- Search Menu
- Browse content in A - General Economics and Teaching
- Browse content in A1 - General Economics
- A11 - Role of Economics; Role of Economists; Market for Economists
- A13 - Relation of Economics to Social Values
- A14 - Sociology of Economics
- Browse content in C - Mathematical and Quantitative Methods
- Browse content in C0 - General
- C02 - Mathematical Methods
- Browse content in C1 - Econometric and Statistical Methods and Methodology: General
- C10 - General
- C11 - Bayesian Analysis: General
- C12 - Hypothesis Testing: General
- C13 - Estimation: General
- C14 - Semiparametric and Nonparametric Methods: General
- C15 - Statistical Simulation Methods: General
- C18 - Methodological Issues: General
- Browse content in C2 - Single Equation Models; Single Variables
- C21 - Cross-Sectional Models; Spatial Models; Treatment Effect Models; Quantile Regressions
- C22 - Time-Series Models; Dynamic Quantile Regressions; Dynamic Treatment Effect Models; Diffusion Processes
- C23 - Panel Data Models; Spatio-temporal Models
- Browse content in C3 - Multiple or Simultaneous Equation Models; Multiple Variables
- C32 - Time-Series Models; Dynamic Quantile Regressions; Dynamic Treatment Effect Models; Diffusion Processes; State Space Models
- C38 - Classification Methods; Cluster Analysis; Principal Components; Factor Models
- Browse content in C4 - Econometric and Statistical Methods: Special Topics
- C45 - Neural Networks and Related Topics
- Browse content in C5 - Econometric Modeling
- C50 - General
- C51 - Model Construction and Estimation
- C52 - Model Evaluation, Validation, and Selection
- C53 - Forecasting and Prediction Methods; Simulation Methods
- C55 - Large Data Sets: Modeling and Analysis
- C58 - Financial Econometrics
- Browse content in C6 - Mathematical Methods; Programming Models; Mathematical and Simulation Modeling
- C61 - Optimization Techniques; Programming Models; Dynamic Analysis
- C62 - Existence and Stability Conditions of Equilibrium
- C65 - Miscellaneous Mathematical Tools
- Browse content in C7 - Game Theory and Bargaining Theory
- C70 - General
- C72 - Noncooperative Games
- C73 - Stochastic and Dynamic Games; Evolutionary Games; Repeated Games
- C78 - Bargaining Theory; Matching Theory
- Browse content in C8 - Data Collection and Data Estimation Methodology; Computer Programs
- C81 - Methodology for Collecting, Estimating, and Organizing Microeconomic Data; Data Access
- Browse content in C9 - Design of Experiments
- C91 - Laboratory, Individual Behavior
- C92 - Laboratory, Group Behavior
- C93 - Field Experiments
- Browse content in D - Microeconomics
- Browse content in D0 - General
- D03 - Behavioral Microeconomics: Underlying Principles
- Browse content in D1 - Household Behavior and Family Economics
- D10 - General
- D11 - Consumer Economics: Theory
- D12 - Consumer Economics: Empirical Analysis
- D14 - Household Saving; Personal Finance
- D15 - Intertemporal Household Choice: Life Cycle Models and Saving
- D18 - Consumer Protection
- Browse content in D2 - Production and Organizations
- D20 - General
- D21 - Firm Behavior: Theory
- D22 - Firm Behavior: Empirical Analysis
- D23 - Organizational Behavior; Transaction Costs; Property Rights
- D24 - Production; Cost; Capital; Capital, Total Factor, and Multifactor Productivity; Capacity
- D25 - Intertemporal Firm Choice: Investment, Capacity, and Financing
- Browse content in D3 - Distribution
- D30 - General
- D31 - Personal Income, Wealth, and Their Distributions
- Browse content in D4 - Market Structure, Pricing, and Design
- D40 - General
- D43 - Oligopoly and Other Forms of Market Imperfection
- D44 - Auctions
- D47 - Market Design
- D49 - Other
- Browse content in D5 - General Equilibrium and Disequilibrium
- D50 - General
- D51 - Exchange and Production Economies
- D52 - Incomplete Markets
- D53 - Financial Markets
- Browse content in D6 - Welfare Economics
- D60 - General
- D61 - Allocative Efficiency; Cost-Benefit Analysis
- D62 - Externalities
- Browse content in D7 - Analysis of Collective Decision-Making
- D70 - General
- D71 - Social Choice; Clubs; Committees; Associations
- D72 - Political Processes: Rent-seeking, Lobbying, Elections, Legislatures, and Voting Behavior
- D73 - Bureaucracy; Administrative Processes in Public Organizations; Corruption
- D74 - Conflict; Conflict Resolution; Alliances; Revolutions
- D78 - Positive Analysis of Policy Formulation and Implementation
- Browse content in D8 - Information, Knowledge, and Uncertainty
- D80 - General
- D81 - Criteria for Decision-Making under Risk and Uncertainty
- D82 - Asymmetric and Private Information; Mechanism Design
- D83 - Search; Learning; Information and Knowledge; Communication; Belief; Unawareness
- D84 - Expectations; Speculations
- D85 - Network Formation and Analysis: Theory
- D86 - Economics of Contract: Theory
- D87 - Neuroeconomics
- Browse content in D9 - Micro-Based Behavioral Economics
- D90 - General
- D91 - Role and Effects of Psychological, Emotional, Social, and Cognitive Factors on Decision Making
- D92 - Intertemporal Firm Choice, Investment, Capacity, and Financing
- Browse content in E - Macroeconomics and Monetary Economics
- Browse content in E0 - General
- E00 - General
- E03 - Behavioral Macroeconomics
- Browse content in E1 - General Aggregative Models
- E17 - Forecasting and Simulation: Models and Applications
- Browse content in E2 - Consumption, Saving, Production, Investment, Labor Markets, and Informal Economy
- E20 - General
- E21 - Consumption; Saving; Wealth
- E22 - Investment; Capital; Intangible Capital; Capacity
- E23 - Production
- E24 - Employment; Unemployment; Wages; Intergenerational Income Distribution; Aggregate Human Capital; Aggregate Labor Productivity
- Browse content in E3 - Prices, Business Fluctuations, and Cycles
- E30 - General
- E31 - Price Level; Inflation; Deflation
- E32 - Business Fluctuations; Cycles
- E37 - Forecasting and Simulation: Models and Applications
- Browse content in E4 - Money and Interest Rates
- E40 - General
- E41 - Demand for Money
- E42 - Monetary Systems; Standards; Regimes; Government and the Monetary System; Payment Systems
- E43 - Interest Rates: Determination, Term Structure, and Effects
- E44 - Financial Markets and the Macroeconomy
- E47 - Forecasting and Simulation: Models and Applications
- Browse content in E5 - Monetary Policy, Central Banking, and the Supply of Money and Credit
- E50 - General
- E51 - Money Supply; Credit; Money Multipliers
- E52 - Monetary Policy
- E58 - Central Banks and Their Policies
- Browse content in E6 - Macroeconomic Policy, Macroeconomic Aspects of Public Finance, and General Outlook
- E60 - General
- E61 - Policy Objectives; Policy Designs and Consistency; Policy Coordination
- E62 - Fiscal Policy
- E63 - Comparative or Joint Analysis of Fiscal and Monetary Policy; Stabilization; Treasury Policy
- E64 - Incomes Policy; Price Policy
- E65 - Studies of Particular Policy Episodes
- E66 - General Outlook and Conditions
- Browse content in E7 - Macro-Based Behavioral Economics
- E71 - Role and Effects of Psychological, Emotional, Social, and Cognitive Factors on the Macro Economy
- Browse content in F - International Economics
- Browse content in F0 - General
- F02 - International Economic Order and Integration
- Browse content in F1 - Trade
- F14 - Empirical Studies of Trade
- Browse content in F2 - International Factor Movements and International Business
- F21 - International Investment; Long-Term Capital Movements
- F22 - International Migration
- F23 - Multinational Firms; International Business
- Browse content in F3 - International Finance
- F30 - General
- F31 - Foreign Exchange
- F32 - Current Account Adjustment; Short-Term Capital Movements
- F33 - International Monetary Arrangements and Institutions
- F34 - International Lending and Debt Problems
- F36 - Financial Aspects of Economic Integration
- F37 - International Finance Forecasting and Simulation: Models and Applications
- F38 - International Financial Policy: Financial Transactions Tax; Capital Controls
- Browse content in F4 - Macroeconomic Aspects of International Trade and Finance
- F40 - General
- F41 - Open Economy Macroeconomics
- F42 - International Policy Coordination and Transmission
- F43 - Economic Growth of Open Economies
- F44 - International Business Cycles
- F47 - Forecasting and Simulation: Models and Applications
- Browse content in F5 - International Relations, National Security, and International Political Economy
- F51 - International Conflicts; Negotiations; Sanctions
- Browse content in F6 - Economic Impacts of Globalization
- F63 - Economic Development
- F65 - Finance
- Browse content in G - Financial Economics
- Browse content in G0 - General
- G00 - General
- G01 - Financial Crises
- G02 - Behavioral Finance: Underlying Principles
- Browse content in G1 - General Financial Markets
- G10 - General
- G11 - Portfolio Choice; Investment Decisions
- G12 - Asset Pricing; Trading volume; Bond Interest Rates
- G13 - Contingent Pricing; Futures Pricing
- G14 - Information and Market Efficiency; Event Studies; Insider Trading
- G15 - International Financial Markets
- G17 - Financial Forecasting and Simulation
- G18 - Government Policy and Regulation
- G19 - Other
- Browse content in G2 - Financial Institutions and Services
- G20 - General
- G21 - Banks; Depository Institutions; Micro Finance Institutions; Mortgages
- G22 - Insurance; Insurance Companies; Actuarial Studies
- G23 - Non-bank Financial Institutions; Financial Instruments; Institutional Investors
- G24 - Investment Banking; Venture Capital; Brokerage; Ratings and Ratings Agencies
- G28 - Government Policy and Regulation
- G29 - Other
- Browse content in G3 - Corporate Finance and Governance
- G30 - General
- G31 - Capital Budgeting; Fixed Investment and Inventory Studies; Capacity
- G32 - Financing Policy; Financial Risk and Risk Management; Capital and Ownership Structure; Value of Firms; Goodwill
- G33 - Bankruptcy; Liquidation
- G34 - Mergers; Acquisitions; Restructuring; Corporate Governance
- G35 - Payout Policy
- G38 - Government Policy and Regulation
- G39 - Other
- Browse content in G4 - Behavioral Finance
- G40 - General
- G41 - Role and Effects of Psychological, Emotional, Social, and Cognitive Factors on Decision Making in Financial Markets
- Browse content in G5 - Household Finance
- G50 - General
- G51 - Household Saving, Borrowing, Debt, and Wealth
- G52 - Insurance
- G53 - Financial Literacy
- Browse content in H - Public Economics
- H0 - General
- Browse content in H1 - Structure and Scope of Government
- H11 - Structure, Scope, and Performance of Government
- H19 - Other
- Browse content in H2 - Taxation, Subsidies, and Revenue
- H22 - Incidence
- H24 - Personal Income and Other Nonbusiness Taxes and Subsidies; includes inheritance and gift taxes
- H25 - Business Taxes and Subsidies
- H26 - Tax Evasion and Avoidance
- Browse content in H3 - Fiscal Policies and Behavior of Economic Agents
- H31 - Household
- Browse content in H4 - Publicly Provided Goods
- H40 - General
- H41 - Public Goods
- Browse content in H5 - National Government Expenditures and Related Policies
- H50 - General
- H52 - Government Expenditures and Education
- H53 - Government Expenditures and Welfare Programs
- H54 - Infrastructures; Other Public Investment and Capital Stock
- H55 - Social Security and Public Pensions
- H56 - National Security and War
- H57 - Procurement
- Browse content in H6 - National Budget, Deficit, and Debt
- H63 - Debt; Debt Management; Sovereign Debt
- Browse content in H7 - State and Local Government; Intergovernmental Relations
- H70 - General
- H72 - State and Local Budget and Expenditures
- H74 - State and Local Borrowing
- H75 - State and Local Government: Health; Education; Welfare; Public Pensions
- Browse content in H8 - Miscellaneous Issues
- H81 - Governmental Loans; Loan Guarantees; Credits; Grants; Bailouts
- Browse content in I - Health, Education, and Welfare
- Browse content in I1 - Health
- I11 - Analysis of Health Care Markets
- I12 - Health Behavior
- I13 - Health Insurance, Public and Private
- I18 - Government Policy; Regulation; Public Health
- Browse content in I2 - Education and Research Institutions
- I22 - Educational Finance; Financial Aid
- I23 - Higher Education; Research Institutions
- I28 - Government Policy
- Browse content in I3 - Welfare, Well-Being, and Poverty
- I30 - General
- I38 - Government Policy; Provision and Effects of Welfare Programs
- Browse content in J - Labor and Demographic Economics
- Browse content in J0 - General
- J00 - General
- Browse content in J1 - Demographic Economics
- J11 - Demographic Trends, Macroeconomic Effects, and Forecasts
- J12 - Marriage; Marital Dissolution; Family Structure; Domestic Abuse
- J13 - Fertility; Family Planning; Child Care; Children; Youth
- J15 - Economics of Minorities, Races, Indigenous Peoples, and Immigrants; Non-labor Discrimination
- J16 - Economics of Gender; Non-labor Discrimination
- J18 - Public Policy
- Browse content in J2 - Demand and Supply of Labor
- J20 - General
- J21 - Labor Force and Employment, Size, and Structure
- J22 - Time Allocation and Labor Supply
- J23 - Labor Demand
- J24 - Human Capital; Skills; Occupational Choice; Labor Productivity
- J26 - Retirement; Retirement Policies
- J28 - Safety; Job Satisfaction; Related Public Policy
- Browse content in J3 - Wages, Compensation, and Labor Costs
- J30 - General
- J31 - Wage Level and Structure; Wage Differentials
- J32 - Nonwage Labor Costs and Benefits; Retirement Plans; Private Pensions
- J33 - Compensation Packages; Payment Methods
- J38 - Public Policy
- Browse content in J4 - Particular Labor Markets
- J41 - Labor Contracts
- J44 - Professional Labor Markets; Occupational Licensing
- J45 - Public Sector Labor Markets
- J46 - Informal Labor Markets
- J49 - Other
- Browse content in J5 - Labor-Management Relations, Trade Unions, and Collective Bargaining
- J51 - Trade Unions: Objectives, Structure, and Effects
- J52 - Dispute Resolution: Strikes, Arbitration, and Mediation; Collective Bargaining
- Browse content in J6 - Mobility, Unemployment, Vacancies, and Immigrant Workers
- J61 - Geographic Labor Mobility; Immigrant Workers
- J62 - Job, Occupational, and Intergenerational Mobility
- J63 - Turnover; Vacancies; Layoffs
- J64 - Unemployment: Models, Duration, Incidence, and Job Search
- J65 - Unemployment Insurance; Severance Pay; Plant Closings
- J68 - Public Policy
- Browse content in J7 - Labor Discrimination
- J71 - Discrimination
- Browse content in J8 - Labor Standards: National and International
- J88 - Public Policy
- Browse content in K - Law and Economics
- Browse content in K1 - Basic Areas of Law
- K12 - Contract Law
- Browse content in K2 - Regulation and Business Law
- K22 - Business and Securities Law
- K23 - Regulated Industries and Administrative Law
- Browse content in K3 - Other Substantive Areas of Law
- K31 - Labor Law
- K32 - Environmental, Health, and Safety Law
- K34 - Tax Law
- K35 - Personal Bankruptcy Law
- Browse content in K4 - Legal Procedure, the Legal System, and Illegal Behavior
- K42 - Illegal Behavior and the Enforcement of Law
- Browse content in L - Industrial Organization
- Browse content in L1 - Market Structure, Firm Strategy, and Market Performance
- L10 - General
- L11 - Production, Pricing, and Market Structure; Size Distribution of Firms
- L13 - Oligopoly and Other Imperfect Markets
- L14 - Transactional Relationships; Contracts and Reputation; Networks
- L15 - Information and Product Quality; Standardization and Compatibility
- Browse content in L2 - Firm Objectives, Organization, and Behavior
- L21 - Business Objectives of the Firm
- L22 - Firm Organization and Market Structure
- L23 - Organization of Production
- L24 - Contracting Out; Joint Ventures; Technology Licensing
- L25 - Firm Performance: Size, Diversification, and Scope
- L26 - Entrepreneurship
- L29 - Other
- Browse content in L3 - Nonprofit Organizations and Public Enterprise
- L33 - Comparison of Public and Private Enterprises and Nonprofit Institutions; Privatization; Contracting Out
- Browse content in L4 - Antitrust Issues and Policies
- L43 - Legal Monopolies and Regulation or Deregulation
- L44 - Antitrust Policy and Public Enterprises, Nonprofit Institutions, and Professional Organizations
- Browse content in L5 - Regulation and Industrial Policy
- L51 - Economics of Regulation
- Browse content in L6 - Industry Studies: Manufacturing
- L66 - Food; Beverages; Cosmetics; Tobacco; Wine and Spirits
- Browse content in L8 - Industry Studies: Services
- L81 - Retail and Wholesale Trade; e-Commerce
- L85 - Real Estate Services
- L86 - Information and Internet Services; Computer Software
- Browse content in L9 - Industry Studies: Transportation and Utilities
- L92 - Railroads and Other Surface Transportation
- L94 - Electric Utilities
- Browse content in M - Business Administration and Business Economics; Marketing; Accounting; Personnel Economics
- Browse content in M0 - General
- M00 - General
- Browse content in M1 - Business Administration
- M12 - Personnel Management; Executives; Executive Compensation
- M13 - New Firms; Startups
- M14 - Corporate Culture; Social Responsibility
- M16 - International Business Administration
- Browse content in M2 - Business Economics
- M20 - General
- M21 - Business Economics
- Browse content in M3 - Marketing and Advertising
- M30 - General
- M31 - Marketing
- M37 - Advertising
- Browse content in M4 - Accounting and Auditing
- M40 - General
- M41 - Accounting
- M42 - Auditing
- M48 - Government Policy and Regulation
- Browse content in M5 - Personnel Economics
- M51 - Firm Employment Decisions; Promotions
- M52 - Compensation and Compensation Methods and Their Effects
- M54 - Labor Management
- Browse content in N - Economic History
- Browse content in N1 - Macroeconomics and Monetary Economics; Industrial Structure; Growth; Fluctuations
- N10 - General, International, or Comparative
- N12 - U.S.; Canada: 1913-
- Browse content in N2 - Financial Markets and Institutions
- N20 - General, International, or Comparative
- N21 - U.S.; Canada: Pre-1913
- N22 - U.S.; Canada: 1913-
- N23 - Europe: Pre-1913
- N24 - Europe: 1913-
- N25 - Asia including Middle East
- N27 - Africa; Oceania
- Browse content in N3 - Labor and Consumers, Demography, Education, Health, Welfare, Income, Wealth, Religion, and Philanthropy
- N32 - U.S.; Canada: 1913-
- Browse content in N4 - Government, War, Law, International Relations, and Regulation
- N43 - Europe: Pre-1913
- Browse content in N7 - Transport, Trade, Energy, Technology, and Other Services
- N71 - U.S.; Canada: Pre-1913
- Browse content in N8 - Micro-Business History
- N80 - General, International, or Comparative
- N82 - U.S.; Canada: 1913-
- Browse content in O - Economic Development, Innovation, Technological Change, and Growth
- Browse content in O1 - Economic Development
- O11 - Macroeconomic Analyses of Economic Development
- O12 - Microeconomic Analyses of Economic Development
- O13 - Agriculture; Natural Resources; Energy; Environment; Other Primary Products
- O16 - Financial Markets; Saving and Capital Investment; Corporate Finance and Governance
- O17 - Formal and Informal Sectors; Shadow Economy; Institutional Arrangements
- Browse content in O2 - Development Planning and Policy
- O23 - Fiscal and Monetary Policy in Development
- Browse content in O3 - Innovation; Research and Development; Technological Change; Intellectual Property Rights
- O30 - General
- O31 - Innovation and Invention: Processes and Incentives
- O32 - Management of Technological Innovation and R&D
- O33 - Technological Change: Choices and Consequences; Diffusion Processes
- O34 - Intellectual Property and Intellectual Capital
- O35 - Social Innovation
- O38 - Government Policy
- Browse content in O4 - Economic Growth and Aggregate Productivity
- O40 - General
- O43 - Institutions and Growth
- Browse content in O5 - Economywide Country Studies
- O53 - Asia including Middle East
- Browse content in P - Economic Systems
- Browse content in P1 - Capitalist Systems
- P16 - Political Economy
- P18 - Energy: Environment
- Browse content in P2 - Socialist Systems and Transitional Economies
- P26 - Political Economy; Property Rights
- Browse content in P3 - Socialist Institutions and Their Transitions
- P31 - Socialist Enterprises and Their Transitions
- P34 - Financial Economics
- P39 - Other
- Browse content in P4 - Other Economic Systems
- P43 - Public Economics; Financial Economics
- P48 - Political Economy; Legal Institutions; Property Rights; Natural Resources; Energy; Environment; Regional Studies
- Browse content in Q - Agricultural and Natural Resource Economics; Environmental and Ecological Economics
- Browse content in Q0 - General
- Q02 - Commodity Markets
- Browse content in Q3 - Nonrenewable Resources and Conservation
- Q31 - Demand and Supply; Prices
- Q32 - Exhaustible Resources and Economic Development
- Browse content in Q4 - Energy
- Q40 - General
- Q41 - Demand and Supply; Prices
- Q42 - Alternative Energy Sources
- Q43 - Energy and the Macroeconomy
- Browse content in Q5 - Environmental Economics
- Q50 - General
- Q51 - Valuation of Environmental Effects
- Q53 - Air Pollution; Water Pollution; Noise; Hazardous Waste; Solid Waste; Recycling
- Q54 - Climate; Natural Disasters; Global Warming
- Q56 - Environment and Development; Environment and Trade; Sustainability; Environmental Accounts and Accounting; Environmental Equity; Population Growth
- Browse content in R - Urban, Rural, Regional, Real Estate, and Transportation Economics
- Browse content in R0 - General
- R00 - General
- Browse content in R1 - General Regional Economics
- R10 - General
- R11 - Regional Economic Activity: Growth, Development, Environmental Issues, and Changes
- R12 - Size and Spatial Distributions of Regional Economic Activity
- Browse content in R2 - Household Analysis
- R20 - General
- R21 - Housing Demand
- R23 - Regional Migration; Regional Labor Markets; Population; Neighborhood Characteristics
- Browse content in R3 - Real Estate Markets, Spatial Production Analysis, and Firm Location
- R30 - General
- R31 - Housing Supply and Markets
- R32 - Other Spatial Production and Pricing Analysis
- R33 - Nonagricultural and Nonresidential Real Estate Markets
- R38 - Government Policy
- Browse content in R4 - Transportation Economics
- R41 - Transportation: Demand, Supply, and Congestion; Travel Time; Safety and Accidents; Transportation Noise
- Browse content in R5 - Regional Government Analysis
- R51 - Finance in Urban and Rural Economies
- Browse content in Z - Other Special Topics
- Browse content in Z1 - Cultural Economics; Economic Sociology; Economic Anthropology
- Z11 - Economics of the Arts and Literature
- Z13 - Economic Sociology; Economic Anthropology; Social and Economic Stratification
- Advance articles
- Editor's Choice
- Author Guidelines
- Submission Site
- Open Access
- About The Review of Financial Studies
- Editorial Board
- Advertising and Corporate Services
- Journals Career Network
- Self-Archiving Policy
- Dispatch Dates
- Terms and Conditions
- Journals on Oxford Academic
- Books on Oxford Academic
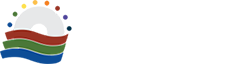
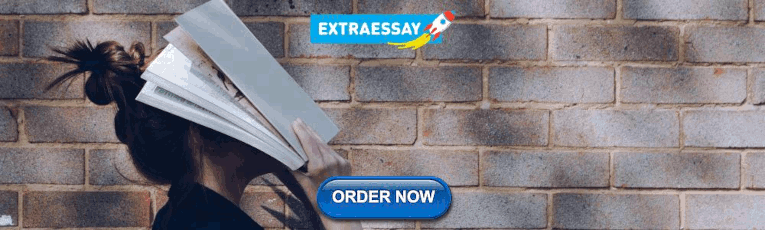
Article Contents
1. empirically grounded models of subjective beliefs, 2. expectation formation in asset pricing, 3. macro and monetary economics connections, 4. macro and monetary drivers of asset prices, 5. intermediary asset pricing and beyond, 6. intermediary asset pricing: assessment and future directions, 7. international finance, 8. an asset pricing view of exchange rates, acknowledgement.
