7.3 Problem Solving
Learning objectives.
By the end of this section, you will be able to:
- Describe problem solving strategies
- Define algorithm and heuristic
- Explain some common roadblocks to effective problem solving and decision making
People face problems every day—usually, multiple problems throughout the day. Sometimes these problems are straightforward: To double a recipe for pizza dough, for example, all that is required is that each ingredient in the recipe be doubled. Sometimes, however, the problems we encounter are more complex. For example, say you have a work deadline, and you must mail a printed copy of a report to your supervisor by the end of the business day. The report is time-sensitive and must be sent overnight. You finished the report last night, but your printer will not work today. What should you do? First, you need to identify the problem and then apply a strategy for solving the problem.
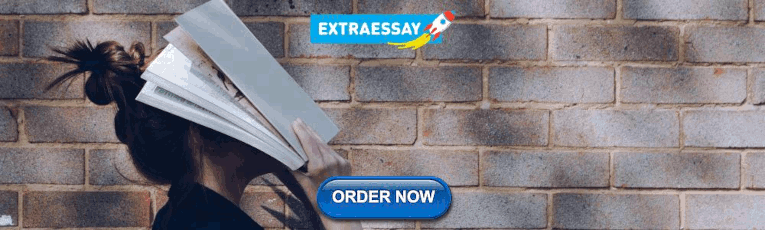
Problem-Solving Strategies
When you are presented with a problem—whether it is a complex mathematical problem or a broken printer, how do you solve it? Before finding a solution to the problem, the problem must first be clearly identified. After that, one of many problem solving strategies can be applied, hopefully resulting in a solution.
A problem-solving strategy is a plan of action used to find a solution. Different strategies have different action plans associated with them ( Table 7.2 ). For example, a well-known strategy is trial and error . The old adage, “If at first you don’t succeed, try, try again” describes trial and error. In terms of your broken printer, you could try checking the ink levels, and if that doesn’t work, you could check to make sure the paper tray isn’t jammed. Or maybe the printer isn’t actually connected to your laptop. When using trial and error, you would continue to try different solutions until you solved your problem. Although trial and error is not typically one of the most time-efficient strategies, it is a commonly used one.
Another type of strategy is an algorithm. An algorithm is a problem-solving formula that provides you with step-by-step instructions used to achieve a desired outcome (Kahneman, 2011). You can think of an algorithm as a recipe with highly detailed instructions that produce the same result every time they are performed. Algorithms are used frequently in our everyday lives, especially in computer science. When you run a search on the Internet, search engines like Google use algorithms to decide which entries will appear first in your list of results. Facebook also uses algorithms to decide which posts to display on your newsfeed. Can you identify other situations in which algorithms are used?
A heuristic is another type of problem solving strategy. While an algorithm must be followed exactly to produce a correct result, a heuristic is a general problem-solving framework (Tversky & Kahneman, 1974). You can think of these as mental shortcuts that are used to solve problems. A “rule of thumb” is an example of a heuristic. Such a rule saves the person time and energy when making a decision, but despite its time-saving characteristics, it is not always the best method for making a rational decision. Different types of heuristics are used in different types of situations, but the impulse to use a heuristic occurs when one of five conditions is met (Pratkanis, 1989):
- When one is faced with too much information
- When the time to make a decision is limited
- When the decision to be made is unimportant
- When there is access to very little information to use in making the decision
- When an appropriate heuristic happens to come to mind in the same moment
Working backwards is a useful heuristic in which you begin solving the problem by focusing on the end result. Consider this example: You live in Washington, D.C. and have been invited to a wedding at 4 PM on Saturday in Philadelphia. Knowing that Interstate 95 tends to back up any day of the week, you need to plan your route and time your departure accordingly. If you want to be at the wedding service by 3:30 PM, and it takes 2.5 hours to get to Philadelphia without traffic, what time should you leave your house? You use the working backwards heuristic to plan the events of your day on a regular basis, probably without even thinking about it.
Another useful heuristic is the practice of accomplishing a large goal or task by breaking it into a series of smaller steps. Students often use this common method to complete a large research project or long essay for school. For example, students typically brainstorm, develop a thesis or main topic, research the chosen topic, organize their information into an outline, write a rough draft, revise and edit the rough draft, develop a final draft, organize the references list, and proofread their work before turning in the project. The large task becomes less overwhelming when it is broken down into a series of small steps.
Everyday Connection
Solving puzzles.
Problem-solving abilities can improve with practice. Many people challenge themselves every day with puzzles and other mental exercises to sharpen their problem-solving skills. Sudoku puzzles appear daily in most newspapers. Typically, a sudoku puzzle is a 9×9 grid. The simple sudoku below ( Figure 7.7 ) is a 4×4 grid. To solve the puzzle, fill in the empty boxes with a single digit: 1, 2, 3, or 4. Here are the rules: The numbers must total 10 in each bolded box, each row, and each column; however, each digit can only appear once in a bolded box, row, and column. Time yourself as you solve this puzzle and compare your time with a classmate.
Here is another popular type of puzzle ( Figure 7.8 ) that challenges your spatial reasoning skills. Connect all nine dots with four connecting straight lines without lifting your pencil from the paper:
Take a look at the “Puzzling Scales” logic puzzle below ( Figure 7.9 ). Sam Loyd, a well-known puzzle master, created and refined countless puzzles throughout his lifetime (Cyclopedia of Puzzles, n.d.).
Pitfalls to Problem Solving
Not all problems are successfully solved, however. What challenges stop us from successfully solving a problem? Imagine a person in a room that has four doorways. One doorway that has always been open in the past is now locked. The person, accustomed to exiting the room by that particular doorway, keeps trying to get out through the same doorway even though the other three doorways are open. The person is stuck—but they just need to go to another doorway, instead of trying to get out through the locked doorway. A mental set is where you persist in approaching a problem in a way that has worked in the past but is clearly not working now.
Functional fixedness is a type of mental set where you cannot perceive an object being used for something other than what it was designed for. Duncker (1945) conducted foundational research on functional fixedness. He created an experiment in which participants were given a candle, a book of matches, and a box of thumbtacks. They were instructed to use those items to attach the candle to the wall so that it did not drip wax onto the table below. Participants had to use functional fixedness to overcome the problem ( Figure 7.10 ). During the Apollo 13 mission to the moon, NASA engineers at Mission Control had to overcome functional fixedness to save the lives of the astronauts aboard the spacecraft. An explosion in a module of the spacecraft damaged multiple systems. The astronauts were in danger of being poisoned by rising levels of carbon dioxide because of problems with the carbon dioxide filters. The engineers found a way for the astronauts to use spare plastic bags, tape, and air hoses to create a makeshift air filter, which saved the lives of the astronauts.
Link to Learning
Check out this Apollo 13 scene about NASA engineers overcoming functional fixedness to learn more.
Researchers have investigated whether functional fixedness is affected by culture. In one experiment, individuals from the Shuar group in Ecuador were asked to use an object for a purpose other than that for which the object was originally intended. For example, the participants were told a story about a bear and a rabbit that were separated by a river and asked to select among various objects, including a spoon, a cup, erasers, and so on, to help the animals. The spoon was the only object long enough to span the imaginary river, but if the spoon was presented in a way that reflected its normal usage, it took participants longer to choose the spoon to solve the problem. (German & Barrett, 2005). The researchers wanted to know if exposure to highly specialized tools, as occurs with individuals in industrialized nations, affects their ability to transcend functional fixedness. It was determined that functional fixedness is experienced in both industrialized and nonindustrialized cultures (German & Barrett, 2005).
In order to make good decisions, we use our knowledge and our reasoning. Often, this knowledge and reasoning is sound and solid. Sometimes, however, we are swayed by biases or by others manipulating a situation. For example, let’s say you and three friends wanted to rent a house and had a combined target budget of $1,600. The realtor shows you only very run-down houses for $1,600 and then shows you a very nice house for $2,000. Might you ask each person to pay more in rent to get the $2,000 home? Why would the realtor show you the run-down houses and the nice house? The realtor may be challenging your anchoring bias. An anchoring bias occurs when you focus on one piece of information when making a decision or solving a problem. In this case, you’re so focused on the amount of money you are willing to spend that you may not recognize what kinds of houses are available at that price point.
The confirmation bias is the tendency to focus on information that confirms your existing beliefs. For example, if you think that your professor is not very nice, you notice all of the instances of rude behavior exhibited by the professor while ignoring the countless pleasant interactions he is involved in on a daily basis. Hindsight bias leads you to believe that the event you just experienced was predictable, even though it really wasn’t. In other words, you knew all along that things would turn out the way they did. Representative bias describes a faulty way of thinking, in which you unintentionally stereotype someone or something; for example, you may assume that your professors spend their free time reading books and engaging in intellectual conversation, because the idea of them spending their time playing volleyball or visiting an amusement park does not fit in with your stereotypes of professors.
Finally, the availability heuristic is a heuristic in which you make a decision based on an example, information, or recent experience that is that readily available to you, even though it may not be the best example to inform your decision . Biases tend to “preserve that which is already established—to maintain our preexisting knowledge, beliefs, attitudes, and hypotheses” (Aronson, 1995; Kahneman, 2011). These biases are summarized in Table 7.3 .
Watch this teacher-made music video about cognitive biases to learn more.
Were you able to determine how many marbles are needed to balance the scales in Figure 7.9 ? You need nine. Were you able to solve the problems in Figure 7.7 and Figure 7.8 ? Here are the answers ( Figure 7.11 ).
As an Amazon Associate we earn from qualifying purchases.
This book may not be used in the training of large language models or otherwise be ingested into large language models or generative AI offerings without OpenStax's permission.
Want to cite, share, or modify this book? This book uses the Creative Commons Attribution License and you must attribute OpenStax.
Access for free at https://openstax.org/books/psychology-2e/pages/1-introduction
- Authors: Rose M. Spielman, William J. Jenkins, Marilyn D. Lovett
- Publisher/website: OpenStax
- Book title: Psychology 2e
- Publication date: Apr 22, 2020
- Location: Houston, Texas
- Book URL: https://openstax.org/books/psychology-2e/pages/1-introduction
- Section URL: https://openstax.org/books/psychology-2e/pages/7-3-problem-solving
© Jan 6, 2024 OpenStax. Textbook content produced by OpenStax is licensed under a Creative Commons Attribution License . The OpenStax name, OpenStax logo, OpenStax book covers, OpenStax CNX name, and OpenStax CNX logo are not subject to the Creative Commons license and may not be reproduced without the prior and express written consent of Rice University.
- Search Menu
- Sign in through your institution
- Browse content in Arts and Humanities
- Browse content in Archaeology
- Anglo-Saxon and Medieval Archaeology
- Archaeological Methodology and Techniques
- Archaeology by Region
- Archaeology of Religion
- Archaeology of Trade and Exchange
- Biblical Archaeology
- Contemporary and Public Archaeology
- Environmental Archaeology
- Historical Archaeology
- History and Theory of Archaeology
- Industrial Archaeology
- Landscape Archaeology
- Mortuary Archaeology
- Prehistoric Archaeology
- Underwater Archaeology
- Zooarchaeology
- Browse content in Architecture
- Architectural Structure and Design
- History of Architecture
- Residential and Domestic Buildings
- Theory of Architecture
- Browse content in Art
- Art Subjects and Themes
- History of Art
- Industrial and Commercial Art
- Theory of Art
- Biographical Studies
- Byzantine Studies
- Browse content in Classical Studies
- Classical History
- Classical Philosophy
- Classical Mythology
- Classical Literature
- Classical Reception
- Classical Art and Architecture
- Classical Oratory and Rhetoric
- Greek and Roman Epigraphy
- Greek and Roman Law
- Greek and Roman Papyrology
- Greek and Roman Archaeology
- Late Antiquity
- Religion in the Ancient World
- Digital Humanities
- Browse content in History
- Colonialism and Imperialism
- Diplomatic History
- Environmental History
- Genealogy, Heraldry, Names, and Honours
- Genocide and Ethnic Cleansing
- Historical Geography
- History by Period
- History of Emotions
- History of Agriculture
- History of Education
- History of Gender and Sexuality
- Industrial History
- Intellectual History
- International History
- Labour History
- Legal and Constitutional History
- Local and Family History
- Maritime History
- Military History
- National Liberation and Post-Colonialism
- Oral History
- Political History
- Public History
- Regional and National History
- Revolutions and Rebellions
- Slavery and Abolition of Slavery
- Social and Cultural History
- Theory, Methods, and Historiography
- Urban History
- World History
- Browse content in Language Teaching and Learning
- Language Learning (Specific Skills)
- Language Teaching Theory and Methods
- Browse content in Linguistics
- Applied Linguistics
- Cognitive Linguistics
- Computational Linguistics
- Forensic Linguistics
- Grammar, Syntax and Morphology
- Historical and Diachronic Linguistics
- History of English
- Language Acquisition
- Language Evolution
- Language Reference
- Language Variation
- Language Families
- Lexicography
- Linguistic Anthropology
- Linguistic Theories
- Linguistic Typology
- Phonetics and Phonology
- Psycholinguistics
- Sociolinguistics
- Translation and Interpretation
- Writing Systems
- Browse content in Literature
- Bibliography
- Children's Literature Studies
- Literary Studies (Asian)
- Literary Studies (European)
- Literary Studies (Eco-criticism)
- Literary Studies (Romanticism)
- Literary Studies (American)
- Literary Studies (Modernism)
- Literary Studies - World
- Literary Studies (1500 to 1800)
- Literary Studies (19th Century)
- Literary Studies (20th Century onwards)
- Literary Studies (African American Literature)
- Literary Studies (British and Irish)
- Literary Studies (Early and Medieval)
- Literary Studies (Fiction, Novelists, and Prose Writers)
- Literary Studies (Gender Studies)
- Literary Studies (Graphic Novels)
- Literary Studies (History of the Book)
- Literary Studies (Plays and Playwrights)
- Literary Studies (Poetry and Poets)
- Literary Studies (Postcolonial Literature)
- Literary Studies (Queer Studies)
- Literary Studies (Science Fiction)
- Literary Studies (Travel Literature)
- Literary Studies (War Literature)
- Literary Studies (Women's Writing)
- Literary Theory and Cultural Studies
- Mythology and Folklore
- Shakespeare Studies and Criticism
- Browse content in Media Studies
- Browse content in Music
- Applied Music
- Dance and Music
- Ethics in Music
- Ethnomusicology
- Gender and Sexuality in Music
- Medicine and Music
- Music Cultures
- Music and Religion
- Music and Media
- Music and Culture
- Music Education and Pedagogy
- Music Theory and Analysis
- Musical Scores, Lyrics, and Libretti
- Musical Structures, Styles, and Techniques
- Musicology and Music History
- Performance Practice and Studies
- Race and Ethnicity in Music
- Sound Studies
- Browse content in Performing Arts
- Browse content in Philosophy
- Aesthetics and Philosophy of Art
- Epistemology
- Feminist Philosophy
- History of Western Philosophy
- Metaphysics
- Moral Philosophy
- Non-Western Philosophy
- Philosophy of Science
- Philosophy of Language
- Philosophy of Mind
- Philosophy of Perception
- Philosophy of Action
- Philosophy of Law
- Philosophy of Religion
- Philosophy of Mathematics and Logic
- Practical Ethics
- Social and Political Philosophy
- Browse content in Religion
- Biblical Studies
- Christianity
- East Asian Religions
- History of Religion
- Judaism and Jewish Studies
- Qumran Studies
- Religion and Education
- Religion and Health
- Religion and Politics
- Religion and Science
- Religion and Law
- Religion and Art, Literature, and Music
- Religious Studies
- Browse content in Society and Culture
- Cookery, Food, and Drink
- Cultural Studies
- Customs and Traditions
- Ethical Issues and Debates
- Hobbies, Games, Arts and Crafts
- Natural world, Country Life, and Pets
- Popular Beliefs and Controversial Knowledge
- Sports and Outdoor Recreation
- Technology and Society
- Travel and Holiday
- Visual Culture
- Browse content in Law
- Arbitration
- Browse content in Company and Commercial Law
- Commercial Law
- Company Law
- Browse content in Comparative Law
- Systems of Law
- Competition Law
- Browse content in Constitutional and Administrative Law
- Government Powers
- Judicial Review
- Local Government Law
- Military and Defence Law
- Parliamentary and Legislative Practice
- Construction Law
- Contract Law
- Browse content in Criminal Law
- Criminal Procedure
- Criminal Evidence Law
- Sentencing and Punishment
- Employment and Labour Law
- Environment and Energy Law
- Browse content in Financial Law
- Banking Law
- Insolvency Law
- History of Law
- Human Rights and Immigration
- Intellectual Property Law
- Browse content in International Law
- Private International Law and Conflict of Laws
- Public International Law
- IT and Communications Law
- Jurisprudence and Philosophy of Law
- Law and Politics
- Law and Society
- Browse content in Legal System and Practice
- Courts and Procedure
- Legal Skills and Practice
- Primary Sources of Law
- Regulation of Legal Profession
- Medical and Healthcare Law
- Browse content in Policing
- Criminal Investigation and Detection
- Police and Security Services
- Police Procedure and Law
- Police Regional Planning
- Browse content in Property Law
- Personal Property Law
- Study and Revision
- Terrorism and National Security Law
- Browse content in Trusts Law
- Wills and Probate or Succession
- Browse content in Medicine and Health
- Browse content in Allied Health Professions
- Arts Therapies
- Clinical Science
- Dietetics and Nutrition
- Occupational Therapy
- Operating Department Practice
- Physiotherapy
- Radiography
- Speech and Language Therapy
- Browse content in Anaesthetics
- General Anaesthesia
- Neuroanaesthesia
- Browse content in Clinical Medicine
- Acute Medicine
- Cardiovascular Medicine
- Clinical Genetics
- Clinical Pharmacology and Therapeutics
- Dermatology
- Endocrinology and Diabetes
- Gastroenterology
- Genito-urinary Medicine
- Geriatric Medicine
- Infectious Diseases
- Medical Toxicology
- Medical Oncology
- Pain Medicine
- Palliative Medicine
- Rehabilitation Medicine
- Respiratory Medicine and Pulmonology
- Rheumatology
- Sleep Medicine
- Sports and Exercise Medicine
- Clinical Neuroscience
- Community Medical Services
- Critical Care
- Emergency Medicine
- Forensic Medicine
- Haematology
- History of Medicine
- Browse content in Medical Dentistry
- Oral and Maxillofacial Surgery
- Paediatric Dentistry
- Restorative Dentistry and Orthodontics
- Surgical Dentistry
- Browse content in Medical Skills
- Clinical Skills
- Communication Skills
- Nursing Skills
- Surgical Skills
- Medical Ethics
- Medical Statistics and Methodology
- Browse content in Neurology
- Clinical Neurophysiology
- Neuropathology
- Nursing Studies
- Browse content in Obstetrics and Gynaecology
- Gynaecology
- Occupational Medicine
- Ophthalmology
- Otolaryngology (ENT)
- Browse content in Paediatrics
- Neonatology
- Browse content in Pathology
- Chemical Pathology
- Clinical Cytogenetics and Molecular Genetics
- Histopathology
- Medical Microbiology and Virology
- Patient Education and Information
- Browse content in Pharmacology
- Psychopharmacology
- Browse content in Popular Health
- Caring for Others
- Complementary and Alternative Medicine
- Self-help and Personal Development
- Browse content in Preclinical Medicine
- Cell Biology
- Molecular Biology and Genetics
- Reproduction, Growth and Development
- Primary Care
- Professional Development in Medicine
- Browse content in Psychiatry
- Addiction Medicine
- Child and Adolescent Psychiatry
- Forensic Psychiatry
- Learning Disabilities
- Old Age Psychiatry
- Psychotherapy
- Browse content in Public Health and Epidemiology
- Epidemiology
- Public Health
- Browse content in Radiology
- Clinical Radiology
- Interventional Radiology
- Nuclear Medicine
- Radiation Oncology
- Reproductive Medicine
- Browse content in Surgery
- Cardiothoracic Surgery
- Gastro-intestinal and Colorectal Surgery
- General Surgery
- Neurosurgery
- Paediatric Surgery
- Peri-operative Care
- Plastic and Reconstructive Surgery
- Surgical Oncology
- Transplant Surgery
- Trauma and Orthopaedic Surgery
- Vascular Surgery
- Browse content in Science and Mathematics
- Browse content in Biological Sciences
- Aquatic Biology
- Biochemistry
- Bioinformatics and Computational Biology
- Developmental Biology
- Ecology and Conservation
- Evolutionary Biology
- Genetics and Genomics
- Microbiology
- Molecular and Cell Biology
- Natural History
- Plant Sciences and Forestry
- Research Methods in Life Sciences
- Structural Biology
- Systems Biology
- Zoology and Animal Sciences
- Browse content in Chemistry
- Analytical Chemistry
- Computational Chemistry
- Crystallography
- Environmental Chemistry
- Industrial Chemistry
- Inorganic Chemistry
- Materials Chemistry
- Medicinal Chemistry
- Mineralogy and Gems
- Organic Chemistry
- Physical Chemistry
- Polymer Chemistry
- Study and Communication Skills in Chemistry
- Theoretical Chemistry
- Browse content in Computer Science
- Artificial Intelligence
- Computer Architecture and Logic Design
- Game Studies
- Human-Computer Interaction
- Mathematical Theory of Computation
- Programming Languages
- Software Engineering
- Systems Analysis and Design
- Virtual Reality
- Browse content in Computing
- Business Applications
- Computer Security
- Computer Games
- Computer Networking and Communications
- Digital Lifestyle
- Graphical and Digital Media Applications
- Operating Systems
- Browse content in Earth Sciences and Geography
- Atmospheric Sciences
- Environmental Geography
- Geology and the Lithosphere
- Maps and Map-making
- Meteorology and Climatology
- Oceanography and Hydrology
- Palaeontology
- Physical Geography and Topography
- Regional Geography
- Soil Science
- Urban Geography
- Browse content in Engineering and Technology
- Agriculture and Farming
- Biological Engineering
- Civil Engineering, Surveying, and Building
- Electronics and Communications Engineering
- Energy Technology
- Engineering (General)
- Environmental Science, Engineering, and Technology
- History of Engineering and Technology
- Mechanical Engineering and Materials
- Technology of Industrial Chemistry
- Transport Technology and Trades
- Browse content in Environmental Science
- Applied Ecology (Environmental Science)
- Conservation of the Environment (Environmental Science)
- Environmental Sustainability
- Environmentalist Thought and Ideology (Environmental Science)
- Management of Land and Natural Resources (Environmental Science)
- Natural Disasters (Environmental Science)
- Nuclear Issues (Environmental Science)
- Pollution and Threats to the Environment (Environmental Science)
- Social Impact of Environmental Issues (Environmental Science)
- History of Science and Technology
- Browse content in Materials Science
- Ceramics and Glasses
- Composite Materials
- Metals, Alloying, and Corrosion
- Nanotechnology
- Browse content in Mathematics
- Applied Mathematics
- Biomathematics and Statistics
- History of Mathematics
- Mathematical Education
- Mathematical Finance
- Mathematical Analysis
- Numerical and Computational Mathematics
- Probability and Statistics
- Pure Mathematics
- Browse content in Neuroscience
- Cognition and Behavioural Neuroscience
- Development of the Nervous System
- Disorders of the Nervous System
- History of Neuroscience
- Invertebrate Neurobiology
- Molecular and Cellular Systems
- Neuroendocrinology and Autonomic Nervous System
- Neuroscientific Techniques
- Sensory and Motor Systems
- Browse content in Physics
- Astronomy and Astrophysics
- Atomic, Molecular, and Optical Physics
- Biological and Medical Physics
- Classical Mechanics
- Computational Physics
- Condensed Matter Physics
- Electromagnetism, Optics, and Acoustics
- History of Physics
- Mathematical and Statistical Physics
- Measurement Science
- Nuclear Physics
- Particles and Fields
- Plasma Physics
- Quantum Physics
- Relativity and Gravitation
- Semiconductor and Mesoscopic Physics
- Browse content in Psychology
- Affective Sciences
- Clinical Psychology
- Cognitive Psychology
- Cognitive Neuroscience
- Criminal and Forensic Psychology
- Developmental Psychology
- Educational Psychology
- Evolutionary Psychology
- Health Psychology
- History and Systems in Psychology
- Music Psychology
- Neuropsychology
- Organizational Psychology
- Psychological Assessment and Testing
- Psychology of Human-Technology Interaction
- Psychology Professional Development and Training
- Research Methods in Psychology
- Social Psychology
- Browse content in Social Sciences
- Browse content in Anthropology
- Anthropology of Religion
- Human Evolution
- Medical Anthropology
- Physical Anthropology
- Regional Anthropology
- Social and Cultural Anthropology
- Theory and Practice of Anthropology
- Browse content in Business and Management
- Business Strategy
- Business Ethics
- Business History
- Business and Government
- Business and Technology
- Business and the Environment
- Comparative Management
- Corporate Governance
- Corporate Social Responsibility
- Entrepreneurship
- Health Management
- Human Resource Management
- Industrial and Employment Relations
- Industry Studies
- Information and Communication Technologies
- International Business
- Knowledge Management
- Management and Management Techniques
- Operations Management
- Organizational Theory and Behaviour
- Pensions and Pension Management
- Public and Nonprofit Management
- Strategic Management
- Supply Chain Management
- Browse content in Criminology and Criminal Justice
- Criminal Justice
- Criminology
- Forms of Crime
- International and Comparative Criminology
- Youth Violence and Juvenile Justice
- Development Studies
- Browse content in Economics
- Agricultural, Environmental, and Natural Resource Economics
- Asian Economics
- Behavioural Finance
- Behavioural Economics and Neuroeconomics
- Econometrics and Mathematical Economics
- Economic Systems
- Economic History
- Economic Methodology
- Economic Development and Growth
- Financial Markets
- Financial Institutions and Services
- General Economics and Teaching
- Health, Education, and Welfare
- History of Economic Thought
- International Economics
- Labour and Demographic Economics
- Law and Economics
- Macroeconomics and Monetary Economics
- Microeconomics
- Public Economics
- Urban, Rural, and Regional Economics
- Welfare Economics
- Browse content in Education
- Adult Education and Continuous Learning
- Care and Counselling of Students
- Early Childhood and Elementary Education
- Educational Equipment and Technology
- Educational Strategies and Policy
- Higher and Further Education
- Organization and Management of Education
- Philosophy and Theory of Education
- Schools Studies
- Secondary Education
- Teaching of a Specific Subject
- Teaching of Specific Groups and Special Educational Needs
- Teaching Skills and Techniques
- Browse content in Environment
- Applied Ecology (Social Science)
- Climate Change
- Conservation of the Environment (Social Science)
- Environmentalist Thought and Ideology (Social Science)
- Natural Disasters (Environment)
- Social Impact of Environmental Issues (Social Science)
- Browse content in Human Geography
- Cultural Geography
- Economic Geography
- Political Geography
- Browse content in Interdisciplinary Studies
- Communication Studies
- Museums, Libraries, and Information Sciences
- Browse content in Politics
- African Politics
- Asian Politics
- Chinese Politics
- Comparative Politics
- Conflict Politics
- Elections and Electoral Studies
- Environmental Politics
- European Union
- Foreign Policy
- Gender and Politics
- Human Rights and Politics
- Indian Politics
- International Relations
- International Organization (Politics)
- International Political Economy
- Irish Politics
- Latin American Politics
- Middle Eastern Politics
- Political Methodology
- Political Communication
- Political Philosophy
- Political Sociology
- Political Behaviour
- Political Economy
- Political Institutions
- Political Theory
- Politics and Law
- Politics of Development
- Public Administration
- Public Policy
- Quantitative Political Methodology
- Regional Political Studies
- Russian Politics
- Security Studies
- State and Local Government
- UK Politics
- US Politics
- Browse content in Regional and Area Studies
- African Studies
- Asian Studies
- East Asian Studies
- Japanese Studies
- Latin American Studies
- Middle Eastern Studies
- Native American Studies
- Scottish Studies
- Browse content in Research and Information
- Research Methods
- Browse content in Social Work
- Addictions and Substance Misuse
- Adoption and Fostering
- Care of the Elderly
- Child and Adolescent Social Work
- Couple and Family Social Work
- Direct Practice and Clinical Social Work
- Emergency Services
- Human Behaviour and the Social Environment
- International and Global Issues in Social Work
- Mental and Behavioural Health
- Social Justice and Human Rights
- Social Policy and Advocacy
- Social Work and Crime and Justice
- Social Work Macro Practice
- Social Work Practice Settings
- Social Work Research and Evidence-based Practice
- Welfare and Benefit Systems
- Browse content in Sociology
- Childhood Studies
- Community Development
- Comparative and Historical Sociology
- Economic Sociology
- Gender and Sexuality
- Gerontology and Ageing
- Health, Illness, and Medicine
- Marriage and the Family
- Migration Studies
- Occupations, Professions, and Work
- Organizations
- Population and Demography
- Race and Ethnicity
- Social Theory
- Social Movements and Social Change
- Social Research and Statistics
- Social Stratification, Inequality, and Mobility
- Sociology of Religion
- Sociology of Education
- Sport and Leisure
- Urban and Rural Studies
- Browse content in Warfare and Defence
- Defence Strategy, Planning, and Research
- Land Forces and Warfare
- Military Administration
- Military Life and Institutions
- Naval Forces and Warfare
- Other Warfare and Defence Issues
- Peace Studies and Conflict Resolution
- Weapons and Equipment

