Null Hypothesis Definition and Examples
PM Images / Getty Images
- Chemical Laws
- Periodic Table
- Projects & Experiments
- Scientific Method
- Biochemistry
- Physical Chemistry
- Medical Chemistry
- Chemistry In Everyday Life
- Famous Chemists
- Activities for Kids
- Abbreviations & Acronyms
- Weather & Climate
- Ph.D., Biomedical Sciences, University of Tennessee at Knoxville
- B.A., Physics and Mathematics, Hastings College
In a scientific experiment, the null hypothesis is the proposition that there is no effect or no relationship between phenomena or populations. If the null hypothesis is true, any observed difference in phenomena or populations would be due to sampling error (random chance) or experimental error. The null hypothesis is useful because it can be tested and found to be false, which then implies that there is a relationship between the observed data. It may be easier to think of it as a nullifiable hypothesis or one that the researcher seeks to nullify. The null hypothesis is also known as the H 0, or no-difference hypothesis.
The alternate hypothesis, H A or H 1 , proposes that observations are influenced by a non-random factor. In an experiment, the alternate hypothesis suggests that the experimental or independent variable has an effect on the dependent variable .
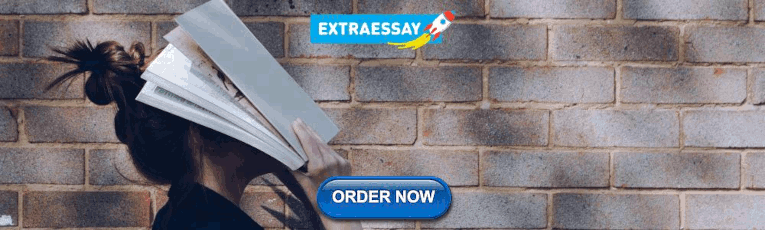
How to State a Null Hypothesis
There are two ways to state a null hypothesis. One is to state it as a declarative sentence, and the other is to present it as a mathematical statement.
For example, say a researcher suspects that exercise is correlated to weight loss, assuming diet remains unchanged. The average length of time to achieve a certain amount of weight loss is six weeks when a person works out five times a week. The researcher wants to test whether weight loss takes longer to occur if the number of workouts is reduced to three times a week.
The first step to writing the null hypothesis is to find the (alternate) hypothesis. In a word problem like this, you're looking for what you expect to be the outcome of the experiment. In this case, the hypothesis is "I expect weight loss to take longer than six weeks."
This can be written mathematically as: H 1 : μ > 6
In this example, μ is the average.
Now, the null hypothesis is what you expect if this hypothesis does not happen. In this case, if weight loss isn't achieved in greater than six weeks, then it must occur at a time equal to or less than six weeks. This can be written mathematically as:
H 0 : μ ≤ 6
The other way to state the null hypothesis is to make no assumption about the outcome of the experiment. In this case, the null hypothesis is simply that the treatment or change will have no effect on the outcome of the experiment. For this example, it would be that reducing the number of workouts would not affect the time needed to achieve weight loss:
H 0 : μ = 6
- Null Hypothesis Examples
"Hyperactivity is unrelated to eating sugar " is an example of a null hypothesis. If the hypothesis is tested and found to be false, using statistics, then a connection between hyperactivity and sugar ingestion may be indicated. A significance test is the most common statistical test used to establish confidence in a null hypothesis.
Another example of a null hypothesis is "Plant growth rate is unaffected by the presence of cadmium in the soil ." A researcher could test the hypothesis by measuring the growth rate of plants grown in a medium lacking cadmium, compared with the growth rate of plants grown in mediums containing different amounts of cadmium. Disproving the null hypothesis would set the groundwork for further research into the effects of different concentrations of the element in soil.
Why Test a Null Hypothesis?
You may be wondering why you would want to test a hypothesis just to find it false. Why not just test an alternate hypothesis and find it true? The short answer is that it is part of the scientific method. In science, propositions are not explicitly "proven." Rather, science uses math to determine the probability that a statement is true or false. It turns out it's much easier to disprove a hypothesis than to positively prove one. Also, while the null hypothesis may be simply stated, there's a good chance the alternate hypothesis is incorrect.
For example, if your null hypothesis is that plant growth is unaffected by duration of sunlight, you could state the alternate hypothesis in several different ways. Some of these statements might be incorrect. You could say plants are harmed by more than 12 hours of sunlight or that plants need at least three hours of sunlight, etc. There are clear exceptions to those alternate hypotheses, so if you test the wrong plants, you could reach the wrong conclusion. The null hypothesis is a general statement that can be used to develop an alternate hypothesis, which may or may not be correct.
- Difference Between Independent and Dependent Variables
- Examples of Independent and Dependent Variables
- What Are Examples of a Hypothesis?
- What Is a Hypothesis? (Science)
- What 'Fail to Reject' Means in a Hypothesis Test
- What Are the Elements of a Good Hypothesis?
- Null Hypothesis and Alternative Hypothesis
- Scientific Hypothesis Examples
- What Is a Control Group?
- Understanding Simple vs Controlled Experiments
- Six Steps of the Scientific Method
- Scientific Method Vocabulary Terms
- Definition of a Hypothesis
- How to Conduct a Hypothesis Test
- Type I and Type II Errors in Statistics
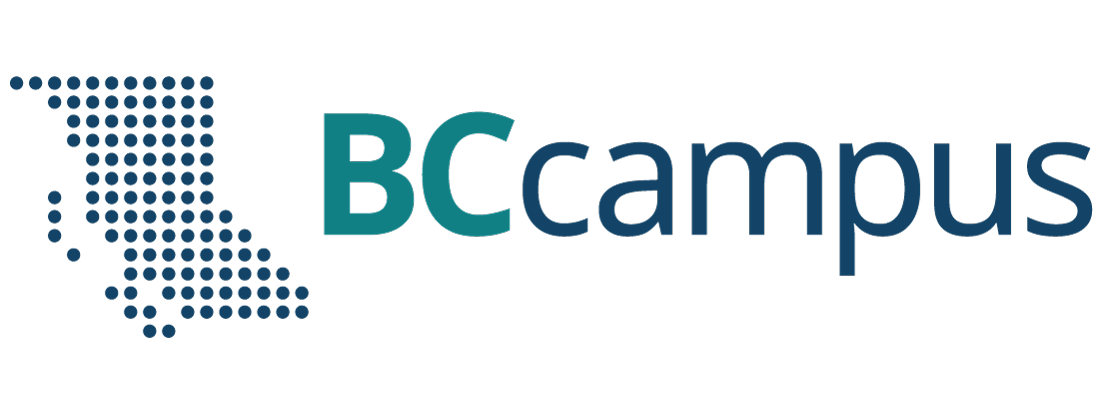
Want to create or adapt books like this? Learn more about how Pressbooks supports open publishing practices.
Chapter 13: Inferential Statistics
Understanding Null Hypothesis Testing
Learning Objectives
- Explain the purpose of null hypothesis testing, including the role of sampling error.
- Describe the basic logic of null hypothesis testing.
- Describe the role of relationship strength and sample size in determining statistical significance and make reasonable judgments about statistical significance based on these two factors.
The Purpose of Null Hypothesis Testing
As we have seen, psychological research typically involves measuring one or more variables for a sample and computing descriptive statistics for that sample. In general, however, the researcher’s goal is not to draw conclusions about that sample but to draw conclusions about the population that the sample was selected from. Thus researchers must use sample statistics to draw conclusions about the corresponding values in the population. These corresponding values in the population are called parameters . Imagine, for example, that a researcher measures the number of depressive symptoms exhibited by each of 50 clinically depressed adults and computes the mean number of symptoms. The researcher probably wants to use this sample statistic (the mean number of symptoms for the sample) to draw conclusions about the corresponding population parameter (the mean number of symptoms for clinically depressed adults).
Unfortunately, sample statistics are not perfect estimates of their corresponding population parameters. This is because there is a certain amount of random variability in any statistic from sample to sample. The mean number of depressive symptoms might be 8.73 in one sample of clinically depressed adults, 6.45 in a second sample, and 9.44 in a third—even though these samples are selected randomly from the same population. Similarly, the correlation (Pearson’s r ) between two variables might be +.24 in one sample, −.04 in a second sample, and +.15 in a third—again, even though these samples are selected randomly from the same population. This random variability in a statistic from sample to sample is called sampling error . (Note that the term error here refers to random variability and does not imply that anyone has made a mistake. No one “commits a sampling error.”)
One implication of this is that when there is a statistical relationship in a sample, it is not always clear that there is a statistical relationship in the population. A small difference between two group means in a sample might indicate that there is a small difference between the two group means in the population. But it could also be that there is no difference between the means in the population and that the difference in the sample is just a matter of sampling error. Similarly, a Pearson’s r value of −.29 in a sample might mean that there is a negative relationship in the population. But it could also be that there is no relationship in the population and that the relationship in the sample is just a matter of sampling error.
In fact, any statistical relationship in a sample can be interpreted in two ways:
- There is a relationship in the population, and the relationship in the sample reflects this.
- There is no relationship in the population, and the relationship in the sample reflects only sampling error.
The purpose of null hypothesis testing is simply to help researchers decide between these two interpretations.
The Logic of Null Hypothesis Testing
Null hypothesis testing is a formal approach to deciding between two interpretations of a statistical relationship in a sample. One interpretation is called the null hypothesis (often symbolized H 0 and read as “H-naught”). This is the idea that there is no relationship in the population and that the relationship in the sample reflects only sampling error. Informally, the null hypothesis is that the sample relationship “occurred by chance.” The other interpretation is called the alternative hypothesis (often symbolized as H 1 ). This is the idea that there is a relationship in the population and that the relationship in the sample reflects this relationship in the population.
Again, every statistical relationship in a sample can be interpreted in either of these two ways: It might have occurred by chance, or it might reflect a relationship in the population. So researchers need a way to decide between them. Although there are many specific null hypothesis testing techniques, they are all based on the same general logic. The steps are as follows:
- Assume for the moment that the null hypothesis is true. There is no relationship between the variables in the population.
- Determine how likely the sample relationship would be if the null hypothesis were true.
- If the sample relationship would be extremely unlikely, then reject the null hypothesis in favour of the alternative hypothesis. If it would not be extremely unlikely, then retain the null hypothesis .
Following this logic, we can begin to understand why Mehl and his colleagues concluded that there is no difference in talkativeness between women and men in the population. In essence, they asked the following question: “If there were no difference in the population, how likely is it that we would find a small difference of d = 0.06 in our sample?” Their answer to this question was that this sample relationship would be fairly likely if the null hypothesis were true. Therefore, they retained the null hypothesis—concluding that there is no evidence of a sex difference in the population. We can also see why Kanner and his colleagues concluded that there is a correlation between hassles and symptoms in the population. They asked, “If the null hypothesis were true, how likely is it that we would find a strong correlation of +.60 in our sample?” Their answer to this question was that this sample relationship would be fairly unlikely if the null hypothesis were true. Therefore, they rejected the null hypothesis in favour of the alternative hypothesis—concluding that there is a positive correlation between these variables in the population.
A crucial step in null hypothesis testing is finding the likelihood of the sample result if the null hypothesis were true. This probability is called the p value . A low p value means that the sample result would be unlikely if the null hypothesis were true and leads to the rejection of the null hypothesis. A high p value means that the sample result would be likely if the null hypothesis were true and leads to the retention of the null hypothesis. But how low must the p value be before the sample result is considered unlikely enough to reject the null hypothesis? In null hypothesis testing, this criterion is called α (alpha) and is almost always set to .05. If there is less than a 5% chance of a result as extreme as the sample result if the null hypothesis were true, then the null hypothesis is rejected. When this happens, the result is said to be statistically significant . If there is greater than a 5% chance of a result as extreme as the sample result when the null hypothesis is true, then the null hypothesis is retained. This does not necessarily mean that the researcher accepts the null hypothesis as true—only that there is not currently enough evidence to conclude that it is true. Researchers often use the expression “fail to reject the null hypothesis” rather than “retain the null hypothesis,” but they never use the expression “accept the null hypothesis.”
The Misunderstood p Value
The p value is one of the most misunderstood quantities in psychological research (Cohen, 1994) [1] . Even professional researchers misinterpret it, and it is not unusual for such misinterpretations to appear in statistics textbooks!
The most common misinterpretation is that the p value is the probability that the null hypothesis is true—that the sample result occurred by chance. For example, a misguided researcher might say that because the p value is .02, there is only a 2% chance that the result is due to chance and a 98% chance that it reflects a real relationship in the population. But this is incorrect . The p value is really the probability of a result at least as extreme as the sample result if the null hypothesis were true. So a p value of .02 means that if the null hypothesis were true, a sample result this extreme would occur only 2% of the time.
You can avoid this misunderstanding by remembering that the p value is not the probability that any particular hypothesis is true or false. Instead, it is the probability of obtaining the sample result if the null hypothesis were true.
Role of Sample Size and Relationship Strength
Recall that null hypothesis testing involves answering the question, “If the null hypothesis were true, what is the probability of a sample result as extreme as this one?” In other words, “What is the p value?” It can be helpful to see that the answer to this question depends on just two considerations: the strength of the relationship and the size of the sample. Specifically, the stronger the sample relationship and the larger the sample, the less likely the result would be if the null hypothesis were true. That is, the lower the p value. This should make sense. Imagine a study in which a sample of 500 women is compared with a sample of 500 men in terms of some psychological characteristic, and Cohen’s d is a strong 0.50. If there were really no sex difference in the population, then a result this strong based on such a large sample should seem highly unlikely. Now imagine a similar study in which a sample of three women is compared with a sample of three men, and Cohen’s d is a weak 0.10. If there were no sex difference in the population, then a relationship this weak based on such a small sample should seem likely. And this is precisely why the null hypothesis would be rejected in the first example and retained in the second.
Of course, sometimes the result can be weak and the sample large, or the result can be strong and the sample small. In these cases, the two considerations trade off against each other so that a weak result can be statistically significant if the sample is large enough and a strong relationship can be statistically significant even if the sample is small. Table 13.1 shows roughly how relationship strength and sample size combine to determine whether a sample result is statistically significant. The columns of the table represent the three levels of relationship strength: weak, medium, and strong. The rows represent four sample sizes that can be considered small, medium, large, and extra large in the context of psychological research. Thus each cell in the table represents a combination of relationship strength and sample size. If a cell contains the word Yes , then this combination would be statistically significant for both Cohen’s d and Pearson’s r . If it contains the word No , then it would not be statistically significant for either. There is one cell where the decision for d and r would be different and another where it might be different depending on some additional considerations, which are discussed in Section 13.2 “Some Basic Null Hypothesis Tests”
Although Table 13.1 provides only a rough guideline, it shows very clearly that weak relationships based on medium or small samples are never statistically significant and that strong relationships based on medium or larger samples are always statistically significant. If you keep this lesson in mind, you will often know whether a result is statistically significant based on the descriptive statistics alone. It is extremely useful to be able to develop this kind of intuitive judgment. One reason is that it allows you to develop expectations about how your formal null hypothesis tests are going to come out, which in turn allows you to detect problems in your analyses. For example, if your sample relationship is strong and your sample is medium, then you would expect to reject the null hypothesis. If for some reason your formal null hypothesis test indicates otherwise, then you need to double-check your computations and interpretations. A second reason is that the ability to make this kind of intuitive judgment is an indication that you understand the basic logic of this approach in addition to being able to do the computations.
Statistical Significance Versus Practical Significance
Table 13.1 illustrates another extremely important point. A statistically significant result is not necessarily a strong one. Even a very weak result can be statistically significant if it is based on a large enough sample. This is closely related to Janet Shibley Hyde’s argument about sex differences (Hyde, 2007) [2] . The differences between women and men in mathematical problem solving and leadership ability are statistically significant. But the word significant can cause people to interpret these differences as strong and important—perhaps even important enough to influence the college courses they take or even who they vote for. As we have seen, however, these statistically significant differences are actually quite weak—perhaps even “trivial.”
This is why it is important to distinguish between the statistical significance of a result and the practical significance of that result. Practical significance refers to the importance or usefulness of the result in some real-world context. Many sex differences are statistically significant—and may even be interesting for purely scientific reasons—but they are not practically significant. In clinical practice, this same concept is often referred to as “clinical significance.” For example, a study on a new treatment for social phobia might show that it produces a statistically significant positive effect. Yet this effect still might not be strong enough to justify the time, effort, and other costs of putting it into practice—especially if easier and cheaper treatments that work almost as well already exist. Although statistically significant, this result would be said to lack practical or clinical significance.
Key Takeaways
- Null hypothesis testing is a formal approach to deciding whether a statistical relationship in a sample reflects a real relationship in the population or is just due to chance.
- The logic of null hypothesis testing involves assuming that the null hypothesis is true, finding how likely the sample result would be if this assumption were correct, and then making a decision. If the sample result would be unlikely if the null hypothesis were true, then it is rejected in favour of the alternative hypothesis. If it would not be unlikely, then the null hypothesis is retained.
- The probability of obtaining the sample result if the null hypothesis were true (the p value) is based on two considerations: relationship strength and sample size. Reasonable judgments about whether a sample relationship is statistically significant can often be made by quickly considering these two factors.
- Statistical significance is not the same as relationship strength or importance. Even weak relationships can be statistically significant if the sample size is large enough. It is important to consider relationship strength and the practical significance of a result in addition to its statistical significance.
- Discussion: Imagine a study showing that people who eat more broccoli tend to be happier. Explain for someone who knows nothing about statistics why the researchers would conduct a null hypothesis test.
- The correlation between two variables is r = −.78 based on a sample size of 137.
- The mean score on a psychological characteristic for women is 25 ( SD = 5) and the mean score for men is 24 ( SD = 5). There were 12 women and 10 men in this study.
- In a memory experiment, the mean number of items recalled by the 40 participants in Condition A was 0.50 standard deviations greater than the mean number recalled by the 40 participants in Condition B.
- In another memory experiment, the mean scores for participants in Condition A and Condition B came out exactly the same!
- A student finds a correlation of r = .04 between the number of units the students in his research methods class are taking and the students’ level of stress.
Long Descriptions
“Null Hypothesis” long description: A comic depicting a man and a woman talking in the foreground. In the background is a child working at a desk. The man says to the woman, “I can’t believe schools are still teaching kids about the null hypothesis. I remember reading a big study that conclusively disproved it years ago.” [Return to “Null Hypothesis”]
“Conditional Risk” long description: A comic depicting two hikers beside a tree during a thunderstorm. A bolt of lightning goes “crack” in the dark sky as thunder booms. One of the hikers says, “Whoa! We should get inside!” The other hiker says, “It’s okay! Lightning only kills about 45 Americans a year, so the chances of dying are only one in 7,000,000. Let’s go on!” The comic’s caption says, “The annual death rate among people who know that statistic is one in six.” [Return to “Conditional Risk”]
Media Attributions
- Null Hypothesis by XKCD CC BY-NC (Attribution NonCommercial)
- Conditional Risk by XKCD CC BY-NC (Attribution NonCommercial)
- Cohen, J. (1994). The world is round: p < .05. American Psychologist, 49 , 997–1003. ↵
- Hyde, J. S. (2007). New directions in the study of gender similarities and differences. Current Directions in Psychological Science, 16 , 259–263. ↵
Values in a population that correspond to variables measured in a study.
The random variability in a statistic from sample to sample.
A formal approach to deciding between two interpretations of a statistical relationship in a sample.
The idea that there is no relationship in the population and that the relationship in the sample reflects only sampling error.
The idea that there is a relationship in the population and that the relationship in the sample reflects this relationship in the population.
When the relationship found in the sample would be extremely unlikely, the idea that the relationship occurred “by chance” is rejected.
When the relationship found in the sample is likely to have occurred by chance, the null hypothesis is not rejected.
The probability that, if the null hypothesis were true, the result found in the sample would occur.
How low the p value must be before the sample result is considered unlikely in null hypothesis testing.
When there is less than a 5% chance of a result as extreme as the sample result occurring and the null hypothesis is rejected.
Research Methods in Psychology - 2nd Canadian Edition Copyright © 2015 by Paul C. Price, Rajiv Jhangiani, & I-Chant A. Chiang is licensed under a Creative Commons Attribution-NonCommercial-ShareAlike 4.0 International License , except where otherwise noted.
Share This Book
Have a thesis expert improve your writing
Check your thesis for plagiarism in 10 minutes, generate your apa citations for free.
- Knowledge Base
- Null and Alternative Hypotheses | Definitions & Examples
Null and Alternative Hypotheses | Definitions & Examples
Published on 5 October 2022 by Shaun Turney . Revised on 6 December 2022.
The null and alternative hypotheses are two competing claims that researchers weigh evidence for and against using a statistical test :
- Null hypothesis (H 0 ): There’s no effect in the population .
- Alternative hypothesis (H A ): There’s an effect in the population.
The effect is usually the effect of the independent variable on the dependent variable .
Table of contents
Answering your research question with hypotheses, what is a null hypothesis, what is an alternative hypothesis, differences between null and alternative hypotheses, how to write null and alternative hypotheses, frequently asked questions about null and alternative hypotheses.
The null and alternative hypotheses offer competing answers to your research question . When the research question asks “Does the independent variable affect the dependent variable?”, the null hypothesis (H 0 ) answers “No, there’s no effect in the population.” On the other hand, the alternative hypothesis (H A ) answers “Yes, there is an effect in the population.”
The null and alternative are always claims about the population. That’s because the goal of hypothesis testing is to make inferences about a population based on a sample . Often, we infer whether there’s an effect in the population by looking at differences between groups or relationships between variables in the sample.
You can use a statistical test to decide whether the evidence favors the null or alternative hypothesis. Each type of statistical test comes with a specific way of phrasing the null and alternative hypothesis. However, the hypotheses can also be phrased in a general way that applies to any test.
The null hypothesis is the claim that there’s no effect in the population.
If the sample provides enough evidence against the claim that there’s no effect in the population ( p ≤ α), then we can reject the null hypothesis . Otherwise, we fail to reject the null hypothesis.
Although “fail to reject” may sound awkward, it’s the only wording that statisticians accept. Be careful not to say you “prove” or “accept” the null hypothesis.
Null hypotheses often include phrases such as “no effect”, “no difference”, or “no relationship”. When written in mathematical terms, they always include an equality (usually =, but sometimes ≥ or ≤).
Examples of null hypotheses
The table below gives examples of research questions and null hypotheses. There’s always more than one way to answer a research question, but these null hypotheses can help you get started.
*Note that some researchers prefer to always write the null hypothesis in terms of “no effect” and “=”. It would be fine to say that daily meditation has no effect on the incidence of depression and p 1 = p 2 .
The alternative hypothesis (H A ) is the other answer to your research question . It claims that there’s an effect in the population.
Often, your alternative hypothesis is the same as your research hypothesis. In other words, it’s the claim that you expect or hope will be true.
The alternative hypothesis is the complement to the null hypothesis. Null and alternative hypotheses are exhaustive, meaning that together they cover every possible outcome. They are also mutually exclusive, meaning that only one can be true at a time.
Alternative hypotheses often include phrases such as “an effect”, “a difference”, or “a relationship”. When alternative hypotheses are written in mathematical terms, they always include an inequality (usually ≠, but sometimes > or <). As with null hypotheses, there are many acceptable ways to phrase an alternative hypothesis.
Examples of alternative hypotheses
The table below gives examples of research questions and alternative hypotheses to help you get started with formulating your own.
Null and alternative hypotheses are similar in some ways:
- They’re both answers to the research question
- They both make claims about the population
- They’re both evaluated by statistical tests.
However, there are important differences between the two types of hypotheses, summarized in the following table.
To help you write your hypotheses, you can use the template sentences below. If you know which statistical test you’re going to use, you can use the test-specific template sentences. Otherwise, you can use the general template sentences.
The only thing you need to know to use these general template sentences are your dependent and independent variables. To write your research question, null hypothesis, and alternative hypothesis, fill in the following sentences with your variables:
Does independent variable affect dependent variable ?
- Null hypothesis (H 0 ): Independent variable does not affect dependent variable .
- Alternative hypothesis (H A ): Independent variable affects dependent variable .
Test-specific
Once you know the statistical test you’ll be using, you can write your hypotheses in a more precise and mathematical way specific to the test you chose. The table below provides template sentences for common statistical tests.
Note: The template sentences above assume that you’re performing one-tailed tests . One-tailed tests are appropriate for most studies.
The null hypothesis is often abbreviated as H 0 . When the null hypothesis is written using mathematical symbols, it always includes an equality symbol (usually =, but sometimes ≥ or ≤).
The alternative hypothesis is often abbreviated as H a or H 1 . When the alternative hypothesis is written using mathematical symbols, it always includes an inequality symbol (usually ≠, but sometimes < or >).
A research hypothesis is your proposed answer to your research question. The research hypothesis usually includes an explanation (‘ x affects y because …’).
A statistical hypothesis, on the other hand, is a mathematical statement about a population parameter. Statistical hypotheses always come in pairs: the null and alternative hypotheses. In a well-designed study , the statistical hypotheses correspond logically to the research hypothesis.
Cite this Scribbr article
If you want to cite this source, you can copy and paste the citation or click the ‘Cite this Scribbr article’ button to automatically add the citation to our free Reference Generator.
Turney, S. (2022, December 06). Null and Alternative Hypotheses | Definitions & Examples. Scribbr. Retrieved 31 May 2024, from https://www.scribbr.co.uk/stats/null-and-alternative-hypothesis/
Is this article helpful?
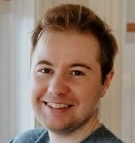
Shaun Turney
Other students also liked, levels of measurement: nominal, ordinal, interval, ratio, the standard normal distribution | calculator, examples & uses, types of variables in research | definitions & examples.
Null Hypothesis
- Reference work entry
- First Online: 01 January 2020
- pp 3267–3270
- Cite this reference work entry
- Tom Booth 3 ,
- Alex Doumas 3 &
- Aja Louise Murray 4
32 Accesses
In formal hypothesis testing, the null hypothesis ( H 0 ) is the hypothesis assumed to be true in the population and which gives rise to the sampling distribution of the test statistic in question (Hays 1994 ). The critical feature of the null hypothesis across hypothesis testing frameworks is that it is stated with enough precision that it can be tested.
Introduction
A hypothesis is a statement or explanation about the nature or causes of some phenomena of interest. In the process of scientific study, we can distinguish two forms of hypotheses. A research hypothesis poses the question of interest, and if well stated, will include the variables under study and the expected relationship between them. A statistical hypothesis translates the research hypothesis into a mathematically precise, statistically testable statement concerning the assumed value of a parameter of interest in the population. The null hypothesis is an example of a statistical hypothesis.
In order to test these...
This is a preview of subscription content, log in via an institution to check access.
Access this chapter
- Available as PDF
- Read on any device
- Instant download
- Own it forever
- Available as EPUB and PDF
- Durable hardcover edition
- Dispatched in 3 to 5 business days
- Free shipping worldwide - see info
Tax calculation will be finalised at checkout
Purchases are for personal use only
Institutional subscriptions
Fisher, R. (1925). Statistical methods for research workers (1st ed.). Edinburgh: Oliver and Boyd.
Google Scholar
Gigerenzer, G. (2004). Mindless statistics. The Journal of Socio-Economics, 33 , 587–606.
Article Google Scholar
Hays, W. L. (1994). Statistics (5th ed.). Belmont: Wadsworth.
Neyman, J., & Pearson, E. S. (1933). On the problem of the most efficient tests of statistical hypotheses. Philosophical Transactions of the Royal Society of London, Series A, 231 , 289–337.
Szucs, D., & Ioannidis, J. P. A. (2016). When null hypothesis significance testing is unsuitable for research: A reassessment. bioRxiv . https://doi.org/10.1101/095570 .
Download references
Author information
Authors and affiliations.
Department of Psychology, University of Edinburgh, Edinburgh, UK
Tom Booth & Alex Doumas
Violence Research Centre, Institute of Criminology, University of Cambridge, Cambridge, UK
Aja Louise Murray
You can also search for this author in PubMed Google Scholar
Corresponding author
Correspondence to Tom Booth .
Editor information
Editors and affiliations.
Oakland University, Rochester, MI, USA
Virgil Zeigler-Hill
Todd K. Shackelford
Section Editor information
Humboldt University, Germany, Berlin, Germany
Matthias Ziegler
Rights and permissions
Reprints and permissions
Copyright information
© 2020 Springer Nature Switzerland AG
About this entry
Cite this entry.
Booth, T., Doumas, A., Murray, A.L. (2020). Null Hypothesis. In: Zeigler-Hill, V., Shackelford, T.K. (eds) Encyclopedia of Personality and Individual Differences. Springer, Cham. https://doi.org/10.1007/978-3-319-24612-3_1335
Download citation
DOI : https://doi.org/10.1007/978-3-319-24612-3_1335
Published : 22 April 2020
Publisher Name : Springer, Cham
Print ISBN : 978-3-319-24610-9
Online ISBN : 978-3-319-24612-3
eBook Packages : Behavioral Science and Psychology Reference Module Humanities and Social Sciences Reference Module Business, Economics and Social Sciences
Share this entry
Anyone you share the following link with will be able to read this content:
Sorry, a shareable link is not currently available for this article.
Provided by the Springer Nature SharedIt content-sharing initiative
- Publish with us
Policies and ethics
- Find a journal
- Track your research
Research Hypothesis In Psychology: Types, & Examples
Saul Mcleod, PhD
Editor-in-Chief for Simply Psychology
BSc (Hons) Psychology, MRes, PhD, University of Manchester
Saul Mcleod, PhD., is a qualified psychology teacher with over 18 years of experience in further and higher education. He has been published in peer-reviewed journals, including the Journal of Clinical Psychology.
Learn about our Editorial Process
Olivia Guy-Evans, MSc
Associate Editor for Simply Psychology
BSc (Hons) Psychology, MSc Psychology of Education
Olivia Guy-Evans is a writer and associate editor for Simply Psychology. She has previously worked in healthcare and educational sectors.
On This Page:
A research hypothesis, in its plural form “hypotheses,” is a specific, testable prediction about the anticipated results of a study, established at its outset. It is a key component of the scientific method .
Hypotheses connect theory to data and guide the research process towards expanding scientific understanding
Some key points about hypotheses:
- A hypothesis expresses an expected pattern or relationship. It connects the variables under investigation.
- It is stated in clear, precise terms before any data collection or analysis occurs. This makes the hypothesis testable.
- A hypothesis must be falsifiable. It should be possible, even if unlikely in practice, to collect data that disconfirms rather than supports the hypothesis.
- Hypotheses guide research. Scientists design studies to explicitly evaluate hypotheses about how nature works.
- For a hypothesis to be valid, it must be testable against empirical evidence. The evidence can then confirm or disprove the testable predictions.
- Hypotheses are informed by background knowledge and observation, but go beyond what is already known to propose an explanation of how or why something occurs.
Predictions typically arise from a thorough knowledge of the research literature, curiosity about real-world problems or implications, and integrating this to advance theory. They build on existing literature while providing new insight.
Types of Research Hypotheses
Alternative hypothesis.
The research hypothesis is often called the alternative or experimental hypothesis in experimental research.
It typically suggests a potential relationship between two key variables: the independent variable, which the researcher manipulates, and the dependent variable, which is measured based on those changes.
The alternative hypothesis states a relationship exists between the two variables being studied (one variable affects the other).
A hypothesis is a testable statement or prediction about the relationship between two or more variables. It is a key component of the scientific method. Some key points about hypotheses:
- Important hypotheses lead to predictions that can be tested empirically. The evidence can then confirm or disprove the testable predictions.
In summary, a hypothesis is a precise, testable statement of what researchers expect to happen in a study and why. Hypotheses connect theory to data and guide the research process towards expanding scientific understanding.
An experimental hypothesis predicts what change(s) will occur in the dependent variable when the independent variable is manipulated.
It states that the results are not due to chance and are significant in supporting the theory being investigated.
The alternative hypothesis can be directional, indicating a specific direction of the effect, or non-directional, suggesting a difference without specifying its nature. It’s what researchers aim to support or demonstrate through their study.
Null Hypothesis
The null hypothesis states no relationship exists between the two variables being studied (one variable does not affect the other). There will be no changes in the dependent variable due to manipulating the independent variable.
It states results are due to chance and are not significant in supporting the idea being investigated.
The null hypothesis, positing no effect or relationship, is a foundational contrast to the research hypothesis in scientific inquiry. It establishes a baseline for statistical testing, promoting objectivity by initiating research from a neutral stance.
Many statistical methods are tailored to test the null hypothesis, determining the likelihood of observed results if no true effect exists.
This dual-hypothesis approach provides clarity, ensuring that research intentions are explicit, and fosters consistency across scientific studies, enhancing the standardization and interpretability of research outcomes.
Nondirectional Hypothesis
A non-directional hypothesis, also known as a two-tailed hypothesis, predicts that there is a difference or relationship between two variables but does not specify the direction of this relationship.
It merely indicates that a change or effect will occur without predicting which group will have higher or lower values.
For example, “There is a difference in performance between Group A and Group B” is a non-directional hypothesis.
Directional Hypothesis
A directional (one-tailed) hypothesis predicts the nature of the effect of the independent variable on the dependent variable. It predicts in which direction the change will take place. (i.e., greater, smaller, less, more)
It specifies whether one variable is greater, lesser, or different from another, rather than just indicating that there’s a difference without specifying its nature.
For example, “Exercise increases weight loss” is a directional hypothesis.
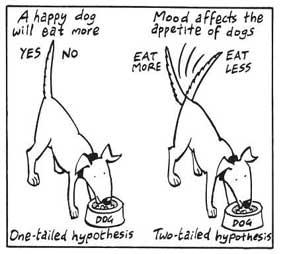
Falsifiability
The Falsification Principle, proposed by Karl Popper , is a way of demarcating science from non-science. It suggests that for a theory or hypothesis to be considered scientific, it must be testable and irrefutable.
Falsifiability emphasizes that scientific claims shouldn’t just be confirmable but should also have the potential to be proven wrong.
It means that there should exist some potential evidence or experiment that could prove the proposition false.
However many confirming instances exist for a theory, it only takes one counter observation to falsify it. For example, the hypothesis that “all swans are white,” can be falsified by observing a black swan.
For Popper, science should attempt to disprove a theory rather than attempt to continually provide evidence to support a research hypothesis.
Can a Hypothesis be Proven?
Hypotheses make probabilistic predictions. They state the expected outcome if a particular relationship exists. However, a study result supporting a hypothesis does not definitively prove it is true.
All studies have limitations. There may be unknown confounding factors or issues that limit the certainty of conclusions. Additional studies may yield different results.
In science, hypotheses can realistically only be supported with some degree of confidence, not proven. The process of science is to incrementally accumulate evidence for and against hypothesized relationships in an ongoing pursuit of better models and explanations that best fit the empirical data. But hypotheses remain open to revision and rejection if that is where the evidence leads.
- Disproving a hypothesis is definitive. Solid disconfirmatory evidence will falsify a hypothesis and require altering or discarding it based on the evidence.
- However, confirming evidence is always open to revision. Other explanations may account for the same results, and additional or contradictory evidence may emerge over time.
We can never 100% prove the alternative hypothesis. Instead, we see if we can disprove, or reject the null hypothesis.
If we reject the null hypothesis, this doesn’t mean that our alternative hypothesis is correct but does support the alternative/experimental hypothesis.
Upon analysis of the results, an alternative hypothesis can be rejected or supported, but it can never be proven to be correct. We must avoid any reference to results proving a theory as this implies 100% certainty, and there is always a chance that evidence may exist which could refute a theory.
How to Write a Hypothesis
- Identify variables . The researcher manipulates the independent variable and the dependent variable is the measured outcome.
- Operationalized the variables being investigated . Operationalization of a hypothesis refers to the process of making the variables physically measurable or testable, e.g. if you are about to study aggression, you might count the number of punches given by participants.
- Decide on a direction for your prediction . If there is evidence in the literature to support a specific effect of the independent variable on the dependent variable, write a directional (one-tailed) hypothesis. If there are limited or ambiguous findings in the literature regarding the effect of the independent variable on the dependent variable, write a non-directional (two-tailed) hypothesis.
- Make it Testable : Ensure your hypothesis can be tested through experimentation or observation. It should be possible to prove it false (principle of falsifiability).
- Clear & concise language . A strong hypothesis is concise (typically one to two sentences long), and formulated using clear and straightforward language, ensuring it’s easily understood and testable.
Consider a hypothesis many teachers might subscribe to: students work better on Monday morning than on Friday afternoon (IV=Day, DV= Standard of work).
Now, if we decide to study this by giving the same group of students a lesson on a Monday morning and a Friday afternoon and then measuring their immediate recall of the material covered in each session, we would end up with the following:
- The alternative hypothesis states that students will recall significantly more information on a Monday morning than on a Friday afternoon.
- The null hypothesis states that there will be no significant difference in the amount recalled on a Monday morning compared to a Friday afternoon. Any difference will be due to chance or confounding factors.
More Examples
- Memory : Participants exposed to classical music during study sessions will recall more items from a list than those who studied in silence.
- Social Psychology : Individuals who frequently engage in social media use will report higher levels of perceived social isolation compared to those who use it infrequently.
- Developmental Psychology : Children who engage in regular imaginative play have better problem-solving skills than those who don’t.
- Clinical Psychology : Cognitive-behavioral therapy will be more effective in reducing symptoms of anxiety over a 6-month period compared to traditional talk therapy.
- Cognitive Psychology : Individuals who multitask between various electronic devices will have shorter attention spans on focused tasks than those who single-task.
- Health Psychology : Patients who practice mindfulness meditation will experience lower levels of chronic pain compared to those who don’t meditate.
- Organizational Psychology : Employees in open-plan offices will report higher levels of stress than those in private offices.
- Behavioral Psychology : Rats rewarded with food after pressing a lever will press it more frequently than rats who receive no reward.