- < Previous
Review Article: Perspectives on the Future of Asset Pricing
- Article contents
- Figures & tables
- Supplementary Data
Markus Brunnermeier, Emmanuel Farhi, Ralph S J Koijen, Arvind Krishnamurthy, Sydney C Ludvigson, Hanno Lustig, Stefan Nagel, Monika Piazzesi, Review Article: Perspectives on the Future of Asset Pricing, The Review of Financial Studies , Volume 34, Issue 4, April 2021, Pages 2126–2160, https://doi.org/10.1093/rfs/hhaa129
- Permissions Icon Permissions
The field of asset pricing is a rich and diverse discipline that has contributed to many areas of discourse, including those of fundamental importance to policy makers, investors, and households. 1 As we look ahead during a time of substantial economic and political change, it is apparent that society faces many pressing questions, both new and old, that the field is uniquely suited to informing.
To contribute to this conversation, the NBER Asset Pricing program convened a panel discussion on “Perspectives on the Future of Asset Pricing” at its November 8, 2019, meeting that took place at Stanford University. The objective of the panel was to identify some of the important questions the field could productively address in the next five to 10 years. The panelists, consisting of experts in several subfields of asset pricing, were invited to share their views on these questions with an eye toward innovative research topics that are ripe for exploring, and the metrics the field could be using to gauge progress.
This article summarizes these views. The list of topics covered by the panelists is by no means exhaustive. We hope that this article shall nevertheless serve as a productive conduit for identifying some key questions for investigation and generating a concerted research effort toward answering them.
Beliefs are central to asset pricing. 2 Asset prices are forward-looking, and essentially any asset-pricing model implies that investors price assets based on their beliefs about the joint distribution of some stochastic discount factor (SDF) |$M_{t+1}$| and payoffs |$X_{t+1}$| . An observer outside the field of asset pricing might therefore guess that a major part of the research efforts in asset pricing are devoted to understanding how investors form beliefs. This is, at least so far, not the case.
The vast majority of theoretical and empirical work in asset pricing is based on the rational expectations (RE) paradigm. Under RE, as in Lucas (1978) , investors are assumed to know the economy’s underlying model and the model parameters, and to forecast rationally.
Within the RE paradigm, there is no role for the study of beliefs: theoretically, beliefs are implied by the model; empirically, an econometrician can recover investor beliefs from the large-sample empirical distribution of |$M_{t+1}$| and |$X_{t+1}$| .
RE-based approaches have been useful to establish a benchmark, but asset prices have not yielded easily to RE-based explanations. Seeing the increasingly complicated dynamics in preferences and endowment processes researchers use to reverse-engineer better-fitting RE models, it is natural to wonder whether more progress could be made by treating belief dynamics as an object of theoretical and empirical study.
I propose that such a research program should be organized around the following three principles:
Research focus should be on motivating, building, calibrating, and estimating models with non-RE beliefs rather than on merely rejecting RE models. To make further progress, we need structural models of belief dynamics that can compete with RE models in explaining asset prices and empirically observed beliefs.
Deviating from RE does not necessarily imply assuming irrationality. For example, models of Bayesian learning relax the RE assumption that agents know the model of the world and its parameter values, while retaining the rational forecasting assumption. Exploration of cognitive limitations, bounded rationality, and heuristics that relax the rational forecasting assumption may have promising insights to offer as well, but even models of rational learning can produce asset-price properties that are quite different from those in an RE setting. 3
Belief dynamics should be disciplined with data on beliefs and micro decisions. While reverse-engineering of preferences and technology to fit asset prices is common in the literature, I would argue that we should not follow this approach with beliefs. Taking beliefs seriously as an object of scientific study also means bringing in empirical data that helps pin down their dynamics.
In line with these principles, a number of areas seem promising for future research:
1.1 Belief measurement
The availability of beliefs data has improved substantially in recent years, but for beliefs data to become a standard ingredient of asset-pricing research, further progress on measurement is necessary.
So far we do not have a good understanding of investor expectations of firm cash flows. Existing work on expectations in asset pricing has often focused on return expectations, but return expectations alone, especially ones limited to relatively short forecast horizons, do not provide a complete picture of how beliefs dynamics explain variation in price levels. Forecasts of earnings and dividends by analysts, used in De la O and Myers (2019) , and by CFOs in the Graham and Harvey (2011) survey are a start, but there is more to be done.
Collecting more data on long-run expectations would be useful. Much of the currently available expectations data is focused on short forecast horizons such as one year. Asset prices, however, depend on expectations over much longer horizons.
In addition to perceived first moments, investors’ subjective perception of risk are also of obvious relevance to asset pricing. 4 Data on beliefs about the perceived downside tails of the distribution would be particularly interesting. Since crashes and disasters are infrequent, objective historical data does little to pin down these tails, leaving lots of room for subjective judgements. Eliciting beliefs about the shape of distributions is a challenging problem, but further research on belief elicitation methods may bring progress in this area, too ( Manski, 2018 ).
Finally, in many asset-pricing applications, we are interested in dynamics of beliefs at frequencies of the business cycle, or even lower. This means that we need long time series. The time series available in survey data have lengthened substantially, but there are still potential benefits from innovations that can help to extend beliefs data backwards in time, for example, with proxies constructed from textual analysis of media, somewhat akin to what Manela and Moreira (2017) have done to extend the VIX index.
1.2 Beliefs and actions
If a respondent in a survey states a belief, this does not necessarily mean that the respondent is ready to act in accordance with this stated belief. In addition to a decision-relevant signal, stated beliefs likely contain measurement noise. For example, it is unlikely that respondents deliberate as carefully when stating beliefs as they would if they actually had to take an action. Moreover, even if stated beliefs truly reflect respondents’ perceptions, actual or cognitive costs of taking an action may prevent respondents from perfectly aligning their choices with these beliefs. To sort out these issues, more research on the connection of beliefs and actions is needed. 5
For asset pricing, we also need to understand the properties of this measurement error when we aggregate across respondents. If measurement error averages out within the population, or within demographic groups, then matching asset-pricing models with beliefs moments based on such aggregates may be fine, even if the links between stated beliefs and actions are distorted by measurement error at the individual level. To the extent that more sophisticated agents play a bigger role in financial markets, how the belief-action relationship varies with sophistication is an important issue as well. 6
Another important question to sort out is whose beliefs matter for pricing. Individual investors’ beliefs can differ from those of professional investors. The relative importance of their beliefs in influencing asset prices likely differs by aggregation level: For allocation decisions at the asset class level (e.g. stocks vs. bonds), it seems likely that individuals exert substantial influence because the investment products they choose from often have predetermined allocations to an asset class; at the individual stock level, fund managers have discretion; at the investment-style level (e.g., small vs. large stocks) there is probably a mix of predetermined choices by individuals and managerial discretion. Sorting this out empirically would also help in linking belief-based approaches with a demand-system analysis as in Koijen and Yogo (2019) .
1.3 Modeling belief formation
Models of investor belief dynamics need to take a stand on the sources of information that investors rely on when they form their beliefs and how they digest this information to produce forecasts. Two questions in this regard seem particularly interesting.
How do investors form beliefs when faced with high-dimensional prediction problems? Existing asset-pricing models with parameter learning typically consider settings with a small number of predictor variables. Reality, however, looks different. For example, to value stocks, investors must forecast cash flows. They observe a vast number of potential predictor variables, but they do not know the precise functional relationship between predictors and future cash flows and need to learn it from observed data. In a simple linear setting with homogeneous investors, Martin and Nagel (2019) show that this can give rise to return predictability and a “factor zoo.” Further efforts to close the gap between the simplistic environments investors face in asset-pricing models and the messy real world seem necessary.
The second question concerns the role of memory. Data can shape beliefs only if it is remembered. In theory, one could imagine a Bayesian learner that takes into account “all available” history when learning about pricing-relevant stochastic processes. But when mapping these models into the real world, it is not clear what “all available” means. Some implementations of learning models set time zero to 1926, because this is where the CRSP database starts, but this is obviously not the true starting point of investors’ learning process. Moreover, there are reasons to expect that memory could be limited. More research, both empirically and theoretically, is needed to better understand investors’ formation of memory, including those of institutions (e.g., through maintenance of data sets or establishment of decision rules). 7
1.4 Beyond asset pricing: Macro-finance
The drivers of stock price dynamics emphasized in asset pricing research are largely disconnected from the drivers of the business cycle that macroeconomists focus on (see, e.g., Cochrane 2017 ). This question should be revisited through the lens of models with non-RE belief dynamics. Shocks to beliefs are potential source of links between asset prices and macro quantities. Exactly how such links could play out is an open question. 8
Beliefs effects could operate in ways that are quite different from time-varying preferences that macro and asset-pricing research has already explored in various ways. For example, belief effects can be specific to technologies or markets. An individual could be optimistic about the housing market but, at the same time, pessimistic about the stock market. Beliefs data will be important to sort out the commonalities and differences between different sectors and markets.
Interactions of beliefs with frictions are potentially interesting. For example, belief heterogeneity can interact with frictions in a way that amplifies shocks ( Caballero and Simsek (2020) ). The housing market seems to be a particularly interesting area to explore these types of mechanisms, as it features substantial frictions and plays a big role in the macroeconomy.
1.5 Conclusion
Asset prices express investors’ beliefs about the future. Our understanding of how investors form these beliefs, how they evolve over time, and how we can measure them is still limited. Empirically grounded research on investor beliefs holds promise to unlock some of the mysteries of asset pricing.
The conventional agenda in the asset pricing literature studies quantitative rational expectation models. 9 This approach is particularly well suited for the study of recurring patterns, such as the comovement of price-dividend ratios on stocks with the business cycle or the seasonality in the housing market during a calendar year. (Volume and prices are above trend during the summer, while activity in housing markets slows down in the winter.) In rational expectation models, the expectations of agents reflect these recurring patterns and are consistent with the equilibrium dynamics of the model. The model is successful if the distributions of equilibrium prices and quantities are consistent with those in the data.
An advantage of this approach is that agents’ expectations do not introduce free parameters. Rational expectations impose cross-equation restrictions on agents’ expectations and equilibrium dynamics that constrain these parameters to be the same. This approach imposes a welcome discipline on the model if there are no data on expectations. By design, the approach assumes that all agents have the same expectations. To study differences in expectations, a researcher has to specify the source of information that only some agents may receive, while others do not.
Recent years have witnessed a massive effort to collect expectational data. These new data enable researchers to be more agnostic about how agents arrive at their expectations. It is now possible to discipline expectations with direct observations on households and firms. More and more surveys that ask respondents about their expectations also ask them about their characteristics (e.g., household or firm age, income, or sales) and choices (e.g., investments). These data allow researchers to study the joint distribution of expectations, characteristics, and choices. Now a model is successful if it can match the observed joint distribution in the data.
Freeing up expectations is especially appealing to study unique episodes that are associated with structural change. In these instances, it is often not clear how agents were forming expectations at the time. For example, what explains postwar house price booms? Two major boom-bust episodes stand out in the United States, because they coincide with booms in other countries (e.g., chapter 4.5 in Piazzesi and Schneider 2016 ). The first boom occurred during the late 1970s and early 1980s, while the second boom occurred in the early 2000s; both episodes had unique features. An important contributor to the first boom was the Great Inflation. How did households form expectations about future inflation during this unique event? Low interest rates during the second boom made it cheap for households to borrow and increased the value of houses, computed as the present value of a stream of future housing services. When house prices collapsed in 2007, interest rates came down further and remained close to zero. Did households during the boom foresee the low rates after the collapse? Did home buyers at the peak of the boom expect house prices to further appreciate, or were they aware that house prices were about to decline? What did renters expect during these years? Again, surveys help us understand households’ expectations and actions during this unique episode. It is difficult to think about these house price booms as recurring patterns.
2.1 Big data collection efforts
Central banks have recently pioneered massive data collection efforts to improve the foundations of their economic models and ultimately their conduct of monetary policy. Many private companies, such as Vanguard, contribute to this effort because they want to better understand their clients. The surveys ask individual households or firms about their expectations for the future. Some surveys ask respondents to forecast aggregate variables: macro-economic indicators (e.g., inflation, GDP growth) or financial variables (e.g., stock returns, bond returns). Other questions ask about individual-specific variables such as income. More and more, surveys ask the same respondents about their expectations and actual choices. For example, households are asked about their stock return forecasts and stock holdings. Other surveys ask firms about their current sales and sales forecasts.
Examples of such surveys include the European Community Household Panel by the European Central Bank and its member banks, which asks a panel of households about their income and living conditions. There are modules in the survey that ask about household expectations. Since 2011, the Bundesbank has been conducting the Panel of Household Finances, which asks households about their expectations and their choices. Since 2013, the Federal Reserve Bank of New York has the Survey of Consumer Expectations, and the Federal Reserve Board has the Survey of Household Economics and Decision Making. The Bank of Canada has conducted the Canadian Survey of Consumer Expectations since 2015.
There has been considerable progress in how to frame the survey questions in a way that enables people without formal training in statistics to express their perceived uncertainty about these forecasts. This progress is often made by academics who are directly involved in the survey. For example, Bachmann et al. (2019) ask German firms about their future sales growth in the Ifo Business Tendency Survey. To get a measure of the uncertainty that firms perceive, the researchers formulate a survey question that asks firms to provide a best- and worst-case scenario for their future sales growth. The span between these scenarios provides a useful measure of uncertainty, since most of these firms routinely use scenario analysis. Bachmann et al. (2020) document that firms find it difficult to express their uncertainty with a probability distribution. Many other surveys also involve researchers directly. For example, the Bundesbank solicits questions from academics for its Online Survey of Consumer Expectations. The Atlanta Fed Survey of Business Uncertainty involves researchers in its survey design. Companies like Vanguard allow researchers to ask questions for a subset of their investors.
2.2 How do survey expectations compare with rational expectations from conventional models?
The conventional agenda has worked hard to come up with models that are successful at generating investor expectations that are consistent with predictability regressions for asset returns. In the data, high ratios of asset valuations relative to fundamentals tend to be followed by low returns on the asset compared with the risk-free rate. For stocks and housing, regressions of excess returns over the next, say, five years on the current price-dividend or price-rent ratio have a negative slope coefficient that is statistically significant. For bonds, the regression is on the difference between the price on a long bond compared with a short bond. Expectations that capture this pattern describe investors who have low return expectations in booms. The reason why assets are highly valued despite this pessimistic outlook is that investors may be less risk averse in asset booms. Alternatively, investors may perceive less risk in booms.
Survey evidence challenges this view. A growing number of papers documents high return expectations in booms. For stocks, Greenwood and Shleifer (2014) provide evidence that investors predict high excess returns on stocks during booms. De la O and Myers (2019) show that high price-dividend ratios are associated with high cash flow expectations in surveys, while expected returns do not change that much over time. In bond markets, Piazzesi et al. (2018) document that forecast errors can account for a substantial component of cyclical movements in bond risk premia. During the postwar period, the Great Inflation is the one episode in which risk premia on long nominal bonds were high.
Similarly, for housing, Case and Shiller (2003) document that households that bought a house at the peak of the housing boom in 2003 were expecting double-digit appreciation rates for houses not only over the next year, but over the next decade. Piazzesi and Schneider (2009) document that there is only a small fraction of households (roughly 10 |$\%$| of all households) that believed it was a good time to buy a house during the early phase of the housing boom (during the years 2000–2003). This fraction doubles during the years 2003–2006. Since there are few housing transactions overall — less than 10 |$\%$| of the housing stock turns over in any given year — a boom in house prices is easily supported by a small fraction of households that are optimistic. The Case-Shiller evidence suggests that these optimistic households select themselves into these few transactions and sustain high valuations.
The conventional wisdom is still dominant. Research that relies on survey answers has to argue that they provide direct evidence about expectations. A question is whether survey respondents are the right people to ask about their expectations — they may not be marginal investors. Some surveys address this issue by focusing on people who recently bought the asset, as does the Case-Shiller survey of recent home buyers. Another question is whether the survey respondents really understand what they are being asked. There has been recent progress on this front by researchers who are involved in the survey design, as I already mentioned. Another important concern is whether survey answers reflect the career concerns of professional forecasters. 10 Finally, surveys may reflect risk-neutral forecasts. Adam et al. (2019) provide evidence against this argument for stocks. These concerns are important because they improve survey design and will lead to better survey evidence in the future.
2.3 Belief heterogeneity
Why do households have heterogeneous expectations? Some differences in beliefs can be explained with informational advantages by certain households. During the recent housing boom in Germany, for example, renters have on average higher rent and house price expectations than owners who severely under-predict these variables ( Kindermann et al. 2020 ). This pattern is consistent with the idea that housing is a unique asset, where non-owners (renters) may have more precise signals about housing dividends (in units of numeraire consumption) than owners of the asset who consume the housing dividend but may not know how much it is worth.
Will we be able to explain all the cross-sectional variation in household expectations? The answer to this question will likely be no. The same observable characteristics (e.g., age, income, and wealth) that have high |$R^2$| s in explaining other choices that households make (e.g., housing tenure) have rather low |$R^2$| s in explaining their expectations.
A more humble approach, which is still very interesting, is to admit that we do not know how households get to their expectations. Successful papers along these lines are Landvoigt (2017) , Lenel (2018) , and Giglio et al. (2019) . Even with a more humble approach, we can describe clusters of people (e.g., Piazzesi and Schneider 2009 ) and study how these clusters evolve over time (e.g., Burnside et al. 2016 ). These approaches may help us to make progress in our understanding of volume in asset markets, which is one of the most important open issues in finance.
2.4 How to use survey beliefs in models?
One way to use survey beliefs as an input into our models is to work with a temporary equilibrium concept (for an introduction, see chapter 3.4 in Piazzesi and Schneider 2016 ). Suppose heterogeneous agents solve dynamic optimization problems given some expectations that may be functions of time |$t$| variables. The outcome of these optimization problems will be an excess demand system for goods and assets at time |$t$| . Equilibrium prices set this system of equations to zero at time |$t$| .
A rational expectations equilibrium is a special case of a temporary equilibrium, that requires the expectations to be consistent with equilibrium dynamics. An alternative approach is to use survey forecasts to discipline expectations. This approach deals with unique episodes, which are reflected in the survey answers. A successful model then matches equilibrium prices and quantities as well as expectational data. An example of such an approach is Landvoigt et al. (2015) , who study the role of credit conditions and expectations during the housing boom of the early 2000s. Another is Leombroni et al. (2020) , who study the role of heterogeneous inflation expectations and inflation uncertainty for house prices and stock prices during the Great Inflation.
The field of finance and asset pricing has the potential to go through a transformational period by enriching other fields of economics and adopting new solution techniques. 11 Four important areas come to mind. First, asset pricing and financial frictions have become the centerpiece of modern monetary and macro economics. Central banks are key drivers of asset prices. This is especially true after the global financial crisis and during the COVID-19 global pandemic. We have witnessed unprecedented central bank activism: Most central banks heavily intervened in asset markets. Nowadays they not only determine the short-term interest rates but also impact many asset prices via quantitative easing programs, active yield curve management, funding for lending programs, and repo programs. Central banks are also not shy to conduct large-scale experiments with the economy, creating an “El Dorado” for empirical researchers. In addition, many interest rates turned negative calling for new fixed income models. Second, safe assets are the focal point of recent debate to understand the low interest rate puzzle and sudden flight-to-safety phenomenon. Safe is not necessarily the same as risk-free (or default risk-free). Third, new quantitative tools, such as neural networks and deep learning algorithms, now enable researchers to numerically solve nonlinear continuous-time macro-finance models with many state variables. These new methods allow us to solve models in which we disaggregate the financial sector and study the impact of various policies and shocks on banks, insurance companies, pension funds, and asset managers separately. Fourth, finance and money are in the middle of a technological revolution. New technology allows us to exploit big data and link various data sources. This makes digital money more attractive than physical cash and mitigates liquidity frictions. Hence, the asset feature of money has become more prominent, and many assets can gain some of the liquidity benefits of money. The main part of these remarks outlines four trends in more detail.
