists, Karlsruhe, Germany, September 3–5, 1860, The Chemical Educator , 15, 309–320.
Klassen, S. (2007). The Construction and Analysis of a Science Story: A Proposed Methodology, Proccedings of the International History and Philosophy of Science Teaching Group Conference , Calgary, Canada.
Klassen, S. (2008). The Photoelectric Effect: Rehabilitating the Story for the Physics Classroom’ Proceedings of the Second International Conference on Story in Science Teaching , Munich, Germany.
Kleppner, D., & Jackiw, R. (2000). One Hundred Years of Quantum Physics, Science , 289(5481), 893–898.
Kohn, W., & Sham, L. J. (1965). Self-consistent equations including exchange and correlation effects, Physical Review ,140, A1133–8.
Kuhn, T. S. (1969). The structure of scientific revolutions , Chicago: University of Chicago Press.
Kuhn, T. S. (1978). Black-Body Theory and the Quantum Discontinuity 1894–1912 , Oxford, UK: Oxford University Press.
Kutzelnigg, W. (1984). Chemical Bonding in Higher Main Group Elements, Angew. Chem. Int. Ed. Engl. 23, 272–295.
Langmuir, I. (1919). The Arrangement of Electrons in Atoms and Molecules, J.Am. Chem.Soc , 41, 868–934
Laloë, F. (2001). Do we really understand quantum mechanics? Strange correlations, paradoxes, and theorems, American Journal of Physics , 69, 655–701.
Laudan, L. (1997). Progress and its Problems: Toward a theory of scientific growth , Berkeley: University of California Press.
Lee, O., Eichinger, D. C., Anderson, C. W., Berkheimer, G. D., & Blakeslee, T.D. (1993). Changing Middle School Student’s Conception of Matter and Molecules, Journal of Research in Science Teaching , 30(3), 249–270.
Lewis, G. N. (1923). Valence and the Structure of Atoms and Molecules , New York: Dover.
Lombardi, O. & Labarca, M. (2005). The Ontological Autonomy of The Chemical World, Foundations of CHemistry , 7, 125–148.
Martin B. & Richards E. (1995). Scientific knowledge, controversy, and public decision-making, in Published in Jasanoff, S., Markle, G.E., Petersen, J.C. & Pinch T. (eds.), Handbook of Science and Technology Studies (Newbury Park, CA: Sage.
Matthews, M. R. (1994/2014). Science teaching: The role of history and philosophy of science. London: Routledge.
Matthews, M.R. (1992). History, Philosophy and Science Teaching: The Present Rapprochement, Science & Education 1(1), 11–48.
Magnasco, V. (2004). A Model for the Chemical Bond, Journal of Chemical Education , 81(3), 427–435.
Marinacci, B. (1995) (Ed) Linus Pauling in his own words , Simon&Schuster, New York
Melrose, M. P., & Scerri, E. R. (1996). Why the 4s Orbital Is Occupied before the 3d, Journal of Chemical Education , 73(6), 498–503.
Metz, D., Klassen, S., Mcmillan, B., Clough, M., & Olson, J. (2007). Building a Foundation for the Use of Historical Narratives, Science & Education , 16(3–5), 313–334.
Morrison M. (2001). History and Metaphysics: On the Reality of Spin, In Buchwald J.Z. & Warwick A. (eds.) Histories of the Electron. The Birth of Microphysics , (Chapter 14 pp. 425–450), Cambridge, Massachusetts: The MIT Press.
Michelini, M., Ragazzon, R., Santi, L., & Stefanel, A. (2000). Proposal for quantum physics in secondary school, Physics Education , 35(6), 406–410.
Moreno-Ramírez, J. E., Gallego-Badillo, R. and Pérez-Miranda, R. (2010). El modelo semicuántico de Bohr en los libros de texto [The semi-quantum Bohr’s model in textbooks], Ciência & Educação , 16(3), 611–629.
Nachtrieb N.H. (1975) Interview with Robert S. Mulliken, Journal of Chemical Education , 52(9), 560–563.
Nash, L. K. (1957). “The Atomic-Molecular Theory.” In James Bryant Conant (Ed.) Harvard Case Histories in Experimental Science , Vol. 1. Cambridge, MA, USA: Harvard University Press.
Niaz, M. (2000). The oil drop experiment: a rational reconstruction of the Millikan-Ehrenhaft controversy and its implications for chemistry textbooks, Journal of Research in Science Teaching , 37(5), 480–508.
Niaz, M. (2001). A rational reconstruction of the origin of the covalent bond and its implications for general chemistry textbooks, International Journal of Science Education , 23, 623–641.
Niaz, M. (2009). Critical Appraisal of Physical Science as a Human Enterprise. Dynamics of Scientific Progress . Dordrecht, The Netherlands: Springer Academic Publishers.
Niaz, M. (2010). Science curriculum and teacher education: The role of presuppositions, contradictions, controversies and speculations vs. Kuhn’s normal science, Teaching and Teacher Education , 26, 891–899.
Niaz, M., & Fernández, R. (2008). Understanding quantum numbers in general chemistry textbooks, International Journal of Science Education , 30(7), 869–901.
Norris, S., Guilbert, M., Smith, M., Shaharam, H., & Phillips, L. (2005). A theoretical Framework for Narrative Explanation in Science, Science Education , 89(4) 535–554.
Novick, S., & Nussbaum, J. (1978). Junior High School Pupils’ Understanding of the Particulate Nature of Matter: An Interview Study, Science Education , 62[3], 273–281.
Novick, S., & Nussbaum, J. (1981). Pupils’ Understanding of the Particulate Nature of Matter: A Cross-Age Study, Science Education , 65[2], 187–196.
Nuffield Foundation (1967). Chemistry. Handbook for teachers , London: Longmans/Penguin Books.
Nussbaum, J. (1985). The Particulate Nature of Matter in the Gaseous Phase. In R. Driver, E. Guesne y A. Tiberghien (Eds.), Children's Ideas in Science , (pp. 125–144) Philadelphia: Open University Press.
Nye, M. J. (1993). From Chemical Philosophy to Theoretical Chemistry , University of California Press, Berkeley
Ogilvie, J. F. (1994). The Nature of the Chemical Bond 1993. There are No Such Things as Orbitals!, in E. S. Kryachko and J. L. Calais (eds.), Conceptual Trends in Quantum Chemistry , (pp. 171–198), Dordrecht, The Netherlands: Kluwer.
Özmen, H. (2004). Some Student Misconceptions in Chemistry: A Literature Review of Chemical Bonding, Journal of Science Education and Technology , 13(2), 147–159.
Pagliaro, M. (2010). On shapes, molecules and models: An insight into chemical methodology, European Journal of Chemistry , 1, 276–281.
Panusch, M., Singh, R., & Heering, P. (2008). How Robert A. Millikan got the Physics Nobel Prize’. Proceedings of the Second International Conference on Story in Science Teaching , Munich, Germany.
Paoloni, L. (1982). Classical mechanics and quantum mechanics: an elementary approach to the comparison of two viewpoints, European Journal of Science Education , 4, 241–251.
Paraskevopoulou, E. and Koliopoulos, D. (2011). Teaching the Nature of Science Through the Millikan-Ehrenhaft Dispute, Science & Education , 20(10), 943–960. Published online 26 September 2010.
Park, E: J. & Light, G. (2009). Identifying Atomic Structure as a Threshold Concept: Student mental models and troublesomeness, International Journal of Science Education , 31(2), 895–930.
Peterson, R. F., Treagust, D. F., & Garnett, P. (1989). Development and application of a diagnostic instrument to evaluate grade 11 and 12 students’ concepts of covalent bonding and structure following a course of instruction, Journal of Research in Science Teaching , 26(4), 301–314.
Petri, J., & Niedderer, H. (1998). A learning pathway in high-school level quantum atomic physics, International Journal of Science Education , 20(9), 1075–1088.
Piaget, J. & Garcia, R. (1983). Psychogenesis and the history of science . New York, Columbia University Press.
Pospiech, G. (2000). Uncertainty and complementarity: The heart of quantum physics, Physics Education , 35(6), 393–399.
Popper, K. (1969). Conjectures and Refutations , London: Routledge and Kegan Paul.
Primas, H. (1983) Chemistry, Quantum Mechanics and Reductionism: Perspectives in theoretical chemistry , Berlin, Springer.
Purser, G. H. (2001). Lewis structure in General Chemistry: Agreement between electron density calculations and Lewis structures, Journal of Chemical Education , 78(7), 981–983.
Reichenbach, H. (1938). Experience and prediction: an analysis of the foundations and the structure of knowledge . Chicago: University of Chicago Press.
Reichenbach, H. (1978, [1929]) The aims and methods of physical knowledge pp 81–125 in Hans Reichenbach: Selected writings 1909–1953 (M. Reichenbach and R.S. Cohen, Eds; principal translations by E.H. Schneewind), volumen II, Dordrecht: Reidel.
Reish, G. A. (2005). How the Cold War transformed Philosophy of Science. To the Icy Slopes of Logic , New York, Cambridge University Press (Versión en español Cómo la Guerra fria transformó la filosofía de la ciencia. Hacia las heladas laderas de la lógica , Buenos Aires, Universidad de Quilmes Editorial, 2009).
Rodríguez, M., & Niaz, M. (2004). A Reconstruction of Structure of the Atom and Its Implications for General Physics Textbooks: A History and Philosophy of Science Perspective, Journal of Science Education and Technology , Vol. 13, No. 3.
Scerri, E. R. (1991). Electronic Configurations, Quantum Mechanics and Reduction, British Journal for the Philosophy of Science , 42(3), 309–25.
Scerri, E. R. (2000a). Have Orbitals Really Been Observed? Journal of Chemical Education , 77(11), 1492–4.
Scerri, E. R. (2000b). The failure of Reduction and How to Resist Disunity of the Sciences in the Context of Chemical Education, Science & Education , 9, 405–425.
Scerri, E. R. (2001). The Recently Claimed Observation of Atomic Orbitals and Some Related Philosophical Issues, Philosophy of Science, 68 (Proceedings) S76-S88, N. Koertge, ed. Philosophy of Science Association, East Lansing, MI
Scerri, E. R. (2007). The Periodic Table: Its Story and Its Significance , Oxford University Press, New York.
Scheffel, L., Brockmeier, W., & Parchmann, L. (2009). Historical material in macro-micro thinking: Conceptual change in chemistry education and the history of chemistry. In Gilbert, J. K. & Treagust, D. F. (Eds.). (2009). Multiple representations in chemical education (pp. 215–250). Springer.
Schummer, J. (1998). The Chemical Core of Chemistry I: A Conceptual Approach, HYLE-International Journal for Philosophy of Chemistry , 4, 129–162.
Schummer, J. (1999). Coping with the Growth of Chemical Knowledge: Challenges for Chemistry Documentation, Education, and Working Chemists, Educación Química , 10(2), 92–101.
Schummer, J. (2008). The philosophy of chemistry in Fritz Allhoff (Ed.), Philosophies of the Sciences , (pp. 163–183), Albany, NY, USA: Blackwell-Wiley.
Schwab, J. J. (1962). The teaching of science as enquiry. In J. J. Schwab & P. F. Brandwein (Eds.), The teaching of science. Cambridge: Harvard University Press.
Seok P. & Jin S. (2011) What Teachers of Science Need to Know about Models: An overview, International Journal of Science Education , 33(8), 1109–1130.
Segrè, E. (2007). From X-rays to Quarks: Modern Physicists and Their Discoveries , New York, USA: Dover Publications.
Shahbazian, S. & Zahedi, M. (2006). The Role of Observables and Non-Observables in Chemistry: A Critique of Chemical Language, Foundations of Chemistry , 8, 37–52.
Shiland, T. W. (1995). What’s the use of all this theory? The role of quantum mechanics in high school chemistry textbooks, Journal of Chemical Education , 72(3), 215–219.
Shiland, T. W. (1997). Quantum mechanics and conceptual change in high school chemistry textbooks, Journal of Research in Science Teaching , 34(5), 535–545.
Shrigley, R.L. & Koballa, T. R. (1989). Anecdotes: What Research Suggests about Their Use in the Science Classroom, School Science and Mathematics , 89, 293–298.
Silberstein, M. (2002). Reduction, Emergence and explanation, en Machamer P., and Silberstein, M., Philosophy of Science , Oxford: Blackwell.
Slater, J. C. (1951). A Simplification of the Hartree-Fock Method, Physical Review , 81, 385–390.
Snooks, R. J. (2006). Another Scientific Practice separating chemistry from Physics: Thought Experiments, Foundations of Chemistry , 8, 255–270.
Snow, C.P. (1981). The Physicists. A generation that changed the world , Macmillan, London
Spence, J. C. H., O’Keeffe, M. and Zuo, J. M. (2001). Have orbitals really been observed? Letter in Journal of Chemical Education , 78(7), 877.
Stewart, I. (2007). Why Beauty is Truth. The history of symmetry . Basic Books.
Stinner, A. (2008). Teaching Modern Physics using Selected Nobel Lectures APS Physics Forum on Education, fall.
Stinner, A. & Williams, H. (1998). History and Philosophy of Science in the Science Curriculum, a chapter in The International Handbook of Science Education . Dordrecht: Kluwer Academic Publishers.
Strong, I. E. (1962). Chemical Systems. Chemical Bond Approach Project , New York, USA: Chemical Education Publishing Company.
Styer, D. F. (2000). The Strange World of Quantum Mechanics . Cambridge: Cambridge University Press.
Sutcliffe, B. T. (1996). The Development of the Idea of a Chemical Bond, International Journal of Quantum Chemistry , 58, 645–55.
Taber, K. S. (1994). Misunderstanding the ionic bond, Education in Chemistry , 31(4), 100–103.
Taber, K. S. (1997). Student understanding of ionic bonding: molecular versus electrostatic framework? School Science Review , 78(285), 85–95.
Taber, K. S. (2002a). Conceptualizing Quanta: Illuminating the Ground State of Student Understanding of Atomic Orbitals, Chemistry Education: Research and Practice , 3(2), 145–158.
Taber, K. S. (2002b). Compounding Quanta: Probing the Frontiers of Student Understanding Of Molecular Orbitals, Chemistry Education: Research and Practice , 3(2), 159–173.
Taber, K.S. (2003). The Atom in the Chemistry Curriculum: Fundamental Concept, Teaching Model or Epistemological Obstacle, Foundations of Chemistry , 5, 43–84.
Taber, K. S. (2005). Learning Quanta: Barriers to Stimulating Transitions in Student Understanding of Orbital Ideas, Science Education , 89, 94–116.
Talanquer, V. (2011, online), School Chemistry: The Need for Transgression, Science & Education , published online 17th September.
Tapio, I. (2007). Models and Modelling in Physics Education: A critical Re-analysis of Philosophical Underpinnings and Suggestions for Revisions, Science & Education , 16, 751–773.
Teichmann, J. (2008). Anecdotes Can Tell Stories—How? And What is Good and What is Bad about Such Stories? Proceedings of the Second International Conference on Story in Science Teaching , Munich, Germany.