Related Articles
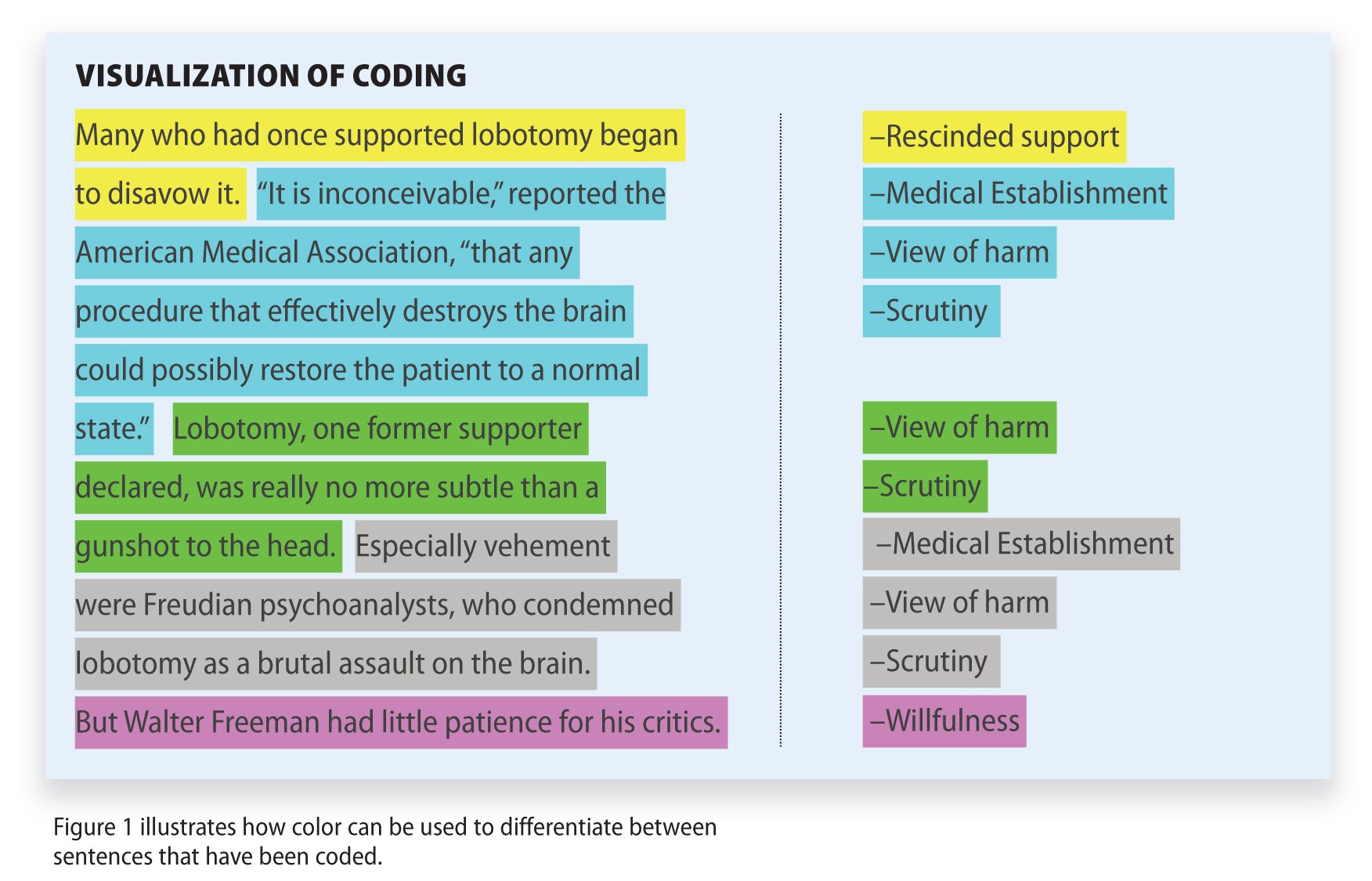
Research Methodology
Qualitative Data Coding
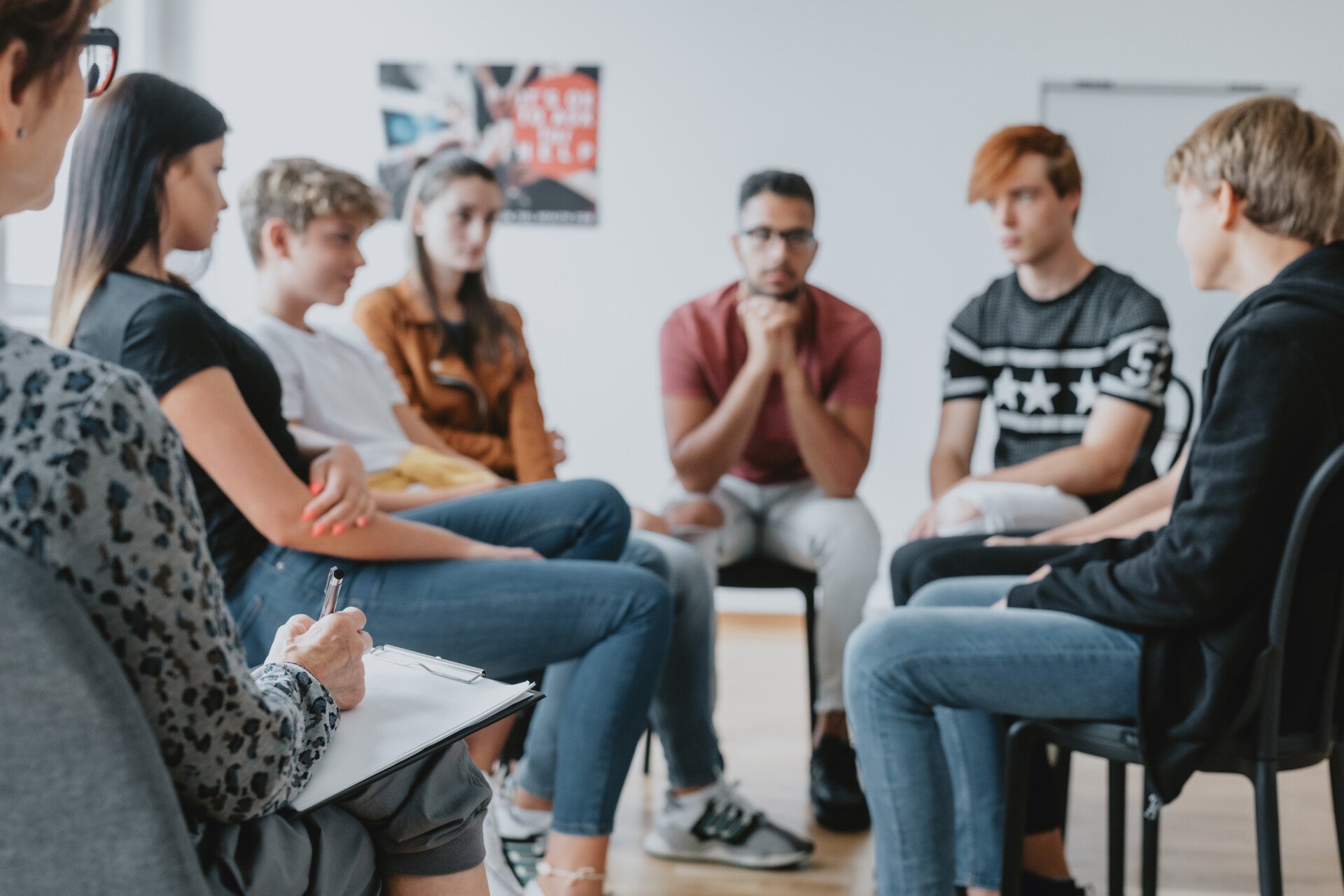
What Is a Focus Group?
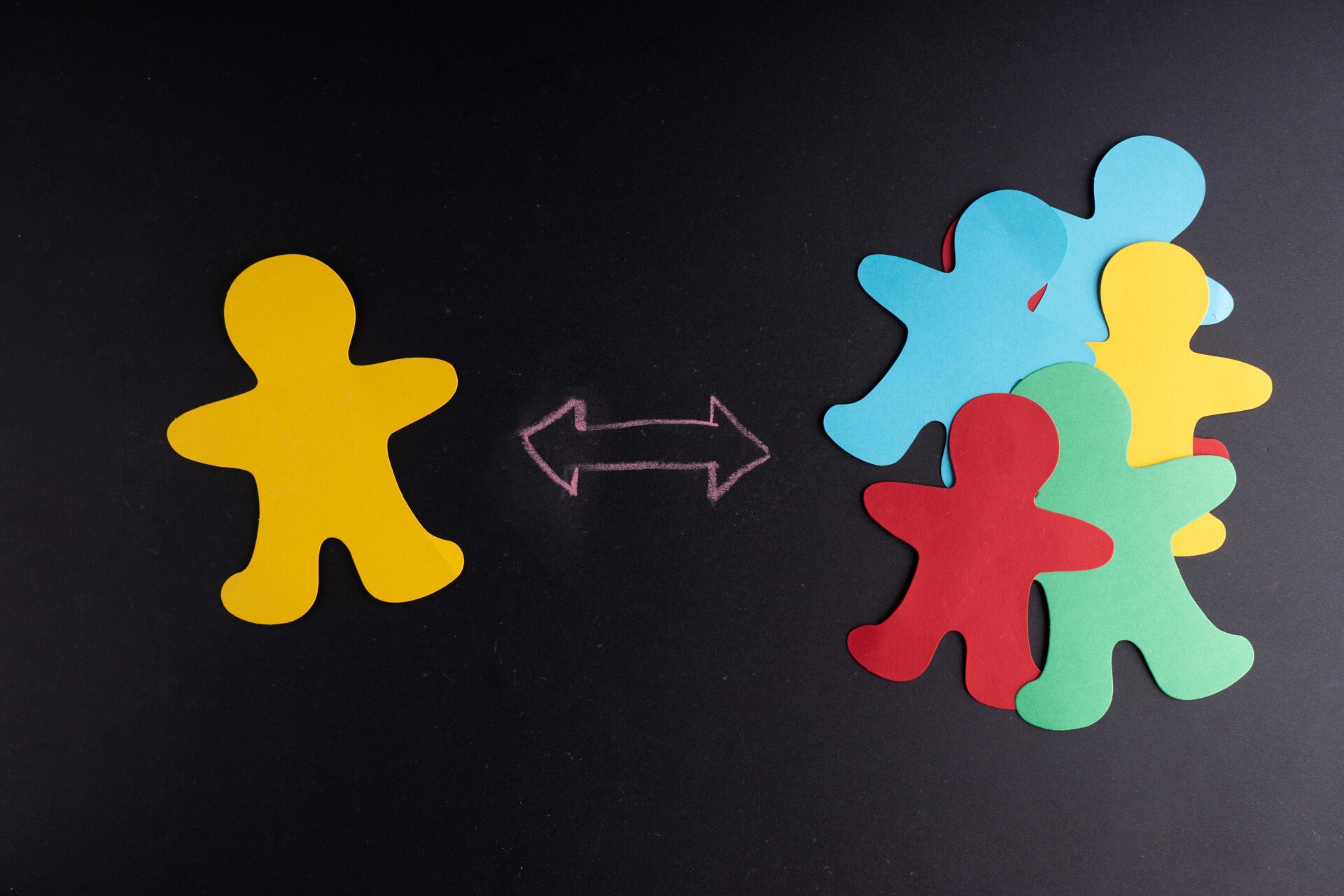
Cross-Cultural Research Methodology In Psychology
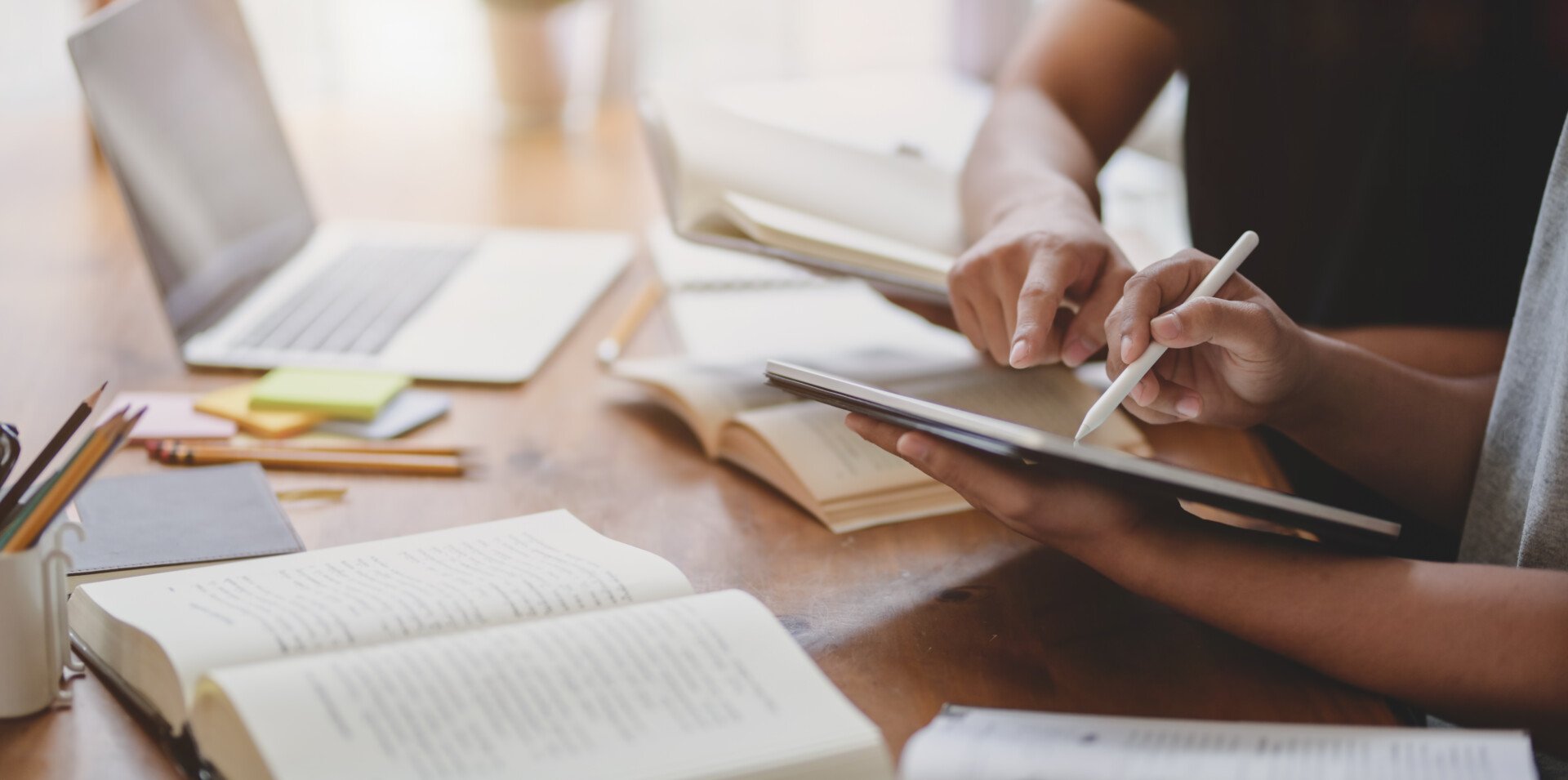
What Is Internal Validity In Research?
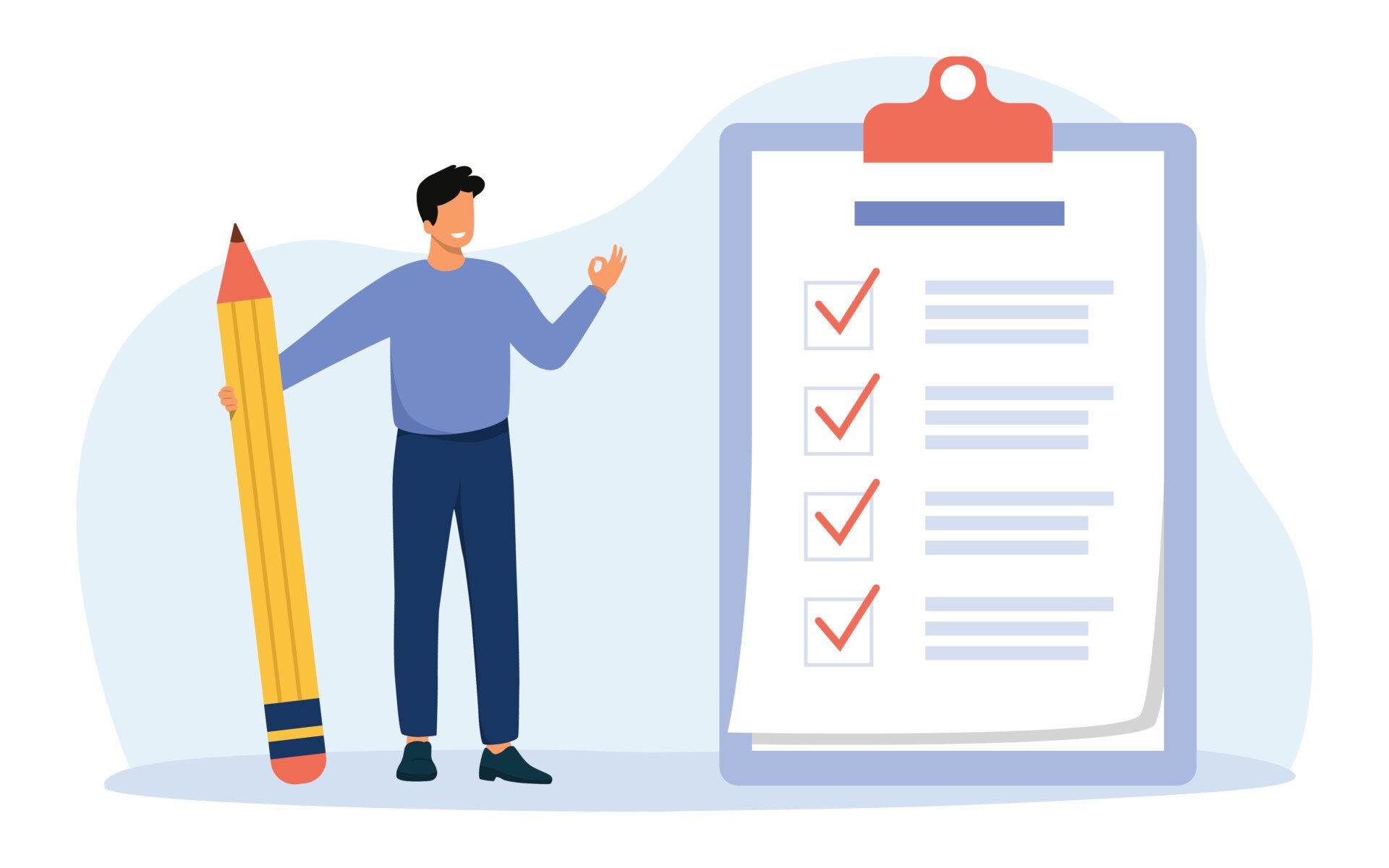
Research Methodology , Statistics
What Is Face Validity In Research? Importance & How To Measure
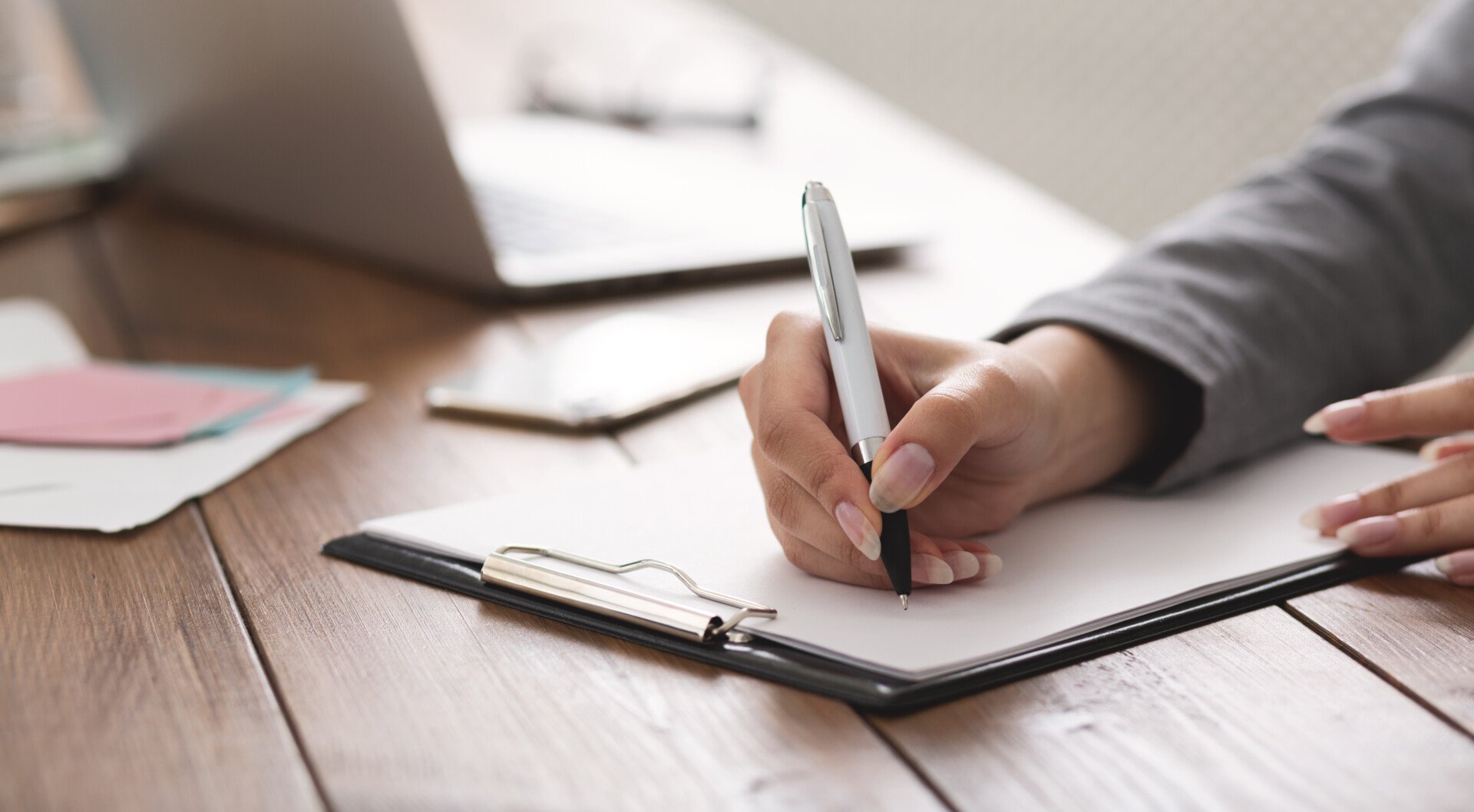
Criterion Validity: Definition & Examples
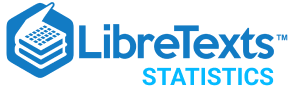
- school Campus Bookshelves
- menu_book Bookshelves
- perm_media Learning Objects
- login Login
- how_to_reg Request Instructor Account
- hub Instructor Commons
Margin Size
- Download Page (PDF)
- Download Full Book (PDF)
- Periodic Table
- Physics Constants
- Scientific Calculator
- Reference & Cite
- Tools expand_more
- Readability
selected template will load here
This action is not available.
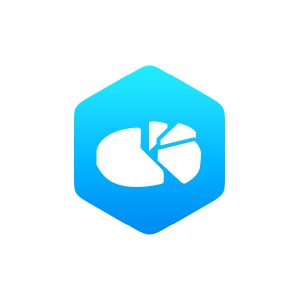
7.3: The Research Hypothesis and the Null Hypothesis
- Last updated
- Save as PDF
- Page ID 18038
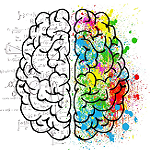
- Michelle Oja
- Taft College
\( \newcommand{\vecs}[1]{\overset { \scriptstyle \rightharpoonup} {\mathbf{#1}} } \)
\( \newcommand{\vecd}[1]{\overset{-\!-\!\rightharpoonup}{\vphantom{a}\smash {#1}}} \)
\( \newcommand{\id}{\mathrm{id}}\) \( \newcommand{\Span}{\mathrm{span}}\)
( \newcommand{\kernel}{\mathrm{null}\,}\) \( \newcommand{\range}{\mathrm{range}\,}\)
\( \newcommand{\RealPart}{\mathrm{Re}}\) \( \newcommand{\ImaginaryPart}{\mathrm{Im}}\)
\( \newcommand{\Argument}{\mathrm{Arg}}\) \( \newcommand{\norm}[1]{\| #1 \|}\)
\( \newcommand{\inner}[2]{\langle #1, #2 \rangle}\)
\( \newcommand{\Span}{\mathrm{span}}\)
\( \newcommand{\id}{\mathrm{id}}\)
\( \newcommand{\kernel}{\mathrm{null}\,}\)
\( \newcommand{\range}{\mathrm{range}\,}\)
\( \newcommand{\RealPart}{\mathrm{Re}}\)
\( \newcommand{\ImaginaryPart}{\mathrm{Im}}\)
\( \newcommand{\Argument}{\mathrm{Arg}}\)
\( \newcommand{\norm}[1]{\| #1 \|}\)
\( \newcommand{\Span}{\mathrm{span}}\) \( \newcommand{\AA}{\unicode[.8,0]{x212B}}\)
\( \newcommand{\vectorA}[1]{\vec{#1}} % arrow\)
\( \newcommand{\vectorAt}[1]{\vec{\text{#1}}} % arrow\)
\( \newcommand{\vectorB}[1]{\overset { \scriptstyle \rightharpoonup} {\mathbf{#1}} } \)
\( \newcommand{\vectorC}[1]{\textbf{#1}} \)
\( \newcommand{\vectorD}[1]{\overrightarrow{#1}} \)
\( \newcommand{\vectorDt}[1]{\overrightarrow{\text{#1}}} \)
\( \newcommand{\vectE}[1]{\overset{-\!-\!\rightharpoonup}{\vphantom{a}\smash{\mathbf {#1}}}} \)
Hypotheses are predictions of expected findings.
The Research Hypothesis
A research hypothesis is a mathematical way of stating a research question. A research hypothesis names the groups (we'll start with a sample and a population), what was measured, and which we think will have a higher mean. The last one gives the research hypothesis a direction. In other words, a research hypothesis should include:
- The name of the groups being compared. This is sometimes considered the IV.
- What was measured. This is the DV.
- Which group are we predicting will have the higher mean.
There are two types of research hypotheses related to sample means and population means: Directional Research Hypotheses and Non-Directional Research Hypotheses
Directional Research Hypothesis
If we expect our obtained sample mean to be above or below the other group's mean (the population mean, for example), we have a directional hypothesis. There are two options:
- Symbol: \( \displaystyle \bar{X} > \mu \)
- (The mean of the sample is greater than than the mean of the population.)
- Symbol: \( \displaystyle \bar{X} < \mu \)
- (The mean of the sample is less than than mean of the population.)
Example \(\PageIndex{1}\)
A study by Blackwell, Trzesniewski, and Dweck (2007) measured growth mindset and how long the junior high student participants spent on their math homework. What’s a directional hypothesis for how scoring higher on growth mindset (compared to the population of junior high students) would be related to how long students spent on their homework? Write this out in words and symbols.
Answer in Words: Students who scored high on growth mindset would spend more time on their homework than the population of junior high students.
Answer in Symbols: \( \displaystyle \bar{X} > \mu \)
Non-Directional Research Hypothesis
A non-directional hypothesis states that the means will be different, but does not specify which will be higher. In reality, there is rarely a situation in which we actually don't want one group to be higher than the other, so we will focus on directional research hypotheses. There is only one option for a non-directional research hypothesis: "The sample mean differs from the population mean." These types of research hypotheses don’t give a direction, the hypothesis doesn’t say which will be higher or lower.
A non-directional research hypothesis in symbols should look like this: \( \displaystyle \bar{X} \neq \mu \) (The mean of the sample is not equal to the mean of the population).
Exercise \(\PageIndex{1}\)
What’s a non-directional hypothesis for how scoring higher on growth mindset higher on growth mindset (compared to the population of junior high students) would be related to how long students spent on their homework (Blackwell, Trzesniewski, & Dweck, 2007)? Write this out in words and symbols.
Answer in Words: Students who scored high on growth mindset would spend a different amount of time on their homework than the population of junior high students.
Answer in Symbols: \( \displaystyle \bar{X} \neq \mu \)
See how a non-directional research hypothesis doesn't really make sense? The big issue is not if the two groups differ, but if one group seems to improve what was measured (if having a growth mindset leads to more time spent on math homework). This textbook will only use directional research hypotheses because researchers almost always have a predicted direction (meaning that we almost always know which group we think will score higher).
The Null Hypothesis
The hypothesis that an apparent effect is due to chance is called the null hypothesis, written \(H_0\) (“H-naught”). We usually test this through comparing an experimental group to a comparison (control) group. This null hypothesis can be written as:
\[\mathrm{H}_{0}: \bar{X} = \mu \nonumber \]
For most of this textbook, the null hypothesis is that the means of the two groups are similar. Much later, the null hypothesis will be that there is no relationship between the two groups. Either way, remember that a null hypothesis is always saying that nothing is different.
This is where descriptive statistics diverge from inferential statistics. We know what the value of \(\overline{\mathrm{X}}\) is – it’s not a mystery or a question, it is what we observed from the sample. What we are using inferential statistics to do is infer whether this sample's descriptive statistics probably represents the population's descriptive statistics. This is the null hypothesis, that the two groups are similar.
Keep in mind that the null hypothesis is typically the opposite of the research hypothesis. A research hypothesis for the ESP example is that those in my sample who say that they have ESP would get more correct answers than the population would get correct, while the null hypothesis is that the average number correct for the two groups will be similar.
In general, the null hypothesis is the idea that nothing is going on: there is no effect of our treatment, no relation between our variables, and no difference in our sample mean from what we expected about the population mean. This is always our baseline starting assumption, and it is what we seek to reject. If we are trying to treat depression, we want to find a difference in average symptoms between our treatment and control groups. If we are trying to predict job performance, we want to find a relation between conscientiousness and evaluation scores. However, until we have evidence against it, we must use the null hypothesis as our starting point.
In sum, the null hypothesis is always : There is no difference between the groups’ means OR There is no relationship between the variables .
In the next chapter, the null hypothesis is that there’s no difference between the sample mean and population mean. In other words:
- There is no mean difference between the sample and population.
- The mean of the sample is the same as the mean of a specific population.
- \(\mathrm{H}_{0}: \bar{X} = \mu \nonumber \)
- We expect our sample’s mean to be same as the population mean.
Exercise \(\PageIndex{2}\)
A study by Blackwell, Trzesniewski, and Dweck (2007) measured growth mindset and how long the junior high student participants spent on their math homework. What’s the null hypothesis for scoring higher on growth mindset (compared to the population of junior high students) and how long students spent on their homework? Write this out in words and symbols.
Answer in Words: Students who scored high on growth mindset would spend a similar amount of time on their homework as the population of junior high students.
Answer in Symbols: \( \bar{X} = \mu \)
Contributors and Attributions
Foster et al. (University of Missouri-St. Louis, Rice University, & University of Houston, Downtown Campus)
Dr. MO ( Taft College )