- < Previous chapter
- Next chapter >
21 Problem Solving
Miriam Bassok, Department of Psychology, University of Washington, Seattle, WA
Laura R. Novick, Department of Psychology and Human Development, Vanderbilt University, Nashville, TN
- Published: 21 November 2012
- Cite Icon Cite
- Permissions Icon Permissions
This chapter follows the historical development of research on problem solving. It begins with a description of two research traditions that addressed different aspects of the problem-solving process: ( 1 ) research on problem representation (the Gestalt legacy) that examined how people understand the problem at hand, and ( 2 ) research on search in a problem space (the legacy of Newell and Simon) that examined how people generate the problem's solution. It then describes some developments in the field that fueled the integration of these two lines of research: work on problem isomorphs, on expertise in specific knowledge domains (e.g., chess, mathematics), and on insight solutions. Next, it presents examples of recent work on problem solving in science and mathematics that highlight the impact of visual perception and background knowledge on how people represent problems and search for problem solutions. The final section considers possible directions for future research.
People are confronted with problems on a daily basis, be it trying to extract a broken light bulb from a socket, finding a detour when the regular route is blocked, fixing dinner for unexpected guests, dealing with a medical emergency, or deciding what house to buy. Obviously, the problems people encounter differ in many ways, and their solutions require different types of knowledge and skills. Yet we have a sense that all the situations we classify as problems share a common core. Karl Duncker defined this core as follows: “A problem arises when a living creature has a goal but does not know how this goal is to be reached. Whenever one cannot go from the given situation to the desired situation simply by action [i.e., by the performance of obvious operations], then there has to be recourse to thinking” (Duncker, 1945 , p. 1). Consider the broken light bulb. The obvious operation—holding the glass part of the bulb with one's fingers while unscrewing the base from the socket—is prevented by the fact that the glass is broken. Thus, there must be “recourse to thinking” about possible ways to solve the problem. For example, one might try mounting half a potato on the broken bulb (we do not know the source of this creative solution, which is described on many “how to” Web sites).
The above definition and examples make it clear that what constitutes a problem for one person may not be a problem for another person, or for that same person at another point in time. For example, the second time one has to remove a broken light bulb from a socket, the solution likely can be retrieved from memory; there is no problem. Similarly, tying shoes may be considered a problem for 5-year-olds but not for readers of this chapter. And, of course, people may change their goal and either no longer have a problem (e.g., take the guests to a restaurant instead of fixing dinner) or attempt to solve a different problem (e.g., decide what restaurant to go to). Given the highly subjective nature of what constitutes a problem, researchers who study problem solving have often presented people with novel problems that they should be capable of solving and attempted to find regularities in the resulting problem-solving behavior. Despite the variety of possible problem situations, researchers have identified important regularities in the thinking processes by which people (a) represent , or understand, problem situations and (b) search for possible ways to get to their goal.
A problem representation is a model constructed by the solver that summarizes his or her understanding of the problem components—the initial state (e.g., a broken light bulb in a socket), the goal state (the light bulb extracted), and the set of possible operators one may apply to get from the initial state to the goal state (e.g., use pliers). According to Reitman ( 1965 ), problem components differ in the extent to which they are well defined . Some components leave little room for interpretation (e.g., the initial state in the broken light bulb example is relatively well defined), whereas other components may be ill defined and have to be defined by the solver (e.g., the possible actions one may take to extract the broken bulb). The solver's representation of the problem guides the search for a possible solution (e.g., possible attempts at extracting the light bulb). This search may, in turn, change the representation of the problem (e.g., finding that the goal cannot be achieved using pliers) and lead to a new search. Such a recursive process of representation and search continues until the problem is solved or until the solver decides to abort the goal.
Duncker ( 1945 , pp. 28–37) documented the interplay between representation and search based on his careful analysis of one person's solution to the “Radiation Problem” (later to be used extensively in research analogy, see Holyoak, Chapter 13 ). This problem requires using some rays to destroy a patient's stomach tumor without harming the patient. At sufficiently high intensity, the rays will destroy the tumor. However, at that intensity, they will also destroy the healthy tissue surrounding the tumor. At lower intensity, the rays will not harm the healthy tissue, but they also will not destroy the tumor. Duncker's analysis revealed that the solver's solution attempts were guided by three distinct problem representations. He depicted these solution attempts as an inverted search tree in which the three main branches correspond to the three general problem representations (Duncker, 1945 , p. 32). We reproduce this diagram in Figure 21.1 . The desired solution appears on the rightmost branch of the tree, within the general problem representation in which the solver aims to “lower the intensity of the rays on their way through healthy tissue.” The actual solution is to project multiple low-intensity rays at the tumor from several points around the patient “by use of lens.” The low-intensity rays will converge on the tumor, where their individual intensities will sum to a level sufficient to destroy the tumor.
A search-tree representation of one subject's solution to the radiation problem, reproduced from Duncker ( 1945 , p. 32).
Although there are inherent interactions between representation and search, some researchers focus their efforts on understanding the factors that affect how solvers represent problems, whereas others look for regularities in how they search for a solution within a particular representation. Based on their main focus of interest, researchers devise or select problems with solutions that mainly require either constructing a particular representation or finding the appropriate sequence of steps leading from the initial state to the goal state. In most cases, researchers who are interested in problem representation select problems in which one or more of the components are ill defined, whereas those who are interested in search select problems in which the components are well defined. The following examples illustrate, respectively, these two problem types.
The Bird-and-Trains problem (Posner, 1973 , pp. 150–151) is a mathematical word problem that tends to elicit two distinct problem representations (see Fig. 21.2a and b ):
Two train stations are 50 miles apart. At 2 p.m. one Saturday afternoon two trains start toward each other, one from each station. Just as the trains pull out of the stations, a bird springs into the air in front of the first train and flies ahead to the front of the second train. When the bird reaches the second train, it turns back and flies toward the first train. The bird continues to do this until the trains meet. If both trains travel at the rate of 25 miles per hour and the bird flies at 100 miles per hour, how many miles will the bird have flown before the trains meet? Fig. 21.2 Open in new tab Download slide Alternative representations of Posner's ( 1973 ) trains-and-bird problem. Adapted from Novick and Hmelo ( 1994 ).
Some solvers focus on the back-and-forth path of the bird (Fig. 21.2a ). This representation yields a problem that would be difficult for most people to solve (e.g., a series of differential equations). Other solvers focus on the paths of the trains (Fig. 21.2b ), a representation that yields a relatively easy distance-rate-time problem.
The Tower of Hanoi problem falls on the other end of the representation-search continuum. It leaves little room for differences in problem representations, and the primary work is to discover a solution path (or the best solution path) from the initial state to the goal state .
There are three pegs mounted on a base. On the leftmost peg, there are three disks of differing sizes. The disks are arranged in order of size with the largest disk on the bottom and the smallest disk on the top. The disks may be moved one at a time, but only the top disk on a peg may be moved, and at no time may a larger disk be placed on a smaller disk. The goal is to move the three-disk tower from the leftmost peg to the rightmost peg.
Figure 21.3 shows all the possible legal arrangements of disks on pegs. The arrows indicate transitions between states that result from moving a single disk, with the thicker gray arrows indicating the shortest path that connects the initial state to the goal state.
The division of labor between research on representation versus search has distinct historical antecedents and research traditions. In the next two sections, we review the main findings from these two historical traditions. Then, we describe some developments in the field that fueled the integration of these lines of research—work on problem isomorphs, on expertise in specific knowledge domains (e.g., chess, mathematics), and on insight solutions. In the fifth section, we present some examples of recent work on problem solving in science and mathematics. This work highlights the role of visual perception and background knowledge in the way people represent problems and search for problem solutions. In the final section, we consider possible directions for future research.
Our review is by no means an exhaustive one. It follows the historical development of the field and highlights findings that pertain to a wide variety of problems. Research pertaining to specific types of problems (e.g., medical problems), specific processes that are involved in problem solving (e.g., analogical inferences), and developmental changes in problem solving due to learning and maturation may be found elsewhere in this volume (e.g., Holyoak, Chapter 13 ; Smith & Ward, Chapter 23 ; van Steenburgh et al., Chapter 24 ; Simonton, Chapter 25 ; Opfer & Siegler, Chapter 30 ; Hegarty & Stull, Chapter 31 ; Dunbar & Klahr, Chapter 35 ; Patel et al., Chapter 37 ; Lowenstein, Chapter 38 ; Koedinger & Roll, Chapter 40 ).
All possible problem states for the three-disk Tower of Hanoi problem. The thicker gray arrows show the optimum solution path connecting the initial state (State #1) to the goal state (State #27).
Problem Representation: The Gestalt Legacy
Research on problem representation has its origins in Gestalt psychology, an influential approach in European psychology during the first half of the 20th century. (Behaviorism was the dominant perspective in American psychology at this time.) Karl Duncker published a book on the topic in his native German in 1935, which was translated into English and published 10 years later as the monograph On Problem-Solving (Duncker, 1945 ). Max Wertheimer also published a book on the topic in 1945, titled Productive Thinking . An enlarged edition published posthumously includes previously unpublished material (Wertheimer, 1959 ). Interestingly, 1945 seems to have been a watershed year for problem solving, as mathematician George Polya's book, How to Solve It , also appeared then (a second edition was published 12 years later; Polya, 1957 ).
The Gestalt psychologists extended the organizational principles of visual perception to the domain of problem solving. They showed that various visual aspects of the problem, as well the solver's prior knowledge, affect how people understand problems and, therefore, generate problem solutions. The principles of visual perception (e.g., proximity, closure, grouping, good continuation) are directly relevant to problem solving when the physical layout of the problem, or a diagram that accompanies the problem description, elicits inferences that solvers include in their problem representations. Such effects are nicely illustrated by Maier's ( 1930 ) nine-dot problem: Nine dots are arrayed in a 3x3 grid, and the task is to connect all the dots by drawing four straight lines without lifting one's pencil from the paper. People have difficulty solving this problem because their initial representations generally include a constraint, inferred from the configuration of the dots, that the lines should not go outside the boundary of the imaginary square formed by the outer dots. With this constraint, the problem cannot be solved (but see Adams, 1979 ). Without this constraint, the problem may be solved as shown in Figure 21.4 (though the problem is still difficult for many people; see Weisberg & Alba, 1981 ).
The nine-dot problem is a classic insight problem (see van Steenburgh et al., Chapter 24 ). According to the Gestalt view (e.g., Duncker, 1945 ; Kohler, 1925 ; Maier, 1931 ; see Ohlsson, 1984 , for a review), the solution to an insight problem appears suddenly, accompanied by an “aha!” sensation, immediately following the sudden “restructuring” of one's understanding of the problem (i.e., a change in the problem representation): “The decisive points in thought-processes, the moments of sudden comprehension, of the ‘Aha!,’ of the new, are always at the same time moments in which such a sudden restructuring of the thought-material takes place” (Duncker, 1945 , p. 29). For the nine-dot problem, one view of the required restructuring is that the solver relaxes the constraint implied by the perceptual form of the problem and realizes that the lines may, in fact, extend past the boundary of the imaginary square. Later in the chapter, we present more recent accounts of insight.
The entities that appear in a problem also tend to evoke various inferences that people incorporate into their problem representations. A classic demonstration of this is the phenomenon of functional fixedness , introduced by Duncker ( 1945 ): If an object is habitually used for a certain purpose (e.g., a box serves as a container), it is difficult to see
A solution to the nine-dot problem.
that object as having properties that would enable it to be used for a dissimilar purpose. Duncker's basic experimental paradigm involved two conditions that varied in terms of whether the object that was crucial for solution was initially used for a function other than that required for solution.
Consider the candles problem—the best known of the five “practical problems” Duncker ( 1945 ) investigated. Three candles are to be mounted at eye height on a door. On the table, for use in completing this task, are some tacks and three boxes. The solution is to tack the three boxes to the door to serve as platforms for the candles. In the control condition, the three boxes were presented to subjects empty. In the functional-fixedness condition, they were filled with candles, tacks, and matches. Thus, in the latter condition, the boxes initially served the function of container, whereas the solution requires that they serve the function of platform. The results showed that 100% of the subjects who received empty boxes solved the candles problem, compared with only 43% of subjects who received filled boxes. Every one of the five problems in this study showed a difference favoring the control condition over the functional-fixedness condition, with average solution rates across the five problems of 97% and 58%, respectively.
The function of the objects in a problem can be also “fixed” by their most recent use. For example, Birch and Rabinowitz ( 1951 ) had subjects perform two consecutive tasks. In the first task, people had to use either a switch or a relay to form an electric circuit. After completing this task, both groups of subjects were asked to solve Maier's ( 1931 ) two-ropes problem. The solution to this problem requires tying an object to one of the ropes and making the rope swing as a pendulum. Subjects could create the pendulum using either the object from the electric-circuit task or the other object. Birch and Rabinowitz found that subjects avoided using the same object for two unrelated functions. That is, those who used the switch in the first task made the pendulum using the relay, and vice versa. The explanations subjects subsequently gave for their object choices revealed that they were unaware of the functional-fixedness constraint they imposed on themselves.
In addition to investigating people's solutions to such practical problems as irradiating a tumor, mounting candles on the wall, or tying ropes, the Gestalt psychologists examined how people understand and solve mathematical problems that require domain-specific knowledge. For example, Wertheimer ( 1959 ) observed individual differences in students' learning and subsequent application of the formula for finding the area of a parallelogram (see Fig. 21.5a ). Some students understood the logic underlying the learned formula (i.e., the fact that a parallelogram can be transformed into a rectangle by cutting off a triangle from one side and pasting it onto the other side) and exhibited “productive thinking”—using the same logic to find the area of the quadrilateral in Figure 21.5b and the irregularly shaped geometric figure in Figure 21.5c . Other students memorized the formula and exhibited “reproductive thinking”—reproducing the learned solution only to novel parallelograms that were highly similar to the original one.
The psychological study of human problem solving faded into the background after the demise of the Gestalt tradition (during World War II), and problem solving was investigated only sporadically until Allen Newell and Herbert Simon's ( 1972 ) landmark book Human Problem Solving sparked a flurry of research on this topic. Newell and Simon adopted and refined Duncker's ( 1945 ) methodology of collecting and analyzing the think-aloud protocols that accompany problem solutions and extended Duncker's conceptualization of a problem solution as a search tree. However, their initial work did not aim to extend the Gestalt findings
Finding the area of ( a ) a parallelogram, ( b ) a quadrilateral, and ( c ) an irregularly shaped geometric figure. The solid lines indicate the geometric figures whose areas are desired. The dashed lines show how to convert the given figures into rectangles (i.e., they show solutions with understanding).
pertaining to problem representation. Instead, as we explain in the next section, their objective was to identify the general-purpose strategies people use in searching for a problem solution.
Search in a Problem Space: The Legacy of Newell and Simon
Newell and Simon ( 1972 ) wrote a magnum opus detailing their theory of problem solving and the supporting research they conducted with various collaborators. This theory was grounded in the information-processing approach to cognitive psychology and guided by an analogy between human and artificial intelligence (i.e., both people and computers being “Physical Symbol Systems,” Newell & Simon, 1976 ; see Doumas & Hummel, Chapter 5 ). They conceptualized problem solving as a process of search through a problem space for a path that connects the initial state to the goal state—a metaphor that alludes to the visual or spatial nature of problem solving (Simon, 1990 ). The term problem space refers to the solver's representation of the task as presented (Simon, 1978 ). It consists of ( 1 ) a set of knowledge states (the initial state, the goal state, and all possible intermediate states), ( 2 ) a set of operators that allow movement from one knowledge state to another, ( 3 ) a set of constraints, and ( 4 ) local information about the path one is taking through the space (e.g., the current knowledge state and how one got there).
We illustrate the components of a problem space for the three-disk Tower of Hanoi problem, as depicted in Figure 21.3 . The initial state appears at the top (State #1) and the goal state at the bottom right (State #27). The remaining knowledge states in the figure are possible intermediate states. The current knowledge state is the one at which the solver is located at any given point in the solution process. For example, the current state for a solver who has made three moves along the optimum solution path would be State #9. The solver presumably would know that he or she arrived at this state from State #5. This knowledge allows the solver to recognize a move that involves backtracking. The three operators in this problem are moving each of the three disks from one peg to another. These operators are subject to the constraint that a larger disk may not be placed on a smaller disk.
Newell and Simon ( 1972 ), as well as other contemporaneous researchers (e.g., Atwood & Polson, 1976 ; Greeno, 1974 ; Thomas, 1974 ), examined how people traverse the spaces of various well-defined problems (e.g., the Tower of Hanoi, Hobbits and Orcs). They discovered that solvers' search is guided by a number of shortcut strategies, or heuristics , which are likely to get the solver to the goal state without an extensive amount of search. Heuristics are often contrasted with algorithms —methods that are guaranteed to yield the correct solution. For example, one could try every possible move in the three-disk Tower of Hanoi problem and, eventually, find the correct solution. Although such an exhaustive search is a valid algorithm for this problem, for many problems its application is very time consuming and impractical (e.g., consider the game of chess).
In their attempts to identify people's search heuristics, Newell and Simon ( 1972 ) relied on two primary methodologies: think-aloud protocols and computer simulations. Their use of think-aloud protocols brought a high degree of scientific rigor to the methodology used by Duncker ( 1945 ; see Ericsson & Simon, 1980 ). Solvers were required to say out loud everything they were thinking as they solved the problem, that is, everything that went through their verbal working memory. Subjects' verbalizations—their think-aloud protocols—were tape-recorded and then transcribed verbatim for analysis. This method is extremely time consuming (e.g., a transcript of one person's solution to the cryptarithmetic problem DONALD + GERALD = ROBERT, with D = 5, generated a 17-page transcript), but it provides a detailed record of the solver's ongoing solution process.
An important caveat to keep in mind while interpreting a subject's verbalizations is that “a protocol is relatively reliable only for what it positively contains, but not for that which it omits” (Duncker, 1945 , p. 11). Ericsson and Simon ( 1980 ) provided an in-depth discussion of the conditions under which this method is valid (but see Russo, Johnson, & Stephens, 1989 , for an alternative perspective). To test their interpretation of a subject's problem solution, inferred from the subject's verbal protocol, Newell and Simon ( 1972 ) created a computer simulation program and examined whether it solved the problem the same way the subject did. To the extent that the computer simulation provided a close approximation of the solver's step-by-step solution process, it lent credence to the researcher's interpretation of the verbal protocol.
Newell and Simon's ( 1972 ) most famous simulation was the General Problem Solver or GPS (Ernst & Newell, 1969 ). GPS successfully modeled human solutions to problems as different as the Tower of Hanoi and the construction of logic proofs using a single general-purpose heuristic: means-ends analysis . This heuristic captures people's tendency to devise a solution plan by setting subgoals that could help them achieve their final goal. It consists of the following steps: ( 1 ) Identify a difference between the current state and the goal (or subgoal ) state; ( 2 ) Find an operator that will remove (or reduce) the difference; (3a) If the operator can be directly applied, do so, or (3b) If the operator cannot be directly applied, set a subgoal to remove the obstacle that is preventing execution of the desired operator; ( 4 ) Repeat steps 1–3 until the problem is solved. Next, we illustrate the implementation of this heuristic for the Tower of Hanoi problem, using the problem space in Figure 21.3 .
As can be seen in Figure 21.3 , a key difference between the initial state and the goal state is that the large disk is on the wrong peg (step 1). To remove this difference (step 2), one needs to apply the operator “move-large-disk.” However, this operator cannot be applied because of the presence of the medium and small disks on top of the large disk. Therefore, the solver may set a subgoal to move that two-disk tower to the middle peg (step 3b), leaving the rightmost peg free for the large disk. A key difference between the initial state and this new subgoal state is that the medium disk is on the wrong peg. Because application of the move-medium-disk operator is blocked, the solver sets another subgoal to move the small disk to the right peg. This subgoal can be satisfied immediately by applying the move-small-disk operator (step 3a), generating State #3. The solver then returns to the previous subgoal—moving the tower consisting of the small and medium disks to the middle peg. The differences between the current state (#3) and the subgoal state (#9) can be removed by first applying the move-medium-disk operator (yielding State #5) and then the move-small-disk operator (yielding State #9). Finally, the move-large-disk operator is no longer blocked. Hence, the solver moves the large disk to the right peg, yielding State #11.
Notice that the subgoals are stacked up in the order in which they are generated, so that they pop up in the order of last in first out. Given the first subgoal in our example, repeated application of the means-ends analysis heuristic will yield the shortest-path solution, indicated by the large gray arrows. In general, subgoals provide direction to the search and allow solvers to plan several moves ahead. By assessing progress toward a required subgoal rather than the final goal, solvers may be able to make moves that otherwise seem unwise. To take a concrete example, consider the transition from State #1 to State #3 in Figure 21.3 . Comparing the initial state to the goal state, this move seems unwise because it places the small disk on the bottom of the right peg, whereas it ultimately needs to be at the top of the tower on that peg. But comparing the initial state to the solver-generated subgoal state of having the medium disk on the middle peg, this is exactly where the small disk needs to go.
Means-ends analysis and various other heuristics (e.g., the hill-climbing heuristic that exploits the similarity, or distance, between the state generated by the next operator and the goal state; working backward from the goal state to the initial state) are flexible strategies that people often use to successfully solve a large variety of problems. However, the generality of these heuristics comes at a cost: They are relatively weak and fallible (e.g., in the means-ends solution to the problem of fixing a hole in a bucket, “Dear Liza” leads “Dear Henry” in a loop that ends back at the initial state; the lyrics of this famous song can be readily found on the Web). Hence, although people use general-purpose heuristics when they encounter novel problems, they replace them as soon as they acquire experience with and sufficient knowledge about the particular problem space (e.g., Anzai & Simon, 1979 ).
Despite the fruitfulness of this research agenda, it soon became evident that a fundamental weakness was that it minimized the importance of people's background knowledge. Of course, Newell and Simon ( 1972 ) were aware that problem solutions require relevant knowledge (e.g., the rules of logical proofs, or rules for stacking disks). Hence, in programming GPS, they supplemented every problem they modeled with the necessary background knowledge. This practice highlighted the generality and flexibility of means-ends analysis but failed to capture how people's background knowledge affects their solutions. As we discussed in the previous section, domain knowledge is likely to affect how people represent problems and, therefore, how they generate problem solutions. Moreover, as people gain experience solving problems in a particular knowledge domain (e.g., math, physics), they change their representations of these problems (e.g., Chi, Feltovich, & Glaser, 1981 ; Haverty, Koedinger, Klahr, & Alibali, 2000 ; Schoenfeld & Herrmann, 1982 ) and learn domain-specific heuristics (e.g., Polya, 1957 ; Schoenfeld, 1979 ) that trump the general-purpose strategies.
It is perhaps inevitable that the two traditions in problem-solving research—one emphasizing representation and the other emphasizing search strategies—would eventually come together. In the next section we review developments that led to this integration.
The Two Legacies Converge
Because Newell and Simon ( 1972 ) aimed to discover the strategies people use in searching for a solution, they investigated problems that minimized the impact of factors that tend to evoke differences in problem representations, of the sort documented by the Gestalt psychologists. In subsequent work, however, Simon and his collaborators showed that such factors are highly relevant to people's solutions of well-defined problems, and Simon ( 1986 ) incorporated these findings into the theoretical framework that views problem solving as search in a problem space.
In this section, we first describe illustrative examples of this work. We then describe research on insight solutions that incorporates ideas from the two legacies described in the previous sections.
Relevance of the Gestalt Ideas to the Solution of Search Problems
In this subsection we describe two lines of research by Simon and his colleagues, and by other researchers, that document the importance of perception and of background knowledge to the way people search for a problem solution. The first line of research used variants of relatively well-defined riddle problems that had the same structure (i.e., “problem isomorphs”) and, therefore, supposedly the same problem space. It documented that people's search depended on various perceptual and conceptual inferences they tended to draw from a specific instantiation of the problem's structure. The second line of research documented that people's search strategies crucially depend on their domain knowledge and on their prior experience with related problems.
Problem Isomorphs
Hayes and Simon ( 1977 ) used two variants of the Tower of Hanoi problem that, instead of disks and pegs, involved monsters and globes that differed in size (small, medium, and large). In both variants, the initial state had the small monster holding the large globe, the medium-sized monster holding the small globe, and the large monster holding the medium-sized globe. Moreover, in both variants the goal was for each monster to hold a globe proportionate to its own size. The only difference between the problems concerned the description of the operators. In one variant (“transfer”), subjects were told that the monsters could transfer the globes from one to another as long as they followed a set of rules, adapted from the rules in the original Tower of Hanoi problem (e.g., only one globe may be transferred at a time). In the other variant (“change”), subjects were told that the monsters could shrink and expand themselves according to a set of rules, which corresponded to the rules in the transfer version of the problem (e.g., only one monster may change its size at a time). Despite the isomorphism of the two variants, subjects conducted their search in two qualitatively different problem spaces, which led to solution times for the change variant being almost twice as long as those for the transfer variant. This difference arose because subjects could more readily envision and track an object that was changing its location with every move than one that was changing its size.
Recent work by Patsenko and Altmann ( 2010 ) found that, even in the standard Tower of Hanoi problem, people's solutions involve object-bound routines that depend on perception and selective attention. The subjects in their study solved various Tower of Hanoi problems on a computer. During the solution of a particular “critical” problem, the computer screen changed at various points without subjects' awareness (e.g., a disk was added, such that a subject who started with a five-disc tower ended with a six-disc tower). Patsenko and Altmann found that subjects' moves were guided by the configurations of the objects on the screen rather than by solution plans they had stored in memory (e.g., the next subgoal).
The Gestalt psychologists highlighted the role of perceptual factors in the formation of problem representations (e.g., Maier's, 1930 , nine-dot problem) but were generally silent about the corresponding implications for how the problem was solved (although they did note effects on solution accuracy). An important contribution of the work on people's solutions of the Tower of Hanoi problem and its variants was to show the relevance of perceptual factors to the application of various operators during search for a problem solution—that is, to the how of problem solving. In the next section, we describe recent work that documents the involvement of perceptual factors in how people understand and use equations and diagrams in the context of solving math and science problems.
Kotovsky, Hayes, and Simon ( 1985 ) further investigated factors that affect people's representation and search in isomorphs of the Tower of Hanoi problem. In one of their isomorphs, three disks were stacked on top of each other to form an inverted pyramid, with the smallest disc on the bottom and the largest on top. Subjects' solutions of the inverted pyramid version were similar to their solutions of the standard version that has the largest disc on the bottom and the smallest on top. However, the two versions were solved very differently when subjects were told that the discs represent acrobats. Subjects readily solved the version in which they had to place a small acrobat on the shoulders of a large one, but they refrained from letting a large acrobat stand on the shoulders of a small one. In other words, object-based inferences that draw on people's semantic knowledge affected the solution of search problems, much as they affect the solution of the ill-defined problems investigated by the Gestalt psychologists (e.g., Duncker's, 1945 , candles problem). In the next section, we describe more recent work that shows similar effects in people's solutions to mathematical word problems.
The work on differences in the representation and solution of problem isomorphs is highly relevant to research on analogical problem solving (or analogical transfer), which examines when and how people realize that two problems that differ in their cover stories have a similar structure (or a similar problem space) and, therefore, can be solved in a similar way. This research shows that minor differences between example problems, such as the use of X-rays versus ultrasound waves to fuse a broken filament of a light bulb, can elicit different problem representations that significantly affect the likelihood of subsequent transfer to novel problem analogs (Holyoak & Koh, 1987 ). Analogical transfer has played a central role in research on human problem solving, in part because it can shed light on people's understanding of a given problem and its solution and in part because it is believed to provide a window onto understanding and investigating creativity (see Smith & Ward, Chapter 23 ). We briefly mention some findings from the analogy literature in the next subsection on expertise, but we do not discuss analogical transfer in detail because this topic is covered elsewhere in this volume (Holyoak, Chapter 13 ).
Expertise and Its Development
In another line of research, Simon and his colleagues examined how people solve ecologically valid problems from various rule-governed and knowledge-rich domains. They found that people's level of expertise in such domains, be it in chess (Chase & Simon, 1973 ; Gobet & Simon, 1996 ), mathematics (Hinsley, Hayes, & Simon, 1977 ; Paige & Simon, 1966 ), or physics (Larkin, McDermott, Simon, & Simon, 1980 ; Simon & Simon, 1978 ), plays a crucial role in how they represent problems and search for solutions. This work, and the work of numerous other researchers, led to the discovery (and rediscovery, see Duncker, 1945 ) of important differences between experts and novices, and between “good” and “poor” students.
One difference between experts and novices pertains to pattern recognition. Experts' attention is quickly captured by familiar configurations within a problem situation (e.g., a familiar configuration of pieces in a chess game). In contrast, novices' attention is focused on isolated components of the problem (e.g., individual chess pieces). This difference, which has been found in numerous domains, indicates that experts have stored in memory many meaningful groups (chunks) of information: for example, chess (Chase & Simon, 1973 ), circuit diagrams (Egan & Schwartz, 1979 ), computer programs (McKeithen, Reitman, Rueter, & Hirtle, 1981 ), medicine (Coughlin & Patel, 1987 ; Myles-Worsley, Johnston, & Simons, 1988 ), basketball and field hockey (Allard & Starkes, 1991 ), and figure skating (Deakin & Allard, 1991 ).
The perceptual configurations that domain experts readily recognize are associated with stored solution plans and/or compiled procedures (Anderson, 1982 ). As a result, experts' solutions are much faster than, and often qualitatively different from, the piecemeal solutions that novice solvers tend to construct (e.g., Larkin et al., 1980 ). In effect, experts often see the solutions that novices have yet to compute (e.g., Chase & Simon, 1973 ; Novick & Sherman, 2003 , 2008 ). These findings have led to the design of various successful instructional interventions (e.g., Catrambone, 1998 ; Kellman et al., 2008 ). For example, Catrambone ( 1998 ) perceptually isolated the subgoals of a statistics problem. This perceptual chunking of meaningful components of the problem prompted novice students to self-explain the meaning of the chunks, leading to a conceptual understanding of the learned solution. In the next section, we describe some recent work that shows the beneficial effects of perceptual pattern recognition on the solution of familiar mathematics problems, as well as the potentially detrimental effects of familiar perceptual chunks to understanding and reasoning with diagrams depicting evolutionary relationships among taxa.
Another difference between experts and novices pertains to their understanding of the solution-relevant problem structure. Experts' knowledge is highly organized around domain principles, and their problem representations tend to reflect this principled understanding. In particular, they can extract the solution-relevant structure of the problems they encounter (e.g., meaningful causal relations among the objects in the problem; see Cheng & Buehner, Chapter 12 ). In contrast, novices' representations tend to be bound to surface features of the problems that may be irrelevant to solution (e.g., the particular objects in a problem). For example, Chi, Feltovich, and Glaser ( 1981 ) examined how students with different levels of physics expertise group mechanics word problems. They found that advanced graduate students grouped the problems based on the physics principles relevant to the problems' solutions (e.g., conservation of energy, Newton's second law). In contrast, undergraduates who had successfully completed an introductory course in mechanics grouped the problems based on the specific objects involved (e.g., pulley problems, inclined plane problems). Other researchers have found similar results in the domains of biology, chemistry, computer programming, and math (Adelson, 1981 ; Kindfield, 1993 / 1994 ; Kozma & Russell, 1997 ; McKeithen et al., 1981 ; Silver, 1979 , 1981 ; Weiser & Shertz, 1983 ).
The level of domain expertise and the corresponding representational differences are, of course, a matter of degree. With increasing expertise, there is a gradual change in people's focus of attention from aspects that are not relevant to solution to those that are (e.g., Deakin & Allard, 1991 ; Hardiman, Dufresne, & Mestre, 1989 ; McKeithen et al., 1981 ; Myles-Worsley et al., 1988 ; Schoenfeld & Herrmann, 1982 ; Silver, 1981 ). Interestingly, Chi, Bassok, Lewis, Reimann, and Glaser ( 1989 ) found similar differences in focus on structural versus surface features among a group of novices who studied worked-out examples of mechanics problems. These differences, which echo Wertheimer's ( 1959 ) observations of individual differences in students' learning about the area of parallelograms, suggest that individual differences in people's interests and natural abilities may affect whether, or how quickly, they acquire domain expertise.
An important benefit of experts' ability to focus their attention on solution-relevant aspects of problems is that they are more likely than novices to recognize analogous problems that involve different objects and cover stories (e.g., Chi et al., 1989 ; Novick, 1988 ; Novick & Holyoak, 1991 ; Wertheimer, 1959 ) or that come from other knowledge domains (e.g., Bassok & Holyoak, 1989 ; Dunbar, 2001 ; Goldstone & Sakamoto, 2003 ). For example, Bassok and Holyoak ( 1989 ) found that, after learning to solve arithmetic-progression problems in algebra, subjects spontaneously applied these algebraic solutions to analogous physics problems that dealt with constantly accelerated motion. Note, however, that experts and good students do not simply ignore the surface features of problems. Rather, as was the case in the problem isomorphs we described earlier (Kotovsky et al., 1985 ), they tend to use such features to infer what the problem's structure could be (e.g., Alibali, Bassok, Solomon, Syc, & Goldin-Meadow, 1999 ; Blessing & Ross, 1996 ). For example, Hinsley et al. ( 1977 ) found that, after reading no more than the first few words of an algebra word problem, expert solvers classified the problem into a likely problem category (e.g., a work problem, a distance problem) and could predict what questions they might be asked and the equations they likely would need to use.
Surface-based problem categorization has a heuristic value (Medin & Ross, 1989 ): It does not ensure a correct categorization (Blessing & Ross, 1996 ), but it does allow solvers to retrieve potentially appropriate solutions from memory and to use them, possibly with some adaptation, to solve a variety of novel problems. Indeed, although experts exploit surface-structure correlations to save cognitive effort, they have the capability to realize that a particular surface cue is misleading (Hegarty, Mayer, & Green, 1992 ; Lewis & Mayer, 1987 ; Martin & Bassok, 2005 ; Novick 1988 , 1995 ; Novick & Holyoak, 1991 ). It is not surprising, therefore, that experts may revert to novice-like heuristic methods when solving problems under pressure (e.g., Beilock, 2008 ) or in subdomains in which they have general but not specific expertise (e.g., Patel, Groen, & Arocha, 1990 ).
Relevance of Search to Insight Solutions
We introduced the notion of insight in our discussion of the nine-dot problem in the section on the Gestalt tradition. The Gestalt view (e.g., Duncker, 1945 ; Maier, 1931 ; see Ohlsson, 1984 , for a review) was that insight problem solving is characterized by an initial work period during which no progress toward solution is made (i.e., an impasse), a sudden restructuring of one's problem representation to a more suitable form, followed immediately by the sudden appearance of the solution. Thus, solving problems by insight was believed to be all about representation, with essentially no role for a step-by-step solution process (i.e., search). Subsequent and contemporary researchers have generally concurred with the Gestalt view that getting the right representation is crucial. However, research has shown that insight solutions do not necessarily arise suddenly or full blown after restructuring (e.g., Weisberg & Alba, 1981 ); and even when they do, the underlying solution process (in this case outside of awareness) may reflect incremental progress toward the goal (Bowden & Jung-Beeman, 2003 ; Durso, Rea, & Dayton, 1994 ; Novick & Sherman, 2003 ).
“Demystifying insight,” to borrow a phrase from Bowden, Jung-Beeman, Fleck, and Kounios ( 2005 ), requires explaining ( 1 ) why solvers initially reach an impasse in solving a problem for which they have the necessary knowledge to generate the solution, ( 2 ) how the restructuring occurred, and ( 3 ) how it led to the solution. A detailed discussion of these topics appears elsewhere in this volume (van Steenburgh et al., Chapter 24 ). Here, we describe briefly three recent theories that have attempted to account for various aspects of these phenomena: Knoblich, Ohlsson, Haider, and Rhenius's ( 1999 ) representational change theory, MacGregor, Ormerod, and Chronicle's ( 2001 ) progress monitoring theory, and Bowden et al.'s ( 2005 ) neurological model. We then propose the need for an integrated approach to demystifying insight that considers both representation and search.
According to Knoblich et al.'s ( 1999 ) representational change theory, problems that are solved with insight are highly likely to evoke initial representations in which solvers place inappropriate constraints on their solution attempts, leading to an impasse. An impasse can be resolved by revising one's representation of the problem. Knoblich and his colleagues tested this theory using Roman numeral matchstick arithmetic problems in which solvers must move one stick to a new location to change a false numerical statement (e.g., I = II + II ) into a statement that is true. According to representational change theory, re-representation may occur through either constraint relaxation or chunk decomposition. (The solution to the example problem is to change II + to III – , which requires both methods of re-representation, yielding I = III – II ). Good support for this theory has been found based on measures of solution rate, solution time, and eye fixation (Knoblich et al., 1999 ; Knoblich, Ohlsson, & Raney, 2001 ; Öllinger, Jones, & Knoblich, 2008 ).
Progress monitoring theory (MacGregor et al., 2001 ) was proposed to account for subjects' difficulty in solving the nine-dot problem, which has traditionally been classified as an insight problem. According to this theory, solvers use the hill-climbing search heuristic to solve this problem, just as they do for traditional search problems (e.g., Hobbits and Orcs). In particular, solvers are hypothesized to monitor their progress toward solution using a criterion generated from the problem's current state. If solvers reach criterion failure, they seek alternative solutions by trying to relax one or more problem constraints. MacGregor et al. found support for this theory using several variants of the nine-dot problem (also see Ormerod, MacGregor, & Chronicle, 2002 ). Jones ( 2003 ) suggested that progress monitoring theory provides an account of the solution process up to the point an impasse is reached and representational change is sought, at which point representational change theory picks up and explains how insight may be achieved. Hence, it appears that a complete account of insight may require an integration of concepts from the Gestalt (representation) and Newell and Simon's (search) legacies.
Bowden et al.'s ( 2005 ) neurological model emphasizes the overlap between problem solving and language comprehension, and it hinges on differential processing in the right and left hemispheres. They proposed that an impasse is reached because initial processing of the problem produces strong activation of information irrelevant to solution in the left hemisphere. At the same time, weak semantic activation of alternative semantic interpretations, critical for solution, occurs in the right hemisphere. Insight arises when the weakly activated concepts reinforce each other, eventually rising above the threshold required for conscious awareness. Several studies of problem solving using compound remote associates problems, involving both behavioral and neuroimaging data, have found support for this model (Bowden & Jung-Beeman, 1998 , 2003 ; Jung-Beeman & Bowden, 2000 ; Jung-Beeman et al., 2004 ; also see Moss, Kotovsky, & Cagan, 2011 ).
Note that these three views of insight have received support using three quite distinct types of problems (Roman numeral matchstick arithmetic problems, the nine-dot problem, and compound remote associates problems, respectively). It remains to be established, therefore, whether these accounts can be generalized across problems. Kershaw and Ohlsson ( 2004 ) argued that insight problems are difficult because the key behavior required for solution may be hindered by perceptual factors (the Gestalt view), background knowledge (so expertise may be important; e.g., see Novick & Sherman, 2003 , 2008 ), and/or process factors (e.g., those affecting search). From this perspective, solving visual problems (e.g., the nine-dot problem) with insight may call upon more general visual processes, whereas solving verbal problems (e.g., anagrams, compound remote associates) with insight may call upon general verbal/semantic processes.
The work we reviewed in this section shows the relevance of problem representation (the Gestalt legacy) to the way people search the problem space (the legacy of Newell and Simon), and the relevance of search to the solution of insight problems that require a representational change. In addition to this inevitable integration of the two legacies, the work we described here underscores the fact that problem solving crucially depends on perceptual factors and on the solvers' background knowledge. In the next section, we describe some recent work that shows the involvement of these factors in the solution of problems in math and science.
Effects of Perception and Knowledge in Problem Solving in Academic Disciplines
Although the use of puzzle problems continues in research on problem solving, especially in investigations of insight, many contemporary researchers tackle problem solving in knowledge-rich domains, often in academic disciplines (e.g., mathematics, biology, physics, chemistry, meteorology). In this section, we provide a sampling of this research that highlights the importance of visual perception and background knowledge for successful problem solving.
The Role of Visual Perception
We stated at the outset that a problem representation (e.g., the problem space) is a model of the problem constructed by solvers to summarize their understanding of the problem's essential nature. This informal definition refers to the internal representations people construct and hold in working memory. Of course, people may also construct various external representations (Markman, 1999 ) and even manipulate those representations to aid in solution (see Hegarty & Stull, Chapter 31 ). For example, solvers often use paper and pencil to write notes or draw diagrams, especially when solving problems from formal domains (e.g., Cox, 1999 ; Kindfield, 1993 / 1994 ; S. Schwartz, 1971 ). In problems that provide solvers with external representation, such as the Tower of Hanoi problem, people's planning and memory of the current state is guided by the actual configurations of disks on pegs (Garber & Goldin-Meadow, 2002 ) or by the displays they see on a computer screen (Chen & Holyoak, 2010 ; Patsenko & Altmann, 2010 ).
In STEM (science, technology, engineering, and mathematics) disciplines, it is common for problems to be accompanied by diagrams or other external representations (e.g., equations) to be used in determining the solution. Larkin and Simon ( 1987 ) examined whether isomorphic sentential and diagrammatic representations are interchangeable in terms of facilitating solution. They argued that although the two formats may be equivalent in the sense that all of the information in each format can be inferred from the other format (informational equivalence), the ease or speed of making inferences from the two formats might differ (lack of computational equivalence). Based on their analysis of several problems in physics and math, Larkin and Simon further argued for the general superiority of diagrammatic representations (but see Mayer & Gallini, 1990 , for constraints on this general conclusion).
Novick and Hurley ( 2001 , p. 221) succinctly summarized the reasons for the general superiority of diagrams (especially abstract or schematic diagrams) over verbal representations: They “(a) simplify complex situations by discarding unnecessary details (e.g., Lynch, 1990 ; Winn, 1989 ), (b) make abstract concepts more concrete by mapping them onto spatial layouts with familiar interpretational conventions (e.g., Winn, 1989 ), and (c) substitute easier perceptual inferences for more computationally intensive search processes and sentential deductive inferences (Barwise & Etchemendy, 1991 ; Larkin & Simon, 1987 ).” Despite these benefits of diagrammatic representations, there is an important caveat, noted by Larkin and Simon ( 1987 , p. 99) at the very end of their paper: “Although every diagram supports some easy perceptual inferences, nothing ensures that these inferences must be useful in the problem-solving process.” We will see evidence of this in several of the studies reviewed in this section.
Next we describe recent work on perceptual factors that are involved in people's use of two types of external representations that are provided as part of the problem in two STEM disciplines: equations in algebra and diagrams in evolutionary biology. Although we focus here on effects of perceptual factors per se, it is important to note that such factors only influence performance when subjects have background knowledge that supports differential interpretation of the alternative diagrammatic depictions presented (Hegarty, Canham, & Fabricant, 2010 ).
In the previous section, we described the work of Patsenko and Altmann ( 2010 ) that shows direct involvement of visual attention and perception in the sequential application of move operators during the solution of the Tower of Hanoi problem. A related body of work documents similar effects in tasks that require the interpretation and use of mathematical equations (Goldstone, Landy, & Son, 2010 ; Landy & Goldstone, 2007a , b). For example, Landy and Goldstone ( 2007b ) varied the spatial proximity of arguments to the addition (+) and multiplication (*) operators in algebraic equations, such that the spatial layout of the equation was either consistent or inconsistent with the order-of-operations rule that multiplication precedes addition. In consistent equations , the space was narrower around multiplication than around addition (e.g., g*m + r*w = m*g + w*r ), whereas in inconsistent equations this relative spacing was reversed (e.g., s * n+e * c = n * s+c * e ). Subjects' judgments of the validity of such equations (i.e., whether the expressions on the two sides of the equal sign are equivalent) were significantly faster and more accurate for consistent than inconsistent equations.
In discussing these findings and related work with other external representations, Goldstone et al. ( 2010 ) proposed that experience with solving domain-specific problems leads people to “rig up” their perceptual system such that it allows them to look at the problem in a way that is consistent with the correct rules. Similar logic guides the Perceptual Learning Modules developed by Kellman and his collaborators to help students interpret and use algebraic equations and graphs (Kellman et al., 2008 ; Kellman, Massey, & Son, 2009 ). These authors argued and showed that, consistent with the previously reviewed work on expertise, perceptual training with particular external representations supports the development of perceptual fluency. This fluency, in turn, supports students' subsequent use of these external representations for problem solving.
This research suggests that extensive experience with particular equations or graphs may lead to perceptual fluency that could replace the more mindful application of domain-specific rules. Fisher, Borchert, and Bassok ( 2011 ) reported results from algebraic-modeling tasks that are consistent with this hypothesis. For example, college students were asked to represent verbal statements with algebraic equations, a task that typically elicits systematic errors (e.g., Clement, Lochhead, & Monk, 1981 ). Fisher et al. found that such errors were very common when subjects were asked to construct “standard form” equations ( y = ax ), which support fluent left-to-right translation of words to equations, but were relatively rare when subjects were asked to construct nonstandard division-format equations (x = y/a) that do not afford such translation fluency.
In part because of the left-to-right order in which people process equations, which mirrors the linear order in which they process text, equations have traditionally been viewed as sentential representations. However, Landy and Goldstone ( 2007a ) have proposed that equations also share some properties with diagrammatic displays and that, in fact, in some ways they are processed like diagrams. That is, spatial information is used to represent and to support inferences about syntactic structure. This hypothesis received support from Landy and Goldstone's ( 2007b ) results, described earlier, in which subjects' judgments of the validity of equations were affected by the Gestalt principle of grouping: Subjects did better when the grouping was consistent rather than inconsistent with the underlying structure of the problem (order of operations). Moreover, Landy and Goldstone ( 2007a ) found that when subjects wrote their own equations they grouped numbers and operators (+, *, =) in a way that reflected the hierarchical structure imposed by the order-of-operations rule.
In a recent line of research, Novick and Catley ( 2007 ; Novick, Catley, & Funk, 2010 ; Novick, Shade, & Catley, 2011 ) have examined effects of the spatial layout of diagrams depicting the evolutionary history of a set of taxa on people's ability to reason about patterns of relationship among those taxa. We consider here their work that investigates the role of another Gestalt perceptual principle—good continuation—in guiding students' reasoning. According to this principle, a continuous line is perceived as a single entity (Kellman, 2000 ). Consider the diagrams shown in Figure 21.6 . Each is a cladogram, a diagram that depicts nested sets of taxa that are related in terms of levels of most recent common ancestry. For example, chimpanzees and starfish are more closely related to each other than either is to spiders. The supporting evidence for their close relationship is their most recent common ancestor, which evolved the novel character of having radial cleavage. Spiders do not share this ancestor and thus do not have this character.
Cladograms are typically drawn in two isomorphic formats, which Novick and Catley ( 2007 ) referred to as trees and ladders. Although these formats are informationally equivalent (Larkin & Simon, 1987 ), Novick and Catley's ( 2007 ) research shows that they are not computationally equivalent (Larkin & Simon, 1987 ). Imagine that you are given evolutionary relationships in the ladder format, such as in Figure 21.6a (but without the four characters—hydrostatic skeleton, bilateral symmetry, radial cleavage, and trocophore larvae—and associated short lines indicating their locations on the cladogram), and your task is to translate that diagram to the tree format. A correct translation is shown in Figure 21.6b . Novick and Catley ( 2007 ) found that college students were much more likely to get such problems correct when the presented cladogram was in the nested circles (e.g., Figure 21.6d ) rather than the ladder format. Because the Gestalt principle of good continuation makes the long slanted line at the base of the ladder appear to represent a single hierarchical level, a common translation error for the ladder to tree problems was to draw a diagram such as that shown in Figure 21.6c .
The difficulty that good continuation presents for interpreting relationships depicted in the ladder format extends to answering reasoning questions as well. Novick and Catley (unpublished data) asked comparable questions about relationships depicted in the ladder and tree formats. For example, using the cladograms depicted in Figures 21.6a and 21.6b , consider the following questions: (a) Which taxon—jellyfish or earthworm—is the closest evolutionary relation to starfish, and what evidence supports your answer? (b) Do the bracketed taxa comprise a clade (a set of taxa consisting of the most recent common ancestor and all of its descendants), and what evidence supports your answer? For both such questions, students had higher accuracy and evidence quality composite scores when the relationships were depicted in the tree than the ladder format.
Four cladograms depicting evolutionary relationships among six animal taxa. Cladogram ( a ) is in the ladder format, cladograms ( b ) and ( c ) are in the tree format, and cladogram ( d ) is in the nested circles format. Cladograms ( a ), ( b ), and ( d ) are isomorphic.
If the difficulty in extracting the hierarchical structure of the ladder format is due to good continuation (which leads problem solvers to interpret continuous lines that depict multiple hierarchical levels as depicting only a single level), then a manipulation that breaks good continuation at the points where a new hierarchical level occurs should improve understanding. Novick et al. ( 2010 ) tested this hypothesis using a translation task by manipulating whether characters that are the markers for the most recent common ancestor of each nested set of taxa were included on the ladders. Figure 21.6a shows a ladder with such characters. As predicted, translation accuracy increased dramatically simply by adding these characters to the ladders, despite the additional information subjects had to account for in their translations.
The Role of Background Knowledge
As we mentioned earlier, the specific entities in the problems people encounter evoke inferences that affect how people represent these problems (e.g., the candle problem; Duncker, 1945 ) and how they apply the operators in searching for the solution (e.g., the disks vs. acrobats versions of the Tower of Hanoi problem; Kotovsky et al., 1985 ). Such object-based inferences draw on people's knowledge about the properties of the objects (e.g., a box is a container, an acrobat is a person who can be hurt). Here, we describe the work of Bassok and her colleagues, who found that similar inferences affect how people select mathematical procedures to solve problems in various formal domains. This work shows that the objects in the texts of mathematical word problems affect how people represent the problem situation (i.e., the situation model they construct; Kintsch & Greeno, 1985 ) and, in turn, lead them to select mathematical models that have a corresponding structure. To illustrate, a word problem that describes constant change in the rate at which ice is melting off a glacier evokes a model of continuous change, whereas a word problem that describes constant change in the rate at which ice is delivered to a restaurant evokes a model of discrete change. These distinct situation models lead subjects to select corresponding visual representations (e.g., Bassok & Olseth, 1995 ) and solutions methods, such as calculating the average change over time versus adding the consecutive changes (e.g., Alibali et al., 1999 ).
In a similar manner, people draw on their general knowledge to infer how the objects in a given problem are related to each other and construct mathematical solutions that correspond to these inferred object relations. For example, a word problem that involves doctors from two hospitals elicits a situation model in which the two sets of doctors play symmetric roles (e.g., work with each other), whereas a mathematically isomorphic problem that involves mechanics and cars elicits a situation model in which the sets play asymmetric roles (e.g., mechanics fix cars). The mathematical solutions people construct to such problems reflect this difference in symmetry (Bassok, Wu, & Olseth, 1995 ). In general, people tend to add objects that belong to the same taxonomic category (e.g., doctors + doctors) but divide functionally related objects (e.g., cars ÷ mechanics). People establish this correspondence by a process of analogical alignment between semantic and arithmetic relations, which Bassok and her colleagues refer to as “semantic alignment” (Bassok, Chase, & Martin, 1998 ; Doumas, Bassok, Guthormsen, & Hummel, 2006 ; Fisher, Bassok, & Osterhout, 2010 ).
Semantic alignment occurs very early in the solution process and can prime arithmetic facts that are potentially relevant to the problem solution (Bassok, Pedigo, & Oskarsson, 2008 ). Although such alignments can lead to erroneous solutions, they have a high heuristic value because, in most textbook problems, object relations indeed correspond to analogous mathematical relations (Bassok et al., 1998 ). Interestingly, unlike in the case of reliance on specific surface-structure correlations (e.g., the keyword “more” typically appears in word problems that require addition; Lewis & Mayer, 1987 ), people are more likely to exploit semantic alignment when they have more, rather than less modeling experience. For example, Martin and Bassok ( 2005 ) found very strong semantic-alignment effects when subjects solved simple division word problems, but not when they constructed algebraic equations to represent the relational statements that appeared in the problems. Of course, these subjects had significantly more experience with solving numerical word problems than with constructing algebraic models of relational statements. In a subsequent study, Fisher and Bassok ( 2009 ) found semantic-alignment effects for subjects who constructed correct algebraic models, but not for those who committed modeling errors.
Conclusions and Future Directions
In this chapter, we examined two broad components of the problem-solving process: representation (the Gestalt legacy) and search (the legacy of Newell and Simon). Although many researchers choose to focus their investigation on one or the other of these components, both Duncker ( 1945 ) and Simon ( 1986 ) underscored the necessity to investigate their interaction, as the representation one constructs for a problem determines (or at least constrains) how one goes about trying to generate a solution, and searching the problem space may lead to a change in problem representation. Indeed, Duncker's ( 1945 ) initial account of one subject's solution to the radiation problem was followed up by extensive and experimentally sophisticated work by Simon and his colleagues and by other researchers, documenting the involvement of visual perception and background knowledge in how people represent problems and search for problem solutions.
The relevance of perception and background knowledge to problem solving illustrates the fact that, when people attempt to find or devise ways to reach their goals, they draw on a variety of cognitive resources and engage in a host of cognitive activities. According to Duncker ( 1945 ), such goal-directed activities may include (a) placing objects into categories and making inferences based on category membership, (b) making inductive inferences from multiple instances, (c) reasoning by analogy, (d) identifying the causes of events, (e) deducing logical implications of given information, (f) making legal judgments, and (g) diagnosing medical conditions from historical and laboratory data. As this list suggests, many of the chapters in the present volume describe research that is highly relevant to the understanding of problem-solving behavior. We believe that important advancements in problem-solving research would emerge by integrating it with research in other areas of thinking and reasoning, and that research in these other areas could be similarly advanced by incorporating the insights gained from research on what has more traditionally been identified as problem solving.
As we have described in this chapter, many of the important findings in the field have been established by a careful investigation of various riddle problems. Although there are good methodological reasons for using such problems, many researchers choose to investigate problem solving using ecologically valid educational materials. This choice, which is increasingly common in contemporary research, provides researchers with the opportunity to apply their basic understanding of problem solving to benefit the design of instruction and, at the same time, allows them to gain a better understanding of the processes by which domain knowledge and educational conventions affect the solution process. We believe that the trend of conducting educationally relevant research is likely to continue, and we expect a significant expansion of research on people's understanding and use of dynamic and technologically rich external representations (e.g., Kellman et al., 2008 ; Mayer, Griffith, Jurkowitz, & Rothman, 2008 ; Richland & McDonough, 2010 ; Son & Goldstone, 2009 ). Such investigations are likely to yield both practical and theoretical payoffs.
Adams, J. L. ( 1979 ). Conceptual blockbusting: A guide to better ideas (2nd ed.). New York: Norton.
Google Scholar
Google Preview
Adelson, B. ( 1981 ). Problem solving and the development of abstract categories in programming languages. Memory and Cognition , 9 , 422–433.
Alibali, M. W., Bassok, M., Solomon, K. O., Syc, S. E., & Goldin-Meadow, S. ( 1999 ). Illuminating mental representations through speech and gesture. Psychological Science , 10 , 327–333.
Allard, F., & Starkes, J. L. ( 1991 ). Motor-skill experts in sports, dance, and other domains. In K. A. Ericsson & J. Smith (Eds.), Toward a general theory of expertise: Prospects and limits (pp. 126–152). New York: Cambridge University Press.
Anderson, J. R. ( 1982 ). Acquisition of cognitive skill. Psychological Review , 89 , 369–406.
Anzai, Y., & Simon, H. A. ( 1979 ). The theory of learning by doing. Psychological Review , 86 , 124–140.
Atwood, M. E, & Polson, P.G. ( 1976 ). A process model for water jug problems. Cognitive Psychology , 8 , 191–216.
Barwise, J., & Etchemendy, J. ( 1991 ). Visual information and valid reasoning. In W. Zimmermann & S. Cunningham (Eds.), Visualization in teaching and learning mathematics (pp. 9–24). Washington, DC: Mathematical Association of America.
Bassok, M., Chase, V. M., & Martin, S. A. ( 1998 ). Adding apples and oranges: Alignment of semantic and formal knowledge. Cognitive Psychology , 35 , 99–134.
Bassok, M., & Holyoak, K. J. ( 1989 ). Interdomain transfer between isomorphic topics in algebra and physics. Journal of Experimental Psychology: Learning, Memory, and Cognition , 15 , 153–166.
Bassok, M., & Olseth, K. L. ( 1995 ). Object-based representations: Transfer between cases of continuous and discrete models of change. Journal of Experimental Psychology: Learning, Memory, and Cognition , 21 , 1522–1538.
Bassok, M., Pedigo, S. F., & Oskarsson, A. T. ( 2008 ). Priming addition facts with semantic relations. Journal of Experimental Psychology: Learning, Memory, and Cognition , 34 , 343–352.
Bassok, M., Wu, L., & Olseth, L. K. ( 1995 ). Judging a book by its cover: Interpretative effects of content on problem solving transfer. Memory and Cognition , 23 , 354–367.
Beilock, S. L. ( 2008 ). Math performance in stressful situations. Current Directions in Psychological Science , 17 , 339–343.
Birch, H. G. & Rabinowitz, H. S. ( 1951 ). The negative effect of previous experience on productive thinking. Journal of Experimental Psychology , 41 , 122–126.
Blessing, S. B., & Ross, B. H. ( 1996 ). Content effects in problem categorization and problem solving. Journal of Experimental Psychology: Learning, Memory, and Cognition , 22 , 792–810.
Bowden, E. M., & Jung-Beeman, M. ( 1998 ). Getting the right idea: Semantic activation in the right hemisphere may help solve insight problems. Psychological Science , 6 , 435–440.
Bowden, E. M., & Jung-Beeman, M. ( 2003 ). Aha! Insight experience correlates with solution activation in the right hemisphere. Psychonomic Bulletin and Review , 10 , 730–737.
Bowden, E. M., Jung-Beeman, M., Fleck, J., & Kounios, J. ( 2005 ). New approaches to demystifying insight. Trends in Cognitive Sciences , 9 , 322–328.
Catrambone, R. ( 1998 ). The subgoal-learning model: Creating better examples so that students can solve novel problems. Journal of Experimental Psychology: General , 127 , 355–376.
Chase, W. G., & Simon, H. A. ( 1973 ). Perception in chess. Cognitive Psychology , 4 , 55–81.
Chen, D., & Holyoak, K. J. ( 2010 ). Enhancing acquisition of intuition versus planning in problem solving. In S. Ohlsson & R. Catrambone (Eds.), Proceedings of the 32nd Annual Conference of the Cognitive Science Society (pp. 1875–1880). Austin, TX: Cognitive Science Society.
Chi, M. T. H., Bassok, M., Lewis, M. W., Reimann, P., & Glaser, R. ( 1989 ). Self-explanations: How students study and use examples in learning to solve problems. Cognitive Science , 13 , 145–182.
Chi, M. T. H., Feltovich, P. J., & Glaser, R. ( 1981 ). Categorization and representation of physics problems by experts and novices. Cognitive Science , 5 , 121–152.
Clement, J., Lochhead, J., & Monk, G. S. ( 1981 ). Translation difficulties in learning mathematics. The American Mathematical Monthly , 88 , 286–290.
Coughlin, L. D., & Patel, V. L. ( 1987 ). Processing of critical information by physicians and medical students. Journal of Medical Education , 62 , 818–828.
Cox, R. ( 1999 ). Representation construction, externalised cognition and individual differences. Learning and Instruction , 9 , 343–363.
Deakin, J. M., & Allard, F. ( 1991 ). Skilled memory in expert figure skaters. Memory and Cognition , 19 , 79–86.
Doumas, L. A. A., Bassok, M., Guthormsen, A., & Hummel, J. E. ( 2006 ). Theory of reflexive relational generalization. In R. Sun & N. Miyake (Eds.), Proceedings of the 28th Annual Conference of the Cognitive Science Society (pp. 1246–1250). Mahwah, NJ: Erlbaum.
Dunbar, K. ( 2001 ). The analogical paradox: Why analogy is so easy in naturalistic settings, yet so difficult in the psychological laboratory. In D. Gentner, K. J. Holyoak, & B. Kokinov (Eds.), Analogy: Perspectives from cognitive science (pp. 313–362). Cambridge, MA: MIT Press.
Duncker, K. ( 1945 ). On problem-solving (L. S. Lees, Trans.). Psychological Monographs , 58 (Whole No. 270). (Original work published 1935).
Durso, F. T., Rea, C. B., & Dayton, T. ( 1994 ). Graph-theoretic confirmation of restructuring during insight. Psychological Science , 5 , 94–98.
Egan, D. E., & Schwartz, B. J. ( 1979 ). Chunking in the recall of symbolic drawings. Memory and Cognition , 7 , 149–158.
Ericsson, K. A., & Simon, H. A. ( 1980 ). Verbal reports as data. Psychological Review , 87 , 215–251.
Ernst, G. W., & Newell, A. ( 1969 ). GPS: A case study in generality and problem solving . New York: Academic Press.
Fisher, K. J., & Bassok, M. ( 2009 ). Analogical alignments in algebraic modeling. In B. Kokinov, D. Gentner, & K. J. Holyoak (Eds.), Proceedings of the 2nd International Analogy Conference (pp. 137–144). Sofia, Bulgaria: New Bulgarian University Press.
Fisher, K. J., Bassok, M., & Osterhout, L. ( 2010 ). When two plus two does not equal four: Event-related potential responses to semantically incongruous arithmetic word problems. In S. Ohlsson & R. Catrambone (Eds.), Proceedings of the 32nd Annual Conference of the Cognitive Science Society (pp. 1571–1576). Austin, TX: Cognitive Science Society.
Fisher, K. J., Borchert, K., & Bassok, M. ( 2011 ). Following the standard form: Effects of equation format on algebraic modeling. Memory and Cognition , 39 , 502–515.
Garber, P., & Goldin-Meadow, S. ( 2002 ). Gesture offers insight into problem solving in adults and children. Cognitive Science , 26 , 817–831.
Gobet, F., & Simon, H. ( 1996 ). Recall of rapidly presented random chess positions is a function of skill. Psychonomic Bulletin and Review , 3 , 159–163.
Goldstone, R. L., Landy, D. H., & Son, J. Y. ( 2010 ). The education of perception. Topics in Cognitive Science , 2 , 265–284.
Goldstone, R. L., & Sakamoto, J. Y. ( 2003 ). The transfer of abstract principles governing complex adaptive systems. Cognitive Psychology , 46 , 414–466.
Greeno, J. G. ( 1974 ). Hobbits and orcs: Acquisition of a sequential concept. Cognitive Psychology , 6 , 270–292.
Hardiman, P. T., Dufresne, R., & Mestre, J. P. ( 1989 ). The relation between problem categorization and problem solving among experts and novices. Memory and Cognition , 17 , 627–638.
Haverty, L. A., Koedinger, K. R., Klahr, D., & Alibali, M. W. ( 2000 ). Solving induction problems in mathematics: Not-so-trivial Pursuit. Cognitive Science , 24 , 249–298.
Hayes, J. R., & Simon, H. A. ( 1977 ). Psychological differences among problem isomorphs. In N. J. Castellan, D. B. Pisoni, & G. R. Potts (Eds.), Cognitive theory (Vol. 2, pp. 21–44). Hillsdale, NJ: Erlbaum.
Hegarty, M., Canham, M. S., & Fabricant, S. I. ( 2010 ). Thinking about the weather: How display salience and knowledge affect performance in a graphic inference task. Journal of Experimental Psychology: Learning, Memory, and Cognition , 36 , 37–53.
Hegarty, M., Mayer, R. E., & Green, C. E. ( 1992 ). Comprehension of arithmetic word problems: Evidence from students' eye fixations. Journal of Educational Psychology , 84 , 76–84.
Hinsley, D. A., Hayes, J. R., & Simon, H. A. ( 1977 ). From words to equations: Meaning and representation in algebra word problems. In D. Hinsley, M. Just., & P. Carpenter (Eds.), Cognitive processes in comprehension (pp. 89–106). Hillsdale, NJ: Erlbaum.
Holyoak, K. J., & Koh, K. ( 1987 ). Surface and structural similarity in analogical transfer. Memory and Cognition , 15 , 332–340.
Jones, G. ( 2003 ). Testing two cognitive theories of insight. Journal of Experimental Psychology: Learning, Memory, and Cognition , 29 , 1017–1027.
Jung-Beeman, M., & Bowden, E. M. ( 2000 ). The right hemisphere maintains solution-related activation for yet-to-be solved insight problems. Memory and Cognition , 28 , 1231–1241.
Jung-Beeman, M., Bowden, E. M., Haberman, J., Frymiare, J. L., Arambel-Liu, S., Greenblatt, R., … Kounios, J. ( 2004 ). Neural activity when people solve verbal problems with insight. PLOS Biology , 2 , 500–510.
Kellman, P. J. ( 2000 ). An update on Gestalt psychology. In B. Landau, J. Sabini, J. Jonides, & E. Newport (Eds.), Perception, cognition, and language: Essays in honor of Henry and Lila Gleitman (pp. 157–190). Cambridge, MA: MIT Press.
Kellman, P. J., Massey, C. M., & Son, J. Y ( 2009 ). Perceptual learning modules in mathematics: Enhancing students' pattern recognition, structure extraction, and fluency. Topics in Cognitive Science , 1 , 1–21.
Kellman, P. J., Massey, C., Roth, Z., Burke, T., Zucker, J., Saw, A., … Wise, J. A. ( 2008 ). Perceptual learning and the technology of expertise. Pragmatics and Cognition , 16 , 356–405.
Kershaw, T. C., & Ohlsson, S. ( 2004 ). Multiple causes of difficulty in insight: The case of the nine-dot problem. Journal of Experimental Psychology: Learning, Memory, and Cognition , 30 , 3–13.
Kindfield, A. C. H. ( 1993 /1994). Biology diagrams: Tools to think with. Journal of the Learning Sciences , 3 , 1–36.
Kintsch, W., & Greeno, J. G. ( 1985 ). Understanding and solving word arithmetic problems. Psychological Review , 92 , 109–129.
Knoblich, G., Ohlsson, S., Haider, H., & Rhenius, D. ( 1999 ). Constraint relaxation and chunk decomposition in insight problem solving. Journal of Experimental Psychology: Learning, Memory, and Cognition , 25 , 1534–1555.
Knoblich, G., Ohlsson, S., & Raney, G. E. ( 2001 ). An eye movement study of insight problem solving. Memory and Cognition , 29 , 1000–1009.
Kohler, W. ( 1925 ). The mentality of apes . New York: Harcourt Brace.
Kotovsky, K., Hayes, J. R., & Simon, H. A. ( 1985 ). Why are some problems hard? Evidence from Tower of Hanoi. Cognitive Psychology , 17 , 248–294.
Kozma, R. B., & Russell, J. ( 1997 ). Multimedia and understanding: Expert and novice responses to different representations of chemical phenomena. Journal of Research in Science Teaching , 34 , 949–968.
Landy, D., & Goldstone, R. L. ( 2007 a). Formal notations are diagrams: Evidence from a production task. Memory and Cognition , 35, 2033–2040.
Landy, D., & Goldstone, R. L. ( 2007 b). How abstract is symbolic thought? Journal of Experimental Psychology: Learning, Memory, and Cognition , 33, 720–733.
Larkin, J. H., McDermott, J., Simon, D. P., & Simon, H. A. ( 1980 ). Models of competence in solving physics problems. Cognitive Science , 4 , 317–345.
Larkin, J. H., & Simon, H. A. ( 1987 ). Why a diagram is (sometimes) worth ten thousand words. Cognitive Science , 11 , 65–99.
Lewis, A. B., & Mayer, R. E. ( 1987 ). students' miscomprehension of relational statements in arithmetic word problems. Journal of Educational Psychology , 79 , 363–371.
Lynch, M. ( 1990 ). The externalized retina: Selection and mathematization in the visual documentation of objects in the life sciences. In M. Lynch & S. Woolgar (Eds.), Representation in scientific practice (pp. 153–186). Cambridge, MA: MIT Press.
MacGregor, J. N., Ormerod, T. C., & Chronicle, E. P. ( 2001 ). Information processing and insight: A process model of performance on the nine-dot and related problems. Journal of Experimental Psychology: Learning, Memory, and Cognition , 27 , 176–201.
Maier, N. ( 1930 ). Reasoning in humans. I. On direction. Journal of Comparative Psychology , 10 , 15–43.
Maier, N. ( 1931 ). Reasoning in humans. II. The solution of a problem and its appearance in consciousness. Journal of Comparative Psychology , 12 , 181–194.
Markman, A. B. ( 1999 ). Knowledge representation . Mahwah, NJ: Erlbaum.
Martin, S. A., & Bassok, M. ( 2005 ). Effects of semantic cues on mathematical modeling: Evidence from word-problem solving and equation construction tasks. Memory and Cognition , 33 , 471–478.
Mayer, R. E., & Gallini, J. K. ( 1990 ). When is an illustration worth ten thousand words? Journal of Educational Psychology , 82 , 715–726.
Mayer, R. E., Griffith, E., Jurkowitz, I. T. N., & Rothman, D. ( 2008 ). Increased interestingness of extraneous details in a multimedia science presentation leads to decreased learning. Journal of Experimental Psychology: Applied , 14 , 329–339.
McKeithen, K. B., Reitman, J. S., Rueter, H. H., & Hirtle, S. C. ( 1981 ). Knowledge organization and skill differences in computer programmers. Cognitive Psychology , 13 , 307–325.
Medin, D. L., & Ross, B. H. ( 1989 ). The specific character of abstract thought: Categorization, problem solving, and induction. In R. J. Sternberg (Ed.), Advances in the psychology of human intelligence (Vol. 5, pp. 189–223). Hillsdale, NJ: Erlbaum.
Moss, J., Kotovsky, K., & Cagan, J. ( 2011 ). The effect of incidental hints when problems are suspended before, during, and after an impasse. Journal of Experimental Psychology: Learning, Memory, and Cognition , 37 , 140–148.
Myles-Worsley, M., Johnston, W. A., & Simons, M. A ( 1988 ). The influence of expertise on X-ray image processing. Journal of Experimental Psychology: Learning, Memory, and Cognition , 14 , 553–557.
Newell, A., & Simon, H. A. ( 1972 ). Human problem solving . Englewood Cliffs, NJ: Prentice-Hall.
Newell, A., & Simon, H. A. ( 1976 ). Computer science as empirical enquiry: Symbols and search. Communications of the ACM , 19 , 113–126.
Novick, L. R. ( 1988 ). Analogical transfer, problem similarity, and expertise. Journal of Experimental Psychology: Learning, Memory, and Cognition , 14 , 510–520.
Novick, L. R. ( 1995 ). Some determinants of successful analogical transfer in the solution of algebra word problems. Thinking and Reasoning , 1 , 5–30.
Novick, L. R., & Catley, K. M. ( 2007 ). Understanding phylogenies in biology: The influence of a Gestalt perceptual principle. Journal of Experimental Psychology: Applied , 13 , 197–223.
Novick, L. R., Catley, K. M., & Funk, D. J. ( 2010 ). Characters are key: The effect of synapomorphies on cladogram comprehension. Evolution: Education and Outreach , 3 , 539–547.
Novick, L. R., & Hmelo, C. E. ( 1994 ). Transferring symbolic representations across non-isomorphic problems. Journal of Experimental Psychology: Learning, Memory, and Cognition , 20 , 1296–1321.
Novick, L. R., & Holyoak, K. J. ( 1991 ). Mathematical problem solving by analogy. Journal of Experimental Psychology: Learning, Memory, and Cognition , 17 , 398–415.
Novick, L. R., & Hurley, S. M. ( 2001 ). To matrix, network, or hierarchy: That is the question. Cognitive Psychology , 42 , 158–216.
Novick, L. R., Shade, C. K., & Catley, K. M. ( 2011 ). Linear versus branching depictions of evolutionary history: Implications for diagram design. Topics in Cognitive Science , 3 (3), 536–559.
Novick, L. R., & Sherman, S. J. ( 2003 ). On the nature of insight solutions: Evidence from skill differences in anagram solution. The Quarterly Journal of Experimental Psychology , 56A , 351–382.
Novick, L. R., & Sherman, S. J. ( 2008 ). The effects of superficial and structural information on on-line problem solving for good versus poor anagram solvers. The Quarterly Journal of Experimental Psychology , 61 , 1098–1120.
Ohlsson, S. ( 1984 ). Restructuring revisited I. Summary and critique of the Gestalt theory of problem solving. Scandinavian Journal of Psychology , 25 , 65–78.
Öllinger, M., Jones, G., & Knoblich, G. ( 2008 ). Investigating the effect of mental set on insight problem solving. Experimental Psychology , 55 , 269–282.
Ormerod, T. C., MacGregor, J. N., & Chronicle, E. P. ( 2002 ). Dynamics and constraints in insight problem solving. Journal of Experimental Psychology: Learning, Memory, and Cognition , 28 , 791–799.
Paige, J. M., & Simon, H. A. ( 1966 ). Cognitive processes in solving algebra word problems. In B. Kleinmuntz (Ed.), Problem solving: Research, method, and theory (pp. 51–119). New York: Wiley
Patel, V. L., Groen, G. J., & Arocha, J. F. ( 1990 ). Medical expertise as a function of task difficulty. Memory and Cognition , 18 , 394–406.
Patsenko, E. G., & Altmann, E. M. ( 2010 ). How planful is routine behavior? A selective attention model of performance in the Tower of Hanoi. Journal of Experimental Psychology: General , 139 , 95–116.
Polya, G. ( 1957 ). How to solve it (2nd ed.). Princeton, NJ: Princeton University Press.
Posner, M. I. ( 1973 ). Cognition: An introduction . Glenview, IL: Scott, Foresman and Company.
Reitman, W. R. ( 1965 ). Cognition and thought . New York: Wiley.
Richland, L. E., & McDonough, I. M. ( 2010 ), Learning by analogy: Discriminating between potential analogs. Contemporary Educational Psychology , 35 , 28–43.
Russo, J. E., Johnson, E. J., & Stephens, D. L. ( 1989 ). The validity of verbal protocols. Memory and Cognition , 17 , 759–769.
Schoenfeld, A. H. ( 1979 ). Explicit heuristic training as a variable in problem-solving performance. Journal for Research in Mathematics Education , 10 , 173–187.
Schoenfeld, A. H., & Herrmann, D. J. ( 1982 ). Problem perception and knowledge structure in expert and novice mathematical problem solvers. Journal of Experimental Psychology: Learning, Memory, and Cognition , 8 , 484–494.
Schwartz, S. H. ( 1971 ). Modes of representation and problem solving: Well evolved is half solved. Journal of Experimental Psychology , 91 , 347–350.
Silver, E. A. ( 1979 ). Student perceptions of relatedness among mathematical verbal problems. Journal for Research in Mathematics Education , 10 , 195–210.
Silver, E. A. ( 1981 ). Recall of mathematical problem information: Solving related problems. Journal for Research in Mathematics Education , 12 , 54–64.
Simon, D. P., & Simon, H. A. ( 1978 ). Individual differences in solving physics problems. In R. Siegler (Ed.), Children's thinking: What develops? (pp. 325–348). Hillsdale, NJ: Erlbaum.
Simon, H. A. ( 1978 ). Information-processing theory of human problem solving. In W. K. Estes (Ed.), Handbook of learning and cognitive processes (Vol. 5, pp. 271–295). Hillsdale, NJ: Erlbaum.
Simon, H. A. ( 1986 ). The information processing explanation of Gestalt Phenomena. Computers in Human Behavior , 2 , 241–255.
Simon, H. A. ( 1990 ). Invariants of human behavior. Annual Review of Psychology , 41 , 1–19.
Son, J. Y., & Goldstone, R. L. ( 2009 ). Fostering general transfer with specific simulations. Pragmatics and Cognition , 17 , 1–42.
Thomas, J. C., Jr., ( 1974 ). An analysis of behavior in the hobbits-orcs problem. Cognitive Psychology , 6 , 257–269.
Weisberg, R. W., & Alba, J. W. ( 1981 ). An examination of the alleged role of “fixation” in the solution of several “insight” problems. Journal of Experimental Psychology: General , 110 , 169–192.
Weiser, M., & Shertz, J. ( 1983 ). Programming problem representation in novice and expert programmers. International Journal of Man-Machine Studies , 19 , 391–398.
Wertheimer, M. ( 1959 ). Productive thinking (Rev. ed.). Chicago, IL: University of Chicago Press.
Winn, W. ( 1989 ). The design and use of instructional graphics. In H. Mandl & J. R. Levin (Eds.), Knowledge acquisition from text and pictures (pp. 125–144). Amsterdam, Netherlands: Elsevier
- About Oxford Academic
- Publish journals with us
- University press partners
- What we publish
- New features
- Open access
- Institutional account management
- Rights and permissions
- Get help with access
- Accessibility
- Advertising
- Media enquiries
- Oxford University Press
- Oxford Languages
- University of Oxford
Oxford University Press is a department of the University of Oxford. It furthers the University's objective of excellence in research, scholarship, and education by publishing worldwide
- Copyright © 2024 Oxford University Press
- Cookie settings
- Cookie policy
- Privacy policy
- Legal notice
This Feature Is Available To Subscribers Only
Sign In or Create an Account
This PDF is available to Subscribers Only
For full access to this pdf, sign in to an existing account, or purchase an annual subscription.
Thank you for visiting nature.com. You are using a browser version with limited support for CSS. To obtain the best experience, we recommend you use a more up to date browser (or turn off compatibility mode in Internet Explorer). In the meantime, to ensure continued support, we are displaying the site without styles and JavaScript.
- View all journals
- My Account Login
- Explore content
- About the journal
- Publish with us
- Sign up for alerts
- Review Article
- Open access
- Published: 11 January 2023
The effectiveness of collaborative problem solving in promoting students’ critical thinking: A meta-analysis based on empirical literature
- Enwei Xu ORCID: orcid.org/0000-0001-6424-8169 1 ,
- Wei Wang 1 &
- Qingxia Wang 1
Humanities and Social Sciences Communications volume 10 , Article number: 16 ( 2023 ) Cite this article
16k Accesses
16 Citations
3 Altmetric
Metrics details
- Science, technology and society
Collaborative problem-solving has been widely embraced in the classroom instruction of critical thinking, which is regarded as the core of curriculum reform based on key competencies in the field of education as well as a key competence for learners in the 21st century. However, the effectiveness of collaborative problem-solving in promoting students’ critical thinking remains uncertain. This current research presents the major findings of a meta-analysis of 36 pieces of the literature revealed in worldwide educational periodicals during the 21st century to identify the effectiveness of collaborative problem-solving in promoting students’ critical thinking and to determine, based on evidence, whether and to what extent collaborative problem solving can result in a rise or decrease in critical thinking. The findings show that (1) collaborative problem solving is an effective teaching approach to foster students’ critical thinking, with a significant overall effect size (ES = 0.82, z = 12.78, P < 0.01, 95% CI [0.69, 0.95]); (2) in respect to the dimensions of critical thinking, collaborative problem solving can significantly and successfully enhance students’ attitudinal tendencies (ES = 1.17, z = 7.62, P < 0.01, 95% CI[0.87, 1.47]); nevertheless, it falls short in terms of improving students’ cognitive skills, having only an upper-middle impact (ES = 0.70, z = 11.55, P < 0.01, 95% CI[0.58, 0.82]); and (3) the teaching type (chi 2 = 7.20, P < 0.05), intervention duration (chi 2 = 12.18, P < 0.01), subject area (chi 2 = 13.36, P < 0.05), group size (chi 2 = 8.77, P < 0.05), and learning scaffold (chi 2 = 9.03, P < 0.01) all have an impact on critical thinking, and they can be viewed as important moderating factors that affect how critical thinking develops. On the basis of these results, recommendations are made for further study and instruction to better support students’ critical thinking in the context of collaborative problem-solving.
Similar content being viewed by others
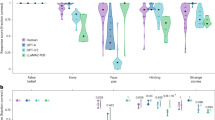
Testing theory of mind in large language models and humans
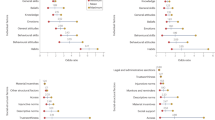
Determinants of behaviour and their efficacy as targets of behavioural change interventions
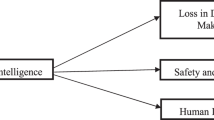
Impact of artificial intelligence on human loss in decision making, laziness and safety in education
Introduction.
Although critical thinking has a long history in research, the concept of critical thinking, which is regarded as an essential competence for learners in the 21st century, has recently attracted more attention from researchers and teaching practitioners (National Research Council, 2012 ). Critical thinking should be the core of curriculum reform based on key competencies in the field of education (Peng and Deng, 2017 ) because students with critical thinking can not only understand the meaning of knowledge but also effectively solve practical problems in real life even after knowledge is forgotten (Kek and Huijser, 2011 ). The definition of critical thinking is not universal (Ennis, 1989 ; Castle, 2009 ; Niu et al., 2013 ). In general, the definition of critical thinking is a self-aware and self-regulated thought process (Facione, 1990 ; Niu et al., 2013 ). It refers to the cognitive skills needed to interpret, analyze, synthesize, reason, and evaluate information as well as the attitudinal tendency to apply these abilities (Halpern, 2001 ). The view that critical thinking can be taught and learned through curriculum teaching has been widely supported by many researchers (e.g., Kuncel, 2011 ; Leng and Lu, 2020 ), leading to educators’ efforts to foster it among students. In the field of teaching practice, there are three types of courses for teaching critical thinking (Ennis, 1989 ). The first is an independent curriculum in which critical thinking is taught and cultivated without involving the knowledge of specific disciplines; the second is an integrated curriculum in which critical thinking is integrated into the teaching of other disciplines as a clear teaching goal; and the third is a mixed curriculum in which critical thinking is taught in parallel to the teaching of other disciplines for mixed teaching training. Furthermore, numerous measuring tools have been developed by researchers and educators to measure critical thinking in the context of teaching practice. These include standardized measurement tools, such as WGCTA, CCTST, CCTT, and CCTDI, which have been verified by repeated experiments and are considered effective and reliable by international scholars (Facione and Facione, 1992 ). In short, descriptions of critical thinking, including its two dimensions of attitudinal tendency and cognitive skills, different types of teaching courses, and standardized measurement tools provide a complex normative framework for understanding, teaching, and evaluating critical thinking.
Cultivating critical thinking in curriculum teaching can start with a problem, and one of the most popular critical thinking instructional approaches is problem-based learning (Liu et al., 2020 ). Duch et al. ( 2001 ) noted that problem-based learning in group collaboration is progressive active learning, which can improve students’ critical thinking and problem-solving skills. Collaborative problem-solving is the organic integration of collaborative learning and problem-based learning, which takes learners as the center of the learning process and uses problems with poor structure in real-world situations as the starting point for the learning process (Liang et al., 2017 ). Students learn the knowledge needed to solve problems in a collaborative group, reach a consensus on problems in the field, and form solutions through social cooperation methods, such as dialogue, interpretation, questioning, debate, negotiation, and reflection, thus promoting the development of learners’ domain knowledge and critical thinking (Cindy, 2004 ; Liang et al., 2017 ).
Collaborative problem-solving has been widely used in the teaching practice of critical thinking, and several studies have attempted to conduct a systematic review and meta-analysis of the empirical literature on critical thinking from various perspectives. However, little attention has been paid to the impact of collaborative problem-solving on critical thinking. Therefore, the best approach for developing and enhancing critical thinking throughout collaborative problem-solving is to examine how to implement critical thinking instruction; however, this issue is still unexplored, which means that many teachers are incapable of better instructing critical thinking (Leng and Lu, 2020 ; Niu et al., 2013 ). For example, Huber ( 2016 ) provided the meta-analysis findings of 71 publications on gaining critical thinking over various time frames in college with the aim of determining whether critical thinking was truly teachable. These authors found that learners significantly improve their critical thinking while in college and that critical thinking differs with factors such as teaching strategies, intervention duration, subject area, and teaching type. The usefulness of collaborative problem-solving in fostering students’ critical thinking, however, was not determined by this study, nor did it reveal whether there existed significant variations among the different elements. A meta-analysis of 31 pieces of educational literature was conducted by Liu et al. ( 2020 ) to assess the impact of problem-solving on college students’ critical thinking. These authors found that problem-solving could promote the development of critical thinking among college students and proposed establishing a reasonable group structure for problem-solving in a follow-up study to improve students’ critical thinking. Additionally, previous empirical studies have reached inconclusive and even contradictory conclusions about whether and to what extent collaborative problem-solving increases or decreases critical thinking levels. As an illustration, Yang et al. ( 2008 ) carried out an experiment on the integrated curriculum teaching of college students based on a web bulletin board with the goal of fostering participants’ critical thinking in the context of collaborative problem-solving. These authors’ research revealed that through sharing, debating, examining, and reflecting on various experiences and ideas, collaborative problem-solving can considerably enhance students’ critical thinking in real-life problem situations. In contrast, collaborative problem-solving had a positive impact on learners’ interaction and could improve learning interest and motivation but could not significantly improve students’ critical thinking when compared to traditional classroom teaching, according to research by Naber and Wyatt ( 2014 ) and Sendag and Odabasi ( 2009 ) on undergraduate and high school students, respectively.
The above studies show that there is inconsistency regarding the effectiveness of collaborative problem-solving in promoting students’ critical thinking. Therefore, it is essential to conduct a thorough and trustworthy review to detect and decide whether and to what degree collaborative problem-solving can result in a rise or decrease in critical thinking. Meta-analysis is a quantitative analysis approach that is utilized to examine quantitative data from various separate studies that are all focused on the same research topic. This approach characterizes the effectiveness of its impact by averaging the effect sizes of numerous qualitative studies in an effort to reduce the uncertainty brought on by independent research and produce more conclusive findings (Lipsey and Wilson, 2001 ).
This paper used a meta-analytic approach and carried out a meta-analysis to examine the effectiveness of collaborative problem-solving in promoting students’ critical thinking in order to make a contribution to both research and practice. The following research questions were addressed by this meta-analysis:
What is the overall effect size of collaborative problem-solving in promoting students’ critical thinking and its impact on the two dimensions of critical thinking (i.e., attitudinal tendency and cognitive skills)?
How are the disparities between the study conclusions impacted by various moderating variables if the impacts of various experimental designs in the included studies are heterogeneous?
This research followed the strict procedures (e.g., database searching, identification, screening, eligibility, merging, duplicate removal, and analysis of included studies) of Cooper’s ( 2010 ) proposed meta-analysis approach for examining quantitative data from various separate studies that are all focused on the same research topic. The relevant empirical research that appeared in worldwide educational periodicals within the 21st century was subjected to this meta-analysis using Rev-Man 5.4. The consistency of the data extracted separately by two researchers was tested using Cohen’s kappa coefficient, and a publication bias test and a heterogeneity test were run on the sample data to ascertain the quality of this meta-analysis.
Data sources and search strategies
There were three stages to the data collection process for this meta-analysis, as shown in Fig. 1 , which shows the number of articles included and eliminated during the selection process based on the statement and study eligibility criteria.
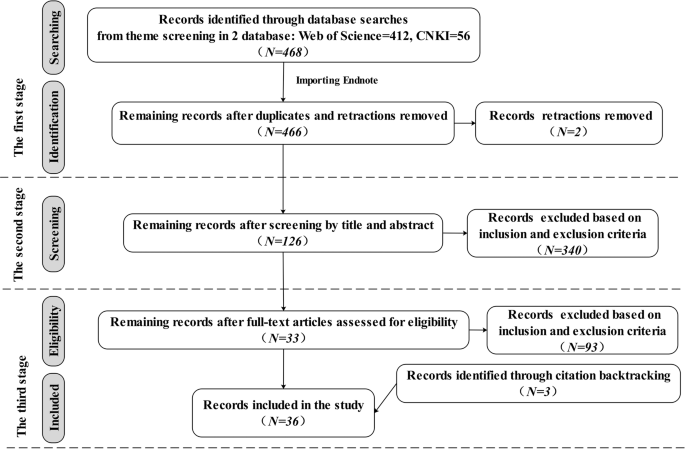
This flowchart shows the number of records identified, included and excluded in the article.
First, the databases used to systematically search for relevant articles were the journal papers of the Web of Science Core Collection and the Chinese Core source journal, as well as the Chinese Social Science Citation Index (CSSCI) source journal papers included in CNKI. These databases were selected because they are credible platforms that are sources of scholarly and peer-reviewed information with advanced search tools and contain literature relevant to the subject of our topic from reliable researchers and experts. The search string with the Boolean operator used in the Web of Science was “TS = (((“critical thinking” or “ct” and “pretest” or “posttest”) or (“critical thinking” or “ct” and “control group” or “quasi experiment” or “experiment”)) and (“collaboration” or “collaborative learning” or “CSCL”) and (“problem solving” or “problem-based learning” or “PBL”))”. The research area was “Education Educational Research”, and the search period was “January 1, 2000, to December 30, 2021”. A total of 412 papers were obtained. The search string with the Boolean operator used in the CNKI was “SU = (‘critical thinking’*‘collaboration’ + ‘critical thinking’*‘collaborative learning’ + ‘critical thinking’*‘CSCL’ + ‘critical thinking’*‘problem solving’ + ‘critical thinking’*‘problem-based learning’ + ‘critical thinking’*‘PBL’ + ‘critical thinking’*‘problem oriented’) AND FT = (‘experiment’ + ‘quasi experiment’ + ‘pretest’ + ‘posttest’ + ‘empirical study’)” (translated into Chinese when searching). A total of 56 studies were found throughout the search period of “January 2000 to December 2021”. From the databases, all duplicates and retractions were eliminated before exporting the references into Endnote, a program for managing bibliographic references. In all, 466 studies were found.
Second, the studies that matched the inclusion and exclusion criteria for the meta-analysis were chosen by two researchers after they had reviewed the abstracts and titles of the gathered articles, yielding a total of 126 studies.
Third, two researchers thoroughly reviewed each included article’s whole text in accordance with the inclusion and exclusion criteria. Meanwhile, a snowball search was performed using the references and citations of the included articles to ensure complete coverage of the articles. Ultimately, 36 articles were kept.
Two researchers worked together to carry out this entire process, and a consensus rate of almost 94.7% was reached after discussion and negotiation to clarify any emerging differences.
Eligibility criteria
Since not all the retrieved studies matched the criteria for this meta-analysis, eligibility criteria for both inclusion and exclusion were developed as follows:
The publication language of the included studies was limited to English and Chinese, and the full text could be obtained. Articles that did not meet the publication language and articles not published between 2000 and 2021 were excluded.
The research design of the included studies must be empirical and quantitative studies that can assess the effect of collaborative problem-solving on the development of critical thinking. Articles that could not identify the causal mechanisms by which collaborative problem-solving affects critical thinking, such as review articles and theoretical articles, were excluded.
The research method of the included studies must feature a randomized control experiment or a quasi-experiment, or a natural experiment, which have a higher degree of internal validity with strong experimental designs and can all plausibly provide evidence that critical thinking and collaborative problem-solving are causally related. Articles with non-experimental research methods, such as purely correlational or observational studies, were excluded.
The participants of the included studies were only students in school, including K-12 students and college students. Articles in which the participants were non-school students, such as social workers or adult learners, were excluded.
The research results of the included studies must mention definite signs that may be utilized to gauge critical thinking’s impact (e.g., sample size, mean value, or standard deviation). Articles that lacked specific measurement indicators for critical thinking and could not calculate the effect size were excluded.
Data coding design
In order to perform a meta-analysis, it is necessary to collect the most important information from the articles, codify that information’s properties, and convert descriptive data into quantitative data. Therefore, this study designed a data coding template (see Table 1 ). Ultimately, 16 coding fields were retained.
The designed data-coding template consisted of three pieces of information. Basic information about the papers was included in the descriptive information: the publishing year, author, serial number, and title of the paper.
The variable information for the experimental design had three variables: the independent variable (instruction method), the dependent variable (critical thinking), and the moderating variable (learning stage, teaching type, intervention duration, learning scaffold, group size, measuring tool, and subject area). Depending on the topic of this study, the intervention strategy, as the independent variable, was coded into collaborative and non-collaborative problem-solving. The dependent variable, critical thinking, was coded as a cognitive skill and an attitudinal tendency. And seven moderating variables were created by grouping and combining the experimental design variables discovered within the 36 studies (see Table 1 ), where learning stages were encoded as higher education, high school, middle school, and primary school or lower; teaching types were encoded as mixed courses, integrated courses, and independent courses; intervention durations were encoded as 0–1 weeks, 1–4 weeks, 4–12 weeks, and more than 12 weeks; group sizes were encoded as 2–3 persons, 4–6 persons, 7–10 persons, and more than 10 persons; learning scaffolds were encoded as teacher-supported learning scaffold, technique-supported learning scaffold, and resource-supported learning scaffold; measuring tools were encoded as standardized measurement tools (e.g., WGCTA, CCTT, CCTST, and CCTDI) and self-adapting measurement tools (e.g., modified or made by researchers); and subject areas were encoded according to the specific subjects used in the 36 included studies.
The data information contained three metrics for measuring critical thinking: sample size, average value, and standard deviation. It is vital to remember that studies with various experimental designs frequently adopt various formulas to determine the effect size. And this paper used Morris’ proposed standardized mean difference (SMD) calculation formula ( 2008 , p. 369; see Supplementary Table S3 ).
Procedure for extracting and coding data
According to the data coding template (see Table 1 ), the 36 papers’ information was retrieved by two researchers, who then entered them into Excel (see Supplementary Table S1 ). The results of each study were extracted separately in the data extraction procedure if an article contained numerous studies on critical thinking, or if a study assessed different critical thinking dimensions. For instance, Tiwari et al. ( 2010 ) used four time points, which were viewed as numerous different studies, to examine the outcomes of critical thinking, and Chen ( 2013 ) included the two outcome variables of attitudinal tendency and cognitive skills, which were regarded as two studies. After discussion and negotiation during data extraction, the two researchers’ consistency test coefficients were roughly 93.27%. Supplementary Table S2 details the key characteristics of the 36 included articles with 79 effect quantities, including descriptive information (e.g., the publishing year, author, serial number, and title of the paper), variable information (e.g., independent variables, dependent variables, and moderating variables), and data information (e.g., mean values, standard deviations, and sample size). Following that, testing for publication bias and heterogeneity was done on the sample data using the Rev-Man 5.4 software, and then the test results were used to conduct a meta-analysis.
Publication bias test
When the sample of studies included in a meta-analysis does not accurately reflect the general status of research on the relevant subject, publication bias is said to be exhibited in this research. The reliability and accuracy of the meta-analysis may be impacted by publication bias. Due to this, the meta-analysis needs to check the sample data for publication bias (Stewart et al., 2006 ). A popular method to check for publication bias is the funnel plot; and it is unlikely that there will be publishing bias when the data are equally dispersed on either side of the average effect size and targeted within the higher region. The data are equally dispersed within the higher portion of the efficient zone, consistent with the funnel plot connected with this analysis (see Fig. 2 ), indicating that publication bias is unlikely in this situation.
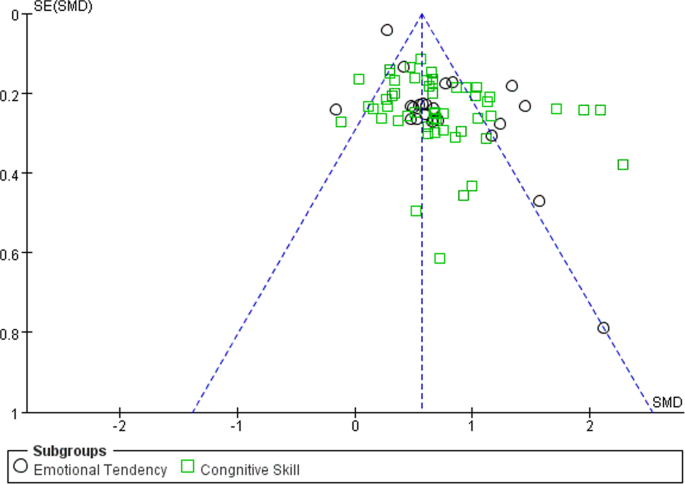
This funnel plot shows the result of publication bias of 79 effect quantities across 36 studies.
Heterogeneity test
To select the appropriate effect models for the meta-analysis, one might use the results of a heterogeneity test on the data effect sizes. In a meta-analysis, it is common practice to gauge the degree of data heterogeneity using the I 2 value, and I 2 ≥ 50% is typically understood to denote medium-high heterogeneity, which calls for the adoption of a random effect model; if not, a fixed effect model ought to be applied (Lipsey and Wilson, 2001 ). The findings of the heterogeneity test in this paper (see Table 2 ) revealed that I 2 was 86% and displayed significant heterogeneity ( P < 0.01). To ensure accuracy and reliability, the overall effect size ought to be calculated utilizing the random effect model.
The analysis of the overall effect size
This meta-analysis utilized a random effect model to examine 79 effect quantities from 36 studies after eliminating heterogeneity. In accordance with Cohen’s criterion (Cohen, 1992 ), it is abundantly clear from the analysis results, which are shown in the forest plot of the overall effect (see Fig. 3 ), that the cumulative impact size of cooperative problem-solving is 0.82, which is statistically significant ( z = 12.78, P < 0.01, 95% CI [0.69, 0.95]), and can encourage learners to practice critical thinking.
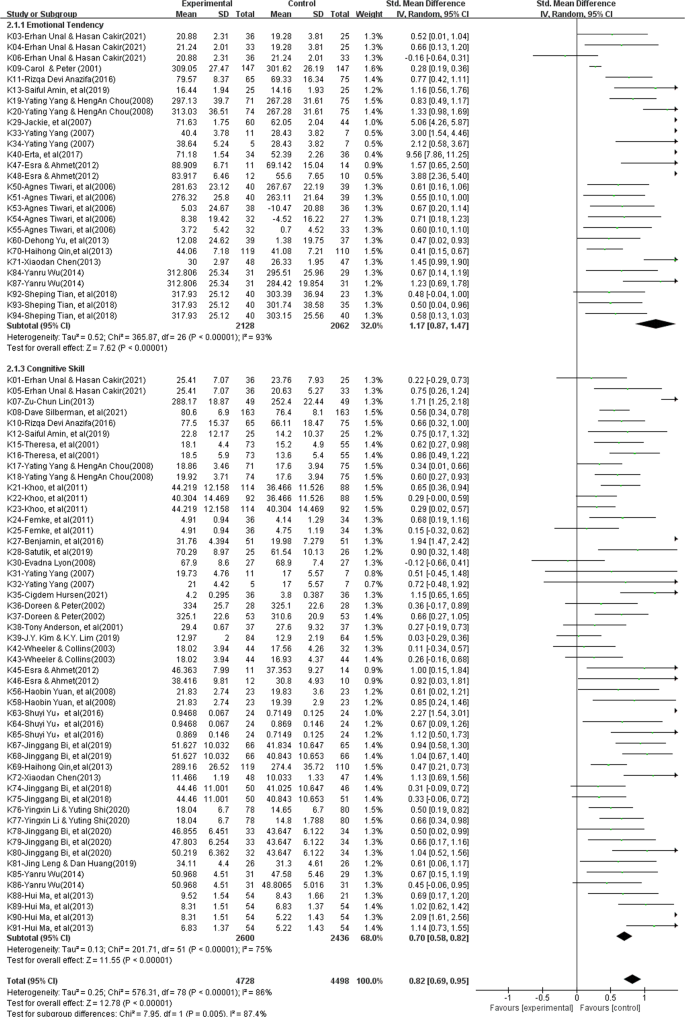
This forest plot shows the analysis result of the overall effect size across 36 studies.
In addition, this study examined two distinct dimensions of critical thinking to better understand the precise contributions that collaborative problem-solving makes to the growth of critical thinking. The findings (see Table 3 ) indicate that collaborative problem-solving improves cognitive skills (ES = 0.70) and attitudinal tendency (ES = 1.17), with significant intergroup differences (chi 2 = 7.95, P < 0.01). Although collaborative problem-solving improves both dimensions of critical thinking, it is essential to point out that the improvements in students’ attitudinal tendency are much more pronounced and have a significant comprehensive effect (ES = 1.17, z = 7.62, P < 0.01, 95% CI [0.87, 1.47]), whereas gains in learners’ cognitive skill are slightly improved and are just above average. (ES = 0.70, z = 11.55, P < 0.01, 95% CI [0.58, 0.82]).
The analysis of moderator effect size
The whole forest plot’s 79 effect quantities underwent a two-tailed test, which revealed significant heterogeneity ( I 2 = 86%, z = 12.78, P < 0.01), indicating differences between various effect sizes that may have been influenced by moderating factors other than sampling error. Therefore, exploring possible moderating factors that might produce considerable heterogeneity was done using subgroup analysis, such as the learning stage, learning scaffold, teaching type, group size, duration of the intervention, measuring tool, and the subject area included in the 36 experimental designs, in order to further explore the key factors that influence critical thinking. The findings (see Table 4 ) indicate that various moderating factors have advantageous effects on critical thinking. In this situation, the subject area (chi 2 = 13.36, P < 0.05), group size (chi 2 = 8.77, P < 0.05), intervention duration (chi 2 = 12.18, P < 0.01), learning scaffold (chi 2 = 9.03, P < 0.01), and teaching type (chi 2 = 7.20, P < 0.05) are all significant moderators that can be applied to support the cultivation of critical thinking. However, since the learning stage and the measuring tools did not significantly differ among intergroup (chi 2 = 3.15, P = 0.21 > 0.05, and chi 2 = 0.08, P = 0.78 > 0.05), we are unable to explain why these two factors are crucial in supporting the cultivation of critical thinking in the context of collaborative problem-solving. These are the precise outcomes, as follows:
Various learning stages influenced critical thinking positively, without significant intergroup differences (chi 2 = 3.15, P = 0.21 > 0.05). High school was first on the list of effect sizes (ES = 1.36, P < 0.01), then higher education (ES = 0.78, P < 0.01), and middle school (ES = 0.73, P < 0.01). These results show that, despite the learning stage’s beneficial influence on cultivating learners’ critical thinking, we are unable to explain why it is essential for cultivating critical thinking in the context of collaborative problem-solving.
Different teaching types had varying degrees of positive impact on critical thinking, with significant intergroup differences (chi 2 = 7.20, P < 0.05). The effect size was ranked as follows: mixed courses (ES = 1.34, P < 0.01), integrated courses (ES = 0.81, P < 0.01), and independent courses (ES = 0.27, P < 0.01). These results indicate that the most effective approach to cultivate critical thinking utilizing collaborative problem solving is through the teaching type of mixed courses.
Various intervention durations significantly improved critical thinking, and there were significant intergroup differences (chi 2 = 12.18, P < 0.01). The effect sizes related to this variable showed a tendency to increase with longer intervention durations. The improvement in critical thinking reached a significant level (ES = 0.85, P < 0.01) after more than 12 weeks of training. These findings indicate that the intervention duration and critical thinking’s impact are positively correlated, with a longer intervention duration having a greater effect.
Different learning scaffolds influenced critical thinking positively, with significant intergroup differences (chi 2 = 9.03, P < 0.01). The resource-supported learning scaffold (ES = 0.69, P < 0.01) acquired a medium-to-higher level of impact, the technique-supported learning scaffold (ES = 0.63, P < 0.01) also attained a medium-to-higher level of impact, and the teacher-supported learning scaffold (ES = 0.92, P < 0.01) displayed a high level of significant impact. These results show that the learning scaffold with teacher support has the greatest impact on cultivating critical thinking.
Various group sizes influenced critical thinking positively, and the intergroup differences were statistically significant (chi 2 = 8.77, P < 0.05). Critical thinking showed a general declining trend with increasing group size. The overall effect size of 2–3 people in this situation was the biggest (ES = 0.99, P < 0.01), and when the group size was greater than 7 people, the improvement in critical thinking was at the lower-middle level (ES < 0.5, P < 0.01). These results show that the impact on critical thinking is positively connected with group size, and as group size grows, so does the overall impact.
Various measuring tools influenced critical thinking positively, with significant intergroup differences (chi 2 = 0.08, P = 0.78 > 0.05). In this situation, the self-adapting measurement tools obtained an upper-medium level of effect (ES = 0.78), whereas the complete effect size of the standardized measurement tools was the largest, achieving a significant level of effect (ES = 0.84, P < 0.01). These results show that, despite the beneficial influence of the measuring tool on cultivating critical thinking, we are unable to explain why it is crucial in fostering the growth of critical thinking by utilizing the approach of collaborative problem-solving.
Different subject areas had a greater impact on critical thinking, and the intergroup differences were statistically significant (chi 2 = 13.36, P < 0.05). Mathematics had the greatest overall impact, achieving a significant level of effect (ES = 1.68, P < 0.01), followed by science (ES = 1.25, P < 0.01) and medical science (ES = 0.87, P < 0.01), both of which also achieved a significant level of effect. Programming technology was the least effective (ES = 0.39, P < 0.01), only having a medium-low degree of effect compared to education (ES = 0.72, P < 0.01) and other fields (such as language, art, and social sciences) (ES = 0.58, P < 0.01). These results suggest that scientific fields (e.g., mathematics, science) may be the most effective subject areas for cultivating critical thinking utilizing the approach of collaborative problem-solving.
The effectiveness of collaborative problem solving with regard to teaching critical thinking
According to this meta-analysis, using collaborative problem-solving as an intervention strategy in critical thinking teaching has a considerable amount of impact on cultivating learners’ critical thinking as a whole and has a favorable promotional effect on the two dimensions of critical thinking. According to certain studies, collaborative problem solving, the most frequently used critical thinking teaching strategy in curriculum instruction can considerably enhance students’ critical thinking (e.g., Liang et al., 2017 ; Liu et al., 2020 ; Cindy, 2004 ). This meta-analysis provides convergent data support for the above research views. Thus, the findings of this meta-analysis not only effectively address the first research query regarding the overall effect of cultivating critical thinking and its impact on the two dimensions of critical thinking (i.e., attitudinal tendency and cognitive skills) utilizing the approach of collaborative problem-solving, but also enhance our confidence in cultivating critical thinking by using collaborative problem-solving intervention approach in the context of classroom teaching.
Furthermore, the associated improvements in attitudinal tendency are much stronger, but the corresponding improvements in cognitive skill are only marginally better. According to certain studies, cognitive skill differs from the attitudinal tendency in classroom instruction; the cultivation and development of the former as a key ability is a process of gradual accumulation, while the latter as an attitude is affected by the context of the teaching situation (e.g., a novel and exciting teaching approach, challenging and rewarding tasks) (Halpern, 2001 ; Wei and Hong, 2022 ). Collaborative problem-solving as a teaching approach is exciting and interesting, as well as rewarding and challenging; because it takes the learners as the focus and examines problems with poor structure in real situations, and it can inspire students to fully realize their potential for problem-solving, which will significantly improve their attitudinal tendency toward solving problems (Liu et al., 2020 ). Similar to how collaborative problem-solving influences attitudinal tendency, attitudinal tendency impacts cognitive skill when attempting to solve a problem (Liu et al., 2020 ; Zhang et al., 2022 ), and stronger attitudinal tendencies are associated with improved learning achievement and cognitive ability in students (Sison, 2008 ; Zhang et al., 2022 ). It can be seen that the two specific dimensions of critical thinking as well as critical thinking as a whole are affected by collaborative problem-solving, and this study illuminates the nuanced links between cognitive skills and attitudinal tendencies with regard to these two dimensions of critical thinking. To fully develop students’ capacity for critical thinking, future empirical research should pay closer attention to cognitive skills.
The moderating effects of collaborative problem solving with regard to teaching critical thinking
In order to further explore the key factors that influence critical thinking, exploring possible moderating effects that might produce considerable heterogeneity was done using subgroup analysis. The findings show that the moderating factors, such as the teaching type, learning stage, group size, learning scaffold, duration of the intervention, measuring tool, and the subject area included in the 36 experimental designs, could all support the cultivation of collaborative problem-solving in critical thinking. Among them, the effect size differences between the learning stage and measuring tool are not significant, which does not explain why these two factors are crucial in supporting the cultivation of critical thinking utilizing the approach of collaborative problem-solving.
In terms of the learning stage, various learning stages influenced critical thinking positively without significant intergroup differences, indicating that we are unable to explain why it is crucial in fostering the growth of critical thinking.
Although high education accounts for 70.89% of all empirical studies performed by researchers, high school may be the appropriate learning stage to foster students’ critical thinking by utilizing the approach of collaborative problem-solving since it has the largest overall effect size. This phenomenon may be related to student’s cognitive development, which needs to be further studied in follow-up research.
With regard to teaching type, mixed course teaching may be the best teaching method to cultivate students’ critical thinking. Relevant studies have shown that in the actual teaching process if students are trained in thinking methods alone, the methods they learn are isolated and divorced from subject knowledge, which is not conducive to their transfer of thinking methods; therefore, if students’ thinking is trained only in subject teaching without systematic method training, it is challenging to apply to real-world circumstances (Ruggiero, 2012 ; Hu and Liu, 2015 ). Teaching critical thinking as mixed course teaching in parallel to other subject teachings can achieve the best effect on learners’ critical thinking, and explicit critical thinking instruction is more effective than less explicit critical thinking instruction (Bensley and Spero, 2014 ).
In terms of the intervention duration, with longer intervention times, the overall effect size shows an upward tendency. Thus, the intervention duration and critical thinking’s impact are positively correlated. Critical thinking, as a key competency for students in the 21st century, is difficult to get a meaningful improvement in a brief intervention duration. Instead, it could be developed over a lengthy period of time through consistent teaching and the progressive accumulation of knowledge (Halpern, 2001 ; Hu and Liu, 2015 ). Therefore, future empirical studies ought to take these restrictions into account throughout a longer period of critical thinking instruction.
With regard to group size, a group size of 2–3 persons has the highest effect size, and the comprehensive effect size decreases with increasing group size in general. This outcome is in line with some research findings; as an example, a group composed of two to four members is most appropriate for collaborative learning (Schellens and Valcke, 2006 ). However, the meta-analysis results also indicate that once the group size exceeds 7 people, small groups cannot produce better interaction and performance than large groups. This may be because the learning scaffolds of technique support, resource support, and teacher support improve the frequency and effectiveness of interaction among group members, and a collaborative group with more members may increase the diversity of views, which is helpful to cultivate critical thinking utilizing the approach of collaborative problem-solving.
With regard to the learning scaffold, the three different kinds of learning scaffolds can all enhance critical thinking. Among them, the teacher-supported learning scaffold has the largest overall effect size, demonstrating the interdependence of effective learning scaffolds and collaborative problem-solving. This outcome is in line with some research findings; as an example, a successful strategy is to encourage learners to collaborate, come up with solutions, and develop critical thinking skills by using learning scaffolds (Reiser, 2004 ; Xu et al., 2022 ); learning scaffolds can lower task complexity and unpleasant feelings while also enticing students to engage in learning activities (Wood et al., 2006 ); learning scaffolds are designed to assist students in using learning approaches more successfully to adapt the collaborative problem-solving process, and the teacher-supported learning scaffolds have the greatest influence on critical thinking in this process because they are more targeted, informative, and timely (Xu et al., 2022 ).
With respect to the measuring tool, despite the fact that standardized measurement tools (such as the WGCTA, CCTT, and CCTST) have been acknowledged as trustworthy and effective by worldwide experts, only 54.43% of the research included in this meta-analysis adopted them for assessment, and the results indicated no intergroup differences. These results suggest that not all teaching circumstances are appropriate for measuring critical thinking using standardized measurement tools. “The measuring tools for measuring thinking ability have limits in assessing learners in educational situations and should be adapted appropriately to accurately assess the changes in learners’ critical thinking.”, according to Simpson and Courtney ( 2002 , p. 91). As a result, in order to more fully and precisely gauge how learners’ critical thinking has evolved, we must properly modify standardized measuring tools based on collaborative problem-solving learning contexts.
With regard to the subject area, the comprehensive effect size of science departments (e.g., mathematics, science, medical science) is larger than that of language arts and social sciences. Some recent international education reforms have noted that critical thinking is a basic part of scientific literacy. Students with scientific literacy can prove the rationality of their judgment according to accurate evidence and reasonable standards when they face challenges or poorly structured problems (Kyndt et al., 2013 ), which makes critical thinking crucial for developing scientific understanding and applying this understanding to practical problem solving for problems related to science, technology, and society (Yore et al., 2007 ).
Suggestions for critical thinking teaching
Other than those stated in the discussion above, the following suggestions are offered for critical thinking instruction utilizing the approach of collaborative problem-solving.
First, teachers should put a special emphasis on the two core elements, which are collaboration and problem-solving, to design real problems based on collaborative situations. This meta-analysis provides evidence to support the view that collaborative problem-solving has a strong synergistic effect on promoting students’ critical thinking. Asking questions about real situations and allowing learners to take part in critical discussions on real problems during class instruction are key ways to teach critical thinking rather than simply reading speculative articles without practice (Mulnix, 2012 ). Furthermore, the improvement of students’ critical thinking is realized through cognitive conflict with other learners in the problem situation (Yang et al., 2008 ). Consequently, it is essential for teachers to put a special emphasis on the two core elements, which are collaboration and problem-solving, and design real problems and encourage students to discuss, negotiate, and argue based on collaborative problem-solving situations.
Second, teachers should design and implement mixed courses to cultivate learners’ critical thinking, utilizing the approach of collaborative problem-solving. Critical thinking can be taught through curriculum instruction (Kuncel, 2011 ; Leng and Lu, 2020 ), with the goal of cultivating learners’ critical thinking for flexible transfer and application in real problem-solving situations. This meta-analysis shows that mixed course teaching has a highly substantial impact on the cultivation and promotion of learners’ critical thinking. Therefore, teachers should design and implement mixed course teaching with real collaborative problem-solving situations in combination with the knowledge content of specific disciplines in conventional teaching, teach methods and strategies of critical thinking based on poorly structured problems to help students master critical thinking, and provide practical activities in which students can interact with each other to develop knowledge construction and critical thinking utilizing the approach of collaborative problem-solving.
Third, teachers should be more trained in critical thinking, particularly preservice teachers, and they also should be conscious of the ways in which teachers’ support for learning scaffolds can promote critical thinking. The learning scaffold supported by teachers had the greatest impact on learners’ critical thinking, in addition to being more directive, targeted, and timely (Wood et al., 2006 ). Critical thinking can only be effectively taught when teachers recognize the significance of critical thinking for students’ growth and use the proper approaches while designing instructional activities (Forawi, 2016 ). Therefore, with the intention of enabling teachers to create learning scaffolds to cultivate learners’ critical thinking utilizing the approach of collaborative problem solving, it is essential to concentrate on the teacher-supported learning scaffolds and enhance the instruction for teaching critical thinking to teachers, especially preservice teachers.
Implications and limitations
There are certain limitations in this meta-analysis, but future research can correct them. First, the search languages were restricted to English and Chinese, so it is possible that pertinent studies that were written in other languages were overlooked, resulting in an inadequate number of articles for review. Second, these data provided by the included studies are partially missing, such as whether teachers were trained in the theory and practice of critical thinking, the average age and gender of learners, and the differences in critical thinking among learners of various ages and genders. Third, as is typical for review articles, more studies were released while this meta-analysis was being done; therefore, it had a time limit. With the development of relevant research, future studies focusing on these issues are highly relevant and needed.
Conclusions
The subject of the magnitude of collaborative problem-solving’s impact on fostering students’ critical thinking, which received scant attention from other studies, was successfully addressed by this study. The question of the effectiveness of collaborative problem-solving in promoting students’ critical thinking was addressed in this study, which addressed a topic that had gotten little attention in earlier research. The following conclusions can be made:
Regarding the results obtained, collaborative problem solving is an effective teaching approach to foster learners’ critical thinking, with a significant overall effect size (ES = 0.82, z = 12.78, P < 0.01, 95% CI [0.69, 0.95]). With respect to the dimensions of critical thinking, collaborative problem-solving can significantly and effectively improve students’ attitudinal tendency, and the comprehensive effect is significant (ES = 1.17, z = 7.62, P < 0.01, 95% CI [0.87, 1.47]); nevertheless, it falls short in terms of improving students’ cognitive skills, having only an upper-middle impact (ES = 0.70, z = 11.55, P < 0.01, 95% CI [0.58, 0.82]).
As demonstrated by both the results and the discussion, there are varying degrees of beneficial effects on students’ critical thinking from all seven moderating factors, which were found across 36 studies. In this context, the teaching type (chi 2 = 7.20, P < 0.05), intervention duration (chi 2 = 12.18, P < 0.01), subject area (chi 2 = 13.36, P < 0.05), group size (chi 2 = 8.77, P < 0.05), and learning scaffold (chi 2 = 9.03, P < 0.01) all have a positive impact on critical thinking, and they can be viewed as important moderating factors that affect how critical thinking develops. Since the learning stage (chi 2 = 3.15, P = 0.21 > 0.05) and measuring tools (chi 2 = 0.08, P = 0.78 > 0.05) did not demonstrate any significant intergroup differences, we are unable to explain why these two factors are crucial in supporting the cultivation of critical thinking in the context of collaborative problem-solving.
Data availability
All data generated or analyzed during this study are included within the article and its supplementary information files, and the supplementary information files are available in the Dataverse repository: https://doi.org/10.7910/DVN/IPFJO6 .
Bensley DA, Spero RA (2014) Improving critical thinking skills and meta-cognitive monitoring through direct infusion. Think Skills Creat 12:55–68. https://doi.org/10.1016/j.tsc.2014.02.001
Article Google Scholar
Castle A (2009) Defining and assessing critical thinking skills for student radiographers. Radiography 15(1):70–76. https://doi.org/10.1016/j.radi.2007.10.007
Chen XD (2013) An empirical study on the influence of PBL teaching model on critical thinking ability of non-English majors. J PLA Foreign Lang College 36 (04):68–72
Google Scholar
Cohen A (1992) Antecedents of organizational commitment across occupational groups: a meta-analysis. J Organ Behav. https://doi.org/10.1002/job.4030130602
Cooper H (2010) Research synthesis and meta-analysis: a step-by-step approach, 4th edn. Sage, London, England
Cindy HS (2004) Problem-based learning: what and how do students learn? Educ Psychol Rev 51(1):31–39
Duch BJ, Gron SD, Allen DE (2001) The power of problem-based learning: a practical “how to” for teaching undergraduate courses in any discipline. Stylus Educ Sci 2:190–198
Ennis RH (1989) Critical thinking and subject specificity: clarification and needed research. Educ Res 18(3):4–10. https://doi.org/10.3102/0013189x018003004
Facione PA (1990) Critical thinking: a statement of expert consensus for purposes of educational assessment and instruction. Research findings and recommendations. Eric document reproduction service. https://eric.ed.gov/?id=ed315423
Facione PA, Facione NC (1992) The California Critical Thinking Dispositions Inventory (CCTDI) and the CCTDI test manual. California Academic Press, Millbrae, CA
Forawi SA (2016) Standard-based science education and critical thinking. Think Skills Creat 20:52–62. https://doi.org/10.1016/j.tsc.2016.02.005
Halpern DF (2001) Assessing the effectiveness of critical thinking instruction. J Gen Educ 50(4):270–286. https://doi.org/10.2307/27797889
Hu WP, Liu J (2015) Cultivation of pupils’ thinking ability: a five-year follow-up study. Psychol Behav Res 13(05):648–654. https://doi.org/10.3969/j.issn.1672-0628.2015.05.010
Huber K (2016) Does college teach critical thinking? A meta-analysis. Rev Educ Res 86(2):431–468. https://doi.org/10.3102/0034654315605917
Kek MYCA, Huijser H (2011) The power of problem-based learning in developing critical thinking skills: preparing students for tomorrow’s digital futures in today’s classrooms. High Educ Res Dev 30(3):329–341. https://doi.org/10.1080/07294360.2010.501074
Kuncel NR (2011) Measurement and meaning of critical thinking (Research report for the NRC 21st Century Skills Workshop). National Research Council, Washington, DC
Kyndt E, Raes E, Lismont B, Timmers F, Cascallar E, Dochy F (2013) A meta-analysis of the effects of face-to-face cooperative learning. Do recent studies falsify or verify earlier findings? Educ Res Rev 10(2):133–149. https://doi.org/10.1016/j.edurev.2013.02.002
Leng J, Lu XX (2020) Is critical thinking really teachable?—A meta-analysis based on 79 experimental or quasi experimental studies. Open Educ Res 26(06):110–118. https://doi.org/10.13966/j.cnki.kfjyyj.2020.06.011
Liang YZ, Zhu K, Zhao CL (2017) An empirical study on the depth of interaction promoted by collaborative problem solving learning activities. J E-educ Res 38(10):87–92. https://doi.org/10.13811/j.cnki.eer.2017.10.014
Lipsey M, Wilson D (2001) Practical meta-analysis. International Educational and Professional, London, pp. 92–160
Liu Z, Wu W, Jiang Q (2020) A study on the influence of problem based learning on college students’ critical thinking-based on a meta-analysis of 31 studies. Explor High Educ 03:43–49
Morris SB (2008) Estimating effect sizes from pretest-posttest-control group designs. Organ Res Methods 11(2):364–386. https://doi.org/10.1177/1094428106291059
Article ADS Google Scholar
Mulnix JW (2012) Thinking critically about critical thinking. Educ Philos Theory 44(5):464–479. https://doi.org/10.1111/j.1469-5812.2010.00673.x
Naber J, Wyatt TH (2014) The effect of reflective writing interventions on the critical thinking skills and dispositions of baccalaureate nursing students. Nurse Educ Today 34(1):67–72. https://doi.org/10.1016/j.nedt.2013.04.002
National Research Council (2012) Education for life and work: developing transferable knowledge and skills in the 21st century. The National Academies Press, Washington, DC
Niu L, Behar HLS, Garvan CW (2013) Do instructional interventions influence college students’ critical thinking skills? A meta-analysis. Educ Res Rev 9(12):114–128. https://doi.org/10.1016/j.edurev.2012.12.002
Peng ZM, Deng L (2017) Towards the core of education reform: cultivating critical thinking skills as the core of skills in the 21st century. Res Educ Dev 24:57–63. https://doi.org/10.14121/j.cnki.1008-3855.2017.24.011
Reiser BJ (2004) Scaffolding complex learning: the mechanisms of structuring and problematizing student work. J Learn Sci 13(3):273–304. https://doi.org/10.1207/s15327809jls1303_2
Ruggiero VR (2012) The art of thinking: a guide to critical and creative thought, 4th edn. Harper Collins College Publishers, New York
Schellens T, Valcke M (2006) Fostering knowledge construction in university students through asynchronous discussion groups. Comput Educ 46(4):349–370. https://doi.org/10.1016/j.compedu.2004.07.010
Sendag S, Odabasi HF (2009) Effects of an online problem based learning course on content knowledge acquisition and critical thinking skills. Comput Educ 53(1):132–141. https://doi.org/10.1016/j.compedu.2009.01.008
Sison R (2008) Investigating Pair Programming in a Software Engineering Course in an Asian Setting. 2008 15th Asia-Pacific Software Engineering Conference, pp. 325–331. https://doi.org/10.1109/APSEC.2008.61
Simpson E, Courtney M (2002) Critical thinking in nursing education: literature review. Mary Courtney 8(2):89–98
Stewart L, Tierney J, Burdett S (2006) Do systematic reviews based on individual patient data offer a means of circumventing biases associated with trial publications? Publication bias in meta-analysis. John Wiley and Sons Inc, New York, pp. 261–286
Tiwari A, Lai P, So M, Yuen K (2010) A comparison of the effects of problem-based learning and lecturing on the development of students’ critical thinking. Med Educ 40(6):547–554. https://doi.org/10.1111/j.1365-2929.2006.02481.x
Wood D, Bruner JS, Ross G (2006) The role of tutoring in problem solving. J Child Psychol Psychiatry 17(2):89–100. https://doi.org/10.1111/j.1469-7610.1976.tb00381.x
Wei T, Hong S (2022) The meaning and realization of teachable critical thinking. Educ Theory Practice 10:51–57
Xu EW, Wang W, Wang QX (2022) A meta-analysis of the effectiveness of programming teaching in promoting K-12 students’ computational thinking. Educ Inf Technol. https://doi.org/10.1007/s10639-022-11445-2
Yang YC, Newby T, Bill R (2008) Facilitating interactions through structured web-based bulletin boards: a quasi-experimental study on promoting learners’ critical thinking skills. Comput Educ 50(4):1572–1585. https://doi.org/10.1016/j.compedu.2007.04.006
Yore LD, Pimm D, Tuan HL (2007) The literacy component of mathematical and scientific literacy. Int J Sci Math Educ 5(4):559–589. https://doi.org/10.1007/s10763-007-9089-4
Zhang T, Zhang S, Gao QQ, Wang JH (2022) Research on the development of learners’ critical thinking in online peer review. Audio Visual Educ Res 6:53–60. https://doi.org/10.13811/j.cnki.eer.2022.06.08
Download references
Acknowledgements
This research was supported by the graduate scientific research and innovation project of Xinjiang Uygur Autonomous Region named “Research on in-depth learning of high school information technology courses for the cultivation of computing thinking” (No. XJ2022G190) and the independent innovation fund project for doctoral students of the College of Educational Science of Xinjiang Normal University named “Research on project-based teaching of high school information technology courses from the perspective of discipline core literacy” (No. XJNUJKYA2003).
Author information
Authors and affiliations.
College of Educational Science, Xinjiang Normal University, 830017, Urumqi, Xinjiang, China
Enwei Xu, Wei Wang & Qingxia Wang
You can also search for this author in PubMed Google Scholar
Corresponding authors
Correspondence to Enwei Xu or Wei Wang .
Ethics declarations
Competing interests.
The authors declare no competing interests.
Ethical approval
This article does not contain any studies with human participants performed by any of the authors.
Informed consent
Additional information.
Publisher’s note Springer Nature remains neutral with regard to jurisdictional claims in published maps and institutional affiliations.
Supplementary information
Supplementary tables, rights and permissions.
Open Access This article is licensed under a Creative Commons Attribution 4.0 International License, which permits use, sharing, adaptation, distribution and reproduction in any medium or format, as long as you give appropriate credit to the original author(s) and the source, provide a link to the Creative Commons license, and indicate if changes were made. The images or other third party material in this article are included in the article’s Creative Commons license, unless indicated otherwise in a credit line to the material. If material is not included in the article’s Creative Commons license and your intended use is not permitted by statutory regulation or exceeds the permitted use, you will need to obtain permission directly from the copyright holder. To view a copy of this license, visit http://creativecommons.org/licenses/by/4.0/ .
Reprints and permissions
About this article
Cite this article.
Xu, E., Wang, W. & Wang, Q. The effectiveness of collaborative problem solving in promoting students’ critical thinking: A meta-analysis based on empirical literature. Humanit Soc Sci Commun 10 , 16 (2023). https://doi.org/10.1057/s41599-023-01508-1
Download citation
Received : 07 August 2022
Accepted : 04 January 2023
Published : 11 January 2023
DOI : https://doi.org/10.1057/s41599-023-01508-1
Share this article
Anyone you share the following link with will be able to read this content:
Sorry, a shareable link is not currently available for this article.
Provided by the Springer Nature SharedIt content-sharing initiative
This article is cited by
Impacts of online collaborative learning on students’ intercultural communication apprehension and intercultural communicative competence.
- Hoa Thi Hoang Chau
- Hung Phu Bui
- Quynh Thi Huong Dinh
Education and Information Technologies (2024)
Exploring the effects of digital technology on deep learning: a meta-analysis
Sustainable electricity generation and farm-grid utilization from photovoltaic aquaculture: a bibliometric analysis.
- A. A. Amusa
- M. Alhassan
International Journal of Environmental Science and Technology (2024)
Quick links
- Explore articles by subject
- Guide to authors
- Editorial policies