In New Keynesian (NK) macroeconomic models, arguably the dominant school in monetary economics (see, e.g., Woodford 2011 or Galí 2015 for nice textbooks), the key friction is price/wage stickiness. The central equation is the Euler equation, which describes the savings-consumption choice, and the most important price is the risk-free interest rate. In contrast, in macro-finance models, the focus is on financial frictions in form of borrowing frictions like in Kiyotaki and Moore (1997) or Bernanke et al. (1999) or incomplete markets like in Brunnermeier and Sannikov (2014 , 2016) . The latter embeds an intermediate asset pricing model in a macro setting in which resource allocation and economic growth are endogenous. The portfolio choice between a risky asset and safe asset, often in the form of money, is key. The price of risk and risk premia are at least as important as the risk-free rate. Resource allocation and the endogenous growth rate feed back into portfolio choices, the risk-free rate, endogenous volatility, and the price of risk. The term premium contains a risk premium, as do credit spreads — that is, credit spreads reflect risk attitudes as well as expected losses. The risk premium is the product of price of risk times the sum of exogenous and endogenous risk. Endogenous risk is subject to amplification and spirals and can reflect risk-on-risk-off phenomena. In short, the price of risk affects and is affected by the resource allocation and endogenous growth of the economy. All variables interact with monetary policy that tries to affect not only the risk-free rate but also risk premia. The emphasis on endogenously time-varying risk and price of risk, and the portfolio choice, is in sharp contrast to Heterogeneous Agent New Keynesian (HANK) models ( Kaplan et al. 2018 ) in which the risk premium is either zero or constant.
Another future trend that affects asset pricing is the recognition of the special role of safe assets. Brunnermeier and Haddad (2014) stress two important characteristics of a safe asset. First, a safe asset is like a good friend, who is around when you need her. Similarly to a good friend, a safe asset is valuable and liquid whenever you need it, at a random horizon. In contrast, a risk-free asset pays off a fixed amount at a prespecified horizon. Second, there is the “safe asset tautology.” A safe asset is safe because it is perceived as safe. Investors coordinate to fly into certain assets in times of crisis. This points in terms of economic modeling to settings with multiple equilibria and/or bubbles. For example, Swiss government debt is considered as a flight-to-safety asset and appreciates in crisis times, while the Swedish krona typically depreciates in value during crises. In “The I Theory of Money” models, money or government bonds are the safe asset and are a bubble.
Another trend that will change asset pricing relates to new modeling and especially numerical techniques. Neural network techniques will open up a new research avenue in macro-finance. Existing macro-finance models aim to keep the number of state variables that describe the evolution of the dynamical system low. This is especially true for macro-models with nonlinearities due to amplifications and runs since they cannot be solved simply by log-linearizing around the steady state. The limitation to a few state variables has prevented researchers from incorporating the richness of the financial intermediary sector. Typically, the financial sector is summarized by a single sector, even though we know that banks, insurance companies, asset management firms, pension funds, and so on have very different risk exposures across the various risk factors. Using novel deep learning techniques will endow researchers with the tools to fully explore the heterogeneity within the financial sector. Duarte (2018) and Lauriere et al. (2020) provide early advances in this area.
Finally, the fintech revolution will also alter asset pricing research. Big data has the potential to fundamentally reshape our economies, including financial intermediation and payment. So far, studies on payments have only played a role on the sidelines. However, with the recognition that payments deliver valuable data that can feed recommender systems and improve various scores, in particular credit scores, payments are moving to center stage. The current “banking-centric” industrial organization structure of financial activities might be replaced with a “payment-centric” structure. Platforms might be at the center rather than deposit-taking and lending banks. Social networks will play a more important role. Such a shift has important implications for money and also asset pricing. Money will become more digital. So far, bank accounts (inside money) are of course already digital. But only a part of outside money, central bank reserves, is digital, whereas cash is not. Digital money has the advantages that it can be linked to digital platforms, it can be traded automatically using smart contracts, and it is more convenient to use. Hence, as we already see in several countries, cash is losing its importance. Central banks have become increasingly concerned over the potential loss of monetary sovereignty— that is, the power to effectively conduct monetary policy. Among the three roles of money, the unit of account, store of value, and medium of exchange, ensuring that central bank-issued money remains a unit of account is essential for retaining monetary sovereignty. In models with incomplete markets, if debt is primarily denominated in the national currency, monetary policy can redistribute wealth (ex post) and be a risk-sharing tool (ex ante), as, for example in the case of the I Theory of Money. This reduces the price of risk and lowers endogenous risk. If transactions are increasingly conducted in and debt is denominated in new forms of digital currencies, for example those introduced by TechGiants, a process labeled as “digital dollarization” kicks in; see Brunnermeier et al. (2019) . Central banks lose their grip on monetary policy to smooth out the business cycle, and seigniorage revenue fades away. New “Digital Currency Areas” can emerge whose borders are more governed by connections to particular platforms and data regulation rather than national boundaries. For these reasons, central banks are seriously considering introducing their own “digital cash” in the form of Central Bank Digital Currencies (CBDC). Foreseeing the transition of the financial sector is not easy, but it is likely that currencies like Bitcoin and recently Libra will serve as catalysts in the same way Napster did for the music industry about 20 years ago.
This document identifies four trends that are likely to have an impact on research in asset pricing. First, central banks have become important players in asset markets, calling for more studies in which asset prices and monetary policy interact in a meaningful way. Second, flight-to-safety phenomena during a switch from a risk-on to a risk-off mood stress the importance of studying the role of safe assets. Third, new numerical techniques based on deep-learning machine-learning algorithms allow us to solve and estimate macro-finance models that reflect the heterogeneity of the financial intermediary sector. Finally, fintech and big data put digital money and payments at center stage with the emergence of digital currency areas.
A long intellectual history in economics considers the state of the macroeconomy among the most important drivers of asset markets, be these stock markets, bond markets, or housing markets 12 . At the same time, vast literatures in macroeconomics have argued that the aggregate state is itself profoundly influenced by the operations of monetary authorities around the globe. Ultimately, the question of whether and to what extent fluctuations in aggregate economic activity and/or monetary policies matter for asset pricing is an empirical one; thus, addressing it requires an empirical research agenda. As we ponder the exciting paths forward for the field of asset pricing, it is worth reflecting on where we are with this agenda.
On the question of whether macroeconomic risk matters for asset pricing, the evidence is mixed. Some research has found that it does, 13 while other research has found that it does not. 14 Confronted with these conflicting findings, it may be tempting to proceed as if macroeconomic conditions are unimportant for asset pricing. And yet, the stock market appears to react strongly to macroeconomic news (e.g., Boyd et al. 2005 ; Ai and Bansal 2018 ; Baker et al. 2019 ), a global financial crisis from 2007 to 2009 laid bare the important feedback loops between financial markets and the real economy, and it is hard to imagine that the tremendous structural change of the past several decades—slowing growth, rising profit shares, growing inequality, low and declining real interest rates—has not affected the pricing of risky assets.
Regarding monetary policy, there is ample evidence that unanticipated actions and announcements by central banks have important consequences for long-lived asset markets. 15 But surprisingly little attention has been given to understanding how this can occur, when all available evidence suggests that monetary policy shocks have transitory effects on the economy.
4.1 What’s the macroeconomy got to do with it?
There are many possible reasons why evidence cited above might be mixed: our models are gross simplifications of reality; the data are mismeasured and limited; our estimation tools are sometimes restrictive; information sets are unobserved. But one feature that is shared by all of the evidence cited earlier is their representative agent perspective, which presumes that growth in aggregate (average) consumption is an appropriate measure of systematic risk. At least when it comes to the pricing of equity, it is worth remembering how at odds this perspective is with even the most basic facts of stock market ownership. According to the Survey of Consumer Finances, just 52 |$\%$| of households owned equity in any amount or any form in 2016. More significantly, because stock market wealth is so heavily concentrated at the top (the top 5 |$\%$| of the stock wealth distribution owned 76 |$\%$| of the stock market in 2016), participation rates on a wealth-weighted basis are much lower than 52 |$\%$| and trending down since 2004. We might reasonably ask whether the representative agent framework is just too much of an abstraction.
Macro-finance trends also suggest an important role for heterogeneity. Indeed, the ratio of market equity for the corporate sector to three different measures of broad aggregate economic activity has trended up over time and is at or near its postwar high by the end of 2017. By contrast, the ratio of market equity to after-tax profits (earnings) for the sector is not trending up and is not near a postwar high. (See Greenwald et al. 2019 for plots.)
One response to these facts is to revisit an earlier literature that stressed the importance for equity pricing of limited stock market participation and heterogeneity ( Mankiw and Zeldes 1991 ; Vissing-Jørgensen 2002 ; Aït-Sahalia et al. 2004 ; Guvenen 2009 ; Malloy et al. 2009 ). Lettau et al. (2019) (LLM), and Greenwald et al. (2019) (GLL) do so by studying the empirical implications of a heterogeneous agent model characterized by two types of agents and imperfect risk sharing between them: wealth is concentrated in the hands of a few investors, or “shareholders,” while most households are “workers” who finance consumption primarily out of wages and salaries. In contrast to the earlier limited participation/heterogenous agent literature, the results in LLM and GLL suggest the relevance of frameworks in which investors are concerned about shocks that have opposite effects on labor compensation and shareholder payout. Such redistributive shocks play no role in the traditional limited participation/heterogeneous agent literature.
As regards the relevance of representative agent frameworks, LLM find that exposure to growth in the capital share of national income is an important determinant of return premia in the cross-section, while, conditional on this, exposure to aggregate consumption growth is not. GLL focus on understanding the factors that drive the real (adjusted for inflation) level of the stock market over time. During the past 30 years, a time when the market grew precipitously, GLL find that the most important driver of the market has been a string of “factor share shocks” that reallocated the rewards of production without affecting the size of those rewards. The realizations of this shock persistently reallocated rewards to shareholders and away from labor compensation, with no effect on economic growth. Economic growth contributed just 25 |$\%$| to the market’s rise since 1989, which could be compared to the period 1952 to 1988 when economic growth powered the stock market, accounting for more than 100 |$\%$| of its increase. But that 37-year period created less than half the equity wealth generated over the 30 years since 1989. These findings suggest not that the macroeconomy is irrelevant for the stock market, but that distributional shocks may be more imporant than aggregate ones.
Important questions remain. Why have factor shares changed so persistently? Will these trends continue? Do the reasons for the changes matter? (I suspect so.) How are these trends related to the broader trends in wealth and income inequality, in economic growth, and real interest rates?
4.2 The how, why, and whether of monetary policy
If the real values of long-term financial assets respond to the actions and announcements of central banks, the question is why? Asset pricing theories can generally rationalize such large responses only if something related to the conduct of monetary policy will have a persistent influence on real variables. Yet the notion that monetary policy could have long-lived effects on real variables is contravened by an agglomeration of foundational New Keynesian macro theories ( Galí 2015 ), and empirical evidence appears consistent with this ( Christiano et al. 2005 ). But if this is so, how does monetary policy influence long-lived assets?
One possibility is that some components of monetary policy do in fact have long-lasting, first-order effects on the aggregate economy, on short-term real interest rates, and on equity market return premia. Such are the implications of evidence reported in Bianchi et al. (2016) (BLL). BLL solve and estimate a novel New Keynesian framework with two key departures from the prototypical model. The first allows for changes in the conduct of monetary policy that take the form of shifts in the parameters of the nominal interest rate rule. Such changes are conceptually distinct from those generated by a monetary policy shock, an innovation in the policy rate that is uncorrelated with inflation, economic growth, and shifts in the policy rule parameters. The second allows the evolution of beliefs about long-term trend inflation to be potentially influenced by both an adaptive learning component as well as a signal about the central bank’s inflation target, with the belief rule disciplined by observations on survey expectations of inflation over time.
With the estimates in hand, one may identify movements in real variables that are attributable solely to the conduct of monetary policy—that is, to regime changes in the policy rule. These estimates imply that changes in the conduct of monetary policy generate large and persistent fluctuations in the short-term real interest rate that last for decades, in contrast to monetary policy shocks, which have far more transitory effects. The reason is that expectations of inflation are found empirically to be highly adaptive, and as a consequence, the central bank must spend a long time “convincing” households that the policy rule has changed. One interpretation of the evidence on central bank announcements is that these announcements are, in part, noisy signals about the possibility of a regime change in the conduct of monetary policy.
This evidence also speaks to the question of why real interest rates have been declining for decades. Specifically, almost all of the downward drift in the real interest rate since 1980 can be explained by regime changes in the conduct of monetary policy. This happens because the policy rule parameters exhibit a decisive shift toward more hawkish values around the time of Paul Volcker’s appointment to the Federal Reserve, but then exhibit an equally decisive shift back to more dovish values in the aftermath of the near collapse of Long Term Capital Management, the tech bust in the stock market, and the 9/11 terrorist attacks. The conduct of monetary policy has remained dovish since, with the exception of a brief interlude from 2006:Q2 to 2008:Q2. Finally, shifts to a more dovish policy rule are associated with declining equity return premia, consistent with a “reach for yield” in equity markets.
As earlier, important unanswered questions remain. Why does the central bank change the conduct of monetary policy in the first place? One possibility is that it does so in part in reaction to markets ( Cochrane and Piazzesi 2002 , Cieslak and Vissing-Jørgensen 2017 ), leaving us in a circuitous loop. Why is a more dovish monetary policy associated with a decline in equity return premia? The BLL model is silent on the mechanisms that could explain their finding in this regard. As a start, the literature could look to recent intermediary-based frameworks with a banking sector ( Drechsler et al. 2018 , Piazzesi and Schneider 2015 , Piazzesi et al. 2018 ). But we must keep in mind that equities are not the heavily intermediated asset class for which reach-for-yield-type phenomena are typically documented. On the contrary, a significant fraction of the equity market is held by wealthy households and retail investors. To fully understand these findings, we must ultimately account for their role too, whatever that may be.
Most capital invested in financial markets flows through the hands of intermediaries. 16 While there is little disagreement that intermediation frictions have some impact on asset prices, 17 and perhaps in particular during times of financial stress, it remains unclear how much various agency, behavioral, and regulatory frictions matter quantitatively.
Recognizing the potential importance of institutional investors, and motivated by recent asset pricing theories featuring intermediaries, a vibrant empirical literature emerged that tests the Euler condition of a particular group of intermediaries using an empirical proxy for their marginal value of wealth, such as the leverage of broker-dealers. 18
However, by testing the Euler equation of a particular group of investors, we can at best establish that their asset demand curve is correctly specified, but not their importance for asset prices. For instance, we do not learn how asset prices would change if we were to shock the leverage of broker-dealers. To make progress on the central question of how much intermediaries matter for asset prices, we need to impose market clearing and understand the asset demand curves of all investors, that is, the asset demand system.
The modern asset demand system consists of households allocating capital to various intermediaries, such as mutual funds, pension funds, and insurance companies and to financial markets directly. Likewise, intermediaries invest in other intermediaries (for instance, pension funds invest in mutual funds) and allocate capital directly. An important goal of asset pricing is to understand investors’ capital allocation decisions, that is, the asset demand curves of households and intermediaries.
5.1 Intermediary asset pricing and beyond: A demand system approach
To estimate models and to test theories of the asset demand system, it is natural to use data on portfolio holdings. This modeling approach to asset pricing and macroeconomics has its roots in the 1960s and 1970s (see, for instance, the work by Brainard and Tobin 1968 ), and has been revived recently by Koijen and Yogo (2019) (KY19).
A central question is how investors’ demand responds to price changes and to changes in asset characteristics, and how investors substitute across various assets and asset classes. For instance, if an intermediary is forced to liquidate some of its holdings due to a binding constraint, we need to know how much prices have to fall for other intermediaries and households to step in to ensure that markets clear.
Two major obstacles in the earlier asset demand literature that resulted in its long period of hibernation were limited data on portfolio holdings as well a lack of instruments to credibly estimate demand elasticities and cross-elasticities.
The first obstacle has been resolved with the improved disclosure of portfolio holdings by institutions over time in many countries, such as, for instance, the 13F filings in the case of U.S. equities. A quick look at these data reveals several basic facts, as documented in KY19, that appear puzzling in the context of modern portfolio theory. First, institutions hold relatively few stocks. The median institution holds 67 stocks in the period from 2015 to 2017. Second, these choice sets are quite persistent over time, even as prices and asset characteristics change. Third, investors’ portfolio holdings, across stocks, are not well explained by standard characteristics that capture risk and expected returns. The residuals, labeled latent demand, are important drivers of prices 19 , and changes in latent demand are important drivers of returns.
To address the second obstacle, we need an instrumental variable. While the Euler equation approach allowed the asset pricing literature to sidestep thorny identification questions, this is no longer possible if one is interested in estimating the demand system. The identification challenge is no different than in the fields of industrial organization or macro-economics. 20 KY19 propose to exploit the exogenous variation in investment mandates to isolate an exogenous component of demand, but it may be possible to construct other instruments. Identifying demand elasticities is a central goal in this literature.
By taking a demand system perspective to asset pricing, a coherent research agenda emerges. For empiricists, the goal is to credibly estimate demand curves for different investors and to uncover which investor characteristics matter, such as institutional type, size, funding structure, regulatory environment, agency frictions, and informational differences. In estimating demand, it is important to explain both the extensive (that is, explaining the sparse and persistent choice sets of investors) and intensive margin (that is, the determinants of latent demand). Existing theories suggest that latent demand may be related to heterogeneity in beliefs or constraints, and it may be interesting to explore whether observable measures of beliefs (such as those from analysts or surveys) or constraints can explain latent demand.
In terms of asset pricing theory, an important avenue for future research is to develop models that explain which agency, behavioral, or regulatory frictions may give rise to sparse portfolios, low elasticities of demand, and volatile latent demand. By taking a structural approach, portfolio holdings can also be used to directly test these theories and to quantify the importance of various frictions. Part of the research agenda can be decentralized by studying a group of intermediaries in isolation, such as mutual funds or pension funds, if more granular data are available.
In summary, a successful asset pricing model must explain not only prices, but both prices and quantities, including portfolio holdings and flows, 21 as is common practice almost anywhere else in economics. Interestingly, the recent work on demand systems suggests that investors do not behave as our models suggest, and understanding the quantitative importance of such deviations may deliver valuable insights to improve our asset pricing theories. Moreover, by understanding investors’ demand, asset pricing becomes more measurable and tangible, and we can hopefully reduce the “dark matter” in modern asset pricing theories ( Chen et al., 2019 ). Instead of abstractly referring to “arbitrageurs,” “intermediaries,” and “noise traders” in our theories, we actually know who they are, what their asset demand curves look like, how large they are, and what their contribution is to fluctuations in asset prices.
5.2 Broader implications
The benefits of developing an asset pricing model that is consistent with prices and quantities extends beyond the academic curiosity of asset pricing researchers. Indeed, many of the salient policy and regulatory questions involve quantities: What is the impact of large-scale asset purchases by central banks? What would happen to credit spreads if a large fraction of BBB bonds are downgraded? What is the impact of growing environmental, social, and governance (ESG) mandates on asset prices? What is the impact of changing the risk regulation of banks or insurance companies? The recent COVID-19 crisis has highlighted once more the importance of being able to answer these questions quantitatively.
Understanding the asset demand system is essential to provide credible answers to these questions. Elasticities and cross-elasticities are often not targeted directly in modern asset pricing models, yet these models are used to address these questions involving large changes in portfolio holdings.
Furthermore, the asset demand of investors depends on firms’ characteristics such as payout policy, leverage, investment and innovation policies, and profitability as they capture growth expectations and the riskiness of future cash flows. By combining models of the asset demand system with models of corporate decision making, 22 we obtain an integrated model of asset pricing and corporate finance that should target to explain asset prices, investors’ portfolio holdings, macro quantities, and firms’ corporate policies. 23
Obviously, this research agenda using asset demand systems only just (re)started. But given the wealth of data on portfolio holdings that is available across countries and asset classes, there is a lot of scope to make progress on this key question in asset pricing using a demand system approach.
Intermediary asset pricing (IAP) seeks to understand the role of financial intermediaries in explaining fluctuations in asset prices. 24 It shifts the focus from a household’s Euler equation, as in consumption-based approaches to asset pricing, to the pricing condition of a trader in a financial intermediary. In so doing, IAP elevates factors such as regulatory and corporate financing considerations that affect financial intermediaries. The big questions in this research agenda are: How much do intermediaries matter for asset prices? In which markets are these effects most pronounced? In which states of the world are the effects most pronounced? What are the central underlying factors driving intermediaries’ effects on asset prices, and how do they vary across types of intermediaries?
IAP should be seen as a branch of heterogeneous agent approaches to asset pricing ( Constantinides and Duffie 1996 ; Heaton and Lucas 1996 ). In these models, all households are on their consumption Euler equations. However, households differ in their preferences and endowment risks. As a result, factors such as the cross-sectional distribution of household income shocks and the wealth distribution drive asset market returns.
In IAP, the financial investments of some households are directed through intermediaries into asset markets. The trader at the intermediary is on her investment Euler equation, while the household who delegates investment to this trader may or may not share this Euler equation. Other households that directly invest in asset markets are on their Euler equations. The action in IAP theory is about the wedge between the Euler equations of the household that delegates and the trader at the intermediary.
Let me turn to corporate finance. A financial intermediary is a firm, with decisions made by the workers (management, traders, etc.) at the firm, accountable to the firm’s shareholders, and raising financing from equity and debt holders. An additional stakeholder, the government, looms large and affects decisions in many financial firms.