What Is A Research (Scientific) Hypothesis? A plain-language explainer + examples
By: Derek Jansen (MBA) | Reviewed By: Dr Eunice Rautenbach | June 2020
If you’re new to the world of research, or it’s your first time writing a dissertation or thesis, you’re probably noticing that the words “research hypothesis” and “scientific hypothesis” are used quite a bit, and you’re wondering what they mean in a research context .
“Hypothesis” is one of those words that people use loosely, thinking they understand what it means. However, it has a very specific meaning within academic research. So, it’s important to understand the exact meaning before you start hypothesizing.
Research Hypothesis 101
- What is a hypothesis ?
- What is a research hypothesis (scientific hypothesis)?
- Requirements for a research hypothesis
- Definition of a research hypothesis
- The null hypothesis
What is a hypothesis?
Let’s start with the general definition of a hypothesis (not a research hypothesis or scientific hypothesis), according to the Cambridge Dictionary:
Hypothesis: an idea or explanation for something that is based on known facts but has not yet been proved.
In other words, it’s a statement that provides an explanation for why or how something works, based on facts (or some reasonable assumptions), but that has not yet been specifically tested . For example, a hypothesis might look something like this:
Hypothesis: sleep impacts academic performance.
This statement predicts that academic performance will be influenced by the amount and/or quality of sleep a student engages in – sounds reasonable, right? It’s based on reasonable assumptions , underpinned by what we currently know about sleep and health (from the existing literature). So, loosely speaking, we could call it a hypothesis, at least by the dictionary definition.
But that’s not good enough…
Unfortunately, that’s not quite sophisticated enough to describe a research hypothesis (also sometimes called a scientific hypothesis), and it wouldn’t be acceptable in a dissertation, thesis or research paper . In the world of academic research, a statement needs a few more criteria to constitute a true research hypothesis .
What is a research hypothesis?
A research hypothesis (also called a scientific hypothesis) is a statement about the expected outcome of a study (for example, a dissertation or thesis). To constitute a quality hypothesis, the statement needs to have three attributes – specificity , clarity and testability .
Let’s take a look at these more closely.
Need a helping hand?
Hypothesis Essential #1: Specificity & Clarity
A good research hypothesis needs to be extremely clear and articulate about both what’ s being assessed (who or what variables are involved ) and the expected outcome (for example, a difference between groups, a relationship between variables, etc.).
Let’s stick with our sleepy students example and look at how this statement could be more specific and clear.
Hypothesis: Students who sleep at least 8 hours per night will, on average, achieve higher grades in standardised tests than students who sleep less than 8 hours a night.
As you can see, the statement is very specific as it identifies the variables involved (sleep hours and test grades), the parties involved (two groups of students), as well as the predicted relationship type (a positive relationship). There’s no ambiguity or uncertainty about who or what is involved in the statement, and the expected outcome is clear.
Contrast that to the original hypothesis we looked at – “Sleep impacts academic performance” – and you can see the difference. “Sleep” and “academic performance” are both comparatively vague , and there’s no indication of what the expected relationship direction is (more sleep or less sleep). As you can see, specificity and clarity are key.
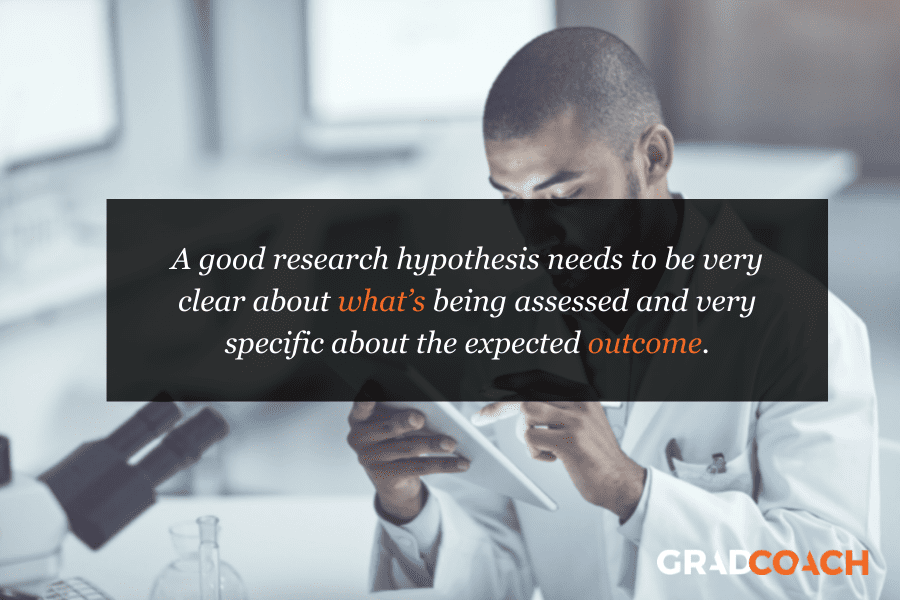
Hypothesis Essential #2: Testability (Provability)
A statement must be testable to qualify as a research hypothesis. In other words, there needs to be a way to prove (or disprove) the statement. If it’s not testable, it’s not a hypothesis – simple as that.
For example, consider the hypothesis we mentioned earlier:
Hypothesis: Students who sleep at least 8 hours per night will, on average, achieve higher grades in standardised tests than students who sleep less than 8 hours a night.
We could test this statement by undertaking a quantitative study involving two groups of students, one that gets 8 or more hours of sleep per night for a fixed period, and one that gets less. We could then compare the standardised test results for both groups to see if there’s a statistically significant difference.
Again, if you compare this to the original hypothesis we looked at – “Sleep impacts academic performance” – you can see that it would be quite difficult to test that statement, primarily because it isn’t specific enough. How much sleep? By who? What type of academic performance?
So, remember the mantra – if you can’t test it, it’s not a hypothesis 🙂
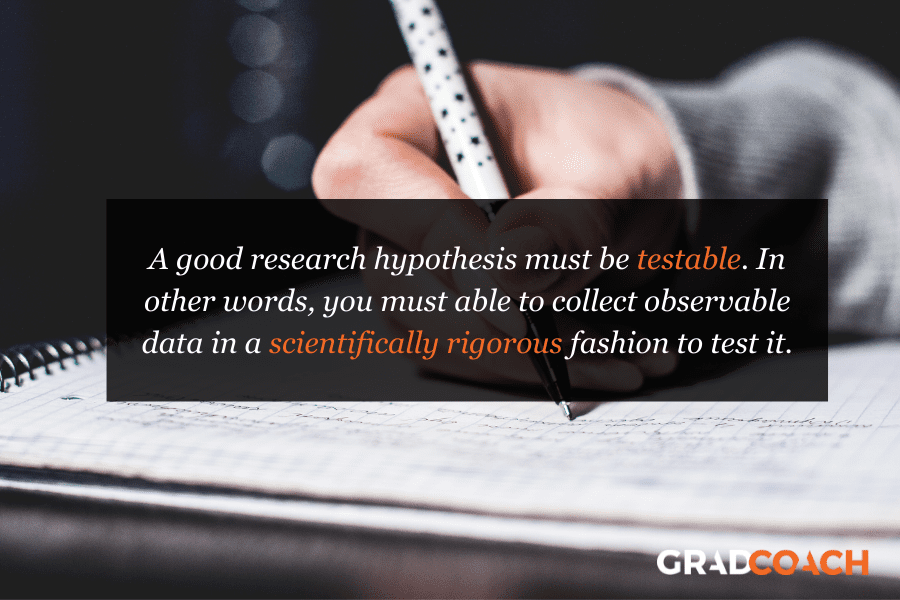
Defining A Research Hypothesis
You’re still with us? Great! Let’s recap and pin down a clear definition of a hypothesis.
A research hypothesis (or scientific hypothesis) is a statement about an expected relationship between variables, or explanation of an occurrence, that is clear, specific and testable.
So, when you write up hypotheses for your dissertation or thesis, make sure that they meet all these criteria. If you do, you’ll not only have rock-solid hypotheses but you’ll also ensure a clear focus for your entire research project.
What about the null hypothesis?
You may have also heard the terms null hypothesis , alternative hypothesis, or H-zero thrown around. At a simple level, the null hypothesis is the counter-proposal to the original hypothesis.
For example, if the hypothesis predicts that there is a relationship between two variables (for example, sleep and academic performance), the null hypothesis would predict that there is no relationship between those variables.
At a more technical level, the null hypothesis proposes that no statistical significance exists in a set of given observations and that any differences are due to chance alone.
And there you have it – hypotheses in a nutshell.
If you have any questions, be sure to leave a comment below and we’ll do our best to help you. If you need hands-on help developing and testing your hypotheses, consider our private coaching service , where we hold your hand through the research journey.
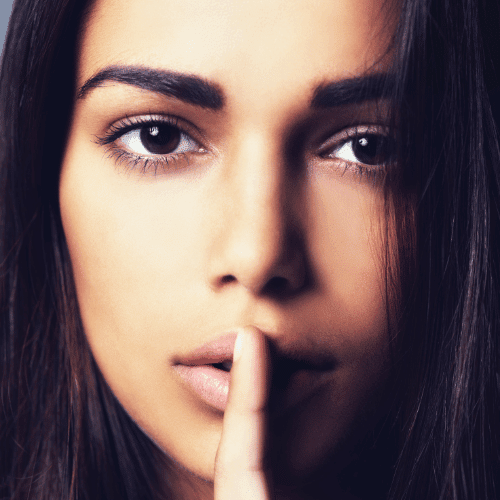
Psst... there’s more!
This post was based on one of our popular Research Bootcamps . If you're working on a research project, you'll definitely want to check this out ...
You Might Also Like:
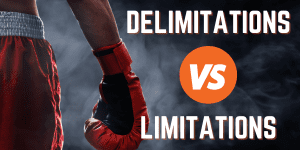
16 Comments
Very useful information. I benefit more from getting more information in this regard.
Very great insight,educative and informative. Please give meet deep critics on many research data of public international Law like human rights, environment, natural resources, law of the sea etc
In a book I read a distinction is made between null, research, and alternative hypothesis. As far as I understand, alternative and research hypotheses are the same. Can you please elaborate? Best Afshin
This is a self explanatory, easy going site. I will recommend this to my friends and colleagues.
Very good definition. How can I cite your definition in my thesis? Thank you. Is nul hypothesis compulsory in a research?
It’s a counter-proposal to be proven as a rejection
Please what is the difference between alternate hypothesis and research hypothesis?
It is a very good explanation. However, it limits hypotheses to statistically tasteable ideas. What about for qualitative researches or other researches that involve quantitative data that don’t need statistical tests?
In qualitative research, one typically uses propositions, not hypotheses.
could you please elaborate it more
I’ve benefited greatly from these notes, thank you.
This is very helpful
well articulated ideas are presented here, thank you for being reliable sources of information
Excellent. Thanks for being clear and sound about the research methodology and hypothesis (quantitative research)
I have only a simple question regarding the null hypothesis. – Is the null hypothesis (Ho) known as the reversible hypothesis of the alternative hypothesis (H1? – How to test it in academic research?
this is very important note help me much more
Trackbacks/Pingbacks
- What Is Research Methodology? Simple Definition (With Examples) - Grad Coach - […] Contrasted to this, a quantitative methodology is typically used when the research aims and objectives are confirmatory in nature. For example,…
Submit a Comment Cancel reply
Your email address will not be published. Required fields are marked *
Save my name, email, and website in this browser for the next time I comment.
- Print Friendly
- Math Article
Null Hypothesis
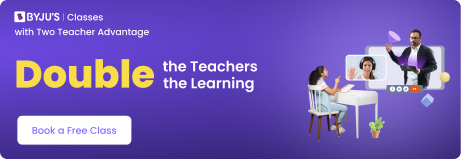
In mathematics, Statistics deals with the study of research and surveys on the numerical data. For taking surveys, we have to define the hypothesis. Generally, there are two types of hypothesis. One is a null hypothesis, and another is an alternative hypothesis .
In probability and statistics, the null hypothesis is a comprehensive statement or default status that there is zero happening or nothing happening. For example, there is no connection among groups or no association between two measured events. It is generally assumed here that the hypothesis is true until any other proof has been brought into the light to deny the hypothesis. Let us learn more here with definition, symbol, principle, types and example, in this article.
Table of contents:
- Comparison with Alternative Hypothesis
Null Hypothesis Definition
The null hypothesis is a kind of hypothesis which explains the population parameter whose purpose is to test the validity of the given experimental data. This hypothesis is either rejected or not rejected based on the viability of the given population or sample . In other words, the null hypothesis is a hypothesis in which the sample observations results from the chance. It is said to be a statement in which the surveyors wants to examine the data. It is denoted by H 0 .
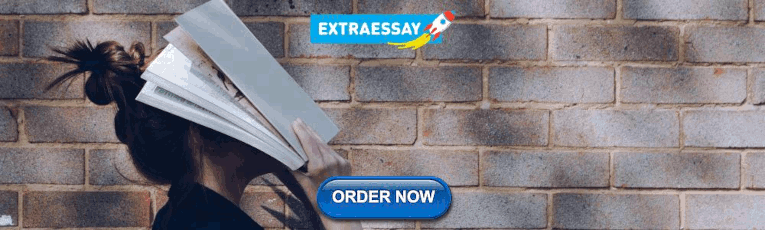
Null Hypothesis Symbol
In statistics, the null hypothesis is usually denoted by letter H with subscript ‘0’ (zero), such that H 0 . It is pronounced as H-null or H-zero or H-nought. At the same time, the alternative hypothesis expresses the observations determined by the non-random cause. It is represented by H 1 or H a .
Null Hypothesis Principle
The principle followed for null hypothesis testing is, collecting the data and determining the chances of a given set of data during the study on some random sample, assuming that the null hypothesis is true. In case if the given data does not face the expected null hypothesis, then the outcome will be quite weaker, and they conclude by saying that the given set of data does not provide strong evidence against the null hypothesis because of insufficient evidence. Finally, the researchers tend to reject that.
Null Hypothesis Formula
Here, the hypothesis test formulas are given below for reference.
The formula for the null hypothesis is:
H 0 : p = p 0
The formula for the alternative hypothesis is:
H a = p >p 0 , < p 0 ≠ p 0
The formula for the test static is:
Remember that, p 0 is the null hypothesis and p – hat is the sample proportion.
Also, read:
Types of Null Hypothesis
There are different types of hypothesis. They are:
Simple Hypothesis
It completely specifies the population distribution. In this method, the sampling distribution is the function of the sample size.
Composite Hypothesis
The composite hypothesis is one that does not completely specify the population distribution.
Exact Hypothesis
Exact hypothesis defines the exact value of the parameter. For example μ= 50
Inexact Hypothesis
This type of hypothesis does not define the exact value of the parameter. But it denotes a specific range or interval. For example 45< μ <60
Null Hypothesis Rejection
Sometimes the null hypothesis is rejected too. If this hypothesis is rejected means, that research could be invalid. Many researchers will neglect this hypothesis as it is merely opposite to the alternate hypothesis. It is a better practice to create a hypothesis and test it. The goal of researchers is not to reject the hypothesis. But it is evident that a perfect statistical model is always associated with the failure to reject the null hypothesis.
How do you Find the Null Hypothesis?
The null hypothesis says there is no correlation between the measured event (the dependent variable) and the independent variable. We don’t have to believe that the null hypothesis is true to test it. On the contrast, you will possibly assume that there is a connection between a set of variables ( dependent and independent).
When is Null Hypothesis Rejected?
The null hypothesis is rejected using the P-value approach. If the P-value is less than or equal to the α, there should be a rejection of the null hypothesis in favour of the alternate hypothesis. In case, if P-value is greater than α, the null hypothesis is not rejected.
Null Hypothesis and Alternative Hypothesis
Now, let us discuss the difference between the null hypothesis and the alternative hypothesis.
Null Hypothesis Examples
Here, some of the examples of the null hypothesis are given below. Go through the below ones to understand the concept of the null hypothesis in a better way.
If a medicine reduces the risk of cardiac stroke, then the null hypothesis should be “the medicine does not reduce the chance of cardiac stroke”. This testing can be performed by the administration of a drug to a certain group of people in a controlled way. If the survey shows that there is a significant change in the people, then the hypothesis is rejected.
Few more examples are:
1). Are there is 100% chance of getting affected by dengue?
Ans: There could be chances of getting affected by dengue but not 100%.
2). Do teenagers are using mobile phones more than grown-ups to access the internet?
Ans: Age has no limit on using mobile phones to access the internet.
3). Does having apple daily will not cause fever?
Ans: Having apple daily does not assure of not having fever, but increases the immunity to fight against such diseases.
4). Do the children more good in doing mathematical calculations than grown-ups?
Ans: Age has no effect on Mathematical skills.
In many common applications, the choice of the null hypothesis is not automated, but the testing and calculations may be automated. Also, the choice of the null hypothesis is completely based on previous experiences and inconsistent advice. The choice can be more complicated and based on the variety of applications and the diversity of the objectives.
The main limitation for the choice of the null hypothesis is that the hypothesis suggested by the data is based on the reasoning which proves nothing. It means that if some hypothesis provides a summary of the data set, then there would be no value in the testing of the hypothesis on the particular set of data.
Frequently Asked Questions on Null Hypothesis
What is meant by the null hypothesis.
In Statistics, a null hypothesis is a type of hypothesis which explains the population parameter whose purpose is to test the validity of the given experimental data.
What are the benefits of hypothesis testing?
Hypothesis testing is defined as a form of inferential statistics, which allows making conclusions from the entire population based on the sample representative.
When a null hypothesis is accepted and rejected?
The null hypothesis is either accepted or rejected in terms of the given data. If P-value is less than α, then the null hypothesis is rejected in favor of the alternative hypothesis, and if the P-value is greater than α, then the null hypothesis is accepted in favor of the alternative hypothesis.
Why is the null hypothesis important?
The importance of the null hypothesis is that it provides an approximate description of the phenomena of the given data. It allows the investigators to directly test the relational statement in a research study.
How to accept or reject the null hypothesis in the chi-square test?
If the result of the chi-square test is bigger than the critical value in the table, then the data does not fit the model, which represents the rejection of the null hypothesis.
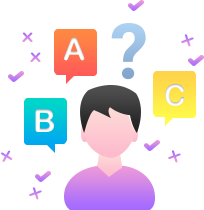
Put your understanding of this concept to test by answering a few MCQs. Click ‘Start Quiz’ to begin!
Select the correct answer and click on the “Finish” button Check your score and answers at the end of the quiz
Visit BYJU’S for all Maths related queries and study materials
Your result is as below
Request OTP on Voice Call