CONCEPTUAL ANALYSIS article
Complex problem solving: what it is and what it is not.
- 1 Department of Psychology, University of Bamberg, Bamberg, Germany
- 2 Department of Psychology, Heidelberg University, Heidelberg, Germany
Computer-simulated scenarios have been part of psychological research on problem solving for more than 40 years. The shift in emphasis from simple toy problems to complex, more real-life oriented problems has been accompanied by discussions about the best ways to assess the process of solving complex problems. Psychometric issues such as reliable assessments and addressing correlations with other instruments have been in the foreground of these discussions and have left the content validity of complex problem solving in the background. In this paper, we return the focus to content issues and address the important features that define complex problems.
Succeeding in the 21st century requires many competencies, including creativity, life-long learning, and collaboration skills (e.g., National Research Council, 2011 ; Griffin and Care, 2015 ), to name only a few. One competence that seems to be of central importance is the ability to solve complex problems ( Mainzer, 2009 ). Mainzer quotes the Nobel prize winner Simon (1957) who wrote as early as 1957:
The capacity of the human mind for formulating and solving complex problems is very small compared with the size of the problem whose solution is required for objectively rational behavior in the real world or even for a reasonable approximation to such objective rationality. (p. 198)
The shift from well-defined to ill-defined problems came about as a result of a disillusion with the “general problem solver” ( Newell et al., 1959 ): The general problem solver was a computer software intended to solve all kind of problems that can be expressed through well-formed formulas. However, it soon became clear that this procedure was in fact a “special problem solver” that could only solve well-defined problems in a closed space. But real-world problems feature open boundaries and have no well-determined solution. In fact, the world is full of wicked problems and clumsy solutions ( Verweij and Thompson, 2006 ). As a result, solving well-defined problems and solving ill-defined problems requires different cognitive processes ( Schraw et al., 1995 ; but see Funke, 2010 ).
Well-defined problems have a clear set of means for reaching a precisely described goal state. For example: in a match-stick arithmetic problem, a person receives a false arithmetic expression constructed out of matchsticks (e.g., IV = III + III). According to the instructions, moving one of the matchsticks will make the equations true. Here, both the problem (find the appropriate stick to move) and the goal state (true arithmetic expression; solution is: VI = III + III) are defined clearly.
Ill-defined problems have no clear problem definition, their goal state is not defined clearly, and the means of moving towards the (diffusely described) goal state are not clear. For example: The goal state for solving the political conflict in the near-east conflict between Israel and Palestine is not clearly defined (living in peaceful harmony with each other?) and even if the conflict parties would agree on a two-state solution, this goal again leaves many issues unresolved. This type of problem is called a “complex problem” and is of central importance to this paper. All psychological processes that occur within individual persons and deal with the handling of such ill-defined complex problems will be subsumed under the umbrella term “complex problem solving” (CPS).
Systematic research on CPS started in the 1970s with observations of the behavior of participants who were confronted with computer simulated microworlds. For example, in one of those microworlds participants assumed the role of executives who were tasked to manage a company over a certain period of time (see Brehmer and Dörner, 1993 , for a discussion of this methodology). Today, CPS is an established concept and has even influenced large-scale assessments such as PISA (“Programme for International Student Assessment”), organized by the Organization for Economic Cooperation and Development ( OECD, 2014 ). According to the World Economic Forum, CPS is one of the most important competencies required in the future ( World Economic Forum, 2015 ). Numerous articles on the subject have been published in recent years, documenting the increasing research activity relating to this field. In the following collection of papers we list only those published in 2010 and later: theoretical papers ( Blech and Funke, 2010 ; Funke, 2010 ; Knauff and Wolf, 2010 ; Leutner et al., 2012 ; Selten et al., 2012 ; Wüstenberg et al., 2012 ; Greiff et al., 2013b ; Fischer and Neubert, 2015 ; Schoppek and Fischer, 2015 ), papers about measurement issues ( Danner et al., 2011a ; Greiff et al., 2012 , 2015a ; Alison et al., 2013 ; Gobert et al., 2015 ; Greiff and Fischer, 2013 ; Herde et al., 2016 ; Stadler et al., 2016 ), papers about applications ( Fischer and Neubert, 2015 ; Ederer et al., 2016 ; Tremblay et al., 2017 ), papers about differential effects ( Barth and Funke, 2010 ; Danner et al., 2011b ; Beckmann and Goode, 2014 ; Greiff and Neubert, 2014 ; Scherer et al., 2015 ; Meißner et al., 2016 ; Wüstenberg et al., 2016 ), one paper about developmental effects ( Frischkorn et al., 2014 ), one paper with a neuroscience background ( Osman, 2012 ) 1 , papers about cultural differences ( Güss and Dörner, 2011 ; Sonnleitner et al., 2014 ; Güss et al., 2015 ), papers about validity issues ( Goode and Beckmann, 2010 ; Greiff et al., 2013c ; Schweizer et al., 2013 ; Mainert et al., 2015 ; Funke et al., 2017 ; Greiff et al., 2017 , 2015b ; Kretzschmar et al., 2016 ; Kretzschmar, 2017 ), review papers and meta-analyses ( Osman, 2010 ; Stadler et al., 2015 ), and finally books ( Qudrat-Ullah, 2015 ; Csapó and Funke, 2017b ) and book chapters ( Funke, 2012 ; Hotaling et al., 2015 ; Funke and Greiff, 2017 ; Greiff and Funke, 2017 ; Csapó and Funke, 2017a ; Fischer et al., 2017 ; Molnàr et al., 2017 ; Tobinski and Fritz, 2017 ; Viehrig et al., 2017 ). In addition, a new “Journal of Dynamic Decision Making” (JDDM) has been launched ( Fischer et al., 2015 , 2016 ) to give the field an open-access outlet for research and discussion.
This paper aims to clarify aspects of validity: what should be meant by the term CPS and what not? This clarification seems necessary because misunderstandings in recent publications provide – from our point of view – a potentially misleading picture of the construct. We start this article with a historical review before attempting to systematize different positions. We conclude with a working definition.
Historical Review
The concept behind CPS goes back to the German phrase “komplexes Problemlösen” (CPS; the term “komplexes Problemlösen” was used as a book title by Funke, 1986 ). The concept was introduced in Germany by Dörner and colleagues in the mid-1970s (see Dörner et al., 1975 ; Dörner, 1975 ) for the first time. The German phrase was later translated to CPS in the titles of two edited volumes by Sternberg and Frensch (1991) and Frensch and Funke (1995a) that collected papers from different research traditions. Even though it looks as though the term was coined in the 1970s, Edwards (1962) used the term “dynamic decision making” to describe decisions that come in a sequence. He compared static with dynamic decision making, writing:
In dynamic situations, a new complication not found in the static situations arises. The environment in which the decision is set may be changing, either as a function of the sequence of decisions, or independently of them, or both. It is this possibility of an environment which changes while you collect information about it which makes the task of dynamic decision theory so difficult and so much fun. (p. 60)
The ability to solve complex problems is typically measured via dynamic systems that contain several interrelated variables that participants need to alter. Early work (see, e.g., Dörner, 1980 ) used a simulation scenario called “Lohhausen” that contained more than 2000 variables that represented the activities of a small town: Participants had to take over the role of a mayor for a simulated period of 10 years. The simulation condensed these ten years to ten hours in real time. Later, researchers used smaller dynamic systems as scenarios either based on linear equations (see, e.g., Funke, 1993 ) or on finite state automata (see, e.g., Buchner and Funke, 1993 ). In these contexts, CPS consisted of the identification and control of dynamic task environments that were previously unknown to the participants. Different task environments came along with different degrees of fidelity ( Gray, 2002 ).
According to Funke (2012) , the typical attributes of complex systems are (a) complexity of the problem situation which is usually represented by the sheer number of involved variables; (b) connectivity and mutual dependencies between involved variables; (c) dynamics of the situation, which reflects the role of time and developments within a system; (d) intransparency (in part or full) about the involved variables and their current values; and (e) polytely (greek term for “many goals”), representing goal conflicts on different levels of analysis. This mixture of features is similar to what is called VUCA (volatility, uncertainty, complexity, ambiguity) in modern approaches to management (e.g., Mack et al., 2016 ).
In his evaluation of the CPS movement, Sternberg (1995) compared (young) European approaches to CPS with (older) American research on expertise. His analysis of the differences between the European and American traditions shows advantages but also potential drawbacks for each side. He states (p. 301): “I believe that although there are problems with the European approach, it deals with some fundamental questions that American research scarcely addresses.” So, even though the echo of the European approach did not enjoy strong resonance in the US at that time, it was valued by scholars like Sternberg and others. Before attending to validity issues, we will first present a short review of different streams.
Different Approaches to CPS
In the short history of CPS research, different approaches can be identified ( Buchner, 1995 ; Fischer et al., 2017 ). To systematize, we differentiate between the following five lines of research:
(a) The search for individual differences comprises studies identifying interindividual differences that affect the ability to solve complex problems. This line of research is reflected, for example, in the early work by Dörner et al. (1983) and their “Lohhausen” study. Here, naïve student participants took over the role of the mayor of a small simulated town named Lohhausen for a simulation period of ten years. According to the results of the authors, it is not intelligence (as measured by conventional IQ tests) that predicts performance, but it is the ability to stay calm in the face of a challenging situation and the ability to switch easily between an analytic mode of processing and a more holistic one.
(b) The search for cognitive processes deals with the processes behind understanding complex dynamic systems. Representative of this line of research is, for example, Berry and Broadbent’s (1984) work on implicit and explicit learning processes when people interact with a dynamic system called “Sugar Production”. They found that those who perform best in controlling a dynamic system can do so implicitly, without explicit knowledge of details regarding the systems’ relations.
(c) The search for system factors seeks to identify the aspects of dynamic systems that determine the difficulty of complex problems and make some problems harder than others. Representative of this line of research is, for example, work by Funke (1985) , who systematically varied the number of causal effects within a dynamic system or the presence/absence of eigendynamics. He found, for example, that solution quality decreases as the number of systems relations increases.
(d) The psychometric approach develops measurement instruments that can be used as an alternative to classical IQ tests, as something that goes “beyond IQ”. The MicroDYN approach ( Wüstenberg et al., 2012 ) is representative for this line of research that presents an alternative to reasoning tests (like Raven matrices). These authors demonstrated that a small improvement in predicting school grade point average beyond reasoning is possible with MicroDYN tests.
(e) The experimental approach explores CPS under different experimental conditions. This approach uses CPS assessment instruments to test hypotheses derived from psychological theories and is sometimes used in research about cognitive processes (see above). Exemplary for this line of research is the work by Rohe et al. (2016) , who test the usefulness of “motto goals” in the context of complex problems compared to more traditional learning and performance goals. Motto goals differ from pure performance goals by activating positive affect and should lead to better goal attainment especially in complex situations (the mentioned study found no effect).
To be clear: these five approaches are not mutually exclusive and do overlap. But the differentiation helps to identify different research communities and different traditions. These communities had different opinions about scaling complexity.
The Race for Complexity: Use of More and More Complex Systems
In the early years of CPS research, microworlds started with systems containing about 20 variables (“Tailorshop”), soon reached 60 variables (“Moro”), and culminated in systems with about 2000 variables (“Lohhausen”). This race for complexity ended with the introduction of the concept of “minimal complex systems” (MCS; Greiff and Funke, 2009 ; Funke and Greiff, 2017 ), which ushered in a search for the lower bound of complexity instead of the higher bound, which could not be defined as easily. The idea behind this concept was that whereas the upper limits of complexity are unbound, the lower limits might be identifiable. Imagine starting with a simple system containing two variables with a simple linear connection between them; then, step by step, increase the number of variables and/or the type of connections. One soon reaches a point where the system can no longer be considered simple and has become a “complex system”. This point represents a minimal complex system. Despite some research having been conducted in this direction, the point of transition from simple to complex has not been identified clearly as of yet.
Some years later, the original “minimal complex systems” approach ( Greiff and Funke, 2009 ) shifted to the “multiple complex systems” approach ( Greiff et al., 2013a ). This shift is more than a slight change in wording: it is important because it taps into the issue of validity directly. Minimal complex systems have been introduced in the context of challenges from large-scale assessments like PISA 2012 that measure new aspects of problem solving, namely interactive problems besides static problem solving ( Greiff and Funke, 2017 ). PISA 2012 required test developers to remain within testing time constraints (given by the school class schedule). Also, test developers needed a large item pool for the construction of a broad class of problem solving items. It was clear from the beginning that MCS deal with simple dynamic situations that require controlled interaction: the exploration and control of simple ticket machines, simple mobile phones, or simple MP3 players (all of these example domains were developed within PISA 2012) – rather than really complex situations like managerial or political decision making.
As a consequence of this subtle but important shift in interpreting the letters MCS, the definition of CPS became a subject of debate recently ( Funke, 2014a ; Greiff and Martin, 2014 ; Funke et al., 2017 ). In the words of Funke (2014b , p. 495):
It is funny that problems that nowadays come under the term ‘CPS’, are less complex (in terms of the previously described attributes of complex situations) than at the beginning of this new research tradition. The emphasis on psychometric qualities has led to a loss of variety. Systems thinking requires more than analyzing models with two or three linear equations – nonlinearity, cyclicity, rebound effects, etc. are inherent features of complex problems and should show up at least in some of the problems used for research and assessment purposes. Minimal complex systems run the danger of becoming minimal valid systems.
Searching for minimal complex systems is not the same as gaining insight into the way how humans deal with complexity and uncertainty. For psychometric purposes, it is appropriate to reduce complexity to a minimum; for understanding problem solving under conditions of overload, intransparency, and dynamics, it is necessary to realize those attributes with reasonable strength. This aspect is illustrated in the next section.
Importance of the Validity Issue
The most important reason for discussing the question of what complex problem solving is and what it is not stems from its phenomenology: if we lose sight of our phenomena, we are no longer doing good psychology. The relevant phenomena in the context of complex problems encompass many important aspects. In this section, we discuss four phenomena that are specific to complex problems. We consider these phenomena as critical for theory development and for the construction of assessment instruments (i.e., microworlds). These phenomena require theories for explaining them and they require assessment instruments eliciting them in a reliable way.
The first phenomenon is the emergency reaction of the intellectual system ( Dörner, 1980 ): When dealing with complex systems, actors tend to (a) reduce their intellectual level by decreasing self-reflections, by decreasing their intentions, by stereotyping, and by reducing their realization of intentions, (b) they show a tendency for fast action with increased readiness for risk, with increased violations of rules, and with increased tendency to escape the situation, and (c) they degenerate their hypotheses formation by construction of more global hypotheses and reduced tests of hypotheses, by increasing entrenchment, and by decontextualizing their goals. This phenomenon illustrates the strong connection between cognition, emotion, and motivation that has been emphasized by Dörner (see, e.g., Dörner and Güss, 2013 ) from the beginning of his research tradition; the emergency reaction reveals a shift in the mode of information processing under the pressure of complexity.
The second phenomenon comprises cross-cultural differences with respect to strategy use ( Strohschneider and Güss, 1999 ; Güss and Wiley, 2007 ; Güss et al., 2015 ). Results from complex task environments illustrate the strong influence of context and background knowledge to an extent that cannot be found for knowledge-poor problems. For example, in a comparison between Brazilian and German participants, it turned out that Brazilians accept the given problem descriptions and are more optimistic about the results of their efforts, whereas Germans tend to inquire more about the background of the problems and take a more active approach but are less optimistic (according to Strohschneider and Güss, 1998 , p. 695).
The third phenomenon relates to failures that occur during the planning and acting stages ( Jansson, 1994 ; Ramnarayan et al., 1997 ), illustrating that rational procedures seem to be unlikely to be used in complex situations. The potential for failures ( Dörner, 1996 ) rises with the complexity of the problem. Jansson (1994) presents seven major areas for failures with complex situations: acting directly on current feedback; insufficient systematization; insufficient control of hypotheses and strategies; lack of self-reflection; selective information gathering; selective decision making; and thematic vagabonding.
The fourth phenomenon describes (a lack of) training and transfer effects ( Kretzschmar and Süß, 2015 ), which again illustrates the context dependency of strategies and knowledge (i.e., there is no strategy that is so universal that it can be used in many different problem situations). In their own experiment, the authors could show training effects only for knowledge acquisition, not for knowledge application. Only with specific feedback, performance in complex environments can be increased ( Engelhart et al., 2017 ).
These four phenomena illustrate why the type of complexity (or degree of simplicity) used in research really matters. Furthermore, they demonstrate effects that are specific for complex problems, but not for toy problems. These phenomena direct the attention to the important question: does the stimulus material used (i.e., the computer-simulated microworld) tap and elicit the manifold of phenomena described above?
Dealing with partly unknown complex systems requires courage, wisdom, knowledge, grit, and creativity. In creativity research, “little c” and “BIG C” are used to differentiate between everyday creativity and eminent creativity ( Beghetto and Kaufman, 2007 ; Kaufman and Beghetto, 2009 ). Everyday creativity is important for solving everyday problems (e.g., finding a clever fix for a broken spoke on my bicycle), eminent creativity changes the world (e.g., inventing solar cells for energy production). Maybe problem solving research should use a similar differentiation between “little p” and “BIG P” to mark toy problems on the one side and big societal challenges on the other. The question then remains: what can we learn about BIG P by studying little p? What phenomena are present in both types, and what phenomena are unique to each of the two extremes?
Discussing research on CPS requires reflecting on the field’s research methods. Even if the experimental approach has been successful for testing hypotheses (for an overview of older work, see Funke, 1995 ), other methods might provide additional and novel insights. Complex phenomena require complex approaches to understand them. The complex nature of complex systems imposes limitations on psychological experiments: The more complex the environments, the more difficult is it to keep conditions under experimental control. And if experiments have to be run in labs one should bring enough complexity into the lab to establish the phenomena mentioned, at least in part.
There are interesting options to be explored (again): think-aloud protocols , which have been discredited for many years ( Nisbett and Wilson, 1977 ) and yet are a valuable source for theory testing ( Ericsson and Simon, 1983 ); introspection ( Jäkel and Schreiber, 2013 ), which seems to be banned from psychological methods but nevertheless offers insights into thought processes; the use of life-streaming ( Wendt, 2017 ), a medium in which streamers generate a video stream of think-aloud data in computer-gaming; political decision-making ( Dhami et al., 2015 ) that demonstrates error-proneness in groups; historical case studies ( Dörner and Güss, 2011 ) that give insights into the thinking styles of political leaders; the use of the critical incident technique ( Reuschenbach, 2008 ) to construct complex scenarios; and simulations with different degrees of fidelity ( Gray, 2002 ).
The methods tool box is full of instruments that have to be explored more carefully before any individual instrument receives a ban or research narrows its focus to only one paradigm for data collection. Brehmer and Dörner (1993) discussed the tensions between “research in the laboratory and research in the field”, optimistically concluding “that the new methodology of computer-simulated microworlds will provide us with the means to bridge the gap between the laboratory and the field” (p. 183). The idea behind this optimism was that computer-simulated scenarios would bring more complexity from the outside world into the controlled lab environment. But this is not true for all simulated scenarios. In his paper on simulated environments, Gray (2002) differentiated computer-simulated environments with respect to three dimensions: (1) tractability (“the more training subjects require before they can use a simulated task environment, the less tractable it is”, p. 211), correspondence (“High correspondence simulated task environments simulate many aspects of one task environment. Low correspondence simulated task environments simulate one aspect of many task environments”, p. 214), and engagement (“A simulated task environment is engaging to the degree to which it involves and occupies the participants; that is, the degree to which they agree to take it seriously”, p. 217). But the mere fact that a task is called a “computer-simulated task environment” does not mean anything specific in terms of these three dimensions. This is one of several reasons why we should differentiate between those studies that do not address the core features of CPS and those that do.
What is not CPS?
Even though a growing number of references claiming to deal with complex problems exist (e.g., Greiff and Wüstenberg, 2015 ; Greiff et al., 2016 ), it would be better to label the requirements within these tasks “dynamic problem solving,” as it has been done adequately in earlier work ( Greiff et al., 2012 ). The dynamics behind on-off-switches ( Thimbleby, 2007 ) are remarkable but not really complex. Small nonlinear systems that exhibit stunningly complex and unstable behavior do exist – but they are not used in psychometric assessments of so-called CPS. There are other small systems (like MicroDYN scenarios: Greiff and Wüstenberg, 2014 ) that exhibit simple forms of system behavior that are completely predictable and stable. This type of simple systems is used frequently. It is even offered commercially as a complex problem-solving test called COMPRO ( Greiff and Wüstenberg, 2015 ) for business applications. But a closer look reveals that the label is not used correctly; within COMPRO, the used linear equations are far from being complex and the system can be handled properly by using only one strategy (see for more details Funke et al., 2017 ).
Why do simple linear systems not fall within CPS? At the surface, nonlinear and linear systems might appear similar because both only include 3–5 variables. But the difference is in terms of systems behavior as well as strategies and learning. If the behavior is simple (as in linear systems where more input is related to more output and vice versa), the system can be easily understood (participants in the MicroDYN world have 3 minutes to explore a complex system). If the behavior is complex (as in systems that contain strange attractors or negative feedback loops), things become more complicated and much more observation is needed to identify the hidden structure of the unknown system ( Berry and Broadbent, 1984 ; Hundertmark et al., 2015 ).
Another issue is learning. If tasks can be solved using a single (and not so complicated) strategy, steep learning curves are to be expected. The shift from problem solving to learned routine behavior occurs rapidly, as was demonstrated by Luchins (1942) . In his water jar experiments, participants quickly acquired a specific strategy (a mental set) for solving certain measurement problems that they later continued applying to problems that would have allowed for easier approaches. In the case of complex systems, learning can occur only on very general, abstract levels because it is difficult for human observers to make specific predictions. Routines dealing with complex systems are quite different from routines relating to linear systems.
What should not be studied under the label of CPS are pure learning effects, multiple-cue probability learning, or tasks that can be solved using a single strategy. This last issue is a problem for MicroDYN tasks that rely strongly on the VOTAT strategy (“vary one thing at a time”; see Tschirgi, 1980 ). In real-life, it is hard to imagine a business manager trying to solve her or his problems by means of VOTAT.
What is CPS?
In the early days of CPS research, planet Earth’s dynamics and complexities gained attention through such books as “The limits to growth” ( Meadows et al., 1972 ) and “Beyond the limits” ( Meadows et al., 1992 ). In the current decade, for example, the World Economic Forum (2016) attempts to identify the complexities and risks of our modern world. In order to understand the meaning of complexity and uncertainty, taking a look at the worlds’ most pressing issues is helpful. Searching for strategies to cope with these problems is a difficult task: surely there is no place for the simple principle of “vary-one-thing-at-a-time” (VOTAT) when it comes to global problems. The VOTAT strategy is helpful in the context of simple problems ( Wüstenberg et al., 2014 ); therefore, whether or not VOTAT is helpful in a given problem situation helps us distinguish simple from complex problems.
Because there exist no clear-cut strategies for complex problems, typical failures occur when dealing with uncertainty ( Dörner, 1996 ; Güss et al., 2015 ). Ramnarayan et al. (1997) put together a list of generic errors (e.g., not developing adequate action plans; lack of background control; learning from experience blocked by stereotype knowledge; reactive instead of proactive action) that are typical of knowledge-rich complex systems but cannot be found in simple problems.
Complex problem solving is not a one-dimensional, low-level construct. On the contrary, CPS is a multi-dimensional bundle of competencies existing at a high level of abstraction, similar to intelligence (but going beyond IQ). As Funke et al. (2018) state: “Assessment of transversal (in educational contexts: cross-curricular) competencies cannot be done with one or two types of assessment. The plurality of skills and competencies requires a plurality of assessment instruments.”
There are at least three different aspects of complex systems that are part of our understanding of a complex system: (1) a complex system can be described at different levels of abstraction; (2) a complex system develops over time, has a history, a current state, and a (potentially unpredictable) future; (3) a complex system is knowledge-rich and activates a large semantic network, together with a broad list of potential strategies (domain-specific as well as domain-general).
Complex problem solving is not only a cognitive process but is also an emotional one ( Spering et al., 2005 ; Barth and Funke, 2010 ) and strongly dependent on motivation (low-stakes versus high-stakes testing; see Hermes and Stelling, 2016 ).
Furthermore, CPS is a dynamic process unfolding over time, with different phases and with more differentiation than simply knowledge acquisition and knowledge application. Ideally, the process should entail identifying problems (see Dillon, 1982 ; Lee and Cho, 2007 ), even if in experimental settings, problems are provided to participants a priori . The more complex and open a given situation, the more options can be generated (T. S. Schweizer et al., 2016 ). In closed problems, these processes do not occur in the same way.
In analogy to the difference between formative (process-oriented) and summative (result-oriented) assessment ( Wiliam and Black, 1996 ; Bennett, 2011 ), CPS should not be reduced to the mere outcome of a solution process. The process leading up to the solution, including detours and errors made along the way, might provide a more differentiated impression of a person’s problem-solving abilities and competencies than the final result of such a process. This is one of the reasons why CPS environments are not, in fact, complex intelligence tests: research on CPS is not only about the outcome of the decision process, but it is also about the problem-solving process itself.
Complex problem solving is part of our daily life: finding the right person to share one’s life with, choosing a career that not only makes money, but that also makes us happy. Of course, CPS is not restricted to personal problems – life on Earth gives us many hard nuts to crack: climate change, population growth, the threat of war, the use and distribution of natural resources. In sum, many societal challenges can be seen as complex problems. To reduce that complexity to a one-hour lab activity on a random Friday afternoon puts it out of context and does not address CPS issues.
Theories about CPS should specify which populations they apply to. Across populations, one thing to consider is prior knowledge. CPS research with experts (e.g., Dew et al., 2009 ) is quite different from problem solving research using tasks that intentionally do not require any specific prior knowledge (see, e.g., Beckmann and Goode, 2014 ).
More than 20 years ago, Frensch and Funke (1995b) defined CPS as follows:
CPS occurs to overcome barriers between a given state and a desired goal state by means of behavioral and/or cognitive, multi-step activities. The given state, goal state, and barriers between given state and goal state are complex, change dynamically during problem solving, and are intransparent. The exact properties of the given state, goal state, and barriers are unknown to the solver at the outset. CPS implies the efficient interaction between a solver and the situational requirements of the task, and involves a solver’s cognitive, emotional, personal, and social abilities and knowledge. (p. 18)
The above definition is rather formal and does not account for content or relations between the simulation and the real world. In a sense, we need a new definition of CPS that addresses these issues. Based on our previous arguments, we propose the following working definition:
Complex problem solving is a collection of self-regulated psychological processes and activities necessary in dynamic environments to achieve ill-defined goals that cannot be reached by routine actions. Creative combinations of knowledge and a broad set of strategies are needed. Solutions are often more bricolage than perfect or optimal. The problem-solving process combines cognitive, emotional, and motivational aspects, particularly in high-stakes situations. Complex problems usually involve knowledge-rich requirements and collaboration among different persons.
The main differences to the older definition lie in the emphasis on (a) the self-regulation of processes, (b) creativity (as opposed to routine behavior), (c) the bricolage type of solution, and (d) the role of high-stakes challenges. Our new definition incorporates some aspects that have been discussed in this review but were not reflected in the 1995 definition, which focused on attributes of complex problems like dynamics or intransparency.
This leads us to the final reflection about the role of CPS for dealing with uncertainty and complexity in real life. We will distinguish thinking from reasoning and introduce the sense of possibility as an important aspect of validity.
CPS as Combining Reasoning and Thinking in an Uncertain Reality
Leading up to the Battle of Borodino in Leo Tolstoy’s novel “War and Peace”, Prince Andrei Bolkonsky explains the concept of war to his friend Pierre. Pierre expects war to resemble a game of chess: You position the troops and attempt to defeat your opponent by moving them in different directions.
“Far from it!”, Andrei responds. “In chess, you know the knight and his moves, you know the pawn and his combat strength. While in war, a battalion is sometimes stronger than a division and sometimes weaker than a company; it all depends on circumstances that can never be known. In war, you do not know the position of your enemy; some things you might be able to observe, some things you have to divine (but that depends on your ability to do so!) and many things cannot even be guessed at. In chess, you can see all of your opponent’s possible moves. In war, that is impossible. If you decide to attack, you cannot know whether the necessary conditions are met for you to succeed. Many a time, you cannot even know whether your troops will follow your orders…”
In essence, war is characterized by a high degree of uncertainty. A good commander (or politician) can add to that what he or she sees, tentatively fill in the blanks – and not just by means of logical deduction but also by intelligently bridging missing links. A bad commander extrapolates from what he sees and thus arrives at improper conclusions.
Many languages differentiate between two modes of mentalizing; for instance, the English language distinguishes between ‘thinking’ and ‘reasoning’. Reasoning denotes acute and exact mentalizing involving logical deductions. Such deductions are usually based on evidence and counterevidence. Thinking, however, is what is required to write novels. It is the construction of an initially unknown reality. But it is not a pipe dream, an unfounded process of fabrication. Rather, thinking asks us to imagine reality (“Wirklichkeitsfantasie”). In other words, a novelist has to possess a “sense of possibility” (“Möglichkeitssinn”, Robert Musil; in German, sense of possibility is often used synonymously with imagination even though imagination is not the same as sense of possibility, for imagination also encapsulates the impossible). This sense of possibility entails knowing the whole (or several wholes) or being able to construe an unknown whole that could accommodate a known part. The whole has to align with sociological and geographical givens, with the mentality of certain peoples or groups, and with the laws of physics and chemistry. Otherwise, the entire venture is ill-founded. A sense of possibility does not aim for the moon but imagines something that might be possible but has not been considered possible or even potentially possible so far.
Thinking is a means to eliminate uncertainty. This process requires both of the modes of thinking we have discussed thus far. Economic, political, or ecological decisions require us to first consider the situation at hand. Though certain situational aspects can be known, but many cannot. In fact, von Clausewitz (1832) posits that only about 25% of the necessary information is available when a military decision needs to be made. Even then, there is no way to guarantee that whatever information is available is also correct: Even if a piece of information was completely accurate yesterday, it might no longer apply today.
Once our sense of possibility has helped grasping a situation, problem solvers need to call on their reasoning skills. Not every situation requires the same action, and we may want to act this way or another to reach this or that goal. This appears logical, but it is a logic based on constantly shifting grounds: We cannot know whether necessary conditions are met, sometimes the assumptions we have made later turn out to be incorrect, and sometimes we have to revise our assumptions or make completely new ones. It is necessary to constantly switch between our sense of possibility and our sense of reality, that is, to switch between thinking and reasoning. It is an arduous process, and some people handle it well, while others do not.
If we are to believe Tuchman’s (1984) book, “The March of Folly”, most politicians and commanders are fools. According to Tuchman, not much has changed in the 3300 years that have elapsed since the misguided Trojans decided to welcome the left-behind wooden horse into their city that would end up dismantling Troy’s defensive walls. The Trojans, too, had been warned, but decided not to heed the warning. Although Laocoön had revealed the horse’s true nature to them by attacking it with a spear, making the weapons inside the horse ring, the Trojans refused to see the forest for the trees. They did not want to listen, they wanted the war to be over, and this desire ended up shaping their perception.
The objective of psychology is to predict and explain human actions and behavior as accurately as possible. However, thinking cannot be investigated by limiting its study to neatly confined fractions of reality such as the realms of propositional logic, chess, Go tasks, the Tower of Hanoi, and so forth. Within these systems, there is little need for a sense of possibility. But a sense of possibility – the ability to divine and construe an unknown reality – is at least as important as logical reasoning skills. Not researching the sense of possibility limits the validity of psychological research. All economic and political decision making draws upon this sense of possibility. By not exploring it, psychological research dedicated to the study of thinking cannot further the understanding of politicians’ competence and the reasons that underlie political mistakes. Christopher Clark identifies European diplomats’, politicians’, and commanders’ inability to form an accurate representation of reality as a reason for the outbreak of World War I. According to Clark’s (2012) book, “The Sleepwalkers”, the politicians of the time lived in their own make-believe world, wrongfully assuming that it was the same world everyone else inhabited. If CPS research wants to make significant contributions to the world, it has to acknowledge complexity and uncertainty as important aspects of it.
For more than 40 years, CPS has been a new subject of psychological research. During this time period, the initial emphasis on analyzing how humans deal with complex, dynamic, and uncertain situations has been lost. What is subsumed under the heading of CPS in modern research has lost the original complexities of real-life problems. From our point of view, the challenges of the 21st century require a return to the origins of this research tradition. We would encourage researchers in the field of problem solving to come back to the original ideas. There is enough complexity and uncertainty in the world to be studied. Improving our understanding of how humans deal with these global and pressing problems would be a worthwhile enterprise.
Author Contributions
JF drafted a first version of the manuscript, DD added further text and commented on the draft. JF finalized the manuscript.
Authors Note
After more than 40 years of controversial discussions between both authors, this is the first joint paper. We are happy to have done this now! We have found common ground!
Conflict of Interest Statement
The authors declare that the research was conducted in the absence of any commercial or financial relationships that could be construed as a potential conflict of interest.
Acknowledgments
The authors thank the Deutsche Forschungsgemeinschaft (DFG) for the continuous support of their research over many years. Thanks to Daniel Holt for his comments on validity issues, thanks to Julia Nolte who helped us by translating German text excerpts into readable English and helped us, together with Keri Hartman, to improve our style and grammar – thanks for that! We also thank the two reviewers for their helpful critical comments on earlier versions of this manuscript. Finally, we acknowledge financial support by Deutsche Forschungsgemeinschaft and Ruprecht-Karls-Universität Heidelberg within their funding programme Open Access Publishing .
- ^ The fMRI-paper from Anderson (2012) uses the term “complex problem solving” for tasks that do not fall in our understanding of CPS and is therefore excluded from this list.
Alison, L., van den Heuvel, C., Waring, S., Power, N., Long, A., O’Hara, T., et al. (2013). Immersive simulated learning environments for researching critical incidents: a knowledge synthesis of the literature and experiences of studying high-risk strategic decision making. J. Cogn. Eng. Deci. Mak. 7, 255–272. doi: 10.1177/1555343412468113
CrossRef Full Text | Google Scholar
Anderson, J. R. (2012). Tracking problem solving by multivariate pattern analysis and hidden markov model algorithms. Neuropsychologia 50, 487–498. doi: 10.1016/j.neuropsychologia.2011.07.025
PubMed Abstract | CrossRef Full Text | Google Scholar
Barth, C. M., and Funke, J. (2010). Negative affective environments improve complex solving performance. Cogn. Emot. 24, 1259–1268. doi: 10.1080/02699930903223766
Beckmann, J. F., and Goode, N. (2014). The benefit of being naïve and knowing it: the unfavourable impact of perceived context familiarity on learning in complex problem solving tasks. Instruct. Sci. 42, 271–290. doi: 10.1007/s11251-013-9280-7
Beghetto, R. A., and Kaufman, J. C. (2007). Toward a broader conception of creativity: a case for “mini-c” creativity. Psychol. Aesthetics Creat. Arts 1, 73–79. doi: 10.1037/1931-3896.1.2.73
Bennett, R. E. (2011). Formative assessment: a critical review. Assess. Educ. Princ. Policy Pract. 18, 5–25. doi: 10.1080/0969594X.2010.513678
Berry, D. C., and Broadbent, D. E. (1984). On the relationship between task performance and associated verbalizable knowledge. Q. J. Exp. Psychol. 36, 209–231. doi: 10.1080/14640748408402156
Blech, C., and Funke, J. (2010). You cannot have your cake and eat it, too: how induced goal conflicts affect complex problem solving. Open Psychol. J. 3, 42–53. doi: 10.2174/1874350101003010042
Brehmer, B., and Dörner, D. (1993). Experiments with computer-simulated microworlds: escaping both the narrow straits of the laboratory and the deep blue sea of the field study. Comput. Hum. Behav. 9, 171–184. doi: 10.1016/0747-5632(93)90005-D
Buchner, A. (1995). “Basic topics and approaches to the study of complex problem solving,” in Complex Problem Solving: The European Perspective , eds P. A. Frensch and J. Funke (Hillsdale, NJ: Erlbaum), 27–63.
Google Scholar
Buchner, A., and Funke, J. (1993). Finite state automata: dynamic task environments in problem solving research. Q. J. Exp. Psychol. 46A, 83–118. doi: 10.1080/14640749308401068
Clark, C. (2012). The Sleepwalkers: How Europe Went to War in 1914 . London: Allen Lane.
Csapó, B., and Funke, J. (2017a). “The development and assessment of problem solving in 21st-century schools,” in The Nature of Problem Solving: Using Research to Inspire 21st Century Learning , eds B. Csapó and J. Funke (Paris: OECD Publishing), 19–31.
Csapó, B., and Funke, J. (eds) (2017b). The Nature of Problem Solving. Using Research to Inspire 21st Century Learning. Paris: OECD Publishing.
Danner, D., Hagemann, D., Holt, D. V., Hager, M., Schankin, A., Wüstenberg, S., et al. (2011a). Measuring performance in dynamic decision making. Reliability and validity of the Tailorshop simulation. J. Ind. Differ. 32, 225–233. doi: 10.1027/1614-0001/a000055
CrossRef Full Text
Danner, D., Hagemann, D., Schankin, A., Hager, M., and Funke, J. (2011b). Beyond IQ: a latent state-trait analysis of general intelligence, dynamic decision making, and implicit learning. Intelligence 39, 323–334. doi: 10.1016/j.intell.2011.06.004
Dew, N., Read, S., Sarasvathy, S. D., and Wiltbank, R. (2009). Effectual versus predictive logics in entrepreneurial decision-making: differences between experts and novices. J. Bus. Ventur. 24, 287–309. doi: 10.1016/j.jbusvent.2008.02.002
Dhami, M. K., Mandel, D. R., Mellers, B. A., and Tetlock, P. E. (2015). Improving intelligence analysis with decision science. Perspect. Psychol. Sci. 10, 753–757. doi: 10.1177/1745691615598511
Dillon, J. T. (1982). Problem finding and solving. J. Creat. Behav. 16, 97–111. doi: 10.1002/j.2162-6057.1982.tb00326.x
Dörner, D. (1975). Wie Menschen eine Welt verbessern wollten [How people wanted to improve a world]. Bild Der Wissenschaft 12, 48–53.
Dörner, D. (1980). On the difficulties people have in dealing with complexity. Simulat. Gam. 11, 87–106. doi: 10.1177/104687818001100108
Dörner, D. (1996). The Logic of Failure: Recognizing and Avoiding Error in Complex Situations. New York, NY: Basic Books.
Dörner, D., Drewes, U., and Reither, F. (1975). “Über das Problemlösen in sehr komplexen Realitätsbereichen,” in Bericht über den 29. Kongreß der DGfPs in Salzburg 1974, Band 1 , ed. W. H. Tack (Göttingen: Hogrefe), 339–340.
Dörner, D., and Güss, C. D. (2011). A psychological analysis of Adolf Hitler’s decision making as commander in chief: summa confidentia et nimius metus. Rev. Gen. Psychol. 15, 37–49. doi: 10.1037/a0022375
Dörner, D., and Güss, C. D. (2013). PSI: a computational architecture of cognition, motivation, and emotion. Rev. Gen. Psychol. 17, 297–317. doi: 10.1037/a0032947
Dörner, D., Kreuzig, H. W., Reither, F., and Stäudel, T. (1983). Lohhausen. Vom Umgang mit Unbestimmtheit und Komplexität. Bern: Huber.
Ederer, P., Patt, A., and Greiff, S. (2016). Complex problem-solving skills and innovativeness – evidence from occupational testing and regional data. Eur. J. Educ. 51, 244–256. doi: 10.1111/ejed.12176
Edwards, W. (1962). Dynamic decision theory and probabiIistic information processing. Hum. Factors 4, 59–73. doi: 10.1177/001872086200400201
Engelhart, M., Funke, J., and Sager, S. (2017). A web-based feedback study on optimization-based training and analysis of human decision making. J. Dynamic Dec. Mak. 3, 1–23.
Ericsson, K. A., and Simon, H. A. (1983). Protocol Analysis: Verbal Reports As Data. Cambridge, MA: Bradford.
Fischer, A., Greiff, S., and Funke, J. (2017). “The history of complex problem solving,” in The Nature of Problem Solving: Using Research to Inspire 21st Century Learning , eds B. Csapó and J. Funke (Paris: OECD Publishing), 107–121.
Fischer, A., Holt, D. V., and Funke, J. (2015). Promoting the growing field of dynamic decision making. J. Dynamic Decis. Mak. 1, 1–3. doi: 10.11588/jddm.2015.1.23807
Fischer, A., Holt, D. V., and Funke, J. (2016). The first year of the “journal of dynamic decision making.” J. Dynamic Decis. Mak. 2, 1–2. doi: 10.11588/jddm.2016.1.28995
Fischer, A., and Neubert, J. C. (2015). The multiple faces of complex problems: a model of problem solving competency and its implications for training and assessment. J. Dynamic Decis. Mak. 1, 1–14. doi: 10.11588/jddm.2015.1.23945
Frensch, P. A., and Funke, J. (eds) (1995a). Complex Problem Solving: The European Perspective. Hillsdale, NJ: Erlbaum.
Frensch, P. A., and Funke, J. (1995b). “Definitions, traditions, and a general framework for understanding complex problem solving,” in Complex Problem Solving: The European Perspective , eds P. A. Frensch and J. Funke (Hillsdale, NJ: Lawrence Erlbaum), 3–25.
Frischkorn, G. T., Greiff, S., and Wüstenberg, S. (2014). The development of complex problem solving in adolescence: a latent growth curve analysis. J. Educ. Psychol. 106, 1004–1020. doi: 10.1037/a0037114
Funke, J. (1985). Steuerung dynamischer Systeme durch Aufbau und Anwendung subjektiver Kausalmodelle. Z. Psychol. 193, 435–457.
Funke, J. (1986). Komplexes Problemlösen - Bestandsaufnahme und Perspektiven [Complex Problem Solving: Survey and Perspectives]. Heidelberg: Springer.
Funke, J. (1993). “Microworlds based on linear equation systems: a new approach to complex problem solving and experimental results,” in The Cognitive Psychology of Knowledge , eds G. Strube and K.-F. Wender (Amsterdam: Elsevier Science Publishers), 313–330.
Funke, J. (1995). “Experimental research on complex problem solving,” in Complex Problem Solving: The European Perspective , eds P. A. Frensch and J. Funke (Hillsdale, NJ: Erlbaum), 243–268.
Funke, J. (2010). Complex problem solving: a case for complex cognition? Cogn. Process. 11, 133–142. doi: 10.1007/s10339-009-0345-0
Funke, J. (2012). “Complex problem solving,” in Encyclopedia of the Sciences of Learning , Vol. 38, ed. N. M. Seel (Heidelberg: Springer), 682–685.
Funke, J. (2014a). Analysis of minimal complex systems and complex problem solving require different forms of causal cognition. Front. Psychol. 5:739. doi: 10.3389/fpsyg.2014.00739
Funke, J. (2014b). “Problem solving: what are the important questions?,” in Proceedings of the 36th Annual Conference of the Cognitive Science Society , eds P. Bello, M. Guarini, M. McShane, and B. Scassellati (Austin, TX: Cognitive Science Society), 493–498.
Funke, J., Fischer, A., and Holt, D. V. (2017). When less is less: solving multiple simple problems is not complex problem solving—A comment on Greiff et al. (2015). J. Intell. 5:5. doi: 10.3390/jintelligence5010005
Funke, J., Fischer, A., and Holt, D. V. (2018). “Competencies for complexity: problem solving in the 21st century,” in Assessment and Teaching of 21st Century Skills , eds E. Care, P. Griffin, and M. Wilson (Dordrecht: Springer), 3.
Funke, J., and Greiff, S. (2017). “Dynamic problem solving: multiple-item testing based on minimally complex systems,” in Competence Assessment in Education. Research, Models and Instruments , eds D. Leutner, J. Fleischer, J. Grünkorn, and E. Klieme (Heidelberg: Springer), 427–443.
Gobert, J. D., Kim, Y. J., Pedro, M. A. S., Kennedy, M., and Betts, C. G. (2015). Using educational data mining to assess students’ skills at designing and conducting experiments within a complex systems microworld. Think. Skills Creat. 18, 81–90. doi: 10.1016/j.tsc.2015.04.008
Goode, N., and Beckmann, J. F. (2010). You need to know: there is a causal relationship between structural knowledge and control performance in complex problem solving tasks. Intelligence 38, 345–352. doi: 10.1016/j.intell.2010.01.001
Gray, W. D. (2002). Simulated task environments: the role of high-fidelity simulations, scaled worlds, synthetic environments, and laboratory tasks in basic and applied cognitive research. Cogn. Sci. Q. 2, 205–227.
Greiff, S., and Fischer, A. (2013). Measuring complex problem solving: an educational application of psychological theories. J. Educ. Res. 5, 38–58.
Greiff, S., Fischer, A., Stadler, M., and Wüstenberg, S. (2015a). Assessing complex problem-solving skills with multiple complex systems. Think. Reason. 21, 356–382. doi: 10.1080/13546783.2014.989263
Greiff, S., Stadler, M., Sonnleitner, P., Wolff, C., and Martin, R. (2015b). Sometimes less is more: comparing the validity of complex problem solving measures. Intelligence 50, 100–113. doi: 10.1016/j.intell.2015.02.007
Greiff, S., Fischer, A., Wüstenberg, S., Sonnleitner, P., Brunner, M., and Martin, R. (2013a). A multitrait–multimethod study of assessment instruments for complex problem solving. Intelligence 41, 579–596. doi: 10.1016/j.intell.2013.07.012
Greiff, S., Holt, D. V., and Funke, J. (2013b). Perspectives on problem solving in educational assessment: analytical, interactive, and collaborative problem solving. J. Problem Solv. 5, 71–91. doi: 10.7771/1932-6246.1153
Greiff, S., Wüstenberg, S., Molnár, G., Fischer, A., Funke, J., and Csapó, B. (2013c). Complex problem solving in educational contexts—something beyond g: concept, assessment, measurement invariance, and construct validity. J. Educ. Psychol. 105, 364–379. doi: 10.1037/a0031856
Greiff, S., and Funke, J. (2009). “Measuring complex problem solving: the MicroDYN approach,” in The Transition to Computer-Based Assessment. New Approaches to Skills Assessment and Implications for Large-Scale Testing , eds F. Scheuermann and J. Björnsson (Luxembourg: Office for Official Publications of the European Communities), 157–163.
Greiff, S., and Funke, J. (2017). “Interactive problem solving: exploring the potential of minimal complex systems,” in The Nature of Problem Solving: Using Research to Inspire 21st Century Learning , eds B. Csapó and J. Funke (Paris: OECD Publishing), 93–105.
Greiff, S., and Martin, R. (2014). What you see is what you (don’t) get: a comment on Funke’s (2014) opinion paper. Front. Psychol. 5:1120. doi: 10.3389/fpsyg.2014.01120
Greiff, S., and Neubert, J. C. (2014). On the relation of complex problem solving, personality, fluid intelligence, and academic achievement. Learn. Ind. Diff. 36, 37–48. doi: 10.1016/j.lindif.2014.08.003
Greiff, S., Niepel, C., Scherer, R., and Martin, R. (2016). Understanding students’ performance in a computer-based assessment of complex problem solving: an analysis of behavioral data from computer-generated log files. Comput. Hum. Behav. 61, 36–46. doi: 10.1016/j.chb.2016.02.095
Greiff, S., Stadler, M., Sonnleitner, P., Wolff, C., and Martin, R. (2017). Sometimes more is too much: a rejoinder to the commentaries on Greiff et al. (2015). J. Intell. 5:6. doi: 10.3390/jintelligence5010006
Greiff, S., and Wüstenberg, S. (2014). Assessment with microworlds using MicroDYN: measurement invariance and latent mean comparisons. Eur. J. Psychol. Assess. 1, 1–11. doi: 10.1027/1015-5759/a000194
Greiff, S., and Wüstenberg, S. (2015). Komplexer Problemlösetest COMPRO [Complex Problem-Solving Test COMPRO]. Mödling: Schuhfried.
Greiff, S., Wüstenberg, S., and Funke, J. (2012). Dynamic problem solving: a new assessment perspective. Appl. Psychol. Measure. 36, 189–213. doi: 10.1177/0146621612439620
Griffin, P., and Care, E. (2015). “The ATC21S method,” in Assessment and Taching of 21st Century Skills , eds P. Griffin and E. Care (Dordrecht, NL: Springer), 3–33.
Güss, C. D., and Dörner, D. (2011). Cultural differences in dynamic decision-making strategies in a non-linear, time-delayed task. Cogn. Syst. Res. 12, 365–376. doi: 10.1016/j.cogsys.2010.12.003
Güss, C. D., Tuason, M. T., and Orduña, L. V. (2015). Strategies, tactics, and errors in dynamic decision making in an Asian sample. J. Dynamic Deci. Mak. 1, 1–14. doi: 10.11588/jddm.2015.1.13131
Güss, C. D., and Wiley, B. (2007). Metacognition of problem-solving strategies in Brazil, India, and the United States. J. Cogn. Cult. 7, 1–25. doi: 10.1163/156853707X171793
Herde, C. N., Wüstenberg, S., and Greiff, S. (2016). Assessment of complex problem solving: what we know and what we don’t know. Appl. Meas. Educ. 29, 265–277. doi: 10.1080/08957347.2016.1209208
Hermes, M., and Stelling, D. (2016). Context matters, but how much? Latent state – trait analysis of cognitive ability assessments. Int. J. Sel. Assess. 24, 285–295. doi: 10.1111/ijsa.12147
Hotaling, J. M., Fakhari, P., and Busemeyer, J. R. (2015). “Dynamic decision making,” in International Encyclopedia of the Social & Behavioral Sciences , 2nd Edn, eds N. J. Smelser and P. B. Batles (New York, NY: Elsevier), 709–714.
Hundertmark, J., Holt, D. V., Fischer, A., Said, N., and Fischer, H. (2015). System structure and cognitive ability as predictors of performance in dynamic system control tasks. J. Dynamic Deci. Mak. 1, 1–10. doi: 10.11588/jddm.2015.1.26416
Jäkel, F., and Schreiber, C. (2013). Introspection in problem solving. J. Problem Solv. 6, 20–33. doi: 10.7771/1932-6246.1131
Jansson, A. (1994). Pathologies in dynamic decision making: consequences or precursors of failure? Sprache Kogn. 13, 160–173.
Kaufman, J. C., and Beghetto, R. A. (2009). Beyond big and little: the four c model of creativity. Rev. Gen. Psychol. 13, 1–12. doi: 10.1037/a0013688
Knauff, M., and Wolf, A. G. (2010). Complex cognition: the science of human reasoning, problem-solving, and decision-making. Cogn. Process. 11, 99–102. doi: 10.1007/s10339-010-0362-z
Kretzschmar, A. (2017). Sometimes less is not enough: a commentary on Greiff et al. (2015). J. Intell. 5:4. doi: 10.3390/jintelligence5010004
Kretzschmar, A., Neubert, J. C., Wüstenberg, S., and Greiff, S. (2016). Construct validity of complex problem solving: a comprehensive view on different facets of intelligence and school grades. Intelligence 54, 55–69. doi: 10.1016/j.intell.2015.11.004
Kretzschmar, A., and Süß, H.-M. (2015). A study on the training of complex problem solving competence. J. Dynamic Deci. Mak. 1, 1–14. doi: 10.11588/jddm.2015.1.15455
Lee, H., and Cho, Y. (2007). Factors affecting problem finding depending on degree of structure of problem situation. J. Educ. Res. 101, 113–123. doi: 10.3200/JOER.101.2.113-125
Leutner, D., Fleischer, J., Wirth, J., Greiff, S., and Funke, J. (2012). Analytische und dynamische Problemlösekompetenz im Lichte internationaler Schulleistungsvergleichsstudien: Untersuchungen zur Dimensionalität. Psychol. Rundschau 63, 34–42. doi: 10.1026/0033-3042/a000108
Luchins, A. S. (1942). Mechanization in problem solving: the effect of einstellung. Psychol. Monogr. 54, 1–95. doi: 10.1037/h0093502
Mack, O., Khare, A., Krämer, A., and Burgartz, T. (eds) (2016). Managing in a VUCA world. Heidelberg: Springer.
Mainert, J., Kretzschmar, A., Neubert, J. C., and Greiff, S. (2015). Linking complex problem solving and general mental ability to career advancement: does a transversal skill reveal incremental predictive validity? Int. J. Lifelong Educ. 34, 393–411. doi: 10.1080/02601370.2015.1060024
Mainzer, K. (2009). Challenges of complexity in the 21st century. An interdisciplinary introduction. Eur. Rev. 17, 219–236. doi: 10.1017/S1062798709000714
Meadows, D. H., Meadows, D. L., and Randers, J. (1992). Beyond the Limits. Vermont, VA: Chelsea Green Publishing.
Meadows, D. H., Meadows, D. L., Randers, J., and Behrens, W. W. (1972). The Limits to Growth. New York, NY: Universe Books.
Meißner, A., Greiff, S., Frischkorn, G. T., and Steinmayr, R. (2016). Predicting complex problem solving and school grades with working memory and ability self-concept. Learn. Ind. Differ. 49, 323–331. doi: 10.1016/j.lindif.2016.04.006
Molnàr, G., Greiff, S., Wüstenberg, S., and Fischer, A. (2017). “Empirical study of computer-based assessment of domain-general complex problem-solving skills,” in The Nature of Problem Solving: Using research to Inspire 21st Century Learning , eds B. Csapó and J. Funke (Paris: OECD Publishing), 125–141.
National Research Council (2011). Assessing 21st Century Skills: Summary of a Workshop. Washington, DC: The National Academies Press.
Newell, A., Shaw, J. C., and Simon, H. A. (1959). A general problem-solving program for a computer. Comput. Automat. 8, 10–16.
Nisbett, R. E., and Wilson, T. D. (1977). Telling more than we can know: verbal reports on mental processes. Psychol. Rev. 84, 231–259. doi: 10.1037/0033-295X.84.3.231
OECD (2014). “PISA 2012 results,” in Creative Problem Solving: Students’ Skills in Tackling Real-Life problems , Vol. 5 (Paris: OECD Publishing).
Osman, M. (2010). Controlling uncertainty: a review of human behavior in complex dynamic environments. Psychol. Bull. 136, 65–86. doi: 10.1037/a0017815
Osman, M. (2012). The role of reward in dynamic decision making. Front. Neurosci. 6:35. doi: 10.3389/fnins.2012.00035
Qudrat-Ullah, H. (2015). Better Decision Making in Complex, Dynamic Tasks. Training with Human-Facilitated Interactive Learning Environments. Heidelberg: Springer.
Ramnarayan, S., Strohschneider, S., and Schaub, H. (1997). Trappings of expertise and the pursuit of failure. Simulat. Gam. 28, 28–43. doi: 10.1177/1046878197281004
Reuschenbach, B. (2008). Planen und Problemlösen im Komplexen Handlungsfeld Pflege. Berlin: Logos.
Rohe, M., Funke, J., Storch, M., and Weber, J. (2016). Can motto goals outperform learning and performance goals? Influence of goal setting on performance, intrinsic motivation, processing style, and affect in a complex problem solving task. J. Dynamic Deci. Mak. 2, 1–15. doi: 10.11588/jddm.2016.1.28510
Scherer, R., Greiff, S., and Hautamäki, J. (2015). Exploring the relation between time on task and ability in complex problem solving. Intelligence 48, 37–50. doi: 10.1016/j.intell.2014.10.003
Schoppek, W., and Fischer, A. (2015). Complex problem solving – single ability or complex phenomenon? Front. Psychol. 6:1669. doi: 10.3389/fpsyg.2015.01669
Schraw, G., Dunkle, M., and Bendixen, L. D. (1995). Cognitive processes in well-defined and ill-defined problem solving. Appl. Cogn. Psychol. 9, 523–538. doi: 10.1002/acp.2350090605
Schweizer, F., Wüstenberg, S., and Greiff, S. (2013). Validity of the MicroDYN approach: complex problem solving predicts school grades beyond working memory capacity. Learn. Ind. Differ. 24, 42–52. doi: 10.1016/j.lindif.2012.12.011
Schweizer, T. S., Schmalenberger, K. M., Eisenlohr-Moul, T. A., Mojzisch, A., Kaiser, S., and Funke, J. (2016). Cognitive and affective aspects of creative option generation in everyday life situations. Front. Psychol. 7:1132. doi: 10.3389/fpsyg.2016.01132
Selten, R., Pittnauer, S., and Hohnisch, M. (2012). Dealing with dynamic decision problems when knowledge of the environment is limited: an approach based on goal systems. J. Behav. Deci. Mak. 25, 443–457. doi: 10.1002/bdm.738
Simon, H. A. (1957). Administrative Behavior: A Study of Decision-Making Processes in Administrative Organizations , 2nd Edn. New York, NY: Macmillan.
Sonnleitner, P., Brunner, M., Keller, U., and Martin, R. (2014). Differential relations between facets of complex problem solving and students’ immigration background. J. Educ. Psychol. 106, 681–695. doi: 10.1037/a0035506
Spering, M., Wagener, D., and Funke, J. (2005). The role of emotions in complex problem solving. Cogn. Emot. 19, 1252–1261. doi: 10.1080/02699930500304886
Stadler, M., Becker, N., Gödker, M., Leutner, D., and Greiff, S. (2015). Complex problem solving and intelligence: a meta-analysis. Intelligence 53, 92–101. doi: 10.1016/j.intell.2015.09.005
Stadler, M., Niepel, C., and Greiff, S. (2016). Easily too difficult: estimating item difficulty in computer simulated microworlds. Comput. Hum. Behav. 65, 100–106. doi: 10.1016/j.chb.2016.08.025
Sternberg, R. J. (1995). “Expertise in complex problem solving: a comparison of alternative conceptions,” in Complex Problem Solving: The European Perspective , eds P. A. Frensch and J. Funke (Hillsdale, NJ: Erlbaum), 295–321.
Sternberg, R. J., and Frensch, P. A. (1991). Complex Problem Solving: Principles and Mechanisms. (eds) R. J. Sternberg and P. A. Frensch. Hillsdale, NJ: Erlbaum.
Strohschneider, S., and Güss, C. D. (1998). Planning and problem solving: differences between brazilian and german students. J. Cross-Cult. Psychol. 29, 695–716. doi: 10.1177/0022022198296002
Strohschneider, S., and Güss, C. D. (1999). The fate of the Moros: a cross-cultural exploration of strategies in complex and dynamic decision making. Int. J. Psychol. 34, 235–252. doi: 10.1080/002075999399873
Thimbleby, H. (2007). Press On. Principles of Interaction. Cambridge, MA: MIT Press.
Tobinski, D. A., and Fritz, A. (2017). “EcoSphere: a new paradigm for problem solving in complex systems,” in The Nature of Problem Solving: Using Research to Inspire 21st Century Learning , eds B. Csapó and J. Funke (Paris: OECD Publishing), 211–222.
Tremblay, S., Gagnon, J.-F., Lafond, D., Hodgetts, H. M., Doiron, M., and Jeuniaux, P. P. J. M. H. (2017). A cognitive prosthesis for complex decision-making. Appl. Ergon. 58, 349–360. doi: 10.1016/j.apergo.2016.07.009
Tschirgi, J. E. (1980). Sensible reasoning: a hypothesis about hypotheses. Child Dev. 51, 1–10. doi: 10.2307/1129583
Tuchman, B. W. (1984). The March of Folly. From Troy to Vietnam. New York, NY: Ballantine Books.
Verweij, M., and Thompson, M. (eds) (2006). Clumsy Solutions for A Complex World. Governance, Politics and Plural Perceptions. New York, NY: Palgrave Macmillan. doi: 10.1057/9780230624887
Viehrig, K., Siegmund, A., Funke, J., Wüstenberg, S., and Greiff, S. (2017). “The heidelberg inventory of geographic system competency model,” in Competence Assessment in Education. Research, Models and Instruments , eds D. Leutner, J. Fleischer, J. Grünkorn, and E. Klieme (Heidelberg: Springer), 31–53.
von Clausewitz, C. (1832). Vom Kriege [On war]. Berlin: Dämmler.
Wendt, A. N. (2017). The empirical potential of live streaming beyond cognitive psychology. J. Dynamic Deci. Mak. 3, 1–9. doi: 10.11588/jddm.2017.1.33724
Wiliam, D., and Black, P. (1996). Meanings and consequences: a basis for distinguishing formative and summative functions of assessment? Br. Educ. Res. J. 22, 537–548. doi: 10.1080/0141192960220502
World Economic Forum (2015). New Vsion for Education Unlocking the Potential of Technology. Geneva: World Economic Forum.
World Economic Forum (2016). Global Risks 2016: Insight Report , 11th Edn. Geneva: World Economic Forum.
Wüstenberg, S., Greiff, S., and Funke, J. (2012). Complex problem solving — more than reasoning? Intelligence 40, 1–14. doi: 10.1016/j.intell.2011.11.003
Wüstenberg, S., Greiff, S., Vainikainen, M.-P., and Murphy, K. (2016). Individual differences in students’ complex problem solving skills: how they evolve and what they imply. J. Educ. Psychol. 108, 1028–1044. doi: 10.1037/edu0000101
Wüstenberg, S., Stadler, M., Hautamäki, J., and Greiff, S. (2014). The role of strategy knowledge for the application of strategies in complex problem solving tasks. Technol. Knowl. Learn. 19, 127–146. doi: 10.1007/s10758-014-9222-8
Keywords : complex problem solving, validity, assessment, definition, MicroDYN
Citation: Dörner D and Funke J (2017) Complex Problem Solving: What It Is and What It Is Not. Front. Psychol. 8:1153. doi: 10.3389/fpsyg.2017.01153
Received: 14 March 2017; Accepted: 23 June 2017; Published: 11 July 2017.
Reviewed by:
Copyright © 2017 Dörner and Funke. This is an open-access article distributed under the terms of the Creative Commons Attribution License (CC BY) . The use, distribution or reproduction in other forums is permitted, provided the original author(s) or licensor are credited and that the original publication in this journal is cited, in accordance with accepted academic practice. No use, distribution or reproduction is permitted which does not comply with these terms.
*Correspondence: Joachim Funke, [email protected]
Disclaimer: All claims expressed in this article are solely those of the authors and do not necessarily represent those of their affiliated organizations, or those of the publisher, the editors and the reviewers. Any product that may be evaluated in this article or claim that may be made by its manufacturer is not guaranteed or endorsed by the publisher.
Cognition and Instruction/Problem Solving, Critical Thinking and Argumentation
We are constantly surrounded by ambiguities, falsehoods, challenges or situations in our daily lives that require our Critical Thinking , Problem Solving Skills , and Argumentation skills . While these three terms are often used interchangeably, they are notably different. Critical thinking enables us to actively engage with information that we are presented with through all of our senses, and to think deeply about such information. This empowers us to analyse, critique, and apply knowledge, as well as create new ideas. Critical thinking can be considered the overarching cognitive skill of problem solving and argumentation. With critical thinking, although there are logical conclusions we can arrive at, there is not necessarily a 'right' idea. What may seem 'right' is often very subjective. Problem solving is a form of critical thinking that confronts learners with decisions to be made about best possible solutions, with no specific right answer for well-defined and ill-defined problems. One method of engaging with Problem Solving is with tutor systems such as Cognitive Tutor which can modify problems for individual students as well as track their progress in learning. Particular to Problem Solving is Project Based Learning which focuses the learner on solving a driving question, placing the student in the centre of learning experience by conducting an extensive investigation. Problem Based Learning focuses on real-life problems that motivate the student with experiential learning. Further, Design Thinking uses a specific scaffold system to encourage learners to develop a prototype to solve a real-world problem through a series of steps. Empathy, practical design principles, and refinement of prototyping demonstrate critical thought throughout this process. Likewise, argumentation is a critical thinking process that does not necessarily involve singular answers, hence the requirement for negotiation in argumentative thought. More specifically, argumentation involves using reasoning to support or refute a claim or idea. In comparison problem solving may lead to one solution that could be considered to be empirical.
This chapter provides a theoretical overview of these three key topics: the qualities of each, their relationship to each other, as well as practical classroom applications.
Learning Outcomes:
- Defining Critical Thought and its interaction with knowledge
- Defining Problem Solving and how it uses Critical Thought to develop solutions to problems
- Introduce a Cognitive Tutor as a cognitive learning tool that employs problem solving to enhance learning
- Explore Project Based Learning as a specific method of Problem Solving
- Examine Design Thinking as a sub-set of Project Based Learning and its scaffold process for learning
- Define Argumentation and how it employs a Critical Though process
- Examine specific methodologies and instruments of application for argumentation
- 1.1 Defining critical thinking
- 1.2 Critical thinking as a western construct
- 1.3 Critical thinking in other parts of the world
- 1.4 Disposition and critical thinking
- 1.5 Self-regulation and critical thinking
- 1.6.1 Venn Diagrams
- 1.6.2.1 The classroom environment
- 1.6.3.1 Socratic Method
- 1.6.3.2 Bloom’s Taxonomy
- 1.6.3.3 Norman Webb’s Depth of Knowledge
- 1.6.3.4 Williams Model
- 1.6.3.5 Wiggins & McTighe’s Six Facets of Understanding
- 2.1.1.1.1 Structure Of The Classroom
- 2.2.1.1 Instructional Implications
- 2.2.2.1 Instructional Implications
- 2.3.1 Mind set
- 2.3.2.1.1 Instructional Implications
- 2.4 Novice Versus Expert In Problem Solving
- 2.5.1 An overview of Cognitive Tutor
- 2.5.2.1 ACT-R theory
- 2.5.2.2 Production rules
- 2.5.2.3 Cognitive model and model tracing
- 2.5.2.4 Knowledge tracing
- 2.5.3.1 Cognitive Tutor® Geometry
- 2.5.3.2 Genetics Cognitive Tutor
- 2.6.1 Theorizing Solutions for Real World Problems
- 2.6.2 Experience is the Foundation of Learning
- 2.6.3 Self-Motivation Furthers Student Learning
- 2.6.4 Educators Find Challenges in Project Based Learning Implementation
- 2.6.5 Learner Need for Authentic Results through Critical Thought
- 2.7.1 Using the Process of Practical Design for Real-World Solutions
- 2.7.2 Critical Thought on Design in the Artificial World
- 2.7.3 Critical Thinking as Disruptive Achievement
- 2.7.4 Designers are Not Scientific?
- 2.7.5 21st Century Learners and the Need for Divergent Thinking
- 3.1 Educators Find Challenges in Project Based Learning Implementation
- 3.2 Learner Need for Authentic Results through Critical Thought
- 3.3 Critical Thinking as Disruptive Achievement
- 3.4.1 Argumentation Stages
- 3.5 The Impact of Argumentation on Learning
- 4.1.1 Production, Analysis, and Evaluation
- 4.2 How Argumentation Improves Critical Thinking
- 5.1 Teaching Tactics
- 5.2.1 The CoRT Thinking Materials
- 5.2.2 The Feuerstein Instrumental Enrichment Program (FIE)
- 5.2.3 The Productive Thinking Program
- 5.2.4 The IDEAL Problem Solver
- 5.3.1 Dialogue and Argumentation
- 5.3.2 Science and Argumentation
- 5.3.3.1 Historical Thinking - The Big Six
- 5.4 Instructing through Academic Controversy
- 7.1 External links
- 8 References
Critical thinking [ edit | edit source ]