If the Modigliani and Miller (1958) propositions apply to this firm, then the intermediary is but a veil and the IAP model collapses back to the heterogeneous agent asset pricing model that focuses on the delegating households. If, however, Modigliani-Miller fails, then the separation between ownership and control has relevance for asset prices. The content of the IAP model is in the specification of how Modigliani-Miller fails. Asset demand from intermediaries then is a function of these frictions. In general equilibrium, asset demand from intermediaries plus asset demand from the direct-investing households clear the asset market.
There have been a number of well-developed specifications that trace the failure of Modigliani-Miller, borrowing from corporate finance theory, to equilibrium asset prices. I will mention two. He and Krishnamurthy (2013) develop an agency-theoretic model where the trader at an intermediary must be provided incentives when making trading decisions. This type of model shows that information frictions, such as what may arise when the trader is responsible for complex trading strategies or what may worsen during turbulent periods, will affect intermediary asset demand and equilibrium asset prices. In the agency-theoretic model, the stake of the insider (management, trader) can alleviate information frictions; thus, metrics that track this stake—for example, past returns of the firm - will affect asset prices. The agency-theoretic model also gives rise to a constraint on raising outside equity finance since such finance may dilute the stake of the insider. Thus, we can understand why equity capital and regulatory capital may affect asset demands. Andersen et al. (2019) develop a model where debt-overhang distorts the investment decision of the trader at a financial intermediary. The trader makes decisions to maximize value to shareholders, but given debt-overhang, such decisions differ from the frictionless benchmark. They show that traders under debt-overhang require a minimum return, roughly equal to the financial firm’s credit spread, to purchase assets. Their approach allows one to understand the high returns on even near riskless trades in the last decade, and the comovement between such returns and financial firms’ credit spreads. In this model, the debt-overhang friction reduces the private incentive to raise equity capital. Thus, the model also speaks to why regulatory capital requirements can affect asset demand and prices.
I view this theoretical research as a work in progress. Opening the box of the financial firm suggests that many other considerations may matter for the trading decisions of the firm. Capital allocation within firms, career concerns of traders, search for yield, and benchmarking effects all seem like considerations that may be of importance. Sorting out which of these are first-order, and in which asset markets and states-of-the-world, is the research agenda that needs to be completed.
There is by now ample evidence that Modigliani-Miller fails for financial firms, and this failure meaningfully affects asset prices (see He and Krishnamurthy 2018 for a review of the evidence). Yet there is heterogeneity within intermediaries. We need to understand better the impact of these failures on different intermediary types and in different asset markets. Commercial banks are players in loan markets, bond markets, and derivatives markets. They are financed largely by deposits, some of which is subject to government insurance and regulatory constraints. Broker/dealers are active in derivatives and market-making activities across a broad array of asset markets. They are financed in short-term funding markets including wholesale money markets and repo markets. Hedge funds engage in complex trading strategies across a range of asset markets. They are financed by insiders’ wealth, outside equity capital, and repo. Placing structure on the “asset demand” functions across these different types of intermediaries, as in the approach taken by Koijen and Yogo (2019) , can shed light on the underlying factors driving trading at these intermediaries. This too is an important research agenda, and will draw on tools from asset pricing, industrial organization, and corporate finance.
Finally, I will mention a parallel stream of research to IAP in macroeconomics. There is a large literature that studies how intermediation frictions affect credit extension, via loan supply/loan rates, and then through such channels affect aggregate investment and consumption. See Brunnermeier et al. (2011) for a survey. If intermediaries are an important driver of asset prices, then we should expect that they will also affect macro quantities. This observation has two further implications. On the theoretical side, the consumption of the direct investing household I alluded to earlier will also be affected by intermediary frictions. This point is often lost in models with exogenously specified agent endowments, as is typical in asset pricing models. On the empirical side, the data studied by macroeconomists offer further moments for intermediary asset pricing models to match. Thus, connecting IAP and macroeconomics is another important avenue in this research agenda.
Twenty years ago, international finance and finance were far apart from each other. 25 Over the past 20 years, a remarkable convergence took place. Will these two fields share a common path in the future, or will they diverge in order to meet specific challenges? I will use this question to organize my thoughts on the future of international finance.
Despite these fundamental similarities, there are differences of emphasis between the two fields. First, heterogeneity is more central to international finance than it is to finance. Heterogeneity simply cannot be avoided in international finance. In some sense, international finance starts with two countries and two investors. Second, frictions are more central to international finance than they are to finance. Indeed, and despite decades of international financial integration, there are many more frictions in financial markets across countries than within countries. Third, currencies are more central to international finance than they are to finance. It does seem to make a big difference that assets are traded in one currency or another. Fourth, the role of governments is more central to international finance than it is to finance. Governments interfere in many different ways in the trading of assets across countries, more so than within countries.
Turning to the future, it is clear that there are both common and specific long-standing problems that are likely to occupy the two fields in the coming decades. Common long-standing problems include the identification of the economic determinants of beliefs |$\mathbb{E}_{t}$| , the economic determinants of risk premia or equivalently of stochastic discount factors |$X_{t,t+1}$| and |$X_{t,t+1}^{*}$| , the economic determinants of portfolios. They also include what I will call the “disconnect” problem: the fact that it seems difficult to connect the stochastic discount factor |$X_{t,t+1}$| to an actual preference-based marginal rate of substitution |$MRS_{t,t+1}$| of a well-identified marginal investor between periods |$t$| and |$t+1$| , or the real exchange rate |$E_{t}P_{F,t}^{*}/P_{H,t}$| to the actual preference-based marginal rates of substitution |$MRS_{F,H,t}$| between foreign goods |$F$| and home goods |$H$| . 26 Other common long-standing problems include the identification of the key market failures and externalities (fire sales from financing constraints, aggregate demand externalities from nominal rigidities, search externalities, etc.) as well as the role and transmission of policy (monetary, fiscal, prudential, etc.). 27
Third, there is the large degree of home bias in portfolios across countries. Fourth, there are the destabilizing effects of volatile capital flows in emerging markets. Fifth, there are the economic determinants of government behavior in these countries. Sixth, there are the economic determinants and implications of exchange rate regimes (fixed exchange rates, floats, managed floats, etc.). 30 Seventh and finally, there are the importance of the international monetary system and the special role of the United States as the preeminent issuer of safe and liquid reserve assets, its associated role as the world banker and the exorbitant privilege that comes with it, and the resulting pattern of global imbalances. 31 How long will the dollar continue to dominate as a reserve and invoicing currency? How long will the United States keep playing a dominant role as a world lender of last resort through its network of swap lines? When will we transition to a more multipolar world? Will that transmission be smooth or turbulent? Will the supreme reign of the dollar come to an end in a Triffiin event similar to that which brought the end of the Bretton Woods system of fixed exchange rates? 32
The two fields are actively tackling these challenges. In doing so, they sometimes take a common approach to deviate from the first and simplest models that rationalized the fundamental equations. For example, both fields now make ample room for market segmentation; recognize the role of financial intermediaries; incorporate financial constraints, illiquidity, and runs; try to capture irrationality and speculation; and allow for inattention. 33 International finance also sometimes breaks away from finance to address some of the central problems that are specific to the field. For example, it makes more room for nominal rigidities and political economy frictions such as limited commitment and the like. A question that arises, as the two fields deviate from the simple unifying paradigms of the fundamental equations, is whether they will splinter into a collection of local explanations to local questions, or whether new and richer global paradigms will emerge. Another is whether the two fields gravitate toward similar local and global theories. It seems that they would probably have a better shot at the truth if they did.
It seems clear that both fields will benefit from the arrival of large quality micro-datasets. It is a safe bet that it will force the fields to recognize the importance of heterogeneity and frictions even more so than they do today. In international finance, new data already allows us to grasp a new reality on disaggregated capital flows, portfolios, and balance sheets, and to recognize the importance of financial derivatives. 34 Similarly, we are beginning to gain an understanding of the heterogeneity and dynamics of beliefs. 35 And we are also making progress on the nature of nominal rigidities using data on disaggregated prices. 36 Similar trends are at work in finance. In the two fields, reduced-form empiricism will be a temptation, and the question is whether new and better structural theories will emerge to explain all this data.
I explore how recent advances in asset pricing have contributed to our understanding of exchange rates, and I outline promising areas for future research, using the United States’ role in the international financial system as a test case. 37
8.1 Exchange rate valuation and decomposition
The exchange rate level reflects a cash flow component, the interest rate differences, and a discount rate component, the currency risk premia. All else equal, an increase in the foreign country’s interest rates will cause the foreign currency to strengthen, that is, to appreciate against the dollar, but an increase in the currency risk premium will cause the currency to depreciate.
Importantly, real interest rate differences across countries are quite persistent. Some countries have persistently low interest rates, and other countries have persistently high interest rates ( Lustig et al. 2011 ; Hassan and Mano 2019 ). According to the high interest rate currencies also need to have high currency risk premia, so that the persistent component of the interest rate differences is (partly) offset by the persistent component of the currency risk premia, if real exchange rates are to be stationary. In other words, Switzerland and Japan have to convince global bond market investors to accept negative currency risk premia to keep their currency from weakening, while Australia and New Zealand need large and positive currency risk premia to keep their currency from strengthening.
This implication of the exchange rate valuation model is borne out by the data. Investors earn an unconditional currency carry trade risk premium by going long in currencies that have high interest rates on average, even if they do not condition on current interest rates ( Lustig et al. 2011 ; Hassan and Mano 2019 ). This is often referred to as the unconditional version of the currency carry trade. In equilibrium, investors have to believe that long positions in low interest rate currencies, such as the Swiss franc and the Japanese yen, offer insurance against aggregate risk that is priced in global securities markets, while high interest rate currencies expose their portfolios to more global risk.
High interest rate currencies depreciate in bad states of the world. Lustig et al. (2011) find that high interest rate currencies depreciate when volatility in global stock markets increases. 38
8.2 The role of the United States in the international financial system
In the typical carry trade pattern of global capital flows, the United States looks like an outlier. The United States is an example of a country with low real interest rates that has been running persistent current account deficits. The United States has accumulated a large, negative net foreign asset position against the rest of the world as a result. The composition of the United States balance sheet against the rest of the world is unusual. The United States borrows by issuing Treasury bonds and other safe assets, and then the United States takes a long position in risky foreign assets. As a result, the United States manages to make money on its negative net foreign assets position. This has been referred to as the “exorbitant privilege” of the United States.
The insurance view. In the neoclassical complete markets benchmark, the exceptional role of the United States has a natural interpretation. The United States is the world’s disaster insurance provider. The United States insures the rest of the world against adverse shocks, as is argued by Gourinchas and Rey (2007) and Gourinchas et al. (2010) . In equilibrium, the least risk-averse investor, in this case the United States, insures other investors against adverse shocks. When disaster strikes, the insurance contract calls for large net transfers from the United States to the rest of the world. To generate these transfers, the dollar depreciates in real terms. 39
The insurance view of the United States role in the international financial system faces two main challenges. I will use the great financial crisis (GFC) of 2007–2008 to highlight these challenges. First, it is not at all clear that there were large net transfers from the United States to the rest of the world during the GFC. There was a striking collapse of global trade during the GFC. Any cross-country transfers that did occur were probably smaller than what would be predicted by the model. Second, the dollar tends to appreciate in the case of large adverse shocks to the global economy. This is exactly what happened during the GFC.
The safe asset view. In the safe asset view, the United States is different because it is the sole supplier of the world’s safe assets, and the dollar is the world’s reserve currency ( Farhi and Maggiori 2018 ; Caballero et al. 2008 ; Gopinath and Stein 2018 ; Caballero and Krishnamurthy 2008 ).
Part of the discount rate component in the exchange rate valuation component has been relabeled as a convenience yield term. An increase in the convenience yield |$\lambda^{\%,\ast}_{t+\tau}$| that foreign investors derive from their holding of dollar-denominated safe assets, relative to the same yield on foreign safe assets |$\lambda^{\ast,\ast}_{t+\tau}$| , causes the dollar to appreciate instantaneously. This prediction has empirical support. The variation in the U.S. Treasury basis has explanatory power for the dollar exchange rate; other bond bases do not have the same explanatory power for other bilateral exchange rates. Part of the discount rate component we measured earlier is now recast as a convenience yield component.
Jiang et al. (2018) find that these extra convenience yields foreign investors earn when holding Treasury bonds are larger than 2 |$\%$| per annum. More than 90 |$\%$| of this convenience yield is attributable to the dollar exposure, not the safety of Treasury bonds, consistent with recent evidence that international bond investors seem to be subject to dollar bias ( Maggiori et al. 2018 ).
This safe asset model has radically different implications from the benchmark model. When the world economy experiences an adverse shock, the flight-to-safety will tend to cause the dollar to appreciate. During the onset of the great financial recession in 2008, the dollar appreciated by 30 |$\%$| , and the United States generated a larger dollar amount of seignorage revenue from the sale of Treasury bonds and other safe assets. In case of a global crisis, there is a net transfer from the rest of the world to the United States.
In this safe asset model, international capital flows can obviously be destabilizing. For example, safe asset demand creates an incentive to produce more dollar-denominated safe assets in the United States, potentially giving rise to excessive leverage in the United States in the run-up to the GFC. In other countries, especially emerging market countries, issuers have an incentive to issue bonds denominated in dollars. This in turn gives rise to currency mismatch. Much more empirical work in the coming years is needed to explore and test the implications of these two different paradigms, particularly their implications for exchange rates.
This article summarizes the perspectives of Markus Brunnermeier, Emmanuel Farhi, Ralph S. J. Koijen, Arvind Krishnamurthy, Sydney C. Ludvigson, Hanno Lustig, Stefan Nagel, and Monika Piazzesi. Each subsection reflects the view of one of the authors only and this will be indicated at the beginning of each subsection.
1 The introduction is by Ralph S.J. Koijen and Sydney C. Ludvigson.
2 This subsection is the perspective of Stefan Nagel.
3 See, e.g., Timmermann (1993) , Lewellen and Shanken (2002) , Collin-Dufresne et al. (2016) .
4 Lochstoer and Muir (2019) show that subjective volatility perceptions can explain a number of asset pricing puzzles.
5 Giglio et al. (2019) is a recent example of work that looks at this question.
6 See, e.g., D’Acunto et al. (2019) for recent research of this kind.
7 Collin-Dufresne et al. (2017) , Nagel and Xu (2019) , and Wachter and Kahana (2019) are recent examples of such research.
8 As an example, work by Kozlowski et al. (2020) suggests that beliefs about disasters could play a role.
9 This subsection is the perspective of Monika Piazzesi.
10 An attractive feature of the Survey of Professional Forecasters conducted by the Philadelphia Federal Reserve is that survey respondents are anonymous, which reduces the importance of career concerns. Bluechip survey respondents are not anonymous but are serving a wide range of clients who may be either long or short in fixed income assets, which also mitigates these concerns.
11 This subsection is the perspective of Markus Brunnermeier.
12 This subsection is the perspective of Sydney C. Ludvigson.
13 Chen et al. (1986) , Lettau and Ludvigson (2001) , Parker and Julliard (2004) , Koijen et al. (2017) , Bansal et al. (2016) , and Ghosh et al. (2016) .
14 Breeden et al. (1989) , Campbell and Mei (1993) , Lewellen and Nagel (2006) , Roussanov (2014) , and Herskovic et al. (2019) .
15 Hanson and Stein (2015) , Gertler and Karadi (2015) , Boyarchenko et al. (2016) , Jarocinski and Karadi (2020) , Cieslak and Schrimpf (2019) , and Kekre and Lenel (2019) .
16 This subsection is the perspective of Ralph S.J. Koijen.
17 The recent work on covered interest parity deviations provides an example ( Du et al., 2018 ).
18 See, for instance, He and Krishnamurthy (2013) , Adrien et al. (2014) , and He et al. (2016) .
19 Koijen et al. (2019) study how much the demand of various investors, differentiated by type, size, and activeness, matters for equity valuations and long-horizon expected returns.
20 For recent advances on identification in macroeconomics, see Nakamura and Steinsson (2018) and Gabaix and Koijen (2020a) .
21 See Gabaix and Koijen (2020b) for a dynamic model of flows, quantities, and asset prices to understand the volatility of movements in the aggregate stock market.
22 A complementary literature develops heterogeneous agent asset pricing models with both an intermediary and a household sector as well as various corporate finance policies; see, for instance, Elenev et al. (2018) . Due to computational limitations, such models can currently only handle a limited amount of heterogeneity, a small number of assets, and the models are calibrated rather than estimated. However, this literature is developing rapidly, and advances in machine learning may enable researchers to estimate larger-scale models in the near future.
23 See, for instance, Baker and Wurgler (2004) .
24 This subsection is the perspective of Arvind Krishnamurthy.
25 This subsection is the perspective of Emmanuel Farhi.
26 See, e.g., Itskhoki and Mukhin (2017) and Lilley et al. (2019) ,
27 See, e.g., Jeanne and Korinek (2010) , Bianchi and Mendoza (2010) , Bianchi (2011) , and Farhi and Werning (2016) .
28 See, e.g., Itskhoki and Mukhin (2017) .
29 See, e.g., Du et al. (2018) .
30 See, e.g., Ilzetzki et al. (2017) .
31 See, e.g., Gourinchas and Rey (2007) , Caballero et al. (2008) , and Gourinchas et al. (2010) .
32 See, e.g., Farhi and Maggiori (2018) , Gopinath and Stein (2018) , He et al. (2019) , and Gourinchas et al. (2019) .
33 See, e.g., Gabaix and Maggiori (2015) and Itskhoki and Mukhin (2017) .
34 See, e.g., Maggiori et al. (2018) and Coppola et al. (2020) .
35 See, e.g., Giglio et al. (2019) .
36 See, e.g., Gopinath et al. (2020) .
37 This subsection is the perspective of Hanno Lustig.
38 Menkhoff et al. (2012) find that baskets of high interest rate currencies depreciate when global FX volatility increases. Lettau et al. (2014) find that the downside betas of high interest rate currencies are higher.
39 Maggiori (2017) reinterprets this insurance arrangement by imputing a central role to financial intermediaries. In Maggiori’s model, United States’ intermediaries are better equipped to hedge against adverse shocks than their foreign counterparts. In this risk-sharing arrangement, the United States can run large and persistent current account deficits in anticipation of surpluses during rare disasters. Similarly, Chien and Naknoi (2015) develop a model with heterogeneous agents in which the United States has a larger mass of sophisticated traders than the foreign countries. Their model has similar predictions.
Adam, K. , Matveev D. , and Nagel S. . 2019 . Do survey expectations of stock returns reflect risk-adjustments? Working Paper, The University of Chicago .
Adrien, T. , Etula E. , and Muir T. . 2014 . Financial intermediaries and the cross-section of asset returns . Journal of Finance 69 : 2557 – 96 .
Google Scholar
Ai, H. and R. Bansal. 2018 . Risk preferences and the macroeconomic announcement premium . Econometrica 86 : 1383 – 1430 .
Aït-Sahalia, Y. , Parker J. A. , and Yogo M. . 2004 . Luxury goods and the equity premium . Journal of Finance 59 : 2959 – 3004 .
Andersen, L. , Duffie D. , and Song Y. . 2019 . Funding value adjustments . Journal of Finance 74 : 145 – 92 .
Bachmann, R. , Carstensen K. , Lautenbacher S. , and Schneider M. . 2019 . Uncertainty and change: Survey evidence of firm’s subjective beliefs . Working Paper, Stanford University .
Bachmann, R. , Carstensen K. , Lautenbacher S. , and Schneider M. . 2020 . Uncertainty is more than risk . Working Paper, Stanford University .
Baker, M. and Wurgler J. . 2004 . A catering theory of dividends . Journal of Finance 59 : 1125 – 65 .
Baker, S. , Bloom N. , Davis S. J. , and Sammon M. . 2019 . What triggers stock market jumps? Unpublished manuscript, Stanford University .
Bansal, R. , Kiku D. , and Yaron A. . 2016 . Risks for the long run: Estimation with time aggregation . Journal of Monetary Economics 82 : 52 – 69 .
Bernanke, B. S. , Gertler M. , and Gilchrist S. . 1999 . The financial accelerator in a quantitative business cycle framework . Handbook of Macroeconomics 1 : 1341 – 93 .
Bianchi, F. , Lettau M. , and Ludvigson S. C. . 2016 . Monetary policy and asset valuation . http://www.econ.nyu.edu/user/ludvigsons/reg.pdf .
Bianchi, J. 2011 . Overborrowing and systemic externalities in the business cycle . American Economic Review 101 : 3400 – 26 .
Bianchi, J. and Mendoza. E. G. 2010 . Overborrowing, financial crises and ‘macro-prudential’ taxes . NBER Working Paper 16091 .
Boyarchenko, N. , Haddad V. , and Plosser M. C. . 2016 . The federal reserve and market confidence . Working Paper, Federal Reserve Bank of New York .
Boyd, J. H. , Hu J. , and Jagannathan R. . 2005 . The stock market’s reaction to unemployment news: Why bad news is usually good for stocks . Journal of Finance 60 : 649 – 72 .
Brainard, W. C. , and Tobin J. . 1968 . Pitfalls in financial model building . American Economic Review: Papers and Proceedings 58 : 99 – 122 .
Breeden, D. , Gibbons M. , and Litzenberger R. . 1989 . Empirical tests of the consumption-oriented CAPM . Journal of Finance 44 : 231 – 62 .
Brunnermeier, M. , and Sannikov Y. . 2014 . A macroeconomic model with a financial sector . American Economic Review 104 : 379 – 421 .
Brunnermeier, M. K. , Eisenbach T. M. , and Sannikov Y. . 2011 . Macroeconomics with financial frictions: A survey . In Advances in Economics and Econometrics: Tenth World Congress, Volume II: Applied Economics , pp. 3 – 94 . Cambridge University Press .
Google Preview
Brunnermeier, M. K. , and Haddad V. . 2014 . Safe assets . Working Paper, Princeton University .
Brunnermeier, M. K. , James H. , and Landau J.-P. . 2019 . The digitalization of money . Working Paper, Princeton University .
Brunnermeier, M. K. , and Sannikov Y. . 2016 . On the optimal inflation rate . American Economic Review 106 : 484 – 89 .
Burnside, C. , Eichenbaum M. , and Rebelo S. . 2016 . Understanding booms and busts in housing markets . Journal of Political Economy 124 : 1088 – 1147 .