Register with BYJU'S & Download Free PDFs
Register with byju's & watch live videos.

Want to create or adapt books like this? Learn more about how Pressbooks supports open publishing practices.
Inferential Statistics
Learning Objectives
- Explain the purpose of null hypothesis testing, including the role of sampling error.
- Describe the basic logic of null hypothesis testing.
- Describe the role of relationship strength and sample size in determining statistical significance and make reasonable judgments about statistical significance based on these two factors.
The Purpose of Null Hypothesis Testing
As we have seen, psychological research typically involves measuring one or more variables in a sample and computing descriptive summary data (e.g., means, correlation coefficients) for those variables. These descriptive data for the sample are called statistics . In general, however, the researcher’s goal is not to draw conclusions about that sample but to draw conclusions about the population that the sample was selected from. Thus researchers must use sample statistics to draw conclusions about the corresponding values in the population. These corresponding values in the population are called parameters . Imagine, for example, that a researcher measures the number of depressive symptoms exhibited by each of 50 adults with clinical depression and computes the mean number of symptoms. The researcher probably wants to use this sample statistic (the mean number of symptoms for the sample) to draw conclusions about the corresponding population parameter (the mean number of symptoms for adults with clinical depression).
Unfortunately, sample statistics are not perfect estimates of their corresponding population parameters. This is because there is a certain amount of random variability in any statistic from sample to sample. The mean number of depressive symptoms might be 8.73 in one sample of adults with clinical depression, 6.45 in a second sample, and 9.44 in a third—even though these samples are selected randomly from the same population. Similarly, the correlation (Pearson’s r ) between two variables might be +.24 in one sample, −.04 in a second sample, and +.15 in a third—again, even though these samples are selected randomly from the same population. This random variability in a statistic from sample to sample is called sampling error . (Note that the term error here refers to random variability and does not imply that anyone has made a mistake. No one “commits a sampling error.”)
One implication of this is that when there is a statistical relationship in a sample, it is not always clear that there is a statistical relationship in the population. A small difference between two group means in a sample might indicate that there is a small difference between the two group means in the population. But it could also be that there is no difference between the means in the population and that the difference in the sample is just a matter of sampling error. Similarly, a Pearson’s r value of −.29 in a sample might mean that there is a negative relationship in the population. But it could also be that there is no relationship in the population and that the relationship in the sample is just a matter of sampling error.
In fact, any statistical relationship in a sample can be interpreted in two ways:
- There is a relationship in the population, and the relationship in the sample reflects this.
- There is no relationship in the population, and the relationship in the sample reflects only sampling error.
The purpose of null hypothesis testing is simply to help researchers decide between these two interpretations.
The Logic of Null Hypothesis Testing
Null hypothesis testing (often called null hypothesis significance testing or NHST) is a formal approach to deciding between two interpretations of a statistical relationship in a sample. One interpretation is called the null hypothesis (often symbolized H 0 and read as “H-zero”). This is the idea that there is no relationship in the population and that the relationship in the sample reflects only sampling error. Informally, the null hypothesis is that the sample relationship “occurred by chance.” The other interpretation is called the alternative hypothesis (often symbolized as H 1 ). This is the idea that there is a relationship in the population and that the relationship in the sample reflects this relationship in the population.
Again, every statistical relationship in a sample can be interpreted in either of these two ways: It might have occurred by chance, or it might reflect a relationship in the population. So researchers need a way to decide between them. Although there are many specific null hypothesis testing techniques, they are all based on the same general logic. The steps are as follows:
- Assume for the moment that the null hypothesis is true. There is no relationship between the variables in the population.
- Determine how likely the sample relationship would be if the null hypothesis were true.
- If the sample relationship would be extremely unlikely, then reject the null hypothesis in favor of the alternative hypothesis. If it would not be extremely unlikely, then retain the null hypothesis .
Following this logic, we can begin to understand why Mehl and his colleagues concluded that there is no difference in talkativeness between women and men in the population. In essence, they asked the following question: “If there were no difference in the population, how likely is it that we would find a small difference of d = 0.06 in our sample?” Their answer to this question was that this sample relationship would be fairly likely if the null hypothesis were true. Therefore, they retained the null hypothesis—concluding that there is no evidence of a sex difference in the population. We can also see why Kanner and his colleagues concluded that there is a correlation between hassles and symptoms in the population. They asked, “If the null hypothesis were true, how likely is it that we would find a strong correlation of +.60 in our sample?” Their answer to this question was that this sample relationship would be fairly unlikely if the null hypothesis were true. Therefore, they rejected the null hypothesis in favor of the alternative hypothesis—concluding that there is a positive correlation between these variables in the population.
A crucial step in null hypothesis testing is finding the probability of the sample result or a more extreme result if the null hypothesis were true (Lakens, 2017). [1] This probability is called the p value . A low p value means that the sample or more extreme result would be unlikely if the null hypothesis were true and leads to the rejection of the null hypothesis. A p value that is not low means that the sample or more extreme result would be likely if the null hypothesis were true and leads to the retention of the null hypothesis. But how low must the p value criterion be before the sample result is considered unlikely enough to reject the null hypothesis? In null hypothesis testing, this criterion is called α (alpha) and is almost always set to .05. If there is a 5% chance or less of a result at least as extreme as the sample result if the null hypothesis were true, then the null hypothesis is rejected. When this happens, the result is said to be statistically significant . If there is greater than a 5% chance of a result as extreme as the sample result when the null hypothesis is true, then the null hypothesis is retained. This does not necessarily mean that the researcher accepts the null hypothesis as true—only that there is not currently enough evidence to reject it. Researchers often use the expression “fail to reject the null hypothesis” rather than “retain the null hypothesis,” but they never use the expression “accept the null hypothesis.”
The Misunderstood p Value
The p value is one of the most misunderstood quantities in psychological research (Cohen, 1994) [2] . Even professional researchers misinterpret it, and it is not unusual for such misinterpretations to appear in statistics textbooks!
The most common misinterpretation is that the p value is the probability that the null hypothesis is true—that the sample result occurred by chance. For example, a misguided researcher might say that because the p value is .02, there is only a 2% chance that the result is due to chance and a 98% chance that it reflects a real relationship in the population. But this is incorrect . The p value is really the probability of a result at least as extreme as the sample result if the null hypothesis were true. So a p value of .02 means that if the null hypothesis were true, a sample result this extreme would occur only 2% of the time.
You can avoid this misunderstanding by remembering that the p value is not the probability that any particular hypothesis is true or false. Instead, it is the probability of obtaining the sample result if the null hypothesis were true.
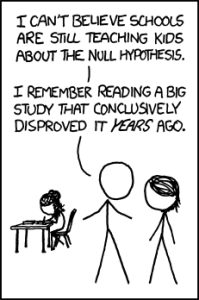
Role of Sample Size and Relationship Strength
Recall that null hypothesis testing involves answering the question, “If the null hypothesis were true, what is the probability of a sample result as extreme as this one?” In other words, “What is the p value?” It can be helpful to see that the answer to this question depends on just two considerations: the strength of the relationship and the size of the sample. Specifically, the stronger the sample relationship and the larger the sample, the less likely the result would be if the null hypothesis were true. That is, the lower the p value. This should make sense. Imagine a study in which a sample of 500 women is compared with a sample of 500 men in terms of some psychological characteristic, and Cohen’s d is a strong 0.50. If there were really no sex difference in the population, then a result this strong based on such a large sample should seem highly unlikely. Now imagine a similar study in which a sample of three women is compared with a sample of three men, and Cohen’s d is a weak 0.10. If there were no sex difference in the population, then a relationship this weak based on such a small sample should seem likely. And this is precisely why the null hypothesis would be rejected in the first example and retained in the second.
Of course, sometimes the result can be weak and the sample large, or the result can be strong and the sample small. In these cases, the two considerations trade off against each other so that a weak result can be statistically significant if the sample is large enough and a strong relationship can be statistically significant even if the sample is small. Table 13.1 shows roughly how relationship strength and sample size combine to determine whether a sample result is statistically significant. The columns of the table represent the three levels of relationship strength: weak, medium, and strong. The rows represent four sample sizes that can be considered small, medium, large, and extra large in the context of psychological research. Thus each cell in the table represents a combination of relationship strength and sample size. If a cell contains the word Yes , then this combination would be statistically significant for both Cohen’s d and Pearson’s r . If it contains the word No , then it would not be statistically significant for either. There is one cell where the decision for d and r would be different and another where it might be different depending on some additional considerations, which are discussed in Section 13.2 “Some Basic Null Hypothesis Tests”
Although Table 13.1 provides only a rough guideline, it shows very clearly that weak relationships based on medium or small samples are never statistically significant and that strong relationships based on medium or larger samples are always statistically significant. If you keep this lesson in mind, you will often know whether a result is statistically significant based on the descriptive statistics alone. It is extremely useful to be able to develop this kind of intuitive judgment. One reason is that it allows you to develop expectations about how your formal null hypothesis tests are going to come out, which in turn allows you to detect problems in your analyses. For example, if your sample relationship is strong and your sample is medium, then you would expect to reject the null hypothesis. If for some reason your formal null hypothesis test indicates otherwise, then you need to double-check your computations and interpretations. A second reason is that the ability to make this kind of intuitive judgment is an indication that you understand the basic logic of this approach in addition to being able to do the computations.
Statistical Significance Versus Practical Significance
Table 13.1 illustrates another extremely important point. A statistically significant result is not necessarily a strong one. Even a very weak result can be statistically significant if it is based on a large enough sample. This is closely related to Janet Shibley Hyde’s argument about sex differences (Hyde, 2007) [3] . The differences between women and men in mathematical problem solving and leadership ability are statistically significant. But the word significant can cause people to interpret these differences as strong and important—perhaps even important enough to influence the college courses they take or even who they vote for. As we have seen, however, these statistically significant differences are actually quite weak—perhaps even “trivial.”
This is why it is important to distinguish between the statistical significance of a result and the practical significance of that result. Practical significance refers to the importance or usefulness of the result in some real-world context. Many sex differences are statistically significant—and may even be interesting for purely scientific reasons—but they are not practically significant. In clinical practice, this same concept is often referred to as “clinical significance.” For example, a study on a new treatment for social phobia might show that it produces a statistically significant positive effect. Yet this effect still might not be strong enough to justify the time, effort, and other costs of putting it into practice—especially if easier and cheaper treatments that work almost as well already exist. Although statistically significant, this result would be said to lack practical or clinical significance.
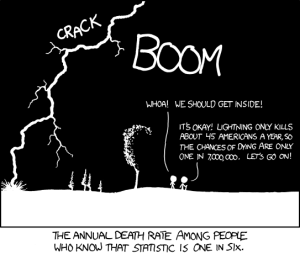
Image Description
“Null Hypothesis” long description: A comic depicting a man and a woman talking in the foreground. In the background is a child working at a desk. The man says to the woman, “I can’t believe schools are still teaching kids about the null hypothesis. I remember reading a big study that conclusively disproved it years ago.” [Return to “Null Hypothesis”]
“Conditional Risk” long description: A comic depicting two hikers beside a tree during a thunderstorm. A bolt of lightning goes “crack” in the dark sky as thunder booms. One of the hikers says, “Whoa! We should get inside!” The other hiker says, “It’s okay! Lightning only kills about 45 Americans a year, so the chances of dying are only one in 7,000,000. Let’s go on!” The comic’s caption says, “The annual death rate among people who know that statistic is one in six.” [Return to “Conditional Risk”]
Media Attributions
- Null Hypothesis by XKCD CC BY-NC (Attribution NonCommercial)
- Conditional Risk by XKCD CC BY-NC (Attribution NonCommercial)
- Lakens, D. (2017, December 25). About p -values: Understanding common misconceptions. [Blog post] Retrieved from https://correlaid.org/en/blog/understand-p-values/ ↵
- Cohen, J. (1994). The world is round: p < .05. American Psychologist, 49 , 997–1003. ↵
- Hyde, J. S. (2007). New directions in the study of gender similarities and differences. Current Directions in Psychological Science, 16 , 259–263. ↵
Descriptive data that involves measuring one or more variables in a sample and computing descriptive summary data (e.g., means, correlation coefficients) for those variables.
Corresponding values in the population.
The random variability in a statistic from sample to sample.
A formal approach to deciding between two interpretations of a statistical relationship in a sample.
The idea that there is no relationship in the population and that the relationship in the sample reflects only sampling error (often symbolized H0 and read as “H-zero”).
An alternative to the null hypothesis (often symbolized as H1), this hypothesis proposes that there is a relationship in the population and that the relationship in the sample reflects this relationship in the population.
A decision made by researchers using null hypothesis testing which occurs when the sample relationship would be extremely unlikely.
A decision made by researchers in null hypothesis testing which occurs when the sample relationship would not be extremely unlikely.
The probability of obtaining the sample result or a more extreme result if the null hypothesis were true.
The criterion that shows how low a p-value should be before the sample result is considered unlikely enough to reject the null hypothesis (Usually set to .05).
An effect that is unlikely due to random chance and therefore likely represents a real effect in the population.
Refers to the importance or usefulness of the result in some real-world context.
Research Methods in Psychology Copyright © 2019 by Rajiv S. Jhangiani, I-Chant A. Chiang, Carrie Cuttler, & Dana C. Leighton is licensed under a Creative Commons Attribution-NonCommercial-ShareAlike 4.0 International License , except where otherwise noted.
Share This Book