Thomson, J. J. (1904). Electricity and matter , Westminster, UK: Archibald Constable & Co. Ltd.
Toulmin, S. (1961). Foresight and Understanding: An Enquiry Into the Aims of Science , Bloomington: Indiana University Press.
Toulmin, S. (1972). Human Understanding , Princeton: Princeton University Press.
Tsaparlis, G., & Papaphotis, G. (2002). Quantum-Chemical Concepts: Are They Suitable for Secondary Students? Chemistry Education: Research and Practice , 3(2), 129–144.
Tsaparlis, G. (1997a). Atomic orbitals, molecular orbitals and related concepts: Conceptual difficulties among chemistry students. Research in Science Education , 27, 271–287.
Tsaparlis, G. (1997b). Atomic and Molecular Structure in Chemical Education, Journal of Chemical Education , 74(8), 922–5.
Tsaparlis, G. (2001). Towards a meaningful introduction to the Schrödinger equation through historical and heuristic approaches, Chemistry Education: Research and Practice in Europe , 2, 203–213.
Tsaparlis, G., & Papaphotis, G. (2009). High-school Students’ Conceptual Difficulties and Attempts at Conceptual Change: The case of basic quantum chemical concepts, International Journal of Science Education , 31(7), 895–930.
Toulmin S. & Goodfield J. (1962). The Architecture of Matter , The University of Chicago Press, Chicago
Valanides, N. (2000). Primary student teachers’ understanding of the particulate nature of matter and its transformations during dissolving, Chemistry Education: Research and Practice in Europe , 1, 249–262.
Van Aalsvoort, J. (2004) ‘Logical positivism as a tool to analyse the problem of chemistry’s lack of relevance in secondary school chemical education’, International Journal of Science Education , 26, 1151–1168.
Van Brakel, J. (2000). Philosophy of Chemistry , Leuven University Press, Louvain.
Van Berkel, B. (2005). The Structure of Current School Chemistry. A Quest for Conditions for Escape , Centrum voor Didactiek van Wiskunde en Natuurwetenschappen, University of Utrech CD-β Press, Utrech.
van Berkel, B., de Vos, W., Veronk, A. H., & Pilot, A. (2000). Normal science education and its dangers: The case of school chemistry. Science & Education , 9, 123–159.
Velmulapalli, G. K. & Byerly H. (1999) Remnants of Reductionism, Foundations of Chemistry 1, 17–41.
Viana, H. E. B. & Porto, P. A. (2010). The development of Dalton’s Atomic Theory as a Case Study in the History of Science: Reflections for Educators in Chemistry, Science & Education , 19(1), 75–90.
Wandersee, J. H., & Griffard, P. B. (2002). The history of chemistry: Potential and actual contributions to chemical education in Gilbert J. et al. (eds), Chemical Education: Towards Research-based Practice , (Chapter 2, pp. 29–46), Dordrecht, The Netherlands: Kluwer.
Weyer, J. (1974) Chemiegeschichtsschreibung von Wiegleb (1790) bis Partington (1970); Gerstenberg: Hildescheim
Wightman, T., Johnston, K., & Scott, P. (1987). Children’s learning in science project in the classroom. Approaches to teaching the particulate theory of matter , Centre for Studies in Science and Mathematics Education: University of Leeds.
Wisniak, J. (2013). Gustav Charles Bonaventure Chancel, Educación Química , 24(1), 23–30.
Wittmann, M. C., Steinberg, R. N., & Redish, E. F. (2002). Investigating student understanding of quantum physics: Spontaneous models of conductivity, American Journal of Physics , 70, 218–226.
Woolley, R.G. (1978). Must a molecule have a shape? Journal of the American Chemical Society , 100, 1073–1078.
Yager, R. E. (2004). Science is Not Written, But It Can Be Written About, in W. Saul (Ed.), Crossing Borders in Literacy and Science Instruction , (pp. 95–107) Washington: NSTA.
Zuo, J.; Kim, M.; O’Keeffe, M.; Spence, J. (1999). Direct observation of d holes and Cu-Cu bonding in. Cu 2 O, Nature , 401, 49–56.
Download references
Author information
Authors and affiliations.
Seminario de Investigación Educativa en Química, Facultad de Química, Universidad Nacional Autónoma de México, Avenida Universidad 3000, Delegación Coyoacán, México, DF, 04510, México
José Antonio Chamizo & Andoni Garritz
You can also search for this author in PubMed Google Scholar
Corresponding author
Correspondence to José Antonio Chamizo .
Editor information
Editors and affiliations.
School of Education, University of New South Wales, Sydney, New South Wales, Australia
Michael R. Matthews
Rights and permissions
Reprints and permissions
Copyright information
© 2014 Springer Science+Business Media Dordrecht
About this chapter
Chamizo, J.A., Garritz, A. (2014). Historical Teaching of Atomic and Molecular Structure. In: Matthews, M. (eds) International Handbook of Research in History, Philosophy and Science Teaching. Springer, Dordrecht. https://doi.org/10.1007/978-94-007-7654-8_12
Download citation
DOI : https://doi.org/10.1007/978-94-007-7654-8_12
Published : 30 December 2013
Publisher Name : Springer, Dordrecht
Print ISBN : 978-94-007-7653-1
Online ISBN : 978-94-007-7654-8
eBook Packages : Humanities, Social Sciences and Law Education (R0)
Share this chapter
Anyone you share the following link with will be able to read this content:
Sorry, a shareable link is not currently available for this article.
Provided by the Springer Nature SharedIt content-sharing initiative
- Publish with us
Policies and ethics
- Find a journal
- Track your research

- school Campus Bookshelves
- menu_book Bookshelves
- perm_media Learning Objects
- login Login
- how_to_reg Request Instructor Account
- hub Instructor Commons
Margin Size
- Download Page (PDF)
- Download Full Book (PDF)
- Periodic Table
- Physics Constants
- Scientific Calculator
- Reference & Cite
- Tools expand_more
- Readability
selected template will load here
This action is not available.
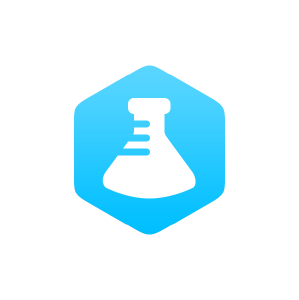
2.2: The Discovery of Atomic Structure
- Last updated
- Save as PDF
- Page ID 21698
\( \newcommand{\vecs}[1]{\overset { \scriptstyle \rightharpoonup} {\mathbf{#1}} } \)
\( \newcommand{\vecd}[1]{\overset{-\!-\!\rightharpoonup}{\vphantom{a}\smash {#1}}} \)
\( \newcommand{\id}{\mathrm{id}}\) \( \newcommand{\Span}{\mathrm{span}}\)
( \newcommand{\kernel}{\mathrm{null}\,}\) \( \newcommand{\range}{\mathrm{range}\,}\)
\( \newcommand{\RealPart}{\mathrm{Re}}\) \( \newcommand{\ImaginaryPart}{\mathrm{Im}}\)
\( \newcommand{\Argument}{\mathrm{Arg}}\) \( \newcommand{\norm}[1]{\| #1 \|}\)
\( \newcommand{\inner}[2]{\langle #1, #2 \rangle}\)
\( \newcommand{\Span}{\mathrm{span}}\)
\( \newcommand{\id}{\mathrm{id}}\)
\( \newcommand{\kernel}{\mathrm{null}\,}\)
\( \newcommand{\range}{\mathrm{range}\,}\)
\( \newcommand{\RealPart}{\mathrm{Re}}\)
\( \newcommand{\ImaginaryPart}{\mathrm{Im}}\)
\( \newcommand{\Argument}{\mathrm{Arg}}\)
\( \newcommand{\norm}[1]{\| #1 \|}\)
\( \newcommand{\Span}{\mathrm{span}}\) \( \newcommand{\AA}{\unicode[.8,0]{x212B}}\)
\( \newcommand{\vectorA}[1]{\vec{#1}} % arrow\)
\( \newcommand{\vectorAt}[1]{\vec{\text{#1}}} % arrow\)
\( \newcommand{\vectorB}[1]{\overset { \scriptstyle \rightharpoonup} {\mathbf{#1}} } \)
\( \newcommand{\vectorC}[1]{\textbf{#1}} \)
\( \newcommand{\vectorD}[1]{\overrightarrow{#1}} \)
\( \newcommand{\vectorDt}[1]{\overrightarrow{\text{#1}}} \)
\( \newcommand{\vectE}[1]{\overset{-\!-\!\rightharpoonup}{\vphantom{a}\smash{\mathbf {#1}}}} \)
Learning Objectives
- To become familiar with the components and structure of the atom.
Long before the end of the 19th century, it was well known that applying a high voltage to a gas contained at low pressure in a sealed tube (called a gas discharge tube) caused electricity to flow through the gas, which then emitted light (Figure \(\PageIndex{1}\)). Researchers trying to understand this phenomenon found that an unusual form of energy was also emitted from the cathode, or negatively charged electrode; this form of energy was called a cathode ray.

In 1897, the British physicist J. J. Thomson (1856–1940) proved that atoms were not the most basic form of matter. He demonstrated that cathode rays could be deflected, or bent, by magnetic or electric fields, which indicated that cathode rays consist of charged particles (Figure \(\PageIndex{2}\)). More important, by measuring the extent of the deflection of the cathode rays in magnetic or electric fields of various strengths, Thomson was able to calculate the mass-to-charge ratio of the particles. These particles were emitted by the negatively charged cathode and repelled by the negative terminal of an electric field. Because like charges repel each other and opposite charges attract, Thomson concluded that the particles had a net negative charge; these particles are now called electrons. Most relevant to the field of chemistry, Thomson found that the mass-to-charge ratio of cathode rays is independent of the nature of the metal electrodes or the gas, which suggested that electrons were fundamental components of all atoms.
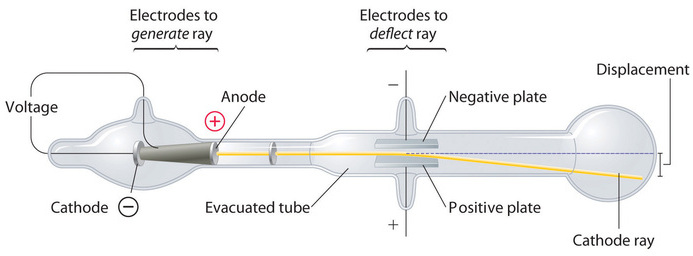
Subsequently, the American scientist Robert Millikan (1868–1953) carried out a series of experiments using electrically charged oil droplets, which allowed him to calculate the charge on a single electron. With this information and Thomson’s mass-to-charge ratio, Millikan determined the mass of an electron:
\[\dfrac {mass}{charge} \times {charge} ={mass} \nonumber \]
It was at this point that two separate lines of investigation began to converge, both aimed at determining how and why matter emits energy. The video below shows how JJ Thompson used such a tube to measure the ratio of charge over mass of an electron
Measuring e/m For an Electron. Video from Davidson College demonstrating Thompson's e/m experiment.
Radioactivity
The second line of investigation began in 1896, when the French physicist Henri Becquerel (1852–1908) discovered that certain minerals, such as uranium salts, emitted a new form of energy. Becquerel’s work was greatly extended by Marie Curie (1867–1934) and her husband, Pierre (1854–1906); all three shared the Nobel Prize in Physics in 1903. Marie Curie coined the term radioactivity (from the Latin radius , meaning “ray”) to describe the emission of energy rays by matter. She found that one particular uranium ore, pitchblende, was substantially more radioactive than most, which suggested that it contained one or more highly radioactive impurities. Starting with several tons of pitchblende, the Curies isolated two new radioactive elements after months of work: polonium, which was named for Marie’s native Poland, and radium, which was named for its intense radioactivity. Pierre Curie carried a vial of radium in his coat pocket to demonstrate its greenish glow, a habit that caused him to become ill from radiation poisoning well before he was run over by a horse-drawn wagon and killed instantly in 1906. Marie Curie, in turn, died of what was almost certainly radiation poisoning.
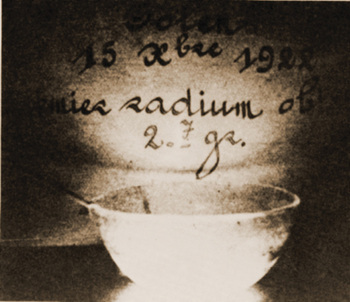
Building on the Curies’ work, the British physicist Ernest Rutherford (1871–1937) performed decisive experiments that led to the modern view of the structure of the atom. While working in Thomson’s laboratory shortly after Thomson discovered the electron, Rutherford showed that compounds of uranium and other elements emitted at least two distinct types of radiation. One was readily absorbed by matter and seemed to consist of particles that had a positive charge and were massive compared to electrons. Because it was the first kind of radiation to be discovered, Rutherford called these substances α particles. Rutherford also showed that the particles in the second type of radiation, β particles, had the same charge and mass-to-charge ratio as Thomson’s electrons; they are now known to be high-speed electrons. A third type of radiation, γ rays, was discovered somewhat later and found to be similar to the lower-energy form of radiation called x-rays, now used to produce images of bones and teeth.
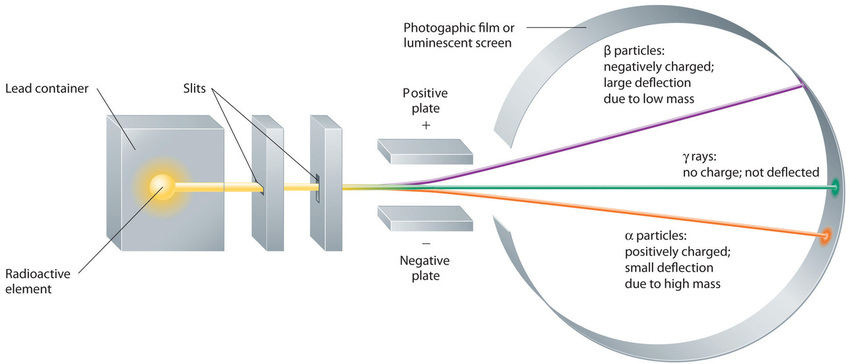
These three kinds of radiation—α particles, β particles, and γ rays—are readily distinguished by the way they are deflected by an electric field and by the degree to which they penetrate matter. As Figure \(\PageIndex{3}\) illustrates, α particles and β particles are deflected in opposite directions; α particles are deflected to a much lesser extent because of their higher mass-to-charge ratio. In contrast, γ rays have no charge, so they are not deflected by electric or magnetic fields. Figure \(\PageIndex{5}\) shows that α particles have the least penetrating power and are stopped by a sheet of paper, whereas β particles can pass through thin sheets of metal but are absorbed by lead foil or even thick glass. In contrast, γ-rays can readily penetrate matter; thick blocks of lead or concrete are needed to stop them.