Critical thinking is an extremely valuable aspect of education. The ability to think critically often increases over the lifespan as knowledge and experience is acquired, but it is crucial to begin the process of this development as early on as possible. Research has indicated that critical thinking skills are correlated with better transfer of knowledge, while a lack of critical thinking skills has been associated with biased reasoning [1] . Before children even begin formal schooling, they develop critical thinking skills at home because of interactions with parents and caregivers [2] . As well, critical thinking appears to improve with explicit instruction [3] . Being able to engage in critical thought is what allows us to make informed decisions in situations like elections, in which candidates present skewed views of themselves and other candidates. Without critical thinking, people would fall prey to fallacious information and biased reasoning. It is therefore important that students are introduced to critical thought and are encouraged to utilize critical thinking skills as they face problems.
Defining critical thinking [ edit | edit source ]
In general, critical thinking can be defined as the process of evaluating arguments and evidence to reach a conclusion that is the most appropriate and valid among other possible conclusions. Critical thinking is a dynamic and reflective process, and it is primarily evidence-based [4] . Thinking critically involves being able to criticize information objectively and explore opposing views, eventually leading to a conclusion based on evidence and careful thought. Critical thinkers are skeptical of information given to them, actively seek out evidence, and are not hesitant to take on decision-making and complex problem solving tasks [5] . Asking questions, debating topics, and critiquing the credibility of sources are all activities that involve thinking critically. As outlined by Glaser (1941), critical thinking involves three main components: a disposition for critical thought, knowledge of critical thinking strategies, and some ability to apply the strategies [6] . Having a disposition for critical thought is necessary for applying known strategies.
Critical thinking, which includes cognitive processes such as weighing and evaluating information, leads to more thorough understanding of an issue or problem. As a type of reflection, critical thinking also promotes an awareness of one's own perceptions, intentions, feelings and actions. [7]
Critical thinking as a western construct [ edit | edit source ]

In modern education, critical thinking is taken for granted as something that people universally need and should acquire, especially at a higher educational level [8] [9] . However, critical thinking is a human construct [10] - not a scientific fact - that is tied to Ancient Greek philosophy and beliefs [11] .
The link to Ancient Greece relates both to Ancient Greek priorities of logic over emotion [11] , as well as its democratic principles. Various authors, including Elder & Paul [12] , Moon [8] , and Stanlick & Strawser [13] share the view that critical thinking questioning back to the time of Socrates . Likewise, Morgan & Saxton (2006) associate critical thinking with a fundamental requirement of all democratic citizens [14] .
An additional connection with Ancient Greece involves the Socratic Method. The Socratic Method involves a conversation between two or more people in which they ask and answer questions to challenge each other’s theses using logic and reason [15] . Such debates are subject to the issue of objective/subjective dualism in that the purpose of debate is the belief that there is a ‘right answer’, yet the ability to conduct such a debate demonstrates the subjectivity of any thesis [15] .
Because of this strong connection to Ancient Greece, critical thinking is generally considered to be a western construct. This is further amplified another western construct called Bloom’s Taxonomy , which is considered to be the essence of critical thinking in modern education [16] .
Since critical thinking is a human construct, notions of what constitutes critical thinking vary considerably from person to person. Moon (2007) lists 21 common notions of critical thinking provided by people from her workshops, and then provides her own 2-page definition of the term [8] . One view of critical thinking is that it involves a set of skills that enables one to reach defensible conclusions and make decisions in a domain or context in which one has some prior knowledge [10] . Another view is that critical thinking involves the use of systematic logic and reasoning, which while not necessarily producing empirical answers nevertheless uses a rational and scientific approach [17] . Ultimately, Moon concludes that there is no right or wrong definition [8] .
Critical thinking in other parts of the world [ edit | edit source ]
Scholars argue that while the critical thinking construct is linked to western, democratic nations, that does not mean that other non-western cultures do not possess or use similar constructs that involve critical thinking [18] . Instead, “there are different ways or forms of reasoning” [19] ; for example, Asian approaches to debates involve finding connections between conflictive arguments in order for such ideas to coexist [18] . This is due to eastern values regarding face-saving [8] . In contrast, western approaches are often viewed as being competitive: attacking the views of others while defending one's own position. Despite this dichotomous generalisation, eastern and western approaches have more similarities than they would first seem. With regards to the diplomatic Asian approach to debating, western approaches also involve compromise and negotiation for the very reason that ideas are often complex and that there can be many ‘right’ answers [14] . Similarly, the extent to which other cultures adopt western notions of critical thinking is determined by cultural values. In Muslim cultures, for example, the value of critical thinking is link to views on the appropriateness of voicing one’s views [20] .
Disposition and critical thinking [ edit | edit source ]
It has been suggested that critical thinking skills alone are not sufficient for the application of critical thinking – a disposition for critical thinking is also necessary [5] . A disposition for critical thought differs from cognitive skills. A disposition is better explained as the ability to consciously choose a skill, rather than just the ability to execute the skill [4] . Having a disposition for critical thinking can include such things as genuine interest and ability in intellectual activities. Perkins et al. (2000) expand on the idea of the necessity for a critical thinking disposition, and indicate three aspects involved in critical thinking disposition: an inclination for engaging in intellectual behaviours; a sensitivity to opportunities, in which such behaviours may be engaged; and a general ability for engaging in critical thought [5] . Halpern (1998) suggests that this critical thinking disposition must include a willingness to continue with tasks that seem difficult, openmindedness, and a habit of planning [5] . In fact, in a cognitive skills study conducted by Clifford et al. (2004), they discovered that a disposition for critical thinking was associated with better overall critical thinking skills [4] .
These are characteristics of one's attitude or personality that facilitate the process of developing CT skills:
- Inquisitive
- Truthseeking
- Open-minded
- Confidence in reasoning
There are many factors that can influence one's disposition towards CT; the first of these is culture [5] . There are many aspects of culture that can impact the ability for people to think critically. For instance, religion can negatively impact the development of CT [5] . Many religions are founded upon faith, which often requires wholehearted belief without evidence or support. The nature of organized religion counters the very premise of CT, which is to evaluate the validity and credibility of any claim. Growing up in an environment such as this can be detrimental to the development of CT skills. This kind of environment can dampen dispositions that question religious views or examine the validity of religion. Another cultural factor that can be detrimental to a CT disposition is that of authority [5] . When a child is raised under the conditions of an authoritarian parenting style, it can be detrimental to many aspects of their lives, but especially to their CT skills, as they are taught not to question the credibility of authority and often receive punishment if they do. This is also applicable in the classroom [5] . Classroom environments that foster a disposition for critical thinking in which teachers who do not foster an atmosphere of openness or allow students to question what they are taught can impact CT development as well. Classrooms where questions are rejected or home environments in which there is a high level of parental power and control can all affect the ability of students to think critically. What is more, students will have been conditioned not to think this way for their entire lives [5] . However, despite these cultural limitations, there are ways in which a disposition for CT can be fostered in both the home and the classroom.
Classroom structure is a primary way in which CT dispositions can be highlighted. Fostering a classroom structure in which students are a part of the decision making process of what they are studying can be very helpful in creating CT dispositions [5] . Such structures help students become invested in what they are learning as well as promote a classroom atmosphere in which students may feel free to question the teacher, as well as other students' opinions and beliefs about different subjects. Allowing the freedom to scrutinize and evaluate information that has been given to students is an effective way of creating a classroom environment that can encourage students to develop CT dispositions. This freedom allows for the students to remain individuals within the larger classroom context, and gives them the power to evaluate and make decisions on their own. Allowing the students to share power in the classroom can be extremely beneficial in helping the students stay motivated and analytical of classroom teachings [5] . Teachers can also employ a variety of techniques that can help students become autonomous in the classroom. Giving students the opportunity to take on different roles can be effective in creating CT dispositions, such as making predictions and contemplating problems [5] . Allowing students to engage with problems that are presented, instead of just teaching them what the teacher or textbook believes to be true, is essential for students to develop their own opinions and individual, though. In addition to this, gathering data and information on the subject is an important part of developing CT dispositions. Doing so allows for students to go out and find resources that they themselves can analyze and come to conclusions on their own [5] . Using these aspects of CT students can most effectively relate to the predictions that were first made and critique the validity of the findings [5] .
Self-regulation and critical thinking [ edit | edit source ]
In conjunction with instructing CT, teachers also need to keep in mind the self-regulation of their students. Students need to be able to maintain motivation and have a proactive attitude towards their own learning when learning a new skill. In an article by Phan (2010), he argues that self-regulated students that have better goal setting have more personal responsibility for their learning, can maintain their motivation, are more cognitively flexible, and hence are more inclined to utilize CT. Since CT skills are highly reflective, they help in self-regulated learning (SRL), and in turn, self-regulatory strategies aid in developing CT skills. These two cognitive practices are assets to students’ growth and development [7] .
Self-Regulation provides students with the basic meta-cognitive awareness required for proactive learning. This pro-activity allows students to engage in the cognitive processes of CT, such as evaluation, reflection and inference. Through one’s meta-cognitive ability to assess one’s own thoughts, one develops the capability to become autonomous in one’s learning [7] . Instead of having a supervisor overlook every task, the learner can progress at their own pace while monitoring their performance, thereby engaging in SRL. Part of this process would include periodic reflection upon the strategies that one uses when completing a task. This reflection can facilitate the student’s learning by using CT to evaluate which strategies best suit their own learning based on their cognitive needs.
The complex nature of CT suggests that it requires a long developmental process requiring guidance, practice and reinforcement. To facilitate this process, self-monitoring as a first step to self-regulation can jump-start reflective thought through assessing one’s own educational performance. This assessment promotes self-efficacy through generating motivational beliefs about one’s academic capabilities [7] . From there, through practice, students can extend their CT skills beyond themselves and into their educational contexts. With practice, students use their meta-cognitive strategies as a basis for developing CT in the long run.
Critical thinking strategies [ edit | edit source ]
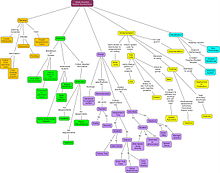
Psychologists and educators have discovered many different strategies for the development of critical thinking. Among these strategies are some that may be very familiar, such as concept maps or Venn diagrams , as well as some that may be less familiar, such as appeal-question stimuli strategies [21] . Concept mapping is particularly useful for illustrating the relationships between ideas and concepts, while Venn diagrams are often used to represent contrasting ideas [21] .
Venn Diagrams [ edit | edit source ]
Venn diagrams are used frequently in elementary grade levels and continue to be used as a contrast/compare tool throughout secondary school. An example of a situation in which a Venn diagram activity may be appropriate is during a science class. Instructors may direct students to develop a Venn diagram comparing and contrasting different plants or animals. Concept maps may be introduced in elementary grades, although they are most often used in the secondary and post-secondary levels. Concept maps are an interactive and versatile way to encourage students to engage with the course material. A key aspect of concept mapping is how it requires students to reflect on previously learned information and make connections. In elementary grades, concept maps can be introduced as a project, while later, possibly in college or university, students may use them as a study strategy. At the elementary level, students can use concept maps to make connections about the characters, settings, or plot in a story they have read. When introducing concept maps, teachers may provide students with a list of words or phrases and instruct the students to illustrate the connections between them in the form of a concept map. Asking questions can also be a simple and engaging way to develop critical thought. Teachers may begin by asking the students questions about the material, and then encouraging students to come up with their own questions. In secondary and post-secondary education, students may use questions as a way to assess the credibility of a source. At the elementary school level, questions can be used to assess students' understanding of the material, while also encouraging them to engage in critical thought by questioning the actions of characters in a story or the validity of an experiment. Appeal-question stimuli, founded by Svobodová, involves a process of students asking questions regarding their reading comprehension [21] .
Discussions [ edit | edit source ]
Using discussions as a way to develop students’ critical thinking skills can be a particularly valuable strategy for teachers. Peer interactions provide a basis for developing particular critical thinking skills, such as perspective taking and cooperation, which may not be as easily taught through instruction. A large part of discussions, of course, is language. Klooster (2002) suggested that critical thinking begins with asking questions [21] . Similarly, Vygotsky has claimed that language skills can be a crucial precursor for higher level thought processes [2] . As children develop larger vocabularies, they are better able to understand reading material and can then begin to think abstractly about the material and engage in thoughtful discussions with peers about what they understood [2] .
Studies have indicated that cross-age peer discussions may be particularly helpful in facilitating the development of critical thinking. Cross-age peer groups can be effective because of the motivation children tend to have when working with peers of different ages [2] . Younger children often look up to the older children as mentors and valuable sources of knowledge and experience, while older children feel a sense of maturity and a responsibility to share their knowledge and experience with younger students [2] . These cross-age peer discussions also provide students with the challenge of tailoring their use of language to the other group members in order to make their points understandable [2] . An example of cross-age peer groups that is relatively common in Canadian schools is the big buddy programs, where intermediate grade students are assigned a primary grade buddy to help over the course of the school year. Big buddies may help their little buddies with projects, advice, or school events. The big buddy/little buddy programs can be effective as younger students look up to their big buddies, and the big buddies feel a responsibility to help their little buddy. One important factor to be considered with cross-age peer discussions, as noted by Hattie (2006), is that these discussions should be highly structured activities facilitated by a teacher in order to ensure that students understand their group responsibilities [2] .
The classroom environment [ edit | edit source ]
Having an environment that is a safe place for students to ask questions and share ideas is extremely valuable for creating a classroom that encourages critical thinking. It has been suggested that students are more likely to develop a disposition for critical thinking when they are able to participate in the organization and planning of their classroom and class activities [5] . In these classrooms, students are legitimately encouraged by their teacher to engage in the decision making process regarding the functioning of the classroom [5] . It is also important for teachers to model the desired types of critical thought, by questioning themselves and other authorities in a respectful and appropriate manner [5] . Studies have indicated higher levels of cognitive engagement among students in classrooms with teachers who are enthusiastic and responsive [22] . Therefore, teachers should be encouraging and inclusive, and allow student engagement in classroom planning processes when possible.
Critical questions [ edit | edit source ]
Research is increasingly supporting the idea that critical thinking can be explicitly taught [23] . The use of critical questioning in education is of particular importance, because by teaching critical questioning, educators are actively modelling critical thinking processes. One of the key issues with teaching critical thinking in education is that students merely witness the product of critical thinking on the part of the teacher, i.e. they hear the conclusions that the teacher has reached through critical thinking [9] . Whereas an experienced critical thinker uses critical questions, these questions are implicit and not normally verbalised. However, for students to understand critical questioning and critical thinking strategies, the students must see the process of critical thinking. Modelling the formation and sequencing of critical questions explicitly demonstrates the thought process of how one can reach a logical conclusion.
There various methods of teaching critical questioning. The frameworks discussed below are among the most famous of these. All have their own strengths and weaknesses in terms of ease-of-use, complexity, and universality. Each of these methods approaches critical thinking with a specific definition of this human concept. As such, one’s own definition of critical thinking will likely affect one’s receptiveness to a specific critical questioning framework.