Want to create or adapt books like this? Learn more about how Pressbooks supports open publishing practices.
13.1 Understanding Null Hypothesis Testing
Learning objectives.
- Explain the purpose of null hypothesis testing, including the role of sampling error.
- Describe the basic logic of null hypothesis testing.
- Describe the role of relationship strength and sample size in determining statistical significance and make reasonable judgments about statistical significance based on these two factors.
The Purpose of Null Hypothesis Testing
As we have seen, psychological research typically involves measuring one or more variables for a sample and computing descriptive statistics for that sample. In general, however, the researcher’s goal is not to draw conclusions about that sample but to draw conclusions about the population that the sample was selected from. Thus researchers must use sample statistics to draw conclusions about the corresponding values in the population. These corresponding values in the population are called parameters . Imagine, for example, that a researcher measures the number of depressive symptoms exhibited by each of 50 clinically depressed adults and computes the mean number of symptoms. The researcher probably wants to use this sample statistic (the mean number of symptoms for the sample) to draw conclusions about the corresponding population parameter (the mean number of symptoms for clinically depressed adults).
Unfortunately, sample statistics are not perfect estimates of their corresponding population parameters. This is because there is a certain amount of random variability in any statistic from sample to sample. The mean number of depressive symptoms might be 8.73 in one sample of clinically depressed adults, 6.45 in a second sample, and 9.44 in a third—even though these samples are selected randomly from the same population. Similarly, the correlation (Pearson’s r ) between two variables might be +.24 in one sample, −.04 in a second sample, and +.15 in a third—again, even though these samples are selected randomly from the same population. This random variability in a statistic from sample to sample is called sampling error . (Note that the term error here refers to random variability and does not imply that anyone has made a mistake. No one “commits a sampling error.”)
One implication of this is that when there is a statistical relationship in a sample, it is not always clear that there is a statistical relationship in the population. A small difference between two group means in a sample might indicate that there is a small difference between the two group means in the population. But it could also be that there is no difference between the means in the population and that the difference in the sample is just a matter of sampling error. Similarly, a Pearson’s r value of −.29 in a sample might mean that there is a negative relationship in the population. But it could also be that there is no relationship in the population and that the relationship in the sample is just a matter of sampling error.
In fact, any statistical relationship in a sample can be interpreted in two ways:
- There is a relationship in the population, and the relationship in the sample reflects this.
- There is no relationship in the population, and the relationship in the sample reflects only sampling error.
The purpose of null hypothesis testing is simply to help researchers decide between these two interpretations.
The Logic of Null Hypothesis Testing
Null hypothesis testing is a formal approach to deciding between two interpretations of a statistical relationship in a sample. One interpretation is called the null hypothesis (often symbolized H 0 and read as “H-naught”). This is the idea that there is no relationship in the population and that the relationship in the sample reflects only sampling error. Informally, the null hypothesis is that the sample relationship “occurred by chance.” The other interpretation is called the alternative hypothesis (often symbolized as H 1 ). This is the idea that there is a relationship in the population and that the relationship in the sample reflects this relationship in the population.
Again, every statistical relationship in a sample can be interpreted in either of these two ways: It might have occurred by chance, or it might reflect a relationship in the population. So researchers need a way to decide between them. Although there are many specific null hypothesis testing techniques, they are all based on the same general logic. The steps are as follows:
- Assume for the moment that the null hypothesis is true. There is no relationship between the variables in the population.
- Determine how likely the sample relationship would be if the null hypothesis were true.
- If the sample relationship would be extremely unlikely, then reject the null hypothesis in favor of the alternative hypothesis. If it would not be extremely unlikely, then retain the null hypothesis .
Following this logic, we can begin to understand why Mehl and his colleagues concluded that there is no difference in talkativeness between women and men in the population. In essence, they asked the following question: “If there were no difference in the population, how likely is it that we would find a small difference of d = 0.06 in our sample?” Their answer to this question was that this sample relationship would be fairly likely if the null hypothesis were true. Therefore, they retained the null hypothesis—concluding that there is no evidence of a sex difference in the population. We can also see why Kanner and his colleagues concluded that there is a correlation between hassles and symptoms in the population. They asked, “If the null hypothesis were true, how likely is it that we would find a strong correlation of +.60 in our sample?” Their answer to this question was that this sample relationship would be fairly unlikely if the null hypothesis were true. Therefore, they rejected the null hypothesis in favor of the alternative hypothesis—concluding that there is a positive correlation between these variables in the population.
A crucial step in null hypothesis testing is finding the likelihood of the sample result if the null hypothesis were true. This probability is called the p value . A low p value means that the sample result would be unlikely if the null hypothesis were true and leads to the rejection of the null hypothesis. A high p value means that the sample result would be likely if the null hypothesis were true and leads to the retention of the null hypothesis. But how low must the p value be before the sample result is considered unlikely enough to reject the null hypothesis? In null hypothesis testing, this criterion is called α (alpha) and is almost always set to .05. If there is less than a 5% chance of a result as extreme as the sample result if the null hypothesis were true, then the null hypothesis is rejected. When this happens, the result is said to be statistically significant . If there is greater than a 5% chance of a result as extreme as the sample result when the null hypothesis is true, then the null hypothesis is retained. This does not necessarily mean that the researcher accepts the null hypothesis as true—only that there is not currently enough evidence to conclude that it is true. Researchers often use the expression “fail to reject the null hypothesis” rather than “retain the null hypothesis,” but they never use the expression “accept the null hypothesis.”
The Misunderstood p Value
The p value is one of the most misunderstood quantities in psychological research (Cohen, 1994). Even professional researchers misinterpret it, and it is not unusual for such misinterpretations to appear in statistics textbooks!
The most common misinterpretation is that the p value is the probability that the null hypothesis is true—that the sample result occurred by chance. For example, a misguided researcher might say that because the p value is .02, there is only a 2% chance that the result is due to chance and a 98% chance that it reflects a real relationship in the population. But this is incorrect . The p value is really the probability of a result at least as extreme as the sample result if the null hypothesis were true. So a p value of .02 means that if the null hypothesis were true, a sample result this extreme would occur only 2% of the time.
You can avoid this misunderstanding by remembering that the p value is not the probability that any particular hypothesis is true or false. Instead, it is the probability of obtaining the sample result if the null hypothesis were true.
Role of Sample Size and Relationship Strength
Recall that null hypothesis testing involves answering the question, “If the null hypothesis were true, what is the probability of a sample result as extreme as this one?” In other words, “What is the p value?” It can be helpful to see that the answer to this question depends on just two considerations: the strength of the relationship and the size of the sample. Specifically, the stronger the sample relationship and the larger the sample, the less likely the result would be if the null hypothesis were true. That is, the lower the p value. This should make sense. Imagine a study in which a sample of 500 women is compared with a sample of 500 men in terms of some psychological characteristic, and Cohen’s d is a strong 0.50. If there were really no sex difference in the population, then a result this strong based on such a large sample should seem highly unlikely. Now imagine a similar study in which a sample of three women is compared with a sample of three men, and Cohen’s d is a weak 0.10. If there were no sex difference in the population, then a relationship this weak based on such a small sample should seem likely. And this is precisely why the null hypothesis would be rejected in the first example and retained in the second.
Of course, sometimes the result can be weak and the sample large, or the result can be strong and the sample small. In these cases, the two considerations trade off against each other so that a weak result can be statistically significant if the sample is large enough and a strong relationship can be statistically significant even if the sample is small. Table 13.1 “How Relationship Strength and Sample Size Combine to Determine Whether a Result Is Statistically Significant” shows roughly how relationship strength and sample size combine to determine whether a sample result is statistically significant. The columns of the table represent the three levels of relationship strength: weak, medium, and strong. The rows represent four sample sizes that can be considered small, medium, large, and extra large in the context of psychological research. Thus each cell in the table represents a combination of relationship strength and sample size. If a cell contains the word Yes , then this combination would be statistically significant for both Cohen’s d and Pearson’s r . If it contains the word No , then it would not be statistically significant for either. There is one cell where the decision for d and r would be different and another where it might be different depending on some additional considerations, which are discussed in Section 13.2 “Some Basic Null Hypothesis Tests”
Table 13.1 How Relationship Strength and Sample Size Combine to Determine Whether a Result Is Statistically Significant
Although Table 13.1 “How Relationship Strength and Sample Size Combine to Determine Whether a Result Is Statistically Significant” provides only a rough guideline, it shows very clearly that weak relationships based on medium or small samples are never statistically significant and that strong relationships based on medium or larger samples are always statistically significant. If you keep this in mind, you will often know whether a result is statistically significant based on the descriptive statistics alone. It is extremely useful to be able to develop this kind of intuitive judgment. One reason is that it allows you to develop expectations about how your formal null hypothesis tests are going to come out, which in turn allows you to detect problems in your analyses. For example, if your sample relationship is strong and your sample is medium, then you would expect to reject the null hypothesis. If for some reason your formal null hypothesis test indicates otherwise, then you need to double-check your computations and interpretations. A second reason is that the ability to make this kind of intuitive judgment is an indication that you understand the basic logic of this approach in addition to being able to do the computations.
Statistical Significance Versus Practical Significance
Table 13.1 “How Relationship Strength and Sample Size Combine to Determine Whether a Result Is Statistically Significant” illustrates another extremely important point. A statistically significant result is not necessarily a strong one. Even a very weak result can be statistically significant if it is based on a large enough sample. This is closely related to Janet Shibley Hyde’s argument about sex differences (Hyde, 2007). The differences between women and men in mathematical problem solving and leadership ability are statistically significant. But the word significant can cause people to interpret these differences as strong and important—perhaps even important enough to influence the college courses they take or even who they vote for. As we have seen, however, these statistically significant differences are actually quite weak—perhaps even “trivial.”
This is why it is important to distinguish between the statistical significance of a result and the practical significance of that result. Practical significance refers to the importance or usefulness of the result in some real-world context. Many sex differences are statistically significant—and may even be interesting for purely scientific reasons—but they are not practically significant. In clinical practice, this same concept is often referred to as “clinical significance.” For example, a study on a new treatment for social phobia might show that it produces a statistically significant positive effect. Yet this effect still might not be strong enough to justify the time, effort, and other costs of putting it into practice—especially if easier and cheaper treatments that work almost as well already exist. Although statistically significant, this result would be said to lack practical or clinical significance.
Key Takeaways
- Null hypothesis testing is a formal approach to deciding whether a statistical relationship in a sample reflects a real relationship in the population or is just due to chance.
- The logic of null hypothesis testing involves assuming that the null hypothesis is true, finding how likely the sample result would be if this assumption were correct, and then making a decision. If the sample result would be unlikely if the null hypothesis were true, then it is rejected in favor of the alternative hypothesis. If it would not be unlikely, then the null hypothesis is retained.
- The probability of obtaining the sample result if the null hypothesis were true (the p value) is based on two considerations: relationship strength and sample size. Reasonable judgments about whether a sample relationship is statistically significant can often be made by quickly considering these two factors.
- Statistical significance is not the same as relationship strength or importance. Even weak relationships can be statistically significant if the sample size is large enough. It is important to consider relationship strength and the practical significance of a result in addition to its statistical significance.
- Discussion: Imagine a study showing that people who eat more broccoli tend to be happier. Explain for someone who knows nothing about statistics why the researchers would conduct a null hypothesis test.
Practice: Use Table 13.1 “How Relationship Strength and Sample Size Combine to Determine Whether a Result Is Statistically Significant” to decide whether each of the following results is statistically significant.
- The correlation between two variables is r = −.78 based on a sample size of 137.
- The mean score on a psychological characteristic for women is 25 ( SD = 5) and the mean score for men is 24 ( SD = 5). There were 12 women and 10 men in this study.
- In a memory experiment, the mean number of items recalled by the 40 participants in Condition A was 0.50 standard deviations greater than the mean number recalled by the 40 participants in Condition B.
- In another memory experiment, the mean scores for participants in Condition A and Condition B came out exactly the same!
- A student finds a correlation of r = .04 between the number of units the students in his research methods class are taking and the students’ level of stress.
Cohen, J. (1994). The world is round: p < .05. American Psychologist, 49 , 997–1003.
Hyde, J. S. (2007). New directions in the study of gender similarities and differences. Current Directions in Psychological Science , 16 , 259–263.
Research Methods in Psychology Copyright © 2016 by University of Minnesota is licensed under a Creative Commons Attribution-NonCommercial-ShareAlike 4.0 International License , except where otherwise noted.
- Privacy Policy

Home » Research Hypothesis Vs Null Hypothesis
Research Hypothesis Vs Null Hypothesis
Table of Contents

The difference between Research Hypothesis Vs Null Hypothesis is as follows:
Research Hypothesis
A Research Hypothesis is a tentative statement that proposes a relationship between two or more variables. It is based on a theoretical or conceptual framework and is typically tested through empirical research.
Null Hypothesis
A Null Hypothesis is a statement that proposes that there is no relationship between two or more variables. It is the opposite of the research hypothesis and is used as a comparison in statistical analysis.
Comparison Table:
In summary, a research hypothesis proposes a relationship between variables, while a null hypothesis proposes no relationship between variables. The research hypothesis guides empirical research, while the null hypothesis is used as a comparison in statistical analysis. The research hypothesis is supported if statistical analysis provides evidence to reject the null hypothesis, while the null hypothesis is rejected if statistical analysis provides evidence to support the research hypothesis.
About the author
Muhammad Hassan
Researcher, Academic Writer, Web developer
You may also like

Inductive Vs Deductive Research

Exploratory Vs Explanatory Research

Basic Vs Applied Research

Generative Vs Evaluative Research

Reliability Vs Validity

Longitudinal Vs Cross-Sectional Research

An official website of the United States government
The .gov means it’s official. Federal government websites often end in .gov or .mil. Before sharing sensitive information, make sure you’re on a federal government site.
The site is secure. The https:// ensures that you are connecting to the official website and that any information you provide is encrypted and transmitted securely.
- Publications
- Account settings
Preview improvements coming to the PMC website in October 2024. Learn More or Try it out now .
- Advanced Search
- Journal List

Why we habitually engage in null-hypothesis significance testing: A qualitative study
Jonah stunt.
1 Department of Health Sciences, Section of Methodology and Applied Statistics, Vrije Universiteit, Amsterdam, The Netherlands
2 Department of Radiation Oncology, Erasmus Medical Center, Rotterdam, The Netherlands
Leonie van Grootel
3 Rathenau Institute, The Hague, The Netherlands
4 Department of Philosophy, Vrije Universiteit, Amsterdam, The Netherlands
5 Department of Epidemiology and Data Science, Amsterdam University Medical Centers, Amsterdam, The Netherlands
David Trafimow
6 Psychology Department, New Mexico State University, Las Cruces, New Mexico, United States of America
Trynke Hoekstra
Michiel de boer.
7 Department of General Practice and Elderly Care, University Medical Center Groningen, Groningen, The Netherlands
Associated Data
A full study protocol, including a detailed data analysis plan, was preregistered ( https://osf.io/4qg38/ ). At the start of this study, preregistration forms for qualitative studies were not developed yet. Therefore, preregistration for this study is based on an outdated form. Presently, there is a preregistration form available for qualitative studies. Information about data collection, data management, data sharing and data storage is described in a Data Management Plan. Sensitive data is stored in Darkstor, an offline archive for storing sensitive information or data (information that involves i.e., privacy or copyright). As the recordings and transcripts of the interviews and focus groups contain privacy-sensitive data, these files are archived in Darkstor and can be accessed only on request by authorized individuals (i.e., the original researcher or a research coordinator)1. Non-sensitive data is stored in DANS ( https://doi.org/10.17026/dans-2at-nzfs ) (Data Archiving and Networked Services; the Netherlands institute for permanent access to digital research resources). 1. Data requests can be send to ln.uv@mdr .
Null Hypothesis Significance Testing (NHST) is the most familiar statistical procedure for making inferences about population effects. Important problems associated with this method have been addressed and various alternatives that overcome these problems have been developed. Despite its many well-documented drawbacks, NHST remains the prevailing method for drawing conclusions from data. Reasons for this have been insufficiently investigated. Therefore, the aim of our study was to explore the perceived barriers and facilitators related to the use of NHST and alternative statistical procedures among relevant stakeholders in the scientific system.
Individual semi-structured interviews and focus groups were conducted with junior and senior researchers, lecturers in statistics, editors of scientific journals and program leaders of funding agencies. During the focus groups, important themes that emerged from the interviews were discussed. Data analysis was performed using the constant comparison method, allowing emerging (sub)themes to be fully explored. A theory substantiating the prevailing use of NHST was developed based on the main themes and subthemes we identified.
Twenty-nine interviews and six focus groups were conducted. Several interrelated facilitators and barriers associated with the use of NHST and alternative statistical procedures were identified. These factors were subsumed under three main themes: the scientific climate, scientific duty, and reactivity. As a result of the factors, most participants feel dependent in their actions upon others, have become reactive, and await action and initiatives from others. This may explain why NHST is still the standard and ubiquitously used by almost everyone involved.
Our findings demonstrate how perceived barriers to shift away from NHST set a high threshold for actual behavioral change and create a circle of interdependency between stakeholders. By taking small steps it should be possible to decrease the scientific community’s strong dependence on NHST and p-values.
Introduction
Empirical studies often start from the idea that there might be an association between a specific factor and a certain outcome within a population. This idea is referred to as the alternative hypothesis (H1). Its complement, the null hypothesis (H0), typically assumes no association or effect (although it is possible to test other effect sizes than no effect with the null hypothesis). At the stage of data-analysis, the probability of obtaining the observed, or a more extreme, association is calculated under the assumption of no effect in the population (H0) and a number of inferential assumptions [ 1 ]. The probability of obtaining the observed, or more extreme, data is known as ‘the p-value’. The p-value demonstrates the compatibility between the observed data and the expected data under the null hypothesis, where 0 is complete incompatibility and 1 is perfect compatibility [ 2 ]. When the p-value is smaller than a prespecified value (labelled as alpha, usually set at 5% (0.05)), results are generally declared to be statistically significant. At this point, researchers commonly reject the null hypothesis and accept the alternative hypothesis [ 2 ]. Assessing statistical significance by means of contrasting the data with the null hypothesis is called Null Hypothesis Significance Testing (NHST). NHST is the best known and most widely used statistical procedure for making inferences about population effects. The procedure has become the prevailing paradigm in empirical science [ 3 ], and reaching and being able to report statistically significant results has become the ultimate goal for many researchers.
Despite its widespread use, NHST and the p-value have been criticized since its inception. Numerous publications have addressed problems associated with NHST and p-values. Arguably the most important drawback is the fact that NHST is a form of indirect or inverse inference: researchers usually want to know if the null or alternative hypothesis can be accepted and use NHST to conclude either way. But with NHST, the probability of a finding, or more extreme findings, given the null hypothesis is calculated [ 4 ]. Ergo, NHST doesn’t tell us what we want to know. In fact, p-values were never meant to serve as a basis to draw conclusions, but as a continuous measure of incompatibility between empirical findings and a statistical model [ 2 ]. Moreover, the procedure promotes a dichotomous way of thinking, by using the outcome of a significance test as a dichotomous indicator for an effect (p<0.05: effect, p>0.05: no effect). Reducing empirical findings to two categories also results in a great loss of information. Further, a significant outcome is often unjustly interpreted as relevant, but a p-value does not convey any information about the strength or importance of the association. Worse yet, the p-values on which NHST is based confound effect size and sample size. A trivial effect size may nevertheless result in statistical significance provided a sufficiently large sample size. Or an important effect size may fail to result in statistical significance if the sample size is too small. P-values do not validly index the size, relevance, or precision of an effect [ 5 ]. Furthermore, statistical models include not only null hypotheses, but additional assumptions, some of which are wrong, such as the ubiquitous assumption of random and independent sampling from a defined population [ 1 ]. Therefore, although p-values validly index the incompatibility of data with models, p-values do not validly index incompatibility of data with hypotheses that are embedded in wrong models. These are important drawbacks rendering NHST unsuitable as the default procedure for drawing conclusions from empirical data [ 2 , 3 , 5 – 13 ].
A number of alternatives have been developed that overcome these pitfalls, such as Bayesian inference methods [ 7 , 11 , 14 , 15 ], informative hypothesis testing [ 9 , 16 ] and a priori inferential statistics [ 4 , 17 ]. These alternatives build on the idea that research usually starts with a more informed research-question than one merely assuming the null hypothesis of no effect. These methods overcome the problem of inverse inference, although the first two might still lead to dichotomous thinking with the use of thresholds. Despite the availability of alternatives, statistical behavior in the research community has hardly changed. Researchers have been slow to adopt alternative methods and NHST is still the prevailing paradigm for making inferences about population effects [ 3 ].
Until now, reasons for the continuous and ubiquitous use of NHST and the p-value have scarcely been investigated. One explanation is that NHST provides a very simple means for drawing conclusions from empirical data, usually based on the 5% cut-off. Secondly, most researchers are unaware of the pitfalls of NHST; it has been shown that NHST and the p-value are often misunderstood and misinterpreted [ 2 , 3 , 8 , 11 , 18 , 19 ]. Thirdly, NHST has a central role in most methods and statistics courses in higher education. Courses on alternative methods are increasingly being offered but are usually not mandatory. To our knowledge, there is a lack of in depth, empirical research, aimed at elucidating why NHST nevertheless remains the dominant approach, or what actions can be taken to shift the sciences away from NHST. Therefore, the aim of our study was to explore the perceived barriers and facilitators, as well as behavioral intentions related to the use of NHST and alternatives statistical procedures, among all relevant stakeholders in the scientific system.
Theoretical framework
In designing our study, we used two theories. Firstly, we used the ‘diffusion of innovation theory’ of Rogers [ 20 ]. This theory describes the dissemination of an innovation as a process consisting of four elements: 1) an innovation is 2) communicated through certain channels 3) over time 4) among the members of a social system [ 20 ]. In the current study, the innovation consists of the idea that we should stop with the default use of NHST and instead consider using alternative methods for drawing conclusions from empirical data. The science system forms the social structure in which the innovation should take place. The most important members, and potential adopters of the innovation, we identified are researchers, lecturers, editors of scientific journals and representatives of funding agencies. Rogers describes phases in the adoption process, which coincide with characteristics of the (potential) adopters of the idea: 1) innovators, 2) early adopters, 3) early majority adopters, 4) late majority adopters and 5) laggards. Innovators are the first to adopt an innovation. There are few innovators but these few are very important for bringing in new ideas. Early adopters form the second group to adopt an innovation. This group includes opinion leaders and role models for other stakeholders. The largest group consists of the early and late majority who follow the early adopters, and then there is a smaller group of laggards who resist the innovation until they are certain the innovation will not fail. The process of innovation adoption by individuals is described as a normal distribution ( Fig 1 ). For these five groups, the adoption of a new idea is influenced by the following five characteristics of the innovative idea and 1) its relative advantage, 2) its compatibility with current experiences, 3) its complexity, 4) its flexibility, and 5) its visibility [ 20 ]. Members of all four stakeholder groups could play an important role in the diffusion of the innovation of replacing NHST by its alternatives.

The innovativeness dimension, measured by the time at which an individual from an adopter category adopts an innovation. Each category is one of more standard deviations removed from the average time of adoption [ 20 ].
Another important theory for our study is the ‘theory of planned behavior’, that was developed in the 1960s [ 21 ]. This theory describes how human behavior in a certain context can be predicted and explained. The theory was updated in 2010, under the name ‘the reasoned action approach’ [ 22 ]. A central factor in this theory is the intention to perform a certain behavior, in this case, to change the default use of NHST. According to the theory, people’s intentions determine their behaviors. An intention indexes to what extent someone is motivated to perform the behavior. Intentions are determined by three independent determinants: the person’s attitudes toward the behavior—the degree to which a person sees the behavior as favorable or unfavorable, perceived subjective norms regarding the behavior—the perceived social pressure to perform the behavior or not, and perceptions of control regarding the behavior—the perceived ease or difficulty of performing the behavior. Underlying (i.e. responsible for) these three constructs are corresponding behavioral, normative, and control beliefs [ 21 , 22 ] (see Fig 2 ).