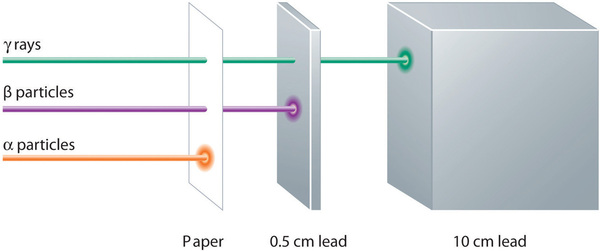
The Atomic Model
Once scientists concluded that all matter contains negatively charged electrons, it became clear that atoms, which are electrically neutral, must also contain positive charges to balance the negative ones. Thomson proposed that the electrons were embedded in a uniform sphere that contained both the positive charge and most of the mass of the atom, much like raisins in plum pudding or chocolate chips in a cookie (Figure \(\PageIndex{6}\)).
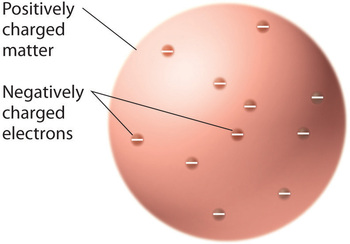
In a single famous experiment, however, Rutherford showed unambiguously that Thomson’s model of the atom was incorrect. Rutherford aimed a stream of α particles at a very thin gold foil target (Figure \(\PageIndex{7a}\)) and examined how the α particles were scattered by the foil. Gold was chosen because it could be easily hammered into extremely thin sheets, minimizing the number of atoms in the target. If Thomson’s model of the atom were correct, the positively-charged α particles should crash through the uniformly distributed mass of the gold target like cannonballs through the side of a wooden house. They might be moving a little slower when they emerged, but they should pass essentially straight through the target (Figure \(\PageIndex{7b}\)). To Rutherford’s amazement, a small fraction of the α particles were deflected at large angles, and some were reflected directly back at the source (Figure \(\PageIndex{7c}\)). According to Rutherford, “It was almost as incredible as if you fired a 15-inch shell at a piece of tissue paper and it came back and hit you.”

The Nuclear Atom: The Nuclear Atom, YouTube(opens in new window) [youtu.be]
Rutherford’s results were not consistent with a model in which the mass and positive charge are distributed uniformly throughout the volume of an atom. Instead, they strongly suggested that both the mass and positive charge are concentrated in a tiny fraction of the volume of an atom, which Rutherford called the nucleus. It made sense that a small fraction of the α particles collided with the dense, positively charged nuclei in either a glancing fashion, resulting in large deflections, or almost head-on, causing them to be reflected straight back at the source.
Although Rutherford could not explain why repulsions between the positive charges in nuclei that contained more than one positive charge did not cause the nucleus to disintegrate, he reasoned that repulsions between negatively charged electrons would cause the electrons to be uniformly distributed throughout the atom’s volume.Today it is known that strong nuclear forces, which are much stronger than electrostatic interactions, hold the protons and the neutrons together in the nucleus. For this and other insights, Rutherford was awarded the Nobel Prize in Chemistry in 1908. Unfortunately, Rutherford would have preferred to receive the Nobel Prize in Physics because he considered physics superior to chemistry. In his opinion, “All science is either physics or stamp collecting.”
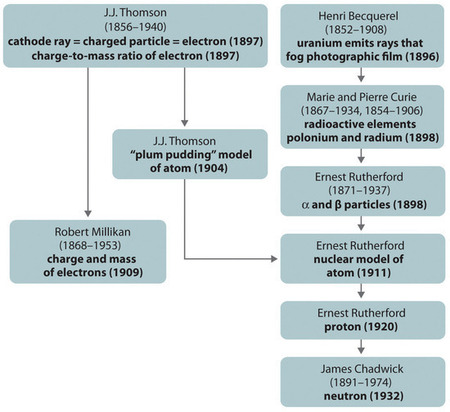
The historical development of the different models of the atom’s structure is summarized in Figure \(\PageIndex{8}\). Rutherford established that the nucleus of the hydrogen atom was a positively charged particle, for which he coined the name proton in 1920. He also suggested that the nuclei of elements other than hydrogen must contain electrically neutral particles with approximately the same mass as the proton. The neutron, however, was not discovered until 1932, when James Chadwick (1891–1974, a student of Rutherford; Nobel Prize in Physics, 1935) discovered it. As a result of Rutherford’s work, it became clear that an α particle contains two protons and neutrons, and is therefore the nucleus of a helium atom.
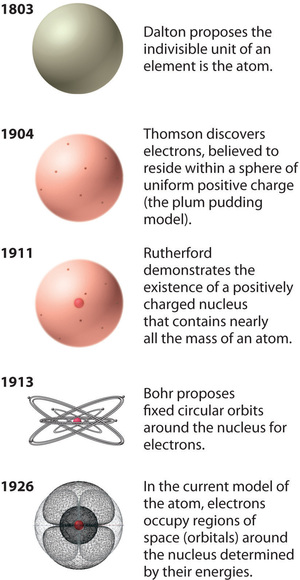
Rutherford’s model of the atom is essentially the same as the modern model, except that it is now known that electrons are not uniformly distributed throughout an atom’s volume. Instead, they are distributed according to a set of principles described by Quantum Mechanics. Figure \(\PageIndex{9}\) shows how the model of the atom has evolved over time from the indivisible unit of Dalton to the modern view taught today.
Atoms are the ultimate building blocks of all matter. The modern atomic theory establishes the concepts of atoms and how they compose matter. Atoms, the smallest particles of an element that exhibit the properties of that element, consist of negatively charged electrons around a central nucleus composed of more massive positively charged protons and electrically neutral neutrons. Radioactivity is the emission of energetic particles and rays (radiation) by some substances. Three important kinds of radiation are α particles (helium nuclei), β particles (electrons traveling at high speed), and γ rays (similar to x-rays but higher in energy).
Academia.edu no longer supports Internet Explorer.
To browse Academia.edu and the wider internet faster and more securely, please take a few seconds to upgrade your browser .
- We're Hiring!
- Help Center
Atomic Structure
- Most Cited Papers
- Most Downloaded Papers
- Newest Papers
- Save to Library
- Last »
- X-ray production Follow Following
- Nucleic Acid Follow Following
- Theoretical and Space Physics Follow Following
- Space Physics Follow Following
- Organic Compound Follow Following
- Hyperfine Structure Follow Following
- Electromagnetic Field Follow Following
- Theoretical Physics Follow Following
- Amino Acid Profile Follow Following
- Mathematical Sciences Follow Following
Enter the email address you signed up with and we'll email you a reset link.
- Academia.edu Publishing
- We're Hiring!
- Help Center
- Find new research papers in:
- Health Sciences
- Earth Sciences
- Cognitive Science
- Mathematics
- Computer Science
- Academia ©2024
Thank you for visiting nature.com. You are using a browser version with limited support for CSS. To obtain the best experience, we recommend you use a more up to date browser (or turn off compatibility mode in Internet Explorer). In the meantime, to ensure continued support, we are displaying the site without styles and JavaScript.
- View all journals
- My Account Login
- Explore content
- About the journal
- Publish with us
- Sign up for alerts
- Open access
- Published: 15 July 2021
Highly accurate protein structure prediction with AlphaFold
- John Jumper ORCID: orcid.org/0000-0001-6169-6580 1 na1 ,
- Richard Evans 1 na1 ,
- Alexander Pritzel 1 na1 ,
- Tim Green ORCID: orcid.org/0000-0002-3227-1505 1 na1 ,
- Michael Figurnov 1 na1 ,
- Olaf Ronneberger 1 na1 ,
- Kathryn Tunyasuvunakool 1 na1 ,
- Russ Bates 1 na1 ,
- Augustin Žídek 1 na1 ,
- Anna Potapenko 1 na1 ,
- Alex Bridgland 1 na1 ,
- Clemens Meyer 1 na1 ,
- Simon A. A. Kohl ORCID: orcid.org/0000-0003-4271-4418 1 na1 ,
- Andrew J. Ballard 1 na1 ,
- Andrew Cowie 1 na1 ,
- Bernardino Romera-Paredes 1 na1 ,
- Stanislav Nikolov 1 na1 ,
- Rishub Jain 1 na1 ,
- Jonas Adler ORCID: orcid.org/0000-0001-9928-3407 1 ,
- Trevor Back 1 ,
- Stig Petersen 1 ,
- David Reiman 1 ,
- Ellen Clancy 1 ,
- Michal Zielinski 1 ,
- Martin Steinegger ORCID: orcid.org/0000-0001-8781-9753 2 , 3 ,
- Michalina Pacholska ORCID: orcid.org/0000-0002-2160-6226 1 ,
- Tamas Berghammer 1 ,
- Sebastian Bodenstein 1 ,
- David Silver ORCID: orcid.org/0000-0002-5197-2892 1 ,
- Oriol Vinyals 1 ,
- Andrew W. Senior ORCID: orcid.org/0000-0002-2401-5691 1 ,
- Koray Kavukcuoglu 1 ,
- Pushmeet Kohli 1 &
- Demis Hassabis ORCID: orcid.org/0000-0003-2812-9917 1 na1
Nature volume 596 , pages 583–589 ( 2021 ) Cite this article
1.58m Accesses
8815 Citations
3561 Altmetric
Metrics details
- Computational biophysics
- Machine learning
- Protein structure predictions
- Structural biology
Proteins are essential to life, and understanding their structure can facilitate a mechanistic understanding of their function. Through an enormous experimental effort 1 , 2 , 3 , 4 , the structures of around 100,000 unique proteins have been determined 5 , but this represents a small fraction of the billions of known protein sequences 6 , 7 . Structural coverage is bottlenecked by the months to years of painstaking effort required to determine a single protein structure. Accurate computational approaches are needed to address this gap and to enable large-scale structural bioinformatics. Predicting the three-dimensional structure that a protein will adopt based solely on its amino acid sequence—the structure prediction component of the ‘protein folding problem’ 8 —has been an important open research problem for more than 50 years 9 . Despite recent progress 10 , 11 , 12 , 13 , 14 , existing methods fall far short of atomic accuracy, especially when no homologous structure is available. Here we provide the first computational method that can regularly predict protein structures with atomic accuracy even in cases in which no similar structure is known. We validated an entirely redesigned version of our neural network-based model, AlphaFold, in the challenging 14th Critical Assessment of protein Structure Prediction (CASP14) 15 , demonstrating accuracy competitive with experimental structures in a majority of cases and greatly outperforming other methods. Underpinning the latest version of AlphaFold is a novel machine learning approach that incorporates physical and biological knowledge about protein structure, leveraging multi-sequence alignments, into the design of the deep learning algorithm.
Similar content being viewed by others
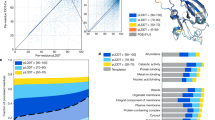
Highly accurate protein structure prediction for the human proteome
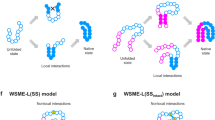
Accurate prediction of protein folding mechanisms by simple structure-based statistical mechanical models
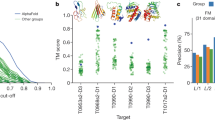
Improved protein structure prediction using potentials from deep learning
The development of computational methods to predict three-dimensional (3D) protein structures from the protein sequence has proceeded along two complementary paths that focus on either the physical interactions or the evolutionary history. The physical interaction programme heavily integrates our understanding of molecular driving forces into either thermodynamic or kinetic simulation of protein physics 16 or statistical approximations thereof 17 . Although theoretically very appealing, this approach has proved highly challenging for even moderate-sized proteins due to the computational intractability of molecular simulation, the context dependence of protein stability and the difficulty of producing sufficiently accurate models of protein physics. The evolutionary programme has provided an alternative in recent years, in which the constraints on protein structure are derived from bioinformatics analysis of the evolutionary history of proteins, homology to solved structures 18 , 19 and pairwise evolutionary correlations 20 , 21 , 22 , 23 , 24 . This bioinformatics approach has benefited greatly from the steady growth of experimental protein structures deposited in the Protein Data Bank (PDB) 5 , the explosion of genomic sequencing and the rapid development of deep learning techniques to interpret these correlations. Despite these advances, contemporary physical and evolutionary-history-based approaches produce predictions that are far short of experimental accuracy in the majority of cases in which a close homologue has not been solved experimentally and this has limited their utility for many biological applications.
In this study, we develop the first, to our knowledge, computational approach capable of predicting protein structures to near experimental accuracy in a majority of cases. The neural network AlphaFold that we developed was entered into the CASP14 assessment (May–July 2020; entered under the team name ‘AlphaFold2’ and a completely different model from our CASP13 AlphaFold system 10 ). The CASP assessment is carried out biennially using recently solved structures that have not been deposited in the PDB or publicly disclosed so that it is a blind test for the participating methods, and has long served as the gold-standard assessment for the accuracy of structure prediction 25 , 26 .
In CASP14, AlphaFold structures were vastly more accurate than competing methods. AlphaFold structures had a median backbone accuracy of 0.96 Å r.m.s.d. 95 (Cα root-mean-square deviation at 95% residue coverage) (95% confidence interval = 0.85–1.16 Å) whereas the next best performing method had a median backbone accuracy of 2.8 Å r.m.s.d. 95 (95% confidence interval = 2.7–4.0 Å) (measured on CASP domains; see Fig. 1a for backbone accuracy and Supplementary Fig. 14 for all-atom accuracy). As a comparison point for this accuracy, the width of a carbon atom is approximately 1.4 Å. In addition to very accurate domain structures (Fig. 1b ), AlphaFold is able to produce highly accurate side chains (Fig. 1c ) when the backbone is highly accurate and considerably improves over template-based methods even when strong templates are available. The all-atom accuracy of AlphaFold was 1.5 Å r.m.s.d. 95 (95% confidence interval = 1.2–1.6 Å) compared with the 3.5 Å r.m.s.d. 95 (95% confidence interval = 3.1–4.2 Å) of the best alternative method. Our methods are scalable to very long proteins with accurate domains and domain-packing (see Fig. 1d for the prediction of a 2,180-residue protein with no structural homologues). Finally, the model is able to provide precise, per-residue estimates of its reliability that should enable the confident use of these predictions.