Socratic Method [ edit | edit source ]
One of the key features of western approaches to critical thinking involves the importance of critical questioning, which is linked to the Socratic Method from Ancient Greece traditions. Whether answering existing questions posed or creating new questions to be considered, critical thinking involves questions, whether explicitly / implicitly, consciously / unconsciously [13] . Browne & Keeley (2006) base their definition of critical thinking specifically on the involvement of critical questions [24] .
Answers to critical questions are not necessarily empirical. They may involve reasoning and be logical, but are nevertheless subject to alternative views from others, thus making all views both subjective and objective at the same time. Elder & Paul (2009) separate such critical questions into three categories [12] :
- Questions that have a correct answer, which can be determined using knowledge
- Questions that are open to subjective answers that cannot be judged
- Questions that produce objective answers that are judged based the quality of evidence and reasoning used
Books on critical questioning tend to be influenced heavily by the Socratic Method, and they make a distinction between ‘good’ and ‘bad’ questions. Good questions are those that are relevant to the topic at hand and that take a logical, systematic approach [14] [13] , while bad questions are those that are not relevant to the topic, are superficial, and are sequenced haphazardly. Elder & Paul (2009) argue that “[i]t is not possible to be a good thinker and a poor questioner.” [25] In other words, if a person cannot thinking of relevant and logical questions, they will be unable to reach any rational conclusions.
Additionally, as indicated above, critical thinking requires more than just asking the right questions. There is a direct relationship between critical thinking and knowledge [23] . One can possess knowledge, but not know how to apply it. Conversely, one can have good critical questioning skills, but lack the knowledge to judge the merits of an answer.
In terms of teaching critical questioning using the Socratic Method, it is essential to appreciate that there is no set of questions that one can follow, since the type of critical questions needed is based on the actual context. Consequently, the examples presented by different authors vary quite considerably. Nevertheless, there are specific guidelines one can follow [26] :
- Use critical questions to identify and understand the situation, issues, viewpoints and conclusions
- Use critical questions to search for assumptions, ambiguity, conflicts, or fallacies
- Use critical questions to evaluate the effects of the ideas
Part 1 of the Socratic Method is more of an information gathering stage, using questions to find out essential details, to clarify ideas or opinions, and to determine objectives. Part 2 uses the information from Part 1 and then uses questions to probe for underlying details that could provide reasons for critiquing the accuracy of the idea. Part 3 uses questions to reflect upon the consequences of such ideas.
Conklin (2012) separates the above three parts into six parts [27] :
- Using questions to understand
- Using questions to determine assumptions
- Using questions to discover reasons / evidence
- Using questions to determine perspectives
- Using questions to determine consequences
- Using questions to evaluate a given question
Here are some sample questions for each part [28] :
Questions for understanding:
- Why do you think that?
- What have you studied about this topic so far?
- How does this relate to what you are studying now?
Questions that determine assumptions
- How could you check that assumption?
- What else could be assumed?
- What are your views on that? Do you agree or disagree?
Questions that discover reasons / evidence
- How can you be sure?
- Why is this happening?
- What evidence do you have to back up your opinion?
Questions that determine perspectives
- How could you look at this argument another way?
- Which perspective is better?
Questions that determine consequences
- How does it affect you?
- What impact does that have?
Questions that evaluate a given question
- Why was I asked this question?
- Which questions led to the most interesting answers?
- What other questions should be asked?
Depending on the text, the Socratic Method can be extraordinarily elaborate, making it challenging for educators to apply. Conklin (2012) states that a teacher would need to spend time planning such questions in advance, rather than expect to produce them during a lesson [27] .
Bloom’s Taxonomy [ edit | edit source ]
Bloom’s Taxonomy was originally designed in 1956 to determine cognitive educational objectives and assess students’ higher-order thinking skills [29] . Since then, though, it has become adapted and used as a useful tool for promoting critical thinking skills, particularly through critical questioning [30] . These critical questions involve Bloom’s categories of understanding, applying, analysing, synthesising and evaluating. Such categories can be seen to relate to the Socratic Method promoted by other authors, i.e. the importance of questioning to understanding, analyse and evaluate. Moon (2007) believes that “‘evaluation’, ‘reflection’ and ‘understanding’” are key aspects of critical thinking [8] , which should therefore appear in any notion of critical thinking. At the same time, Bloom’s Taxonomy generates a natural set of questions that can be adapted to various contexts [31] .
In one example, a teacher uses a picture of a New York speakeasy bar. Using Bloom’s Taxonomy, the teacher could ask and model the following critical questions [14] :
- KNOWLEDGE: What do you see in the picture?
- COMPREHENSION: What do people do in places like that?
- ANALYSIS: Why are there so many policemen in the picture?
- APPLICATION: What similar situations do we see nowadays?
- SYNTHESIS: What if there were no laws prohibiting such behaviour?
- EVALUATION: How would you feel if you were one of these people? Why?

Norman Webb’s Depth of Knowledge [ edit | edit source ]
Webb’s Depth of Knowledge (DOK) taxonomy was produced in 2002 in response to Bloom’s Taxonomy [32] . In contrast with Bloom’s Taxonomy, Webb’s DOK focuses on considering thinking in terms of complexity of thinking rather than difficulty [32] .
Webb’s DOK has four levels:
- Recall & reproduction
- Working with skills & concepts
- Short-term strategic thinking
- Extended strategic thinking
Level 1 aligns with Bloom’s level of remembering and recalling information. Example critical questions in this level would include:
- What is the name of the protagonist?
- What did Oliver Twist ask Fagin?
Level 2 involves various skills, such as classifying, comparing, predicting, gathering, and displaying. Critical questions can be derived from these skill sets, including the following:
- How do these two ideas compare?
- How would you categorise these objects?
- How would you summarize the text?
Level 3 involves analysis and evaluation, once again aligning with Bloom’s Taxonomy.
- What conclusions can you reach?
- What theory can you generate to explain this?
- What is the best answer? Why?
At the same time, Level 3 of DOK shares similarities with the Socratic Method in that the individual must defend their views.
Level 4 is the most elaborate and challenging level. It involves making interdisciplinary connections and the creation of new ideas / solutions.
Since DOK becomes increasingly elaborate with levels and leads to the requirement to defend one’s position using logic and evidence, there are parallels with the Socratic Method. At the same time, because is used to develop standards in assessing critical thinking, it shares similarities with Bloom’s Taxonomy.
Williams Model [ edit | edit source ]

The Williams Model was designed by Frank Williams in the 1970s [27] . Unlike other methods, the Williams Model was designed specifically to promote creative thinking using critical questioning [27] . This model involves the following aspects:
- Flexibility
- Elaboration
- Originality
- Risk taking
- Imagination
Critical questions regarding fluency follow a sort of brainstorming approach in that the questions are designed to generates ideas and options [27] . For ‘flexibility’, the questions are designed to produce variations on existing ideas. ‘Elaboration’ questions are about building upon existing ideas and developing the level of detail. As the name suggests, critical questions for ‘originality’ are for promoting the development of new ideas. The ‘curiosity’ aspect of the Williams Model bears a similarity with that of the ‘Wonder’ stage of the Know Wonder Learn (KWL) system [33] . ‘Risk taking’ questions are designed to provoke experimentation. Although the name ‘complexity’ may sound similar to ‘elaboration’, it is instead about finding order among chaos, making connections, and filling in gaps of information. The final aspect is ‘Imagination’, which involves using questions to visualise.
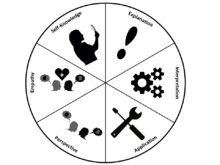
Wiggins & McTighe’s Six Facets of Understanding [ edit | edit source ]
Wiggins & McTighe’s ‘Six Facets of Understanding’ are all based on deep understanding aspects of critical thinking [34] . The method is used for teachers to design questions for students to promote critical thinking [34] . The six facets are Explanation, Interpretation, Application, Perspective, Empathy, and Self-Knowledge [35] .
‘Why’ and ‘How’ questions dominate the ‘Explanation’ facet in developing theory and reasoning [36] :
- How did this happen? Why do you think this?
- How does this connect to the other theory?
Interpretation questions encourage reading between the lines, creating analogies or metaphors, and creating written or visual scenarios to illustrate the idea. Questions include:
- How would you explain this idea in other words?
- Why do you think that there is conflict between the two sides?
- Why is it important to know this?
Application questions are about getting students to use knowledge. Part of this comes from predicting what will happen based on prior experience. Another aspect involves learning from the past. Critical questions in this facet include:
- How might we prevent this happening again?
- What do you think will happen?
- How does this work?
Perspective questions involves not only looking at ideas from other people’s perspectives, but also determining what people’s points of views are. In comparison with Empathy questions, though, Perspective questions involve more of an analytical and critical examination [35] . Here are some example questions:
- What are the different points of view concerning this topic?
- Whose is speaking in the poem?
- Whose point of view is being expressed?
- How might this look from the other person’s perspective?
Empathy questions involve perspective-taking, including empathy, in order to show an open mind to considering what it would feel like to walk in another person’s shoes.
- How would you feel in the same situation?
- What would it be like to live in those conditions?
- How would you react if someone did that your family?
Self-knowledge questions are primarily designed to encourage self reflection and to develop greater self awareness [35] . In particular, Self-Knowledge questions reveal one’s biases, values, and prejudices and how they influence our judgment of others. Critical questions in this facet include:
- How has my life shaped my view on this topic?
- What do I really know about the lives of people in that community?
- What knowledge or experience do I lack?
- How do I know what I know? Where did that information / idea come from?
Questions within the Six Facets of Understanding all incorporate the following attributes [36] :
- They are open ended
- They require deep thought
- They require critical thinking
- They promote transfer of knowledge
- They are designed to lead to follow-up questions
- They require answers that are substantiated
For examples of critical questioning in action in a classroom environment, view the External Link section at the bottom of this page.
Problem Solving [ edit | edit source ]
In everyday life we are surrounded by a plethora of problems that require solutions and our attention to resolve them to reach our goals [37] . We may be confronted with problems such as: needing to determine the best route to get to work, what to wear for an interview, how to do well on an argumentative essay or needing to find the solution to a quadratic equation. A problem is present in situations where there is a desire to solve the problem, however the solution is not obvious to the solver [38] . Problem solving is the process of finding the solutions to these problems. [39] . Although they are related, critical thinking differs fundamentally from problem solving. Critical thought is actually a process that can be applied to problem solving. For example, students may find themselves engaging in critical thought when they encounter ill-defined problems that require them to consider many options or possible answers. In essence, those who are able to think critically are able to solve problems effectively [40] .

This chapter on problem solving will first differentiate between Well-defined Problems and Ill-defined Problems , then explain uses of conceptualizing and visually representing problems within the context of problem solving and finally we will discuss how mental set may impede successful problem solving.
Well-defined and Ill-defined Problems [ edit | edit source ]
Problems can be categorized into two types: ill-defined or well-defined [37] Cognitive Psychology and Instruction (5th Ed). New York: Pearson.</ref> to the problem at hand. An example of a well-defined problem is an algebraic problem (ex: 2x - 29 = 7) where one must find the value of x. Another example may be converting the weight of the turkey from kilograms to pounds. In both instances these represent well-defined problems as there is one correct solution and a clearly defined way of finding that solution.
In contrast, ill-defined problems represent those we may face in our daily lives, the goals are unclear and they have information that is conflicting, incomplete or inconclusive [41] . An example of an ill-defined problem may be “how do we solve climate change?” or “how should we resolve poverty” as there is no one right answer to these problems. These problems yield the possibility to many different solutions as there isn’t a universally agreed upon strategy for solving them. People approach these problems differently depending on their assumptions, application of theory or values that they use to inform their approach [42] . Furthermore, each solution to a problem has its own unique strengths and weaknesses. [42] .
Table 1. Summarizes the difference between well-defined and ill-defined problems.
Differences in Solving Ill-defined and Well-defined Problems [ edit | edit source ]
In earlier times, researchers assumed both types of problems were solved in similar ways [44] , more contemporary research highlights some distinct differences between processes behind finding a solution.
Kitchener (1983) proposed that well-defined problems did not involve assumptions regarding Epistemological Beliefs [37] because they have a clear and definite solution, while ill-defined problems require these beliefs due to not having a clear and particular solution [45] . In support of this idea, Schraw, Dunkle and Bendixen conducted an experiment with 200 participants, where they found that performance in well-defined problems is not predictive of one's performance on ill-defined problems, as ill-defined problems activated different beliefs about knowledge. [46]
Furthermore Shin, Jonassen and McGee (2003), [43] found that solving ill-defined problems brought forth different skills than those found in well-structured problems. In well-structured problems domain knowledge and justification skills highly predicted problem-solving scores, whereas scores on ill-structured tasks were predictive of argumentation, attitudes and metacognition in an astronomy simulation.
Aligned with these findings, Cho and Jonassen (2002) [47] found that groups solving ill-structured problems produced more argumentation and problem solving strategies due to the importance of considering a wide variety of solutions and perspectives. In contrast, the same argumentation technique distracted the participant's activities when they dealt with well-defined problems. This research highlights the potential differences in the processes behind solving ill-defined and well-defined problems.
Implications Of The Classroom Environment [ edit | edit source ]
The fundamental differences between well-structured and ill-structured problems implicate that solving ill-structured problems calls for different skills, strategies, and approaches than well-structured problems [43] . Meanwhile, most tasks in the educational setting are designed around engaging learners in solving well-structured problems that are found at the end of textbook chapters or on standardized tests. [48] . Unfortunately the strategies used for well-defined problems have little application to ill-defined problems that are likely to be encountered day to day [49] as simplified problem solving strategies used for the well-structured designs have been found to have almost no similarities to real-life problems [48]
This demonstrates the need to restructure classrooms in a way that facilitates the student problem solving of ill-structured problems. One way we may facilitate this is through asking students questions that exemplify the problems found in everyday life [50] . This type of approach is called problem based learning and this type of classroom structure students are given the opportunity to address questions by collecting and compiling evidence, data and information from a plethora of sources [51] . In doing so students learn to analyze the information,data and information, while taking into consideration the vast interpretations and perspectives in order to present and explain their findings [51] .
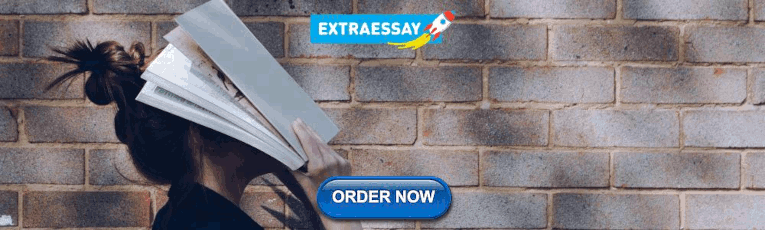
Structure Of The Classroom [ edit | edit source ]
In problem-based learning, students work in small groups to where they explore meaningful problems, identify the information needed to solve the given problem, and devise effective approaches for the solution [50] . Students utilize these strategies, analyze and consider their results to devise new strategies until they have come up with an effective solution [50] . The teacher’s role in this classroom structure is to guide the process, facilitate participation and pose questions to elicit reflections and critical thinking about their findings [50] . In addition teachers may also provide traditional lectures and explanations that are intended to support student inquiry [50] .
In support of the argument to implement a problem-based approach to problem solving, a meta-analysis conducted by Dochy, Segers, Van den Bossche, & Gijbels (2003), found problem-based learning to be superior to traditional styles of learning though in supporting flexible problem solving, application of knowledge, and hypothesis generation. [52] Furthermore, Williams, Hemstreet, Liu, and Smith (1998) found that this approach fostered greater gains in conceptual understanding in science [53] . Lastly Gallagher, Stepien, & Rosenthal (1992), found that in comparing traditional vs. project-based approaches students in problem-based learning demonstrate an ability to define problems. [54] These findings highlight the benefits of problem-based learning on understanding and defining problems in science. Given the positive effects of defining problems this education approach may also be applied to our next sub-topic of conceptualizing problems.
Steps to Problem Solving [ edit | edit source ]
There have been five stages consistently found within the literature of problem solving: (1) identifying the problem, (2) representing the problem, (3) choosing the appropriate strategy, (4) implementing the strategy, and (5) assessing the solutions [37] . This overview will focus on the first two stages of problem solving and examine how they influence problem solving.
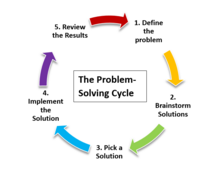
Conceptualizing Problems [ edit | edit source ]
One of the most tedious and taxing aspects of problem solving is identifying the problem as it requires one to consider the problem through multiple lenses and perspectives without being attached to one particular solution to early on in the task [39] . In addition it is also important to spend time clearly identifying the problem due to the association between time spent "conceptualizing a particular problem and the quality of one's solutions". [37] For example consider the following problem:
Becka baked a chocolate cake in her oven for twenty five minutes. How long would it take her to bake three chocolate cakes?
Most people would jump to the conclusion to multiply twenty five by three, however if we place all three cakes in the oven at a time we find it would take the same time to bake three cakes as it would take to bake one. This example highlights the need to properly conceptualize the problem and look at it from different viewpoints, before rushing to solutions.
Taking this one step further, break down the five steps as the would be used to conceptualize the problem:
Stage 1 - Define the Problem
Stage 2 - Brainstorm Solutions
Stage 3 - Pick a Solution
Stage 4 - Implement the Solution
Stage 5 - Review the Result
Research also supports the importance of taking one's time to clearly identifying the problem before proceeding to other stages. In support of this argument, Getzel and Csikszentmihalyi found that artist students that spend more time identifying the problem when producing their art were rated as having more creative and original pieces than artists who spent less time at this stage [37] . These researchers postulated that in considering a wider scope of options during this initial stage they were able to come up with more original and dynamic solutions.
Furthermore, when comparing the approaches of experienced teachers and novice post-secondary students studying to be teachers, it was found that experienced teachers spent a greater amount of time lesson planning in comparison to post-secondary students when in a placed in a hypothetical classroom. [37] In addition these teachers offered significantly more solutions to problems posed in both ill-defined and well-defined problems. Therefore it is implicated that successful problem solving is associated with the time spent finding the correct problem and the consideration of multiple solutions.
Instructional Implications [ edit | edit source ]
One instructional implication we may draw from the literature that supports that the direct relationship between time spent on conceptualizing a problem and the quality of the solution, is that teachers should encourage students to spend as much time as possible at this stage [37] . In providing this knowledge and by monitoring student’s problem solving processes to ensure that they “linger” when conceptualizing problems, we may facilitate effective problem solving [37] .
Representing the Problem [ edit | edit source ]
Problem Representation refers to how the known information about a particular problem is organized [37] . In abstract representation of a problem, we merely think or speak about the problem without externally visually representing [37] . In representing a problem tangibly this is done by creating a visual representation on paper, computer, etc. of the data though graphs, stories, symbols, pictures or equations. These visual representations [37] may be helpful they can help us keep track of solutions and steps to a problem, which can particularly be useful when encountering complex problems.
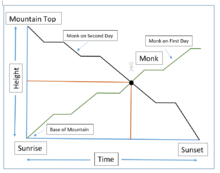
For example if we look at Dunker's Buddhist Monk example [37] :
In the morning a Buddhist monk walks outside at sunrise to climb up the mountain to get to the temple at the peak. He reaches the temple just prior to sunset. A couple days later, he departs from the temple at sunrise to climb back down the mountain, travelling quicker than he did during his ascent as he is going down the mountain. Can you show a location along the path that the monk would have passed on both at the exact time of the day? [37]
In solely using abstraction, this problem is seemingly impossible to solve due to the vast amount of information, how it is verbally presented and the amount of irrelevant information present in the question. In using a visual representation we are able to create a mental image of where the two points would intersect and are better able to come up with a solution [55] .
Research supports the benefits of visual representation when confronted with difficult problems. Martin and Schwartz [56] found greater usage of external representations when confronted with a difficult task and they had intermittent access to resources, which suggests that these representations are used as a tool when problems are too complex without external aids. Results found that while creating the initial visual representation itself took up time, those who created these visual representations solved tasks with greater efficiency and accuracy.
Another benefit is that these visual representations may foster problem solving abilities by enabling us to overcome our cognitive biases. In a study conducted by Chambers and Reisberg [57] , participants were asked to look at the image below then close their eyes and form a mental image. When asked to recall their mental image of the photo and see if there were any alternate possibilities of what the photo could be, none of the participants were able to do so. However when participants were given the visual representation of the photo they were quickly able to manipulate the position of the photo to come up with an alternate explanation of what the photo could be. This shows how visual representations may be used in education by learners to counteract mental sets, which will be discussed in the next section.
As shown above, relying on abstraction can often overload one’s cognitive resources due to short- term memory being limited to seven items of information at a time [37] . Many problems surpass these limits disabling us being able to hold all the relevant information needed to solve a problem in our working memory [37] . Therefore it is implicated that in posing problems teachers should represent them written or visually in order to reduce the cognitive load. Lastly another implication is that as teachers we may increase problem-solving skills through demonstrating to students different types of external representations that can be used to show the relevant information pertaining to the problem. These representations may include different types of graphs, charts and imagery, which all can serve as tools for students in coming up with an effective solution, representing relevant information and reducing cognitive load
Challenges of Problem Solving [ edit | edit source ]
As discussed above there are many techniques to facilitate the problem solving process, however there are factors that can also hinder this process. For example: one’s past experiences can often impede problem solving as they can provide a barrier in looking at novel solutions, approaches or ideas [58] .
Mind set [ edit | edit source ]
A mind set refers to one's tendency to be influenced by one's past experiences in approaching tasks. [58] Mental set refers to confining ourselves to using solutions that have worked in the past rather than seeking out alternative approaches. Mental sets can be functional in certain situation as in using strategies that have worked before we are quickly able to come up with solutions. However, they can also eliminate other potential and more effective solutions.
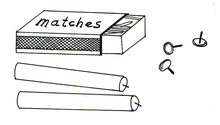
Functional Fixedness [ edit | edit source ]
Functional Fixedness is a type of mental set that refers to our tendency to focus on a specific function of an object (ie. what we traditionally use it for) while overlooking other potential novel functions of that object. [37]
A classic example of functional fixedness is the candle problem [59] . Consider you are at a table with a box full of tacks, one candle, and matches, you are then asked to mount the lit candle on the wall corkscrew board wall as quickly as possible, and make sure that this doesn't cause any wax to melt on the table. Due to functional fixedness you might first be inclined to pin the candle to the wall as that is what tacks are typically used for, similar to participants in this experiment. However, this is the incorrect solution as it would cause the wax to melt on the table.
The most effective solution requires you to view the box containing the tacks as a platform for the candle rather than it's traditional use as a receptacle. In emptying the box, we may use it as a platform for the candle and then use the tacks inside to attach the box to the wall. It is difficult to initially arrive at this solution as we tend to fixate on the function of the box of holding the tacks and have difficulty designating an alternate function to the box (ie. as a platform as opposed to a receptacle). This experiment demonstrates how prior knowledge can lead to fixation and can hinder problem solving.
Techniques to Overcome Functional Fixedness [ edit | edit source ]
As proposed by McCaffrey (2012), [60] one way to overcome functional fixedness is to break the object into parts. In doing so we may ask two fundamental questions “can it be broken down further” and “does my description of the part imply a use”. To explain this we can use McCaffrey’s steel ring figure-8 example. In this scenario the subject is given two steel rings, a candle and a match, they are asked to make the two steel rings into a figure 8. Looking at the tools provided to the subject they might decide that the wax from the candle could potentially hold the two pieces of steel together when heated up. However the wax would not be strong enough. It leaves them with a problem, how do they attach the two steel rings to make them a figure eight.
In being left with the wick as a tool, and labelling it as such we become fixated on seeing the primary function of the wick as giving off light, which hinders our ability to come up with a solution for creating a figure-8. In order to effectively solve problem we must break down our concept of the wick down further. In seeing a wick as just a waxed piece of string, we are able to get past functional fixedness and see the alternate functions of the string. In doing so we may come to the conclusion and see the waxed string as being able to be used to tie the two rings together. In showing the effectiveness of this approach McCaffrey (2012) found that people trained to use this technique solved 67% more problems than the control group [60] .
Given the effectiveness of this approach, it is implicated that one way we may promote Divergent Thinking is through teaching students to consider: "whether the object may be broken down further" [60] and "whether the description of the part imply a use" in doing so we may teach students to break down objects to their purest form and make salient the obscure features of a problem. This connects to the previously discussed idea of conceptualization where problem solving effectiveness can be increased through focusing time on defining the problem rather than jumping to conclusions based on our own preconceptions. In the following section we will discuss what strategies experts use when solving problems.
Novice Versus Expert In Problem Solving [ edit | edit source ]
Many researchers view effective problem solving as being dependent on two important variables: the amount of experience we have in trying to solve a particular category of problems [61] , which we addressed earlier by demonstrating that in practicing problem solving through engaging in a problem-based approach we may increase problem solving skills. However, the second factor to consider is the amount of domain-specific knowledge that we have to draw upon [61] . Experts possess a vast amount of domain knowledge, which allows them to efficiently apply their knowledge to relevant problems. Experts have a well-organized knowledge of their domain, which impacts they notice and how they arrange, represent and interpret information, this in turn enables them to better recall, reason and solve problems in comparison to novices. [62]
In comparing experts to novices in their problem strategies, experts are able to organize their knowledge around the deep structure in important ideas or concepts in their domain, such as what kind of solution strategy is required to solve the problem [63] . In contrast novices group problems based on surface structure of the problems, such as the objects that appear in the problem. [63]
Experts also spend more time than novices analyzing and identifying problems at the beginning of the problem-solving process. Experts take more time in thinking and planning before implementing solutions and use a limited set of strategies that are optimal in allowing them to richer and more effective solutions to the given problem. [64]
In addition experts will engage in deeper and more complete problem representation novices, in using external representations such as sketches and diagrams to represent information and solve problems. In doing so they are able to solve problems quicker and come up with better solutions. [65]
Given the literature above it is evident that problem solving and expertise overlap as the key strategies that experts utilize are also provided as effective problem solving strategies. Therefore, we may conclude that experts not only have a vast knowledge of their domain, they also know and implement the most effective strategies in order to solve problem more efficiently and effectively in comparison to novices. [65] In the next section we will discuss the connection between problem solving and critical thinking.
Cognitive Tutor for Problem Solving [ edit | edit source ]
Cognitive Tutor is a kind of Intelligent Tutoring Systems. [66] It can assign different problems to students according to their individual basis, trace users’ solution steps, provide just-in-time feedback and hint, and implement mastery learning criteria. [67]
According to Anderson and colleague, [67] the students who worked with LISP tutors completed the problems 30% faster and 43% outperformed than their peers with the help of teachers in mini-course. Also, college students who employed ACT Programming Tutor (APT) with the function of immediate feedback finished faster on a set of problems and 25% better on tests than the students who received the conventional instruction. [68] In addition, in high school geometry school settings, students who used Geometry Proof Tutor (GPT) for in- class problem solving had a letter grade scores higher than their peers who participated in traditional classroom problem-solving activities on a subsequent test. [69]
An overview of Cognitive Tutor [ edit | edit source ]
In 1985, Anderson, Boyle, and Reigser added the discipline of cognitive psychology to the Intelligent Tutoring Systems. Since then, the intelligent tutoring system adopted this approach to construct cognitive models for students to gain knowledge was named Cognitive Tutors. [67] The most widely used Cognitive Tutor is Cognitive Tutor® Algebra I. [69] Carnegie Learning, Inc., the trademark owner, is developing full- scale Cognitive Tutor®, including Algebra I, II, Bridge to Algebra, Geometry, and Integrated Math I, II, III. Cognitive Tutor® now includes Spanish Modules, as well.
Cognitive Tutors support the idea of learning by doing, an important part of human tutoring, which to provide students the performance opportunities to apply the objective skills or concepts and content related feedback. [69] To monitor students’ performance, Cognitive Tutors adopt two Algorithms , model tracing and knowledge tracing. Model tracing can provide immediate feedback, and give content-specific advice based on every step of the students’ performance trace. [67] Knowledge tracing can select appropriate tasks for every user to achieve mastery learning according to the calculation of one’s prior knowledge. [67] [69]
Cognitive Tutors can be created and applied to different curriculum or domains to help students learn, as well as being integrated into classroom learning as adaptive software. The curriculum and domains include mathematics in middle school and high school, [66] [68] [70] genetics in post-secondary institutions, [71] and programming. [67] [68] [72] [73]
Cognitive Tutors yielded huge impacts on the classroom, student motivation, and student achievement. [74] Regarding the effectiveness of Cognitive Tutors, research evidence supports more effectiveness of Cognitive Tutors than classroom instruction. [67] [75] [76] [68]
The Theoretical Background of Cognitive Tutor [ edit | edit source ]
Act-r theory [ edit | edit source ].
The theoretical background of Cognitive Tutors is ACT-R theory of learning and performance, which distinguishes between procedural knowledge and declarative knowledge. [67] According to the ACT-R theory, procedural knowledge cannot be directly absorbed into people’s heads, and it can be presented in the notation of if-then Production rules. The only way to acquire procedural knowledge is learning by doing.
Production rules [ edit | edit source ]
Production rules characterize how students, whether they beginning learners or advanced learners, think in a domain or subject. [67] Production rules can represent students' informal or intuitive thinking. [77] The informal or intuitive forms of thinking are usually different from what textbook taught, and students might gain such patterns of thinking outside from school. [78] Heuristic methods, such us providing a plan of actions for problem-solving instead of giving particular operation; [79] and non-traditional strategies, such as working with graphics rather than symbols when solving equation, [69] can be represented in production rules as well.
Cognitive model and model tracing [ edit | edit source ]
Cognitive model is constructed on both ACT-R theory and empirical studies of learners. [69] All the solutions and typical misconceptions of learners are represented in the production system of the cognitive model.
For example, there are three strategies of solving an algebra equation, 2(3+X)=10. Strategy 1 is multiplying 2 across the sum (3+X); Strategy 2 is dividing both sides of the equation by 2; Strategy 3 shows the misconception of failing to multiply 2 across the sum (3+X). Since there are various methods of each task, students can choose their way of solving problems.
Model tracing is an algorithm that can run forward along every student’s learning steps and provide instant context-specific feedback. If a student chooses the correct answer, for example, using strategy 1 or strategy 2 to solve the equation, the Cognitive Tutor® will accept the action and provide the student next task. If the student’s mistake match a common misconception, such as using strategy 3, the Cognitive Tutor will highlight this step as incorrect and provide a just-in- time feedback, such as you also need to multiply X by 2. If the student’s mistake does not match any of the production rule in the cognitive model, which means that the student does not use any of the strategies above, the Cognitive Tutor® will flag this step as an error in red and italicized. Students can ask for advice or hint any time when solving problems. According to Corbett, [68] there are three levels of advice. The first level is to accomplish a particular goal; the second level is to offer general ideas of achieving the goal, and the third level is to give students detailed advice on how to solve the problem in the current context.
Knowledge tracing [ edit | edit source ]
Knowledge tracing can monitor the growing number of production rules during the problem solving process. Every student can choose one production rule every step of his or her way of solving problems, and Cognitive Tutors can calculate an updated estimate of the probability of the student has learned the particular rule. [68] [69] The probability estimates of the rules are integrated into the interface and displayed in the skill-meter. Using probability estimates, the Cognitive Tutors can select appropriate tasks or problems according to students’ individual needs.
Effectiveness [ edit | edit source ]
Cognitive tutor® geometry [ edit | edit source ].
Aleven and Koedinger conducted two experiments to examine whether Cognitive Tutor® can scaffold self-explanation effectively in high school geometry class settings. [66] The findings suggested that “problem-solving practice with a Cognitive Tutor® is even more effective when the students explain their steps by providing references to problem-solving principles.” [80]
In geometry learning, it could happen when students have over-generalized production rules in their prior knowledge, and thus leading shallow encoding and learning. For instance, a student may choose the correct answer and go to next step base on the over-generalized production rule, if an angle looks equal to another, then it is , instead of real understanding. According to Aleven & Koedinger, self-explanation can promote more general encoding during problem-solving practice for it can push students to think more and reflect explicitly on the rules in the domain of geometry. [66]
All the geometry class in the experiments includes classroom discussion, small-group activities, lectures, and solving problems with Cognitive Tutor®. In both of the experiments, students are required to solve problems with the help of the Cognitive Tutor®. However, the Cognitive Tutor® were provided with two different versions, the new version can support self-explanation which is also called guided learning by doing and explaining, [66] and the other cannot. Theses additional features of the new version required students to justify each step by entering geometry principles or referring the principles to an online glossary of geometry knowledge, as well as providing explanations and solutions according to students’ individual choice. Also, the form of explanation in the new version is different from speech-based explanations mentioned in another experiment on self-explanation. The researchers found that students who use the new version of the Cognitive Tutor® were not only better able to give accurate explanation, but also able to deeper understand the domain rules. Thus, the students were able to transfer those learned rules to new situations better, avoiding shallow encoding and learning.
Genetics Cognitive Tutor [ edit | edit source ]
Corbett et al. (2010) conducted two evaluations of the Genetics Cognitive Tutor in seven different kinds of biology courses in 12 universities in America. The findings suggested the effectiveness of implementing Genetics Cognitive Tutor in post-secondary institution genetic problem-solving practice settings. [81]
In the first evaluation, the participants used the Genetics Cognitive Tutor with their class activities or homework assignments. The software has 16 modules with about 125 problems in five general genetic topics. Genetics Cognitive Tutor utilized the cognitive model of genetics problem solving knowledge to provide step-by-step help, and both model tracing and knowledge tracing. With the average correctness of pretest (43%) and post-test (61%), the average improvements of using Genetic Cognitive Tutors was 18%. In the second empirical evaluations, the researchers examined whether the knowledge tracing can correctly predict students’ knowledge. The finding suggested that the algorithm of knowledge tracing is capable of accurately estimating every student performance on the paper- and-pencil post-test.
Project Based Learning and Design Thinking [ edit | edit source ]
Theorizing solutions for real world problems [ edit | edit source ].
Project Based Learning is a concept that is meant to place the student at the center of learning. The learner is expected to take on an active role in their learning by responding to a complex challenge or question through an extended period of investigation. Project Based Learning is meant for students to acknowledge the curriculum of their class, but also access the knowledge that they already have to solve the problem challenge. At its roots, project-based learning is an activity in which students develop an understanding of a topic based on a real-life problem or issue and requires learners to have a degree of responsibility in designing their learning activity [82] . Blummenfeld et al. (1991) states that Project Based Learning allows students to be responsible for both their initial question, activities, and nature of their artifacts [83] .
Project based learning is based on five criteria [84]

Challenges are based on authentic, real-world problems that require learners to engage through an inquiry process and demonstrate understanding through active or experiential learning. An example would be elementary or secondary students being asked by their teacher to solve a school problem – such as how to deal with cafeteria compost. Students would be encouraged to work in groups to develop solutions for this problem within specific criteria for research, construction, and demonstration of their idea as learners are cognitively engaged with subject matter over an extended period of time keeping them motivated [83] . The result is complex learning that defines its success is more than as more than the sum of the parts [85] . Project Based Learning aims at learners coordinating skills of knowledge, collaboration, and a final project presentation. This type of schema construction allows learners to use concrete training to perform concrete results. The learner uses previous knowledge to connect with new information and elaborate on their revised perception of a topic [85] . In Project Based Learning this would constitute the process of information gathering and discussing this information within a team to decide on a final solution for the group-instructed problem.
Unlike Problem-Based Learning, experiential learning within a constructivist pedagogy, is the basis of Project Based Learning, and learners show their knowledge, or lack there of, by working towards a real solution through trial and error on a specific driving question. The philosophy of Experiential experiential learning education comes from the theories developed by John Dewey in his work Education and Experience. Dewey argues that experience is shown to be a continuous process of learning by arousing curiosity, strengthen initiative, and is a force in moving the learner towards further knowledge [86] . The experiential aspect of Project Based Learning through working towards solutions for real world problems ties learner’s solutions to practical constructs. Learners must make up the expected gap in their knowledge through research and working together in a collaborative group. The experiential learning through Project Based Learning is focused on a driving question usually presented by the teacher. It is this focus that students must respond to with a designed artifact to show acquired knowledge.
The constructivist methodology of Project Based Learning is invoked through the guided discovery process set forth by the instructor, unlike pure discovery which has been criticised for student having too much freedom [87] , Project Based Learning involves a specific question driven by the instructor to focus the process of investigation. This form of constructivist pedagogy has shown to promote cognitive processing that is most effective in this type of learning environment [87] . Project Based Learning provides a platform for learners to find their own solutions to the teacher driven question, but also have a system in which to discover, analyze, and present. Therefore, Project Based Learning delivers beneficial cognitive meaningful learning by selecting, organizing, and integrating knowledge [87] .
Experience is the Foundation of Learning [ edit | edit source ]
Project Based Learning is a branch of education theory that is based on the idea of learning through doing. John Dewey indicated that teachers and schools should help learners to achieve greater depth in correlation between theory and real-world through experiential and constructivist methods. Dewey stated that education should contain an experiential continuum and a democratization of education to promote a better quality of human experience [86] . These two elements are consistent with Project Based Learning through the application of authentic, real world problems and production of artifacts as solutions, and the learner finding their own solutions through a collaborative effort with in a group. Blumenfeld et al. mentions that the value in Project Based Learning comes from questions that students can relate to including personal health and welfare, community concerns, or current events [83] .
Project Based Learning has basis also in the work of Jean Piaget who surmised that the learner is best served to learn in a constructivist manner – using previous knowledge as a foundation for new learning and connections. The learner’s intelligence is progressed from the assimilation of things in the learner’s environment to alter their original schema by accommodating multiple new schema and assimilating all of this experienced knowledge [88] . Piaget believed in the learner discovering new knowledge for themselves, but that without collaboration the individual would not be able to coherently organize their solution [87] . Project Based Learning acknowledges Piaget’s beliefs on the need for collective communication and its use in assembling new knowledge for the learner.
Self-Motivation Furthers Student Learning [ edit | edit source ]
Project Based Learning is perceived as beneficial to learners in various ways including gained knowledge, communication, and creativity. While engaging on a single challenge, learners obtain a greater depth of knowledge. Moreover, abilities in communication, leadership, and inter-social skills are strengthened due to the collaborative nature of Project Based Learning. Students retain content longer and have a better understanding of what they are learning. There are at least four strands of cognitive research to support Project Based Learning [84] – motivation, expertise, contextual factors, and technology.
Motivation of students that is centred on the learning and mastery of subject matter are more inclined to have sustained engagement with their work [89] . Therefore, Project Based Learning discourages public competition in favour of cooperative goals to reduce the threat to individual students and increase focus on learning and mastery [84] . Project Based Learning is designed to allow students to reach goals together, without fear of reprisal or individual criticism. For instance, Helle, et al. completed a study of information system design students who were asked to work on a specific assignment over a seven-month timeline. Students were given questionnaires about their experience during this assignment to determine their motivation level. Helle, et al. examined the motivation of learners in project groups and found intrinsic motivation increased by 0.52 standard deviations, showing that Project Based learner groups used self-motivation more often to complete assignments. Further, the study implied intrinsic motivation increase substantially for those who were lowest in self-regulation [90] .
Learner metacognitive and self-regulation skills are lacking in many students and these are important to master in student development in domains [84] . In the Project Based Learning system the relationship between student and teacher allows the instructor to use scaffolding to introduce more advance forms of inquiry for students to model, thus middle school students and older are very capable of meaningful learning and sophisticated results [91] . Learners would then become experts over time of additional skills sets that they developed on their own within this system.
Contextually, situated cognition is best realized when the material to be used resembles real-life as much as possible [84] , therefore, Project Based Learning provides confidence in learners to succeed in similar tasks outside of school because they no longer associate subjects as artificial boundaries to knowledge transfer. Gorges and Goke (2015) investigated the relationship between student perception of their abilities in major high school subjects and their relating these skills to real-world problem application through an online survey. Learners showed confidence in problem-solving skills and how to apply their learning to real-life situations, as Gorges and Goke [92] report, and that students who used Project Based Learning style learning have increased self-efficacy and self-concepts of ability in math (SD .77), history (SD .72), etc. [92] . Therefore, students are more likely to use domain-specific knowledge outside of an academic setting through increased confidence. Further, a comparison between students immediately after finishing a course and 12 weeks to 2 years provided effect sizes that showed Project Based Learning helped retain much knowledge [92] .
Technology use allows learners to have a more authentic experience by providing users with an environment that includes data, expanded interaction and collaboration, and emulates the use of artifacts [84] . The learner, in accessing technology, can enhance the benefits of Project Based Learning by having more autonomy is finding knowledge and connecting with group members. Creativity is enhanced as students must find innovative solutions to their authentic problem challenges. For instance, using digital-story-telling techniques through Project Based Learning, as stated by Hung and Hwang [93] , to collect data (photos) in elementary class to help answer a specific project question on global warming in science provided a significant increase in tests results (SD 0.64). As well, in order to find answers, learners must access a broad range of knowledge, usually crossing over various disciplines. The end result is that projects are resolved by student groups that use their knowledge and access to additional knowledge (usually through technology) to build a solution to the specific problem.
Educators Find Challenges in Project Based Learning Implementation [ edit | edit source ]
One of the main arguments against this type of learning is that the project can become unfocused and not have the appropriate amount of classroom time to build solutions. Educators themselves marginalized Project Based Learning because they lack the training and background knowledge in its implementation. Further financial constraints to provide effective evaluation through technology dissuades teachers as well [94] . The information gained by students could be provided in a lecture-style instruction and can be just as effective according to critics. Further, the danger is in learners becoming off-task in their time spent in the classroom, and if they are not continually focused on the task and the learning content, then the project will not be successful. Educators with traditional backgrounds in teaching find Project Based Learning requires instructors to maintain student connection to content and management of their time – this is not necessarily a style that all teachers can accomplish [94] .Blumenfeld et al. (1998) state that real success from Project Based Learning begins and ends with a focused structure that allows teacher modelling, examples, suggested strategies, distributing guidelines, giving feedback during the activity, and allowing for revision of work [91] .
Learner Need for Authentic Results through Critical Thought [ edit | edit source ]

Project Based Learning is applicable to a number of different disciplines since it has various applications in learning, and is specifically relevant with the 21st century redefinition of education (differentiated, technologically-focused, collaboration, cross-curricular). STEM (Science, Technology, Engineering, Mathematics) is one form of 21st century education that benefits from instructors using Project Based Learning since it natural bridges between domains. The focus of STEM is to prepare secondary students for the rigors of post-secondary education and being able to solve complex problems in teams as would be expected when performing these jobs in the real world after graduation. Many potential occupational areas could benefit from Project Based Learning including medical, engineering, computer design, and education. Project Based Learning allows secondary students the opportunity to broaden their knowledge and become successful in high-stakes situation [95] . Moreover, these same students then develop a depth in knowledge when it comes to reflecting upon their strengths and limitations [95] . The result would be a learner who has developed critical thinking and has had a chance to apply it to real situations. Further the construction of a finished product is a realistic expectation in presenting an authentic result from learning. The product result demands accountability, and learner adherent to instructor expectations as well as constraints for the project [95] .
The learner is disciplined to focus on specific outcomes, understand the parameters of the task, and demonstrate a viable artifact. The implication is that students will be ready to meet the challenges of a high-technology, fast-paced work world where innovation, collaboration, and results-driven product is essential for success. Technology is one area where Project Based Learning can be applied by developing skills in real-world application, thus cognitive tools aforded by new technology will be useful if perceived as essential for the project (as is the case in many real-world applications) [83] .. For example, designers of computer systems with prior knowledge may be able to know how to trouble-shoot an operating system, but they do not really understand how things fit or work together, and they have a false sense of security about their skills [96] .
Design-Thinking as a Sub-set of Project-Based Learning [ edit | edit source ]
Using the process of practical design for real-world solutions [ edit | edit source ].
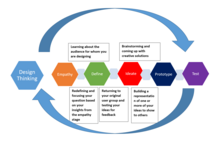
Design Thinking is a pedagogical approach to teaching through a constructionist methodology of challenge-based problem solving branching off of Project Based learning. It should be understood as a combination of sub-disciplines having design as the subject of their cognitive interests [97] .
An example of design-thinking would be learners engaged with finding a solution to a real-world problem. However, unlike Project Based Learning, design-thinking asks the learner to create a practical solution within a scaffolding process (Figure 3) such as finding a method to deliver clean drinking water to a village. Designers would consider social, economic, and political considerations, but would deliver a final presentation of a working prototype that could be marketable. Hence a water system could be produced to deliver water to villagers, but within the limits of the materials, finances, and local policies in mind. It designates cores principles of empathy, define, ideate, prototype, and test to fulfill the challenges of design. Starting with a goal (solution) in mind, empathise is placed upon creative and practical decision making through design to achieve an improved future result. It draws upon a thinking that requires investigation into the details of a problem to find hidden parameters for a solution-based result. The achieved goal then becomes the launching point for further goal-setting and problem solving. [97]
This type of approach to education is based on the premise that the modern world is full of artificial constructs, and that our civilization historically has relied upon these artifacts to further our progress in technological advances. Herbert Simon, a founder of design-thinking, states that the world that students find themselves in today is much more man-made and artificial that it is a natural world [98] . The challenge of design-thinking is to foster innovation by enhancing student creative thinking abilities [99] . Design-thinking is a tool for scaffolding conventional educational projects into Project Based thinking. Van Merrienbroer (2004) views design-learning as a scaffolding for whole-task practice. It decreases intrinsic cognitive load while learners can practice on the simplest of worked-out examples [87] . Therefore, Design-thinking is currently becoming popular due to its ability to bridge between the justification of what the learner knows and what the learner discovers within the context of 21st century skills and learning. A further example of this process is the design of a product that children will use to increase their physical activity (see video on Design Thinking) and can be explained using the scaffold of Design Thinking:
Critical Thought on Design in the Artificial World [ edit | edit source ]
Design-thinking is can be traced back to a specific scholars including Herbert Simon, Donald Schon, and Nigel Cross. Simon published his findings on the gap he found in education of professions in 1969. He observed that techniques in the natural sciences and that just as science strove to show simplicity in the natural world of underlying complex systems, and Simon determined the it was the same for the artificial world as well [100] . Not only should this include the process behind the sciences, but the arts and humanities as well since music, for example involves formal patterns like mathematics (Simon, 136). Hence, the creative designs of everyone is based upon a common language and its application. While Schon builds upon the empathetic characteristics of design-thinking as a Ford Professor of Urban Planning and Education at MIT, referring to this process as an artistic and intuitive process for problem-solving [101] . Schon realized that part of the design process was also the reflection-in-action that must be involved during critical thinking and ideating. Moreover, the solutions for problems do not lie in text-books, but in the designer’s ability to frame their own understanding of the situation [100] . Cross fuses these earlier ideas into a pedagogy surrounding education stating that design-thinking should be part of the general education of both sciences and humanities [97] . He implies that students encouraged to use this style of thinking will improve cognitive development of non-verbal thought and communication [97] .
Critical Thinking as Disruptive Achievement [ edit | edit source ]
Design-thinking follows a specific flow from theoretical to practical. It relies upon guided learning to promote effective learner solutions and goes beyond inquiry which has been argued does not work because it goes beyond the limits of long-term memory [97] . Design-thinking requires the learner to have a meta-analysis of their process. Creativity (innovative thought) is evident in design thinking through studies in defocused and focused attention to stimuli in memory activation [97] . Hu et al. (2010) developed a process of disrupted thinking in elementary students by having them use logical methods of critical thought towards specific design projects, over a four-year period, through specific lesson techniques. The results show that these students had increased thinking ability (SD .78) and that these effects have a long-term transfer increasing student academic achievement [102] . This shows use of divergent and convergent thinking in the creative process, and both of these process of thought has been noted to be important in the process of creativity (Goldschmidt, 2016, p 2) and demonstrates the Higher Order Thinking that is associated with long-term memory. Design-thinking specifically demonstrates the capability of having learners develop
Designers are Not Scientific? [ edit | edit source ]
Design-thinking critics comment that design is in itself not a science or cognitive method of learning, and is a non-scientific activity due to the use of intuitive processes [97] . The learner is not truly involved within a cognitive practice (scientific process of reasoning). However, the belief of Cross is that design itself is a science to be studied, hence it can be investigated with systematic and reliable methods of investigation [97] . Further, Schon states that there is connection between theory and practice that in design thinking means that there is a loyalty to developing a theoretical idea into a real world prototype [101] . Design-thinking is a process of scientific cognitive practice that does constitute technical rationality [101] and using this practice to understand the limits of their design that includes a reflective practice and meta. Further, this pedagogy is the application for the natural gap between theory and practice for most ideas, by allowing the learner to step beyond normal instruction and practice to try something new and innovative to come up with a solution. Design-thinking rejects heuristically-derived responses based on client or expert appreciation to take on an unforeseen form [101] .
21st Century Learners and the Need for Divergent Thinking [ edit | edit source ]
Design-thinking is exceptionally positioned for use with 21st century skills based around technological literacy. Specifically, it is meant to assist the learner in developing creative and critical skills towards the application of technology. Designing is a distinct form of thinking that creates a qualitative relationship to satisfy a purpose [103] . Moreover, in a world that is rapidly becoming technologized, design-thinking the ability to make decisions based upon feel, be able to pay attention to nuances, and appraise the consequences of one’s actions [103] . The designer needs to be able to think outside the perceived acceptable solution and look to use current technology. Therefore, learners using design thinking are approaching all forms of technology as potential applications for a solution. Prototyping might include not just a hardware application, but also the use of software. Cutting-edge technologies such as Augmented Reality and Virtual Reality would be acceptable forms of solutions for design challenges. Specific application of design-thinking is, therefore applicable to areas of study that require technological adaptation and innovation. Specifically, the K-12 BC new curriculum (2016) has a specific focus on Applied Design, Skills, and Technologies that calls for all students to have knowledge of design-thinking throughout their entire education career and its application towards the advancement of technology. Therefore, Design Thinking is a relative and essential component to engaging student critical thought process.
Argumentation [ edit | edit source ]
Argumentation is the process of assembling and communicating reasons for or against an idea, that is, the act of making and presenting arguments. CT in addition to clear communication makes a good argument. It is the process through which one rationally solves problems, issues and disputes as well as resolving questions [104] .
The practice of argumentation consists of two dimensions: dialogue and structure [105] . The dialogue in argumentative discussions focus on specific speech acts – actions done through language (i.e. accept, reject, refute, etc.) – that help advance the speaker’s position. The structure of an argument helps distinguish the different perspectives in discussion and highlight positions for which speakers are arguing [105] .
One of the main arguments against this type of learning is that the project can become unfocused and not have the appropriate amount of classroom time to build solutions. Educators themselves marginalize PBL* because they lack the training and background knowledge in its implementation. Further financial constraints to provide effective evaluation through technology dissuades teachers as well (Efstratia, 2014, p 1258). The information gained by students could be provided in a lecture-style instruction and can be just as effective according to critics. Further, the danger is in learners becoming off-task in their time spent in the classroom, and if they are not continually focused on the task and the learning content, then the project will not be successful. Educators with traditional backgrounds in teaching find Project Based Learning requires instructors to maintain student connection to content and management of their time – this is not necessarily a style that all teachers can accomplish (Efstratia, 2014, p 1258).
Project Based Learning is applicable to a number of different disciplines since it has various applications in learning, and is specifically relevant with the 21st century redefinition of education (differentiated, technologically-focused, collaboration, cross-curricular). STEM (Science, Technology, Engineering, Mathematics) is one form of 21st century education that benefits from instructors using Project Based Learning since it natural bridges between domains. The focus of STEM is to prepare secondary students for the rigors of post-secondary education and being able to solve complex problems in teams as would be expected when performing these jobs in the real world after graduation. Many potential occupational areas could benefit from Project Based Learning including medical, engineering, computer design, and education.
Project Based Learning allows secondary students the opportunity to broaden their knowledge and become successful in high-stakes situation (Capraro, et al., 2013, p 2). Moreover, these same students then develop a depth in knowledge when it comes to reflecting upon their strengths and limitations (Capraro, et al., 2013, p 2). The result would be a learner who has developed critical thinking and has had a chance to apply it to real situations. Further the construction of a finished product is a realistic expectation in presenting an authentic result from learning. The product result demands accountability, and learner adherent to instructor expectations as well as constraints for the project (Capraro, et al., 2013, p 2). The learner is disciplined to focus on specific outcomes, understand the parameters of the task, and demonstrate a viable artifact. The implication is that students will be ready to meet the challenges of a high-technology, fast-paced work world where innovation, collaboration, and results-driven product is essential for success. Technology is one area where Project Based Learning can be applied by developing skills in real-world application. For example, designers of computer systems with prior knowledge may be able to know how to trouble-shoot an operating system, but they do not really understand how things fit or work together, and they have a false sense of security about their skills (Gary, 2013, p 1).
Design-thinking follows a specific flow from theoretical to practical. It relies upon guided learning to promote effective learner solutions and goes beyond inquiry which has been argued does not work because it goes beyond the limits of long-term memory (Lazonder and Harmsen, 2016, p 2). Design-thinking requires the learner to have a meta-analysis of their process. Creativity (innovative thought) is evident in design thinking through studies in defocused and focused attention to stimuli in memory activation (Goldschmidt, 2016, p 1). Hu et al. (2010) developed a process of disrupted thinking in elementary students by having them use logical methods of critical thought towards specific design projects, over a four-year period, through specific lesson techniques. The results show that these students had increased thinking ability (SD .78) and that these effects have a long-term transfer increasing student academic achievement (Hu, et al. 2010, p 554). This shows use of divergent and convergent thinking in the creative process, and both of these process of thought has been noted to be important in the process of creativity (Goldschmidt, 2016, p 2) and demonstrates the Higher Order Thinking that is associated with long-term memory. Design-thinking specifically demonstrates the capability of having learners develop.
The Process of Argumentation [ edit | edit source ]
Argumentation stages [ edit | edit source ].
The psychological process of argumentation that allows one the produce, analyze and evaluate arguments [106] . These stages will be discussed in more detail later in this chapter.
The Impact of Argumentation on Learning [ edit | edit source ]
Argumentation does not only impact the development of CT and vice versa, it affects many other aspects of learning as well. For instance, a study conducted in a junior high school science class showed that when students engaged in argumentation, they drew heavily on their prior knowledge and experiences [107] . Not only did argumentation enable the students to use their prior knowledge, it also helped them consolidate knowledge and elaborate on their understanding of the subject at a higher level [107] . These are just a few of the ways in which argumentation can be seen to impact aspects of learning other than the development of CT.
Video: Argumentation in Education: https://www.youtube.com/watch?v=YHm5xUZmCDg
The Relationship between Critical Thinking and Argumentation [ edit | edit source ]
Argumentation and CT appear to have a close relationship in instruction. Many studies have shown the impact that both of these elements can have on one another. Data suggests that when CT is infused into instruction it impacts the ability of students to argue [108] tasks that involve both critical thinking and creative thinking must be of an argumentative nature [109] , and that argument analysis and storytelling can improve CT [110] . In other words it would appear that both CT and argumentation impact the development of each other in students and that both impact other aspects of learning and cognition.
How Critical Thinking Improves Argumentation [ edit | edit source ]
CT facilitates the evaluation of the information necessary to make an argument. It aids in the judgement of the validity of each position. It is used to assess the credibility of sources and helps in approaching the issue from multiple points of view. The elements of CT and argumentation have many common features. For example, examining evidence and counter-evidence of a statement and the information that backs up these claims are both facets of creating a sound argument and thinking critically.
The impact of how CT explicitly impacts one’s ability to argue and reason with reference to the aforementioned four CT components will be examined in this section. First, there needs to be an examination of the aspects of CT and how they can be impacted by argumentation. The first component, knowledge, as stated by Bruning et. al (2011), actively shapes the way in which one resolves problems [111] . Therefore, it is essential that students have a solid foundation of knowledge of whatever it is that they are arguing. The ability to use well founded information in order to effectively analyze the credibility of new information is imperative for students who wish to increase their argumentative abilities. The second component of CT that is important for argumentation is inference . As Chesñevar and Simari (2007) discuss in their examination of how we develop arguments, inference and deduction are essential aspects of reaching new conclusions from knowledge that is already known or proven [112] .
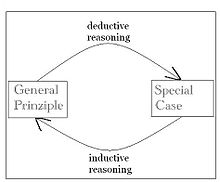
In other words, the ability to reach conclusions from known information is pivotal in developing and elaborating an argument. As well, the use of induction , a part of the CT process, is important to argumentation. As Bruning et al. suggest, the ability to make a general conclusion from known information is an essential part of the CT process [111] . Ontañón and Plaza (2015) make the argument that induction can be used in argumentation through communication with one another. Moreover, making inductions of general conclusions using the complete information that every member of the group can provide shows how interaction can be helpful through the use of induction in argumentation [113] . Therefore, it can be seen how induction, an important part of CT, can have a significant impact on argumentation and collaboration. The final component of CT, that may be the most important in its relationship to argumentation, is evaluation . The components of Evaluation indicated by Bruning et al. are analyzing, judging and weighing. These are three essential aspects of creating a successful argument [111] . Hornikx and Hahn (2012) provide a framework for three key elements of argumentation that are heavily attached in these Bruning et al.'s three aspects of CT [106] .
Production, Analysis, and Evaluation [ edit | edit source ]
The three aspects of argumentation that Hornikx and Hahn focus on in their research is the production , analysis and evaluation of arguments [106] . Producing an argument uses the key aspects of CT; there must be evaluation, analysis, judgement and weighing of the argument that one wishes to make a stand on. Analysis of arguments and analysis in CT go hand in hand, there must be a critical analysis of information and viewpoints in order to create a successful and fully supported argument. As well, evaluation is used similarly in argumentation as it is derived from CT. Assessing the credibility of sources and information is an essential part in finding articles and papers that can assist someone in making an informed decision. The final aspect of evaluation in critical thinking is metacognition, thinking about thinking or monitoring one's own thoughts [111] . Monitoring one's own thoughts and taking time to understand the rationality of the decisions that one makes is also a significant part of argumentation. According to Pinto et al.’s research, there is a strong correlation between one's argumentation ability and metacognition. [114] In other words, the ability to think about one’s own thoughts and the validity of those thoughts correlates positively with the ability to formulate sound arguments. The transfer of thoughts into speech/argumentation shows that CT influences argumentation dramatically, however some research suggests that the two interact in different ways as well. It can clearly be seen through the research presented that argumentation is heavily influenced by CT skills, such as knowledge, inference, evaluation and metacognition. However there are also strong implications that instruction of CT in a curriculum can bolster argumentation. A study conducted by Bensley et. al (2010) suggests that when CT skills are directly infused into a course compared to groups that received no CT instruction, those who received CT instruction showed significant gains in their ability of argument analysis [115] . There can be many arguments made for the implication of specific CT skills to impact argumentation, but this research shows that explicit teaching of CT in general can increase the ability of students to more effectively analyze arguments as well. This should be taken into account that Skills Programs mentioned later in this chapter should be instituted if teachers wish to foster argumentation as well as CT in the classroom.
How Argumentation Improves Critical Thinking [ edit | edit source ]
Argumentation is a part of the CT process, it clarifies reasoning and the increases one's ability to assess viable information. It is a part of metacognition in the sense that one needs to evaluate their own ideas. CT skills such as induction and/or deduction are used to create a structured and clear argument.
Research by Glassner and Schwarz (2007) shows that argumentation lies at the intersection of critical and creative thinking. They argue that reasoning, which is both critical and creative, is done through argumentation in adolescents. They suggest that reasoning is constantly being influenced by other perspectives and information. The ability to think creatively as well as critically about new information is managed by argumentation [116] . The back and forth process of accommodating, evaluating, and being open minded to new information can be argued as critical and creative thinking working together. However, the way in which one reaches conclusions from information is created from the ability to weigh this information, and then to successfully draw a conclusion regarding the validity of the solution that students come to. There is also a clear correlation of how argumentation helps students to nurture CT skills as well.
It is clear that CT can directly impact argumentation, but this relationship can also be seen as bidirectional, with argumentation instruction developing the CT skills. A study by Gold et al. shows that CT skills can be fostered through the use of argument analysis and storytelling in instruction [117] . This research suggests that argumentation and argument analysis are not only be beneficial to students, but also to older adults. This study was conducted using mature adult managers as participants. The article outlines four skills of CT that can be impacted by the use of argument analysis and storytelling: critique of rhetoric, tradition, authority, and knowledge. These four skills of CT are somewhat deeper than many instructed in high schools and extremely important to develop. The ability of argumentation to impact CT in a way that enables a person to gain a better perspective on their view about these things is essential to developing personal values as well as being able to use argumentation and CT to critique those values when presented with new information. The ability of argumentation to influence the ability of individuals to analyze their own traditions and knowledge is important for all students as it can give them better insight into what they value.
Argumentation is beneficial to CT skills as well as creative thinking skills in high school students. Research done by Demir and İsleyen (2015) shows that argumentation based a science learning approach in 9th graders improves both of types of thinking [118] . The ability of students to use argumentation to foster CT as well as creative thinking can be seen as being very beneficial, as mentioned earlier creative and CT skills use argumentation as a means of reasoning to draw conclusions, it is therefore not surprising that argumentation in instruction also fosters both of these abilities. In summation, it can clearly be seen that there is a link between both argumentation and CT along with many skills in the subset of CT skills. Explicit instruction of both of these concepts seems to foster the growth of the other and can be seen as complementary. In the next sections of this chapter how these aspects can be beneficial if taught within the curriculum and how they go hand in hand in fostering sound reasoning as well as skills that will help students throughout their lives will be examined.
Instructional Application of Argumentation and Critical Thinking [ edit | edit source ]