Both theories have served as a lens for both data collection and analysis. We used sensitizing concepts [ 23 ] within the framework of the grounded theory approach [ 24 ] from both theories as a starting point for this qualitative study, and more specifically, for the topic list for the interviews and focus groups, providing direction and guidance for the data collection and data analysis.
Many of the concepts of Rogers’ and Fishbein and Ajzen’s theory can be seen as facilitators and barriers for embracing and implementing innovation in the scientific system.
A qualitative study among stakeholders using semi-structured interviews and focus groups was performed. Data collection and analysis were guided by the principle of constant comparison traditional to the grounded theory approach we followed [ 24 ]. The grounded theory is a methodology that uses inductive reasoning, and aims to construct a theory through the collection and analysis of data. Constant comparison is the iterative process whereby each part of the data that emerges from the data analysis is compared with other parts of the data to thoroughly explore and validate the data. Concepts that have been extracted from the data are tagged with codes that are grouped into categories. These categories constitute themes, which (may) become the basis for a new theory. Data collection and analysis were continued until no new information was gained and data saturation had likely occurred within the identified themes.
The target population consisted of stakeholders relevant to our topic: junior and senior researchers, lecturers in statistics, editors of scientific journals and program leaders of funding agencies (see Tables Tables1 1 and and2). 2 ). We approached participants in the field of medical sciences, health- and life sciences and psychology. In line with the grounded theory approach, theoretical sampling was used to identify and recruit eligible participants. Theoretical sampling is a form of purposive sampling. This means that we aimed to purposefully select participants, based on their characteristics that fit the parameters of the research questions [ 25 ]. Recruitment took place by approaching persons in our professional networks and or the networks of the approached persons.
*The numbers between brackets represents the number of participants that were also interviewed.
Data collection
We conducted individual semi-structured interviews followed by focus groups. The aim of the interviews was to gain insight into the views of participants on the use of NHST and alternative methods and to examine potential barriers and facilitators related to these methods. The aim of the focus groups was to validate and further explore interview findings and to develop a comprehensive understanding of participants’ views and beliefs.
For the semi-structured interviews, we used a topic list (see Appendix 1 in S1 Appendix ). Questions addressed participants’ knowledge and beliefs about the concept of NHST, their familiarity with NHST, perceived attractiveness and drawbacks of the use of NHST, knowledge of the current NHST debate, knowledge of and views on alternative procedures and their views on the future of NHST. The topic list was slightly adjusted based on the interviews with editors and representatives from funding agencies (compared to the topic list for interviews with researchers and lecturers). Questions particularly focused on research and education were replaced by questions focused on policy (see Appendix 1 in S1 Appendix ).
The interviews were conducted between October 2017 and June 2018 by two researchers (L.v.G. and J.S.), both trained in qualitative research methods. Interviews lasted about one hour (range 31–86 minutes) and were voice-recorded. One interview was conducted by telephone; all others were face to face and took place at a location convenient for the participants, in most cases the participants’ work location.
Focus groups
During the focus groups, important themes that emerged from the interviews were discussed and explored. These include perceptions on NHST and alternatives and essential conditions to shift away from the default use of NHST.
Five focus groups included representatives from the different stakeholder groups. One focus group was homogenous, including solely lecturers. The focus groups consisted of ‘old’ as well as ‘new’ participants, that is, some of the participants of the focus groups were also in the interview sample. We also selected persons that were open for further contribution to the NHST debate and were willing to help think about (implementing) alternatives for NHST.
The focus groups were conducted between September and December 2018 by three researchers (L.v.G., J.S. and A.d.K.), all trained in qualitative research methods. The focus groups lasted about one-and-a-half hours (range 86–100 minutes).
Data analysis
All interviews and focus groups were transcribed verbatim. Atlas.ti 8.0 software was used for data management and analysis. All transcripts were read thoroughly several times to identify meaningful and relevant text fragments and analyzed by two researchers (J.S. and L.v.G.). Deductive predefined themes and theoretical concepts were used to guide the development of the topic list for the semi-structured interviews and focus groups, and were used as sensitizing concepts [ 23 ] in data collection and data analysis. Inductive themes were identified during the interview process and analysis of the data [ 26 ].
Transcripts were open-, axial- and selectively coded by two researchers (J.S. and L.v.G.). Open coding is the first step in the data-analysis, whereby phenomena found in the text are identified and named (coded). With axial coding, connections between codes are drawn. Selective coding is the process of selecting one central category and relating all other categories to that category, capturing the essence of the research. The constant comparison method [ 27 ] was applied allowing emerging (sub)themes to be fully explored. First, the two researchers independently developed a set of initial codes. Subsequently, findings were discussed until consensus was reached. Codes were then grouped into categories that were covered under subthemes, belonging to main themes. Finally, a theory substantiating the prevailing use of NHST was developed based on the main themes and subthemes.
Ethical issues
This research was conducted in accordance with the Dutch "General Data Protection Regulation" and the “Netherland’s code of conduct for research integrity”. The research protocol had been submitted for review and approved by the ethical review committee of the VU Faculty of Behavioral and Movement Sciences. In addition, the project had been submitted to the Medical Ethics Committee (METC) of the Amsterdam University Medical Centre who decided that the project is not subject to the Medical Research (Human Subjects) Act ( WMO). At the start of data collection, all participants signed an informed consent form.
A full study protocol, including a detailed data analysis plan, was preregistered ( https://osf.io/4qg38/ ). At the start of this study, preregistration forms for qualitative studies were not developed yet. Therefore, preregistration for this study is based on an outdated form. Presently, there is a preregistration form available for qualitative studies [ 28 ]. Information about data collection, data management, data sharing and data storage is described in a Data Management Plan. Sensitive data is stored in Darkstor, an offline archive for storing sensitive information or data (information that involves i.e., privacy or copyright). As the recordings and transcripts of the interviews and focus groups contain privacy-sensitive data, these files are archived in Darkstor and can be accessed only on request by authorized individuals (i.e., the original researcher or a research coordinator) (Data requests can be send to ln.uv@mdr ). Non-sensitive data is stored in DANS ( https://doi.org/10.17026/dans-2at-nzfs ) (Data Archiving and Networked Services; the Netherlands institute for permanent access to digital research resources).
Participant characteristics
Twenty-nine individual interviews and six focus groups were conducted. The focus groups included four to six participants per session. A total of 47 participants were included in the study (13 researchers, 15 lecturers, 11 editors of scientific journals and 8 representatives of funding agencies). Twenty-nine participants were interviewed. Twenty-seven participants took part in the focus group. Nine of the twenty-seven participants were both interviewed and took part in the focus groups. Some participants had multiple roles (i.e., editor and researcher, editor and lecturer or lecturer and researcher) but were classified based on their primary role (assistant professors were classified as lecturers). The lecturers in statistics in our sample were not statisticians themselves. Although they all received training in statistics, they were primarily trained as psychologists, medical doctors, or health scientists. Some lecturers in our sample taught an applied subject, with statistics as part of it. Other lectures taught Methodology and Statistics courses. Statistical skills and knowledge among lecturers varied from modest to quite advanced. Statistical skills and knowledge among participants from the other stakeholder groups varied from poor to quite advanced. All participants were working in the Netherlands. A general overview of the participants is presented in Table 1 . Participant characteristics split up by interviews and focus groups are presented in Table 2 .
Three main themes with sub-themes and categories emerged ( Fig 3 ): the green-colored compartments hold the three main themes: The scientific climate , The scientific duty and Reactivity . Each of these three main themes consists of subthemes, depicted by the yellow-colored compartments. In turn, some (but not all) of the 9 subthemes also have categories. These ‘lower level’ findings are not included in the figure but will be mentioned in the elaboration on the findings and are depicted in Appendix 2 in S1 Appendix . Fig 3 shows how the themes are related to each other. The blue arrows indicate that the themes are interrelated; factors influence each other. The scientific climate affects the way stakeholders perceive and fulfil their scientific duty, the way stakeholders give substance to their scientific duty shapes and maintain the scientific climate. The scientific duty and the scientific climate cause a state of reactivity. Many participants have adopted a ’wait and see’ attitude regarding behavioral changes with respect to statistical methods. They feel dependent on someone else’s action. This leads to a reactive (instead of a proactive) attitude and a low sense of responsibility. ‘Reactivity’ is the core theme, explaining the most critical problem with respect to the continuous and ubiquitous use of NHST.