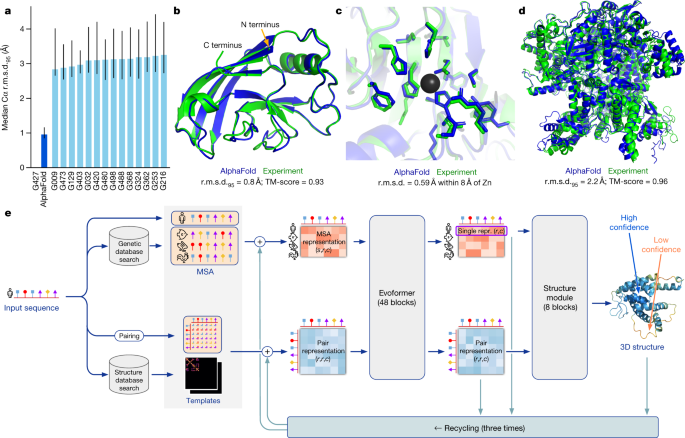
a , The performance of AlphaFold on the CASP14 dataset ( n = 87 protein domains) relative to the top-15 entries (out of 146 entries), group numbers correspond to the numbers assigned to entrants by CASP. Data are median and the 95% confidence interval of the median, estimated from 10,000 bootstrap samples. b , Our prediction of CASP14 target T1049 (PDB 6Y4F, blue) compared with the true (experimental) structure (green). Four residues in the C terminus of the crystal structure are B -factor outliers and are not depicted. c , CASP14 target T1056 (PDB 6YJ1). An example of a well-predicted zinc-binding site (AlphaFold has accurate side chains even though it does not explicitly predict the zinc ion). d , CASP target T1044 (PDB 6VR4)—a 2,180-residue single chain—was predicted with correct domain packing (the prediction was made after CASP using AlphaFold without intervention). e , Model architecture. Arrows show the information flow among the various components described in this paper. Array shapes are shown in parentheses with s , number of sequences ( N seq in the main text); r , number of residues ( N res in the main text); c , number of channels.
We demonstrate in Fig. 2a that the high accuracy that AlphaFold demonstrated in CASP14 extends to a large sample of recently released PDB structures; in this dataset, all structures were deposited in the PDB after our training data cut-off and are analysed as full chains (see Methods , Supplementary Fig. 15 and Supplementary Table 6 for more details). Furthermore, we observe high side-chain accuracy when the backbone prediction is accurate (Fig. 2b ) and we show that our confidence measure, the predicted local-distance difference test (pLDDT), reliably predicts the Cα local-distance difference test (lDDT-Cα) accuracy of the corresponding prediction (Fig. 2c ). We also find that the global superposition metric template modelling score (TM-score) 27 can be accurately estimated (Fig. 2d ). Overall, these analyses validate that the high accuracy and reliability of AlphaFold on CASP14 proteins also transfers to an uncurated collection of recent PDB submissions, as would be expected (see Supplementary Methods 1.15 and Supplementary Fig. 11 for confirmation that this high accuracy extends to new folds).
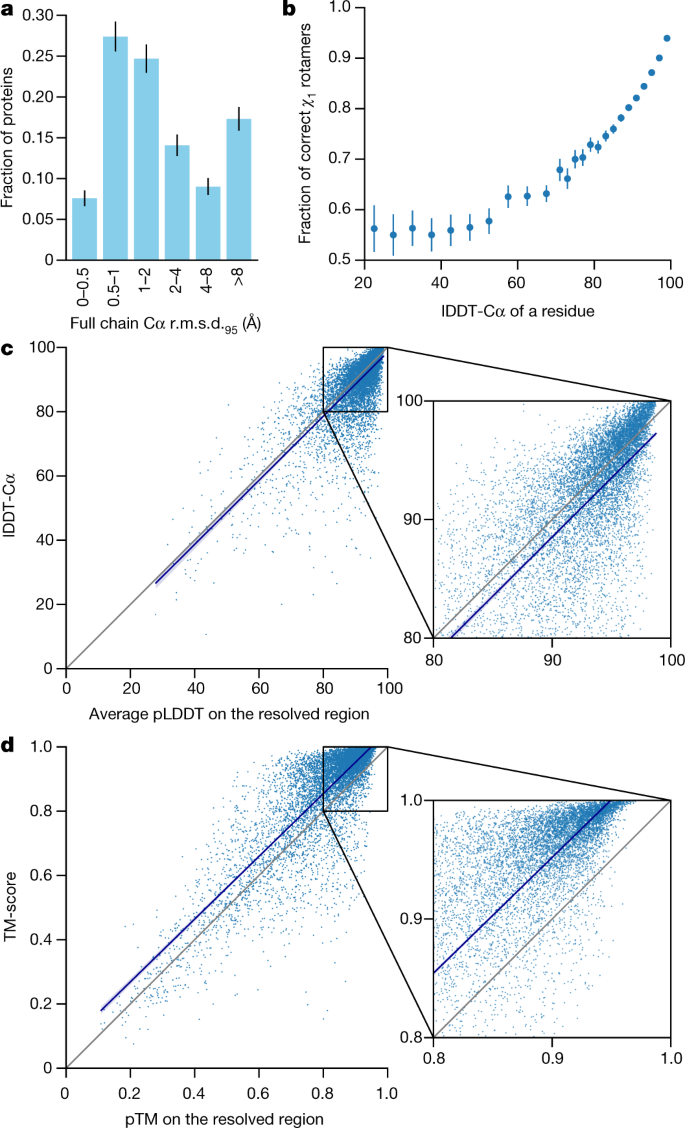
The analysed structures are newer than any structure in the training set. Further filtering is applied to reduce redundancy (see Methods ). a , Histogram of backbone r.m.s.d. for full chains (Cα r.m.s.d. at 95% coverage). Error bars are 95% confidence intervals (Poisson). This dataset excludes proteins with a template (identified by hmmsearch) from the training set with more than 40% sequence identity covering more than 1% of the chain ( n = 3,144 protein chains). The overall median is 1.46 Å (95% confidence interval = 1.40–1.56 Å). Note that this measure will be highly sensitive to domain packing and domain accuracy; a high r.m.s.d. is expected for some chains with uncertain packing or packing errors. b , Correlation between backbone accuracy and side-chain accuracy. Filtered to structures with any observed side chains and resolution better than 2.5 Å ( n = 5,317 protein chains); side chains were further filtered to B -factor <30 Å 2 . A rotamer is classified as correct if the predicted torsion angle is within 40°. Each point aggregates a range of lDDT-Cα, with a bin size of 2 units above 70 lDDT-Cα and 5 units otherwise. Points correspond to the mean accuracy; error bars are 95% confidence intervals (Student t -test) of the mean on a per-residue basis. c , Confidence score compared to the true accuracy on chains. Least-squares linear fit lDDT-Cα = 0.997 × pLDDT − 1.17 (Pearson’s r = 0.76). n = 10,795 protein chains. The shaded region of the linear fit represents a 95% confidence interval estimated from 10,000 bootstrap samples. In the companion paper 39 , additional quantification of the reliability of pLDDT as a confidence measure is provided. d , Correlation between pTM and full chain TM-score. Least-squares linear fit TM-score = 0.98 × pTM + 0.07 (Pearson’s r = 0.85). n = 10,795 protein chains. The shaded region of the linear fit represents a 95% confidence interval estimated from 10,000 bootstrap samples.
The AlphaFold network
AlphaFold greatly improves the accuracy of structure prediction by incorporating novel neural network architectures and training procedures based on the evolutionary, physical and geometric constraints of protein structures. In particular, we demonstrate a new architecture to jointly embed multiple sequence alignments (MSAs) and pairwise features, a new output representation and associated loss that enable accurate end-to-end structure prediction, a new equivariant attention architecture, use of intermediate losses to achieve iterative refinement of predictions, masked MSA loss to jointly train with the structure, learning from unlabelled protein sequences using self-distillation and self-estimates of accuracy.
The AlphaFold network directly predicts the 3D coordinates of all heavy atoms for a given protein using the primary amino acid sequence and aligned sequences of homologues as inputs (Fig. 1e ; see Methods for details of inputs including databases, MSA construction and use of templates). A description of the most important ideas and components is provided below. The full network architecture and training procedure are provided in the Supplementary Methods .
The network comprises two main stages. First, the trunk of the network processes the inputs through repeated layers of a novel neural network block that we term Evoformer to produce an N seq × N res array ( N seq , number of sequences; N res , number of residues) that represents a processed MSA and an N res × N res array that represents residue pairs. The MSA representation is initialized with the raw MSA (although see Supplementary Methods 1.2.7 for details of handling very deep MSAs). The Evoformer blocks contain a number of attention-based and non-attention-based components. We show evidence in ‘Interpreting the neural network’ that a concrete structural hypothesis arises early within the Evoformer blocks and is continuously refined. The key innovations in the Evoformer block are new mechanisms to exchange information within the MSA and pair representations that enable direct reasoning about the spatial and evolutionary relationships.
The trunk of the network is followed by the structure module that introduces an explicit 3D structure in the form of a rotation and translation for each residue of the protein (global rigid body frames). These representations are initialized in a trivial state with all rotations set to the identity and all positions set to the origin, but rapidly develop and refine a highly accurate protein structure with precise atomic details. Key innovations in this section of the network include breaking the chain structure to allow simultaneous local refinement of all parts of the structure, a novel equivariant transformer to allow the network to implicitly reason about the unrepresented side-chain atoms and a loss term that places substantial weight on the orientational correctness of the residues. Both within the structure module and throughout the whole network, we reinforce the notion of iterative refinement by repeatedly applying the final loss to outputs and then feeding the outputs recursively into the same modules. The iterative refinement using the whole network (which we term ‘recycling’ and is related to approaches in computer vision 28 , 29 ) contributes markedly to accuracy with minor extra training time (see Supplementary Methods 1.8 for details).
The key principle of the building block of the network—named Evoformer (Figs. 1 e, 3a )—is to view the prediction of protein structures as a graph inference problem in 3D space in which the edges of the graph are defined by residues in proximity. The elements of the pair representation encode information about the relation between the residues (Fig. 3b ). The columns of the MSA representation encode the individual residues of the input sequence while the rows represent the sequences in which those residues appear. Within this framework, we define a number of update operations that are applied in each block in which the different update operations are applied in series.
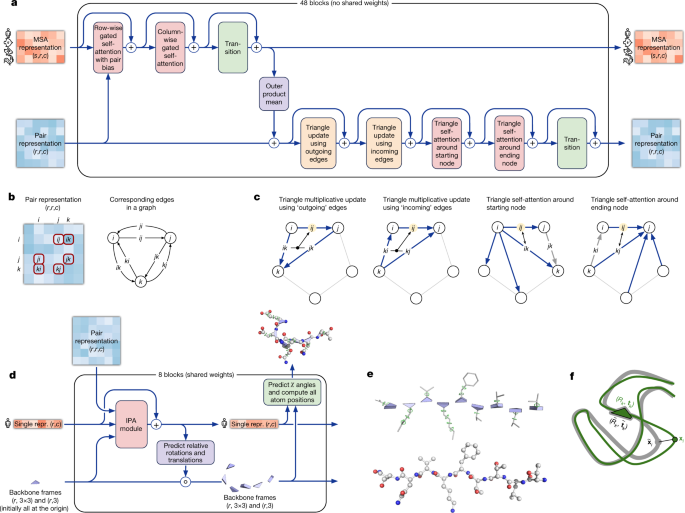
a , Evoformer block. Arrows show the information flow. The shape of the arrays is shown in parentheses. b , The pair representation interpreted as directed edges in a graph. c , Triangle multiplicative update and triangle self-attention. The circles represent residues. Entries in the pair representation are illustrated as directed edges and in each diagram, the edge being updated is ij . d , Structure module including Invariant point attention (IPA) module. The single representation is a copy of the first row of the MSA representation. e , Residue gas: a representation of each residue as one free-floating rigid body for the backbone (blue triangles) and χ angles for the side chains (green circles). The corresponding atomic structure is shown below. f , Frame aligned point error (FAPE). Green, predicted structure; grey, true structure; ( R k , t k ), frames; x i , atom positions.
The MSA representation updates the pair representation through an element-wise outer product that is summed over the MSA sequence dimension. In contrast to previous work 30 , this operation is applied within every block rather than once in the network, which enables the continuous communication from the evolving MSA representation to the pair representation.
Within the pair representation, there are two different update patterns. Both are inspired by the necessity of consistency of the pair representation—for a pairwise description of amino acids to be representable as a single 3D structure, many constraints must be satisfied including the triangle inequality on distances. On the basis of this intuition, we arrange the update operations on the pair representation in terms of triangles of edges involving three different nodes (Fig. 3c ). In particular, we add an extra logit bias to axial attention 31 to include the ‘missing edge’ of the triangle and we define a non-attention update operation ‘triangle multiplicative update’ that uses two edges to update the missing third edge (see Supplementary Methods 1.6.5 for details). The triangle multiplicative update was developed originally as a more symmetric and cheaper replacement for the attention, and networks that use only the attention or multiplicative update are both able to produce high-accuracy structures. However, the combination of the two updates is more accurate.
We also use a variant of axial attention within the MSA representation. During the per-sequence attention in the MSA, we project additional logits from the pair stack to bias the MSA attention. This closes the loop by providing information flow from the pair representation back into the MSA representation, ensuring that the overall Evoformer block is able to fully mix information between the pair and MSA representations and prepare for structure generation within the structure module.
End-to-end structure prediction
The structure module (Fig. 3d ) operates on a concrete 3D backbone structure using the pair representation and the original sequence row (single representation) of the MSA representation from the trunk. The 3D backbone structure is represented as N res independent rotations and translations, each with respect to the global frame (residue gas) (Fig. 3e ). These rotations and translations—representing the geometry of the N-Cα-C atoms—prioritize the orientation of the protein backbone so that the location of the side chain of each residue is highly constrained within that frame. Conversely, the peptide bond geometry is completely unconstrained and the network is observed to frequently violate the chain constraint during the application of the structure module as breaking this constraint enables the local refinement of all parts of the chain without solving complex loop closure problems. Satisfaction of the peptide bond geometry is encouraged during fine-tuning by a violation loss term. Exact enforcement of peptide bond geometry is only achieved in the post-prediction relaxation of the structure by gradient descent in the Amber 32 force field. Empirically, this final relaxation does not improve the accuracy of the model as measured by the global distance test (GDT) 33 or lDDT-Cα 34 but does remove distracting stereochemical violations without the loss of accuracy.
The residue gas representation is updated iteratively in two stages (Fig. 3d ). First, a geometry-aware attention operation that we term ‘invariant point attention’ (IPA) is used to update an N res set of neural activations (single representation) without changing the 3D positions, then an equivariant update operation is performed on the residue gas using the updated activations. The IPA augments each of the usual attention queries, keys and values with 3D points that are produced in the local frame of each residue such that the final value is invariant to global rotations and translations (see Methods ‘IPA’ for details). The 3D queries and keys also impose a strong spatial/locality bias on the attention, which is well-suited to the iterative refinement of the protein structure. After each attention operation and element-wise transition block, the module computes an update to the rotation and translation of each backbone frame. The application of these updates within the local frame of each residue makes the overall attention and update block an equivariant operation on the residue gas.
Predictions of side-chain χ angles as well as the final, per-residue accuracy of the structure (pLDDT) are computed with small per-residue networks on the final activations at the end of the network. The estimate of the TM-score (pTM) is obtained from a pairwise error prediction that is computed as a linear projection from the final pair representation. The final loss (which we term the frame-aligned point error (FAPE) (Fig. 3f )) compares the predicted atom positions to the true positions under many different alignments. For each alignment, defined by aligning the predicted frame ( R k , t k ) to the corresponding true frame, we compute the distance of all predicted atom positions x i from the true atom positions. The resulting N frames × N atoms distances are penalized with a clamped L 1 loss. This creates a strong bias for atoms to be correct relative to the local frame of each residue and hence correct with respect to its side-chain interactions, as well as providing the main source of chirality for AlphaFold ( Supplementary Methods 1.9.3 and Supplementary Fig. 9 ).