Teaching Tactics [ edit | edit source ]
An effective method for structuring the instruction of CT is to organize the thinking skills into a clear and sequential steps. The order in which these steps aid in guiding the student towards internalizing those steps in order to apply them in their daily lives. By taking a deductive approach, starting from broader skills and narrowing them down to task-specific skills helps the student begin from what they know and generate something that they hadn't known before through CT. In the spirit of CT, a student's awareness of their own skills also plays an important role in their learning. In the classroom, they should be encouraged to reflect upon the process through which they completed a goal rather than just the result. Through the encouragement of reflection, students can become more aware of the necessary thinking skills necessary for tasks, such as Argumentation.
Instructing CT and Argumentation predisposes the instruction to using CT skills first. In designing a plan to teach CT, one must be able to critically evaluate and assess different methods and make an informed decision on which would work best for one's class. There are a variety of approaches towards instructing CT. Descriptive Models consist of explanations of how "good" thinking occurs. Specifically, it focuses on thinking strategies such as heuristics to assess information and how to make decisions. Prescriptive Models consist of explanations of what good thinking should be. In a sense, these models give a prototype, a "prescription", of what good thinking is. This approach is comparatively less applicable and sets a high standard of what is expected of higher order thinking. In addition to evaluating which approach would work best for them, prior to teaching CT, instructors need to carefully select the specific types of CT skills that they want students to learn. This process involves assessing factors such as age range, performance level as well as cognitive ability of one's class in order to create a program that can benefit most of, if not all, the students. A final aspect of instruction to consider as an educator is whether direct or indirect instruction will be used to teach CT. Direct Instruction refers to the explicit teaching of CT skills that emphasizes rules and steps for thinking. This is most effective when solutions to problems are limited or when the cognitive task is easy. In contrast, Indirect Instruction refers to a learner-oriented type of teaching that focuses on the student building their own understanding of thinking. This is most effective when problems are ambiguous, unclear or open to interpretation such as moral or ethical decisions [111] .
One example of indirect CT instruction is through the process of writing literature reviews. According to Chandler and Dedman, having the skills to collect, assess and write literature reviews as well as summarize results of studies requires CT. In a teaching note, they evaluated a BSW (Baccalaureate of Social Work) program that strived to improve CT in undergraduate students. Specifically, they assert that practical writing assignments, such as creating literature reviews, help students combine revision and reflection while expanding their thinking to evaluate multiple perspectives on a topic. They found that upon reframing the assignment as a tool to facilitate students in becoming critical reviewers, students viewed the literature review as a summation of course material in addition to an opportunity to improve critical reading and writing skills. Through questioning during discussions, students were guided to analyze the authority and credibility of their articles. The students actively sought for more evidence to support articles on their topics. They found that students successfully created well synthesized literature reviews at the end of the BSW program [119] . This program used implicit instruction of CT skills through dialogue between instructor and students as well as peer engagement. Instead of explicitly stating specific skills or steps to learn CT, the instructors lead the students to practice CT through an assignment. As students worked on the assignment, they needed to use reasoning, analysis and inferential skills in order to synthesize and draw conclusions around the evidence they found on their topics. Practical application of CT skills through an assignment helped students develop CT through indirect instruction.
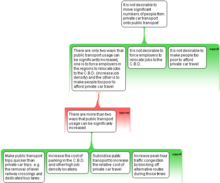
Argument mapping is a way to visualize argumentation. The following are links to argument mapping software: https://www.rationaleonline.com/ http://www.argunet.org/editor/ http://debategraph.org/planet https://www.truthmapping.com/map/1021/#s7164
Skills Programs for CT [ edit | edit source ]
These programs aid in the formulation of critical thinking skills through alternative methods of instruction such as problem-solving. They are usually targeted towards special populations such as students with learning disabilities or cognitive deficits.
The CoRT Thinking Materials [ edit | edit source ]
The CoRT (Cognitive Research Trust) program is based on de Bono’s idea that thinking skills should be taught in school as a subject [120] . The Thinking Materials are geared towards the improvement of thinking skills. This skills program takes on a Gestalt approach and emphasizes the perceptual factor of problem solving. It usually spans over the course of 2 years and is suitable for a wide age range of children. The lessons strive to develop creative thinking, problem-solving as well as interpersonal skills. The materials are split into 6 units and cover topics such as planning, analyzing, comparing, selecting, evaluating and generating alternatives. A typical unit has leaflets covering a single topic, followed by examples using practice items. The leaflets are usually effective in group settings. The focus of these units are to practice thinking skills, therefore much of the instructional time is spent on practicing the topics brought up in the leaflets [111] .
Much of the empirical research on this stand-alone program revolves around the development of creative thinking, however, it is relatively more extensive in comparison to the other programs mentioned in this chapter. The CoRT program has been shown to improve creativity in gifted students. Al-Faoury and Khwaileh (2014) assessed the effectiveness of the CoRT on gifted students’ creative writing abilities. The students were given a pretest that evaluated the fluency, flexibility and originality in writing creative short stories [120] . Students in the experimental group were taught 20 CoRT lessons in total with 10 from CoRT 1 “Breadth” and 10 from CoRT 4 “Creativity” over the course of three months while the control group received traditional lessons on creative writing. The posttest followed the same parameters as the pretest and the results were analyzed by comparing pre and posttest scores. The researchers found a statistically significant effect of CoRT on the experimental group’s fluency, flexibility and originality scores. The mean scores of the experimental groups in all three elements were higher than the control group [120] . These findings suggest that the CoRT program aids gifted students in creative writing skills as indicated through the use of rhetorical devices (metaphor, analogy, etc.), developing characters through dialogue and the control of complex structures [120] . The flexibility and fluency of writing is also applicable to the practice of argumentation and CT. In developing the ability to articulate and modify ideas, students can transfer these skills from creative writing towards higher-order cognitive processes such as CT and argumentation.
The Feuerstein Instrumental Enrichment Program (FIE) [ edit | edit source ]
The FIE is a specialized program focused on mediated learning experiences that strives to develop critical thinking and problem solving skills. Mediation is learning through interaction between the student and the mediator. Similar to Vygotsky's scaffolding, mediation is student-oriented and hinges upon 4 parameters: Intentionality, Reciprocity, Transcendence and Meaning. [121] Intentionality emphasizes the differences between mediation and interaction where the student and mediator have a common goal in mind. Reciprocity involves the student-oriented mentality of mediation, the response of the student hold most importance over academic results. Transcendence focuses on the connectivity of the mediation, it encourages the formation of associations and applications that stretch beyond the scope of the immediate material. Lastly, Meaning in mediation is where the student and mediator explicitly identify "why" and "what for" which promotes dialogue between the two during mediation. [121] [122]
The "instruments" used to facilitate instruction are a series of paper and pencil exercises geared towards practicing internalizing higher order thinking strategies. The instruments cover domains such as analytic perception, spatial organization, categorization, comparison and many more. The implementation of this program varies across countries and is also dependent on the targeted population. A typical program contains 14 units with 3-4 sessions for a few hours every week administered by trained IE staff and teachers. [121]
The Productive Thinking Program [ edit | edit source ]
The Productive Thinking Program consists of the development of planning skills, generating and checking hypotheses as well as creating new ideas. This program is designed as a set of 15 lessons aimed at being completed over one semester. The target population of the program is upper-level elementary school students. The lessons are administered through the use of narrative booklets, often taking a detective-like approach to problem solving where the student is the detective solving a mystery. A structured sequence of steps guides the student to attain an objective specific to the lesson at hand. [123] Following the booklet or story, supplementary problems are given in order for students to apply and practice learned skills. [111]
The IDEAL Problem Solver [ edit | edit source ]
The IDEAL Problem Solver structures problem-solving as 5 steps using the acronym IDEAL. First, (I)dentify the problem, the solver needs to find out what the problem is. Second, (D)efine the problem involves having a clear picture of the entire problem before trying to solve it. Third, (E)xplore the alternatives, meaning that the solver needs to assess the potential solutions available. Fourth, (A)cting on a plan, that is, applying the solution and doing the act of solving. Lastly, (L)ooking at the effects which encompasses the evaluation of the consequences of the chosen solution. IDEAL is flexible in that it can be adapted to suit a wide age range and different levels of ability in its application. It can also be applied to different domains such as composition or physics. [111]
Instructing Argumentation [ edit | edit source ]
Research on argumentation is a comparatively new field of study for education, but has been noted to be of significant importance to almost all educational settings. Grade schools, high schools, and colleges now emphasize the use of argumentation in the classroom as it is seen as the best way for communication and debate in a both vocational and educational settings around the world. [124] A longitudinal study done by Crowell and Kuhn showed that an effective way to help students gain argumentative skills was through consistent and dense application of argumentation in the classroom and as homework. [124] During this longitudinal study, students were exposed to a variety of different methods from which they gained argumentative abilities. The activities employed such as peer collaboration, using computers, reflection activities, individual essays, and small group work all have implications for being valuable in teaching argumentation although it is not clear which ones are the most effective. [124] Data also showed that students all rose to a similar level of argumentative ability, no matter what they scored on argumentative tests before the study began. This shows that even students with seemingly no argumentative skills can be instructed to become as skilled or more skilled than their peers who tested higher than them at the beginning of the study. [124]
Dialogue and Argumentation [ edit | edit source ]
Research by Crowell and Kuhn (2011) highlights collaborative dialogical activities as practical interventions in the development of argumentative skills. The researchers implemented a longitudinal argumentative intervention that used topic cycles to structure a middle school philosophy class [125] . The students had class twice a week for 50 minutes each class over the span of three years. The intervention is as follows: first, students were split into small groups on the same side of the argument to generate ideas around the topic (“for” and “against” teams). Then individuals from either side argue with an opponent through an electronic medium. Finally, the students engage in a whole class debate. These three stages were termed Pregame, Game and Endgame, respectively. After the intervention, students were required to write individual essays regarding the topic through which their argumentative skills would be assessed [125] . The results showed an increased in the generation of dual perspective arguments in the intervention group. Such arguments require the arguer to assume the opposing stance to one’s own and reason its implications. This type of argument reflects a higher-order reasoning that requires critical assessment of multiple perspectives. These results did not begin to appear until year two and was only found statistically significant in year three suggesting that argumentative skills have a longer development trajectory than other lower-level cognitive skills [125] . Through this stand-alone intervention, the collaborative aspect of dialogical activities facilitates the development of intellectual dispositions necessary for good argumentation [125] .

Further research suggests that teaching through the use of collaborative discussions and argumentative dialogue is an effective teaching strategy [105] . Through argumentation, students can acquire knowledge of concepts as well as the foundational ideas behind these concepts. In formulating arguments, students need to generate premises that provide structure to an argument through accepted definitions or claims. Argumentation helps students reveal and clarify misconceptions as well as elaborate on background knowledge. The two aforementioned dimensions of argumentation – dialogue and structure – are often used in assessing and measuring argumentative performance [105] . Specifically, through student-expert dialogue, the students can be guided to give certain arguments and counterarguments depending on the expert’s dialectical decisions [105] . This scaffolding helps the student engage in more critical evaluations that delve deeper into the topic in discussion.
In a study using content and functional coding schemes of argumentative behavior during peer-peer and peer-expert dialogue pairings, Macagno, Mayweg-Paus and Kuhn (2014) found that through student-expert dialogues, students were able to later formulate arguments that dealt with abstract concepts at the root of the issue at hand (i.e. ethical principles, conflict of values) in comparison to peer-peer dialogues [105] . The expert used more specific and sophisticated ways of attacking the student’s argument, such as suggesting an alternative solution to the problem at hand, which in turn enhanced the performance of the student in later meta-dialogues [105] . The results suggest that the practical application of argumentation through collaborate activities facilitates the development of argumentation skills. Similar to CT skills development, rather than teaching, implicit instruction through the practice of argumentation in interactive settings helps its development.
Science and Argumentation [ edit | edit source ]
Much of the literature surrounding the application of argumentation in the classroom revolves around the scientific domain. Argumentation is often used as a tool in scientific learning to enhance CT skills, improve class engagement and activate prior knowledge and beliefs around the subject [105] . In order to articulate and refine scientific theories and knowledge, scientists themselves utilize argumentation [104] . Jonassen and Kim (2010) assert that science educators often emphasize the role of argumentation more than other disciplines [126] . Argumentation supports the learning of how to solve well-structures problems as well as ill-structured ones in science, and from there by extension, in daily life. Specifically, the ill-structured ones reflect more practical everyday problems where goals and limitations are unclear and there are multiple solution pathways as well as multiple factors for evaluating possible solutions [104] .
Through argumentation, students learn to use sound reasoning and CT in order to assess and justify their solution to a problem. For example, a well-structured problem would be one posed in a physics class where concrete laws and formulas dictate the solution pathway to a problem or review questions found at the end textbook chapters which require the application of a finite set of concepts and theories. An ill-structured problem would be finding the cause of heart disease in an individual. Multiple developmental and lifestyle factors contribute to this one problem in addition to the various different forms of heart disease that need to be evaluated. This sort of problem requires the application of knowledge from other domains such as nutrition, emotional well-being and genetics. Since ill-structured problems do not have a definite answer, students are provided with an opportunity to formulate arguments that justify their solutions [104] . Through the practice of resolving problems in science, such as these, students can use CT to develop their argumentative ability.
One’s willingness to argue as well as one's ability to argue also play a significant role in learning science [127] . For one science is at its core, extremely argumentative.
If students have to ability to engage in argumentation at an early age then there knowledge of specific content such as science can grow immensely. The main reason for this is argumentative discourse, being able to disagree with others is extremely important because for adolescents they are at an age which is fundamentally social (ie junior to senior high) using this social ability is pivotal as students at this point may have the confidence to disagree with one another. When a student disagrees with another in argument in a classroom setting it gives them an opportunity to explain the way in which they think about the material. This verbalization of one’s own thoughts and ideas on a subject can help with learning the subject immensely [127] . It also allows for the student to reflect upon and expand their ideas as they have to present them to the class which helps with learning. This also provides the opportunity for the student to identify any misconceptions they have about the subject at hand as more than likely they will receive rebuttal arguments from others in their class [127] . All these factors are aspects of CT and contribute to the learning of the concept and conceptual change in the student which is what learning is all about. The nature of adolescent social behaviour could provide a window through which argumentation could benefit their learning in dramatic ways in learning science [127] .
Argumentation, Problem Solving and Critical Thinking in History Education [ edit | edit source ]
History education offers learners an abundant opportunity to develop their problem solving and critical thinking skills while broadening their perspective on the human condition. The study of history addresses a knowledge gap; specifically, it is the difference between our knowledge of present day and the “infinite, unorganized and unknowable everything that ever happened”. [128] It has long been understood that the study of history requires critical thought and analytical problem-solving skills. In order to become proficient at the study of history, learners must interpret and construct how we come to know about the past and navigate the connection between the past and the body of knowledge we call history. [129] Unfortunately, history education has been demoted to simply recalling factual information - via the overuse of rote memorization and multiple-choice testing - all of which is placed outside the context of present day. This approach does little to inspire a love of history nor does it support the learner’s ability to construct an understanding of how the past and present are connected.
On the other hand, the study of science and mathematics has for many years been centred around developing skills through problem-solving activities. Students learn basic skills and build upon these skills through a progression of increasingly complex problems in order to further their understanding of scientific theory and mathematical relationships. Specific to science education, learners are taught to think like scientists and approach problems using the scientific method. If this approach works well for science and math education, why should it not be utilized for the teaching of history? [128] . Therefore, to develop historical thinking skills it is necessary for instructors to teach the strategies and problem-solving approaches that are used by professional historians. However, unlike science and mathematics, the problems we solve in history are often ill-defined and may be unanswerable in a definitive sense making it more challenging for students to learn and transfer these skills. The following section will address these challenges and provide support for teaching historical thinking via The Big Six Historical Thinking Concepts (2013).
Historical Thinking - The Big Six [ edit | edit source ]
Based upon years of research and first-hand classroom experience, Seixas and Morton (2013) established a set of six competencies essential to the development of historical thinking skills. Much like science and mathematics education discussed above, the Big Six approach to history education allows the learner to progress from simplistic to advanced tasks. Moreover, the Big Six approach is intended to help the learner “move from depending on easily available, commonsense notions of the past to using the culture’s most powerful intellectual tools for understanding history”. (pg 1) [128] Additionally, the Big Six concepts reveal to the learner the difficulties we encounter while attempting to construct a history of the past. The Big Six competencies include the following: historical significance, evidence, continuity and change, cause and consequence, historical perspectives, and the ethical dimension.
Historical Significance
To develop a critical view of history the learner must recognize and define the qualities that makes something (e.g., person, event, social change) historically significant and why they should spend their time learning about this thing. Behaviourist approaches to history education, focusing on the textbook as the main source of information, have caused learners to become passive in their approach to learning about the past. The textbook becomes the authority on what they need to know. Moreover, the sole use of textbooks to teach national history may contribute to the creation of a “master narrative” that limits a student’s access to what is controversial about their country’s past. [130] By shifting the focus away from the textbook, learners may be able to further their critical thinking skills by following the steps historians take to study the past and constructing their own “reasoned decisions about historical significance”. [128] However, even if a learner is provided primary source evidence to construct a narrative of the past but is not taught to recognize the subjective side to historical thinking - why these pieces of evidence were selected, why this topic was selected, and why they are both historically significant - they may not recognize the impacts of human motivation on the construction of historic understanding. Unlike scientific inquiry that relies on a “positivistic definition of rationality”, historical thinking requires learners to acknowledge human motivation - their own motivation in studying the past, their instructors motivation for selecting certain topics of study, and the motivation of those living in the past [131]
Seixas & Morton (2013) cite two elements involved in constructing historical significance: “big, compelling concerns that exist in our lives today, such as environmental sustainability, justice, power, [and] welfare” and “particular events, objects, and people whose historical significance is in question” (pg 16) [128] The intersection between these two elements is where historical significance is found. It is useful here to add Freedman’s (2015), definition of critical historical reasoning . Critical historical reasoning requires us to recognize that the study of history is not objective. Historians “frame their investigations through the questions they pose and the theories they advance” and therefore, learners of history must analyze the “integrity of historical narratives and their pattern of emphasis and omission” (pg 360). [131] Critical historical reasoning aims towards “conscious awareness of the frame one has adopted and the affordances and constraints it imposes” (pg 360) [131] . Therefore, both historians and learners of history must recognize that historical significance is assigned and not an inherent feature of the past, and, importantly, is subject to change.
The second set of competencies described by Seixas and Morton (2013) are based on using evidence to address an inquiry about the past. In a study of the cognitive processes involved in evaluating source documents, Wineburg (1991) lists three heuristics: corroboration, sourcing, and contextualization. Corroboration refers to comparing one piece of evidence to another, sourcing is identifying the author(s) of the evidence prior to reading or viewing the material, and contextualization refers to situating evidence in a specific time and place (pg 77). [132]
This study utilized an expert/novice design to compare how historians and high school students make sense of historic documents. Wineburg (1991) argues that the historians were more successful in the task not because of the “schema-driven processing” common to science and mathematics, but by building a model of the [historic] event through the construction of “context-specific schema tailored to this specific event” (pg 83). [132] Additionally, historians demonstrated greater appreciation for the source of the historic documents compared to the students. This suggests that the students did not make the connection between a document's author and the reliability of the source. As Wineburg states, the historian understands “that there are no free-floating details, only details tied to witnesses, and if witnesses are suspect, so are their details” (pg. 84). [132] This study suggests the potential for historical understanding to be improved by teaching the cognitive strategies historians use to construct history.
Multiple narratives of the past exist as individuals bring their own values and experiences to their interpretations of historical evidence. Recognizing this may push learners beyond accepting historic accounts at face value and pull them towards a more critical approach to history. Inquiry-based guided discovery activities, such as Freedman’s (2015) Vietnam war narrative study, suggest that students may gain an awareness of the way they and others “frame” history through exploring primary source documents and comparing their accounts with standardized accounts (i.e. a textbook). [133] By allowing learners to view history as an interpretation of evidence rather than a fixed body of knowledge, we can promote critical thought through the learners’ creation of inferences based on evidence and construction of arguments to support their inferences.
Continuity and Change
Developing an understanding of continuity and change requires the learner to recognize that these two elements overlap over the chronology of history; some things are changing at the same time that other things remain the same. If students are able to recognize continuity and the processes of change in their own lives they should be able to transfer this understanding to their study of the past. [134] Students should be encouraged to describe and question the rate and depth of historic change as well as consider whether the change should be viewed as progress or decline. [134] The evaluation of historic change as positive or negative is, of course, dependent on the perspective taken by the viewer. An example of continuity through history is the development of cultural identity. Carretero and van Alphen (2014), explored this concept in their study of master narratives in Argentinian high school students. They suggest that identity can be useful to facilitate history education, but could also create misconceptions by the learner confounding past with present (or, presentism), as demonstrated when using “we” to discuss people involved in victorious battles or revolutions of the past which gave shape to a nation (pg 308-309). [130] It is useful, then to teach students to differentiate between periods of history. However, periodization of history, much like everything else in the knowledge domain, is based on interpretation and is dependent on the questions historians ask [134]
Educational technology such as interactive timelines, narrative history games, and online discussion groups may help learners make connections between the past and present. For example, the Museum of Civilization offers a teaching tool on the history of Canadian medicare ( http://www.museedelhistoire.ca/cmc/exhibitions/hist/medicare/medic01e.shtml ). Interactive timelines allow students to see connections between continuity, change, cause, and consequences by visually representing where these elements can be found over historic time. Also, guiding the learners’ exploration of interactive timelines by selecting strong inquiry questions may improve students understanding and facilitate the development of historical thinking. For example, an investigation into the European Renaissance could be framed by the following question: “Did everyone in Europe experience the Renaissance the same way?” Questions such as this are open-ended so as to not restrict where the students takes their inquiry but also suggest a relationship between the changes of the Renaissance and the continuity of European society. Other examples of educational technology that support historical thinking include the “Wold History for us All” ( http://worldhistoryforusall.sdsu.edu/ ) project. This website offers world history units separated into large-scale and local-scale topics and organized by historic period. The lesson plans and resources may allow the learner to making connections between local issues and the broader, global conditions affecting world history. Finally, a case study by Blackenship (2009) suggests that online discussion groups are a useful for developing critical thinking by allowing the teacher to view the students’ thought processes and thereby facilitating formative assessment and informing the type of instructional interventions required by the teacher. Blackenship (2009) cites additional research supporting the use of online discussion because it allows the learners to collect their thoughts before responding to a discussion prompt; they have more time to access prior knowledge and consider their own ideas. [135]
Cause and Consequence
The historical thinking competencies of cause and consequence require learners to become proficient at identifying direct and indirect causes of historic events as well as their immediate and long-term consequences. Effective understanding of the causes of historic change requires the recognition of both the actions of individuals as well as the prevailing conditions of the time. Historical thinking requires students to go beyond simplistic immediate causes and think of history as web of “interrelated causes and consequences, each with various influences” (pg 110). [134] In addition to improving understanding of the past, these competencies may help learners to better understand present-day conflicts and issues. Shreiner (2014) used the novice/expert format to evaluate how people utilize their knowledge of history to make reasoned conclusions about events of the present. Similar to the Wineburg (1991) study discussed above, Shreiner (2014) found the experts were better at contextualizing and using sourcing to critically analyze documents for reliability and utility in establishing a reasoned judgement. Additionally, the study found that while students would use narrative to construct meaning, they typically created schematic narrative templates - general statements about the past which lack specific details & events. [136] Seixas and Morton (2013) caution the use of overly-simplistic timelines of history because they could create a misconception that history is nothing more than a list of isolated events.The study indicates that historical narratives that follow periodization schemes and are characterized by cause-and-effect relationships, as well as change over time, are helpful for understanding contemporary issues. [134] Therefore, it is important that educators work to develop these competencies in students. Much like historic change, the consequences of certain actions in history can be viewed as positive and negative, depending on perspective. This will be discussed in further detail below.
Historical Perspectives and Ethics
The final two historical thinking competencies proposed by Seixas and Morton are historical perspectives and ethics. Historical perspectives refers to analyzing the historical context for conditions that would influence a historic figure to view an event or act in a particular way. This could include religious beliefs, social status, geographic location, time period, prevailing economic and political conditions, and social/cultural conditions. This again requires some interpretation of evidence as oftentimes we do not have evidence that explicitly describes a historic figure’s attitudes and reasons for acting. Primary source documents, such as letters and journals can provide insight but still require the historian to use inference to make sense of the documents and connect the information to a wider historical narrative or biographical sketch of an individual. Additionally, “[h]ard statistics, such as birth and death rates, ages of marriage, literacy rates, and family size... can all help us make inferences about people's experiences, thoughts, and feelings” (pg 143). [134] There are, of course, limitations to how much we can infer about the past; however, Seixas and Morton (2013) suggest that acknowledging the limitations of what we can know about the past is part of “healthy historical thinking” (pg 143). [134] Learners can develop their understanding of historical perspective by observing the contrast between past and present ways of life and worldviews, identifying universal human traits that transcend time periods (e.g., love for a child), and avoiding presentism and anachronism . [134] A greater understanding of historical perspective will be useful for students when encountering conflicting historical accounts as they will be able to see where the historical actors are “coming from” and therefore better understand their actions. Historical perspective and ethics are related. Seixas and Morton (2013) argue that “the ethical dimension of historical thinking helps to imbue the study of history with meaning” (pg 170). [134] To understand the moral reasons for an individual's actions we need to understand the influence of historical, geographical, and cultural context. Additionally, to understand ethical consequences of the past we make moral judgments which require “empathetic understanding[;] an understanding of the differences between our moral universe and theirs” (Seixas and Peck, 2004, pg 113). [137] People with little experience with historical thinking have difficulty separating the moral standards of today’s society with the societies of the past. Additionally, students tend to judge other cultures more critically than their own; oftentimes defending or justifying actions of their own nations. [138] Therefore, Lopez, Carretero and Rodriguez-Moneo (2014) suggest using national narratives of nations different from the learner’s own nation to more effectively develop critical historical thinking. As the learner becomes proficient at analyzing the ethical decisions of the past, they can translate these skills to analyzing present-day ethical questions. Role playing is a useful instructional strategy for teaching historical perspective. Traditional, face-to-face classrooms allow for dramatic role play activities, debates, and mock trials where students can take on the role of an individual or social group from history. Additionally, educational games and websites allow for the integration of technology while using the role play strategy. Whitworth and Berson (2003) found that, in the 1990-2000s, technology in the social studies classroom was focused mostly on using the internet as a digital version of material that would have otherwise been presented in the classroom. They suggest that alternative uses of technology - such as inquiry-based webquests, simulations, and collaborative working environments - promote interaction and critical thinking skills. [139] One example of a learning object that promotes critical thinking through role playing is the Musee-Mccord’s online game collection ( http://www.mccord-museum.qc.ca/en/keys/games/ ). Specifically, the Victorian Period and the Roaring Twenties games allow the learner to progress through the time period and make decisions appropriate to the historic context of the period. These games are paired with relevant resources from the museum collections which can enhance the learner’s depth of understanding of the period. In terms of teaching strategies for the ethical component of history can be explored through historical narratives, debating ethical positions on historic events, and evaluating and critiquing secondary sources of information for ethical judgements.
To summarize, introducing professional historians’ strategies for studying history is widely regarded as a way to improve historical thinking in students. Professional historian’s cognitive processes of corroborating accounts, critically analyzing sources, and establishing historic context are reflected well by Seixas and Morton’s Big Six Historical Thinking Concepts (2013). Historical thinking gives students the skills to problem solve within the context of history and make sense of the past and connect it to the present in order to broaden the learner’s perspective, understand prevailing social conditions, and influence how they interact with the world. See the Historical Thinking Project’s webpage ( http://historicalthinking.ca/lessons ) for instructional ideas for all the historical competencies.
Instructing through Academic Controversy [ edit | edit source ]
Using the technique of Academic Controversy could be an effective way of teaching both argumentation and CT skills to students. Academic controversy involves dividing a cooperative group of four in two pairs of students and assigning them opposing positions of an argument or issue, after which the two pairs each argue for their position. The groups then switch their positions and argue again, finally the group of four is asked to come up with an all-around solution to the problem [140] . This activity can be effective in instructing both aspects of argumentation and CT, though it may be a bit dated. The activity is argumentative by nature, making students come up with reasons and claims for two sets of arguments. This equilibrium is important to the argumentative process because provides the students with an opportunity to evaluate the key points of their argument and the opposition's which could be beneficial in any debate. As well, this activity is geared to engage students in a few aspects of CT such as evaluation, since the students must assess each side of the argument. It also engages metacognitive processes as the students must come up with a synthesized conclusion with their peers of their own arguments, a process which requires them to be both analytical and open minded. This activity is a good way of increasing both CT skills and argumentation as it requires students to be open-minded, but also engage in analytical debate.
Glossary [ edit | edit source ]
Suggested readings [ edit | edit source ].
- Abrami, P.C., Bernard, R.M., Borokhovski, E., Wade, A., Surkes, M.A., Tamim, R., & Zhang, D. (2008). Instructional Interventions Affecting Critical Thinking Skills and Dispositions: A Stage 1 Meta-Analysis. Review of Educational Research, 78(4). 1102-1134. DOI: 10.3102/0034654308326084.
- Phan, H.P. (2010). Critical thinking as a self-regulatory process component in teaching and learning. Psicothema, 22(2). 284-292.
- Kozulin, A. & Presseisen, B.Z. (1995). Mediated Learning Experience and Psychological Tools: Vygotsky’s and Feuerstein’s Perspective in a Study of Student Learning. Educational Psychologist, 30(2), 67-75.
- Crowell, A., & Kuhn, D. (2011). Dialogic Argumentation as a Vehicle for Developing Young Adolescents’ Thinking. Psychological Science, 22(4), 545-552. DOI: 10.1177/0956797611402512.
External links [ edit | edit source ]
- Critical Thinking: How Children Can Start Thinking Deeply, Part 1
- Critical Thinking for Kids In Action, Part 2
- Critical Thinking for Kids In Action, Part 3
- Critical Thinking for Kids In Action, Part 4
- Critical Thinking Exercises for Kids
References [ edit | edit source ]
- ↑ Heijltjes, A., Van Gog, T., & Paas, F. (2014). Improving Students' Critical Thinking: Empirical Support for Explicit Instructions Combined with Practice. Applied Cognitive Psychology, 28(4), 518-530.
- ↑ a b c d e f g Murphy, K. P., Rowe, M. L., Ramani, G., & Silverman, R. (2014). Promoting Critical-Analytic Thinking in Children and Adolescents at Home and in School. Educational Psychology Review, 26(4), 561-578.
- ↑ Gick, M. L. (1986). Problem-Solving Strategies. Educational Psychologist, 21(1/2), 99-121.
- ↑ a b c Ku, K. Y., Ho, I. T., Hau, K., & Lau, E. C. (2014). Integrating direct and Inquiry_Based Instruction in the teaching of critical thinking: An intervention study. Instructional Science, 42(2), 251-169.
- ↑ a b c d e f g h i j k l m n o p q Mathews, S. R., & Lowe, K. (2011). Classroom environments that foster a Disposition for Critical Thinking . Learning Environments Research, 14(1), 59-73.
- ↑ Glaser, E. M. (1941). An Experiment in the Development of Critical Thinking. Columbia University.
- ↑ a b c d Phan, H.P. (2010). Critical thinking as a self-regulatory process component in teaching and learning. Psicothema, 22(2). 284-292.
- ↑ a b c d e f Moon, J. (2007). Critical Thinking: An Exploration of Theory and Practice (1st ed.). London ; New York: Routledge.
- ↑ a b Kurfiss, J. G. (1988). Critical Thinking: Theory, Research, Practice, and Possibilities: ASHE-ERIC/Higher Education Research Report, Volume 17, Number 2, 1988 (1st ed.). Washington, D.C: Jossey-Bass.
- ↑ a b Board on Testing and Assessment, Division of Behavioral and Social Sciences and Education, & National Research Council. (2011). Assessing 21st Century Skills: Summary of a Workshop. National Academies Press.
- ↑ a b Mason, M. (2009). Critical Thinking and Learning. John Wiley & Sons.
- ↑ a b Elder, L., & Paul, R. (2009). The Art of Asking Essential Questions (5th Edition). Dillon Beach, CA: Foundation for Critical Thinking
- ↑ a b c Paul, R., & Elder, L. (2007). The Thinker’s Guide to The Art of Socratic Questioning. Dillon Beach, CA: The Foundation for Critical Thinking.
- ↑ a b c d Morgan, N., & Saxton, J. (2006). Asking Better Questions (2nd ed.). Markham, ON: Pembroke Publishers.
- ↑ a b Cain, R. B. (2007). The Socratic Method: Plato’s Use of Philosophical Drama. A&C Black.
- ↑ Harmon, D. A., & Jones, T. S. (2005). Elementary Education: A Reference Handbook. ABC-CLIO.
- ↑ Stanley, T., & Moore, B. (2013). Critical Thinking and Formative Assessments: Increasing the Rigor in Your Classroom. Routledge.
- ↑ a b Jung, I., Nishimura, M., & Sasao, T. (2016). Liberal Arts Education and Colleges in East Asia: Possibilities and Challenges in the Global Age. Springer.
- ↑ Mason, M. (2009). Critical Thinking and Learning. John Wiley & Sons. p. 8.
- ↑ Davies, M., & Barnett, R. (2015). The Palgrave Handbook of Critical Thinking in Higher Education. Springer.
- ↑ a b c d Cibáková, D. (2015). Methods of developing critical thinking when working with educative texts. E-Pedagogium, (2), 135-145.
- ↑ Garcia, T., & Pintrich, P. R. (1992). Critical Thinking and Its Relationship to Motivation, Learning Strategies, and Classroom Experience. 2-30.
- ↑ a b Halpern, D. F. (2013). Thought and Knowledge: An Introduction to Critical Thinking. Psychology Press.
- ↑ Browne, M. N., & Keeley, S. M. (2006). Asking the Right Questions: A Guide to Critical Thinking (8th ed.). Upper Saddle River, N.J: Prentice Hall.
- ↑ Elder, L., & Paul, R. (2009). The Art of Asking Essential Questions (5th Edition). Dillon Beach, CA: Foundation for Critical Thinking. p. 3.
- ↑ Micarelli, A., Stamper, J., & Panourgia, K. (2016). Intelligent Tutoring Systems: 13th International Conference, ITS 2016, Zagreb, Croatia, June 7-10, 2016. Proceedings. Springer.
- ↑ a b c d e Conklin, W., & Teacher Created Materials. (2012). Strategies for Developing Higher-Order Thinking Skills, Grades 6 - 12. Shell Education.
- ↑ http://www.janinesmusicroom.com/socratic-questioning-part-i-the-framework.html
- ↑ Bloom, B. S., Krathwohl, D. R., & Masia, B. B. (1984). Taxonomy of educational objectives: the classification of educational goals. Longman.
- ↑ Blosser, P. E. (1991). How to Ask the Right Questions. NSTA Press.
- ↑ Wang, J.-F., & Lau, R. (2013). Advances in Web-Based Learning -- ICWL 2013: 12th International Conference, Kenting, Taiwan, October 6-9, 2013, Proceedings. Springer.
- ↑ a b Gregory, G., & Kaufeldt, M. (2015). The Motivated Brain: Improving Student Attention, Engagement, and Perseverance. ASCD.
- ↑ Carol, K., & Sandi, Z. (2014). Q Tasks, 2nd Edition: How to empower students to ask questions and care about answers. Pembroke Publishers Limited.
- ↑ a b Doubet, K. J., & Hockett, J. A. (2015). Differentiation in Middle and High School: Strategies to Engage All Learners. ASCD.
- ↑ a b c http://www.educ.kent.edu/fundedprojects/tspt/units/sixfacets.htm
- ↑ a b McTighe, J., & Wiggins, G. (2013). Essential Questions: Opening Doors to Student Understanding (1st ed.). Alexandria, Va. USA: Association for Supervision & Curriculum Development.
- ↑ a b c d e f g h i j k l m n o p q Bruning, G.J. Schraw & M.M. Norby (2011) Cognitive Psychology and Instruction (5th Ed). New York: Pearson.
- ↑ Anderson, J. R. Cognitive Psychology and Its Implications. New York: Freeman, 1980
- ↑ a b Mayer, R. E., & Wittrock, R. C. (2006). Problem solving. In P. A. Alexander & P. H. Winne (Eds.), Handbook of educational psychology (2nd ed., pp. 287–304). Mahwah, NJ: Erlbaum.
- ↑ Snyder, M. J., & Snyder, L. G. (2008). Teaching critical thinking and Problem solving skills. Delta Pi Epsilon Journal, L(2), 90-99.
- ↑ a b c Voss, J. F. (1988). Problem solving and reasoning in ill-structured domains. In C. Antaki (Ed.), Analyzing everyday explanation: A casebook of methods (pp. 74-93). London: SAGE Publications.
- ↑ a b Pretz, J. E., Naples, A. J., & Sternberg, R. J. (2003). Recognizing, defining, and representing problems. In J. E. Davidson and R. J. Sternberg (Eds.), The psychology of problem solving (pp. 3–30). Cambridge, UK: Cam- bridge University Press.
- ↑ a b c d Shin, N., Jonassen, D. H., & McGee, S. (2003). Predictors of Well-Structured and Ill-Structured Problem Solving in an Astronomy Simulation. Journal Of Research In Science Teaching, 40(1), 6-33.8
- ↑ Simon, D. P. (1978). Information processing theory of human problem solving. In W. K. Estes (Ed.), Handbook of learning and cognitive process. Hillsdale, NJ: Lawrence Erlbau
- ↑ Kitchener, K.S., Cognition, metacognition, and epistemic cognition. Human Development, 1983. 26: p. 222-232.
- ↑ Schraw G., Dunkle, M. E., & Bendixen L. D. (1995). Cognitive processes in well-structured and ill-structured problem solving. Applied Cognitive Psychology, 9, 523–538.
- ↑ Cho, K. L., & Jonassen, D. H. (2002) The effects of argumentation scaffolds on argumentation and problem solving. Educational Technology: Research & Development, 50(3), 5-22.
- ↑ a b Jonassen, D. H. (2000). Revisiting activity theory as a framework for designing student-centered learning environments. In D. H. Jonassen, S. M. Land, D. H. Jonassen, S. M. Land (Eds.) , Theoretical foundations of learning environments (pp. 89-121). Mahwah, NJ, US: Lawrence Erlbaum Associates Publishers.
- ↑ Spiro, R. J., Feltovich, P. J., Jacobson, M. J., & Coulson, R. L. (1992). Cognitive flexibility, constructivism, and hypertext: Random access instruction for advanced knowledge acquisition in ill-structured domains. In T. M. Duffy, D. H. Jonassen, T. M. Duffy, D. H. Jonassen (Eds.) , Constructivism and the technology of instruction: A conversation (pp. 57-75). Hillsdale, NJ, England: Lawrence Erlbaum Associates, Inc.
- ↑ a b c d e Barrows, H. S. (1996). “Problem-based learning in medicine and beyond: A brief overview.” In L. Wilkerson & W. H. Gijselaers (Eds.), Bringing Problem-Based Learning to higher education: Theory and practice (pp. 3-12). San Francisco: Jossey Bass.
- ↑ a b (Barron, B., & Darling-Hammond, L. (2008). Teaching for meaningful learning: A review of research on inquiry-based and cooperative learning. Powerful Learning: What We Know About Teaching for Understanding (pp. 11-70). San Francisco, CA: Jossey-Bass.)
- ↑ Dochy, F., Segers, M., Van den Bossche, P., & Gijbels, D. (2003). Effects of problembased learning: A meta-analysis. Learning and Instruction, 13, 533–568.
- ↑ Williams, D., Hemstreet, S., Liu, M.& Smith, V. (1998). Examining how middle school students use problem-based learning software. Unpublished paper presented at the ED-Media/ED Telecom ‘98 world Conference on Educational Multimedia and Hypermedia & World Conference on Educational Telecommunications, Freiberg, Germany.
- ↑ Gallagher, S. A., Stepien, W. J., & Rosenthal, H. (1992). The effects of problem based learning on problem solving. Gifted Child Quarterly, 36, 195–200. Gertzman, A., & Kolodner, J. L.
- ↑ Bruning, G.J. Schraw & M.M. Norby (2011) Cognitive Psychology and Instruction (5th Ed). New York: Pearson.
- ↑ Martin, L., and D. L. Schwartz. 2009. “Prospective Adaptation in the Use of External Representations.” Cognition and Instruction 27 (4): 370–400. doi:10.1080/
- ↑ Chambers, D., and D. Reisberg. 1985. “Can Mental Images Be Ambiguous?” Journal of Experimental Psychology: Human A pragmatic perspective on visual representation and creative thinking Perception and Performance 11 (3): 317–328.
- ↑ a b Öllinger, M., Jones, G., & Knoblich, G. (2008). Investigating the effect of mental set on insight problem solving. Experimental Psychology, 55(4), 269-282. doi:10.1027/1618-3169.55.4.269
- ↑ Duncker, K. (1945). On Problem Solving. Psychological Monograph, Whole No. 270.
- ↑ a b c McCaffrey, T. (2012). Innovation relies on the obscure: A key to overcoming the classic problem of functional fixedness. Psychological Science, 23(3), 215-218.
- ↑ a b Taconis, R., Ferguson-Hessler, M. G. M., & Broekkamp, H. (2002). Teaching science problem solving: An overview of experimental work. Journal of Research in Science Teaching, 38, 442–46
- ↑ Bransford, J. D., Brown, A. L., & Cocking, R. R. (Eds.). (2000). How people learn: Brain, mind, experience, and school. Washington, DC: National Academies Press.
- ↑ a b Novick, L. R., & Bassok, M. (2005). Problem solving. In K. Holyoak & R. Morrison (Eds.), The Cambridge handbook of thinking and reasoning (pp. 321–350). Cambridge, UK: Cambridge University Press.
- ↑ Fuchs, L. S., Fuchs, D., Stuebing, K., Fletcher, J. M., Hamlett, C. L., & Lambert, W. (2008). Problem solving and computational skills: Are they shared or distinct aspects of mathematical cognition? Journal of Educa- tional Psychology, 100, 30–
- ↑ a b McNeill, K., & Krajcik, J. (2008). Scientific explanations: Characterizing and evaluating the effects of teachers’ instructional practices on student learning. Journal of Research in Science Teaching, 45, 53–78.
- ↑ a b c d e Aleven, V. A. W. M. M., & Koedinger, K. R. (2002) An effective meta-cognitive strategy: learning by doing and explaining with a computer-based Cognitive Tutor. Cognitive Science, 26(2), 147–179.
- ↑ a b c d e f g h i Anderson, J. R., Corbett, A. T., Koedinger, K. R., & Pelletier, R. (1995). Cognitive Tutors: Lessons learned. Journal of the Learning Sciences, 4(2), 167.
- ↑ a b c d e f Corbett, A. T., & Anderson, J. R. (2001). Locus of feedback control in computer-based tutoring: Impact on learning rate, achievement and attitudes. In Proceedings of the SIGCHI Conference on Human Factors in Computing Systems (pp. 245–252). New York, NY, USA: ACM.
- ↑ a b c d e f g Koedinger, K. R., & Corbett, A. (2006). Cognitive tutors. The Cambridge handbook of the learning sciences, 61-77.
- ↑ Koedinger, K. R. (2002). Toward evidence for instructional design principles: Examples from Cognitive Tutor Math 6. In Proceedings of PME-NA XXXIII (The North American Chapter of the International Group for the Psychology of Mathematics Education).
- ↑ Corbett, A., Kauffman, L., Maclaren, B., Wagner, A., & J,.ones, E. (2010). A Cognitive Tutor for genetics problem solving: Learning gains and student modeling. Journal of Educational Computing Research, 42(2), 219–239.
- ↑ Corbett, A. T., & Anderson, J. R. (2008). Knowledge decomposition and sub-goal reification in the ACT programming tutor. Department of Psychology, 81.
- ↑ Corbett, A. T., & Bhatnagar, A. (1997). Student modelling in the ACT programming tutor: Adjusting a procedural learning model with declarative knowledge. In User modelling (pp. 243-254). Springer Vienna.
- ↑ Corbett, A. (2002). Cognitive tutor algebra I: Adaptive student modelling in widespread classroom use. In Technology and assessment: Thinking ahead. proceedings from a workshop (pp. 50-62).
- ↑ Koedinger, K. R. & Anderson, J. R. (1993). Effective use of intelligent software in high school math classrooms. In Proceedings of the World Conference on Artificial Intelligence in Education, (pp. 241-248). Charlottesv
- ↑ Koedinger, K., Anderson, J., Hadley, W., & Mark, M. (1997). Intelligent tutoring goes to school in the big city. Human-Computer Interaction Institute.
- ↑ Koedinger, K. R., & Corbett, A. (2006). Cognitive tutors. The Cambridge handbook of the learning sciences, 61-77.
- ↑ Resnick, L. B. (1987). Learning in school and out. Educational Researcher, 16(9), 13-20.
- ↑ Polya, G. (1957). How to Solve It: A New Aspect of Mathematical Method. (2nd ed.). Princeton, NJ: Princeton University Press.
- ↑ <Aleven, V. A. W. M. M., & Koedinger, K. R. (2002). An effective metacognitive strategy: learning by doing and explaining with a computer-based Cognitive Tutor. Cognitive Science, 26(2), 147–179. p. 173
- ↑ Corbett, A., Kauffman, L., Maclaren, B., Wagner, A., & Jones, E. (2010). A Cognitive Tutor for genetics problem solving: Learning gains and student modelling. Journal of Educational Computing Research, 42(2), 219–239.
- ↑ Morgan, Alistar. (1983). Theoretical Aspects of Project-Based Learning in Higher Education. British Journal of Educational Technology, 14(1), 66-78.
- ↑ a b c d Blumenfeld, Phyllis C., Elliot Soloway, Ronald W. MArx, Joseph S. Krajick, Mark Guzdial, Annemarie Palincsar.. (1991). Motivating Project-Based Learning: Sustaining the Doing, Supporting the Learning. Educational Psychologist, 26(3&4), 369-398.
- ↑ a b c d e f Thomas, John W. (2000). A Review of Research on Project-Based Learning. San Rafael: Autodesk Press.
- ↑ a b van Merriënboer, J. J. G., Clark, R. E., & de Croock, M. B. M. (2002). Blueprints for complex learning: The 4C/ID-model. Educational Technology Research and Development, 50(2), 39–61. doi:10.1007/bf02504993.
- ↑ a b Dewey, J. (1938). Experience and education. New York: Macmillan.
- ↑ a b c d e van Merrienboer, J. J. G., Paul A. Kirschner, & Liesbeth Kester. (2004). Taking the Load off a Learner’s Mind: Instructional Design for Complex Learning. Amsterdam: Open University of the Netherlands.
- ↑ Piaget, Jean; Cook, Margaret (Trans). The construction of reality in the child. New York, NY, US: Basic Books The construction of reality in the child. (1954). xiii 386 pp. http://dx.doi.org/10.1037/11168-000 .
- ↑ Ames, C. (1992). Classrooms: goals, structures, and student motivation. Journal of Educational Psychology, 84, 261-271.
- ↑ Helle, Laura, Paivi Tynjara, Erkki Olkinora, Kristi Lonka. (2007). “Aint nothin’ like the real thing”. Motivation and study processes on a work-based project course in information systems design. British Journal of Educational Psychology, 70(2), 397-411.
- ↑ a b Joseph Krajcik , Phyllis C. Blumenfeld , Ronald W. Marx , Kristin M. Bass , Jennifer Fredricks & Elliot Soloway (1998) Inquiry in Project-Based Science Classrooms: Initial Attempts by Middle School Students, Journal of the Learning Sciences, 7:3-4, 313-350, DOI: 10.1080/10508406.1998.9672057
- ↑ a b c Gorges, Julia, Thomas Goke. (2015). How do I Know what I can do? Anticipating expectancy of success regarding novel academic tasks. British Journal of Educational Psyschology, 85(1), 75-90.
- ↑ Hung, C.-M., Hwang, G.-J., & Huang, I. (2012). A Project-based Digital Storytelling Approach for Improving Students' Learning Motivation, Problem-Solving Competence and Learning Achievement. Educational Technology & Society , 15 (4), 368–379.
- ↑ a b Efstratia, Douladeli. (2014). Experiential education through project based learning. Procedia – Social and Behavioral Sciences . 152, 1256-1260.
- ↑ a b c Capraro, R. M., Capraro, M. M., & Morgan, J. (2013). STEM Project-Based Learning: An Integrated Science, Technology, Engineering, and Mathematics (STEM) Approach (2nd Edition). New York, NY: Sense.
- ↑ Gary, Kevin. (2013), Project-Based Learning. Computer. (Vol 48:9). Tempe: Arizona State.
- ↑ a b c d e f g h Cross, N. (2007). Designerly ways of knowing . Basel, Switzerland: Birkha¨user.
- ↑ Simon, H. A. (1996). The sciences of the artificial . Cambridge, MA: MIT Press.
- ↑ Aflatoony, Leila & Ron Wakkary, (2015). Thoughtful Thinkers: Secondary Schooler’s Learning about Design Thinking. Simon Fraser University: Surrey, BC.
- ↑ a b Koh, Joyce Hwee Ling, Chin Sing Chai, Benjamin Wong, & Huang-Yao Hong. (2015) Design Thinking for Education. Singapore: Springer Science + Business Media.
- ↑ a b c d Schon, D. A. (1983). The reflective practitioner: How professionals think in action (Vol. 5126). New York, NY: Basic Books.
- ↑ Hu, Weiping, Philip Adey, Xiaojuan Jia, Jia Liu, Lei Zhang, Jing Li, Xiaomei Dong. (2010). Effects of a “Learn to Think” Intervention programme on primary school students. British Journal of Educational Psychology . 81(4) 537-557.
- ↑ a b Wells, Alastair. (2013). The importance of design thinking for technological literacy: a phenomenological perspective . International Journal Technol Des Educ. (23:623-636). DOI 10.1007/s10798-012-9207-7.
- ↑ a b c d Jonassen, D.H., & Kim, B. (2010). Arguing to learn ad learning to argue: design justifications and guidelines. Education Technology & Research Development, 58(4), 439-457. DOI 10.1007/s11423-009-9143-8.
- ↑ a b c d e f g h Macagno, F., Mayweg-Paus, W., & Kuhn, D. (2014). Argumentation theory in Education Studies: Coding and Improving Students’ Argumentative Strategies. Topoi, 34, 523-537.
- ↑ a b c Hornikx, J., & Hahn, U. (2012). Reasoning and argumentation: Towards an integrated psychology of argumentation. Thinking & Reasoning, 18(3), 225-243. DOI: 10.1080/13546783.2012.674715.
- ↑ a b Aufschnaiter, C., Erduran, S., Osborne, J., & Simon, S. (2008). Arguing to learn and learning to argue: Case studies of how students' argumentation relates to their scientific knowledge. Journal of Research in Science Teaching, 45(1), 101-131. doi:10.1002/tea.20213
- ↑ Bensley, A., Crowe, D., Bernhardt, P., Buckner, C., & Allman, A. (2010). Teaching and assessing CT skills for argument analysis in psychology. Teaching of Psychology, 37(2), 91-96. doi:10.1080/00986281003626656
- ↑ Glassner, A., & Schwarz, B. B. (2007). What stands and develops between creative and critical thinking? argumentation?. Thinking Skills and Creativity, 2(1), 10-18. doi:10.1016/j.tsc.2006.10.001
- ↑ Gold J., Holman D., & Thorpe R. (2002). The role of argument analysis and story telling in facilitating critical thinking. Management Learning, 33(3), 371-388. doi:10.1177/1350507602333005
- ↑ a b c d e f g h Bruning, R. H., Schraw, G. J., & Norby, M. M. (2011). Cognitive psychology and instruction (5th ed.) Pearson.
- ↑ Chesñevar, I., & Simari, G. (2007). Modelling inference in argumentation through labelled deduction: Formalization and logical properties. Logica Universalis, 2007, Volume 1, Number 1, Page 93, 1(1), 93-124. doi:10.1007/s11787-006-0005-4
- ↑ Ontañón, S., & Plaza, E. (2015). Coordinated inductive learning using argumentation-based communication. Autonomous Agents and Multi-Agent Systems, 29(2), 266-304. doi:10.1007/s10458-014-9256-2
- ↑ Pinto, M., Iliceto, P., & Melagno, S. (2012). Argumentative abilities in metacognition and in metalinguistics: A study on university students. European Journal of Psychology of Education, 27(1), 35-58. doi:10.1007/s10212-011-0064-7
- ↑ Bensley, A., Crowe, D., Bernhardt, P., Buckner, C., & Allman, A. (2010). Teaching and assessing critical thinking skills for argument analysis in psychology. Teaching of Psychology, 37(2), 91-96. doi:10.1080/00986281003626656
- ↑ Demir, B., & İsleyen, T. (2015). The effects of argumentation based science learning approach on creative thinking skills of students. Educational Research Quarterly, 39(1), 49-82.
- ↑ Chandler, S. & Dedman, D.E. (2012). Writing a Literature Review: An Essential Component of Critical Thinking. The Journal of Baccalaureate Social Work, 17. 160-165.
- ↑ a b c d Al-Faoury, O.H., & Khwaileh, F. (2014). The Effect of Teaching CoRT Program No. (4) Entitles “Creativity” on the Gifted Learners’ Writing in Ein El-Basha Center for Gifted Students. Theory and Practice in Language Studies, 4(11), 2249-2257. doi:10.4304/tpls.4.11.2249-2257.
- ↑ a b c Kozulin, A. & Presseisen, B.Z. (1995). Mediated Learning Experience and Psychological Tools: Vygotsky’s and Feuerstein’s Perspective in a Study of Student Learning. Educational Psychologist, 30(2), 67-75.
- ↑ Presseisen, B.Z. & Kozulin, A. (1992). Mediated Learning – The Contributions of Vygotsky and Feuerstein in Theory and Practice.
- ↑ Schuler, G. (1974). The Effectiveness of the Productive Thinking Program. Paper presented at the Annual Meeting of the American Educational Research Association. Retrieved from: http://www.eric.ed.gov/contentdelivery/servlet/ERICServlet?accno=ED103479 .
- ↑ a b c d Crowell, A., & Kuhn, D. (2014). Developing dialogic argumentation skills: A 3-year intervention study. Journal of Cognition and Development, 15(2), 363-381. doi:10.1080/15248372.2012.725187
- ↑ a b c d Crowell, A., & Kuhn, D. (2011). Dialogic Argumentation as a Vehicle for Developing Young Adolescents’ Thinking. Psychological Science, 22(4), 545-552. DOI: 10.1177/0956797611402512.
- ↑ Jonassen, D.H., & Kim, B. (2010). Arguing to learn ad learning to argue: design justifications and guidelines. Education Technology & Research Development, 58(4), 439-457. DOI 10.1007/s11423-009-9143-8.
- ↑ a b c d Bathgate, M., Crowell, A., Schunn, C., Cannady, M., & Dorph, R. (2015). The learning benefits of being willing and able to engage in scientific argumentation. International Journal of Science Education, 37(10), 1590-1612. doi:10.1080/09500693.2015.1045958
- ↑ a b c d e Seixas, P., Morton, T., Colyer, J., & Fornazzari, S. (2013). The Big Six: Historical thinking Concepts. Toronto: Nelson Education.
- ↑ Osborne, K. (2013). Forward. Seixas, P., Morton, T., Colyer, J., & Fornazzari, S. The Big Six: Historical thinking Concepts. Toronto: Nelson Education.
- ↑ a b Carretero, M., & van Alphen, F. (2014). Do Master Narratives Change Among High School Students? A Characterization of How National History Is Represented. Cognition and Instruction, 32(3), 290–312. http://doi.org/10.1080/07370008.2014.919298
- ↑ a b c Freedman, E. B. (2015). “What Happened Needs to Be Told”: Fostering Critical Historical Reasoning in the Classroom. Cognition and Instruction, 33(4), 357–398. http://doi.org/10.1080/07370008.2015.1101465
- ↑ a b c Wineburg, S. S. (1991). Historical problem solving: A study of the cognitive processes used in the evaluation of documentary and pictorial evidence. Journal of Educational Psychology, 83(1), 73–87. http://doi.org/10.1037/0022-0663.83.1.73
- ↑ Freedman, E. B. (2015). “What Happened Needs to Be Told”: Fostering Critical Historical Reasoning in the Classroom. Cognition and Instruction, 33(4), 357–398. http://doi.org/10.1080/07370008.2015.1101465
- ↑ a b c d e f g h i Seixas, P., Morton, T., Colyer, J., & Fornazzari, S. (2013). The Big Six: Historical thinking Concepts. Toronto: Nelson Education.
- ↑ Blackenship, W. (2009). Making connections: Using online discussion forums to engage students in historical inquiry. Social Education, 73(3), 127-130.
- ↑ Shreiner, T. L. (2014). Using Historical Knowledge to Reason About Contemporary Political Issues: An Expert–Novice Study. Cognition and Instruction, 32(4), 313–352. http://doi.org/10.1080/07370008.2014.948680
- ↑ Seixas, P., & Peck, C. (2004). Teaching Historical Thinking. Challenges and Prospects for Canadian Social Studies, 109–117.
- ↑ Lopez, C., Carretero, M., & Rodriguez-Moneo, M. (2014). Telling a national narrative that is not your own. Does it enable critical historical consumption? Culture & Psychology , 20 (4 ), 547–571. http://doi.org/10.1177/1354067X14554156
- ↑ Whitworth, S. A., & Berson, M. J. (2003). Computer technology in the social studies: An examination of the effectiveness literature (1996-2001). Contemporary Issues in Technology and Teacher Education [Online serial], 2(4). Retrieved from http://www.citejournal.org/volume-2/issue-4-02/social-studies/computer-technology-in-the-social-studies-an-examination-of-the-effectiveness-literature-1996-2001
- ↑ Johnson, D. W., & Johnson, R. T. (1993). Creative and critical thinking through academic controversy. The American Behavioral Scientist, 37(1), 40-53. Retrieved from https://www.proquest.com/docview/1306753602
- Book:Cognition and Instruction
Navigation menu