Main themes and subthemes are numbered. Categories are mentioned in the body of the text in bold. ‘P’ stands for participant; ‘I’ stands for interviewer.
1. The scientific climate
The theme, ‘the scientific climate’, represents researchers’ (Dutch) perceptions of the many written and unwritten rules they face in the research environment. This theme concerns the opportunities and challenges participants encounter when working in the science system. Dutch academics feel pressured to publish fast and regularly, and to follow conventions and directions of those on whom they depend. They feel this comes at the expense of the quality of their work. Thus, the scientific climate in the Netherlands has a strong influence on the behavior of participants regarding how they set their priorities and control the quality of their work.
1 . 1 Quality control . Monitoring the quality of research is considered very important. Researchers, funding agencies and editors indicate they rely on their own knowledge, expertise, and insight, and those of their colleagues, to guarantee this quality. However, editors or funding agencies are often left with little choice when it comes to compiling an evaluation committee or a review panel. The choice is often like-knows-like-based. Given the limited choice, they are forced to trust the opinion of their consultants, but the question is whether this is justified.
I: “The ones who evaluate the statistics, do they have sufficient statistical knowledge?” P: “Ehhr, no, I don’t think so.” I: “Okay, interesting. So, there are manuscripts published of which you afterwards might think….” P: “Yes yes.” (Interview 18; Professor/editor, Medical Sciences)
1 . 2 Convention . The scientific system is built on mores and conventions, as this participant describes:
P: “There is science, and there is the sociology of science, that is, how we talk to each other, what we believe, how we connect. And at some point, it was agreed upon that we would talk to each other in this way.” (Interview 28, researcher, Medical Sciences)
And to these conventions, one (naturally) conforms. Stakeholders copy behavior and actions of others within their discipline, thereby causing particular behaviors and values to become conventional or normative. One of those conventions is the use of NHST and p-values. Everyone is trained with NHST and is used to applying this method. Another convention is the fact that significant results mean ‘success’, in the sense of successful research and being a successful researcher. Everyone is aware that ‘p is smaller than 0.05’ means the desired results are achieved and that publication and citation chances are increased.
P: “You want to find a significant result so badly. (…) Because people constantly think: I must find a significant result, otherwise my study is worthless.” (Focus group 4, lecturer, Medical Sciences)
Stakeholders rigidly hold on to the above-mentioned conventions and are not inclined to deviate from existing norms; they are, in other words, quite conservative . ‘We don’t know any better’ has been brought up as a valid argument by participants from various stakeholder groups to stick to current rules and conventions. Consequently, the status quo in the scientific system is being maintained.
P: “People hold on to….” I: ‘Everyone maintains the system?’ P: ‘Yes, we kind of hang to the conservative manner. This is what we know, what someone, everyone, accepts.” (Interview 17, researcher, Health Sciences)
Everyone is trained with NHST and considers it an accessible and easy to interpret method. The familiarity and perceived simplicity of NHST, user-friendly software such as SPSS and the clear cut-off value for significance are important facilitators for the use of NHST and at the same time barriers to start using alternative methods. Applied researchers stressed the importance of the accessibility of NHST as a method to test hypotheses and draw conclusions. This accessibility also justifies the use of NHST when researchers want to communicate their study results and messages in understandable ways to their readership.
P: “It is harder, also to explain, to use an alternative. So, I think, but maybe I’m overstepping, but if you want to go in that direction [alternative methods] it needs to be better facilitated for researchers. Because at the moment… I did some research, but, you know, there are those uncommon statistical packages.” (Interview 16, researcher/editor, Medical Sciences)
1 . 3 Publication pressure . Most researchers mentioned that they perceive publication pressure. This motivates them to use NHST and hope for significant results, as ‘significant p-values’ increase publication chances. They perceive a high workload and the way the scientific reward system is constructed as barriers for behavioral change pertaining to the use of statistical methods; potential negative consequences for publication and career chances prevent researchers from deviating from (un)written rules.
P: “I would like to learn it [alternative methods], but it might very well be that I will not be able to apply it, because I will not get my paper published. I find that quite tricky.” (Interview 1, Assistant Professor, Health Sciences)
2. The scientific duty
Throughout the interviews, participants reported a sense of duty in several variations. “What does it mean to be a scientific researcher?” seemed to be a question that was reflected upon during rather than prior to the interview, suggesting that many scientists had not really thought about the moral and professional obligations of being a scientist in general—let alone what that would mean for their use of NHST. Once they had given it some thought, the opinions concerning what constitutes the scientific duty varied to a large extent. Some participants attached great importance to issues such as reproducibility and transparency in scientific research and continuing education and training for researchers. For others, these topics seemed to play a less important role. A distinction was made between moral and professional obligations that participants described concerning their scientific duty.
2 . 1 Moral obligation . The moral obligation concerns issues such as doing research in a thorough and honest way, refraining from questionable research practices (QRPs) and investing in better research. It concerns tasks and activities that are not often rewarded or acknowledged.
Throughout the interviews and the focus groups, participants very frequently touched upon the responsibility they felt for doing ‘the right thing’ and making the right choice in doing research and using NHST, in particular. The extent to which they felt responsible varied among participants. When it comes to choices during doing research—for example, drawing conclusions from data—participants felt a strong sense of responsibility to do this correctly. However, when it comes to innovation and new practices, and feeling responsible for your own research, let alone improving scientific practice in general, opinions differed. This quotation from one of the focus groups illustrates that:
P1: “If you people [statisticians, methodologists] want me to improve the statistics I use in my research, then you have to hand it to me. I am not going to make any effort to improve that myself. “P3: “No. It is your responsibility as an academic to keep growing and learning and so, also to start familiarizing yourself when you notice that your statistics might need improvement.” (Focus group 2, participant 1 (PhD researcher, Medical Sciences) and 3 (Associate Professor, Health Sciences)
The sense of responsibility for improving research practices regarding the use of NHST was strongly felt and emphasized by a small group of participants. They emphasized the responsibility of the researcher to think, interpret and be critical when interpreting the p -value in NHST. It was felt that you cannot leave that up to the reader. Moreover, scrutinizing and reflecting upon research results was considered a primary responsibility of a scientist, and failing to do so, as not living up to what your job demands you to do:
P: “Yes, and if I want to be very provocative—and I often want that, because then people tend to wake up and react: then I say that hiding behind alpha.05 is just scientific laziness. Actually, it is worse: it is scientific cowardice. I would even say it is ‘relieving yourself from your duty’, but that may sound a bit harsh…” (Interview 2, Professor, Health Sciences)
These participants were convinced that scientists have a duty to keep scientific practice in general at the highest level possible.
The avoidance of questionable research practices (QRPs) was considered a means or a way to keep scientific practices high level and was often touched upon during the interviews and focus groups as being part of the scientific duty. Statisticians saw NHST as directly facilitating QRPs and providing ample examples of how the use of NHST leads to QRPs, whereas most applied researchers perceived NHST as the common way of doing research and were not aware of the risks related to QRPs. Participants did mention the violation of assumptions underlying NHST as being a QRP. Then, too, participants considered overinterpreting results as a QRP, including exaggerating the degree of significance. Although participants stated they were careful about interpreting and reporting p-values, they ‘admitted’ that statistical significance was a starting point for them. Most researchers indicated they search for information that could get their study published, which usually includes a low p-value (this also relates to the theme ‘Scientific climate’).
P: “We all know that a lot of weight is given to the p-value. So, if it is not significant, then that’s the end of it. If it ís significant, it just begins.” (Interview 5, lecturer, Psychology)
The term ‘sloppy science’ was mentioned in relation to efforts by researchers to reduce the p -value (a.k.a. p-hacking, data-dredging, and HARKing. HARKing is an acronym that refers to the questionable research question of Hypothesizing After the Results are Known. It occurs when researchers formulate a hypothesis after the data have been collected and analyzed, but make it look like it is an a priori hypothesis [ 29 ]). Preregistration and replication were mentioned as being promising solutions for some of the problems caused by NHST.
2 . 2 . Professional obligation . The theme professional obligation reflects participants’ expressions about what methodological knowledge scientists should have about NHST. In contrast moral obligations, there appeared to be some consensus about scientists’ professional obligations. Participants considered critical evaluation of research results a core professional obligation. Also, within all the stakeholder groups, participants agreed that sufficient statistical knowledge is required for using NHST, but they varied in their insights in the principles, potential and limitations of NHST. This also applied to the extent to which participants were aware of the current debate about NHST.
Participants considered critical thinking as a requirement for fulfilling their professional obligation. It specifically refers to the process of interpreting outcomes and taking all relevant contextual information into consideration. Critical thinking was not only literally referred to by participants, but also emerged by interpreting text fragments on the emphasis within their research. Researchers differed quite strongly in where the emphasis of their research outcomes should be put and what kind of information is required when reporting study results. Participants mentioned the proven effectiveness of a particular treatment, giving a summary of the research results, effect sizes, clinical relevance, p-values, or whether you have made a considerable contribution to science or society.
P: “I come back to the point where I said that people find it arbitrary to state that two points difference on a particular scale is relevant. They prefer to hide behind an alpha of 0.05, as if it is a God given truth, that it counts for one and for all. But it is just as well an invented concept and an invented guideline, an invented cut-off value, that isn’t more objective than other methods?” (Interview 2, Professor, Health Sciences)
For some participants, especially those representing funding agencies, critical thinking was primarily seen as a prerequisite for the utility of the research. The focus, when formulating the research question and interpreting the results, should be on practical relevance and the contribution the research makes to society.
The term ‘ignorance’ arose in the context of the participants’ concern regarding the level of statistical knowledge scientists and other stakeholders have versus what knowledge they should have to adequately apply statistical analysis in their research. The more statistically competent respondents in the sample felt quite strongly about how problematic the lack of knowledge about NHST is among those who regularly use it in their research, let alone the lack of knowledge about alternative methods. They felt that regularly retraining yourself in research methods is an essential part of the professional obligation one has. Applied researchers in the sample agreed that a certain level of background knowledge on NHST was required to apply it properly to research and acknowledged their own ignorance. However, they had different opinions about what level of knowledge is required. Moreover, not all of them regarded it as part of their scientific duty to be informed about all ins and outs of NHST. Some saw it as the responsibility of statisticians to actively inform them (see also the subtheme periphery). Some participants were not aware of their ignorance or stated that some of their colleagues are not aware of their ignorance, i.e., that they are unconsciously incompetent and without realizing it, poorly understood what the p-value and associated outcome measures actually mean.
P: “The worst, and I honestly think that this is the most common, is unconsciously incompetent, people don’t even understand that…” I: “Ignorance.” P: “Yes, but worse, ignorant and not even knowing you are ignorant.” (Interview 2, Professor, Health Sciences)
The lack of proper knowledge about statistical procedures was especially prevalent in the medical sciences. Participants working in or with the medical sciences all confirmed that there is little room for proper statistical training for medical students and that the level of knowledge is fairly low. NHST is often used because of its simplicity. It is especially attractive for medical PhD students because they need their PhD to get ahead in their medical career instead of pursuing a scientific career.
P: “I am not familiar with other ways of doing research. I would really like to learn, but I do not know where I could go. And I do not know whether there are better ways. So sometimes I do read studies of which I think: ‘this is something I could investigate with a completely different test. Apparently, this is also possible, but I don’t know how.’ Yes, there are courses, but I do not know what they are. And here in the medical center, a lot of research is done by medical doctors and these people have hardly been taught any statistics. Maybe they will get one or two statistics courses, they know how to do a t-test and that is about it. (…) And the courses have a very low level of statistics, so to say.” (Interview 1, Assistant Professor, Health Sciences)
Also, the term ‘ awareness ’ arose. Firstly, it refers to being conscious about the limitations of NHST. Secondly, it refers to the awareness of the ongoing discussions about NHST and more broadly, about the replication crisis. The statisticians in the sample emphasized the importance of knowing that NHST has limitations and that it cannot be considered the holy grail of data analysis. They also emphasized the importance of being aware of the debate. A certain level of awareness was considered a necessary requirement for critical thinking. There was variation in that awareness. Some participants were quite informed and were also fairly engaged in the discussion whereas others were very new to the discussion and larger contextual factors, such as the replication crisis.
I: “Are you aware of the debate going on in academia on this topic [NHST]? P: “No, I occasionally see some article sent by a colleague passing by. I have the idea that something is going on, but I do not know how the debate is conducted and how advanced it is. (Interview 6, lecturer, Psychology)
With respect to the theme, ‘the scientific duty’, participants differed to what extent they felt responsible for better and open science, for pioneering, for reviewing, and for growing and learning as a scientist. Participants had one commonality: although they strived for adherence to the norms of good research, the rampant feeling is that this is very difficult, due to the scientific climate. Consequently, participants perceive an internal conflict : a discrepancy between what they want or believe , and what they do . Participants often found themselves struggling with the responsibility they felt they had. Making the scientifically most solid choice was often difficult due to feasibility, time constraints, or certain expectations from supervisors (this is also directly related to the themes ‘Scientific climate’ and ‘Reactivity’). Thus, the scientific climate strongly influences the behavior of scientists regarding how they set their priorities and fulfill their scientific duties. The strong sense of scientific duty was perceived by some participants as a facilitator and by others as a barrier for the use of alternative methods.
3. Reactivity
A consequence of the foregoing factors is that most stakeholders have adopted a reactive attitude and behave accordingly. People are disinclined to take responsibility and await external signals and initiatives of others. This might explain why NHST is being continuously used and remains the default procedure to make inferences about population effects.
The core theme ‘reactivity’ can be explained by the following subthemes and categories:
3 . 1 Periphery . The NHST-problem resides in the periphery in several ways. First, it is a subject that is not given much priority. Secondly, some applied researchers and editors believe that methodological knowledge, as it is not their field of expertise, should not be part of their job requirement. This also applies to the NHST debate. Thirdly, and partly related to the second point, there is a lack of cooperation within and between disciplines.
The term ‘ priority’ was mentioned often when participants were asked to what extent the topic of NHST was subject of discussion in their working environment. Participants indicated that (too) little priority is given to statistics and the problems related to the subject. There is simply a lot going on in their research field and daily work, so there are always more important or urgent issues on the agenda.
P: “Discussions take place in the periphery; many people find it complicated. Or are just a little too busy.” (Interview 5, lecturer, Psychology)
As the NHST debate is not prioritized, initiatives with respect to this issue are not forthcoming. Moreover, researchers and lecturers claim there is neither time nor money available for training in statistics in general or acquiring more insight and skills with respect to (the use of) alternative methods. Busy working schedules were mentioned as an important barrier for improving statistical knowledge and skills.
P: “Well you can use your time once, so it is an issue low on the priority list.” (Focus group 5, researcher, Medical Sciences)
The NHST debate is perceived as the domain of statisticians and methodologists. Also, cooperation between different domains and domain-specific experts is perceived as complicated, as different perceptions and ways of thinking can clash. Therefore, some participants feel that separate worlds should be kept separate; put another way: stick to what you know!
P: “This part is not our job. The editorial staff, we have the assignment to ensure that it is properly written down. But the discussion about that [alternatives], that is outside our territory.” (Interview 26, editor, Medical Sciences)
Within disciplines, individuals tend to act on their own, not being aware that others are working on the same subject and that it would be worthwhile to join forces. The interviews and focus groups exposed that a modest number of participants actively try to change the current situation, but in doing that, feel like lone voices in the wilderness.
P1: “I mean, you become a lone voice in the wilderness.” P2: “Indeed, you don’t want that.” P1: “I get it, but no one listens. There is no audience.” (Focus Group 3, P1: MD, lecturer, medical Sciences, P2: editor, Medical Sciences)
To succeed at positive change, participants emphasized that it is essential that people (interdisciplinary) cooperate and join forces, rather than operate on individual levels, focusing solely on their own working environment.
The caution people show with respect to taking initiative is reenforced by the fear of encountering resistance from their working environment when one voices that change regarding the use of NHST is needed. A condition that was mentioned as essential to bring about change was tactical implementation , that is, taking very small steps. As everyone is still using NHST, taking big steps brings the risk of losing especially the more conservative people along the way. Also, the adjustment of policy, guidelines and educational programs are processes for which we need to provide time and scope.
P: “Everyone still uses it, so I think we have to be more critical, and I think we have to look at some kind of culture change, that means that we are going to let go of it (NHST) more and we will also use other tests, that in the long term will overthrow NHST. I: and what about alternatives? P: I think you should never be too fanatic in those discussion, because then you will provoke resistance. (…) That is not how it works in communication. You will touch them on a sore spot, and they will think: ‘and who are you?’ I: “and what works?” P: “well, gradualness. Tell them to use NHST, do not burn it to the ground, you do not want to touch peoples work, because it is close to their hearts. Instead, you say: ‘try to do another test next to NHST’. Be a pioneer yourself.” (Interview 5, lecturer, Psychology)
3 . 2 . Efficacy . Most participants stated they feel they are not in the position to initiate change. On the one hand, this feeling is related to their hierarchical positions within their working environments. On the other hand, the feeling is caused by the fact that statistics is perceived as a very complex field of expertise and people feel they lack sufficient knowledge and skills, especially about alternative methods.
Many participants stated they felt little sense of empowerment, or self-efficacy. The academic system is perceived as hierarchical, having an unequal balance of power. Most participants believe that it is not in their power to take a lead in innovative actions or to stand up against establishment, and think that this responsibility lies with other stakeholders, that have more status .
P: “Ideally, there would be a kind of an emergency letter from several people whose names open up doors, in which they indicate that in the medical sciences we are throwing away money because research is not being interpreted properly. Well, if these people that we listen to send such an emergency letter to the board of The Netherlands Organization for Health Research and Development [the largest Dutch funding agency for innovation and research in healthcare], I can imagine that this will initiate a discussion.” (…) I: “and with a big name you mean someone from within the science system? P: well, you know, ideally a chairman, or chairmen of the academic medical center. At that level. If they would put a letter together. Yes, that of course would have way more impact. Or some prominent medical doctors, yes, that would have more impact, than if some other person would send a letter yes.” (Interview 19, representative from funding agency, Physical Sciences)
Some participants indicated that they did try to make a difference but encountered too much resistance and therefore gave up their efforts. PhD students feel they have insufficient power to choose their own directions and make their own choices.
P: I am dependent on funding agencies and professors. In the end, I will write a grant application in that direction that gives me the greatest chance of eventually receiving that grant. Not primarily research that I think is the most optimal (…) If I know that reviewers believe the p-value is very important, well, of course I write down a method in which the p-value is central.” (Focus group 2, PhD-student, Medical Sciences)
With a sense of imperturbability, most participants accept that they cannot really change anything.
Lastly, the complexity of the subject is an obstacle for behavioral change. Statistics is perceived as a difficult subject. Participants indicate that they have a lack of knowledge and skills and that they are unsure about their own abilities. This applies to the ‘standard’ statistical methods (NHST), but to a greater extent to alternative methods. Many participants feel that they do not have the capacity to pursue a true understanding of (alternative) statistical methods.
P: “Statistics is just very hard. Time and again, research demonstrates that scientists, even the smartest, have a hard time with statistics.” (Focus group 3, PhD researcher, Psychology)
3 . 3 . Interdependency . As mentioned, participants feel they are not in a sufficiently strong position to take initiative or to behave in an anti-establishment manner. Therefore, they await external signals from people within the scientific system with more status, power, or knowledge. This can be people within their own stakeholder group, or from other stakeholder groups. As a consequence of this attitude, a situation arises in which peoples’ actions largely depend on others. That is, a complex state of interdependency evolves: scientists argue that if the reward system does not change, they are not able to alter their statistical behavior. According to researchers, editors and funding agencies are still very much focused on NHST and especially (significant) p-values, and thus, scientists wait for editors and funders to adjust their policy regarding statistics:
P: “I wrote an article and submitted it to an internal medicine journal. I only mentioned confidence intervals. Then I was asked to also write down the p-values. So, I had to do that. This is how they [editors] can use their power. They decide.” (Interview 1, Assistant Professor, Health Sciences)
Editors and funders in their turn claim they do not maintain a strict policy. Their main position is that scientists should reach consensus about the best statistical procedure, and they will then adjust their policy and guidelines.
P: “We actually believe that the research field itself should direct the quality of its research, and thus, also the discussions.” (Interview 22, representative from funding agency, Neurosciences)
Lecturers, for their part, argue that they cannot revise their educational programs due to the academic system, and university policies are adapted to NHST and p-values.
As most participants seem not to be aware of this process, a circle of interdependency arises that is difficult to break.
P: “Yes, the stupid thing about this perpetual circle is that you are educating people, let’s say in the department of cardiology. They must of course grow, and so they need to publish. If you want to publish you must meet the norms and values of the cardiology journals, so they will write down all those p-values. These people are trained and in twenty years they are on the editorial board of those journals, and then you never get rid of it [the p-value].” (Interview 18, Professor, editor, Medical Sciences)
3 . 4 . Degree of eagerness . Exerting certain behavior or behavioral change is (partly) determined by the extent to which people want to employ particular behavior, their behavioral intention [ 22 ]. Some participants indicated they are willing to change their behavior regarding the use of statistical methods, but only if it is absolutely necessary, imposed or if they think that the current conventions have too many negative consequences. Thus, true, intrinsic will-power to change behavior is lacking among these participants. Instead, they have a rather opportunistic attitude, meaning that their behavior is mostly driven by circumstances, not by principles.
P: “If tomorrow an alternative is offered by people that make that call, than I will move along. But I am not the one calling the shots on this issue.” (Interview 26, editor, Medical Sciences)
In addition, pragmatism often outweighs the perceived urgency to change. Participants argue they ‘just want to do their jobs’ and consider the practical consequences mainly in their actions. This attitude creates a certain degree of inertia. Although participants claim they are willing to change their behavior, this would contain much more than ‘doing their jobs, and thus, in the end, the NHST-debate is subject to ‘coffee talk’. People are open to discussion, but when it comes to taking action (and motivating others to do so), no one takes action.
P: “The endless analysis of your data to get something with a p-value less than 0.05… There are people that are more critical about that, and there are people that are less critical. But that is a subject for during the coffee break.” (Interview 18, professor, editor, Medical Sciences)
The goal of our study was to acquire in-depth insight into reasons why so many stakeholders from the scientific system keep using NHST as the default method to draw conclusions, despite its many well-documented drawbacks. Furthermore, we wanted to gain insight into the reasons for their reluctance to apply alternative methods. Using a theoretical framework [ 20 , 21 ], several interrelated facilitators and barriers associated with the use of NHST and alternative methods were identified. The identified factors are subsumed under three main themes: the scientific climate, the scientific duty and reactivity. The scientific climate is dominated by conventions, behavioral rules, and beliefs, of which the use of NHST and p-values is part. At the same time, stakeholders feel they have a (moral or professional) duty. For many participants, these two sides of the same coin are incompatible, leading to internal conflicts. There is a discrepancy between what participants want and what they do . As a result of these factors, the majority feels dependent on others and have thereby become reactive. Most participants are not inclined to take responsibility themselves but await action and initiatives from others. This may explain why NHST is still the standard and used by almost everyone involved.
The current study is closely related to the longstanding debate regarding NHST which recently increased to a level not seen before. In 2015, the editors of the journal ‘Basic and Applied Social Psychology’ (BASP) prohibited the use of NHST (and p-values and confidence intervals) [ 30 ]. Subsequently, in 2016, the American Statistical Association published the so-called ‘Statement on p-values’ in the American Statistician. This statement consists of critical standpoints regarding the use of NHST and p-values and warns against the abuse of the procedure. In 2019, the American Statistician devoted an entire edition to the implementation of reforms regarding the use of NHST; in more than forty articles, scientists debated statistical significance, advocated to embrace uncertainty, and suggested alternatives such as the use of s-values, False Positive Risks, reporting results as effect sizes and confidence intervals and more holistic approaches to p-values and outcome measures [ 31 ]. In addition, in the same year, several articles appeared in which an appeal was made to stop using statistical significance testing [ 32 , 33 ]. A number of counter-reactions were published [ 34 – 36 ], stating (i.e.) that banning statistical significance and, with that, abandoning clear rules for statistical analyses may create new problems with regard to statistical interpretation, study interpretations and objectivity. Also, some methodologists expressed the view that under certain circumstances the use of NHST and p-values is not problematic and can in fact provide useful answers [ 37 ]. Until recently, the NHST-debate was limited to mainly methodologists and statisticians. However, a growing number of scientists are getting involved in this lively debate and believe that a paradigm shift is desirable or even necessary.
The aforementioned publications have constructively contributed to this debate. In fact, since the publication of the special edition of the American Statistician, numerous scientific journals published editorials or revised, to a greater or lesser extent, their author guidelines [ 38 – 45 ]. Furthermore, following the American Statistical Association (ASA), the National Institute of Statistical Sciences (NISS) in the United States has also taken up the reform issue. However, real changes are still barely visible. It takes a long time before these kinds of initiatives translate into behavioral changes, and the widespread adoption by most of the scientific community is still far from accomplished. Debate alone will not lead to real changes, and therefore, our efforts to elucidate behavioral barriers and facilitators could provide a framework for potential effective initiatives that could be taken to reduce the default use of NHST. In fact, the debate could counteract behavioral change. If there is no consensus among statisticians and methodologists (the innovators), changing behavior cannot be expected from stakeholders with less statistical and methodological expertise. In other words, without agreement among innovators, early adopters might be reluctant to adopt the innovation.
Research has recently been conducted to explore the potential of behavioral change to improve Open Science behaviors. The adoption of open science behavior has increased in the last years, but uptake has been slow, due to firm barriers such as a lack of awareness about the subject, concerns about constrainment of the creative process, worries about being “scooped” and holding on to existing working practices [ 46 ]. The development regarding open science practices and the parallels these lines of research shows with the current study, might be of benefit to subserve behavioral change regarding the use of statistical methods.
The described obstacles to change behavior are related to features of both the ‘innovative idea’ and the potential adopters of the idea. First, there are characteristics of ‘the innovation’ that form barriers. The first barrier is the complexity of the innovation: most participants perceive alternative methods as difficult to understand and to use. A second barrier concerns the feasibility of trying the innovation; most people do not feel flexible about trying out or experimenting with the new idea. There is a lack of time and monetary resources to get acquainted with alternative methods (for example, by following a course). Also, the possible negative consequences of the use of alternatives (lower publications chances, the chance that the statistical method and message is too complicated for one’s readership) is holding people back from experimenting with these alternatives. And lastly, it is unclear for most participants what the visibility of the results of the new idea are. Up until now, the debate has mainly taken place among a small group of statisticians and methodologists. Many researchers are still not aware of the NHST debate and the idea to shift away from NHST and use alternative methods instead. Therefore, the question is how easily the benefits of the innovation can be made visible for a larger part of the scientific community. Thus, our study shows that, although the compatibility of the innovation is largely consistent with existing values (participants are critical about (the use of) NHST and the p-value and believe that there are better alternatives to NHST), important attributes of the innovative idea negatively affect the rate of adoption and consequently the diffusion of the innovation.
Due to the barriers mentioned above, most stakeholders do not have the intention to change their behavior and adopt the innovative idea. From the theory of planned behavior [ 21 ], it is known that behavioral intentions directly relate to performances of behaviors. The strength of the intention is shaped by attitudes, subjective norms, and perceived power. If people evaluate the suggested behavior as positive (attitude), and if they think others want them to perform the behavior (subjective norm), this leads to a stronger intention to perform that behavior. When an individual also perceives they have enough control over the behavior, they are likely to perform it. Although most participants have a positive attitude towards the behavior, or the innovative idea at stake, many participants think that others in their working environment believe that they should not perform the behavior—i.e., they do not approve of the use of alternative methods (social normative pressure). This is expressed, for example, in lower publication chances, negative judgements by supervisors or failing the requirements that are imposed by funding agencies. Thus, the perception about a particular behavior—the use of alternative methods—is negatively influenced by the (perceived) judgment of others. Moreover, we found that many participants have a low self-efficacy, meaning that there is a perceived lack of behavioral control, i.e., their perceived ability to engage in the behavior at issue is low. Also, participants feel a lack of authority (in the sense of knowledge and skills, but also power) to initiate behavioral change. The existing subjective norms and perceived behavioral control, and the negative attitudes towards performing the behavior, lead to a lower behavioral intention, and, ultimately, a lower chance of the performance of the actual behavior.
Several participants mentioned there is a need for people of stature (belonging to the group of early adopters) to take the lead and break down perceived barriers. Early adopters serve as role models and have opinion leadership, and form the next group (after the innovators, in this case statisticians and methodologists) to adopt an innovative idea [ 20 ] ( Fig 2 ). If early adopters would stand up, conveying a positive attitude towards the innovation, breaking down the described perceived barriers and facilitating the use of alternatives (for example by adjusting policy, guidelines and educational programs and making available financial resources for further training), this could positively affect the perceived social norms and self-efficacy of the early and late majority and ultimately laggards, which could ultimately lead to behavioral change among all stakeholders within the scientific community.
A strength of our study is that it is the first empirical study on views on the use of NHST, its alternatives and reasons for the prevailing use of NHST. Another strength is the method of coding which corresponds to the thematic approach from Braun & Clarke [ 47 ], which allows the researcher to move beyond just categorizing and coding the data, but also analyze how the codes are related to each other [ 47 ]. It provides a rich description of what is studied, linked to theory, but also generating new hypotheses. Moreover, two independent researchers coded all transcripts, which adds to the credibility of the study. All findings and the coding scheme were discussed by the two researchers, until consensus was reached. Also, interview results were further explored, enriched and validated by means of (mixed) focus groups. Important themes that emanated from the interviews, such as interdependency, perceptions on the scientific duty, perceived disadvantages of alternatives or the consequences of the current scientific climate, served as starting points and main subjects of the focus groups. This set-up provided more data, and more insight about the data and validation of the data. Lastly, the use of a theoretical framework [ 20 , 21 ] to develop the topic list, guide the interviews and focus groups, and guide their analysis is a strength as it provides structure to the analysis and substantiation of the results.
A limitation of this study is its sampling method. By using the network of members of the project group, and the fact that a relatively high proportion of those invited to participate refused because they thought they knew too little about the subject to be able to contribute, our sample was biased towards participants that are (somewhat) aware of the NHST debate. Our sample may also consist of people that are relatively critical towards the use of NHST, compared to the total population of researchers. It was not easy to include participants who were indifferent about or who were pro-NHST, as those were presumably less willing to make time and participate in this study. Even in our sample we found that the majority of our participants solely used NHST and perceived it as difficult if not impossible to change their behavior. These perceptions are thus probably even stronger in the target population. Another limitation, that is inherent to qualitative research, is the risk of interviewer bias. Respondents are unable, unwilling, or afraid to answer questions in good conscience, and instead provide socially desirable answers. In the context of our research, people are aware that, especially as a scientist, it does not look good to be conservative, complacent, or ignorant, or not to be open to innovation and new ideas. Therefore, some participants might have given a too favorable view of themselves. The interviewer bias can also take the other direction when values and expectations of the interviewer consciously or unconsciously influence the answers of the respondents. Although we have tried to be as neutral and objective as possible in asking questions and interpreting answers, we cannot rule out the chance that our views and opinions on the use of NHST have at times steered the respondents somewhat, potentially leading to the foregoing desirable answers.
Generalizability is a topic that is often debated in qualitative research methodology. Many researchers do not consider generalizability the purpose of qualitative research, but rather finding in-depth insights and explanations. However, this is an unjustified simplification, as generalizing of findings from qualitative research is possible. Three types of generalization in qualitative research are described: representational generalization (whether what is found in a sample can be generalized to the parent population of the sample), inferential generalization (whether findings from the study can be generalized to other settings), and theoretical generalization (where one draws theoretical statements from the findings of the study for more general application) [ 48 ]. The extent to which our results are generalizable is uncertain, as we used a theoretical sampling method, and our study was conducted exclusively in the Netherlands. We expect that the generic themes (reactivity, the scientific duty and the scientific climate) are applicable to academia in many countries across the world (inferential generalization). However, some elements, such as the Dutch educational system, will differ to a more or lesser extent from other countries (and thus can only be representationally generalized). In the Netherlands there is, for example, only one educational route after secondary school that has an academic orientation (scientific education, equivalent to the US university level education). This route consists of a bachelor’s program (typically 3 years), and a master’s program (typically 1, 2 or 3 years). Not every study program contains (compulsory) statistical courses, and statistical courses differ in depth and difficulty levels depending on the study program. Thus, not all the results will hold for other parts of the world, and further investigation is required.
Our findings demonstrate how perceived barriers to shift away from NHST set a high threshold for behavioral change and create a circle of interdependency. Behavioral change is a complex process. As ‘the stronger the intention to engage in a behavior, the more likely should be its performance’[ 21 ], further research on this subject should focus on how to influence the intention of behavior; i.e. which perceived barriers for the use of alternatives are most promising to break down in order to increase the intention for behavioral change. The present study shows that negative normative beliefs and a lack of perceived behavioral control regarding the innovation among individuals in the scientific system is a substantial problem. When social norms change in favor of the innovation, and control over the behavior increases, then the behavioral intention becomes a sufficient predictor of behavior [ 49 ]. An important follow-up question will therefore be: how can people be enthused and empowered, to ultimately take up the use of alternative methods instead of NHST? Answering this question can, in the long run, lead to the diffusion of the innovation through the scientific system as a whole.
NHST has been the leading paradigm for many decades and is deeply rooted in our science system, despite longstanding criticism. The aim of this study was to gain insight as to why we continue to use NHST. Our findings have demonstrated how perceived barriers to make a shift away from NHST set a high threshold for actual behavioral change and create a circle of interdependency between stakeholders in the scientific system. Consequently, people find themselves in a state of reactivity, which limits behavioral change with respect to the use of NHST. The next step would be to get more insight into ways to effectively remove barriers and thereby increase the intention to take a step back from NHST. A paradigm shift within a couple of years is not realistic. However, we believe that by taking small steps, one at a time, it is possible to decrease the scientific community’s strong dependence on NHST and p-values.
Supporting information
S1 appendix, acknowledgments.
The authors are grateful to Anja de Kruif for her contribution to the design of the study and for moderating one of the focus groups.
Funding Statement
This research was funded by the NWO (Nederlandse Organisatie voor Wetenschappelijk Onderzoek; Dutch Organization for Scientific Research) ( https://www.nwo.nl/ ) The funders had no role in study design, data collection and analysis, decision to publish, or preparation of the manuscript.
Data Availability