Training with labelled and unlabelled data
The AlphaFold architecture is able to train to high accuracy using only supervised learning on PDB data, but we are able to enhance accuracy (Fig. 4a ) using an approach similar to noisy student self-distillation 35 . In this procedure, we use a trained network to predict the structure of around 350,000 diverse sequences from Uniclust30 36 and make a new dataset of predicted structures filtered to a high-confidence subset. We then train the same architecture again from scratch using a mixture of PDB data and this new dataset of predicted structures as the training data, in which the various training data augmentations such as cropping and MSA subsampling make it challenging for the network to recapitulate the previously predicted structures. This self-distillation procedure makes effective use of the unlabelled sequence data and considerably improves the accuracy of the resulting network.
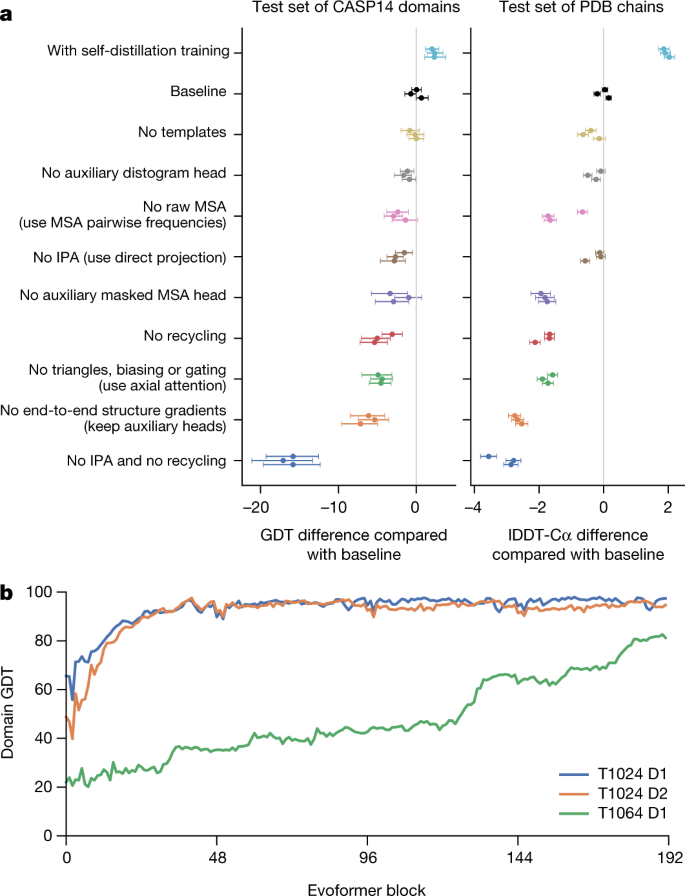
a , Ablation results on two target sets: the CASP14 set of domains ( n = 87 protein domains) and the PDB test set of chains with template coverage of ≤30% at 30% identity ( n = 2,261 protein chains). Domains are scored with GDT and chains are scored with lDDT-Cα. The ablations are reported as a difference compared with the average of the three baseline seeds. Means (points) and 95% bootstrap percentile intervals (error bars) are computed using bootstrap estimates of 10,000 samples. b , Domain GDT trajectory over 4 recycling iterations and 48 Evoformer blocks on CASP14 targets LmrP (T1024) and Orf8 (T1064) where D1 and D2 refer to the individual domains as defined by the CASP assessment. Both T1024 domains obtain the correct structure early in the network, whereas the structure of T1064 changes multiple times and requires nearly the full depth of the network to reach the final structure. Note, 48 Evoformer blocks comprise one recycling iteration.
Additionally, we randomly mask out or mutate individual residues within the MSA and have a Bidirectional Encoder Representations from Transformers (BERT)-style 37 objective to predict the masked elements of the MSA sequences. This objective encourages the network to learn to interpret phylogenetic and covariation relationships without hardcoding a particular correlation statistic into the features. The BERT objective is trained jointly with the normal PDB structure loss on the same training examples and is not pre-trained, in contrast to recent independent work 38 .
Interpreting the neural network
To understand how AlphaFold predicts protein structure, we trained a separate structure module for each of the 48 Evoformer blocks in the network while keeping all parameters of the main network frozen ( Supplementary Methods 1.14 ). Including our recycling stages, this provides a trajectory of 192 intermediate structures—one per full Evoformer block—in which each intermediate represents the belief of the network of the most likely structure at that block. The resulting trajectories are surprisingly smooth after the first few blocks, showing that AlphaFold makes constant incremental improvements to the structure until it can no longer improve (see Fig. 4b for a trajectory of accuracy). These trajectories also illustrate the role of network depth. For very challenging proteins such as ORF8 of SARS-CoV-2 (T1064), the network searches and rearranges secondary structure elements for many layers before settling on a good structure. For other proteins such as LmrP (T1024), the network finds the final structure within the first few layers. Structure trajectories of CASP14 targets T1024, T1044, T1064 and T1091 that demonstrate a clear iterative building process for a range of protein sizes and difficulties are shown in Supplementary Videos 1 – 4 . In Supplementary Methods 1.16 and Supplementary Figs. 12 , 13 , we interpret the attention maps produced by AlphaFold layers.
Figure 4a contains detailed ablations of the components of AlphaFold that demonstrate that a variety of different mechanisms contribute to AlphaFold accuracy. Detailed descriptions of each ablation model, their training details, extended discussion of ablation results and the effect of MSA depth on each ablation are provided in Supplementary Methods 1.13 and Supplementary Fig. 10 .
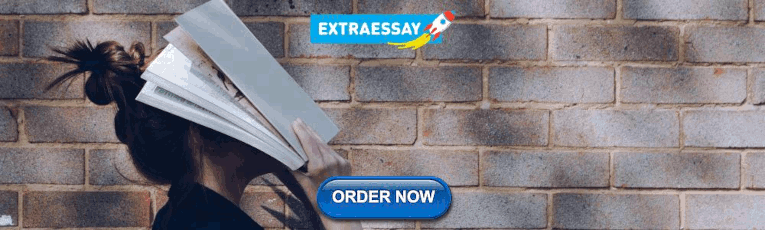
MSA depth and cross-chain contacts
Although AlphaFold has a high accuracy across the vast majority of deposited PDB structures, we note that there are still factors that affect accuracy or limit the applicability of the model. The model uses MSAs and the accuracy decreases substantially when the median alignment depth is less than around 30 sequences (see Fig. 5a for details). We observe a threshold effect where improvements in MSA depth over around 100 sequences lead to small gains. We hypothesize that the MSA information is needed to coarsely find the correct structure within the early stages of the network, but refinement of that prediction into a high-accuracy model does not depend crucially on the MSA information. The other substantial limitation that we have observed is that AlphaFold is much weaker for proteins that have few intra-chain or homotypic contacts compared to the number of heterotypic contacts (further details are provided in a companion paper 39 ). This typically occurs for bridging domains within larger complexes in which the shape of the protein is created almost entirely by interactions with other chains in the complex. Conversely, AlphaFold is often able to give high-accuracy predictions for homomers, even when the chains are substantially intertwined (Fig. 5b ). We expect that the ideas of AlphaFold are readily applicable to predicting full hetero-complexes in a future system and that this will remove the difficulty with protein chains that have a large number of hetero-contacts.
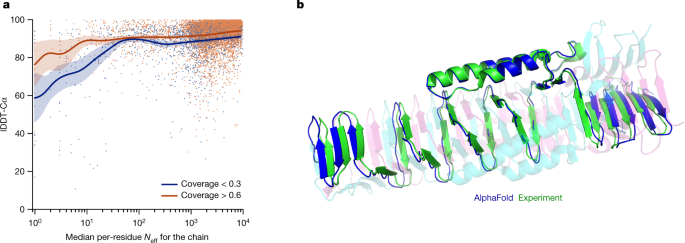
a , Backbone accuracy (lDDT-Cα) for the redundancy-reduced set of the PDB after our training data cut-off, restricting to proteins in which at most 25% of the long-range contacts are between different heteromer chains. We further consider two groups of proteins based on template coverage at 30% sequence identity: covering more than 60% of the chain ( n = 6,743 protein chains) and covering less than 30% of the chain ( n = 1,596 protein chains). MSA depth is computed by counting the number of non-gap residues for each position in the MSA (using the N eff weighting scheme; see Methods for details) and taking the median across residues. The curves are obtained through Gaussian kernel average smoothing (window size is 0.2 units in log 10 ( N eff )); the shaded area is the 95% confidence interval estimated using bootstrap of 10,000 samples. b , An intertwined homotrimer (PDB 6SK0) is correctly predicted without input stoichiometry and only a weak template (blue is predicted and green is experimental).
Related work
The prediction of protein structures has had a long and varied development, which is extensively covered in a number of reviews 14 , 40 , 41 , 42 , 43 . Despite the long history of applying neural networks to structure prediction 14 , 42 , 43 , they have only recently come to improve structure prediction 10 , 11 , 44 , 45 . These approaches effectively leverage the rapid improvement in computer vision systems 46 by treating the problem of protein structure prediction as converting an ‘image’ of evolutionary couplings 22 , 23 , 24 to an ‘image’ of the protein distance matrix and then integrating the distance predictions into a heuristic system that produces the final 3D coordinate prediction. A few recent studies have been developed to predict the 3D coordinates directly 47 , 48 , 49 , 50 , but the accuracy of these approaches does not match traditional, hand-crafted structure prediction pipelines 51 . In parallel, the success of attention-based networks for language processing 52 and, more recently, computer vision 31 , 53 has inspired the exploration of attention-based methods for interpreting protein sequences 54 , 55 , 56 .
The methodology that we have taken in designing AlphaFold is a combination of the bioinformatics and physical approaches: we use a physical and geometric inductive bias to build components that learn from PDB data with minimal imposition of handcrafted features (for example, AlphaFold builds hydrogen bonds effectively without a hydrogen bond score function). This results in a network that learns far more efficiently from the limited data in the PDB but is able to cope with the complexity and variety of structural data.
In particular, AlphaFold is able to handle missing the physical context and produce accurate models in challenging cases such as intertwined homomers or proteins that only fold in the presence of an unknown haem group. The ability to handle underspecified structural conditions is essential to learning from PDB structures as the PDB represents the full range of conditions in which structures have been solved. In general, AlphaFold is trained to produce the protein structure most likely to appear as part of a PDB structure. For example, in cases in which a particular stochiometry, ligand or ion is predictable from the sequence alone, AlphaFold is likely to produce a structure that respects those constraints implicitly.
AlphaFold has already demonstrated its utility to the experimental community, both for molecular replacement 57 and for interpreting cryogenic electron microscopy maps 58 . Moreover, because AlphaFold outputs protein coordinates directly, AlphaFold produces predictions in graphics processing unit (GPU) minutes to GPU hours depending on the length of the protein sequence (for example, around one GPU minute per model for 384 residues; see Methods for details). This opens up the exciting possibility of predicting structures at the proteome-scale and beyond—in a companion paper 39 , we demonstrate the application of AlphaFold to the entire human proteome 39 .
The explosion in available genomic sequencing techniques and data has revolutionized bioinformatics but the intrinsic challenge of experimental structure determination has prevented a similar expansion in our structural knowledge. By developing an accurate protein structure prediction algorithm, coupled with existing large and well-curated structure and sequence databases assembled by the experimental community, we hope to accelerate the advancement of structural bioinformatics that can keep pace with the genomics revolution. We hope that AlphaFold—and computational approaches that apply its techniques for other biophysical problems—will become essential tools of modern biology.
Full algorithm details
Extensive explanations of the components and their motivations are available in Supplementary Methods 1.1–1.10 , in addition, pseudocode is available in Supplementary Information Algorithms 1–32 , network diagrams in Supplementary Figs. 1 – 8 , input features in Supplementary Table 1 and additional details are provided in Supplementary Tables 2 , 3 . Training and inference details are provided in Supplementary Methods 1.11–1.12 and Supplementary Tables 4 , 5 .
The IPA module combines the pair representation, the single representation and the geometric representation to update the single representation (Supplementary Fig. 8 ). Each of these representations contributes affinities to the shared attention weights and then uses these weights to map its values to the output. The IPA operates in 3D space. Each residue produces query points, key points and value points in its local frame. These points are projected into the global frame using the backbone frame of the residue in which they interact with each other. The resulting points are then projected back into the local frame. The affinity computation in the 3D space uses squared distances and the coordinate transformations ensure the invariance of this module with respect to the global frame (see Supplementary Methods 1.8.2 ‘Invariant point attention (IPA)’ for the algorithm, proof of invariance and a description of the full multi-head version). A related construction that uses classic geometric invariants to construct pairwise features in place of the learned 3D points has been applied to protein design 59 .
In addition to the IPA, standard dot product attention is computed on the abstract single representation and a special attention on the pair representation. The pair representation augments both the logits and the values of the attention process, which is the primary way in which the pair representation controls the structure generation.
Inputs and data sources
Inputs to the network are the primary sequence, sequences from evolutionarily related proteins in the form of a MSA created by standard tools including jackhmmer 60 and HHBlits 61 , and 3D atom coordinates of a small number of homologous structures (templates) where available. For both the MSA and templates, the search processes are tuned for high recall; spurious matches will probably appear in the raw MSA but this matches the training condition of the network.
One of the sequence databases used, Big Fantastic Database (BFD), was custom-made and released publicly (see ‘Data availability’) and was used by several CASP teams. BFD is one of the largest publicly available collections of protein families. It consists of 65,983,866 families represented as MSAs and hidden Markov models (HMMs) covering 2,204,359,010 protein sequences from reference databases, metagenomes and metatranscriptomes.
BFD was built in three steps. First, 2,423,213,294 protein sequences were collected from UniProt (Swiss-Prot&TrEMBL, 2017-11) 62 , a soil reference protein catalogue and the marine eukaryotic reference catalogue 7 , and clustered to 30% sequence identity, while enforcing a 90% alignment coverage of the shorter sequences using MMseqs2/Linclust 63 . This resulted in 345,159,030 clusters. For computational efficiency, we removed all clusters with less than three members, resulting in 61,083,719 clusters. Second, we added 166,510,624 representative protein sequences from Metaclust NR (2017-05; discarding all sequences shorter than 150 residues) 63 by aligning them against the cluster representatives using MMseqs2 64 . Sequences that fulfilled the sequence identity and coverage criteria were assigned to the best scoring cluster. The remaining 25,347,429 sequences that could not be assigned were clustered separately and added as new clusters, resulting in the final clustering. Third, for each of the clusters, we computed an MSA using FAMSA 65 and computed the HMMs following the Uniclust HH-suite database protocol 36 .