An official website of the United States government
The .gov means it’s official. Federal government websites often end in .gov or .mil. Before sharing sensitive information, make sure you’re on a federal government site.
The site is secure. The https:// ensures that you are connecting to the official website and that any information you provide is encrypted and transmitted securely.
- Publications
- Account settings
Preview improvements coming to the PMC website in October 2024. Learn More or Try it out now .
- Advanced Search
- Journal List
- Front Psychol
The Efficacy and Development of Students' Problem-Solving Strategies During Compulsory Schooling: Logfile Analyses
Gyöngyvér molnár.
1 Department of Learning and Instruction, University of Szeged, Szeged, Hungary
Benő Csapó
2 MTA-SZTE Research Group on the Development of Competencies, University of Szeged, Szeged, Hungary
The purpose of this study was to examine the role of exploration strategies students used in the first phase of problem solving. The sample for the study was drawn from 3 rd - to 12 th -grade students (aged 9–18) in Hungarian schools ( n = 4,371). Problems designed in the MicroDYN approach with different levels of complexity were administered to the students via the eDia online platform. Logfile analyses were performed to ascertain the impact of strategy use on the efficacy of problem solving. Students' exploration behavior was coded and clustered through Latent Class Analyses. Several theoretically effective strategies were identified, including the vary-one-thing-at-a-time (VOTAT) strategy and its sub-strategies. The results of the analyses indicate that the use of a theoretically effective strategy, which extract all information required to solve the problem, did not always lead to high performance. Conscious VOTAT strategy users proved to be the best problem solvers followed by non-conscious VOTAT strategy users and non-VOTAT strategy users. In the primary school sub-sample, six qualitatively different strategy class profiles were distinguished. The results shed new light on and provide a new interpretation of previous analyses of the processes involved in complex problem solving. They also highlight the importance of explicit enhancement of problem-solving skills and problem-solving strategies as a tool for knowledge acquisition in new contexts during and beyond school lessons.
Introduction
Computer-based assessment has presented new challenges and opportunities in educational research. A large number of studies have highlighted the importance and advantages of technology-based assessment over traditional paper-based testing (Csapó et al., 2012 ). Three main factors support and motivate the use of technology in educational assessment: (1) the improved efficiency and greater measurement precision in the already established assessment domains (e.g., Csapó et al., 2014 ); (2) the possibility of measuring constructs that would be impossible to measure by other means (e.g., Complex Problem Solving (CPS) 1 ; see Greiff et al., 2012 , 2013 ); and (3) the opportunity of logging and analyzing not only observed variables, but metadata as well (Lotz et al., 2017 ; Tóth et al., 2017 ; Zoanetti and Griffin, 2017 ). Analyzing logfiles may contribute to a deeper and better understanding of the phenomenon under examination. Logfile analyses can provide answers to research questions which could not be answered with traditional assessment techniques.
This study focuses on problem solving, especially on complex problem solving (CPS), which reflects higher-order cognitive processes. Previous research identified three different ways to measure CPS competencies: (1) Microworlds (e.g., Gardner and Berry, 1995 ), (2) formal frameworks (Funke, 2001 , 2010 ) and (3) minimal complex systems (Funke, 2014 ). In this paper, the focus is on the MicroDYN approach, which is a specific form of complex problem solving (CPS) in interactive situations using minimal complex systems (Funke, 2014 ). Recent analyses provide both a new theory and data-based evidence for a global understanding of different problem-solving strategies students employ or could employ in a complex problem-solving environment based on minimal complex systems.
The problem scenarios within the MicroDYN approach consist of a small number of variables and causal relations. From the perspective of the problem solver, solving a MicroDYN problem requires a sequence of continuous activities, in which the outcome of one activity is the input for the next. First, students interact with the simulated system, set values for the input variables, and observe the impacts of these settings on the target (dependent) variable. Then, they plot their conclusion about the causal relationships between the input and output variables on a graph (Phase 1). Next, they manipulate the independent variables again to set their values so that they result in the required values for the target variables (Phase 2).
When it comes to gathering information about a complex problem, as in the MicroDYN scenarios, there may be differences between the exploration strategies in terms of efficacy. Some of them may be more useful for generating knowledge about the system. Tschirgi ( 1980 ) identified different exploration strategies. When control of variables strategies (Greiff et al., 2014 ) were explored, findings showed that the vary-one-thing-at-a-time (VOTAT, Tschirgi, 1980 ; Funke, 2014 ) was the most effective strategy for identifying causal relations between the input and output variables in a minimal complex system (Fischer et al., 2012 ). Participants who employed this strategy tended to acquire more structural knowledge than those who used other strategies (Vollmeyer et al., 1996 ; Kröner et al., 2005 ). With the VOTAT strategy, the problem solver systematically varies only one input variable, while the others remain unchanged. This way, the effect of the variable that has just been changed can be observed directly by monitoring the changes in the output variables. There exist several types of VOTAT strategies.
Using this approach—defining the effectiveness of a strategy on a conceptual level, independently of empirical effectiveness—we developed a labeling system and a mathematical model based on all theoretically effective strategies. Thus, effectiveness was defined and linked to the amount of information extracted. An exploration strategy was defined as theoretically effective if the problem solver was able to extract all the information needed to solve the problem, independently of the application level of the information extracted and of the final achievement. We split the effectiveness of the exploration strategy and the usage and application of the information extracted to be able to solve the problem and control the system with respect to the target values based on the causal knowledge acquired. Systematicity was defined on the level of effectiveness based on the amount of information extracted and on the level of awareness based on the implementation of systematicity in time.
Students' actions were logged and coded according to our input behavior model and then clustered for comparison. We were able to distinguish three different VOTAT strategies and two successful non-VOTAT ones. We empirically tested awareness of the input behavior used in time. Awareness of strategy usage was analyzed by the sequence of the trials used, that is, by the systematicity of the trials used in time. We investigated the effectiveness of and differences in problem-solving behavior between three age groups by conducting latent class analyses to explore and define patterns in qualitatively different VOTAT strategy uses.
Although the assessment of problem solving within the MicroDYN approach is a relatively new area of research, its processes have already been studied in a number of different contexts, including a variety of educational settings with several age groups. Our cross-sectional design allows us to describe differences between age groups and outline the developmental tendencies of input behavior and strategy use among children in the age range covered by our data collection.
Reasoning strategies in complex problem solving
Problem-solving skills have been among the most extensively studied transversal skills over the last decade; they have been investigated in the most prominent comprehensive international large-scale assessments today (e.g., OECD, 2014 ). The common aspects in the different theoretical models are that a problem is characterized by a gap between the current state and the goal state with no immediate solution available (Mayer and Wittrock, 1996 ).
Parallel to the definition of the so-called twenty first-century skills (Griffin et al., 2012 ), recent research on problem solving disregards content knowledge and domain-specific processes. The reason for this is that understanding the structure of unfamiliar problems is more effective when it relies on abstract representation schemas and metacognitive strategies than on specifically relevant example problems (Klahr et al., 2007 ). That is, the focus is more on assessing domain-general problem-solving strategies (Molnár et al., 2017 ), such as complex problem solving, which can be used to solve novel problems, even those arising in interactive situations (Molnár et al., 2013 ).
Logfile analyses make it possible to divide the continuum of a problem-solving process into several scoreable phases by extracting information from the logfile that documents students' problem-solving behavior. In our case, latent class analysis extracts information from the file that logs students' interaction with the simulated system at the beginning of the problem-solving process. The way students manipulate the input (independent) variables represents their reasoning strategy. Log data, on the one hand, make it possible to analyze qualitative differences in these strategies and then their efficiency in terms of how they generate knowledge resulting in the correct plotting of the causal relationship in Phase 1 and then the proper setting to reach the required target value in Phase 2. On the other hand, qualitative strategy data can be quantified, and an alternative scoring system can be devised.
From the perspective of the traditional psychometric approach and method of scoring, these problems form a test task consisting of two scoreable items. The first phase is a knowledge acquisition process, where scores are assigned based on how accurately the causal relationship was plotted. The second phase is knowledge application, where the correctness of the value for the target variable is scored. Such scoring based on two phases of solving MicroDYN problems has been used in a number of previous studies (e.g., Greiff et al., 2013 , 2015 ; Wüstenberg et al., 2014 ; Csapó and Molnár, 2017 ; Greiff and Funke, 2017 ).
To sum up, there is great potential to investigate and cluster the problem-solving behavior and exploration strategy usage of the participants at the beginning of the problem-solving process and correlate the use of a successful exploration strategy with the model-building solution (achievement in Phase 1) observed directly in these simulated problem scenarios. Using logfile analyses (Greiff et al., 2015 ), the current article wishes to contribute insights into students' approaches to explore and solve problems related to minimal complex systems. By addressing research questions on the problem-solving strategies used, the study aims to understand students' exploration behavior in a complex problem-solving environment and the underlying causal relations. In this study, we show that such scoring can be developed through latent class analysis and that this alternative method of scoring may produce more reliable tests. Furthermore, such scoring can be automated and then employed in a large-scale assessment.
There are two major theoretical approaches to cognition relevant to our study; both offer general principles to interpret cognitive development beyond the narrower domain of problem solving. Piaget proposed the first comprehensive theory to explain the development of children's thinking as a sequence of four qualitatively different stages, the formal operational stage being the last one (Inhelder and Piaget, 1958 ), while the information processing approach describes human cognition by using terms and analogies borrowed from computer science. The information processing paradigm was not developed into an original developmental theory; it was rather aimed at reinterpreting and extending Piaget's theory (creating several Neo-Piagetian models) and synthesizing the main ideas of the two theoretical frameworks (Demetriou et al., 1993 ; Siegler, 1999 ). One of the focal points of these models is to explain the development of children's scientific reasoning, or, more closely, the way children understand how scientific experiments can be designed and how causal relationships can be explored by systematically changing the values of (independent) variables and observing their impact on other (target) variables.
From the perspective of the present study, the essential common element of cognitive developmental research is the control of variables strategy. Klahr and Dunbar ( 1988 ) distinguished two related skills in scientific thinking, hypothesis formation and experimental design, and they integrated these skills into a coherent model for a process of scientific discovery. The underlying assumption is that knowledge acquisition requires an iterative process involving both. System control as knowledge application tends to include both processes, especially when acquired knowledge turns out to be insufficient or dysfunctional (J. F. Beckmann, personal communication, August 16, 2017). Furthermore, they separated the processes of rule induction and problem solving, defining the latter as a search in a space of rules (Klahr and Dunbar, 1988 , p. 5).
de Jong and van Joolingen ( 1998 ) provided an overview of studies in scientific discovery learning with computer simulations. They concluded that a number of specific skills are needed for successful discovery, like systematic variation of variable values, which is in a focus of the present paper, and the use of high-quality heuristics for experimentation. They identified several characteristic problems in the discovery process and stressed that learners often have trouble interpreting data.
In one of the earliest systematic studies of students' problem-solving strategies, Vollmeyer et al. ( 1996 ) explored the impact of strategy systematicity and effectiveness on complex problem-solving performance. Based on previous studies, they distinguished the VOTAT strategy from other possible strategies [Change All (CA) and Heterogeneous (HT) other strategies], as VOTAT allows systematic exploration of the behavior of a system and a disconfirmation of hypotheses. In one of their experiments, they examined the hypothesis that VOTAT was more effective for acquiring knowledge than less systematic strategies. According to the results, the 36 undergraduate students had clearly shown strategy development. After interacting with the simulated system in several rounds, they tended to use the VOTAT strategy more frequently. In a second experiment, it was also demonstrated that goal specificity influences strategy use as well (Vollmeyer et al., 1996 ).
Beckmann and Goode ( 2014 ) analyzed the systematicity in exploration behavior in a study involving 80 first-year psychology students and focusing on the semantic context of a problem and its effect on the problem solvers' behavior in complex and dynamic systems. According to the results, a semantically familiar problem context invited a high number of a priori assumptions on the interdependency of system variables. These assumptions were less likely tested during the knowledge acquisition phase, this proving to be the main barrier to the acquisition of new knowledge. Unsystematic exploration behavior tended to produce non-informative system states that complicated the extraction of knowledge. A lack of knowledge ultimately led to poor control competency.
Beckmann et al. ( 2017 ) confirmed research results by Beckmann and Goode ( 2014 ) and demonstrated how a differentiation between complexity and difficulty leads to a better understanding of the cognitive mechanism behind CPS. According to findings from a study with 240 university students, the performance differences observed in the context of the semantic effect were associated with differences in the systematicity of the exploration behavior, and the systematicity of the exploration behavior was reflected in a specific sequence of interventions. They argued that it is only the VOTAT strategy—supplemented with the vary- none -at-a-time strategy in the case of noting autonomous changes—that creates informative system state transitions which enable problem solvers to derive knowledge of the causal structure of a complex, dynamic system.
Schoppek and Fischer ( 2017 ) also investigated VOTAT and the related “PULSE” strategy (all input variables to zero), which enables the problem solver to observe the eigendynamics of the system in a transfer experiment. They proposed that besides VOTAT and PULSE, other comprehensive knowledge elements and strategies, which contribute to successful CPS, should be investigated.
In a study with 2 nd - to 4 th -grade students, Chen and Klahr found little spontaneous development when children interacted with physical objects (in situations similar to that of Piaget's experiments), while more direct teaching of the control of variables strategy resulted in good effect sizes and older children were able to transfer the knowledge they had acquired (improved control of variable strategy) to remote contexts (Chen and Klahr, 1999 ). In a more recent study, Kuhn et al. ( 2008 ) further extended the scope of studies on scientific thinking, identifying three further aspects beyond the control of variables strategy, including coordinating effects of multiple influences, understanding the epistemological foundations of science and engaging in argumentation. In their experiment with 91 6th-grade students, they explored how students were able to estimate the impact of five independent variables simultaneously on a particular phenomenon, and they found that most students considered only one or two variables as possible causes.
In this paper, we explore several research questions on effective and less effective problem-solving strategies used in a complex problem-solving environment and detected by logfile analyses. We use logfile analyses to empirically test the success of different input behavior and strategy usage in CPS tasks within the MicroDYN framework. After constructing a mathematical model based on all theoretically effective strategies, which provide the problem solver with all the information needed to solve the problem, and defining several sub-strategies within the VOTAT strategy based on the amount of effort expended to extract the necessary information, we empirically distinguish different VOTAT and non-VOTAT strategies, which can result in good CPS performance and which go beyond the isolated variation strategy as an effective strategy for rule induction (Vollmeyer et al., 1996 ). We highlight the most and least effective VOTAT strategies used in solving MicroDYN problems and empirically investigate the awareness of the strategy used based on the sequence of the sub-strategies used. Based on these results, we conduct latent class analyses to explore and define patterns in qualitatively different VOTAT strategy uses.
We thus intend to answer five research questions:
- RQ1: Does the use of a theoretically effective strategy occur prior to high performance? In other words, does the use of a theoretically effective strategy result in high performance?
- RQ2: Do all VOTAT strategies result in a high CPS performance? What is the most effective VOTAT strategy?
- RQ3: How does awareness of the exploration strategy used influence overall performance on CPS tasks?
- RQ4: What profiles characterize the various problem solvers and explorers?
- RQ5: Do exploration strategy profiles differ across grade levels, which represent different educational stages during compulsory schooling?
In this study, we investigated qualitatively different classes of students' exploration behavior in CPS environments. We used latent class analysis (LCA) to study effective and non-effective input behavior and strategy use, especially the principle of isolated variation, across several CPS tasks. We compared the effectiveness of students' exploration behavior based on the amount of information they extracted with their problem-solving achievement. We posed five separate hypotheses.
Hypothesis 1: We expect that high problem-solving achievement is not closely related to expert exploration behavior.
Vollmeyer et al. ( 1996 ) explored the impact of strategy effectiveness on problem-solving performance and reported that effectiveness correlated negatively and weakly to moderately with solution error ( r = −0.32 and r = −0.54, p < 0.05). They reported that “most participants eventually adopted the most systematic strategy, VOTAT, and the more they used it, the better they tended to perform. However, even those using the VOTAT strategy generally did not solve the problem completely” (p. 88). Greiff et al. ( 2015 ) confirmed that different exploration behaviors are relevant to CPS and that the number of sub-strategies implemented was related to overall problem-solving achievement.
Hypothesis 2: We expect that students who use the isolated variation strategy in exploring CPS problems have a significantly better overall performance than those who use a theoretically effective, but different strategy.
Sonnleiter et al. ( 2017 ) noted that “A more effective exploration strategy leads to a higher system knowledge score and the higher the gathered knowledge, the better the ability to achieve the target values. Thus, system knowledge can be seen as a reliable and valid measure of students' mental problem representations” (p. 169). According to Wüstenberg et al. ( 2012 ), students who consistently apply the principle of isolated variation—the most systematic VOTAT strategy—in CPS environments show better overall CPS performance, compared to those who use different exploration strategies. Kröner et al. ( 2005 ) reported a positive correlation between using the principle of isolated variation and the likelihood of solving the overall problem.
Hypothesis 3: We expected that more aware CPS exploration behavior would be more effective than exploration behavior that generally results in extracting all the necessary information from the system to solve the problem, but within which the steps have no logically built structure and no systematicity in time.
Vollmeyer et al. ( 1996 ) explored the impact of strategy systematicity on problem-solving performance. They emphasized that “the systematicity of participants' spontaneous hypothesis-testing strategies predicted their success on learning the structure of the biology lab problem space” (p. 88). Vollmeyer and her colleagues restricted systematic strategy users to isolated variation strategy users; this corresponds to our terminology usage of aware isolated variation strategy users.
Hypothesis 4: We expected to find a distinct number of classes with statistically distinguishable profiles of CPS exploration behavior. Specifically, we expected to find classes of proficient, intermediate and low-performing explorers.
Several studies (Osman and Speekenbrink, 2011 ; Wüstenberg et al., 2012 ; Greiff et al., 2015 ) have indicated that there exist quantitative differences between different exploration strategies, which are relevant to a CPS environment. The current study is the first to investigate whether a relatively small number of qualitatively different profiles of students' exploration proficiency can be derived from their behavior detected in a CPS environment in a broad age range.
Hypothesis 5: We expected that more proficient CPS exploration behavior would be more dominant at later grade levels as an indication of cognitive maturation and of increasing abilities to explore CPS environments.
The cognitive development in children between Grades 3 and 12 is immense. According to Piaget's stage theory, they move from concrete operations to formal operations and they will be able to think logically and abstractly. According to Galotti ( 2011 ) and Molnár et al. ( 2013 ), the ability to solve problems effectively and to make decisions in CPS environments increases in this period of time; Grades 6–8 seem especially crucial for development. Thus, we expect that cognitive maturation will also be reflected in more proficient exploration behavior.
Participants
The sample was drawn from 3 rd - to 12 th -grade students (aged 9–18) in Hungarian primary and secondary schools ( N = 4,371; Table Table1). 1 ). School classes formed the sampling unit. 180 classes from 50 schools in different regions were involved in the study, resulting in a wide-ranging distribution of students' background variables. The proportion of boys and girls was about the same.
Composition of samples.
The MicroDYN approach was employed to develop a measurement device for CPS. CPS tasks within the MicroDYN approach are based on linear structural equations (Funke, 2001 ), in which up to three input variables and up to three output variables are related (Greiff et al., 2013 ). Because of the small set of input and output variables, the MicroDYN problems could be understood completely with precise causal analyses (Funke, 2014 ). The relations are not presented to the problem solver in the scenario. To explore these relations, the problem solver must interact directly with the problem situation by manipulating the input variables (Greiff and Funke, 2010 ), an action that can influence the output variables (direct effects), and they must use the feedback provided by the computer to acquire and employ new knowledge (Fischer et al., 2012 ). Output variables can change spontaneously and can consist of internal dynamics, meaning they can change without changing the input variables (indirect effects; Greiff et al., 2013 ). Both direct and indirect effects can be detected with an adequate problem-solving strategy (Greiff et al., 2012 ). The interactions between the problem situation and the test taker play an important role, but they can only be identified in a computerized environment based on log data collected during test administration.
In this study, different versions with different levels of item complexity were used (Greiff et al., 2013 ), which varied by school grade (Table (Table2; 2 ; six MicroDYN scenarios were administered in total in Grades 3–4; eight in Grade 5: nine in Grades 6–8; and twelve in Grades 9–12); however, we only involved those six tasks where the principle of isolated variation was the optimal exploration strategy. That is, we excluded problems with an external manipulation-independent, internal dynamic effect or multiple dependence effect from the analyses, and there were no delayed or accumulating effects used in the problem environments created. Complexity was defined by the number of input and output variables and the number of relations based on Cognitive Load Theory (Sweller, 1994 ). “Findings show that increases in the number of relations that must be processed in parallel in reasoning tasks consistently lead to increases in task difficulty” (Beckmann and Goode, 2017 ).
The design of the whole study: the complexity of the systems administered and the structure and anchoring of the tests applied in different grades.
The tasks were designed so that all causal relations could be identified with systematic manipulation of the inputs. The tasks contained up to three input variables and up to three output variables with different fictitious cover stories. The values of the input variables were changed by clicking on a button with a + or – sign or by using a slider connected to the respective variable (see Figure Figure1). 1 ). The controllers of the input variables range from “– –” (value = −2) to “++” (value = +2). The history of the values of the input variables within the same scenario was presented on a graph connected to each input variable. Beyond the input and output variables, each scenario contained a Help, Reset, Apply and Next button. The Reset button set the system back to its original status. The Apply button made it possible to test the effect of the currently set values of the input variables on the output variables, which appeared in the form of a diagram of each output variable. According to the user interface, within the same phase of each of the problem scenarios, the input values remained at the level at which they were set for the previous input until the Reset button was pressed or they were changed manually. The Next button implemented the navigation between the different MicroDYN scenarios and the different phases within a MicroDYN scenario.

Exploration in phase 1 of the MicroDYN problems (two input variables and two output variables).
In the knowledge acquisition phase, participants were freely able to change the values of the input variables and attempt as many trials for each MicroDYN scenario as they liked within 180 s. During this 180 s, they had to draw the concept map (or causal diagram; Beckmann et al., 2017 ); that is, they had to draw the arrows between the variables presented on the concept map under the MicroDYN scenario on screen. In the knowledge application phase, students had to check their respective system using the right concept map presented on screen by reaching the given target values within a given time frame (90 s) in no more than four trials, that is, with a maximum of four clicks on the Apply button. This applied equally to all participants.
All of the CPS problems were administered online via the eDia platform. At the beginning, participants were provided with instructions about the usage of the user interface, including a warm-up task. Subsequently, participants had to explore, describe and operate unfamiliar systems. The assessment took place in the schools' ICT labs using the available school infrastructure. The whole CPS test took approximately 45 min to complete. Testing sessions were supervised by teachers who had been thoroughly trained in test administration. Students' problem-solving performance in the knowledge acquisition and application phases was automatically scored as CPS performance indicators; thus, problem solvers received immediate performance feedback at the end of the testing session. We split the sample into three age groups, whose achievement differed significantly (Grades 3–5, N = 1,871; Grades 6–7, N = 1,284; Grades 8–12, N = 1,216; F = 122.56, p < 0.001; t level_1_2 = −6.22, p < 0.001; t level_2_3 = −8.92, p < 0.001). This grouping corresponds to the changes in the developmental curve relevant to complex problem solving. The most intensive development takes place in Grades 6–7 (see Molnár et al., 2013 ). Measurement invariance, that is, the issue of structural stability, has been demonstrated with regard to complex problem solving in the MicroDYN approach already (e.g., Greiff et al., 2013 ) and was confirmed in the present study (Table (Table3). 3 ). Between group differences can be interpreted as true and not as psychometric differences in latent ability. The comparisons across grade levels are valid.
Goodness of fit indices for measurement invariance of MicroDYN problems.
χ 2 and df were estimated by the weighted least squares mean and variance adjusted estimator (WLSMV). Δχ 2 and Δdf were estimated by the Difference Test procedure in MPlus. Chi-square differences between models cannot be compared by subtracting χ 2 s and dfs if WLSMV estimators are used. CFI, comparative fit index; TLI, Tucker Lewis index; RMSEA, root mean square error of approximation .
The latent class analysis (Collins and Lanza, 2010 ) employed in this study seeks students whose problem-solving strategies show similar patterns. It is a probabilistic or model-based technique, which is a variant of the traditional cluster analysis (Tein et al., 2013 ). The indicator variables observed were re-coded strategy scores. Robust maximum likelihood estimation was used and two to seven cluster solutions were examined. The process of latent class analysis is similar to that of cluster analysis. Information theory methods, likelihood ratio statistical test methods and entropy-based criteria were used in reducing the number of latent classes. As a measure of the relative model fit, AIC (Akaike Information Criterion), which considers the number of model parameters, and BIC (Bayesian Information Criterion), which considers the number of parameters and the number of observations, are the two original and most commonly used information theory methods for model selection. The adjusted Bayesian Information Criterion (aBIC) is the sample size-adjusted BIC. Lower values indicated a better model fit for each criterion (see Dziak et al., 2012 ). Entropy represents the precision of the classification for individual cases. MPlus reports the relative entropy index of the model, which is a re-scaled version of entropy on a [0,1] scale. Values near one, indicating high certainty in classification, and values near zero, indicating low certainty, both point to a low level of homogeneity of the clusters. Finally, the Lo–Mendell–Rubin Adjusted Likelihood Ratio Test (Lo et al., 2001 ) was employed to compare the model containing n latent classes with that containing n −1 latent classes. A significant p -value ( p < 0.05) indicates that the n −1 model is rejected in favor of a model with n classes, as it fits better than the previous one (Muthén and Muthén, 2012 ).
As previous research has found (Greiff et al., 2013 ), achievement in the first and second phases of the problem-solving process can be directly linked to the concept of knowledge acquisition (representation) and knowledge application (generating a solution) and was scored dichotomously. For knowledge acquisition, students' responses were scored as correct (“1”) if the connections between the variables were accurately indicated on the concept map (students' drawings fully matched the underlying problem structure); otherwise, the response was scored as incorrect (“0”). For knowledge application, students' responses were scored as correct (“1”) if students reached the given target values within a given time frame and in no more than four steps, that is, with a maximum of four clicks on the Apply button; otherwise, the response was scored as incorrect (“0”).
We developed a labeling procedure to divide the continuum of the problem-solving process into more scoreable phases and to score students' activity and behavior in the exploration phase at the beginning of the problem-solving process. For the different analyses and the most effective clustering, we applied a categorization, distinguishing students' use of the full, basic and minimal input behavior within a single CPS task (detailed description see later). The unit of this labeling process was a trial, a setting of the input variables, which was tested by clicking on the Apply button during the exploration phase of a problem, thus between receiving the problem and clicking on the Next button to reach the second part, the application part of the problem. The sum of these trials, within the same problem environment is called the input behavior. The input behavior was called a strategy if it followed meaningful regularities.
By our definition, the full input behavior model describes what exactly was done throughout the exploration phase and what kinds of trials were employed in the problem-solving process. It consists of all the activities with the sliders and Apply buttons in the order they were executed during the first phase, the exploration phase of the problem-solving process. The basic input behavior is part of the full input behavior model by definition, when the order of the trials attempted was still being taken into account, but it only consists of activities where students were able to acquire new information on the system. This means that the following activities and trials were not included in the basic input behavior model (they were deleted from the full input behavior model to obtain the basic behavior model):
- - where the same scenario, the same slider adjustment, was employed earlier within the task (that is, we excluded the role of ad hoc control behavior from the analyses),
- - where the value (position) of more than one input variable (slider) was changed and where the effect of the input variable on the operation of the system was still theoretically unknown to the problem solver,
- - where a new setting or new slider adjustment was employed, though the effect of the input variables used was known from previous settings.
- - As the basic input behavior involves timing, that is, the order of the trials used, it is suitable for the analyses with regard to the awareness of the input behavior employed.
Finally, we generated the students' minimal input behavior model from the full input behavior model. By our definition, the minimal input behavior focuses on those untimed activities (a simple list, without the real order of the trials), where students were able to obtain new information from the system and were able to do so by employing the most effective trials.
Each of the activities in which the students engaged and each of the trials which they used were labeled according to the following labeling system to be able to define students' full input behavior in a systematic format (please note that the numerical labels are neither scores nor ordinal or metric information):
- Only one single input variable was manipulated, whose relationship to the output variables was unknown (we considered a relationship unknown if its effect cannot be known from previous settings), while the other variables were set at a neutral value like zero. We labeled this trial +1.
- One single input variable was changed, whose relationship to the output variables was unknown. The others were not at zero, but at a setting used earlier. We labeled this trial +2.
- One single input variable was changed, whose relationship to the output variables was unknown, and the others were not at zero; however, the effect of the other input variable(s) was known from earlier settings. Even so, this combination was not attempted earlier. We labeled this trial +3.
- Everything was maintained in a neutral (zero) position. This trial is especially important for CPS problems with their own internal dynamics. We labeled this +A.
- The value of more than one input variable, whose relationship to the output variables was unknown, was changed at the same time, resulting in no additional information on the system. It was labeled –X.
- The same trial, the slider adjustment, had already been employed earlier within the task, resulting in no additional information on the system. It was labeled −0.
- A new slider adjustment was employed; however, the effect of the manipulated input variables was known from previous settings. This trial offered no additional information on the system and was labeled +0.
Although several input variables were changed by the scenario, it was theoretically possible to count the effect of the input variables on the output variables based on the information from the previous and present settings by using and solving linear equations. It was labeled +4.
An extra code (+5) was employed in the labeling process, but only for the basic input behavior, when the problem solver was able to figure out the structure of the problem based on the information obtained in the last trial used. This labeling has no meaning in the case of the minimal input behavior.
The full, basic and minimal input behavior models as well as the labeling procedure can be employed by analyzing problem solvers' exploration behavior and strategies for problems that are based on minimal complex systems. The user interface can preserve previous input values, and the values are not reset to zero after each exploration input. According to Fischer et al. ( 2012 ), VOTAT strategies are best for identifying causal relations between variables and they maximize the successful strategic behavior in minimal complex systems, such as CPS. By using a VOTAT strategy, the problem solver systematically varies only one input variable, while the others remain unchanged. This way, the effect of the changed variable can be found in the system by monitoring the changes in the output variables. There exist several types of VOTAT strategies based on the different combinations of VOTAT-centered trials +1, +2, and +3. The most obvious systematic strategy is when only one input variable is different from the neutral level in each trial and all the other input variables are systematically maintained at the neutral level. Thus, the strategy is a combination of so-called +1 trials, where it is employed for every input variable. Known as the isolated variation strategy (Müller et al., 2013 ), this strategy has been covered extensively in the literature. It must be noted that the isolated variation strategy is not appropriate to detect multiple dependence effects within the MicroDYN approach. We hypothesize that there are more and less successful input behaviors and strategies. We expect that theoretically effective, non-VOTAT strategies do not work as successfully as VOTAT strategies and that the most effective VOTAT strategy will be the isolated variation strategy.
We will illustrate the labeling and coding process and the course of generating a minimal input behavior out of a basic or full input behavior through the following two examples.
Figure Figure1 1 shows an example with two input variables and two output variables. (The word problem reads as follows: “When you get home in the evening, there is a cat lying on your doorstep. It is exhausted and can barely move. You decide to feed it, and a neighbor gives you two kinds of cat food, Miaow and Catnip. Figure out how Miaow and Catnip impact activity and purring.”). The student who mapped the operation of the system as demonstrated in the figure pressed the Apply button six times in all, using the various settings for the Miaow and Catnip input variables.
In mapping the system, the problem solver kept the value of both the input variables at 0 in the first two steps (making no changes to the base values of the input variables), as a result of which the values of the output variables remained unchanged. In steps 3 and 4, he set the value of the Miaow input variable at 2, while the value of the Catnip variable remained at 0 (the bar chart by the name of each variable shows the history of these settings). Even making this change had no effect on the values of the output variables; that is, the values in each graph by the purring and activity variables are constantly horizontal. In steps 5 and 6, the student left the value of the Miaow input variable at 2, but a value of 2 was added to this for the Catnip input variable. As a result, the values of both output variables (purring and activity) began to grow by the same amount. The coding containing all the information (the full input behavior) for this sequence of steps was as follows: +A, −0, +1, −0, +2, −0. The reason for this is since steps 2, 4, and 6 were repetitions of previous combinations, we coded them as −0. Step 3 involved the purest use of a VOTAT strategy [changing the value of one input variable at a time, while keeping the values of the other input values at a neutral level (+1)], while the trial used in step 5 was also a VOTAT strategy. After all, only the value of one input variable changed compared to step 4. This is therefore not the same trial as we described in step 3 (+2). After step 5, all the necessary information was available to the problem solver. The basic input behavior for the same sequence of steps was +A, +1, +2, since the rest of the steps did not lead the problem solver to acquire unknown information. Independently of the time factor, the minimal input behavior in this case was also +A, +1, +2. The test taker was able to access new information on the operation of the system through these steps. From the point of view of awareness, this +1+2 strategy falls under aware strategy usage, as the +1 and +2 sub-strategies were not applied far apart (excluding the simple repetition of the executed trials next to each other) from each other in time. A good indicator of aware strategy usage is if there is no difference between minimal and basic input behavior.
In the second example (Figure (Figure2), 2 ), we demonstrate the sequence of steps taken in mapping another problem as well as the coding we used. Here the students needed to solve a problem consisting of two input variables and one output variable. The word problem reads as follows: “Your mother has bought two new kinds of fruit drink mix. You want to make yourself a fruit drink with them. Figure out how the green and blue powders impact the sweetness of the drink. Plot your assumptions in the model.” The test taker attempted eight different trials in solving this problem, which were coded as follows: +1, +2, +0, +0, +0, +0, −0, −0. After step 2, the student had access to practically all the information required to plot the causal diagram. (In step 1, the problem solver checked the impact of one scoop of green powder and left the quantity of blue powder at zero. Once mixed, the resultant fruit drink became sweeter. In step 2, the problem solver likewise measured out one scoop of green powder for the drink but also added a scoop of blue powder. The sweetness of the drink changed as much as it had in step 1. After that, the student measured out various quantities of blue and then green powder, and looked at the impact.) The basic input behavior coded from the full input behavior used by the problem solver was +1+2, and the minimal input behavior was +1+1 because the purest VOTAT strategy was used in steps 1 and 6. (Thus, both variables separately confirmed the effects of the blue and the green powder on the sweetness of the drink.) From the point of view of awareness, this +1+1 strategy falls under non-aware strategy usage, as the two applications of the +1 trial occurred far apart from each other in time.

Exploration in phase 1 of the problems based on minimal complex systems (two input variables and one output variable).
Based on students' minimal input behavior we executed latent class analyses. We narrowed the focus to the principle of isolated variation, especially to the extent to which this special strategy was employed in the exploration phase as an indicator of students' ability to proficiently explore the problem environment. We added an extra variable to each of the problems, describing students' exploration behavior based on the following three categories: (1) no isolated variation at all (e.g., isolated variation was employed for none of the input variables – 0 points); (2) partially isolated variation (e.g., isolated variation was employed for some but not all the input variables – 1 point); and (3) fully isolated variation (e.g., isolated variation was employed for all the input variables – 2 points). Thus, depending on the level of optimal exploration strategy used, all the students received new categorical scores based on their input exploration behavior, one for each of the CPS tasks. Let us return to the example provided in Figures Figures1, 1 , ,2. 2 . In the first example, a partially isolated strategy was applied, since the problem solver only used this strategy to test the effect of the Miaow input variables (in trials 3 and 4). In the second example, a full isolated strategy was applied, as the problem solver used this isolated variation strategy for both the input variables during the exploration phase in the first and sixth trials.
The reliability of the test improved when scoring was based on the log data
The reliability of the MicroDYN problems as a measure of knowledge acquisition and knowledge application, the traditional CPS indicators for phases 1 and 2, were acceptable at α = 0.72–0.86 in all grades (Table (Table4). 4 ). After we re-scored the problem solvers' behavior at the beginning of the problem-solving process, coded the log data and assigned new variables for the effectiveness of strategy usage during the exploration phase of the task for each task and person, the overall reliability of the test scores improved. This phenomenon was noted in all grades and in both coding procedures, when the amount of information obtained was examined (Cronbach's α ranged from 0.86 to 0.96) and when the level of optimal exploration strategy used was analyzed (Cronbach's α ranged from 0.83 to 0.98; the answers to the warm-up tasks were excluded from these analyses).
Internal consistencies in scoring the MicroDYN problems: analyses based on both traditional CPS indicators and re-coded log data based on student behavior at the beginning of the problem-solving process.
Use of a theoretically effective strategy does not result in high performance (RQ1)
Use of a theoretically effective strategy did not always result in high performance. The percentage of effective strategy use and high CPS performance varied from 20 to 80%, depending on the complexity of the CPS tasks and the age group. The percentage of theoretically effective strategy use in each cohort increased by 20% for age when problems with the same complexity were compared (Table (Table5) 5 ) and decreased about 20% for the increasing number of input variables in the problems.
Percentage of theoretically effective and non-effective strategy use and high CPS performance.
The percentage of theoretically effective strategy use was the same for the less complex problems in Grades 3–5 and for the most complex tasks in Grades 8–12 (58%). More than 80% of these students solved the problem correctly in the first case, but only 60% had the correct solution in the second case. There was a 50% probability of effective and non-effective strategy use for problems with two input and two output variables in Grades 3–5 and for problems with three input and three output variables in Grades 6–7. In Grades 8–12, the use of a theoretically effective strategy was always higher than 50%, independently of the complexity of the problems (with no internal dynamic). The guessing factor, that is, the ad hoc optimization (use of a theoretically non-effective strategy with the correct solution) also changed, mostly based on the complexity and position of the tasks in the test. The results confirmed our hypothesis that the use of a theoretically effective strategy does not necessary represent the correct solution and that the correct solution does not always represent the use of an even theoretically effective problem-solving strategy.
Not all the VOTAT strategies result in high CPS performance (RQ2)
On average, only 15% of the theoretically effective strategy uses involved non-VOTAT strategies. The isolated variation strategy comprised 45% of the VOTAT strategies employed. It was the only theoretically effective strategy which always resulted in the correct solution to the problem with higher probability independently of problem complexity or the grade of the students. The real advantage of this strategy was most remarkable in the case of the third cohort, where an average of 80% of the students who employed this strategy solved the problems correctly (Figures (Figures3, 3 , ,4 4 ).

Efficacy of the most frequently employed VOTAT strategies on problems with two input variables and one or two output variables in Grades 3–5, 6–7, and 8–12.