Caballero, R. , and Simsek A. . 2020 . A risk-centric model of demand recessions and speculation . Quarterly Journal of Economics 135 : 1493 – 1566 .
Caballero, R. J. , Farhi E. , and Gourinchas P.-O. . 2008 . An equilibrium model of global imbalances and low interest rates . American Economic Review 98 : 358 – 93 .
Caballero, R. J. , and Krishnamurthy A. . 2008 . Collective risk management in a flight to quality episode . Journal of Finance 63 : 2195 – 2230 .
Campbell, J. Y. , and Clarida R. H. . 1987 . The dollar and real interest rates . Carnegie-Rochester Conference Series on Public Policy 27 : 103 – 139 .
Campbell, J. Y. , and Mei J. . 1993 . Where do betas come from? asset price dynamics and the sources of systematic risk . Review of Financial Studies 6 : 567 – 92 .
Case, K. , and Shiller R. . 2003 . Is there a bubble in the housing market? Brookings Papers on Economic Activity 34 : 299 – 362 .
Chen, H. , Dou W. , and Kogan L. . 2019 . Measuring “dark matter” in asset pricing models . Working Paper, Massachusetts Institute of Technology .
Chen, N.-F. , Roll R. , and Ross S. A. . 1986 . Economic forces and the stock market . Journal of Business 59 : 383 – 403 .
Chien, Y. , and Naknoi K. . 2015 . The risk premium and long-run global imbalances . Journal of Monetary Economics 76 : 299 – 315 .
Christiano, L. J. , Eichenbaum M. , and Evans C. L. . 2005 . Nominal rigidities and the dynamic effects of a shock to monetary policy . Journal of Political Economy 113 : 1 – 45 .
Cieslak, A. , and Schrimpf A. . 2019 . Non-monetary news in central bank communication . Journal of International Economics 118 : 293 – 315 .
Cieslak, A. , and Vissing-Jørgensen A. . 2017 . The economics of the fed put . Working Paper, Duke University .
Clarida, R. , and Gali J. . 1994 . Sources of real exchange-rate fluctuations: How important are nominal shocks? Carnegie-Rochester Conference Series on Public Policy 41 : 1 – 56 .
Cochrane, J. H. 2017 . Macro-finance . Review of Finance 21 : 945 – 85 .
Cochrane, J. H. , and Piazzesi M. . 2002 . The fed and interest rates-a high-frequency identification . AEA Papers and Proceedings 92 : 90 – 5 .
Collin-Dufresne, P. , Johannes M. , and Lochstoer L. A. . 2016 . Parameter learning in general equilibrium: The asset pricing implications . American Economic Review 106 : 664 – 98 .
Collin-Dufresne, P. , Johannes M. , and Lochstoer L. A. . 2017 . Asset pricing when `this time is different’ . Review of Financial Studies 30 : 505 – 35 .
Constantinides, G. M. , and Duffie D. . 1996 . Asset pricing with heterogeneous consumers . Journal of Political Economy 104 : 219 – 40 .
Coppola, A. , Maggiori M. , Neiman B. , and Schreger J. . 2020 . Redrawing the Map of Global Capital Flows: The Role of Cross-Border Financing and Tax Havens} . Working Paper, Harvard University .
D’Acunto, F. , Hoang D. , Paloviita M. , and Weber M. . 2019 . Iq, expectations, and choice . Working Paper, Boston College .
De la O, R. , and Myers S. . 2019 . Subjective cash flow and discount rate expectations . Working Paper, Stanford University .
Drechsler, I. , Savov A. , and Schnabl P. . 2018 . A model of monetary policy and risk premia . Journal of Finance 71 : 317 – 373 .
Du, W. , and Schreger J. . 2016 . Local currency sovereign risk . Journal Finance 71 : 1027 – 1070 .
Du, W. , Tepper A. , and Verdelhan A. . 2018 . Deviations from covered interest rate parity . Journal of Finance 73 : 915 – 957 .
Duarte, V. 2018 . Machine learning for continuous-time economics .
Elenev, V. , Landvoigt T. , and Van Nieuwerburgh S. . 2018 . A macroeconomic model with financially constrained producers and intermediaries . Working Paper, Johns Hopkins Carey Business School .
Farhi, E. , and Maggiori M. . 2018 . A model of the international monetary system . Quarterly Journal of Economics 133 : 295 – 355 .
Farhi, E. , and Werning I. . 2016 . A theory of macroprudential policies in the presence of nominal rigidities . Econometrica 84 : 1645 – 1704 .
Froot, K. A. , and Ramadorai T. . 2005 . Currency returns, intrinsic value, and institutional-investor flows . Journal of Finance 60 : 1535 – 66 .
Gabaix, X. , and Koijen R. S. . 2020a . Granular instrumental variables . Working Paper, Harvard University .
Gabaix, X. , and Koijen R. S. . 2020b . In search of the origins of financial fluctuations: The inelastic markets hypothesis . Working Paper, Harvard University .
Gabaix, X. , and Maggiori M. . 2015 . International liquidity and exchange rate dynamics . Quarterly Journal of Economics 130 : 1369 – 1420 .
Galí, J. 2015 . Monetary policy, inflation, and the business cycle: An introduction to the new Keynesian framework and its applications . Princeton, NJ : Princeton University Press .
Gertler, M. , and Karadi P. . 2015 . Monetary policy surprises, credit costs, and economic activity . American Economic Journal: Macroeconomics 7 : 44 – 76 .
Ghosh, A. , Julliard C. , and Taylor A. P. . 2016 . What is the consumption-capm missing? an information-theoretic framework for the analysis of asset pricing models . Review of Financial Studies 30 : 442 – 504 .
Giglio, S. , Maggiori M. , Stroebel J. , and Utkus S. . 2019 . Five facts about beliefs and portfolios . Working Paper, Yale University .
Gilchrist, S. , López-Salido D. , and Zakrajšek E. . 2015 . Monetary policy and real borrowing costs at the zero lower bound . American Economic Journal: Macroeconomics 7 : 77 – 109 .
Gopinath, G. , Boz E. , Casas C. , Dà ez F. J. , Gourinchas P.-O. , and Plagborg-Møller M. . 2020 . Dominant currency paradigm . American Economic Review 110 : 677 – 719 .
Gopinath, G. , and Stein J. C. . 2018 . Banking, trade, and the making of a dominant currency . Working Paper, Harvard University .
Gourinchas, P.-O. , and Rey H. . 2007 . From world banker to venture capitalists: Us external adjustment and exhorbitant privilege . In Clarida R. (Ed.), G-7 Current Account Imbalances: Sustainability and Adjustment , pp. 11 – 55 . Chicago : North Holland .
Gourinchas, P.-O. , Rey H. , and Govillot N. . 2010 . Exorbitant privilege and exorbitant duty . Working Paper, University of California at Berkeley .
Gourinchas, P.-O. , Rey H. , and Sauzet M. . 2019 . The international monetary and financial system . Working Paper, University of California at Berkeley .
Graham, J. R. , and Harvey C. R. . 2011 . The duke/cfo business outlook survey . Duke CFO Global Business Outlook . www.cfosurvey.org .
Greenwald, D. , Lettau M. , and Ludvigson S. C. . 2019 . How the wealth was won: Factors shares as market fundamentals . National Bureau of Economic Research Working Paper No. 25769 .
Greenwood, R. , and Shleifer A. . 2014 . Expectations of returns and expected returns . Review of Financial Studies 27 : 714 – 46 .
Guvenen, M. F. 2009 . A parsimonious macroeconomic model for asset pricing . Econometrica 77 : 1711 – 40 .
Hanson, S. G. , and Stein J. C. . 2015 . Monetary policy and long-term real rates . Journal of Financial Economics 115 : 429 – 48 .
Hassan, T. A. , and Mano R. C. . 2019 . Forward and spot exchange rates in a multi-currency world . Quarterly Journal of Economics 134 : 397 – 450 .
He, Z. , Kelly B. , and Manela A. . 2016 . Intermediary asset pricing: New evidence from many asset classes . Journal of Financial Economics 126 : 1 – 35 .
He, Z. ,and A. Krishnamurthy. 2013 . Intermediary asset pricing . American Economic Review 103 : 732 – 70 .
He, Z. , and Krishnamurthy A. . 2018 . Intermediary asset pricing and the financial crisis . Annual Review of Financial Economics 10 : 173 – 197 .
He, Z. , Krishnamurthy A. , and Milbradt K. . 2019 . A model of safe asset determination . American Economic Review 109 : 1230 – 62 .
Heaton, J. , and Lucas D. . 1996 . Evaluating the effects of incomplete markets on risk sharing and asset pricing . Journal of Political Economy 104 : 443 – 87 .
Herskovic, B. , Moreira A. , and Muir T. . 2019 . Hedging risk factors . Working Paper, UCLA .
Ilzetzki, E. , Reinhart C. M. , and Rogoff K. S. . 2017 . Exchange Arrangements Entering the 21st Century: Which Anchor Will Hold? Working Paper, London School of Economics .
Itskhoki, O. , and Mukhin D. . 2017 . Exchange rate disconnect in general equilibrium . Working Paper, Princeton University .
Jarocinski, M. , and Karadi P. . 2020 . Deconstructing monetary policy surprises: the role of information shocks . American Economic Journal: Macroeconomics 12 : 1 – 43 .
Jeanne, O. , and Korinek A. . 2010 . Excessive Volatility in Capital Flows: A Pigouvian Taxation Approach . American Economic Review 100 : 403 – 407 .
Jiang, Z. , Krishnamurthy A. , and Lustig H. N. . 2018 . Foreign safe asset demand and the dollar exchange rate . Working Paper, Stanford University .
Kaplan, G. , Moll B. , and Violante G. L. . 2018 . Monetary policy according to hank . American Economic Review 108 : 697 – 743 .
Kekre, R. , and Lenel M. . 2019 . Monetary policy, redistribution, and risk premia . Working Paper, The University of Chicago .
Kindermann, F. , Blanc J. L. , Piazzesi M. , and Schneider M. . 2020 . Learning about housing cost: Survey evidence from the german house price boom . Working Paper, Stanford University .
Kiyotaki, N. , and Moore J. . 1997 . Credit cycles . Journal of Political Economy 105 .
Koijen, R. S. , Lustig H. , and Van Nieuwerburgh S. . 2017 . The cross-section and time series of stock and bond returns . Journal of Monetary Economics 88 : 50 – 69 .
Koijen, R. S. , Richmond R. J. , and Yogo M. . 2019 . Which investors matter for equity valuations and expected returns? Working Paper, University of Chicago .
Koijen, R. S. J. , and Yogo M. . 2019 . A demand system approach to asset pricing . Journal of Political Economy 127 : 1475 – 1515 .
Kozlowski, J. , Venkateswaran V. , and Veldkamp L. . 2020 . The tail that wags the economy: Belief-driven business cycles and persistent stagnation . Journal of Political Economy 128 : 2839 – 2879 .
Landvoigt, T. 2017 . Housing demand during the boom: The role of expectations and credit constraints . Review of Financial Studies 30 : 1865 – 1902 .
Landvoigt, T. , Piazzesi M. , and Schneider M. . 2015 . The housing market(s) of san diego . American Economic Review 105 : 1371 – 1407 .
Lauriere, M. , Merkel S. , and Payne J. . 2020 . Solving heterogeneous agent macroeconomic models using neural nets . Mimeo .
Lenel, M. 2018 . Safe assets, collateralized lending and monetary policy . Working Paper, Princeton University .
Leombroni, M. , Piazzesi M. , Rogers C. , and Schneider M. . 2020 . Inflation and the price of real assets . Working Paper, Stanford University .
Lettau, M. , and Ludvigson S. C. . 2001 . Resurrecting the (C)CAPM: A cross-sectional test when risk premia are time-varying . Journal of Political Economy 109 : 1238 – 87 .
Lettau, M. , Ludvigson S. C. , and Ma S. . 2019 . Capital share risk in u.s. asset pricing . Journal of Finance 74 : 1753 – 92 .
Lettau, M. , Maggiori M. , and Weber M. . 2014 . Conditional risk premia in currency markets and other asset classes . Journal of Financial Economics 114 : 197 – 225 .
Lewellen, J. , and Shanken J. . 2002 . Learning, asset-pricing tests, and market efficiency . Journal of Finance 57 : 1113 – 45 .
Lewellen, J. W. , and Nagel S. . 2006 . The conditional capm does not explain asset pricing anomalies . Journal of Financial Economics 82 : 289 – 314 .
Lilley, A. , Maggiori M. , Neiman B. , and Schreger J. . 2019 . Exchange rate reconnect . Working Paper, Harvard University .
Lochstoer, L. A. , and Muir T. . 2019 . Volatility expectations and returns . Working Paper, UCLA .
Lucas, R. E. 1978 . Asset prices in an exchange economy . Econometrica 46 : 1429 – 45 .
Lustig, H. , Roussanov N. , and Verdelhan A. . 2011 . Common risk factors in currency markets . Review of Financial Studies 24 : 3731 – 77 .
Maggiori, M. 2017 . Financial intermediation, international risk sharing, and reserve currencies . American Economic Review 107 : 3038 – 71 .
Maggiori, M. , Neiman B. , and Schreger J. . 2018 . International currencies and capital allocation . Working Paper, Harvard University .
Malloy, C. J. , Moskowitz T. J. , and Vissing-Jørgensen A. . 2009 . Long-run stockholder consumption risk and asset returns . Journal of Finance 64 : 2427 – 79 .
Manela, A. , and Moreira A. . 2017 . News implied volatility and disaster concerns . Journal of Financial Economics 123 : 137 – 62 .
Mankiw, N. G. , and Zeldes S. P. . 1991 . The consumption of stockholders and nonstockholders . Journal of Financial Economics 29 : 97 – 112 .
Manski, C. F. 2018 . Survey measurement of probabilistic macroeconomic expectations: Progress and promise . NBER Macroeconomics Annual 32 : 411 – 471 .
Martin, I. , and Nagel S. . 2019 . Market efficiency in the age of big data . Working Paper, University of Chicago .
Menkhoff, L. , Sarno L. , Schmeling M. , and Schrimpf A. . 2012 . Carry trades and global foreign exchange volatility . Journal of Finance 67 : 681 – 718 .
Modigliani, F. , and Miller M. H. . 1958 . The cost of capital, corporation finance and the theory of investment . American Economic Review 48 : 261 – 97 .
Nagel, S. , and Xu Z. . 2019 . Asset pricing with fading memory . Working Paper, University of Chicago .
Nakamura, E. , and Steinsson J. . 2018 . Identification in macroeconomics . Journal of Economic Perspectives 32 : 59 – 86 .
Parker, J. , and Julliard C. . 2004 . Ultimate consumption risk and the cross-section of expected returns . Journal of Political Economy 113 : 185 – 222 .
Piazzesi, M. , Rogers C. , and Schneider M. . 2018 . Money and banking in a new keynesian model . Working Paper, Stanford University .
Piazzesi, M. , Salomao J. , and Schneider M. . 2018 . Trend and cycle in bond premia . Working Paper, Stanford University .
Piazzesi, M. , and Schneider M. . 2009 . Momentum traders in the housing market: Survey evidence and a search model . American Economic Review 99 : 406 – 411 .
Piazzesi, M. , and Schneider M. . 2015 . Payments, credit and asset prices . Unpublished manuscript, Stanford University .
Piazzesi, M. , and Schneider M. . 2016 . Housing and macroeconomics . Handbook of Macroeconomics , John B. Taylor and Uhlig Harald (ed.), edition 1, volume 2 , chapter 19, Elsevier , 1547 – 1640 .
Roussanov, N. 2014 . Composition of wealth, conditioning information, and the cross-section of stock returns . Journal of Financial Economics 111 : 352 – 380 .
Timmermann, A. G. 1993 . How learning in financial markets generates excess volatility and predictability in stock prices . Quarterly Journal of Economics 108 : 1135 – 1145 .
Vissing-Jørgensen, A. 2002 . Limited asset market participation and intertemporal substitution . Journal of Political Economy 110 : 825 – 853 .
Wachter, J. A. , and Kahana M. J. . 2019 . A retrieved-context theory of financial decisions .
Woodford, M. 2011 . Interest and prices: Foundations of a theory of monetary policy . Princeton University Press .
Email alerts
Companion article.
- Editor’s Note: Introducing the Review Article “Perspectives on the Future of Asset Pricing”
Citing articles via
- Recommend to your Library
Affiliations
- Online ISSN 1465-7368
- Print ISSN 0893-9454
- Copyright © 2024 Society for Financial Studies
- About Oxford Academic
- Publish journals with us
- University press partners
- What we publish
- New features
- Open access
- Institutional account management
- Rights and permissions
- Get help with access
- Accessibility
- Advertising
- Media enquiries
- Oxford University Press
- Oxford Languages
- University of Oxford
Oxford University Press is a department of the University of Oxford. It furthers the University's objective of excellence in research, scholarship, and education by publishing worldwide
- Copyright © 2024 Oxford University Press
- Cookie settings
- Cookie policy
- Privacy policy
- Legal notice
This Feature Is Available To Subscribers Only
Sign In or Create an Account
This PDF is available to Subscribers Only
For full access to this pdf, sign in to an existing account, or purchase an annual subscription.
- Help & FAQ
A review of capital asset pricing models
- Econometrics & Business Statistics
Research output : Contribution to journal › Article › Research › peer-review
Purpose – The main aspect of security analysis is its valuation through a relationship between the security return and the associated risk. The purpose of this paper is to review the traditional capital asset pricing model (CAPM) and its variants adopted in empirical investigations of asset pricing. Design/methodology/approach – Pricing models are discussed under five categories: the single-factor model, multifactor models, CAPM with higher order systematic co-moments, CAPM conditional on market movements and time-varying volatility models. Findings – The paper finds that the last half-century has witnessed the proliferation of empirical studies testing on the validity of the CAPM. A growing number of studies find that the cross-asset variation in expected returns cannot be explained by the systematic risk alone. Therefore a variety of models have been developed to predict asset returns. Research limitations/implications – There is no consensus in the literature as to what a suitable measure of risk is, and consequently, as to what is a suitable measure for evaluating risk-adjusted performance. So the quest for robust asset pricing models continues. Originality/value – From its beginning to its possible demise the paper reviews the history of the CAPM assuring that we are all up to speed with what has been done.
- Capital asset pricing model
- Financial risk
Access to Document
- 10.1108/03074350710779269
Other files and links
- Link to publication in Scopus
T1 - A review of capital asset pricing models
AU - Galagedera, Don U.A.
PY - 2007/9/4
Y1 - 2007/9/4
N2 - Purpose – The main aspect of security analysis is its valuation through a relationship between the security return and the associated risk. The purpose of this paper is to review the traditional capital asset pricing model (CAPM) and its variants adopted in empirical investigations of asset pricing. Design/methodology/approach – Pricing models are discussed under five categories: the single-factor model, multifactor models, CAPM with higher order systematic co-moments, CAPM conditional on market movements and time-varying volatility models. Findings – The paper finds that the last half-century has witnessed the proliferation of empirical studies testing on the validity of the CAPM. A growing number of studies find that the cross-asset variation in expected returns cannot be explained by the systematic risk alone. Therefore a variety of models have been developed to predict asset returns. Research limitations/implications – There is no consensus in the literature as to what a suitable measure of risk is, and consequently, as to what is a suitable measure for evaluating risk-adjusted performance. So the quest for robust asset pricing models continues. Originality/value – From its beginning to its possible demise the paper reviews the history of the CAPM assuring that we are all up to speed with what has been done.
AB - Purpose – The main aspect of security analysis is its valuation through a relationship between the security return and the associated risk. The purpose of this paper is to review the traditional capital asset pricing model (CAPM) and its variants adopted in empirical investigations of asset pricing. Design/methodology/approach – Pricing models are discussed under five categories: the single-factor model, multifactor models, CAPM with higher order systematic co-moments, CAPM conditional on market movements and time-varying volatility models. Findings – The paper finds that the last half-century has witnessed the proliferation of empirical studies testing on the validity of the CAPM. A growing number of studies find that the cross-asset variation in expected returns cannot be explained by the systematic risk alone. Therefore a variety of models have been developed to predict asset returns. Research limitations/implications – There is no consensus in the literature as to what a suitable measure of risk is, and consequently, as to what is a suitable measure for evaluating risk-adjusted performance. So the quest for robust asset pricing models continues. Originality/value – From its beginning to its possible demise the paper reviews the history of the CAPM assuring that we are all up to speed with what has been done.
KW - Capital asset pricing model
KW - Financial risk
KW - Securities
UR - http://www.scopus.com/inward/record.url?scp=85015699953&partnerID=8YFLogxK
U2 - 10.1108/03074350710779269
DO - 10.1108/03074350710779269
M3 - Article
SN - 0307-4358
JO - Managerial Finance
JF - Managerial Finance
Academia.edu no longer supports Internet Explorer.
To browse Academia.edu and the wider internet faster and more securely, please take a few seconds to upgrade your browser .
Enter the email address you signed up with and we'll email you a reset link.
- We're Hiring!
- Help Center
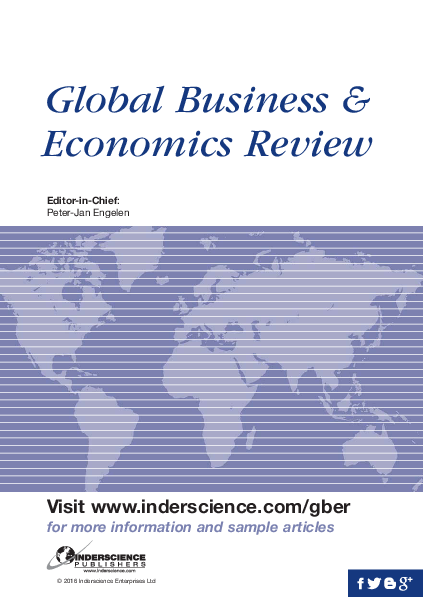
The capital asset pricing model: a critical literature review

What is the relationship between the risk and expected return of an investment? The capital asset pricing model (CAPM) provides an initial framework for answering this question. The CAPM (Sharpe, 1964; Lintner, 1965) marks the birth of asset pricing theory. This model is based on the idea that not all risk should affect asset prices. The model thus provides insight into the kind of risk that is related to return. Four decades later, the CAPM is still widely used in applications. The CAPM provides a methodology for translating risk into estimates of expected ROE. Its application continues to generate debate: many scholars argued that the CAPM is based on unrealistic assumptions. This paper lays out the key ideas of the CAPM, the history of empirical work on the CAPM and the implications of this work on the shortcomings of the CAPM.