The following versions of public datasets were used in this study. Our models were trained on a copy of the PDB 5 downloaded on 28 August 2019. For finding template structures at prediction time, we used a copy of the PDB downloaded on 14 May 2020, and the PDB70 66 clustering database downloaded on 13 May 2020. For MSA lookup at both training and prediction time, we used Uniref90 67 v.2020_01, BFD, Uniclust30 36 v.2018_08 and MGnify 6 v.2018_12. For sequence distillation, we used Uniclust30 36 v.2018_08 to construct a distillation structure dataset. Full details are provided in Supplementary Methods 1.2 .
For MSA search on BFD + Uniclust30, and template search against PDB70, we used HHBlits 61 and HHSearch 66 from hh-suite v.3.0-beta.3 (version 14/07/2017). For MSA search on Uniref90 and clustered MGnify, we used jackhmmer from HMMER3 68 . For constrained relaxation of structures, we used OpenMM v.7.3.1 69 with the Amber99sb force field 32 . For neural network construction, running and other analyses, we used TensorFlow 70 , Sonnet 71 , NumPy 72 , Python 73 and Colab 74 .
To quantify the effect of the different sequence data sources, we re-ran the CASP14 proteins using the same models but varying how the MSA was constructed. Removing BFD reduced the mean accuracy by 0.4 GDT, removing Mgnify reduced the mean accuracy by 0.7 GDT, and removing both reduced the mean accuracy by 6.1 GDT. In each case, we found that most targets had very small changes in accuracy but a few outliers had very large (20+ GDT) differences. This is consistent with the results in Fig. 5a in which the depth of the MSA is relatively unimportant until it approaches a threshold value of around 30 sequences when the MSA size effects become quite large. We observe mostly overlapping effects between inclusion of BFD and Mgnify, but having at least one of these metagenomics databases is very important for target classes that are poorly represented in UniRef, and having both was necessary to achieve full CASP accuracy.
Training regimen
To train, we use structures from the PDB with a maximum release date of 30 April 2018. Chains are sampled in inverse proportion to cluster size of a 40% sequence identity clustering. We then randomly crop them to 256 residues and assemble into batches of size 128. We train the model on Tensor Processing Unit (TPU) v3 with a batch size of 1 per TPU core, hence the model uses 128 TPU v3 cores. The model is trained until convergence (around 10 million samples) and further fine-tuned using longer crops of 384 residues, larger MSA stack and reduced learning rate (see Supplementary Methods 1.11 for the exact configuration). The initial training stage takes approximately 1 week, and the fine-tuning stage takes approximately 4 additional days.
The network is supervised by the FAPE loss and a number of auxiliary losses. First, the final pair representation is linearly projected to a binned distance distribution (distogram) prediction, scored with a cross-entropy loss. Second, we use random masking on the input MSAs and require the network to reconstruct the masked regions from the output MSA representation using a BERT-like loss 37 . Third, the output single representations of the structure module are used to predict binned per-residue lDDT-Cα values. Finally, we use an auxiliary side-chain loss during training, and an auxiliary structure violation loss during fine-tuning. Detailed descriptions and weighting are provided in the Supplementary Information .
An initial model trained with the above objectives was used to make structure predictions for a Uniclust dataset of 355,993 sequences with the full MSAs. These predictions were then used to train a final model with identical hyperparameters, except for sampling examples 75% of the time from the Uniclust prediction set, with sub-sampled MSAs, and 25% of the time from the clustered PDB set.
We train five different models using different random seeds, some with templates and some without, to encourage diversity in the predictions (see Supplementary Table 5 and Supplementary Methods 1.12.1 for details). We also fine-tuned these models after CASP14 to add a pTM prediction objective ( Supplementary Methods 1.9.7 ) and use the obtained models for Fig. 2d .
Inference regimen
We inference the five trained models and use the predicted confidence score to select the best model per target.
Using our CASP14 configuration for AlphaFold, the trunk of the network is run multiple times with different random choices for the MSA cluster centres (see Supplementary Methods 1.11.2 for details of the ensembling procedure). The full time to make a structure prediction varies considerably depending on the length of the protein. Representative timings for the neural network using a single model on V100 GPU are 4.8 min with 256 residues, 9.2 min with 384 residues and 18 h at 2,500 residues. These timings are measured using our open-source code, and the open-source code is notably faster than the version we ran in CASP14 as we now use the XLA compiler 75 .
Since CASP14, we have found that the accuracy of the network without ensembling is very close or equal to the accuracy with ensembling and we turn off ensembling for most inference. Without ensembling, the network is 8× faster and the representative timings for a single model are 0.6 min with 256 residues, 1.1 min with 384 residues and 2.1 h with 2,500 residues.
Inferencing large proteins can easily exceed the memory of a single GPU. For a V100 with 16 GB of memory, we can predict the structure of proteins up to around 1,300 residues without ensembling and the 256- and 384-residue inference times are using the memory of a single GPU. The memory usage is approximately quadratic in the number of residues, so a 2,500-residue protein involves using unified memory so that we can greatly exceed the memory of a single V100. In our cloud setup, a single V100 is used for computation on a 2,500-residue protein but we requested four GPUs to have sufficient memory.
Searching genetic sequence databases to prepare inputs and final relaxation of the structures take additional central processing unit (CPU) time but do not require a GPU or TPU.
The predicted structure is compared to the true structure from the PDB in terms of lDDT metric 34 , as this metric reports the domain accuracy without requiring a domain segmentation of chain structures. The distances are either computed between all heavy atoms (lDDT) or only the Cα atoms to measure the backbone accuracy (lDDT-Cα). As lDDT-Cα only focuses on the Cα atoms, it does not include the penalty for structural violations and clashes. Domain accuracies in CASP are reported as GDT 33 and the TM-score 27 is used as a full chain global superposition metric.
We also report accuracies using the r.m.s.d. 95 (Cα r.m.s.d. at 95% coverage). We perform five iterations of (1) a least-squares alignment of the predicted structure and the PDB structure on the currently chosen Cα atoms (using all Cα atoms in the first iteration); (2) selecting the 95% of Cα atoms with the lowest alignment error. The r.m.s.d. of the atoms chosen for the final iterations is the r.m.s.d. 95 . This metric is more robust to apparent errors that can originate from crystal structure artefacts, although in some cases the removed 5% of residues will contain genuine modelling errors.
Test set of recent PDB sequences
For evaluation on recent PDB sequences (Figs. 2 a–d, 4 a, 5a ), we used a copy of the PDB downloaded 15 February 2021. Structures were filtered to those with a release date after 30 April 2018 (the date limit for inclusion in the training set for AlphaFold). Chains were further filtered to remove sequences that consisted of a single amino acid as well as sequences with an ambiguous chemical component at any residue position. Exact duplicates were removed, with the chain with the most resolved Cα atoms used as the representative sequence. Subsequently, structures with less than 16 resolved residues, with unknown residues or solved by NMR methods were removed. As the PDB contains many near-duplicate sequences, the chain with the highest resolution was selected from each cluster in the PDB 40% sequence clustering of the data. Furthermore, we removed all sequences for which fewer than 80 amino acids had the alpha carbon resolved and removed chains with more than 1,400 residues. The final dataset contained 10,795 protein sequences.
The procedure for filtering the recent PDB dataset based on prior template identity was as follows. Hmmsearch was run with default parameters against a copy of the PDB SEQRES fasta downloaded 15 February 2021. Template hits were accepted if the associated structure had a release date earlier than 30 April 2018. Each residue position in a query sequence was assigned the maximum identity of any template hit covering that position. Filtering then proceeded as described in the individual figure legends, based on a combination of maximum identity and sequence coverage.
The MSA depth analysis was based on computing the normalized number of effective sequences ( N eff ) for each position of a query sequence. Per-residue N eff values were obtained by counting the number of non-gap residues in the MSA for this position and weighting the sequences using the N eff scheme 76 with a threshold of 80% sequence identity measured on the region that is non-gap in either sequence.
Reporting summary
Further information on research design is available in the Nature Research Reporting Summary linked to this paper.
Data availability
All input data are freely available from public sources.
Structures from the PDB were used for training and as templates ( https://www.wwpdb.org/ftp/pdb-ftp-sites ; for the associated sequence data and 40% sequence clustering see also https://ftp.wwpdb.org/pub/pdb/derived_data/ and https://cdn.rcsb.org/resources/sequence/clusters/bc-40.out ). Training used a version of the PDB downloaded 28 August 2019, while the CASP14 template search used a version downloaded 14 May 2020. The template search also used the PDB70 database, downloaded 13 May 2020 ( https://wwwuser.gwdg.de/~compbiol/data/hhsuite/databases/hhsuite_dbs/ ).
We show experimental structures from the PDB with accession numbers 6Y4F 77 , 6YJ1 78 , 6VR4 79 , 6SK0 80 , 6 FES 81 , 6W6W 82 , 6T1Z 83 and 7JTL 84 .
For MSA lookup at both the training and prediction time, we used UniRef90 v.2020_01 (https://ftp.ebi.ac.uk/pub/databases/uniprot/previous_releases/release-2020_01/uniref/), BFD ( https://bfd.mmseqs.com ), Uniclust30 v.2018_08 ( https://wwwuser.gwdg.de/~compbiol/uniclust/2018_08/ ) and MGnify clusters v.2018_12 ( https://ftp.ebi.ac.uk/pub/databases/metagenomics/peptide_database/2018_12/ ). Uniclust30 v.2018_08 was also used as input for constructing a distillation structure dataset.
Code availability
Source code for the AlphaFold model, trained weights and inference script are available under an open-source license at https://github.com/deepmind/alphafold .
Neural networks were developed with TensorFlow v.1 ( https://github.com/tensorflow/tensorflow ), Sonnet v.1 ( https://github.com/deepmind/sonnet ), JAX v.0.1.69 ( https://github.com/google/jax/ ) and Haiku v.0.0.4 ( https://github.com/deepmind/dm-haiku ). The XLA compiler is bundled with JAX and does not have a separate version number.
For MSA search on BFD+Uniclust30, and for template search against PDB70, we used HHBlits and HHSearch from hh-suite v.3.0-beta.3 release 14/07/2017 ( https://github.com/soedinglab/hh-suite ). For MSA search on UniRef90 and clustered MGnify, we used jackhmmer from HMMER v.3.3 ( http://eddylab.org/software/hmmer/ ). For constrained relaxation of structures, we used OpenMM v.7.3.1 ( https://github.com/openmm/openmm ) with the Amber99sb force field.
Construction of BFD used MMseqs2 v.925AF ( https://github.com/soedinglab/MMseqs2 ) and FAMSA v.1.2.5 ( https://github.com/refresh-bio/FAMSA ).
Data analysis used Python v.3.6 ( https://www.python.org/ ), NumPy v.1.16.4 ( https://github.com/numpy/numpy ), SciPy v.1.2.1 ( https://www.scipy.org/ ), seaborn v.0.11.1 ( https://github.com/mwaskom/seaborn ), Matplotlib v.3.3.4 ( https://github.com/matplotlib/matplotlib ), bokeh v.1.4.0 ( https://github.com/bokeh/bokeh ), pandas v.1.1.5 ( https://github.com/pandas-dev/pandas ), plotnine v.0.8.0 ( https://github.com/has2k1/plotnine ), statsmodels v.0.12.2 ( https://github.com/statsmodels/statsmodels ) and Colab ( https://research.google.com/colaboratory ). TM-align v.20190822 ( https://zhanglab.dcmb.med.umich.edu/TM-align/ ) was used for computing TM-scores. Structure visualizations were created in Pymol v.2.3.0 ( https://github.com/schrodinger/pymol-open-source ).
Thompson, M. C., Yeates, T. O. & Rodriguez, J. A. Advances in methods for atomic resolution macromolecular structure determination. F1000Res . 9 , 667 (2020).
Article CAS Google Scholar
Bai, X.-C., McMullan, G. & Scheres, S. H. W. How cryo-EM is revolutionizing structural biology. Trends Biochem. Sci . 40 , 49–57 (2015).
Article CAS PubMed Google Scholar
Jaskolski, M., Dauter, Z. & Wlodawer, A. A brief history of macromolecular crystallography, illustrated by a family tree and its Nobel fruits. FEBS J . 281 , 3985–4009 (2014).
Article CAS PubMed PubMed Central Google Scholar
Wüthrich, K. The way to NMR structures of proteins. Nat. Struct. Biol . 8 , 923–925 (2001).
Article PubMed Google Scholar
wwPDB Consortium. Protein Data Bank: the single global archive for 3D macromolecular structure data. Nucleic Acids Res . 47 , D520–D528 (2018).
Article Google Scholar
Mitchell, A. L. et al. MGnify: the microbiome analysis resource in 2020. Nucleic Acids Res . 48 , D570–D578 (2020).
CAS PubMed Google Scholar
Steinegger, M., Mirdita, M. & Söding, J. Protein-level assembly increases protein sequence recovery from metagenomic samples manyfold. Nat. Methods 16 , 603–606 (2019).
Dill, K. A., Ozkan, S. B., Shell, M. S. & Weikl, T. R. The protein folding problem. Annu. Rev. Biophys . 37 , 289–316 (2008).
Article ADS CAS PubMed PubMed Central Google Scholar
Anfinsen, C. B. Principles that govern the folding of protein chains. Science 181 , 223–230 (1973).
Article ADS CAS PubMed Google Scholar
Senior, A. W. et al. Improved protein structure prediction using potentials from deep learning. Nature 577 , 706–710 (2020).
Wang, S., Sun, S., Li, Z., Zhang, R. & Xu, J. Accurate de novo prediction of protein contact map by ultra-deep learning model. PLOS Comput. Biol . 13 , e1005324 (2017).
Article ADS PubMed PubMed Central Google Scholar
Zheng, W. et al. Deep-learning contact-map guided protein structure prediction in CASP13. Proteins 87 , 1149–1164 (2019).
Abriata, L. A., Tamò, G. E. & Dal Peraro, M. A further leap of improvement in tertiary structure prediction in CASP13 prompts new routes for future assessments. Proteins 87 , 1100–1112 (2019).
Pearce, R. & Zhang, Y. Deep learning techniques have significantly impacted protein structure prediction and protein design. Curr. Opin. Struct. Biol . 68 , 194–207 (2021).
Moult, J., Fidelis, K., Kryshtafovych, A., Schwede, T. & Topf, M. Critical assessment of techniques for protein structure prediction, fourteenth round. CASP 14 Abstract Book https://www.predictioncenter.org/casp14/doc/CASP14_Abstracts.pdf (2020).
Brini, E., Simmerling, C. & Dill, K. Protein storytelling through physics. Science 370 , eaaz3041 (2020).
Sippl, M. J. Calculation of conformational ensembles from potentials of mean force. An approach to the knowledge-based prediction of local structures in globular proteins. J. Mol. Biol . 213 , 859–883 (1990).
Šali, A. & Blundell, T. L. Comparative protein modelling by satisfaction of spatial restraints. J. Mol. Biol . 234 , 779–815 (1993).
Roy, A., Kucukural, A. & Zhang, Y. I-TASSER: a unified platform for automated protein structure and function prediction. Nat. Protocols 5 , 725–738 (2010).