Efficacy of the most frequently employed VOTAT strategies on problems with three input variables and one or two output variables in Grades 3–5, 6–7, and 8–12.
The second most frequently employed and successful VOTAT strategy was the +1+2 type or the +1+2+2 type, depending on the number of input variables. In the +1+2 type, only one single input variable was manipulated in the first step, while the other variable remained at a neutral value; in the second step, only the other input variable was changed and the first retained the setting used previously. This proved to be relatively successful on problems with a low level of complexity independently of age, but it generally resulted in a good solution with a low level of probability on more complex problems.
VOTAT strategies of the +1+3 type (in the case of two input variables) and of the +1+1+2 type (in the case of three input variables) were employed even less frequently and with a lower level of efficacy than all the other VOTAT strategies (+1+1+3, +1+2+1, +1+2+2, +1+2+3, +1+3+1, +1+3+2 and +1+3+3 in the case of three input variables) and theoretically effective, non-VOTAT strategies (e.g., +4 in the case of two input variables or +1+4, +4+2 and +4+3 in the case of three input variables). In the following, we provide an example of the +4+2 type, where the MicroDyn problem has three input variables (A, B, and C) and three output variables. In the first trial, the problem solver set the input variables to the following values: 0 (for variable A), 1 (for variable B), and 1 (for variable C); that is, he or she changed two input variables at the same time. In the second trial, he or she changed the value of two input variables at the same time again and applied the following setting: 0 (for variable A), −2 (for variable B), and −1 (for variable C). In the third trial, he set variable A to 1, and left variables B and C unchanged. That is, the problem solver's input behavior can be described with the following trials: –X +4 +2. Based on this strategy, it was possible to map the relationships between the input and output variables without using any VOTAT strategy in the exploration phase.
Aware explorers perform significantly higher on the CPS tasks (RQ3)
We compared the achievement of the aware, isolated strategy users with that of the non-aware explorers (Table (Table6). 6 ). The percentage of high achievers among the non-aware explorers seemed to be almost independent of age, but strongly influenced by the complexity of the problem and the learning effect we noted in the testing procedure (see RQ5). Results for problems with two input variables and one output variable confirmed our previous results, which showed that the probability of providing the correct solution is very high even without aware use of a theoretically effective strategy (60–70%). With more complex problems, the difference between the percentages of aware and non-aware explorers was huge. Generally, 85% of the non-aware explorers failed on the problems, while at least 80% of the aware, isolated strategy users were able to solve the problems correctly.
Percentage of high achievers among aware and non-aware explorers by grade and problem complexity.
Six qualitatively different explorer class profiles can be distinguished at the end of the elementary level and five at the end of the secondary level (RQ4 and RQ5)
In all three cohorts, each of the information theory criteria used (AIC, BIC, and aBIC) indicated a continuous decrease in an increasing number of latent classes. The likelihood ratio statistical test (Lo–Mendell Rubin Adjusted Likelihood Ratio Test) showed the best model fit in Grades 3–5 for the 4-class model, in Grades 6–7 for the 6-class model and in Grades 8–12 for the 5-class model. The entropy-based criterion reached the maximum values for the 2- and 3-class solutions, but it was also high for the best-fitting models based on the information theory and likelihood ratio criteria. Thus, the entropy index for the 4-class model showed that 80% of the 3 rd - to 5 th -graders, 82% of the 6 th - to 7 th -graders and 85% of the 8 th - to 12 th -graders were accurately categorized based on their class membership (Table (Table7 7 ).
Information theory, likelihood ratio and entropy-based fit indices for latent class analyses.
AIC, Akaike Information Criterion; BIC, Bayesian Information Criterion; aBIC, adjusted Bayesian Information Criterion; L–M–R test, Lo–Mendell–Rubin Adjusted Likelihood Ratio Test. The best fitting model solution is in italics .
We distinguished four latent classes in the lower grades based on the exploration strategy employed and the level of isolated variation strategy used (Table (Table8): 8 ): 40.5% of the students proved to be non-performing explorers on the basis of their strategic patterns in the CPS environments. They did not use any isolated or partially isolated variation at all; 23.6% of the students were among the low-performing explorers who only rarely employed a fully or partially isolated variation strategy (with 0–20% probability on the less complex problems and 0–5% probability on the more complex problems). 24.7% of the 3 rd - to 5 th -graders were categorized as slow learners who were intermediate performers with regard to the efficiency of the exploration strategy they used on the easiest problems with a slow learning effect, but low-performing explorers on the complex ones. In addition, 11.1% of the students proved to be proficient explorers, who used the isolated or partially isolated variation strategy with 80–100% probability on all the proposed CPS problems.
Relative frequencies and average latent class probabilities across grade levels 3–5, 6–7, and 8–12.
In Grades 6–7, in which achievement proved to be significantly higher on average, 10% fewer students were observed in each of the first two classes (non-performing explorers and low-performing explorers). The percentage of intermediate explorers remained almost the same (26%), and we noted two more classes with the analyses: the class of rapid learners (4.4%) and that of slow learners, who are almost proficient explorers on the easiest problems, employing the fully or partially isolated variation strategy with 60–80% probability, but low-performing explorers on the complex ones (10.3%). The frequency of proficient strategy users was also increased (to 14.2%) compared to students in the lower grades. Finally, there was almost no change detected in the low-performing explorers' classes in Grades 8–12. We did not detect anyone in the class of intermediate explorers; they must have developed further and become (1) rapid learners (7.7%), (2) slow learners with almost high achievement with regard to the exploration strategy they used on the easiest problems, but low achievers on the complex ones (17.6%), or (3) proficient strategy users (26.3%), whose achievement was high both on the simplest and the most complex problems.
Based on these results, the percentage of non- and low explorers, who have very low exploration skills and do not learn during testing, decreased from almost 65 to 50% between the lower and higher primary school levels and then remained constant at the secondary level. There was a slight increase in respect of the percentage of students among the rapid learners. The students in that group used the fully or partially isolated strategy at very low levels at the beginning of the test, but they learned very quickly and detected these effective exploration strategies; thus, by the end of the test, their proficiency level with regard to exploration was equal to the top performers' achievement. However, we were unable to detect the class of rapid learners among 3 rd - to 5 th -graders.
Generally, students' level of exploration expertise with regard to fully and partially isolated variation improved significantly with age ( F = 70.376, p < 0.001). According to our expectations based on the achievement differences among students in Grades 3–5, 6–8 and 9–12, there were also significant differences in the level of expertise in fully or partially isolated strategy use during problem exploration between 3 rd - to 5 th - and 6 th - to 7 th -grade students ( t = −6.833, p < 0.001, d = 0.03) and between 6 th - to 7 th - and 8 th - to 12 th -grade students ( t = −6.993, p < 0.001, d = 0.03).
In this study, we examined 3 rd - to 12 th -grade (aged 9–18) students' problem-solving behavior by means of a logfile analysis to identify qualitatively different exploration strategies. Students' activity in the first phase of the problem-solving process was coded according to a mathematical model that was developed based on strategy effectiveness and then clustered for comparison. Reliability analyses of students' strategy use indicated that strategies used in the knowledge acquisition phase described students' development (ability level) better than traditional quantitative psychometric indicators, including the goodness of the model. The high reliability indices indicate that there are untapped possibilities in analyzing log data. Our analyses of logfiles extracted from a simulation-based assessment of problem solving have expanded the scope of previous studies and made it possible to identify a central component of children's scientific reasoning: the way students understand how scientific experiments can be designed and how causal relationships can be explored by systematically changing the values of (independent) variables and observing their impact on other (target) variables.
In this way, we have introduced a new labeling and scoring method that can be employed in addition to the two scores that have already been used in previous studies. We have found that using this scoring method (based on student strategy use) improves the reliability of the test. Further studies are needed to examine the validity of the scale based on this method and to determine what this scale really measures. We may assume that the general idea of varying the values of the independent variables and connecting them to the resultant changes in the target variable is the essence of scientific reasoning and that the systematic manipulation of variables is related to combinatorial reasoning, while summarizing one's observations and plotting a model is linked to rule induction. Such further studies have to place CPS testing in the context of other cognitive tests and may contribute to efforts to determine the place of CPS in a system of cognitive abilities (see e.g., Wüstenberg et al., 2012 ).
We have found that the use of a theoretically effective strategy does not always result in high performance. This is not surprising, and it confirms research results by de Jong and van Joolingen ( 1998 ), who argue that learners often have trouble interpreting data. As we observed earlier, using a systematic strategy requires combinatorial thinking, while drawing a conclusion from one's observations requires rule induction (inductive reasoning). Students showing systematic strategies but failing to solve the problem may possess combinatorial skills but lack the necessary level of inductive reasoning. It is more difficult to find an explanation for the other direction of discrepancy, when students actually solve the problem without an effective (complete) strategy. Thus, solving the problem does not require the use of a strategy which provides the problem solver with sufficient information about the problem environment to be able to form the correct solution. This finding is similar to results from previous research (e.g., Vollmeyer et al., 1996 ; Greiff et al., 2015 ). Goode and Beckmann ( 2010 ) reported two qualitatively different, but equally effective approaches: knowledge- based and ad hoc control.
In the present study, the contents of the problems were not based on real knowledge, and the causal relationships between the variables were artificial. Content knowledge was therefore no help to the students in filling the gap between the insufficient information acquired from interaction and the successful solution to the problem. We may assume that students guessed intuitively in such a case. Further studies may ascertain how students guess in such situations.
The percentage of success is influenced by the complexity of the CPS tasks, the type of theoretically effective strategy used, the age group and, finally, the degree to which the strategy was consciously employed.
The most frequently employed effective strategies fell within the class of VOTAT strategies. Almost half the VOTAT strategies were of the isolated variation strategy type, which resulted with higher probability in the correct solution independently of the complexity of the problem or the grade of the students. As noted earlier, not all the VOTAT strategies resulted in high CPS performance; moreover, all the other VOTAT strategies proved to be significantly less successful. Some of them worked with relative success on problems with a low level of complexity, but failed with a high level of probability on more complex problems independently of age group. Generally, the advantage of the isolated variation strategy (Wüstenberg et al., 2014 ) compared to the other VOTAT and non-VOTAT, theoretically effective strategies is clearly evident from the outcome. The use of the isolated variation strategy, where students examined the effect of the input variables on the output variables independently, resulted in a good solution with the highest probability and proved to be the most effective VOTAT strategy independently of student age or problem complexity.
Besides the type of strategy used, awareness also played an influential role. Aware VOTAT strategy users proved to be the most successful explorers. They were followed in effectiveness by non-aware VOTAT strategy users and theoretically effective, but non-VOTAT strategy users. They managed to represent the information that they had obtained from the system more effectively and made good decisions in the problem-solving process compared to their peers.
We noted both qualitative and quantitative changes of problem-solving behavior in the age range under examination. Using latent class analyses, we identified six qualitatively different class profiles during compulsory schooling. (1) Non-performing and (2) low-performing students who usually employed no fully or partially isolated variation strategy at all or, if so, then rarely. They basically demonstrated unsystematic exploration behavior. (3) Proficient strategy users who consistently employed optimal exploration strategies from the very first problem as well as the isolated variation strategy and the partially isolated variation, but only seldom. They must have more elaborated schemas available. (4) Slow learners who are intermediate performers on the easiest problems, but low performers on the complex ones or (5) high performers on the easiest problems, but low performers on the complex ones. Most members of this group managed to employ the principle of isolated or partially isolated variation and had an understanding of it, but they were only able to use it on the easiest task and then showed a rapid decline on the more complex CPS problems. They might have been cognitively overloaded by the increasingly difficult problem-solving environments they faced. (6) Rapid learners, a very small group from an educational point of view. These students started out as non-performers in their exploration behavior on the first CPS tasks, showed a rapid learning curve afterwards and began to use the partially isolated variation strategy increasingly and then the fully isolated variation strategy. By the end of the test, they reached the same high level of exploration behavior as the proficient explorers. We observed no so-called intermediate strategy users, i.e., those who used the partially isolated variation strategy almost exclusively on the test. As we expected, class membership increased significantly in the more proficient classes at the higher grade levels due to the effects of cognitive maturation and schooling, but this did not change noticeably in the two lowest-level classes.
Limitations of the study include the low sample size for secondary school students; further, repetition is required for validation. The generalizability of the results is also limited by the effects of semantic embedding (i.e., cover stories and variable labels), that is, the usage of different fictitious cover stories “with the intention of minimizing the uncontrollable effects of prior knowledge, beliefs or suppositions” (Beckmann and Goode, 2017 ). An assumption triggered by semantic contexts has an impact on exploration behavior (e.g., the range of interventions, or strategies employed by the problem solver; Beckmann and Goode, 2014 ), that is, how the problem solver interacts with the system. Limitations also include the characteristics of the interface used. In our view, analyses with regard to VOTAT strategies are only meaningful in systems with an interface where inputs do not automatically reset to zero from one input to the next (Beckmann and Goode, 2017 ). That is, we excluded problem environments from the study where the inputs automatically reset to zero from one input to the next. A further limitation of the generalizability of the results is that we have omitted problems with autonomic changes from the analyses.
The main reason why we have excluded systems that contain autoregressive dependencies from the analyses is that different strategy usage is required on problems which also involve the use of trial +A (according to our coding of sub-strategies), which is not among the effective sub-strategies for problems without autonomic changes. Analyses of students' behavior on problems with autonomic changes will form part of further studies, as well as a refinement of the definition of what makes a problem complex and difficult. We plan to adapt the Person, Task and Situation framework published by Beckmann and Goode ( 2017 ). The role of ad hoc control behavior was excluded from the analyses; further studies are required to ascertain the importance of the repetitive control behavior. Another limitation of the study could be the interpretation of the differences across age group clusters as indicators of development and not as a lack of stability of the model employed.
These results shed new light on and provide a new interpretation of previous analyses of complex problem solving in the MicroDYN approach. They also highlight the importance of explicit enhancement of problem-solving skills and problem-solving strategies as a tool for applying knowledge in a new context during school lessons.
Ethics statement
Ethical approval was not required for this study based on national and institutional guidelines. The assessments which provided data for this study were integrated parts of the educational processes of the participating schools. The coding system for the online platform masked students' identity; the data cannot be connected to the students. The results from the no-stakes diagnostic assessments were disclosed only to the participating students (as immediate feedback) and to their teachers. Because of the anonymity and no-stakes testing design of the assessment process, it was not required or possible to request and obtain written informed parental consent from the participants.
Author contributions
Both the authors, GM and BC, certify that they have participated sufficiently in the work to take responsibility for the content, including participation in the concept, design and analysis as well as the writing and final approval of the manuscript. Each author agrees to be accountable for all aspects of the work.
Conflict of interest statement
The authors declare that the research was conducted in the absence of any commercial or financial relationships that could be construed as a potential conflict of interest.
1 With regard to terminology, please note that different terms are used for the subject at hand (e.g., complex problem solving, dynamic problem solving, interactive problem solving and creative problem solving). In this paper, we use the modifier “complex” (see Csapó and Funke, 2017 ; Dörner and Funke, 2017 ).
Funding. This study was funded by OTKA K115497.
- OECD (2014). PISA 2012 Results: Creative Problem Solving. Students' Skills in Tackling Real-Life Problems (Volume V) . Paris: OECD. [ Google Scholar ]
- Beckmann J. F., Goode N. (2014). The benefit of being näive and knowing it? The unfavourable impact of perceived context familiarity on learning in complex problem solving tasks . Instructional Sci . 42 , 271–290. 10.1007/s11251-013-9280-7 [ CrossRef ] [ Google Scholar ]
- Beckmann J. F., Birney D. P., Goode N. (2017). Beyond psychometrics: the difference between difficult problem solving and complex problem solving . Front. Psychol . 8 :1739. 10.3389/fpsyg.2017.01739 [ PMC free article ] [ PubMed ] [ CrossRef ] [ Google Scholar ]
- Beckmann J. F., Goode N. (2017). Missing the wood for the wrong trees: On the difficulty of defining the complexity of complex problem solving scenarios . J. Intell . 5 :2 10.3390/jintelligence5020015 [ PMC free article ] [ PubMed ] [ CrossRef ] [ Google Scholar ]
- Chen Z., Klahr D. (1999). All other things being equal: acquisition and transfer of the control of variables strategy . Child Dev. 70 , 1098–1120. 10.1111/1467-8624.00081 [ PubMed ] [ CrossRef ] [ Google Scholar ]
- Collins L. M., Lanza S. T. (2010). Latent Class and Latent Transition Analysis: With Applications in the Social, Behavioral, and Health Sciences . New York, NY: Wiley. [ Google Scholar ]
- Csapó B., Ainley J., Bennett R., Latour T., Law N. (2012). Technological issues of computer-based assessment of 21st century skills , in Assessment and Teaching of 21st Century Skills , eds Griffin P., McGaw B., Care E. (New York, NY: Springer; ), 143–230. [ Google Scholar ]
- Csapó B., Funke J. (eds.). (2017). The Nature of Problem Solving. Using Research to Inspire 21st Century Learning. Paris: OECD. [ Google Scholar ]
- Csapó B., Molnár G. (2017). Potential for assessing dynamic problem-solving at the beginning of higher education studies . Front. Psychol. 8 :2022. 10.3389/fpsyg.2017.02022 [ PMC free article ] [ PubMed ] [ CrossRef ] [ Google Scholar ]
- Csapó B., Molnár G., Nagy J. (2014). Computer-based assessment of school-readiness and reasoning skills . J. Educ. Psychol. 106 , 639–650. 10.1037/a0035756 [ CrossRef ] [ Google Scholar ]
- de Jong T., van Joolingen W. R. (1998). Scientific discovery learning with computer simulations of conceptual domains . Rev. Educ. Res . 68 , 179–201. 10.3102/00346543068002179 [ CrossRef ] [ Google Scholar ]
- Demetriou A., Efklides A., Platsidou M. (1993). The architecture and dynamics of developing mind: experiential structuralism as a frame for unifying cognitive developmental theories . Monogr. Soc. Res. Child Dev. 58 , 1–205. 10.2307/1166053 [ PubMed ] [ CrossRef ] [ Google Scholar ]
- Dörner D., Funke J. (2017). Complex problem solving: what it is and what it is not . Front. Psychol. 8 :1153 10.3389/fpsyg.2017.01153 [ PMC free article ] [ PubMed ] [ CrossRef ] [ Google Scholar ]
- Dziak J. J., Coffman D. L., Lanza S. T., Li R. (2012). Sensitivity and Specificity of Information Criteria . The Pennsylvania State University: The Methodology Center and Department of Statistics. Available online at: https://methodology.psu.edu/media/techreports/12-119.pdf
- Fischer A., Greiff S., Funke J. (2012). The process of solving complex problems . J. Probl. Solving . 4 , 19–42. 10.7771/1932-6246.1118 [ CrossRef ] [ Google Scholar ]
- Funke J. (2001). Dynamic systems as tools for analysing human judgement . Think. Reason. 7 , 69–89. 10.1080/13546780042000046 [ CrossRef ] [ Google Scholar ]
- Funke J. (2010). Complex problem solving: a case for complex cognition? Cogn. Process. 11 , 133–142. 10.1007/s10339-009-0345-0 [ PubMed ] [ CrossRef ] [ Google Scholar ]
- Funke J. (2014). Analysis of minimal complex systems and complex problem solving require different forms of causal cognition . Front. Psychol. 5 :739. 10.3389/fpsyg.2014.00739 [ PMC free article ] [ PubMed ] [ CrossRef ] [ Google Scholar ]
- Galotti K. M. (2011). Cognitive Development . Thousand Oaks, CA: SAGE. [ Google Scholar ]
- Gardner P. H., Berry D. C. (1995). The effect of different forms of advice on the control of a simulated complex system . Appl. Cogn. Psychol. 9 :7 10.1002/acp.2350090706 [ CrossRef ] [ Google Scholar ]
- Goode N., Beckmann J. F. (2010). You need to know: there is a causal relationship between structural knowledge and control performance in complex problem solving tasks . Intelligence 38 , 345–352. 10.1016/j.intell.2010.01.001 [ CrossRef ] [ Google Scholar ]
- Greiff S., Funke J. (2010). Systematische Erforschung komplexer Problemlösefähigkeit anhand minimal komplexer Systeme . Zeitschrift für Pädagogik 56 , 216–227. [ Google Scholar ]
- Greiff S., Funke J. (2017). Interactive problem solving: exploring the potential of minimal complex systems , in The Nature of Problem Solving. Using Research to Inspire 21st Century Learning , eds Csapó B., Funke J. (Paris: OECD; ), 93–105. [ Google Scholar ]
- Greiff S., Wüstenberg S., Avvisati F. (2015). Computer-generated log-file analyses as a window into students' minds? A showcase study based on the PISA 2012 assessment of problem solving . Computers Educ . 91 , 92–105. 10.1016/j.compedu.2015.10.018 [ CrossRef ] [ Google Scholar ]
- Greiff S., Wüstenberg S., Csapó B., Demetriou A., Hautamäki H., Graesser A. C., et al. (2014). Domain-general problem solving skills and education in the 21st century . Educ. Res. Rev . 13 , 74–83. 10.1016/j.edurev.2014.10.002 [ CrossRef ] [ Google Scholar ]
- Greiff S., Wüstenberg S., Funke J. (2012). Dynamic problem solving: a new assessment perspective . Appl. Psychol. Meas. 36 , 189–213. 10.1177/0146621612439620 [ CrossRef ] [ Google Scholar ]
- Greiff S., Wüstenberg S., Goetz T., Vainikainen M.-P., Hautamäki J., Bornstein M. H. (2015). A longitudinal study of higher-order thinking skills: working memory and fluid reasoning in childhood enhance complex problem solving in adolescence . Front. Psychol. 6 :1060. 10.3389/fpsyg.2015.01060 [ PMC free article ] [ PubMed ] [ CrossRef ] [ Google Scholar ]
- Greiff S., Wüstenberg S., Molnár G., Fischer A., Funke J., Csapó B. (2013). Complex problem solving in educational contexts – Something beyond g: Concept, assessment, measurement invariance, and construct validity . J. Educ. Psychol . 105 , 364–379. 10.1037/a0031856 [ CrossRef ] [ Google Scholar ]
- Griffin P., McGaw B., Care E. (2012). Assessment and Teaching of 21st Century Skills . Dordrecht: Springer. [ Google Scholar ]
- Inhelder B., Piaget J. (1958). The Growth of Logical Thinking from Childhood to Adolescence . New York, NY: Basic Books. [ Google Scholar ]
- Klahr D., Dunbar K. (1988). Dual space search during scientific reasoning . Cogn. Sci. 12 , 1–48. 10.1207/s15516709cog1201_1 [ CrossRef ] [ Google Scholar ]
- Klahr D., Triona L. M., Williams C. (2007). Hands on what? The relative effectiveness of physical versus virtual materials in an engineering design project by middle school children . J. Res. Sci. Teaching 44 , 183–203. 10.1002/tea.20152 [ CrossRef ] [ Google Scholar ]
- Kröner S., Plass J. L., Leutner D. (2005). Intelligence assessment with computer simulations . Intelligence 33 , 347–368. 10.1016/j.intell.2005.03.002 [ CrossRef ] [ Google Scholar ]
- Kuhn D., Iordanou K., Pease M., Wirkala C. (2008). Beyond control of variables: what needs to develop to achieve skilled scientific thinking? Cogn. Dev . 23 , 435–451. 10.1016/j.cogdev.2008.09.006 [ CrossRef ] [ Google Scholar ]
- Lo Y., Mendell N. R., Rubin D. B. (2001). Testing the number of components in a normal mixture . Biometrika 88 , 767–778. 10.1093/biomet/88.3.767 [ CrossRef ] [ Google Scholar ]
- Lotz C., Scherer R., Greiff S., Sparfeldt J. R. (2017). Intelligence in action – Effective strategic behaviors while solving complex problems . Intelligence 64 , 98–112. 10.1016/j.intell.2017.08.002 [ CrossRef ] [ Google Scholar ]
- Mayer R. E., Wittrock M. C. (1996). Problem-solving transfer in Handbook of Educational Psychology , eds Berliner D. C., Calfee R. C. (New York, NY; London: Routledge; ), 47–62. [ Google Scholar ]
- Molnár G., Greiff S., Csapó B. (2013). Inductive reasoning, domain specific and complex problem solving: relations and development . Think. Skills Creat. 9 , 35–45. 10.1016/j.tsc.2013.03.002 [ CrossRef ] [ Google Scholar ]
- Molnár G., Greiff S., Wüstenberg S., Fischer A. (2017). Empirical study of computer-based assessment of domain-general complex problem-solving skills , in The Nature of Problem Solving. Using Research to Inspire 21st Century Learning , eds Csapó B., Funke J. (Paris: OECD; ), 125–140. [ Google Scholar ]
- Müller J. C., Kretzschmar A., Greiff S. (2013). Exploring exploration: Inquiries into exploration behavior in complex problem solving assessment , in Proceedings of the 6th International Conference on Educational Data Mining , eds D'Mello S. K., Calvo R. A., Olney A. 336–337. Available online at: http://citeseerx.ist.psu.edu/viewdoc/download?doi=10.1.1.666.6664&rep=rep1&type=pdf [ Google Scholar ]
- Muthén L. K., Muthén B. O. (2012). Mplus User's Guide, 7th Edn . Los Angeles, CA: Muthén and Muthén. [ Google Scholar ]
- Osman M., Speekenbrink M. (2011). Cue utilization and strategy application in stable and unstable dynamic environments . Cogn. Syst. Res . 12 , 355–364. 10.1016/j.cogsys.2010.12.004 [ CrossRef ] [ Google Scholar ]
- Schoppek W., Fischer A. (2017). Common process demands of two complex dynamic control tasks: transfer is mediated by comprehensive strategies . Front. Psychol . 8 :2145. 10.3389/fpsyg.2017.02145 [ PMC free article ] [ PubMed ] [ CrossRef ] [ Google Scholar ]
- Siegler R. S. (1999). Strategic development . Trends Cogn. Sci. 3 , 430–435. [ PubMed ] [ Google Scholar ]
- Sonnleiter P., Keller U., Martin R., Latour T., Brunner M. (2017). Assessing complex problem solving in the classroom: meeting challenges and opportunities , in The Nature of Problem Solving. Using Research to Inspire 21st Century Learning , eds Csapó B., Funke J. (Paris: OECD; ), 159–174. [ Google Scholar ]
- Sweller J. (1994). Cognitive load theory, learning difficulty, and instructional design . Learn. Instruct . 4 , 295–312. 10.1016/0959-4752(94)90003-5 [ CrossRef ] [ Google Scholar ]
- Tein J. Y., Coxe S., Cham H. (2013). Statistical power to detect the correct number of classes in latent profile analysis . Struct. Equ. Modeling. 20 , 640–657. 10.1080/10705511.2013.824781 [ PMC free article ] [ PubMed ] [ CrossRef ] [ Google Scholar ]
- Tóth K., Rölke H., Goldhammer F., Barkow I. (2017). Educational process mining: New possibilities for understanding students' problem-solving skills , in The Nature of Problem Solving. Using Research to Inspire 21st Century Learning , eds. Csapó B., Funke J. (Paris: OECD; ), 193–210. [ Google Scholar ]
- Tschirgi J. E. (1980). Sensible reasoning: a hypothesis about hypotheses . Child Dev. 51 , 1–10. 10.2307/1129583 [ CrossRef ] [ Google Scholar ]
- Vollmeyer R., Burns B. D., Holyoak K. J. (1996). The impact of goal specificity on strategy use and the acquisition of problem structure . Cogn. Sci. 20 , 75–100. 10.1207/s15516709cog2001_3 [ CrossRef ] [ Google Scholar ]
- Wüstenberg S., Greiff S., Funke J. (2012). Complex problem solving. More than reasoning? Intelligence 40 , 1–14. 10.1016/j.intell.2011.11.003 [ CrossRef ] [ Google Scholar ]
- Wüstenberg S., Stadler M., Hautamäki J., Greiff S. (2014). The role of strategy knowledge for the application of strategies in complex problem solving tasks . Technol. Knowledge Learn. 19 , 127–146. 10.1007/s10758-014-9222-8 [ CrossRef ] [ Google Scholar ]
- Zoanetti N., Griffin P. (2017). Log-file data as indicators for problem-solving processes , in The Nature of Problem Solving. Using Research to Inspire 21st Century Learning , eds Csapó B., Funke J. (Paris: OECD; ), 177–191. [ Google Scholar ]

- SUGGESTED TOPICS
- The Magazine
- Newsletters
- Managing Yourself
- Managing Teams
- Work-life Balance
- The Big Idea
- Data & Visuals
- Reading Lists
- Case Selections
- HBR Learning
- Topic Feeds
- Account Settings
- Email Preferences
Share Podcast

A Better Framework for Solving Tough Problems
Start with trust and end with speed.
- Apple Podcasts
When it comes to solving complicated problems, the default for many organizational leaders is to take their time to work through the issues at hand. Unfortunately, that often leads to patchwork solutions or problems not truly getting resolved.
But Anne Morriss offers a different framework. In this episode, she outlines a five-step process for solving any problem and explains why starting with trust and ending with speed is so important for effective change leadership. As she says, “Let’s get into dialogue with the people who are also impacted by the problem before we start running down the path of solving it.”
Morriss is an entrepreneur and leadership coach. She’s also the coauthor of the book, Move Fast and Fix Things: The Trusted Leader’s Guide to Solving Hard Problems .
Key episode topics include: strategy, decision making and problem solving, strategy execution, managing people, collaboration and teams, trustworthiness, organizational culture, change leadership, problem solving, leadership.
HBR On Strategy curates the best case studies and conversations with the world’s top business and management experts, to help you unlock new ways of doing business. New episodes every week.
- Listen to the full HBR IdeaCast episode: How to Solve Tough Problems Better and Faster (2023)
- Find more episodes of HBR IdeaCast
- Discover 100 years of Harvard Business Review articles, case studies, podcasts, and more at HBR.org .
HANNAH BATES: Welcome to HBR On Strategy , case studies and conversations with the world’s top business and management experts, hand-selected to help you unlock new ways of doing business.
When it comes to solving complicated problems, many leaders only focus on the most apparent issues. Unfortunately that often leads to patchwork or partial solutions. But Anne Morriss offers a different framework that aims to truly tackle big problems by first leaning into trust and then focusing on speed.
Morriss is an entrepreneur and leadership coach. She’s also the co-author of the book, Move Fast and Fix Things: The Trusted Leader’s Guide to Solving Hard Problems . In this episode, she outlines a five-step process for solving any problem. Some, she says, can be solved in a week, while others take much longer. She also explains why starting with trust and ending with speed is so important for effective change leadership.
This episode originally aired on HBR IdeaCast in October 2023. Here it is.
CURT NICKISCH: Welcome to the HBR IdeaCast from Harvard Business Review. I’m Curt Nickisch.
Problems can be intimidating. Sure, some problems are fun to dig into. You roll up your sleeves, you just take care of them; but others, well, they’re complicated. Sometimes it’s hard to wrap your brain around a problem, much less fix it.
And that’s especially true for leaders in organizations where problems are often layered and complex. They sometimes demand technical, financial, or interpersonal knowledge to fix. And whether it’s avoidance on the leaders’ part or just the perception that a problem is systemic or even intractable, problems find a way to endure, to keep going, to keep being a problem that everyone tries to work around or just puts up with.
But today’s guest says that just compounds it and makes the problem harder to fix. Instead, she says, speed and momentum are key to overcoming a problem.
Anne Morriss is an entrepreneur, leadership coach and founder of the Leadership Consortium and with Harvard Business School Professor Francis Frei, she wrote the new book, Move Fast and Fix Things: The Trusted Leaders Guide to Solving Hard Problems . Anne, welcome back to the show.
ANNE MORRISS: Curt, thank you so much for having me.
CURT NICKISCH: So, to generate momentum at an organization, you say that you really need speed and trust. We’ll get into those essential ingredients some more, but why are those two essential?
ANNE MORRISS: Yeah. Well, the essential pattern that we observed was that the most effective change leaders out there were building trust and speed, and it didn’t seem to be a well-known observation. We all know the phrase, “Move fast and break things,” but the people who were really getting it right were moving fast and fixing things, and that was really our jumping off point. So when we dug into the pattern, what we observed was they were building trust first and then speed. This foundation of trust was what allowed them to fix more things and break fewer.
CURT NICKISCH: Trust sounds like a slow thing, right? If you talk about building trust, that is something that takes interactions, it takes communication, it takes experiences. Does that run counter to the speed idea?
ANNE MORRISS: Yeah. Well, this issue of trust is something we’ve been looking at for over a decade. One of the headlines in our research is it’s actually something we’re building and rebuilding and breaking all the time. And so instead of being this precious, almost farbege egg, it’s this thing that is constantly in motion and this thing that we can really impact when we’re deliberate about our choices and have some self-awareness around where it’s breaking down and how it’s breaking down.
CURT NICKISCH: You said break trust in there, which is intriguing, right? That you may have to break trust to build trust. Can you explain that a little?
ANNE MORRISS: Yeah, well, I’ll clarify. It’s not that you have to break it in order to build it. It’s just that we all do it some of the time. Most of us are trusted most of the time. Most of your listeners I imagine are trusted most of the time, but all of us have a pattern where we break trust or where we don’t build as much as could be possible.
CURT NICKISCH: I want to talk about speed, this other essential ingredient that’s so intriguing, right? Because you think about solving hard problems as something that just takes a lot of time and thinking and coordination and planning and designing. Explain what you mean by it? And also, just how we maybe approach problems wrong by taking them on too slowly?
ANNE MORRISS: Well, Curt, no one has ever said to us, “I wish I had taken longer and done less.” We hear the opposite all the time, by the way. So what we really set out to do was to create a playbook that anyone can use to take less time to do more of the things that are going to make your teams and organizations stronger.
And the way we set up the book is okay, it’s really a five step process. Speed is the last step. It’s the payoff for the hard work you’re going to do to figure out your problem, build or rebuild trust, expand the team in thoughtful and strategic ways, and then tell a real and compelling story about the change you’re leading.
Only then do you get to go fast, but that’s an essential part of the process, and we find that either people under emphasize it or speed has gotten a bad name in this world of moving fast and breaking things. And part of our mission for sure was to rehabilitate speed’s reputation because it is an essential part of the change leader’s equation. It can be the difference between good intentions and getting anything done at all.
CURT NICKISCH: You know, the fact that nobody ever tells you, “I wish we had done less and taken more time.” I think we all feel that, right? Sometimes we do something and then realize, “Oh, that wasn’t that hard and why did it take me so long to do it? And I wish I’d done this a long time ago.” Is it ever possible to solve a problem too quickly?
ANNE MORRISS: Absolutely. And we see that all the time too. What we push people to do in those scenarios is really take a look at the underlying issue because in most cases, the solution is not to take your foot off the accelerator per se and slow down. The solution is to get into the underlying problem. So if it’s burnout or a strategic disconnect between what you’re building and the marketplace you’re serving, what we find is the anxiety that people attach to speed or the frustration people attach to speed is often misplaced.
CURT NICKISCH: What is a good timeline to think about solving a problem then? Because if we by default take too long or else jump ahead and we don’t fix it right, what’s a good target time to have in your mind for how long solving a problem should take?
ANNE MORRISS: Yeah. Well, we’re playful in the book and talking about the idea that many problems can be solved in a week. We set the book up five chapters. They’re titled Monday, Tuesday, Wednesday, Thursday, Friday, and we’re definitely having fun with that. And yet, if you count the hours in a week, there are a lot of them. Many of our problems, if you were to spend a focused 40 hours of effort on a problem, you’re going to get pretty far.
But our main message is, listen, of course it’s going to depend on the nature of the problem, and you’re going to take weeks and maybe even some cases months to get to the other side. What we don’t want you to do is take years, which tends to be our default timeline for solving hard problems.
CURT NICKISCH: So you say to start with identifying the problem that’s holding you back, seems kind of obvious. But where do companies go right and wrong with this first step of just identifying the problem that’s holding you back?
ANNE MORRISS: And our goal is that all of these are going to feel obvious in retrospect. The problem is we skip over a lot of these steps and this is why we wanted to underline them. So this one is really rooted in our observation and I think the pattern of our species that we tend to be overconfident in the quality of our thoughts, particularly when it comes to diagnosing problems.
And so we want to invite you to start in a very humble and curious place, which tends not to be our default mode when we’re showing up for work. We convince ourselves that we’re being paid for our judgment. That’s exactly what gets reinforced everywhere. And so we tend to counterintuitively, given what we just talked about, we tend to move too quickly through the diagnostic phase.
CURT NICKISCH: “I know what to do, that’s why you hired me.”
ANNE MORRISS: Exactly. “I know what to do. That’s why you hired me. I’ve seen this before. I have a plan. Follow me.” We get rewarded for the expression of confidence and clarity. And so what we’re inviting people to do here is actually pause and really lean into what are the root causes of the problem you’re seeing? What are some alternative explanations? Let’s get into dialogue with the people who are also impacted by the problem before we start running down the path of solving it.
CURT NICKISCH: So what do you recommend for this step, for getting to the root of the problem? What are questions you should ask? What’s the right thought process? What do you do on Monday of the week?
ANNE MORRISS: In our experience of doing this work, people tend to undervalue the power of conversation, particularly with other people in the organization. So we will often advocate putting together a team of problem solvers, make it a temporary team, really pull in people who have a particular perspective on the problem and create the space, make it as psychologically safe as you can for people to really, as Chris Argyris so beautifully articulated, discuss the undiscussable.
And so the conditions for that are going to look different in every organization depending on the problem, but if you can get a space where smart people who have direct experience of a problem are in a room and talking honestly with each other, you can make an extraordinary amount of progress, certainly in a day.
CURT NICKISCH: Yeah, that gets back to the trust piece.
ANNE MORRISS: Definitely.
CURT NICKISCH: How do you like to start that meeting, or how do you like to talk about it? I’m just curious what somebody on that team might hear in that meeting, just to get the sense that it’s psychologically safe, you can discuss the undiscussable and you’re also focusing on the identification part. What’s key to communicate there?
ANNE MORRISS: Yeah. Well, we sometimes encourage people to do a little bit of data gathering before those conversations. So the power of a quick anonymous survey around whatever problem you’re solving, but also be really thoughtful about the questions you’re going to ask in the moment. So a little bit of preparation can go a long way and a little bit of thoughtfulness about the power dynamic. So who’s going to walk in there with license to speak and who’s going to hold back? So being thoughtful about the agenda, about the questions you’re asking about the room, about the facilitation, and then courage is a very infectious emotion.
So if you can early on create the conditions for people to show up bravely in that conversation, then the chance that you’re going to get good information and that you’re going to walk out of that room with new insight in the problem that you didn’t have when you walked in is extraordinarily high.
CURT NICKISCH: Now, in those discussions, you may have people who have different perspectives on what the problem really is. They also bear different costs of addressing the problem or solving it. You talked about the power dynamic, but there’s also an unfairness dynamic of who’s going to actually have to do the work to take care of it, and I wonder how you create a culture in that meeting where it’s the most productive?
ANNE MORRISS: For sure, the burden of work is not going to be equitably distributed around the room. But I would say, Curt, the dynamic that we see most often is that people are deeply relieved that hard problems are being addressed. So it really can create, and more often than not in our experience, it does create this beautiful flywheel of action, creativity, optimism. Often when problems haven’t been addressed, there is a fair amount of anxiety in the organization, frustration, stagnation. And so credible movement towards action and progress is often the best antidote. So even if the plan isn’t super clear yet, if it’s credible, given who’s in the room and their decision rights and mandate, if there’s real momentum coming out of that to make progress, then that tends to be deeply energizing to people.
CURT NICKISCH: I wonder if there’s an organization that you’ve worked with that you could talk about how this rolled out and how this took shape?
ANNE MORRISS: When we started working with Uber, that was wrestling with some very public issues of culture and trust with a range of stakeholders internally, the organization, also external, that work really started with a campaign of listening and really trying to understand where trust was breaking down from the perspective of these stakeholders?
So whether it was female employees or regulators or riders who had safety concerns getting into the car with a stranger. This work, it starts with an honest internal dialogue, but often the problem has threads that go external. And so bringing that same commitment to curiosity and humility and dialogue to anyone who’s impacted by the problem is the fastest way to surface what’s really going on.
CURT NICKISCH: There’s a step in this process that you lay out and that’s communicating powerfully as a leader. So we’ve heard about listening and trust building, but now you’re talking about powerful communication. How do you do this and why is it maybe this step in the process rather than the first thing you do or the last thing you do?
ANNE MORRISS: So in our process, again, it’s the days of the week. On Monday you figured out the problem. Tuesday you really got into the sandbox in figuring out what a good enough plan is for building trust. Wednesday, step three, you made it better. You created an even better plan, bringing in new perspectives. Thursday, this fourth step is the day we’re saying you got to go get buy-in. You got to bring other people along. And again, this is a step where we see people often underinvest in the power and payoff of really executing it well.
CURT NICKISCH: How does that go wrong?
ANNE MORRISS: Yeah, people don’t know the why. Human behavior and the change in human behavior really depends on a strong why. It’s not just a selfish, “What’s in it for me?” Although that’s helpful, but where are we going? I may be invested in a status quo and I need to understand, okay, if you’re going to ask me to change, if you’re going to invite me into this uncomfortable place of doing things differently, why am I here? Help me understand it and articulate the way forward and language that not only I can understand, but also that’s going to be motivating to me.
CURT NICKISCH: And who on my team was part of this process and all that kind of stuff?
ANNE MORRISS: Oh, yeah. I may have some really important questions that may be in the way of my buy-in and commitment to this plan. So certainly creating a space where those questions can be addressed is essential. But what we found is that there is an architecture of a great change story, and it starts with honoring the past, honoring the starting place. Sometimes we’re so excited about the change and animated about the change that what has happened before or what is even happening in the present tense is low on our list of priorities.
Or we want to label it bad, because that’s the way we’ve thought about the change, but really pausing and honoring what came before you and all the reasonable decisions that led up to it, I think can be really helpful to getting people emotionally where you want them to be willing to be guided by you. Going back to Uber, when Dara Khosrowshahi came in.
CURT NICKISCH: This is the new CEO.
ANNE MORRISS: The new CEO.
CURT NICKISCH: Replaced Travis Kalanick, the founder and first CEO, yeah.
ANNE MORRISS: Yeah, and had his first all-hands meeting. One of his key messages, and this is a quote, was that he was going to retain the edge that had made Uber, “A force of nature.” And in that meeting, the crowd went wild because this is also a company that had been beaten up publicly for months and months and months, and it was a really powerful choice. And his predecessor, Travis was in the room, and he also honored Travis’ incredible work and investment in bringing the company to the place where it was.
And I would use words like grace to also describe those choices, but there’s also an incredible strategic value to naming the starting place for everybody in the room because in most cases, most people in that room played a role in getting to that starting place, and you’re acknowledging that.
CURT NICKISCH: You can call it grace. Somebody else might call it diplomatic or strategic. But yeah, I guess like it or not, it’s helpful to call out and honor the complexity of the way things have been done and also the change that’s happening.
ANNE MORRISS: Yeah, and the value. Sometimes honoring the past is also owning what didn’t work or what wasn’t working for stakeholders or segments of the employee team, and we see that around culture change. Sometimes you’ve got to acknowledge that it was not an equitable environment, but whatever the worker, everyone in that room is bringing that pass with them. So again, making it discussable and using it as the jumping off place is where we advise people to start.
Then you’ve earned the right to talk about the change mandate, which we suggest using clear and compelling language about the why. “This is what happened, this is where we are, this is the good and the bad of it, and here’s the case for change.”
And then the last part, which is to describe a rigorous and optimistic way forward. It’s a simple past, present, future arc, which will be familiar to human beings. We love stories as human beings. It’s among the most powerful currency we have to make sense of the world.
CURT NICKISCH: Yeah. Chronological is a pretty powerful order.
ANNE MORRISS: Right. But again, the change leaders we see really get it right, are investing an incredible amount of time into the storytelling part of their job. Ursula Burns, the Head of Xerox is famous for the months and years she spent on the road just telling the story of Xerox’s change, its pivot into services to everyone who would listen, and that was a huge part of her success.
CURT NICKISCH: So Friday or your fifth step, you end with empowering teams and removing roadblocks. That seems obvious, but it’s critical. Can you dig into that a little bit?
ANNE MORRISS: Yeah. Friday is the fun day. Friday’s the release of energy into the system. Again, you’ve now earned the right to go fast. You have a plan, you’re pretty confident it’s going to work. You’ve told the story of change the organization, and now you get to sprint. So this is about really executing with urgency, and it’s about a lot of the tactics of speed is where we focus in the book. So the tactics of empowerment, making tough strategic trade-offs so that your priorities are clear and clearly communicated, creating mechanisms to fast-track progress. At Etsy, CEO Josh Silverman, he labeled these projects ambulances. It’s an unfortunate metaphor, but it’s super memorable. These are the products that get to speed out in front of the other ones because the stakes are high and the clock is sticking.
CURT NICKISCH: You pull over and let it go by.
ANNE MORRISS: Yeah, exactly. And so we have to agree as an organization on how to do something like that. And so we see lots of great examples both in young organizations and big complex biotech companies with lots of regulatory guardrails have still found ways to do this gracefully.
And I think we end with this idea of conflict debt, which is a term we really love. Leanne Davey, who’s a team scholar and researcher, and anyone in a tech company will recognize the idea of tech debt, which is this weight the organization drags around until they resolve it. Conflict debt is a beautiful metaphor because it is this weight that we drag around and slows us down until we decide to clean it up and fix it. The organizations that are really getting speed right have figured out either formally or informally, how to create an environment where conflict and disagreements can be gracefully resolved.
CURT NICKISCH: Well, let’s talk about this speed more, right? Because I think this is one of those places that maybe people go wrong or take too long, and then you lose the awareness of the problem, you lose that urgency. And then that also just makes it less effective, right? It’s not just about getting the problem solved as quickly as possible. It’s also just speed in some ways helps solve the problem.
ANNE MORRISS: Oh, yeah. It really is the difference between imagining the change you want to lead and really being able to bring it to life. Speed is the thing that unlocks your ability to lead change. It needs a foundation, and that’s what Monday through Thursday is all about, steps one through four, but the finish line is executing with urgency, and it’s that urgency that releases the system’s energy, that communicates your priorities, that creates the conditions for your team to make progress.
CURT NICKISCH: Moving fast is something that entrepreneurs and tech companies certainly understand, but there’s also this awareness that with big companies, the bigger the organization, the harder it is to turn the aircraft carrier around, right? Is speed relative when you get at those levels, or do you think this is something that any company should be able to apply equally?
ANNE MORRISS: We think this applies to any company. The culture really lives at the level of team. So we believe you can make a tremendous amount of progress even within your circle of control as a team leader. I want to bring some humility to this and careful of words like universal, but we do think there’s some universal truths here around the value of speed, and then some of the byproducts like keeping fantastic people. Your best people want to solve problems, they want to execute, they want to make progress and speed, and the ability to do that is going to be a variable in their own equation of whether they stay or they go somewhere else where they can have an impact.
CURT NICKISCH: Right. They want to accomplish something before they go or before they retire or finish something out. And if you’re able to just bring more things on the horizon and have it not feel like it’s going to be another two years to do something meaningful.
ANNE MORRISS: People – I mean, they want to make stuff happen and they want to be around the energy and the vitality of making things happen, which again, is also a super infectious phenomenon. One of the most important jobs of a leader, we believe, is to set the metabolic pace of their teams and organizations. And so what we really dig into on Friday is, well, what does that look like to speed something up? What are the tactics of that?
CURT NICKISCH: I wonder if that universal truth, that a body in motion stays in motion applies to organizations, right? If an organization in motion stays in motion, there is something to that.
ANNE MORRISS: Absolutely.
CURT NICKISCH: Do you have a favorite client story to share, just where you saw speed just become a bit of a flywheel or just a positive reinforcement loop for more positive change at the organization?
ANNE MORRISS: Yeah. We work with a fair number of organizations that are on fire. We do a fair amount of firefighting, but we also less dramatically do a lot of fire prevention. So we’re brought into organizations that are working well and want to get better, looking out on the horizon. That work is super gratifying, and there is always a component of, well, how do we speed this up?
What I love about that work is there’s often already a high foundation of trust, and so it’s, well, how do we maintain that foundation but move this flywheel, as you said, even faster? And it’s really energizing because often there’s a lot of pent-up energy that… There’s a lot of loyalty to the organization, but often it’s also frustration and pent-up energy. And so when that gets released, when good people get the opportunity to sprint for the first time in a little while, it’s incredibly energizing, not just for us, but for the whole organization.
CURT NICKISCH: Anne, this is great. I think finding a way to solve problems better but also faster is going to be really helpful. So thanks for coming on the show to talk about it.
ANNE MORRISS: Oh, Curt, it was such a pleasure. This is my favorite conversation. I’m delighted to have it anytime.
HANNAH BATES: That was entrepreneur, leadership coach, and author Anne Morriss – in conversation with Curt Nickisch on HBR IdeaCast.
We’ll be back next Wednesday with another hand-picked conversation about business strategy from Harvard Business Review. If you found this episode helpful, share it with your friends and colleagues, and follow our show on Apple Podcasts, Spotify, or wherever you get your podcasts. While you’re there, be sure to leave us a review.
When you’re ready for more podcasts, articles, case studies, books, and videos with the world’s top business and management experts, you’ll find it all at HBR.org.
This episode was produced by Mary Dooe, Anne Saini, and me, Hannah Bates. Ian Fox is our editor. Special thanks to Rob Eckhardt, Maureen Hoch, Erica Truxler, Ramsey Khabbaz, Nicole Smith, Anne Bartholomew, and you – our listener. See you next week.
- Subscribe On:
Latest in this series
This article is about strategy.
- Decision making and problem solving
- Strategy execution
- Leadership and managing people
- Collaboration and teams
- Trustworthiness
- Organizational culture
Partner Center
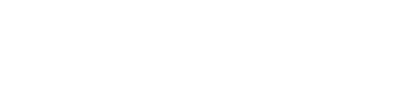
Along with Stanford news and stories, show me:
- Student information
- Faculty/Staff information
We want to provide announcements, events, leadership messages and resources that are relevant to you. Your selection is stored in a browser cookie which you can remove at any time using “Clear all personalization” below.
For everyone whose relationship with mathematics is distant or broken, Jo Boaler , a professor at Stanford Graduate School of Education (GSE), has ideas for repairing it. She particularly wants young people to feel comfortable with numbers from the start – to approach the subject with playfulness and curiosity, not anxiety or dread.
“Most people have only ever experienced what I call narrow mathematics – a set of procedures they need to follow, at speed,” Boaler says. “Mathematics should be flexible, conceptual, a place where we play with ideas and make connections. If we open it up and invite more creativity, more diverse thinking, we can completely transform the experience.”
Boaler, the Nomellini and Olivier Professor of Education at the GSE, is the co-founder and faculty director of Youcubed , a Stanford research center that provides resources for math learning that has reached more than 230 million students in over 140 countries. In 2013 Boaler, a former high school math teacher, produced How to Learn Math , the first massive open online course (MOOC) on mathematics education. She leads workshops and leadership summits for teachers and administrators, and her online courses have been taken by over a million users.
In her new book, Math-ish: Finding Creativity, Diversity, and Meaning in Mathematics , Boaler argues for a broad, inclusive approach to math education, offering strategies and activities for learners at any age. We spoke with her about why creativity is an important part of mathematics, the impact of representing numbers visually and physically, and how what she calls “ishing” a math problem can help students make better sense of the answer.
What do you mean by “math-ish” thinking?
It’s a way of thinking about numbers in the real world, which are usually imprecise estimates. If someone asks how old you are, how warm it is outside, how long it takes to drive to the airport – these are generally answered with what I call “ish” numbers, and that’s very different from the way we use and learn numbers in school.
In the book I share an example of a multiple-choice question from a nationwide exam where students are asked to estimate the sum of two fractions: 12/13 + 7/8. They’re given four choices for the closest answer: 1, 2, 19, or 21. Each of the fractions in the question is very close to 1, so the answer would be 2 – but the most common answer 13-year-olds gave was 19. The second most common was 21.
I’m not surprised, because when students learn fractions, they often don’t learn to think conceptually or to consider the relationship between the numerator or denominator. They learn rules about creating common denominators and adding or subtracting the numerators, without making sense of the fraction as a whole. But stepping back and judging whether a calculation is reasonable might be the most valuable mathematical skill a person can develop.
But don’t you also risk sending the message that mathematical precision isn’t important?
I’m not saying precision isn’t important. What I’m suggesting is that we ask students to estimate before they calculate, so when they come up with a precise answer, they’ll have a real sense for whether it makes sense. This also helps students learn how to move between big-picture and focused thinking, which are two different but equally important modes of reasoning.
Some people ask me, “Isn’t ‘ishing’ just estimating?” It is, but when we ask students to estimate, they often groan, thinking it’s yet another mathematical method. But when we ask them to “ish” a number, they're more willing to offer their thinking.
Ishing helps students develop a sense for numbers and shapes. It can help soften the sharp edges in mathematics, making it easier for kids to jump in and engage. It can buffer students against the dangers of perfectionism, which we know can be a damaging mindset. I think we all need a little more ish in our lives.
You also argue that mathematics should be taught in more visual ways. What do you mean by that?
For most people, mathematics is an almost entirely symbolic, numerical experience. Any visuals are usually sterile images in a textbook, showing bisecting angles, or circles divided into slices. But the way we function in life is by developing models of things in our minds. Take a stapler: Knowing what it looks like, what it feels and sounds like, how to interact with it, how it changes things – all of that contributes to our understanding of how it works.
There’s an activity we do with middle-school students where we show them an image of a 4 x 4 x 4 cm cube made up of smaller 1 cm cubes, like a Rubik’s Cube. The larger cube is dipped into a can of blue paint, and we ask the students, if they could take apart the little cubes, how many sides would be painted blue? Sometimes we give the students sugar cubes and have them physically build a larger 4 x 4 x 4 cube. This is an activity that leads into algebraic thinking.
Some years back we were interviewing students a year after they’d done that activity in our summer camp and asked what had stayed with them. One student said, “I’m in geometry class now, and I still remember that sugar cube, what it looked like and felt like.” His class had been asked to estimate the volume of their shoes, and he said he’d imagined his shoes filled with 1 cm sugar cubes in order to solve that question. He had built a mental model of a cube.
When we learn about cubes, most of us don’t get to see and manipulate them. When we learn about square roots, we don’t take squares and look at their diagonals. We just manipulate numbers.
I wonder if people consider the physical representations more appropriate for younger kids.
That’s the thing – elementary school teachers are amazing at giving kids those experiences, but it dies out in middle school, and by high school it’s all symbolic. There’s a myth that there’s a hierarchy of sophistication where you start out with visual and physical representations and then build up to the symbolic. But so much of high-level mathematical work now is visual. Here in Silicon Valley, if you look at Tesla engineers, they're drawing, they're sketching, they're building models, and nobody says that's elementary mathematics.
There’s an example in the book where you’ve asked students how they would calculate 38 x 5 in their heads, and they come up with several different ways of arriving at the same answer. The creativity is fascinating, but wouldn’t it be easier to teach students one standard method?
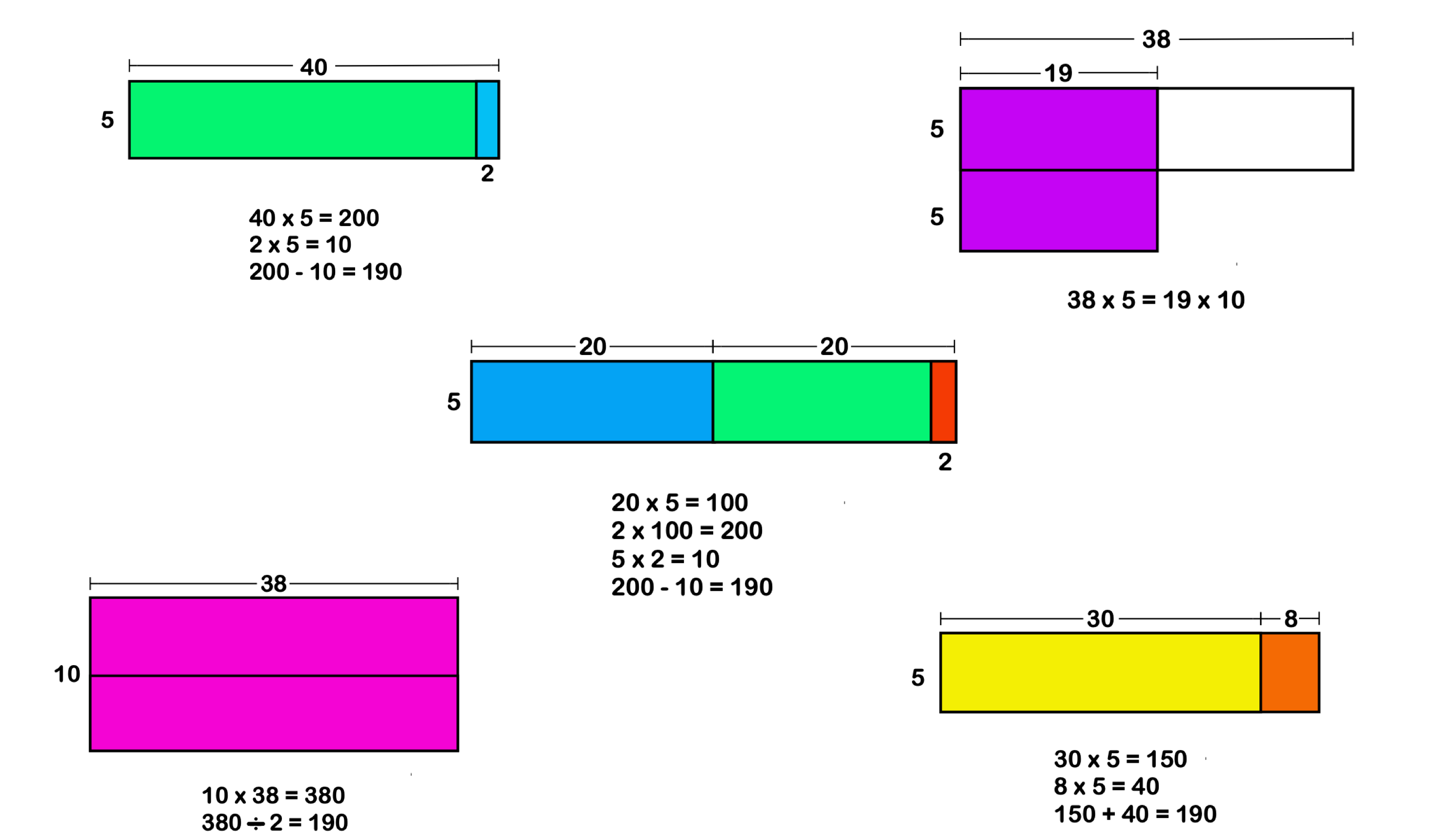
A depiction of various ways to calculate 38 x 5, numerically and visually. | Courtesy Jo Boaler
That narrow, rigid version of mathematics where there’s only one right approach is what most students experience, and it’s a big part of why people have such math trauma. It keeps them from realizing the full range and power of mathematics. When you only have students blindly memorizing math facts, they’re not developing number sense. They don’t learn how to use numbers flexibly in different situations. It also makes students who think differently believe there’s something wrong with them.
When we open mathematics to acknowledge the different ways a concept or problem can be viewed, we also open the subject to many more students. Mathematical diversity, to me, is a concept that includes both the value of diversity in people and the diverse ways we can see and learn mathematics. When we bring those forms of diversity together, it’s powerful. If we want to value different ways of thinking and problem-solving in the world, we need to embrace mathematical diversity.
Problem Solving Versus Modeling
- First Online: 30 November 2009
Cite this chapter
- Judith Zawojewski 5
2788 Accesses
13 Citations
This chapter is the third in a series intended to prompt discussion and debate in the Problem Solving vs. Modeling Theme Group. The chapter addresses distinctions between problem solving and modeling as a means to understand and conduct research by considering three main issues: What constitutes a problem-solving vs. modeling task?; What constitutes problem-solving vs. modeling processes?; and What are some implications for research?
This is a preview of subscription content, log in via an institution to check access.
Access this chapter
- Available as PDF
- Read on any device
- Instant download
- Own it forever
- Available as EPUB and PDF
- Compact, lightweight edition
- Dispatched in 3 to 5 business days
- Free shipping worldwide - see info
- Durable hardcover edition
Tax calculation will be finalised at checkout
Purchases are for personal use only
Institutional subscriptions
Kelly, E. A., Lesh, R. A., and Baek, J. (Eds.) (2008). Handbook of design research methods in education: Innovations in science, technology, engineering and mathematics learning and teaching . London: Routledge.
Google Scholar
Lesh, R., Hoover, M., Hole, B., Kelly, E., and Post, T. (2000). Principles for developing thought-revealing activities for students and teachers. In A. Kelly, and R. Lesh (Eds.), Handbook of Research Design in Mathematics and Science Education. Mahwah, NJ: Erlbaum.
Lesh, R., and Kelly, E. (2000). Multi-tiered teaching experiments. In E. Kelly, and R. Lesh (Eds.), Handbook of Research Design in Mathematics and Science Education. Mahwah, NJ: Erlbaum.
Lesh, R., and Zawojewski, J. S. (2007). Problem solving and modeling. In F. Lester (Ed.), Second Handbook of Research on Mathematics Teaching and Learning (pp. 763–804). Charlotte, NC: Information Age Publishing.
Newell, A., and Simon, H. A. (1972). Human Problem Solving . Englewood Cliffs, NJ: Prentice Hall.
Schoenfeld, A. H. (1992). Learning to think mathematically: Problem solving, metacognition, and sense making in mathematics. In D. Grouws (Ed.), Handbook of Research on Mathematics Teaching and Learning (pp. 334–370) . New York: McMillan.
Silver, E. A. (Ed.). (1985). Teaching and Learning Mathematical Problem Solving: Multiple Research Perspectives . Hillsdale, NJ: Erlbaum.
Vygotsky, L. S. (1978). Mind in Society: The Development of Higher Psychological Processes . Cambridge, MA: Harvard University Press.
Download references
Acknowledgment
Thank you to Frank K. Lester for his valuable feedback and suggestions, which led to revisions in this manuscript.
Author information
Authors and affiliations.
Illinois Institute of Technology, Chicago, IL, 60616, USA
Judith Zawojewski
You can also search for this author in PubMed Google Scholar
Corresponding author
Correspondence to Judith Zawojewski .
Editor information
Editors and affiliations.
School of Education, Indiana University, N. Rose Ave. 201, Bloomington, 47405-1006, USA
Richard Lesh
Graduate School of Education, University of Queensland, Brisbane, 4074, Australia
Peter L. Galbraith
Dept. Continuing Education, City University, Northampton Square, London, EC1V 0HB, United Kingdom
Christopher R. Haines
School of Education, University of Utah, Campus Center Drive 1705, Salt Lake City, 84112, USA
Andrew Hurford
Rights and permissions
Reprints and permissions
Copyright information
© 2010 Springer Science+Business Media, LLC
About this chapter
Zawojewski, J. (2010). Problem Solving Versus Modeling. In: Lesh, R., Galbraith, P., Haines, C., Hurford, A. (eds) Modeling Students' Mathematical Modeling Competencies. Springer, Boston, MA. https://doi.org/10.1007/978-1-4419-0561-1_20
Download citation
DOI : https://doi.org/10.1007/978-1-4419-0561-1_20
Published : 30 November 2009
Publisher Name : Springer, Boston, MA
Print ISBN : 978-1-4419-0560-4
Online ISBN : 978-1-4419-0561-1
eBook Packages : Humanities, Social Sciences and Law Education (R0)
Share this chapter
Anyone you share the following link with will be able to read this content:
Sorry, a shareable link is not currently available for this article.
Provided by the Springer Nature SharedIt content-sharing initiative
- Publish with us
Policies and ethics
- Find a journal
- Track your research
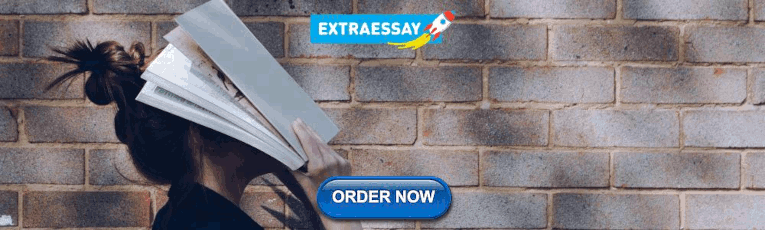
IMAGES
VIDEO
COMMENTS
The conventional problem-solving strategies usually taught have been to: Understanding the Problem; Devise a Plan; Carry Out the Plan; Look Back With her 5-7 year old students, Skinner moved through a sequence of activities that resulted in the students' being able to:
The conventional problem-solving strategies usually taught have been : understand the problem, devise a plan, carry out the plan, and look back. Evaluating the possible solutions to a problem and revising the solutions is the second step of problem solving.
The major cognitive processes in problem solving are representing, planning, executing, and monitoring. The major kinds of knowledge required for problem solving are facts, concepts, procedures, strategies, and beliefs. Classic theoretical approaches to the study of problem solving are associationism, Gestalt, and information processing.
In this educational context, problem solving always refers to the simultaneous use of strong methods for routine aspects of performance and knowledge-based methods for non-routine aspects of performance (e.g., reasoning, decision making). The remainder of this article will therefore focus on real-life problem solving. 3.
that problem solving can be thought of in three different ways: 1. Problem solving is a subject for study in and of itself. 2. Problem solving is an approach to a particular problem. 3. Problem solving is a way of teaching. Above all, teachers must focus their attention on their own ability to become competent problem solvers.
Before finding a solution to the problem, the problem must first be clearly identified. After that, one of many problem solving strategies can be applied, hopefully resulting in a solution. A problem-solving strategy is a plan of action used to find a solution. Different strategies have different action plans associated with them . For example ...
Abstract. This chapter follows the historical development of research on problem solving. It begins with a description of two research traditions that addressed different aspects of the problem-solving process: (1) research on problem representation (the Gestalt legacy) that examined how people understand the problem at hand, and (2) research on search in a problem space (the legacy of Newell ...
Collaborative problem-solving has been widely embraced in the classroom instruction of critical thinking, which is regarded as the core of curriculum reform based on key competencies in the field ...
1 Department of Psychology, University of Bamberg, Bamberg, Germany; 2 Department of Psychology, Heidelberg University, Heidelberg, Germany; Computer-simulated scenarios have been part of psychological research on problem solving for more than 40 years. The shift in emphasis from simple toy problems to complex, more real-life oriented problems has been accompanied by discussions about the best ...
Humans are innate creative problem-solvers. Since early humans developed the first stone tools to crack open fruit and nuts more than 2 million years ago, the application of creative thinking to solve problems has been a distinct competitive advantage for our species (Puccio 2017).Originally used to solve problems related to survival, the tendency toward the use of creative problem-solving to ...
The term problem solving is applied to a variety of activities ranging from puzzle solving, such as cleaning up a toxic spill, to the more complex and uncertain tasks of planning and policy making. In all cases, the typical problem-solving process is less rational than some would like to see. 1 Consequently, practical problem solving strays far from the rational-comprehensive ideal so revered ...
There have been five stages consistently found within the literature of problem solving: (1) identifying the problem, (2) representing the problem, (3) choosing the appropriate strategy, (4) implementing the strategy, and (5) assessing the solutions. This overview will focus on the first two stages of problem solving and examine how they ...
was theorized that the search strategies used, although important in attaining problem goals, were ineffective as learning devices. The extraneous cognitive load imposed by the problem-solving strategy interfered with learning. There are many experiments demonstrating that conventional problem solv-ing can have negative learning consequences.
Reasoning strategies in complex problem solving. Problem-solving skills have been among the most extensively studied transversal skills over the last decade; they have been investigated in the most prominent comprehensive international large-scale assessments today (e.g., OECD, 2014).The common aspects in the different theoretical models are that a problem is characterized by a gap between the ...
Some of the major difficulties novice learners face, when engaged in problem-solving processes, are concerned with the early stages of the solution design (Soloway 1986; Reed 1999; Robins et al. 2003) .In what follows, we present three strategies that may be employed in this stage of the solution design, i.e., defining the problem variables (Sect. 8.4.1), stepwise refinements (Sect. 8.4.2 ...
When teaching complex cognitive skills to no vices, the instructional strategy of presenting a set of worked examples for learners to study has been repeatedly found more effective than the conventional problem solving strategy in which they are provided problems to solve immediately after presentati on of information in
This has been stated by teachers who have joined the national council of teachers of mathematics (NCTM) since the 1980s, and advocated problem solving must be the focus of school mathematics (Sobel & Maletsky, 1988). Problem solving has been one of the general goals overall in the finish curriculum (Pehkonen, 2007). The problem solving is
Results indicated that the general problem-solving group solved more similar and transfer test problems than the conventional problem-solving group. It was concluded that previously acquired general problem-solving strategies can foster both learning and transfer, provided novice learners are directed to use them.
Disciplinary Foundations for Solving Interdisciplinary Scientific Problems. ERIC Educational Resources Information Center. Zhang, Dongmei; Shen, Ji. 2015-01-01. Problem-solving has been one of the major strands in science education research. But much of the problem-solving research has been conducted on discipline-based contexts; little research has been done on how students, especially ...
Problem-solving processes are common to many disciplines. In the mathematics education research community, for example, an intensive discussion takes place about problem-solving processes and techniques, as well as on learners' difficulties and ways of teaching problem-solving strategies. See, for example, Polya and Schoenfeld .
Arguments in favor of a problem-solving first approach (problem solving followed by instruction, or PS-I) are based on preparing students for future learning (Schwartz & Martin, 2004) by giving them opportunities to notice and encode critical domain features on their own (Loibl et al., 2017).By confronting students with challenging experiences (rather than shrinking the problem-space upfront ...
In this episode, she outlines a five-step process for solving any problem and explains why starting with trust and ending with speed is so important for effective change leadership. As she says ...
The Collaborative Problem Solving Approach. The Collaborative Problem Solving (CPS) approach represents a novel, practical, compassionate, and highly effective model for helping challenging children and those who work and live with them. The CPS approach was first articulated in the widely read book, The Explosive Child [ 3 ], and subsequently ...
The case for 'math-ish' thinking. In a new book, Jo Boaler argues for a more flexible, creative approach to math. "Stepping back and judging whether a calculation is reasonable might be the ...
Definitions of problem solving have been posed over the years, with no one definition emerging as the accepted one in the field. In the process of co-writing a chapter with Richard Lesh on mathematical problem solving and modeling for The Second Handbook of Research on Mathematics Teaching and Learning, I read a large number of definitions and discussions about the characteristics of problem ...