Related Papers
International Journal of Economics and Finance
Mona Elbannan
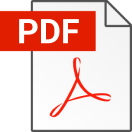
Managerial Finance
Maria Mumtaz
PurposeThe main aspect of security analysis is its valuation through a relationship between the security return and the associated risk. The purpose of this paper is to review the traditional capital asset pricing model (CAPM) and its variants adopted in empirical investigations of asset pricing.Design/methodology/approachPricing models are discussed under five categories: the single‐factor model, multifactor models, CAPM with higher order systematic co‐moments, CAPM conditional on market movements and time‐varying volatility models.FindingsThe paper finds that the last half‐century has witnessed the proliferation of empirical studies testing on the validity of the CAPM. A growing number of studies find that the cross‐asset variation in expected returns cannot be explained by the systematic risk alone. Therefore a variety of models have been developed to predict asset returns.Research limitations/implicationsThere is no consensus in the literature as to what a suitable measure of ri...
Xuezhang Hua
Jibran Sheikh
The capital asset pricing model (CAPM) states that assets are priced commensurate with a trade-off between undiversifiable risk and expectations of return. The model underpins the status of academic finance, as well as the belief that asset pricing is an appropriate subject for economic study. Notwithstanding, our findings imply that in adhering to the CAPM, we are choosing to encounter the market on our own terms of rationality, rather than the market's.
Monirul Hossain
Ashley Olson
Journal of Financial Research
Najabat Ali
This research study attempts to explain the risk and returns of Capital asset pricing model in stocks and investments scenario. In this present study, the model (CAPM) is validated both theoretically and empirically subsequently. Capital asset pricing model is one of the tools for investors to take a decision regarding their investments in various situations by offering risk adjusted returns. This paper creates a concrete underlying building blocks for an investor keeping in view the Modern Portfolio Theory and also the risk and return considerations. Beta is used to prioritize the understanding of different available funds in the market, fund strategies and also to identify instruments for the investors to diversify portfolios of investments. Finally readers would get the idea of how much exactly the investor would get return in order to put his investment in a riskier asset management company instead of a sovereign entity i.e. bank. This paper provides a general investment opportunities for investors not only in order to quantify the risk but also explaining the best risk adjusted returns into estimates of equities expected return.
Leonie Banton
The purpose of this paper is to test the Capital Asset Pricing Model on individual stocks included in the Financial Times Stock Exchange 100 Index (FTSE 100 index) for the period 2004-2014. Both unconditional and conditional CAPM models are used. Also an unconditional CAPM model will be used to determine if the stocks in the FTSE 100 index are correctly priced during the period of study. This research also shows how the financial crisis of 2008 and the behaviour of market participants may impact the CAPM. Based on the evidence from this research, the CAPM could not be validated using the conditional model and the unconditional model for the full periods (2004 -2014). However, the CAPM was validated for the sub-group: the industrials sector of the full period (2004- 2014) and the post-crisis period (2009-2014) using the unconditional CAPM. It was seen that there was a greater proportion of under-priced stocks than over-priced stocks for all test periods and that the financial crisis of 2008 and the behaviour of the participants in the capital market and had an impact on the CAPM testing for the period of the study. Specifically, the financial crisis of 2008 affected sectors of the economy differently. It was concluded that CAPM is affected by the test period, the model used and the sector of the stocks but it still has some merits. Therefore, improvements to the model should include other measures of systematic risk other than beta.
LJubisa Stamatovic
In making an investment in an instrument, investors need to know how much risk they are taking. They have to assess whether the instrument is properly priced, or whether they are getting sufficient returns for the chance they are taking. The CAPM can be defined as 'a mathematical model that seeks to explain the relationship between risk and return in a rational equilibrium market' and ' an economic model for valuing stocks, securities, derivatives and/or assets by relating risk and expected return'. For this study, CAPM is tested using a basket of 20 randomly selected stocks from the Dow Jones Industrial index, a U.S. stock market index consisting of 30 large, industrial companies. The coverage period is from January 1997 to December 2006, which is prior to the credit crisis that engulfed the financial world back in 2007, to enable us to take a much deeper look at the CAPM. Inclusion of the post-crisis data could distort the resulting models, consequently leading to ...
RELATED PAPERS
Journal of American Studies
Malcolm McLaughlin
Revista de las Cortes Generales
pablo garcía mexía
Eero P Simoncelli
Journal of the Korean Geotechnical Society
In-hyun Nam
… : Revista de la …
carolina alonso
Journal of Evolution of Medical and Dental Sciences
Roshny Jacob
Psychological Reports
Marloes van Engen
Polymer Science Series B
Vadim Irzhak
Comércio, Consumo & Governança Urbana
MARINA GABOLEIRO CARREIRAS
Research, Society and Development
victoria cavalari
ABCD. Arquivos Brasileiros de Cirurgia Digestiva (São Paulo)
İsa Özaydın
44th AIAA Plasmadynamics and Lasers Conference
Raffaele Pepe
British Journal of Sports Medicine
Britt Elin Øiestad
IFIP Advances in Information and Communication Technology
Giulia Bruno
Latin American Journal of Trade Policy
Niall Meagher
Perspectivas Revista de Ciencias Sociales
Sandra Liliana Pinzón
Robert Maxson
Nucleic Acids Research
Ruy.anconetani Anconetani
Linguistics and Literature Studies
Chilingaryan Kamo
Psychology in the Schools
Scott Martin
The Laryngoscope
Rusty Jennings
Eighth IFAC Symposium on Advances in Control Education (2009)
Miguel Mañanas
Ukashatu Abubakar
Yongfei Yang
RELATED TOPICS
- We're Hiring!
- Help Center
- Find new research papers in:
- Health Sciences
- Earth Sciences
- Cognitive Science
- Mathematics
- Computer Science
- Academia ©2024
A literature review of new methods in empirical asset pricing: omitted-variable and errors-in-variable bias
- Published: 09 June 2020
- Volume 35 , pages 77–100, ( 2021 )
Cite this article
- Solène Collot ORCID: orcid.org/0000-0003-4359-0357 1 &
- Tobias Hemauer 1
2056 Accesses
6 Citations
3 Altmetric
Explore all metrics
Standard procedures in empirical asset pricing suffer from various issues that are common to all regression-based methods. This work reviews recently introduced approaches that aim to mitigate problems associated with omitted factors and errors-in-variables. New methods addressing the omitted-variable bias suggest procedures for selecting appropriate control variables, aggregating the information from a large set of factors, or making existing methods robust against omitted factors. While the omitted-variable problem is present in almost all standard empirical asset pricing methods, the errors-in-variables problem is largely limited to the estimation of factor premia via two-pass regressions. New methods addressing the errors-in-variable bias implement an instrumental variable approach, suggest a generalized version of the widely used portfolio sorts procedure, or correct estimates based on an analytic expression for the bias. Ultimately, all of these new methods represent highly relevant advances for the area of empirical asset pricing, and the possibility to synthesize the most promising approaches might be worthwhile to investigate in the future.
This is a preview of subscription content, log in via an institution to check access.
Access this article
Price includes VAT (Russian Federation)
Instant access to the full article PDF.
Rent this article via DeepDyve
Institutional subscriptions
Similar content being viewed by others
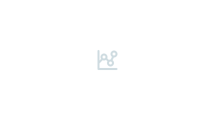
Diversification and portfolio theory: a review
Gilles Boevi Koumou
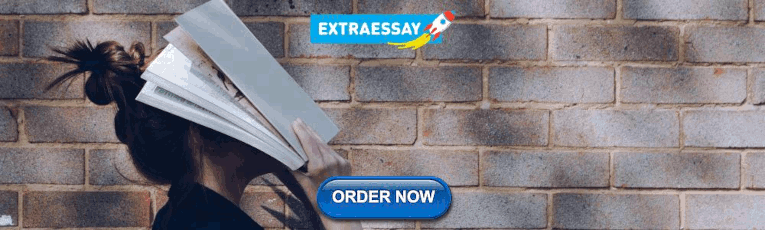
Factor investing and asset allocation strategies: a comparison of factor versus sector optimization
Wolfgang Bessler, Georgi Taushanov & Dominik Wolff
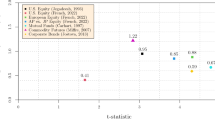
Momentum: what do we know 30 years after Jegadeesh and Titman’s seminal paper?
Tobias Wiest
Harvey et al. ( 2016 ) list 316 factors and Hou et al. ( 2019 ) even 452 factors that have been suggested as potential factors for the explanation and prediction of expected stock returns.
Note that this expression for the SDF assumes the test assets to be excess returns and the intercept of the SDF to be normalized to \(1 + \sum _{j=1}^k b_{j} E(f_{j,t})\) .
Note that selecting the full set of factors as controls is not feasible due to potential in-sample overfitting and the curse of dimensionality.
Acharya, V.V., Pedersen, L.H.: Asset pricing with liquidity risk. J. Financ. Econ. 77 , 375–410 (2005)
Article Google Scholar
Barillas, F., Shanken, J.: Comparing asset pricing models. J. Finance 73 , 715–754 (2018)
Belloni, A., Chernozhukov, V., Hansen, C.: Inference on treatment effects after selection among high-dimensional controls. Rev. Econ. Stud. 81 , 608–650 (2014)
Benjamini, Y., Hochberg, Y.: Controlling the false discovery rate: a practical and powerful approach to multiple testing. J. R. Stat. Soc.: Ser. B (Methodol.) 57 , 289–300 (1995)
Google Scholar
Berk, J.B., Green, R.C., Naik, V.: Optimal investment, growth options, and security returns. J. Finance 54 , 1553–1607 (1999)
Black, F., Jensen, M.C., Scholes, M.: The capital asset pricing model: some empirical tests. In: Studies in the Theory of Capital Markets, pp. 79–124. Praeger Publishers, New York (1972)
Carhart, M.M.: On persistence in mutual fund performance. J. Finance 52 , 57–82 (1997)
Carlson, M., Fisher, A., Giammarino, R.: Corporate investment and asset pricing dynamics: implications for the cross-section of returns. J. Finance 59 , 2577–2603 (2004)
Carlson, M., Fisher, A., Giammarino, R.: Corporate investment and asset price dynamics: implications for SEO event studies and long-run performance. J. Finance 61 , 1009–1034 (2006)
Chen, R., Kan, R.: Finite sample analysis of two-pass cross-sectional regressions. In: Working Paper (2005)
Chordia, T., Goyal, A., Shanken, J.: Cross-sectional asset pricing with individual stocks: betas versus characteristics. In: Working Paper (2017)
Cochrane, J.H.: Asset Pricing. Princeton University Press, Princeton (2005)
Cochrane, J.H.: Presidential address: discount rates. J. Finance 66 , 1047–1108 (2011)
Cooper, I., Ma, L., Maio, P., Philip, D.: New factor models and the APT. In: Working Paper (2019)
Fama, E.F., French, K.R.: Common risk factors in the returns on stocks and bonds. J. Financ. Econ. 33 , 3–56 (1993)
Fama, E.F., French, K.R.: Size and book-to-market factors in earnings and returns. J. Finance 50 , 131–155 (1995)
Fama, E.F., French, K.R.: Multifactor explanations of asset pricing anomalies. J. Finance 51 , 55–84 (1996)
Fama, E.F., French, K.R.: A five-factor asset pricing model. J. Financ. Econ. 116 , 1–22 (2015)
Fama, E.F., MacBeth, J.D.: Risk, return, and equilibrium: empirical tests. J. Polit. Econ. 81 , 607–636 (1973)
Feng, G., Giglio, S., Xiu, D.: Taming the factor zoo: a test of new factors. J. Finance (2020)
Gagliardini, P., Ossola, E., Scaillet, O.: A diagnostic criterion for approximate factor structure. J. Econom. 212 , 503–521 (2019)
Gibbons, M.R.: Multivariate tests of financial models: a new approach. J. Financ. Econ. 10 , 3–27 (1982)
Giglio, S., Liao, Y., Xiu, D.: Thousands of alpha tests. Chicago Booth Research Paper No. 18–09 (2019)
Giglio, S., Xiu, D.: Asset Pricing with Omitted Factors. Chicago Booth Research Paper No. 16–21 (2019)
Goyal, A.: Empirical cross-sectional asset pricing: a survey. Fin. Mark. Portf. Mgmt. 26 , 3–38 (2012)
Gu, S., Kelly, B., Xiu, D.: Empirical Asset Pricing via Machine Learning. Chicago Booth Research Paper No. 18–04 (2019)
Han, Y., He, A., Rapach, D.E., Zhou, G.: Firm characteristics and expected stock returns. In: Working Paper (2019)
Hansen, L.P.: Large sample properties of generalized method of moments estimators. Econometrica 50 , 1029–1054 (1982)
Harvey, C.R., Liu, Y., Zhu, H.:. and the cross-section of expected returns. Rev. Financ. Stud. 29 , 5–68 (2016)
He, Z., Kelly, B., Manela, A.: Intermediary asset pricing: new evidence from many asset classes. J. Financ. Econ. 126 , 1–35 (2017)
Hoechle, D., Schmid, M., Zimmermann, H.: Does unobservable heterogeneity matter for portfolio-based asset pricing tests? In: Working Paper (2019)
Hou, K., Xue, C., Zhang, L.: Digesting anomalies: an investment approach. Rev. Financ. Stud. 28 , 650–705 (2015)
Hou, K., Xue, C., Zhang, L.: Replicating anomalies. Rev. Financ. Stud. (2019)
Huang, D., Li, J., Zhou, G.: Shrinking factor dimension: a reduced-rank approach. In: Working Paper (2019)
Jagannathan, R., Wang, Z.: Empirical evaluation of asset-pricing models: a comparison of the SDF and beta methods. J. Finance 57 , 2337–2367 (2002)
Jegadeesh, N., Noh, J., Pukthuanthong, K., Roll, R., Wang, J.: Empirical tests of asset pricing models with individual assets: resolving the errors-in-variables bias in risk premium estimation. J. Financ. Econ. 133 , 273–298 (2019)
Kamstra, M.J.: Momentum, reversals, and other puzzles in fama–macbeth cross-sectional regressions. In: Working Paper (2017)
Kazemi, M.M.: Semi-parametric estimation of factor risk premia. In: Working Paper (2019)
Kim, S., Skoulakis, G.: Ex-post risk premia estimation and asset pricing tests using large cross sections: the regression-calibration approach. J. Econom. 204 , 159–188 (2018)
Kozak, S., Nagel, S., Santosh, S.: Shrinking the cross-section. J. Financ. Econ. (2019)
Lettau, M., Pelger, M.: Estimating latent asset-pricing factors. In: Working Paper (2018)
Lettau, M., Pelger, M.: Factors that fit the time series and cross-section of stock returns. In: Working Paper (2019)
Light, N., Maslov, D., Rytchkov, O.: Aggregation of information about the cross section of stock returns: a latent variable approach. Rev. Financ. Stud. 30 , 1339–1381 (2017)
Litzenberger, R.H., Ramaswamy, K.: The effect of personal taxes and dividends on capital asset prices: theory and empirical evidence. J. Financ. Econ. 7 , 163–195 (1979)
Merton, R.C.: An intertemporal capital asset pricing model. Econometrica 41 , 867–887 (1973)
Novy-Marx, R.: The other side of value: the gross profitability premium. J. Financ. Econ. 108 , 1–28 (2013)
Ross, S.A.: The arbitrage theory of capital asset pricing. J. Econ. Theory 13 , 341–360 (1976)
Shanken, J.: On the estimation of beta-pricing models. Rev. Financ. Stud. 5 , 1–35 (1992)
Shanken, J., Zhou, G.: Estimating and testing beta pricing models: alternative methods and their performance in simulations. J. Financ. Econ. 84 , 40–86 (2007)
Song, Y., Zhao, Q.: Performance evaluation with latent factors. In: Working Paper (2018)
Zhang, L.: The value premium. J. Finance 60 , 67–103 (2005)
Download references
Acknowledgements
The authors thank Manuel Ammann, Markus Schmid, and participants at a PhD seminar at the University of St. Gallen as well as an anonymous referee for helpful comments and suggestions.
Author information
Authors and affiliations.
School of Finance, University of St. Gallen, St. Gallen, Switzerland
Solène Collot & Tobias Hemauer
You can also search for this author in PubMed Google Scholar
Corresponding author
Correspondence to Solène Collot .
Additional information
Publisher's note.
Springer Nature remains neutral with regard to jurisdictional claims in published maps and institutional affiliations.
Rights and permissions
Reprints and permissions
About this article
Collot, S., Hemauer, T. A literature review of new methods in empirical asset pricing: omitted-variable and errors-in-variable bias. Financ Mark Portf Manag 35 , 77–100 (2021). https://doi.org/10.1007/s11408-020-00358-0
Download citation
Published : 09 June 2020
Issue Date : March 2021
DOI : https://doi.org/10.1007/s11408-020-00358-0
Share this article
Anyone you share the following link with will be able to read this content:
Sorry, a shareable link is not currently available for this article.
Provided by the Springer Nature SharedIt content-sharing initiative
- Empirical asset pricing
- Omitted factors
- Errors-in-variables
- Factor models
- Fama–MacBeth regressions
- Portfolio sorts
JEL Classification
- Find a journal
- Publish with us
- Track your research
Asset pricing in global scenario: a bibliometric analysis
IIM Ranchi Journal of Management Studies
ISSN : 2754-0138
Article publication date: 16 August 2022
Issue publication date: 7 February 2023
This study aims to organise and present the development of asset pricing models in the international environment. The stock market integration and cross-listing lead us to another objective of bibliometric analysis for “International Asset Pricing” to provide a complete overview and give scope and directions for future research.
Design/methodology/approach
Web of Science database is used to search with “International Asset Pricing.” Of 3,438 articles, 2,487 articles are selected for the final bibliometric analysis. Various research such as citation analysis, keyword analysis, author’s and corresponding author's analysis have been conducted.
The bibliometric analysis finds that the USA comes out to be the country where the maximum research was conducted on the topic. The keyword analysis was also analysed to evaluate the significant areas of the research. Risk, return and international asset pricing are the most frequently used keywords. The year 2020 has the maximum number of published research articles and citations due to the change in the market structure worldwide and the effect of Covid-19 across the world.
Originality/value
The present paper provides the collection, classification and comprehensive analysis of “International Asset pricing,” which may help the academicians, researchers and practitioners for future research for the relevant subject area.
Bibliometric analysis
- International asset pricing
- Conditional CAPM
- Arbitrage pricing theory
- Portfolio theory
Keshari, A. and Gautam, A. (2023), "Asset pricing in global scenario: a bibliometric analysis", IIM Ranchi Journal of Management Studies , Vol. 2 No. 1, pp. 48-69. https://doi.org/10.1108/IRJMS-02-2022-0025
Emerald Publishing Limited
Copyright © 2022, Aditya Keshari and Amit Gautam
Published in IIM Ranchi Journal of Management Studies . Published by Emerald Publishing Limited. This article is published under the Creative Commons Attribution (CC BY 4.0) licence. Anyone may reproduce, distribute, translate and create derivative works of this article (for both commercial and non-commercial purposes), subject to full attribution to the original publication and authors. The full terms of this licence may be seen at http://creativecommons.org/licences/by/4.0/legalcode
Introduction
Financial market integration of stock markets worldwide appears to be a key and critical aspect of economic development. Every country across the globe depends on one another for its success ( Pirinsky & Wang, 2011 ). The contemporary period is of globalisation, and the removal of international barriers resulted in the seamless movement of capital and trade. Economic ties have grown stronger as the world has evolved into a global community. The financial market structure of every country has a significant impact on determining asset pricing. It is crucial to business profitability and the stability and expansion of the financial markets. The entire value of a company's outstanding shares is used to calculate the country's stock market capitalisation. Multiply the stock market price by the number of shares outstanding to get a company's market capitalisation ( Ray, 2012 ). Market capitalisation is an essential factor to consider while deciding the asset pricing strategy to be followed, as it aids in selecting the appropriate valuation method ( Ali Khrawish, Zakaria Siam, & Jaradat, 2010 ). Various research have been conducted on asset pricing ( Christensen & Feltham, 2009 ; Connor & Sehgal, 2001 ; Fama & French, 2015 ; Greenwood, Hanson, & Liao, 2018 ; Guasoni & Wong, 2020 ; Karolyi & Stulz, 2002 ; Khudoykulov, 2020 ; Krause, 2001 ; Patro, 2001 ; Ranjan Dash & Mahakud, 2013 ; Sehgal & Tripathi, 2006 ; Sehrawat, Kumar, Nigam, Singh, & Goyal, 2020 ; Stulz, 1981 ; Taneja, 2010 ) in domestic as well in an international setting, but still there is a lot problem at the time of selecting the suitable model for the study. The current research focuses on the three aspects of asset pricing. The first is the development of various asset pricing models over time. The second aspect is crucial, which deals with the theoretical background of the model “how these models perform in domestic and international settings.” The third aspect, which is also the most important, focuses on the bibliometric analysis of 2,487 research articles from 2001 to 2021. The study focused on the area where more research is needed in international asset pricing.
Justification of the study
Asset pricing emerged as a well-versed and predominant area in corporate finance and stock analysis; asset-pricing models have been a vital area of research in finance ( Khudoykulov, 2020 ). The underlying worth of an asset must be distinguished from the market price of that asset. As described by Adam Smith, the natural price can be used to identify the fundamental value, and it defines the genuine price as providing a reasonable profit to the owner ( Krause, 2001 ).