Altschuh, D., Lesk, A. M., Bloomer, A. C. & Klug, A. Correlation of co-ordinated amino acid substitutions with function in viruses related to tobacco mosaic virus. J. Mol. Biol . 193 , 693–707 (1987).
Shindyalov, I. N., Kolchanov, N. A. & Sander, C. Can three-dimensional contacts in protein structures be predicted by analysis of correlated mutations? Protein Eng . 7 , 349–358 (1994).
Weigt, M., White, R. A., Szurmant, H., Hoch, J. A. & Hwa, T. Identification of direct residue contacts in protein–protein interaction by message passing. Proc. Natl Acad. Sci. USA 106 , 67–72 (2009).
Marks, D. S. et al. Protein 3D structure computed from evolutionary sequence variation. PLoS ONE 6 , e28766 (2011).
Jones, D. T., Buchan, D. W. A., Cozzetto, D. & Pontil, M. PSICOV: precise structural contact prediction using sparse inverse covariance estimation on large multiple sequence alignments. Bioinformatics 28 , 184–190 (2012).
Moult, J., Pedersen, J. T., Judson, R. & Fidelis, K. A large-scale experiment to assess protein structure prediction methods. Proteins 23 , ii–iv (1995).
Kryshtafovych, A., Schwede, T., Topf, M., Fidelis, K. & Moult, J. Critical assessment of methods of protein structure prediction (CASP)-round XIII. Proteins 87 , 1011–1020 (2019).
Zhang, Y. & Skolnick, J. Scoring function for automated assessment of protein structure template quality. Proteins 57 , 702–710 (2004).
Tu, Z. & Bai, X. Auto-context and its application to high-level vision tasks and 3D brain image segmentation. IEEE Trans. Pattern Anal. Mach. Intell . 32 , 1744–1757 (2010).
Carreira, J., Agrawal, P., Fragkiadaki, K. & Malik, J. Human pose estimation with iterative error feedback. In Proc. IEEE Conference on Computer Vision and Pattern Recognition 4733–4742 (2016).
Mirabello, C. & Wallner, B. rawMSA: end-to-end deep learning using raw multiple sequence alignments. PLoS ONE 14 , e0220182 (2019).
Huang, Z. et al. CCNet: criss-cross attention for semantic segmentation. In Proc. IEEE/CVF International Conference on Computer Vision 603–612 (2019).
Hornak, V. et al. Comparison of multiple Amber force fields and development of improved protein backbone parameters. Proteins 65 , 712–725 (2006).
Zemla, A. LGA: a method for finding 3D similarities in protein structures. Nucleic Acids Res . 31 , 3370–3374 (2003).
Mariani, V., Biasini, M., Barbato, A. & Schwede, T. lDDT: a local superposition-free score for comparing protein structures and models using distance difference tests. Bioinformatics 29 , 2722–2728 (2013).
Xie, Q., Luong, M.-T., Hovy, E. & Le, Q. V. Self-training with noisy student improves imagenet classification. In Proc. IEEE/CVF Conference on Computer Vision and Pattern Recognition 10687–10698 (2020).
Mirdita, M. et al. Uniclust databases of clustered and deeply annotated protein sequences and alignments. Nucleic Acids Res . 45 , D170–D176 (2017).
Devlin, J., Chang, M.-W., Lee, K. & Toutanova, K. BERT: pre-training of deep bidirectional transformers for language understanding. In Proc. 2019 Conference of the North American Chapter of the Association for Computational Linguistics: Human Language Technologies 1, 4171–4186 (2019).
Rao, R. et al. MSA transformer. In Proc. 38th International Conference on Machine Learning PMLR 139, 8844–8856 (2021).
Tunyasuvunakool, K. et al. Highly accurate protein structure prediction for the human proteome. Nature https://doi.org/10.1038/s41586-021-03828-1 (2021).
Kuhlman, B. & Bradley, P. Advances in protein structure prediction and design. Nat. Rev. Mol. Cell Biol . 20 , 681–697 (2019).
Marks, D. S., Hopf, T. A. & Sander, C. Protein structure prediction from sequence variation. Nat. Biotechnol . 30 , 1072–1080 (2012).
Qian, N. & Sejnowski, T. J. Predicting the secondary structure of globular proteins using neural network models. J. Mol. Biol . 202 , 865–884 (1988).
Fariselli, P., Olmea, O., Valencia, A. & Casadio, R. Prediction of contact maps with neural networks and correlated mutations. Protein Eng . 14 , 835–843 (2001).
Yang, J. et al. Improved protein structure prediction using predicted interresidue orientations. Proc. Natl Acad. Sci. USA 117 , 1496–1503 (2020).
Li, Y. et al. Deducing high-accuracy protein contact-maps from a triplet of coevolutionary matrices through deep residual convolutional networks. PLOS Comput. Biol . 17 , e1008865 (2021).
He, K., Zhang, X., Ren, S. & Sun, J. Deep residual learning for image recognition. In Proc. IEEE Conference on Computer Vision and Pattern Recognition 770–778 (2016).
AlQuraishi, M. End-to-end differentiable learning of protein structure. Cell Syst . 8 , 292–301 (2019).
Senior, A. W. et al. Protein structure prediction using multiple deep neural networks in the 13th Critical Assessment of Protein Structure Prediction (CASP13). Proteins 87 , 1141–1148 (2019).
Ingraham, J., Riesselman, A. J., Sander, C. & Marks, D. S. Learning protein structure with a differentiable simulator. in Proc. International Conference on Learning Representations (2019).
Li, J. Universal transforming geometric network. Preprint at https://arxiv.org/abs/1908.00723 (2019).
Xu, J., McPartlon, M. & Li, J. Improved protein structure prediction by deep learning irrespective of co-evolution information. Nat. Mach. Intell . 3 , 601–609 (2021).
Article PubMed PubMed Central Google Scholar
Vaswani, A. et al. Attention is all you need. In Advances in Neural Information Processing Systems 5998–6008 (2017).
Wang, H. et al. Axial-deeplab: stand-alone axial-attention for panoptic segmentation. in European Conference on Computer Vision 108–126 (Springer, 2020).
Alley, E. C., Khimulya, G., Biswas, S., AlQuraishi, M. & Church, G. M. Unified rational protein engineering with sequence-based deep representation learning. Nat. Methods 16 , 1315–1322 (2019).
Heinzinger, M. et al. Modeling aspects of the language of life through transfer-learning protein sequences. BMC Bioinformatics 20 , 723 (2019).
Rives, A. et al. Biological structure and function emerge from scaling unsupervised learning to 250 million protein sequences. Proc. Natl Acad. Sci. USA 118 , e2016239118 (2021).
Pereira, J. et al. High-accuracy protein structure prediction in CASP14. Proteins https://doi.org/10.1002/prot.26171 (2021).
Gupta, M. et al. CryoEM and AI reveal a structure of SARS-CoV-2 Nsp2, a multifunctional protein involved in key host processes. Preprint at https://doi.org/10.1101/2021.05.10.443524 (2021).
Ingraham, J., Garg, V. K., Barzilay, R. & Jaakkola, T. Generative models for graph-based protein design. in Proc. 33rd Conference on Neural Information Processing Systems (2019).
Johnson, L. S., Eddy, S. R. & Portugaly, E. Hidden Markov model speed heuristic and iterative HMM search procedure. BMC Bioinformatics 11 , 431 (2010).
Remmert, M., Biegert, A., Hauser, A. & Söding, J. HHblits: lightning-fast iterative protein sequence searching by HMM-HMM alignment. Nat. Methods 9 , 173–175 (2012).
The UniProt Consortium. UniProt: the universal protein knowledgebase in 2021. Nucleic Acids Res . 49 , D480–D489 (2020).
Steinegger, M. & Söding, J. Clustering huge protein sequence sets in linear time. Nat. Commun . 9 , 2542 (2018).
Steinegger, M. & Söding, J. MMseqs2 enables sensitive protein sequence searching for the analysis of massive data sets. Nat. Biotechnol . 35 , 1026–1028 (2017).
Deorowicz, S., Debudaj-Grabysz, A. & Gudyś, A. FAMSA: fast and accurate multiple sequence alignment of huge protein families. Sci. Rep . 6 , 33964 (2016).
Steinegger, M. et al. HH-suite3 for fast remote homology detection and deep protein annotation. BMC Bioinformatics 20 , 473 (2019).
Suzek, B. E., Wang, Y., Huang, H., McGarvey, P. B. & Wu, C. H. UniRef clusters: a comprehensive and scalable alternative for improving sequence similarity searches. Bioinformatics 31 , 926–932 (2015).
Eddy, S. R. Accelerated profile HMM searches. PLOS Comput. Biol . 7 , e1002195 (2011).
Article ADS MathSciNet CAS PubMed PubMed Central Google Scholar
Eastman, P. et al. OpenMM 7: rapid development of high performance algorithms for molecular dynamics. PLOS Comput. Biol . 13 , e1005659 (2017).
Ashish, A. M. A. et al. TensorFlow: large-scale machine learning on heterogeneous systems. Preprint at https://arxiv.org/abs/1603.04467 (2015).
Reynolds, M. et al. Open sourcing Sonnet – a new library for constructing neural networks. DeepMind https://deepmind.com/blog/open-sourcing-sonnet/ (7 April 2017).
Harris, C. R. et al. Array programming with NumPy. Nature 585 , 357–362 (2020).
Van Rossum, G. & Drake, F. L. Python 3 Reference Manual (CreateSpace, 2009).
Bisong, E. in Building Machine Learning and Deep Learning Models on Google Cloud Platform: A Comprehensive Guide for Beginners 59–64 (Apress, 2019).
TensorFlow. XLA: Optimizing Compiler for TensorFlow. https://www.tensorflow.org/xla (2018).
Wu, T., Hou, J., Adhikari, B. & Cheng, J. Analysis of several key factors influencing deep learning-based inter-residue contact prediction. Bioinformatics 36 , 1091–1098 (2020).
Jiang, W. et al. MrpH, a new class of metal-binding adhesin, requires zinc to mediate biofilm formation. PLoS Pathog . 16 , e1008707 (2020).
Dunne, M., Ernst, P., Sobieraj, A., Pluckthun, A. & Loessner, M. J. The M23 peptidase domain of the Staphylococcal phage 2638A endolysin. PDB https://doi.org/10.2210/pdb6YJ1/pdb (2020).
Drobysheva, A. V. et al. Structure and function of virion RNA polymerase of a crAss-like phage. Nature 589 , 306–309 (2021).
Flaugnatti, N. et al. Structural basis for loading and inhibition of a bacterial T6SS phospholipase effector by the VgrG spike. EMBO J . 39 , e104129 (2020).
ElGamacy, M. et al. An interface-driven design strategy yields a novel, corrugated protein architecture. ACS Synth. Biol . 7 , 2226–2235 (2018).
Lim, C. J. et al. The structure of human CST reveals a decameric assembly bound to telomeric DNA. Science 368 , 1081–1085 (2020).
Debruycker, V. et al. An embedded lipid in the multidrug transporter LmrP suggests a mechanism for polyspecificity. Nat. Struct. Mol. Biol . 27 , 829–835 (2020).
Flower, T. G. et al. Structure of SARS-CoV-2 ORF8, a rapidly evolving immune evasion protein. Proc. Natl Acad. Sci. USA 118 , e2021785118 (2021).
Download references
Acknowledgements
We thank A. Rrustemi, A. Gu, A. Guseynov, B. Hechtman, C. Beattie, C. Jones, C. Donner, E. Parisotto, E. Elsen, F. Popovici, G. Necula, H. Maclean, J. Menick, J. Kirkpatrick, J. Molloy, J. Yim, J. Stanway, K. Simonyan, L. Sifre, L. Martens, M. Johnson, M. O’Neill, N. Antropova, R. Hadsell, S. Blackwell, S. Das, S. Hou, S. Gouws, S. Wheelwright, T. Hennigan, T. Ward, Z. Wu, Ž. Avsec and the Research Platform Team for their contributions; M. Mirdita for his help with the datasets; M. Piovesan-Forster, A. Nelson and R. Kemp for their help managing the project; the JAX, TensorFlow and XLA teams for detailed support and enabling machine learning models of the complexity of AlphaFold; our colleagues at DeepMind, Google and Alphabet for their encouragement and support; and J. Moult and the CASP14 organizers, and the experimentalists whose structures enabled the assessment. M.S. acknowledges support from the National Research Foundation of Korea grant (2019R1A6A1A10073437, 2020M3A9G7103933) and the Creative-Pioneering Researchers Program through Seoul National University.
Author information
These authors contributed equally: John Jumper, Richard Evans, Alexander Pritzel, Tim Green, Michael Figurnov, Olaf Ronneberger, Kathryn Tunyasuvunakool, Russ Bates, Augustin Žídek, Anna Potapenko, Alex Bridgland, Clemens Meyer, Simon A. A. Kohl, Andrew J. Ballard, Andrew Cowie, Bernardino Romera-Paredes, Stanislav Nikolov, Rishub Jain, Demis Hassabis
Authors and Affiliations
DeepMind, London, UK
John Jumper, Richard Evans, Alexander Pritzel, Tim Green, Michael Figurnov, Olaf Ronneberger, Kathryn Tunyasuvunakool, Russ Bates, Augustin Žídek, Anna Potapenko, Alex Bridgland, Clemens Meyer, Simon A. A. Kohl, Andrew J. Ballard, Andrew Cowie, Bernardino Romera-Paredes, Stanislav Nikolov, Rishub Jain, Jonas Adler, Trevor Back, Stig Petersen, David Reiman, Ellen Clancy, Michal Zielinski, Michalina Pacholska, Tamas Berghammer, Sebastian Bodenstein, David Silver, Oriol Vinyals, Andrew W. Senior, Koray Kavukcuoglu, Pushmeet Kohli & Demis Hassabis
School of Biological Sciences, Seoul National University, Seoul, South Korea
Martin Steinegger
Artificial Intelligence Institute, Seoul National University, Seoul, South Korea
You can also search for this author in PubMed Google Scholar
Contributions
J.J. and D.H. led the research. J.J., R.E., A. Pritzel, M.F., O.R., R.B., A. Potapenko, S.A.A.K., B.R.-P., J.A., M.P., T. Berghammer and O.V. developed the neural network architecture and training. T.G., A.Ž., K.T., R.B., A.B., R.E., A.J.B., A.C., S.N., R.J., D.R., M.Z. and S.B. developed the data, analytics and inference systems. D.H., K.K., P.K., C.M. and E.C. managed the research. T.G. led the technical platform. P.K., A.W.S., K.K., O.V., D.S., S.P. and T. Back contributed technical advice and ideas. M.S. created the BFD genomics database and provided technical assistance on HHBlits. D.H., R.E., A.W.S. and K.K. conceived the AlphaFold project. J.J., R.E. and A.W.S. conceived the end-to-end approach. J.J., A. Pritzel, O.R., A. Potapenko, R.E., M.F., T.G., K.T., C.M. and D.H. wrote the paper.
Corresponding authors
Correspondence to John Jumper or Demis Hassabis .
Ethics declarations
Competing interests.
J.J., R.E., A. Pritzel, T.G., M.F., O.R., R.B., A.B., S.A.A.K., D.R. and A.W.S. have filed non-provisional patent applications 16/701,070 and PCT/EP2020/084238, and provisional patent applications 63/107,362, 63/118,917, 63/118,918, 63/118,921 and 63/118,919, each in the name of DeepMind Technologies Limited, each pending, relating to machine learning for predicting protein structures. The other authors declare no competing interests.
Additional information
Peer review information Nature thanks Mohammed AlQuraishi, Charlotte Deane and Yang Zhang for their contribution to the peer review of this work.
Publisher’s note Springer Nature remains neutral with regard to jurisdictional claims in published maps and institutional affiliations.
Supplementary information
Supplementary information.
Description of the method details of the AlphaFold system, model, and analysis, including data pipeline, datasets, model blocks, loss functions, training and inference details, and ablations. Includes Supplementary Methods, Supplementary Figures, Supplementary Tables and Supplementary Algorithms.
Reporting Summary
Supplementary video 1.
Video of the intermediate structure trajectory of the CASP14 target T1024 (LmrP) A two-domain target (408 residues). Both domains are folded early, while their packing is adjusted for a longer time.
Supplementary Video 2
Video of the intermediate structure trajectory of the CASP14 target T1044 (RNA polymerase of crAss-like phage). A large protein (2180 residues), with multiple domains. Some domains are folded quickly, while others take a considerable amount of time to fold.
Supplementary Video 3
Video of the intermediate structure trajectory of the CASP14 target T1064 (Orf8). A very difficult single-domain target (106 residues) that takes the entire depth of the network to fold.
Supplementary Video 4
Video of the intermediate structure trajectory of the CASP14 target T1091. A multi-domain target (863 residues). Individual domains’ structure is determined early, while the domain packing evolves throughout the network. The network is exploring unphysical configurations throughout the process, resulting in long ‘strings’ in the visualization.
Rights and permissions
Open Access This article is licensed under a Creative Commons Attribution 4.0 International License, which permits use, sharing, adaptation, distribution and reproduction in any medium or format, as long as you give appropriate credit to the original author(s) and the source, provide a link to the Creative Commons license, and indicate if changes were made. The images or other third party material in this article are included in the article’s Creative Commons license, unless indicated otherwise in a credit line to the material. If material is not included in the article’s Creative Commons license and your intended use is not permitted by statutory regulation or exceeds the permitted use, you will need to obtain permission directly from the copyright holder. To view a copy of this license, visit http://creativecommons.org/licenses/by/4.0/ .
Reprints and permissions
About this article
Cite this article.
Jumper, J., Evans, R., Pritzel, A. et al. Highly accurate protein structure prediction with AlphaFold. Nature 596 , 583–589 (2021). https://doi.org/10.1038/s41586-021-03819-2
Download citation
Received : 11 May 2021
Accepted : 12 July 2021
Published : 15 July 2021
Issue Date : 26 August 2021
DOI : https://doi.org/10.1038/s41586-021-03819-2
Share this article
Anyone you share the following link with will be able to read this content:
Sorry, a shareable link is not currently available for this article.
Provided by the Springer Nature SharedIt content-sharing initiative
This article is cited by
Misfolded protein oligomers: mechanisms of formation, cytotoxic effects, and pharmacological approaches against protein misfolding diseases.
- Dillon J. Rinauro
- Fabrizio Chiti
- Ryan Limbocker
Molecular Neurodegeneration (2024)
Identification of Phytophthora cinnamomi CRN effectors and their roles in manipulating cell death during Persea americana infection
- Kayla A. Midgley
- Noëlani van den Berg
- Velushka Swart
BMC Genomics (2024)
Base-editing mutagenesis maps alleles to tune human T cell functions
- Ralf Schmidt
- Carl C. Ward
- Alexander Marson
Nature (2024)
Mammalian PIWI–piRNA–target complexes reveal features for broad and efficient target silencing
- Zhenzhen Li
- En-Zhi Shen
Nature Structural & Molecular Biology (2024)
Good reasons for structural biology
- Patrick Cramer
By submitting a comment you agree to abide by our Terms and Community Guidelines . If you find something abusive or that does not comply with our terms or guidelines please flag it as inappropriate.
Quick links
- Explore articles by subject
- Guide to authors
- Editorial policies
Sign up for the Nature Briefing newsletter — what matters in science, free to your inbox daily.


Producing novel liquid crystals by stacking antiaromatic units
Innovative molecular designs could pave the way to advances in organic semiconductors, optoelectronics, and other applied fields.
Whereas π-stacking systems involving aromatic compounds are quite common, those made from antiaromatic units are rare. In a recent study, researchers from Japan developed modified norcorrole molecules whose side chains favored the formation of columnar π-stacking structures. Using these compounds, they produced liquid crystals with high electrical conductivity and thermotropic properties. Their findings open up new design avenues for materials useful in electronics, sensing, optics, and biomedicine.
In organic chemistry, π-stacking systems are supramolecular structures that arise due to the dispersion force, a type of intermolecular noncovalent interaction. They are a common occurrence in nature; the stabilized structure of DNA is a very prominent example of a π-stacking system, and so are the arrangement of amino acids in certain proteins. Interestingly, π-stacking can be leveraged in the design of materials with useful electronic and optical properties. These include organic semiconductors of various kinds, as well as conjugated polymers for sensing and biomedical applications.
Thus far, a good portion of technologically relevant π-stacking system has been limited to aromatic compounds, which have inherent π-electron clouds. On the other hand, antiaromatic compounds, though promising candidates for developing electric conductors, have been scarcely reported as the building units of π-stacking systems.
Surprisingly, in a recent study, a research team led by Professor Hiromitsu Maeda from Ritsumeikan University, Japan, reported a novel antiaromatic π-stacking system that enabled the formation of a highly conductive liquid crystal. Their findings were published on April 16, 2024, in the journal Chemical Science . Worth noting, this paper was co-authored by Prof. Go Watanabe from Kitasato University, Prof. Shu Seki from Kyoto University, and Prof. Hiroshi Shinokubo from Nagoya University.
The reported compounds in question are Ni II -coordinated norcorroles with modified aryl moieties as side chains. Previously, achieving π-stacking in similar norcorroles failed because hydrogen-bonding interactions between the side chains opposed the face-to-face stacking of the planar antiaromatic units. This time, however, the research team had an ingenious idea. "We hypothesized that the introduction of side interacting moieties with less directionality would enhance the stacking between norcorrole units," explains Prof. Maeda. "Thus, we attempted the simple introduction of aliphatic chains, which induce van der Waals interactions. These interactions can be effective for modulating the stacking structure of a material," adds Prof. Maeda.
As evidenced through various experiments and molecular dynamics simulations, the proposed strategy worked as intended. The norcorrole units formed columnar structures through the stacking of arrangements known as 'triple-decker.' In these arrangements, a planarized molecule is sandwiched between two slightly bowl-shaped molecules.
Using the proposed molecular design, the researchers then synthesized liquid crystals. Thanks to the triple-decker stacking, a liquid crystal exhibited remarkable electricconductivity as well as thermotropicity; that is, an order parameter that depends on temperature. "The control of molecular interactions based on molecular design and synthesis, as demonstrated in our study, will be crucial for future applications," remarks Prof. Maeda. "Properties such as high electric conductivity in liquid crystals may be used for the fabrication of electronic devices. In addition, stimuli-responsive behaviors in soft materials can be used to modulate relevant properties, like photoluminescence, according to pressure and temperature," explains Prof. Maeda.
Taken together, the findings of this study bring to light a promising strategy for designing new compounds based on molecular assemblies of antiaromatic units. With any luck, this will open up new avenues for materials design, ultimately leading to better organic electronics, optoelectronics, and sensing devices.
- Nature of Water
- Materials Science
- Organic Chemistry
- Engineering and Construction
- Spintronics Research
- Computer Modeling
- Mobile Computing
- Pyroelectricity
- Electrical engineering
- Carbon nanotube
- Materials science
- Semiconductor
- Organic chemistry
- Phase (matter)
Story Source:
Materials provided by Ritsumeikan University . Note: Content may be edited for style and length.
Journal Reference :
- Soh Ishikawa, Kazuhisa Yamasumi, Shinya Sugiura, Shunsuke Sato, Go Watanabe, Yun Hee Koo, Shu Seki, Yuya Bando, Yohei Haketa, Hiroshi Shinokubo, Hiromitsu Maeda. Norcorroles as antiaromatic π-electronic systems that form dimension-controlled assemblies . Chemical Science , 2024; 15 (20): 7603 DOI: 10.1039/D4SC01633E
Cite This Page :
Explore More
- Charge Your Laptop in a Minute?
- Caterpillars Detect Predators by Electricity
- 'Electronic Spider Silk' Printed On Human Skin
- Engineered Surfaces Made to Shed Heat
- Innovative Material for Sustainable Building
- Human Brain: New Gene Transcripts
- Epstein-Barr Virus and Resulting Diseases
- Origins of the Proton's Spin
- Symbiotic Bacteria Communicate With Plants
- Birdsong and Human Voice: Same Genetic Blueprint
Trending Topics
Strange & offbeat.
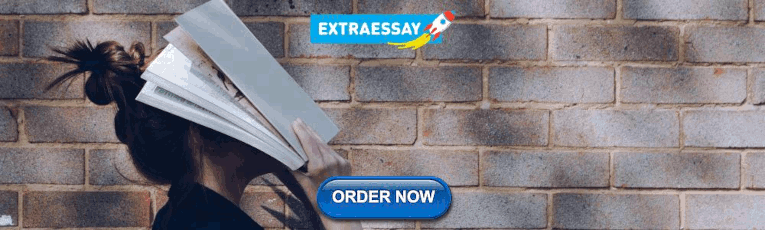
IMAGES
VIDEO
COMMENTS
PDF | On Apr 18, 2018, Ramesh Duraisamy published Atomic Structure & Basic Concepts of Chemistry | Find, read and cite all the research you need on ResearchGate
Atomic and molecular physics it the study of the properties, dynamics and interactions of the basic (but not fundamental) building blocks of matter. ... Electronic structure of atoms and molecules ...
Figure 2.2.1 2.2. 1: The Structure of the Atom. Atoms have protons and neutrons in the center, making the nucleus, while the electrons orbit the nucleus. The modern atomic theory states that atoms of one element are the same, while atoms of different elements are different.
The distribution of representations of the atomic structure in tasks 1, 2a and 2b is presented in Table 4. When students represented atomic structure independent of any context (task 1), the nuclear and Bohr's models (categories C and D) were the most frequent, whereas the quantum mechanical model (category E) appeared only in 1.9% of the ...
Atoms. Atoms is an international, peer-reviewed, open access journal on all aspects of the atom published monthly online by MDPI. Open Access — free for readers, with article processing charges (APC) paid by authors or their institutions. High Visibility: indexed within Scopus, ESCI (Web of Science), Astrophysics Data System, Inspec, CAPlus ...
Feature papers represent the most advanced research with significant potential for high impact in the field. A Feature Paper should be a substantial original Article that involves several techniques or approaches, provides an outlook for future research directions and describes possible research applications. ... Accurate atomic structure and ...
Chemistry Education Research and Practice. ... Three essential concepts - models of atomic structure, effective core charge and principles of electrostatic force, and quantum mechanics descriptions - were identified that may explain students' failure to learn the necessary aspects of molecular geometry and polarity. ... Paper. Submitted ...
Atomic radius is an important periodic descriptor used in understanding a variety of physico-chemical and bio-chemical processes. Numerous scales are suggested to define atomic radii. The aim of the current study is to find out the most reliable and universal scale among different (experimental and theoretical) scales of radii. For this, we have used different types of radii to compute some ...
Progress in the macroscopic (or, ab initio) theory has been quite notable and it has been supported by two major pillars: First, thanks to the advent of Effective Field Theories (EFTs), we can now systematically develop nuclear Hamiltonians that are rooted in the fundamental properties and symmetries of the underlying theory of QCD. Second, advances in computational resources and novel ...
Atomic electron tomography (AET) allows researchers to resolve the 3D atomic positions of crystal defects and amorphous materials4. In our study, we used it to determine the 3D atomic structure of ten
The purpose of this paper is to argue that history and philosophy of chemistry and physics are central strategies in the teaching of atomic and molecular structure, from the Dalton model (for an earlier approach see Chalmers 1998) to modern quantum mechanics and quantum chemistry.Therefore, in addition to the presentation and conclusions, the chapter is divided into two equally important sections.
Three important kinds of radiation are α particles (helium nuclei), β particles (electrons traveling at high speed), and γ rays (similar to x-rays but higher in energy). 2.2: The Discovery of Atomic Structure is shared under a license and was authored, remixed, and/or curated by LibreTexts. Atoms, the smallest particles of an element that ...
Protons and neutrons reside in the atomic nucleus and account for almost all of the mass of the atom. The number of protons present in an atom's nucleus, its atomic number, determines the identity of that atom as an element. The elements are numbered and arranged on the periodic table by their atomic numbers.
Atomic-scale observation of the grain-boundary structure of Yb-doped and heat-treated silicon nitride ceramics. The effect of secondary sintering additives and/or a post-sintering heat treatment on the semicrystalline atomic structure of the intergranular phase in silicon nitride ceramics is investigated.
atom, the basic building block of all matter and chemistry. Atoms can combine with other atoms to form molecules but cannot be divided into smaller parts by ordinary chemical processes. Most of the atom is empty space. The rest consists of three basic types of subatomic particles: protons, neutrons, and electrons.
History of atomic theory. The current theoretical model of the atom involves a dense nucleus surrounded by a probabilistic "cloud" of electrons. Atomic theory is the scientific theory that matter is composed of particles called atoms. The definition of the word "atom" has changed over the years in response to scientific discoveries.
The all-atom accuracy of AlphaFold was 1.5 Å r.m.s.d. 95 (95% confidence interval = 1.2-1.6 Å) compared with the 3.5 Å r.m.s.d. 95 (95% confidence interval = 3.1-4.2 Å) of the best ...
The emergence of atomic-level structure in language models shows a high-resolution picture of protein structure encoded by evolution into protein sequences that can be captured with unsupervised learning. Our current models are very far from the limit of scale in parameters, sequence data, and computing power that can in principle be applied.
Decent Essays. 465 Words. 2 Pages. Open Document. Atomic structure In the nucleus of an atom there are protons and neutrons the number of protons and neutrons depends on the element and ,if it is an isotope of that element. E.g. carbon 12, carbon 12 has six neutrons six protons and 6 electrons . Electrons are located around the nucleus of the atom.
Chicago. Ritsumeikan University. "Producing novel liquid crystals by stacking antiaromatic units." ScienceDaily. ScienceDaily, 23 May 2024. <www.sciencedaily.com / releases / 2024 / 05 ...