Various researchers have looked into multiple aspects of asset pricing theory, supplementing the field with better risk-return explanations like the efficient market hypothesis ( Fama, 1970 ), arbitrage pricing theory (APT) ( Roll & Ross, 1980 ) and the inter-temporal capital asset pricing model (CAPM; Merton, 1973 ). Raei et al. (2011) in his paper discussed the development of consumption-based CAPM ( Breeden, Gibbons & Litzenberger, 2016 ; Chen, 2003 ; Darrat, Li & Park, 2011 ), downside-CAPM ( Bawa & Lindenberg, 1977 ; Harlow & Rao, 1989 ), adjusted CAPM where liquidity risk estimated in investor's Beta ( Amihud & Mendelson, 1989 ; Pástor & Stambaugh, 2000 ; Acharya & Pedersen, 2005 ), revised CAPM in which Beta is revised including financial and operating leverage ( Rahnamaie Roodposhti et al. , 2009 ; Moradian et al. , 2010 ), consumption CAPM in which Beta is explained in terms of growing consumption in the market ( Iqbal & Brooks, 2007 ). The international asset pricing model (IAPM) has been popularised in recent years due to its innovative implementations. Firstly, it helps the firms to cross-list their assets in a relatively segmented market which helps in integrating the international capital markets. Secondly, firms with non-tradable assets indirectly benefit from global integration in terms of lower cost of capital and higher asset prices, without incurring any additional cost due to the international pricing spillover effect. As a result, “International Asset Pricing” was chosen as a significant aspect, and further analysis will provide new dimensions in the field.
What is the pattern of publication and citations during these years?
Who are the most influential authors in the field of asset pricing in terms of the number of publications and citations?
Which asset pricing articles are most cited in the journals?
What are the main dimensions of changes occurred in the international asset pricing during these periods?
Which are the most prominent keywords used in the articles and areas which are less explored in this period?
Conceptual development of model
Several authors have studied asset pricing in a national and international setting, including the research of Bai and Green (2020 ), Buckberg (1995 ), De Santis and Gerard (1997 ), Harshita et al. (2015) , Harvey (1991 ), Musawa et al. (2020) , Singh and Yadav (2015) , Wang et al. (2013) in which they talked about the importance of using the international version of CAPM for valuing the asset pricing models. Researcher's also focussed on the market structure of economies, whether the world market is segmented or integrated ( Errunza & Miller, 2016 ; Errunza, Losq, & Padmanabhan, 1992 ; El Hedi Arouri, Rault, Sova, Sova, & Teulon, 2013 ; Patro, 2001 ; Pirinsky & Wang, 2011 ; Stulz, 1995 ; Thomadakis & Usmen, 1991 ). Cross-listing of shares is another important phenomenon in assessing the pricing of assets in an international setting. We must have to consider the cross-listed share, which is internationally tradable and non-cross-listed shares which are non-tradable assets, and they both occur within the same economy; now the question arises whether the non-tradable assets should also be priced according to the world systematic risk or it should be priced according to domestic pricing portfolios ( Alpanda & Kabaca, 2020 ; Fama & French, 1992 ; Hail & Leuz, 2009 ; Li, 2019 ). Most of the asset pricing models have been tested in the domestic setting and in the closed economies in which assets are priced in the domestic setting and assets are just diversified in the domestic assets. Fama et al. (1973) and Roll & Ross (1980) show the single economy test of CAPM and APT. Various anomalies are seen in single-country studies, like mispricing the securities, seasonal variation, size of the firms and dividend yields ( Korajczyk & Viallet, 1989 ). The International CAPM model is a financial model that helps investors evaluate the return they seek for a certain level of risk, including foreign risk associated with various currencies ( Stulz, 1981 ). Alternative domestic and foreign asset pricing models' price performance. The models are compared when pricing assets in the domestic economy and pricing assets across economies in their international form ( Lee, Ng, & Swaminathan, 2016 ). In International CAPM, apart from the compensation for the time value of money and premium for taking the market risk, investors also get the reward for exposure to foreign currency ( Buckberg, 1995 ). It accounts for the sensitivity to changes in foreign currency when the investor holds the assets.
Despite being prominent features of the theory, it helped in understanding the IAPM because if the risk commands the price, then the linkage among the markets can be studied for the firms operating in these markets.
Research methodology
A systematic and repeatable approach to locating, analysing and synthesising an existing body of knowledge is known as a literature review. A literature review informs the researcher about the study that has already been done; the direction in which the body of knowledge moves; and what can be investigated further. Conducting comprehensive research is difficult without a thorough understanding of the available literature. As a result, in management, a comprehensive systematic literature evaluation is required. Systematic literature reviews are commonly used by researchers and practitioners because they are more transparent and evidence-based.
As a result, we employ bibliometric analysis to give a holistic view of asset pricing theory, filling a vacuum in asset pricing research. This study includes a thorough review of the available literature, which aids in identifying current research areas and future scope. We followed the steps outlined in the subsections below in this study.
Data collection
First, we searched the WOS database with the key term “International Asset Pricing” as the primary key term. The initial results were 3,438 articles.
The analysis was conducted from 2001 to 2021 in order to capture the most recent trends and developments. Between 1989 and 2000, only 20 research publications were found on the concept, and that too were scattered throughout the period concerned. The field received its most substantial research contribution after the year 2000 and henceforth.
The related business domain is the key to accurate analysis, so for that, the disciplines of Economics, Business Finance and Management are considered.
To enhance the study's reliability, only the published research articles are included, and the conference papers, thesis and books are excluded from the list.
The list of the relevant research papers related to International Asset Pricing has been exported from the WOS database in .txt format. After that, the papers were downloaded from the respective databases, i.e. ProQuest, Science Direct, JSTOR, Emerald Insight and Google Scholar ( Figure 1 ).
Based on the criteria mentioned above, 2,487 articles are obtained, which are used for further analysis. The open-source software VOS viewer is being used for the analysis.
Data analysis
To find the important keywords and network diagrams of co-authorship, the data is analysed using the open-source software VOS viewer and Biblioshiny. The publication year, most cited document, authors with the most numbers of research articles and the pioneer publisher in the field of International Asset Pricing are shown with the help of a line graph, bar graph, network tree and tables. Table 1 provides a brief summary of the data for the period 2001–2021, which is represented by the total number of publications (2,487), the annual growth rate of the publication (9.21%) and the average number of citations per document (16.34). The classification of research papers is based on the number of published research articles (2,214), published book chapters (10), early access articles (69) and review articles (193), which provide information on the sample's composition.
Thematic mapping
We employed the theme mapping technique to split the research papers into relevance degree (centrality) and development degree (Density), which provides better insights into the area where the most focus is presented in Figure 2 . The first quadrant presents the niche theme, with the exchange rate, US market and futures as the three main aspects; the second quadrant presents the motor themes, which are extremely pertinent to studies, such as risk, market and cross-section. The emerging themes, where the least amount of research has been done, are shown in the third quadrant constitute consumption, long-run and asset return. The basic themes, which are primarily asset returns, prices and various models of asset pricing, which is the study's core theme, are shown in the fourth quadrant. The central area which is a cross-section of these four quadrants shows information, performance and determinants as the key factor of the study.
Publication year
The publication trend of the international pricing is given in Figure 3 . The earliest publication in WOS is in the year 1989. But significantly fewer research papers were published between the period 1989 and 2000. Although the research publication rate was slow during these years, the trend surged after 2013. The publication in International Asset Pricing increased over time and reflected a remarkable increase of attention towards the development of pricing models.
Figure 2 shows the citation trend which also shows an uprising trend which shows the importance of this topic in the current time. A good number of papers published in the past few years is also reflected in the citation graph.
We classified our study into four areas for bibliometric analysis: authors, keywords, journals and title and abstract text analysis. For clarity, these categories are further subdivided into many subcategories.
In terms of citations, Table 1 summarises the most prominent authors in international asset pricing. The authors' publications give a thorough development in this area by showing the development of international asset pricing from a different perspective. By undertaking diverse empirical tests and outcomes, these authors have contributed to the development of pricing models, providing a stronger foundation for future research.
From 2001 to 2021, Zaremba and Adam published the maximum number of articles (26), followed by Wang and Xingchun, who published 18 papers, being the second most productive author in the field of International Asset Pricing, as shown in Figure 3 .
Author's impact
The author's impact in the area can be evaluated through the citations of their papers ( Figure 4 ). Table 2 shows that Maggiori and Motto have the highest citation of 277 with an average of 55.4 citations per article, making them the most impactful authors in the International Asset Pricing field, followed by Ramdorai Tarun with a total citation of 240 with an average of 48 citations per articles.
Corresponding author's country
Figure 6 shows the corresponding author's country and visualises the linkages of authors from the major countries. The USA has the associations for the maximum countries, including England, Australia and China which shows a strong association and a weak link to the countries like Chile, Indonesia and Argentina.
The data from the table helps to comprehend the contribution of a country to the overall research as well as the level of collaboration between scholars from different countries. It is possible to establish which countries publish the most research on a certain topic, as well as the authors' desire to collaborate, based on this information. The information in the table can be used to determine a country's contribution to the overall study as well as the level of collaboration between researchers from other countries. It is possible to establish which countries publish the most research on a certain topic, as well as the authors' desire to collaborate, based on this information. Table 2 reveals that the USA has published the most articles (860) and has the highest number of citations (21,995), followed by England published 382 articles with citations 7,415 showing the level of awareness of International Asset Pricing in the developed stock market. In contrast, the stock market that is still emerging has published fewer articles and very weak collaboration and linkages to other countries, as depicted in Figure 6.
Each subfield of a research topic can be investigated further in multiple dimensions using various frameworks and methodologies. Every domain can be explored further in other dimensions utilising different frameworks and appropriate procedures. As a result, the literature develops several relevant keywords that emphasise the relevance of research in the field. As a result, the depth and breadth of the research field can be determined using keyword analysis. In a nutshell, keyword analysis establishes a relationship between study subfields and emphasises their importance. Figure 5–7 suggests the most frequent keywords across the author's keywords, keyword plus and all keywords. The keywords help the researcher decide their topic accordingly, whether to choose the selected topic or drop it. The first-word cloud of all keywords shows that “Risk” is the most used keyword in the cloud. As risk is a crucial part of the valuation and in International Asset Pricing, risk acts as one of the most important criteria. The research being conducted across the theme of risk. Other frequently used keywords are return, volatility, cross-section and stock prices. The second cloud shows asset pricing and international asset pricing as the most used keyword, which is also the research theme. The third figure, which is a network visualisation of the keywords, concludes that international asset pricing goes with the theme of the volatility of the stock market, the cross-section of stock price movement, risk and return as a crucial factor, financial crisis as a significant event and monetary policies of the countries, market information and equilibrium. These are some of the essential areas which are being studied over time. But some areas are rising in the current years, and the works are not much conducted in these areas: asymptotic theory, cryptocurrencies, rational bubbles, fiscal policy, endogenous growth, co-variance matrix, risk premia idiosyncratic risk and stochastic dominance ( Figure 8 ).
Table 3 shows the top 10 publishers in the field of economics, finance and management. These publishers are shown in terms of their publications regarding the topic. Elsevier is a leading publisher in this field which published 1,287 journal articles in the past 20 years in international pricing, followed by Wiley (406), Taylor and Francis (169) and Springer (142). Table 4 shows the top 10 best journals in the field of International Asset pricing regarding the number of articles published.
Title and abstract analysis
Knowing the concentration of sectors in which the studies are mainly conducted is crucial for forming new research topics. It gives the direction to researchers for better framing. For this purpose, the text analysis is undertaken for the titles of research papers and their abstract. Figure 9 depicts the network visualisation, it is presented to focus on the most relevant areas.
From Figure 11 , it is clear that the studies related to the cross-section of stock return, international evidence, pricing of assets, monetary policies impact, valuation and risk are critical areas of research where most topics are being researched.
Figure 10 shows the network diagram of the frequent words used in the abstracts of the authors that shows the three clusters consisting of Red (1), Green (2) and Blue (3), showing the different linkages path, models, financial crisis, monetary policy and asset prices are the primarily used words in Cluster 1. In Cluster 2, investor, strategy, value, investment and funds are the most often used words, while factor model, stock return and stock market are the essential words used in Cluster 3 ( Figure 11 ).
Recent trends in publication
The area of international asset pricing has seen various developments in recent years, including studies related to investing and investment behaviour ( Chauhan, Ahmad, Aggarwal, & Chandra, 2020 ; Dharani, Hassan, & Paltrinieri, 2019 ; Javed, Husain, & Ali, 2020 ; Keshari & Gautam, 2022 ; Pandey & Sehgal, 2019 ), showing the role of faith and investor sentiment in asset pricing ( Anand, Basu, Pathak, & Thampy, 2021 ; Kazmaier & van Vuuren, 2020 ; Zhang, Gong, Wang, & Ye, 2021 ). On the other hand, studies have focused on the use of machine learning, deep learning and encoders in asset pricing, which gives better insights and accurate results regarding the dimensions of asset pricing ( Gu, Kelly, & Xiu, 2020 , 2021 ; Lettau & Pelger, 2020 ). In recent years, the domestic asset pricing model (CAPM) and more advanced models like the IAPM, the conditional asset pricing model (Co-CAPM), the liquidity adjusted CAPM and the Fama–French three-factor and five-factor models have been used a lot ( Fama & French, 2015 ; Joshi & Joshi, 2021 ; Kumar & Misra, 2019 ; Lewellen & Nagel, 2016 ). Market structure and co-integration of stock markets are another yet very important phenomena in asset pricing. Researchers have extensively considered these areas to uncover new dimensions in asset pricing ( Caporale, Gil-Alana, & You, 2022 ; Greenwood et al. , 2018 ; Guasoni & Wong, 2020 ; Joshi & Joshi, 2021 ). Cross-listing and tradable assets have also given a strong basis to the development of asset pricing models, and recent studies have focused on these dimensions in detail to encourage global investing and better diversification of portfolios ( Alpanda & Kabaca, 2020 ; Li, 2019 ; Adler & Dumas, 2016 ; Das & Barai, 2017 ).
Citation analysis
Citations measure an article's, author's and Journal's influence in academics. Authors with highly cited works are thought to be significant in their fields of study, and journals are ranked based on the number of citations they receive. Citations are seen as feedback from the academic community, and they are used to assess the quality and contribution of a given publication in a given field. The journals which meet the criteria are presented below. The below density diagram shows the most cited research journals with a minimum of 100 citations in International Asset Pricing. Journal of Finance (10,667) is the most influential journal in terms of citations, followed by the Journal of F inancial E conomics (6,604) and Review of F inancial S tudies (4,423) ( Figures 12 and 13 ).
Table 4 presents the top 10 most cited research articles of International Asset Pricing. The articles are ranked in terms of citations from highest to lowest. The article “Size, Value and Momentum” in international stock returns published in the Journal of Financial Economics is the most cited. In this paper, Fama and French (2012) empirically tested whether traditional asset pricing models represent the value and momentum patterns in international average returns and whether asset pricing appears to be integrated across countries. It was the first study to examine the three-factor and four-factor global asset pricing models on size-B/M and size momentum portfolios returns. The study also compared the local and domestic versions of CAPM and with the given cases explains which model performs better in the case of the integration of the global market. Later, many researchers applied the same models to different countries' portfolios and popularised this methodology. A common pattern has been found in the average returns of developed markets. Betting against Beta is the second most cited paper authored by Frazzini and Pedersen (2014) , which presented a model for leverage with margin constraints that vary across investor and time. The paper became popular among the investors due to its implications, because their findings are evidently consistent with five central predictions. They use the international model for pricing the securities as the use of betting against the beta factor involves 20 countries in their study. Findings show that the high-beta asset portfolios have much less alphas and Sharpe ratios than low-beta asset portfolios and the increased funding liquidity risk compresses beta towards one. The findings of the study give very interesting results and open up the different dimensions of the studies for the researchers. The increased number of citations received by these papers reflects their importance and contribution to asset pricing research. The articles' authors are well-known in their fields and have contributed relevant literature ( Table 5 ).
The analysis reveals the USA as the top country in terms of publication, followed by England, China, France, Australia and other European countries. Very little presence has been seen from countries of emerging economies as India is at 26th rank in terms of publication – finding is consistent with the research of Ali and Bashir (2021 ). The author and article-based analysis show Fama and French, Frazzini and Pedersen, Gorton and Rouwenhorst, RJ Shiller published the most influential paper in the study. Zaremba and Adam and Wang and Xingchun are the most productive authors in terms of the most influential author. These outcomes also emphasise that they mainly belong to the developed nations, which shows that the research is concentrated primarily in those areas.
Furthermore, the keyword analysis shows risk, return, volatility, cross-section, monetary policy, market information and equilibrium are some main dimensions of international asset pricing. Keyword network visualisation shows the international asset pricing goes with the theme of the volatility of the stock market, the cross-section of stock price movement, risk and return as a crucial factor and financial crisis as a significant event, whereas it gives us the direction to the emerging areas where less research has been conducted over the period and needs to assess in future; these include asymptotic theory, cryptocurrencies, rational bubbles, fiscal policy, endogenous growth, co-variance matrix, risk premia idiosyncratic risk and stochastic dominance.
Managerial implications
The study explains the background of various asset pricing models and the development of an international version of CAPM. The paper answers the question, “How does international asset pricing develop over time?” An understanding of these models will help the finance manager in decreasing the equity cost by comparing the local and domestic versions of asset pricing models. The criticism of the single-factor model “CAPM” gives rise to the conditional CAPM and multifactor model “Arbitrage Pricing Theory”. Multifactor APT model incorporates the macroeconomics factors and these factors influence the decision of raise capital from abroad and application of these models will help the managers in risk valuation and management. Researchers also explained the international version of CAPM and APT ( Buckberg, 1995 ; Korajczyk & Viallet, 1989 ; Lee et al. , 2016 ; Solnik, 2018 ; Stulz, 1981 ). The study implicates and gives direction to the future of international asset pricing by using modern techniques for the calculation of their assets and encourages cross-border listing. IAPM model will give managers of the companies a better edge to value their assets and also help them raise capital outside of the domestic market. For investors, the study will help in identifying the best market where they can diversify their risk and make their portfolio optimised.
Asset pricing is a fundamental concept in financial market theory and a well-studied phenomenon in finance ( Khudoykulov, 2020 ) which have different but essential functions in investing. When asset pricing valuation is done in a global scenario, the importance of the study becomes more critical. As research is a never-ending phenomenon, and a lot of research has been conducted in this area. Still, in the current dynamic world where things are happening quickly, the study needs continuous improvement. The inadequacy of contemporary models to adequately explain returns was one of the motivators for developing various forms of asset pricing models. By altering the list of explanatory variables, each subsequent model seeks to provide a more comprehensive explanation. Anomaly patterns in returns, observed from time to time, drive the identification of additional explanatory variables ( Harshita et al. , 2015 ). Though it is highly researched, the publications rose in the past few years from 2015. Still, the publications are maximum in 2020, which shows the new opportunity and importance of this area. As a result, the purpose of this research is to examine the evolution of international asset pricing and to identify areas for further research. The study identifies and highlights the most important research articles, authors and keywords based on a literature review and bibliometric analysis of 2,487 documents published over the past 20 years (2001–2021), accessed from the WOS database, to provide a new direction for future research in international asset pricing. The abstract network analysis reveals that many research works have been conducted, including Japan as a country, but Japan does not contribute much to publishing papers. This may happen due to the language constraint; many of the documents are published in the Japanese language.
Suggestions for future research
Regarding the scope of future research, the study gives the following directions to researchers:
Firstly, the study shows that “International Asset Pricing” is a well-established phenomenon in developed stock markets. The studies are mainly concentrated in the USA and other European countries. Still, there is much scope for the study in emerging markets to examine IAPMs and check the performance of developing markets in this integrating market structure.
Secondly, the keyword analysis emphasises the development of specific areas that are prominent in the current time and can provide new aspects to the asset pricing literature, such as rational bubbles, fiscal policy, endogenous growth, co-variance matrix, risk premia, idiosyncratic risk and stochastic dominance and the introduction of cryptocurrencies, so this can also be one of the crucial aspects for analysing asset pricing considering these trending areas of research.
Thirdly, the stock markets are interdependent and co-integrated in the current context due to the flow of money across borders, cross-listed stocks and cross-section returns. As a result, the issue of international politics and economic stability will open new areas of research.
In addition to the above, many instances affected the stock market worldwide, like the Global Financial Crisis (2007–2009), the European Bond Crisis (2012–2014) and the recent occurrence of Covid-19 across the world. At these events, a surge in the literature can be seen as these events affect the market and its spillover effect is visible across the globe, which can be seen in the last year as well where the maximum number of research papers have been published worldwide.
Flowchart according to PRISMA model
Thematic mapping for the article type classification
Publications over the years
Citations over the years
Most relevant authors in terms of the number of articles published
Word cloud of all the keywords
Word cloud of author's keywords
Network analysis keyword plus
Publishers with number of publications
Network diagram of title analysis
Network diagram of abstract analysis
Citation analysis of journals
Main information about data
Source(s): The authors
Acharya , V .V. , & Pedersen , L. H. ( 2005 ). Asset pricing with liquidity risk . Journal of financial Economics , 77 ( 2 ), 375 – 410 .
Adler , M. , & Dumas , B. ( 2016 ). International portfolio choice and corporation finance: A synthesis . The Journal of Finance , 38 ( 3 ), 925 – 984 .
Ali , A. , & Bashir , H. A. ( 2021 ). Bibliometric study on asset pricing . Qualitative Research in Financial Markets , ahead-of-print ( ahead-of-print ). doi: 10.1108/qrfm-07-2020-0114 .
Ali Khrawish , H. , Zakaria Siam , W. , & Jaradat , M. ( 2010 ). The relationships between stock market capitalization rate and interest rate: Evidence from Jordan . Business and Economic Horizons , 2 , 60 – 66 .
Alpanda , S. , & Kabaca , S. ( 2020 ). International spillovers of large-scale asset purchases . Journal of the European Economic Association , 18 ( 1 ), 342 – 391 .
Amihud , Y. , & Mendelson , H. ( 1989 ). The effects of beta, bid‐ask spread, residual risk, and size on stock returns . The Journal of Finance , 44 ( 2 ), 479 – 486 .
Anand , A. , Basu , S. , Pathak , J. , & Thampy , A. ( 2021 ). The impact of sentiment on emerging stock markets . International Review of Economics and Finance , Elsevier , 75 , 161 – 177 .
Andersen , T. G. , Bollerslev , T. , Diebold , F. X. , & Vega , C. ( 2007 ). Real-time price discovery in global stock, bond and foreign exchange markets . Journal of International Economics , 73 ( 2 ), 251 – 277 .
Ang , A. , & Bekaert , G. ( 2007 ). Stock return predictability: Is it there? The Review of Financial Studies , 20 ( 3 ), 651 – 707 .
Bai , Y. , & Green , C. J. ( 2020 ). Country and industry factors in tests of capital asset pricing models for partially integrated emerging markets . Economic Modelling , 92 , 180 – 194 .
Bawa , V. S. , & Lindenberg , E. B. ( 1977 ). Capital market equilibrium in a mean-lower partial moment framework . Journal of financial economics , 5 ( 2 ), 189 – 200 .
Breeden , D. T. , Gibbons , M. R. , & Litzenberger , R. H. ( 2016 ). Empirical tests of the consumption-oriented . CAPM , 44 ( 2 ), 231 – 262 .
Buckberg , E. ( 1995 ). Emerging stock markets and international asset pricing . World Bank Economic Review , 9 ( 1 ), 51 – 74 .
Caporale , G. M. , Gil-Alana , L. A. , & You , K. ( 2022 ). Stock market linkages between the Asian countries, China and the US: A fractional integration/cointegration approach . Emerging Markets Finance and Trade , Routledge , 58 ( 5 ), 1502 – 1514 .
Chauhan , Y. , Ahmad , N. , Aggarwal , V. , & Chandra , A. ( 2020 ). Herd behaviour and asset pricing in the Indian stock market; herd behavior and asset pricing . IIMB Management Review , Elsevier , 32 ( 2 ), 143 – 152 .
Chen , M. H. ( 2003 ). Risk and return: CAPM and CCAPM . Quarterly Review of Economics and Finance , 43 ( 2 ), 369 – 393 .
Christensen , P. O. , & Feltham , G. A. ( 2009 ). Equity valuation . Foundations and Trends in Accounting , 4 ( 1-2 ), 1 – 112 .
Connor , G. , & Sehgal , S. ( 2001 ). Tests of the Fama and French model in India . London School of Economics and Political Science , 379 , 1 – 23 , Discussion Paper .
Darrat , A. F. , Li , B. , & Park , J. C. ( 2011 ). Consumption-based CAPM models: International evidence . Journal of Banking and Finance , Elsevier B.V. , 35 ( 8 ), 2148 – 2157 .
Das , S. , & Barai , P. ( 2017 ). The role of non-marketable assets to determine the cost of capital: Evidence from India . Asian Journal of Business and Accounting , 10 ( 2 ), 77 – 104 .
De Santis , G. , & Gerard , B. ( 1997 ). International asset pricing and portfolio diversification with time-varying risk . Journal of Finance , 52 ( 5 ), 1881 – 1912 .
Dharani , M. , Hassan , M. K. , & Paltrinieri , A. ( 2019 ). Faith-based norms and portfolio performance: Evidence from India . Global Finance Journal , Elsevier , 41 , 79 – 89 .
El Hedi Arouri , M. , Rault , C. , Sova , A. , Sova , R. , & Teulon , F. ( 2013 ). Market structure and the cost of capital . Economic Modelling , Elsevier B.V. , 31 ( 1 ), 664 – 671 .
Errunza , V. , Losq , E. , & Padmanabhan , P. ( 1992 ). Tests of integration, mild segmentation and segmentation hypotheses . Journal of Banking and Finance , 16 ( 5 ), 949 – 972 .
Errunza , V. R. , & Miller , D. P. ( 2016 ). Market segmentation and the cost of capital in international equity markets . The Journal of Financial and Quantitative Analysis , 35 ( 4 ), 577 – 600 .
Fama , E. F. ( 1970 ). Efficient capital markets: A review of theory and empirical work . The Journal of Finance , 25 ( 2 ), 383 – 417 .
Fama , Eugene , F. , & MacBeth James , D. ( 1973 ). Risk, return, and equilibrium: empirical tests . Journal of Political Economy , 38 , 607 – 636 .
Fama , E. F. , & French , K. R. ( 1992 ). The cross-section of expected stock returns . The Journal of Finance , 47 ( 2 ), 427 – 465 .
Fama , E. F. , & French , K. R. ( 2012 ). Size, value, and momentum in international stock returns . Journal of Financial Economics , Elsevier , 105 ( 3 ), 457 – 472 .
Fama , E. F. , & French , K. R. ( 2015 ). A five-factor asset pricing model . Journal of Financial Economics , Elsevier , 116 ( 1 ), 1 – 22 .
Frazzini , A. , & Pedersen , L. H. ( 2014 ). Betting against beta . Journal of Financial Economics , Elsevier , 111 ( 1 ), 1 – 25 .
Gorton , G. , & Rouwenhorst , K. G. ( 2006 ). Facts and fantasies about commodity futures . Financial Analysts Journal , 62 ( 2 ), 47 – 68 .
Greenwood , R. , Hanson , S. G. , & Liao , G. Y. ( 2018 ). Asset price dynamics in partially segmented markets . Review of Financial Studies , 31 ( 9 ), 3307 – 3343 .
Gu , S. , Kelly , B. , & Xiu , D. ( 2020 ). Empirical asset pricing via machine learning . Review of Financial Studies , 33 ( 5 ), 2223 – 2273 .
Gu , S. , Kelly , B. , & Xiu , D. ( 2021 ). Autoencoder asset pricing models . Journal of Econometrics , Elsevier B.V. , 222 ( 1 ), 429 – 450 .
Guasoni , P. , & Wong , K. C. ( 2020 ). Asset prices in segmented and integrated markets . Finance and Stochastics , 24 ( 4 ), 939 – 980 .
Hail , L. , & Leuz , C. ( 2009 ). Cost of capital effects and changes in growth expectations around U.S. cross-listings . Journal of Financial Economics , Elsevier , 93 ( 3 ), 428 – 454 .
Harlow , W. V. , & Rao , R. K. ( 1989 ). Asset pricing in a generalized mean-lower partial moment framework: Theory and evidence . Journal of financial and quantitative analysis , 24 ( 3 ), 285 – 311 .
Harshita , Singh , S. , & Yadav , S. S. ( 2015 ). Indian stock market and the asset pricing models . Procedia Economics and Finance , Elsevier B.V. , 30 ( 15 ), 294 – 304 .
Harvey , C. R. ( 1991 ). The world price of covariance risk . The Journal of Finance , 46 ( 1 ), 111 – 157 .
Iqbal , J. , & Brooks , R. ( 2007 ). Alternative beta risk estimators and asset pricing tests in emerging markets: the case of Pakistan . Journal of Multinational Financial Management , 17 ( 1 ), 75 – 93 .
Javed , S. , Husain , U. , & Ali , S. ( 2020 ). Relevancy of investment decisions and consumption with asset pricing: GMM and CCAPM model approach . International Journal of Management , 11 ( 8 ), 10 – 17 .
Joshi , N. A. , & Joshi , M. ( 2021 ). Co-Integration and causality among stock market indices: A study of 35 indices across 5 . SCMS Journal of Indian Management , 18 ( 1 ), 131 – 153 .
Karolyi , G. A. & Stulz , R. M. , ( 2002 ). Nber working paper series are financial assets priced locally or globally? Available from: http://www.nber.org/papers/w8994 .
Kazmaier , J. , & van Vuuren , J. H. ( 2020 ). A generic framework for sentiment analysis: Leveraging opinion-bearing data to inform decision making . Decision Support Systems , Elsevier B.V. , 135 , 113304 .
Keshari , A. , & Gautam , A. ( 2022 ). Empirical testing of co-integration of international financial markets with reference to India, the USA, Japan, and Hong Kong . Jindal Journal of Business Research , 11 ( 1 ), 1 – 10 .
Khudoykulov , K. ( 2020 ). Asset-pricing models: A case of Indian capital market . Cogent Economics and Finance , Cogent , 8 ( 1 ). doi: 10.1080/23322039.2020.1832732 .
Kodres , L. E. , & Pritsker , M. ( 2002 ). A rational expectations model of financial contagion . The Journal of Finance , 57 ( 2 ), 769 – 799 .
Korajczyk , R. A. , & Viallet , C. J. ( 1989 ). An empirical investigation of international asset pricing . The Review of Financial Studies , 2 ( 4 ), 553 – 585 .
Krause , A. ( 2001 ). An overview of asset pricing models (p. 154 ). University of Bath, School of Management . https://people.bath.ac.uk/mnsak/Research/Asset_pricing.pdf [Accessed 25 March, 2022 ].
Kumar , G. , & Misra , A. K. ( 2019 ). Liquidity-adjusted CAPM – An empirical analysis on Indian stock market . Cogent Economics and Finance , Cogent , 7 ( 1 ), 1 – 15 .
Lee , C. , Ng , D. , & Swaminathan , B. ( 2016 ). Testing international asset pricing models using implied costs of capital . The Journal of Financial and Quantitative Analysis , 44 ( 2 ), 307 – 335 .
Lettau , M. , & Pelger , M. ( 2020 ). Estimating latent asset-pricing factors . Journal of Econometrics , Elsevier B.V. , 218 ( 1 ), 1 – 31 .
Lewellen , J. , & Nagel , S. ( 2016 ). The conditional CAPM does not explain asset-pricing anomalies . The Journal of Finance , Routledge , 16 ( 4 ), 1 – 114 .
Li , H. ( 2019 ). Direct overseas listing versus cross-listing: A multivalued treatment effects analysis of Chinese listed firms . International Review of Financial Analysis , Elsevier , 66 , 101391 .
Marín-Marín , J. A. , Moreno-Guerrero , A. J. , Dúo-Terrón , P. , & López-Belmonte , J. ( 2021 ). STEAM in education: A bibliometric analysis of performance and co-words in web of science . International Journal of STEM Education , 8 ( 1 ). doi: 10.1186/s40594-021-00296-x .
Merton , R. C. ( 1973 ). An intertemporal capital asset pricing model . Econometrica: Journal of the Econometric Society , 41 ( 5 ), 867 – 887 .
Moradian , M. , Khalaj‐Amirhosseini , M. , & Tayarani , M. ( 2010 ). Design of planar slotted array antenna fed by single ridge waveguide . International Journal of RF and Microwave Computer‐Aided Engineering , 20 ( 6 ), 593 – 602 .
Moskowitz , T. J. , Ooi , Y. H. , & Pedersen , L. H. ( 2012 ). Time series momentum . Journal of Financial Economics , 228 – 250 .
Musawa , N. , Kapena , S. , & Shikaputo , C. ( 2020 ). A better stock pricing model: A systematic literature review . Journal of Economic and Financial Sciences , 13 ( 1 ), 1 – 6 .
Pandey , P. , & Sehgal , S. ( 2019 ). Investor sentiment and its role in asset pricing: An empirical study for India . IIMB Management Review , Elsevier , 31 ( 2 ), 127 – 144 .
Pástor , Ľ. , & Stambaugh , R. F. ( 2000 ). Comparing asset pricing models: an investment perspective . Journal of Financial Economics , 56 ( 3 ), 335 – 381 .
Patro , D. K. ( 2001 ). Market segmentation and international asset prices: Evidence from the listing of world equity benchmark shares . Journal of Financial Research , 24 ( 1 ), 83 – 98 .
Phillips , P. C. , Wu , Y. , & Yu , J. ( 2011 ). Explosive behavior in the 1990s Nasdaq: When did exuberance escalate asset values? International Economic Review , 52 ( 1 ), 201 – 226 .
Pirinsky , C. A. , & Wang , Q. ( 2011 ). Market segmentation and the cost of capital in a domestic market: Evidence from municipal bonds . Financial Management , 40 ( 2 ), 455 – 481 .
Raei , R. , Ahmadinia , H. , & Hasbaei , A. ( 2011 ). A study on developing of asset pricing models . International Business Research , 4 ( 4 ). doi: 10.5539/ibr.v4n4p139 .
Rahnamaie Roodposhti , F. , Alikhani , R. , & Maranjory , M. ( 2009 ). Applicational investigation of Altman and Fulmer bankruptcy prediction models in Tehran stock exchange . Accounting and Auditing Review , 16 ( 2 ).
Ranjan Dash , S. , & Mahakud , J. ( 2013 ). Conditional multifactor asset pricing model and market anomalies . Journal of Indian Business Research , 5 ( 4 ), 271 – 294 .
Ray , S. ( 2012 ). Foreign exchange reserve and its impact on stock market capitalization: Evidence from India . Research on Humanities and Social Sciences , 2 ( 2 ), 46 – 61 .
Roll , R. , & Ross , S. A. ( 1980 ). An empirical investigation of the arbitrage pricing theory . The Journal of Finance , 35 ( 5 ), 1073 – 1103 .
Sehgal , S. , & Tripathi , V. ( 2006 ). Sources of size effect: evidence from INDIAN stock market . The Icfai Journal of Applied Finance , 12 ( 3 ), 18 – 28 .
Sehrawat , N. , Kumar , A. , Nigam , N. K. , Singh , K. , & Goyal , K. ( 2020 ). Test of capital market integration using Fama-French three-factor model: Empirical evidence from India . Investment Management and Financial Innovations , 17 ( 2 ), 113 – 127 .
Shackelford , D. A. , & Shevlin , T. ( 2001 ). Empirical tax research in accounting . Journal of accounting and economics , 31 ( 1–3 ), 321 – 387 .
Shiller , R. J. ( 2003 ). From efficient markets theory to behavioral finance . Journal of economic perspectives , 17 ( 1 ), 83 – 104 .
Singh , S. , & Yadav , S. S. ( 2015 ). Indian stock market and the asset pricing models . Procedia Economics and Finance , 30 , 294 – 304 .
Solnik , B. H. ( 2018 ). Testing international asset pricing: Some pessimistic views . The Journal of Finance , 32 ( 2 ), 503 – 512 .
Stulz , R. M. ( 1981 ). A model of international asset pricing . Journal of Financial Economics , 9 ( 4 ), 383 – 406 .
Stulz , R. M. ( 1995 ). The cost of capital in internationally integrated markets: The case of Nestlé . European Financial Management , 1 ( 1 ), 11 – 22 .
Taneja , Y. P. ( 2010 ). Revisiting Fama French three-factor model in Indian stock market . Vision: The Journal of Business Perspective , 14 ( 4 ), 267 – 274 .
Thomadakis , S. , & Usmen , N. ( 1991 ). Foreign project financing in segmented capital markets: Equity versus debt . Financial Management , 20 ( 4 ), 42 .
Wang , M. C. , Fang , M. , & Ye , J. K. ( 2013 ). Financial integration of large-and small-cap stocks in emerging markets . Emerging Markets Finance and Trade , 49 ( Suppl. 4 ), 17 – 31 .
Zhang , W. , Gong , X. , Wang , C. , & Ye , X. ( 2021 ). Predicting stock market volatility based on textual sentiment: A nonlinear analysis . Journal of Forecasting , 40 ( 8 ), 1479 – 1500 .
Zhu , J. , & Liu , W. ( 2020 ). A tale of two databases: The use of web of science and Scopus in academic papers . Scientometrics , Springer International Publishing , 123 ( 1 ), 321 – 335 .
Acknowledgements
Aditya Keshari is the awardee of the ICSSR Doctoral Fellowship. The paper “Asset Pricing in Global Scenario: A bibliometric analysis” is largely an outcome of the Post-Doctoral Fellowship sponsored by the Indian Council of Social Science Research (ICSSR). However, the responsibility for the facts stated, opinions expressed and conclusions drawn entirely belongs to the author.
Corresponding author
Related articles, we’re listening — tell us what you think, something didn’t work….
Report bugs here
All feedback is valuable
Please share your general feedback
Join us on our journey
Platform update page.
Visit emeraldpublishing.com/platformupdate to discover the latest news and updates
Questions & More Information
Answers to the most commonly asked questions here
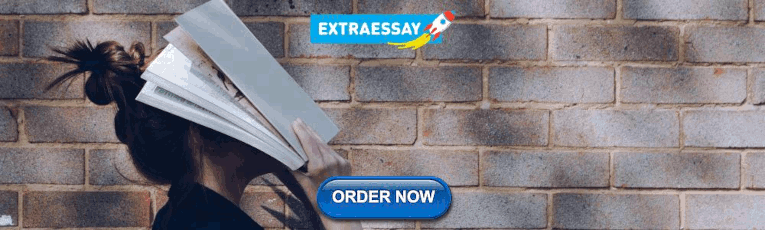
IMAGES
VIDEO
COMMENTS
The CAPM (Sharpe, 1964; Lintner, 1965) marks the birth of asset pricing theory. This model is based on the idea that not all risk should affect asset prices. The model thus provides insight into ...
The conventional agenda in the asset pricing literature studies quantitative rational expectation models. 9 This approach is particularly well suited for the study of recurring patterns, such as the comovement of price-dividend ratios on stocks with the business cycle or the seasonality in the housing market during a calendar year. (Volume and ...
This paper re-examines the presence of the Sharpe-Treynor-Lintner-Mossin capital asset pricing model (CAPM) in the finance literature and is accompanied by a bibliometric summary analysis. The popular model is in its sixth decade; we summarized the relevance of the CAPM using publication and citation trends, as well as identifying its most prolific and impactful contributors.
The CAPM (Sharpe, 1964; Lintner, 1965) marks the birth of asset pricing theory. This model is based on the idea that not all risk should affect asset prices. The model thus provides insight into the kind of risk that is related to return. Four decades later, the CAPM is still widely used in applications. The CAPM provides a methodology for ...
Introduction. The capital asset pricing model (CAPM) of Sharpe (1964), Treynor (1999), Lintner (1965a, 1965b), and Mossin (1966) rightfully occupies a central place in the asset pricing literature. Not surprisingly, an enormous research effort has been devoted to the testing of the CAPM over the past six decades due to its relatively simple and ...
2 Literature Review 2.1 Fundamentals of Asset Pricing Theory ... (2004) pointed out, every asset pricing model is a capital asset pricing model, but the finance profession refers to the specific model of Sharpe (1964), Lintner (1965) and Black (1972) by the acronym CAPM. Thus, throughout this study, I will also use the CAPM terminology in
The Capital Asset Pricing Model (hereafter CAPM) (Black, 1972; Lintner, 1965; Sharpe, 1964) has proven to be the most commonly applied financial model for explaining stock returns.This model claims that the expected required return on an individual stock i E(r i) is equal to the risk-free rate r f (for approximation, a 1-month T-bill return) with an addition of the systematic risk coefficient ...
In this paper we provide a literature review of the main factors-based asset pricing models, focusing in particular on factors related to firm characteristics. After presenting the Capital Asset ...
The asset pricing models of financial economics describe the prices and expected rates of return of securities based on arbitrage or equilibrium theories. These models are reviewed from an empirical perspective, emphasizing the relationships among the various models. ... , and Cochrane review this literature; Sarkissian provides a recent ...
In this paper we provide a literature review of the main factors-based asset pricing models, focusing in particular on factors related to firm characteristics. After presenting the Capital Asset Pricing Model, we describe first the most important empirical evidence that led to the well-known Fama-French three-factors model. Next, we highlight the most widely used multi-factors pricing models ...
A Review of Capital Asset Pricing Models Don U.A.Galagedera* Department of Econometrics and Business Statistics Monash University PO Box 197 Caulfield East Victoria 3145 Australia Abstract This paper provides a review of the main features of asset pricing models. The review includes single-factor and multifactor models, extended forms of the
1 Review of literature on multifactor asset pricing models Mario Pitsillis Abstract The purpose of asset pricing theory is to understand the prices or values or returns of claims to uncertain payments, for example stocks, bonds and options. The most important factor in the valuation is the risk of payments of the asset under examination.
The purpose of this paper is to review the traditional capital asset pricing model (CAPM) and its variants adopted in empirical investigations of asset pricing. Design/methodology/approach - Pricing models are discussed under five categories: the single-factor model, multifactor models, CAPM with higher order systematic co-moments, CAPM ...
Abstract. This article presents an overview of literature on behavioural and experimental asset pricing theory. We systematically review the evolution and current devel-opment of behavioural asset pricing models as an alternate approach to asset pricing in financial economics literature. A review and synthesis of research carried out in ...
Literature Review. Gaston Michel. Chapter. 943 Accesses. The literature has shown that there are numerous empirical regularities that are unexplained by market beta leading to the development of the Fama-French model. This section provides an overview of the relevant asset pricing theories that motivate this model and build the theoretical ...
Download Free PDF. Jibran Sheikh. The capital asset pricing model (CAPM) states that assets are priced commensurate with a trade-off between undiversifiable risk and expectations of return. The model underpins the status of academic finance, as well as the belief that asset pricing is an appropriate subject for economic study.
Jegadeesh et al. focus on ex-ante risk premia estimation of asset pricing models and resort to the original Fama-MacBeth approach for computing standard errors and test ... Collot, S., Hemauer, T. A literature review of new methods in empirical asset pricing: omitted-variable and errors-in-variable bias. Financ Mark Portf Manag 35 ...
Before going for bibliometric analysis, a detailed literature review is being conducted to assess the various asset pricing models, and their latest development and development over time. The current list considers the articles published in the past 20 years between (2001 and 2021); for that, we adopted the Web of Science (WOS) as our source ...
The CAPM (Sharpe, 1964; Lintner, 1965) marks the birth of asset pricing theory. This model is based on the idea that not all risk should affect asset prices. The model thus provides insight into ...
The study provides a comprehensive systematic literature review of empirical findings on neoclassical asset pricing tests, during the period from 1997 to 2017 in Sri Lanka.
The current study presents a systematic review of macroeconomic risk factors and stock market performance under the broader theme of multi-factor asset pricing or the Arbitrage Pricing Theory (APT). Based on the review of relevant literature, the study summarizes key findings of different research works and identifies significant research gaps ...
Abstract. Capital Asset Pricing Model (CAPM) is an important theory in financial economics. It was based on Markowitz's Modern Asset Allocation Theory (MPT) and proposed by Sharpe. This model ...