- Resources / Blog
Data Analysis for Quantitative Data: Techniques and Tools for Accurate Insights
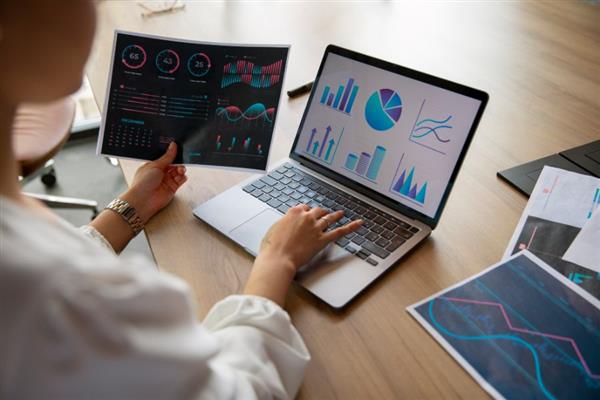
Data is the lifeblood of your organization, especially in a constantly evolving marketplace. Every day, you make decisions that impact achieving your organizational goals. If your organization isn’t digging into and exploring all the data it collects, it risks becoming less relevant in your customers’ eyes. Everyone in your organization, from top-level executives to entry-level employees, must understand how to interpret data to make impactful decisions.
Without data literacy , your organization is blind to what is happening and will make decisions based on guesswork and intuition, which may or may not yield the best decisions. Whether attempting to streamline your organization’s operations, boost sales growth, or improve customer satisfaction, understanding your data is the cornerstone of success.
To become data literate, you need to understand the two distinct types of data: quantitative and qualitative. After introducing these distinct types of data, we will look specifically at quantitative data and how to analyze it.
On this page :
What is Quantitative Data?
What's the difference between quantitative and qualitative data, 10 steps to analyzing quantitative data, the role of machine learning in quantitative data analysis, real-world applications of quantitative data analysis, future trends in quantitative data analysis.
Quantitative data refers to information that can be measured and expressed numerically. This type of data deals with numbers and things that can be measured objectively, such as height, width, length, temperature, humidity, and prices. Quantitative data can be analyzed using various statistical methods to uncover patterns, relationships, and trends within the data set.
Here are several examples of quantitative data:
- Height : A person's height of 175 centimeters.
- Temperature : The temperature is 25 Celsius.
- Income : A person's monthly salary is $3000.
- Age : Someone is 35 years old.
- Test Scores : A student scores 85 out of 100 on a math test.
- Number of Items Sold : A store sells 100 product units monthly.
- Distance : A car travels 50 kilometers an hour.
- Speed : A train might travel 100 kilometers per hour.
- Population : A city’s population is 1,000,000 people.
- Time : An event lasts 2 hours and 30 minutes.
As you can see, these examples represent quantities measured and expressed using numerical values.
Quantitative and qualitative data are the two primary types of data used in research and analysis, and they differ in terms of their nature, characteristics, and how they are collected and analyzed. The table below shows the differences between both data types:
Now that you know what each data type represents, the following table illustrates the differences between quantitative and qualitative data. The 'Price' column provides quantitative information, representing the cost of each product in dollars, whereas the 'Customer Review' column contains qualitative data featuring customer feedback regarding their experiences with the products.
While the two data types represent different data, one thing to remember is that quantitative and qualitative data complement each other. Quantitative data provides you with statistical insight, while qualitative data provides you with depth and context.
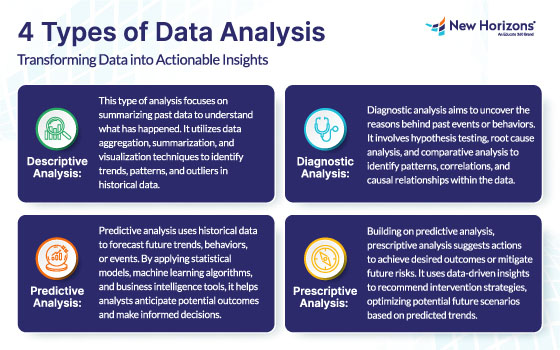
Now that we have defined quantitative data let’s look at the steps you would take to perform quantitative data analysis. Analyzing quantitative data will allow you to unlock insights that can help you make informed decisions. From defining your research objectives to interpreting your analysis results, each step will help you unleash the power of quantitative data.
Following the steps above will provide you with the means to perform effective quantitative data analysis. Using the insights you uncover from your data will help guide your decision-making processes, helping your organization to meet or surpass its goals and objectives.
Any organization's picture can be more precise with more quantitative data. However, if you manually sift through gigabytes of data, you may not be able to see the forest for the trees, leading to incorrect assumptions and decisions. Machine Learning (ML), a subset of Artificial Intelligence (AI), comes to the rescue, helping you see the forest.
The power of ML is that it can identify patterns and trends using algorithms, revealing hidden insights that would take a human analyst a much longer time to discover, if at all. Additionally, ML can help predict future trends by looking at historical data. So, how does ML learn to read quantitative data and provide valuable insights? Let's look at how you teach ML.
There are many different learning methods for ML, but we will define three: supervised, unsupervised, and reinforcement learning.
- Supervised Learning uses labeled training data, which contains input-output pairs. The ML algorithms then analyze a large data set containing these pairs to learn the desired output when asked to make a prediction using new data.
- Unsupervised Learning uses unlabeled training data. The ML algorithms try to find patterns and structures in the input data without guidance on the outcomes they should predict.
- Reinforcement Learning is when an algorithm learns decision-making by performing an action and receiving reward or penalty feedback. The algorithm's overall goal is to discover a strategy that maximizes reward feedback.
While machine learning has become an indispensable tool for quantitative data analysis, it's important to recognize that it does not replace the need for human judgment and decision-making. Python, R, and specialized platforms like TensorFlow and Azure ML have made it easier than ever to integrate machine learning into the data analysis workflow, dramatically enhancing the efficiency and depth of insights that can be uncovered.
When leveraging these resources, analysts can uncover hidden patterns and transform raw data into actionable intelligence. However, rather than replacing human expertise, machine learning augments it by delivering more precise outcomes, freeing up analysts to concentrate on high-level strategic planning and choices that require a human touch. The power of machine learning lies in its ability to work in tandem with human judgment, providing the raw insights that inform critical decisions and drive meaningful change.
Example: Predictive Analytics in E-commerce
Imagine you're a data scientist working for an online retail organization that wants to improve customer retention and increase sales by predicting purchasing behavior and preferences. By leveraging predictive analytics, you can analyze historical transaction data to forecast future buying patterns and identify high-value customers.
- Data Collection and Preparation: You first collect and preprocess quantitative transactional data, including customer demographics, purchase history, purchase frequency, transaction amounts, customer lifetime value, product attributes, and website interactions. This process will also collect qualitative data such as product categories and customer feedback.
- Feature Engineering: Next, you extract meaningful features from the raw data through feature engineering, such as average purchase value or total number of orders, and you create derived variables such as recency and frequency. This information provides the inputs for predictive modeling.
- Predictive Modeling: To forecast customer churn, identify cross-selling opportunities, and create personalized product recommendations, you use ML algorithms to build predictive models. These models use historical data to predict the likelihood of customers’ purchasing in a specific timeframe or which products they will likely purchase next.
- Model Evaluation and Validation: You evaluate the performance of the predictive models using metrics such as accuracy, precision, recall, and area under the receiver operating characteristic curve (AUC-ROC). You then use cross-validation techniques and holdout validation to assess model generalization and ensure robustness against overfitting.
- Deployment and Integration: Once validated, the predictive models are deployed into production systems, integrated with the company's e-commerce platform, and used to generate real-time recommendations and personalized marketing campaigns. Customers receive targeted offers, product suggestions, and promotional discounts based on their predicted preferences and behaviors.
- Monitoring and Iteration: Continuous monitoring of model performance and customer feedback enables iterative refinement and optimization of the predictive analytics pipeline.
Quantitative data analysis is constantly evolving, driven by advancements in technology, new research methodologies, and emerging trends in data science. To remain ahead of the curve, it is crucial that you are aware of future trends that will impact how you perform data analysis. Some of the future trends that will potentially impact quantitative analysis include:
- Increased Use of Artificial Intelligence and Machine Learning : Integrating AI and ML techniques is expected to become more prevalent, enabling more sophisticated and automated data analysis.
- Growth of Big Data : As data grows in volume, variety, and velocity, quantitative analysis methods must adapt to handle larger datasets more efficiently.
- Advancements in Data Privacy Regulations : Increasing concerns and regulations around data privacy will impact how data is collected, stored, and analyzed, prompting the development of new methods that protect individual privacy.
- Rise of Edge Computing : With the rise of IoT devices, edge computing will become more critical. It will push data analysis closer to where data is generated to improve speed and reduce data transfer costs.
- Quantum Computing : The potential rise of quantum computing could revolutionize data analysis by providing the power to process enormous and complex datasets much faster than traditional computers.
- Proliferation of Data-as-a-Service (DaaS) : DaaS will provide increased access to high-quality and specialized data streams, enabling more refined and real-time analyses.
- Augmented Analytics : Augmented analytics, which incorporates natural language processing and automated algorithms, will make data analysis more accessible to non-experts and enhance decision-making processes.
- Focus on Predictive and Prescriptive Analytics : There will be a shift towards more predictive and prescriptive analytics, moving beyond descriptive analytics to offer foresight and guidance on future actions.
As quantitative data analysis evolves, it will be defined by a trifecta of innovation, integration, and integrity. Cutting-edge advancements will reshape analytical methods and tools, while the fusion of diverse data sources and techniques will unearth groundbreaking insights.
However, the true measure of success will lie in the ethical application of these capabilities, prioritizing transparency, privacy, and fairness. The future of data analysis will demand continuous learning, collaboration, and unwavering commitment to using data for the greater good, unlocking transformative opportunities for data-driven decision-making that benefits all.
Quantitative data is a powerful tool for uncovering insights, identifying patterns, and informing decision-making. We've explored the fundamental concepts of quantitative data, delved into the differences between quantitative and qualitative data, and navigated through a step-by-step guide to conducting data analysis using quantitative data. From defining research objectives to deploying advanced ML techniques, each step in the quantitative data analysis process helps you extract meaningful insights from numerical data.
The future of quantitative data analysis is bright, with advancements in technology, methodologies, and interdisciplinary collaboration driving innovation and progress. With the right tools, techniques, and mindset, the vast amount of quantitative data available to you becomes an opportunity to unlock hidden truths, drive informed decisions, and shape a better future through data-driven insights. The power of quantitative data analysis will propel you and your organization forward to greater success.
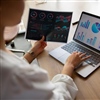
- Expand/Collapse Microsoft Office 38 RSS
- Expand/Collapse Training Trends 107 RSS
- Expand/Collapse CyberSecurity 59 RSS
- Expand/Collapse DevOps 2 RSS
- Expand/Collapse Modern Workplace 41 RSS
- Expand/Collapse Cloud 16 RSS
- Expand/Collapse Programming 10 RSS
- Expand/Collapse Artificial Intelligence (AI) 8 RSS
- Expand/Collapse ITIL 17 RSS
- Expand/Collapse Data & Analytics 26 RSS
- Expand/Collapse Business Analyst 13 RSS
- Expand/Collapse Training By Job Role 1 RSS
- Expand/Collapse Leadership and Professional Development 14 RSS
- Expand/Collapse Managed Learning Services 3 RSS
- Expand/Collapse Design & Multi-Media 1 RSS
- USC Libraries
- Research Guides
Organizing Your Social Sciences Research Paper
Glossary of research terms.
- Purpose of Guide
- Design Flaws to Avoid
- Independent and Dependent Variables
- Reading Research Effectively
- Narrowing a Topic Idea
- Broadening a Topic Idea
- Extending the Timeliness of a Topic Idea
- Academic Writing Style
- Applying Critical Thinking
- Choosing a Title
- Making an Outline
- Paragraph Development
- Research Process Video Series
- Executive Summary
- The C.A.R.S. Model
- Background Information
- The Research Problem/Question
- Theoretical Framework
- Citation Tracking
- Content Alert Services
- Evaluating Sources
- Primary Sources
- Secondary Sources
- Tiertiary Sources
- Scholarly vs. Popular Publications
- Qualitative Methods
- Quantitative Methods
- Insiderness
- Using Non-Textual Elements
- Limitations of the Study
- Common Grammar Mistakes
- Writing Concisely
- Avoiding Plagiarism
- Footnotes or Endnotes?
- Further Readings
- Generative AI and Writing
- USC Libraries Tutorials and Other Guides
- Bibliography
This glossary is intended to assist you in understanding commonly used terms and concepts when reading, interpreting, and evaluating scholarly research. Also included are common words and phrases defined within the context of how they apply to research in the social and behavioral sciences.
- Acculturation -- refers to the process of adapting to another culture, particularly in reference to blending in with the majority population [e.g., an immigrant adopting American customs]. However, acculturation also implies that both cultures add something to one another, but still remain distinct groups unto themselves.
- Accuracy -- a term used in survey research to refer to the match between the target population and the sample.
- Affective Measures -- procedures or devices used to obtain quantified descriptions of an individual's feelings, emotional states, or dispositions.
- Aggregate -- a total created from smaller units. For instance, the population of a county is an aggregate of the populations of the cities, rural areas, etc. that comprise the county. As a verb, it refers to total data from smaller units into a large unit.
- Anonymity -- a research condition in which no one, including the researcher, knows the identities of research participants.
- Baseline -- a control measurement carried out before an experimental treatment.
- Behaviorism -- school of psychological thought concerned with the observable, tangible, objective facts of behavior, rather than with subjective phenomena such as thoughts, emotions, or impulses. Contemporary behaviorism also emphasizes the study of mental states such as feelings and fantasies to the extent that they can be directly observed and measured.
- Beliefs -- ideas, doctrines, tenets, etc. that are accepted as true on grounds which are not immediately susceptible to rigorous proof.
- Benchmarking -- systematically measuring and comparing the operations and outcomes of organizations, systems, processes, etc., against agreed upon "best-in-class" frames of reference.
- Bias -- a loss of balance and accuracy in the use of research methods. It can appear in research via the sampling frame, random sampling, or non-response. It can also occur at other stages in research, such as while interviewing, in the design of questions, or in the way data are analyzed and presented. Bias means that the research findings will not be representative of, or generalizable to, a wider population.
- Case Study -- the collection and presentation of detailed information about a particular participant or small group, frequently including data derived from the subjects themselves.
- Causal Hypothesis -- a statement hypothesizing that the independent variable affects the dependent variable in some way.
- Causal Relationship -- the relationship established that shows that an independent variable, and nothing else, causes a change in a dependent variable. It also establishes how much of a change is shown in the dependent variable.
- Causality -- the relation between cause and effect.
- Central Tendency -- any way of describing or characterizing typical, average, or common values in some distribution.
- Chi-square Analysis -- a common non-parametric statistical test which compares an expected proportion or ratio to an actual proportion or ratio.
- Claim -- a statement, similar to a hypothesis, which is made in response to the research question and that is affirmed with evidence based on research.
- Classification -- ordering of related phenomena into categories, groups, or systems according to characteristics or attributes.
- Cluster Analysis -- a method of statistical analysis where data that share a common trait are grouped together. The data is collected in a way that allows the data collector to group data according to certain characteristics.
- Cohort Analysis -- group by group analytic treatment of individuals having a statistical factor in common to each group. Group members share a particular characteristic [e.g., born in a given year] or a common experience [e.g., entering a college at a given time].
- Confidentiality -- a research condition in which no one except the researcher(s) knows the identities of the participants in a study. It refers to the treatment of information that a participant has disclosed to the researcher in a relationship of trust and with the expectation that it will not be revealed to others in ways that violate the original consent agreement, unless permission is granted by the participant.
- Confirmability Objectivity -- the findings of the study could be confirmed by another person conducting the same study.
- Construct -- refers to any of the following: something that exists theoretically but is not directly observable; a concept developed [constructed] for describing relations among phenomena or for other research purposes; or, a theoretical definition in which concepts are defined in terms of other concepts. For example, intelligence cannot be directly observed or measured; it is a construct.
- Construct Validity -- seeks an agreement between a theoretical concept and a specific measuring device, such as observation.
- Constructivism -- the idea that reality is socially constructed. It is the view that reality cannot be understood outside of the way humans interact and that the idea that knowledge is constructed, not discovered. Constructivists believe that learning is more active and self-directed than either behaviorism or cognitive theory would postulate.
- Content Analysis -- the systematic, objective, and quantitative description of the manifest or latent content of print or nonprint communications.
- Context Sensitivity -- awareness by a qualitative researcher of factors such as values and beliefs that influence cultural behaviors.
- Control Group -- the group in an experimental design that receives either no treatment or a different treatment from the experimental group. This group can thus be compared to the experimental group.
- Controlled Experiment -- an experimental design with two or more randomly selected groups [an experimental group and control group] in which the researcher controls or introduces the independent variable and measures the dependent variable at least two times [pre- and post-test measurements].
- Correlation -- a common statistical analysis, usually abbreviated as r, that measures the degree of relationship between pairs of interval variables in a sample. The range of correlation is from -1.00 to zero to +1.00. Also, a non-cause and effect relationship between two variables.
- Covariate -- a product of the correlation of two related variables times their standard deviations. Used in true experiments to measure the difference of treatment between them.
- Credibility -- a researcher's ability to demonstrate that the object of a study is accurately identified and described based on the way in which the study was conducted.
- Critical Theory -- an evaluative approach to social science research, associated with Germany's neo-Marxist “Frankfurt School,” that aims to criticize as well as analyze society, opposing the political orthodoxy of modern communism. Its goal is to promote human emancipatory forces and to expose ideas and systems that impede them.
- Data -- factual information [as measurements or statistics] used as a basis for reasoning, discussion, or calculation.
- Data Mining -- the process of analyzing data from different perspectives and summarizing it into useful information, often to discover patterns and/or systematic relationships among variables.
- Data Quality -- this is the degree to which the collected data [results of measurement or observation] meet the standards of quality to be considered valid [trustworthy] and reliable [dependable].
- Deductive -- a form of reasoning in which conclusions are formulated about particulars from general or universal premises.
- Dependability -- being able to account for changes in the design of the study and the changing conditions surrounding what was studied.
- Dependent Variable -- a variable that varies due, at least in part, to the impact of the independent variable. In other words, its value “depends” on the value of the independent variable. For example, in the variables “gender” and “academic major,” academic major is the dependent variable, meaning that your major cannot determine whether you are male or female, but your gender might indirectly lead you to favor one major over another.
- Deviation -- the distance between the mean and a particular data point in a given distribution.
- Discourse Community -- a community of scholars and researchers in a given field who respond to and communicate to each other through published articles in the community's journals and presentations at conventions. All members of the discourse community adhere to certain conventions for the presentation of their theories and research.
- Discrete Variable -- a variable that is measured solely in whole units, such as, gender and number of siblings.
- Distribution -- the range of values of a particular variable.
- Effect Size -- the amount of change in a dependent variable that can be attributed to manipulations of the independent variable. A large effect size exists when the value of the dependent variable is strongly influenced by the independent variable. It is the mean difference on a variable between experimental and control groups divided by the standard deviation on that variable of the pooled groups or of the control group alone.
- Emancipatory Research -- research is conducted on and with people from marginalized groups or communities. It is led by a researcher or research team who is either an indigenous or external insider; is interpreted within intellectual frameworks of that group; and, is conducted largely for the purpose of empowering members of that community and improving services for them. It also engages members of the community as co-constructors or validators of knowledge.
- Empirical Research -- the process of developing systematized knowledge gained from observations that are formulated to support insights and generalizations about the phenomena being researched.
- Epistemology -- concerns knowledge construction; asks what constitutes knowledge and how knowledge is validated.
- Ethnography -- method to study groups and/or cultures over a period of time. The goal of this type of research is to comprehend the particular group/culture through immersion into the culture or group. Research is completed through various methods but, since the researcher is immersed within the group for an extended period of time, more detailed information is usually collected during the research.
- Expectancy Effect -- any unconscious or conscious cues that convey to the participant in a study how the researcher wants them to respond. Expecting someone to behave in a particular way has been shown to promote the expected behavior. Expectancy effects can be minimized by using standardized interactions with subjects, automated data-gathering methods, and double blind protocols.
- External Validity -- the extent to which the results of a study are generalizable or transferable.
- Factor Analysis -- a statistical test that explores relationships among data. The test explores which variables in a data set are most related to each other. In a carefully constructed survey, for example, factor analysis can yield information on patterns of responses, not simply data on a single response. Larger tendencies may then be interpreted, indicating behavior trends rather than simply responses to specific questions.
- Field Studies -- academic or other investigative studies undertaken in a natural setting, rather than in laboratories, classrooms, or other structured environments.
- Focus Groups -- small, roundtable discussion groups charged with examining specific topics or problems, including possible options or solutions. Focus groups usually consist of 4-12 participants, guided by moderators to keep the discussion flowing and to collect and report the results.
- Framework -- the structure and support that may be used as both the launching point and the on-going guidelines for investigating a research problem.
- Generalizability -- the extent to which research findings and conclusions conducted on a specific study to groups or situations can be applied to the population at large.
- Grey Literature -- research produced by organizations outside of commercial and academic publishing that publish materials, such as, working papers, research reports, and briefing papers.
- Grounded Theory -- practice of developing other theories that emerge from observing a group. Theories are grounded in the group's observable experiences, but researchers add their own insight into why those experiences exist.
- Group Behavior -- behaviors of a group as a whole, as well as the behavior of an individual as influenced by his or her membership in a group.
- Hypothesis -- a tentative explanation based on theory to predict a causal relationship between variables.
- Independent Variable -- the conditions of an experiment that are systematically manipulated by the researcher. A variable that is not impacted by the dependent variable, and that itself impacts the dependent variable. In the earlier example of "gender" and "academic major," (see Dependent Variable) gender is the independent variable.
- Individualism -- a theory or policy having primary regard for the liberty, rights, or independent actions of individuals.
- Inductive -- a form of reasoning in which a generalized conclusion is formulated from particular instances.
- Inductive Analysis -- a form of analysis based on inductive reasoning; a researcher using inductive analysis starts with answers, but formulates questions throughout the research process.
- Insiderness -- a concept in qualitative research that refers to the degree to which a researcher has access to and an understanding of persons, places, or things within a group or community based on being a member of that group or community.
- Internal Consistency -- the extent to which all questions or items assess the same characteristic, skill, or quality.
- Internal Validity -- the rigor with which the study was conducted [e.g., the study's design, the care taken to conduct measurements, and decisions concerning what was and was not measured]. It is also the extent to which the designers of a study have taken into account alternative explanations for any causal relationships they explore. In studies that do not explore causal relationships, only the first of these definitions should be considered when assessing internal validity.
- Life History -- a record of an event/events in a respondent's life told [written down, but increasingly audio or video recorded] by the respondent from his/her own perspective in his/her own words. A life history is different from a "research story" in that it covers a longer time span, perhaps a complete life, or a significant period in a life.
- Margin of Error -- the permittable or acceptable deviation from the target or a specific value. The allowance for slight error or miscalculation or changing circumstances in a study.
- Measurement -- process of obtaining a numerical description of the extent to which persons, organizations, or things possess specified characteristics.
- Meta-Analysis -- an analysis combining the results of several studies that address a set of related hypotheses.
- Methodology -- a theory or analysis of how research does and should proceed.
- Methods -- systematic approaches to the conduct of an operation or process. It includes steps of procedure, application of techniques, systems of reasoning or analysis, and the modes of inquiry employed by a discipline.
- Mixed-Methods -- a research approach that uses two or more methods from both the quantitative and qualitative research categories. It is also referred to as blended methods, combined methods, or methodological triangulation.
- Modeling -- the creation of a physical or computer analogy to understand a particular phenomenon. Modeling helps in estimating the relative magnitude of various factors involved in a phenomenon. A successful model can be shown to account for unexpected behavior that has been observed, to predict certain behaviors, which can then be tested experimentally, and to demonstrate that a given theory cannot account for certain phenomenon.
- Models -- representations of objects, principles, processes, or ideas often used for imitation or emulation.
- Naturalistic Observation -- observation of behaviors and events in natural settings without experimental manipulation or other forms of interference.
- Norm -- the norm in statistics is the average or usual performance. For example, students usually complete their high school graduation requirements when they are 18 years old. Even though some students graduate when they are younger or older, the norm is that any given student will graduate when he or she is 18 years old.
- Null Hypothesis -- the proposition, to be tested statistically, that the experimental intervention has "no effect," meaning that the treatment and control groups will not differ as a result of the intervention. Investigators usually hope that the data will demonstrate some effect from the intervention, thus allowing the investigator to reject the null hypothesis.
- Ontology -- a discipline of philosophy that explores the science of what is, the kinds and structures of objects, properties, events, processes, and relations in every area of reality.
- Panel Study -- a longitudinal study in which a group of individuals is interviewed at intervals over a period of time.
- Participant -- individuals whose physiological and/or behavioral characteristics and responses are the object of study in a research project.
- Peer-Review -- the process in which the author of a book, article, or other type of publication submits his or her work to experts in the field for critical evaluation, usually prior to publication. This is standard procedure in publishing scholarly research.
- Phenomenology -- a qualitative research approach concerned with understanding certain group behaviors from that group's point of view.
- Philosophy -- critical examination of the grounds for fundamental beliefs and analysis of the basic concepts, doctrines, or practices that express such beliefs.
- Phonology -- the study of the ways in which speech sounds form systems and patterns in language.
- Policy -- governing principles that serve as guidelines or rules for decision making and action in a given area.
- Policy Analysis -- systematic study of the nature, rationale, cost, impact, effectiveness, implications, etc., of existing or alternative policies, using the theories and methodologies of relevant social science disciplines.
- Population -- the target group under investigation. The population is the entire set under consideration. Samples are drawn from populations.
- Position Papers -- statements of official or organizational viewpoints, often recommending a particular course of action or response to a situation.
- Positivism -- a doctrine in the philosophy of science, positivism argues that science can only deal with observable entities known directly to experience. The positivist aims to construct general laws, or theories, which express relationships between phenomena. Observation and experiment is used to show whether the phenomena fit the theory.
- Predictive Measurement -- use of tests, inventories, or other measures to determine or estimate future events, conditions, outcomes, or trends.
- Principal Investigator -- the scientist or scholar with primary responsibility for the design and conduct of a research project.
- Probability -- the chance that a phenomenon will occur randomly. As a statistical measure, it is shown as p [the "p" factor].
- Questionnaire -- structured sets of questions on specified subjects that are used to gather information, attitudes, or opinions.
- Random Sampling -- a process used in research to draw a sample of a population strictly by chance, yielding no discernible pattern beyond chance. Random sampling can be accomplished by first numbering the population, then selecting the sample according to a table of random numbers or using a random-number computer generator. The sample is said to be random because there is no regular or discernible pattern or order. Random sample selection is used under the assumption that sufficiently large samples assigned randomly will exhibit a distribution comparable to that of the population from which the sample is drawn. The random assignment of participants increases the probability that differences observed between participant groups are the result of the experimental intervention.
- Reliability -- the degree to which a measure yields consistent results. If the measuring instrument [e.g., survey] is reliable, then administering it to similar groups would yield similar results. Reliability is a prerequisite for validity. An unreliable indicator cannot produce trustworthy results.
- Representative Sample -- sample in which the participants closely match the characteristics of the population, and thus, all segments of the population are represented in the sample. A representative sample allows results to be generalized from the sample to the population.
- Rigor -- degree to which research methods are scrupulously and meticulously carried out in order to recognize important influences occurring in an experimental study.
- Sample -- the population researched in a particular study. Usually, attempts are made to select a "sample population" that is considered representative of groups of people to whom results will be generalized or transferred. In studies that use inferential statistics to analyze results or which are designed to be generalizable, sample size is critical, generally the larger the number in the sample, the higher the likelihood of a representative distribution of the population.
- Sampling Error -- the degree to which the results from the sample deviate from those that would be obtained from the entire population, because of random error in the selection of respondent and the corresponding reduction in reliability.
- Saturation -- a situation in which data analysis begins to reveal repetition and redundancy and when new data tend to confirm existing findings rather than expand upon them.
- Semantics -- the relationship between symbols and meaning in a linguistic system. Also, the cuing system that connects what is written in the text to what is stored in the reader's prior knowledge.
- Social Theories -- theories about the structure, organization, and functioning of human societies.
- Sociolinguistics -- the study of language in society and, more specifically, the study of language varieties, their functions, and their speakers.
- Standard Deviation -- a measure of variation that indicates the typical distance between the scores of a distribution and the mean; it is determined by taking the square root of the average of the squared deviations in a given distribution. It can be used to indicate the proportion of data within certain ranges of scale values when the distribution conforms closely to the normal curve.
- Statistical Analysis -- application of statistical processes and theory to the compilation, presentation, discussion, and interpretation of numerical data.
- Statistical Bias -- characteristics of an experimental or sampling design, or the mathematical treatment of data, that systematically affects the results of a study so as to produce incorrect, unjustified, or inappropriate inferences or conclusions.
- Statistical Significance -- the probability that the difference between the outcomes of the control and experimental group are great enough that it is unlikely due solely to chance. The probability that the null hypothesis can be rejected at a predetermined significance level [0.05 or 0.01].
- Statistical Tests -- researchers use statistical tests to make quantitative decisions about whether a study's data indicate a significant effect from the intervention and allow the researcher to reject the null hypothesis. That is, statistical tests show whether the differences between the outcomes of the control and experimental groups are great enough to be statistically significant. If differences are found to be statistically significant, it means that the probability [likelihood] that these differences occurred solely due to chance is relatively low. Most researchers agree that a significance value of .05 or less [i.e., there is a 95% probability that the differences are real] sufficiently determines significance.
- Subcultures -- ethnic, regional, economic, or social groups exhibiting characteristic patterns of behavior sufficient to distinguish them from the larger society to which they belong.
- Testing -- the act of gathering and processing information about individuals' ability, skill, understanding, or knowledge under controlled conditions.
- Theory -- a general explanation about a specific behavior or set of events that is based on known principles and serves to organize related events in a meaningful way. A theory is not as specific as a hypothesis.
- Treatment -- the stimulus given to a dependent variable.
- Trend Samples -- method of sampling different groups of people at different points in time from the same population.
- Triangulation -- a multi-method or pluralistic approach, using different methods in order to focus on the research topic from different viewpoints and to produce a multi-faceted set of data. Also used to check the validity of findings from any one method.
- Unit of Analysis -- the basic observable entity or phenomenon being analyzed by a study and for which data are collected in the form of variables.
- Validity -- the degree to which a study accurately reflects or assesses the specific concept that the researcher is attempting to measure. A method can be reliable, consistently measuring the same thing, but not valid.
- Variable -- any characteristic or trait that can vary from one person to another [race, gender, academic major] or for one person over time [age, political beliefs].
- Weighted Scores -- scores in which the components are modified by different multipliers to reflect their relative importance.
- White Paper -- an authoritative report that often states the position or philosophy about a social, political, or other subject, or a general explanation of an architecture, framework, or product technology written by a group of researchers. A white paper seeks to contain unbiased information and analysis regarding a business or policy problem that the researchers may be facing.
Elliot, Mark, Fairweather, Ian, Olsen, Wendy Kay, and Pampaka, Maria. A Dictionary of Social Research Methods. Oxford, UK: Oxford University Press, 2016; Free Social Science Dictionary. Socialsciencedictionary.com [2008]. Glossary. Institutional Review Board. Colorado College; Glossary of Key Terms. Writing@CSU. Colorado State University; Glossary A-Z. Education.com; Glossary of Research Terms. Research Mindedness Virtual Learning Resource. Centre for Human Servive Technology. University of Southampton; Miller, Robert L. and Brewer, John D. The A-Z of Social Research: A Dictionary of Key Social Science Research Concepts London: SAGE, 2003; Jupp, Victor. The SAGE Dictionary of Social and Cultural Research Methods . London: Sage, 2006.
- << Previous: Independent and Dependent Variables
- Next: 1. Choosing a Research Problem >>
- Last Updated: May 30, 2024 9:38 AM
- URL: https://libguides.usc.edu/writingguide
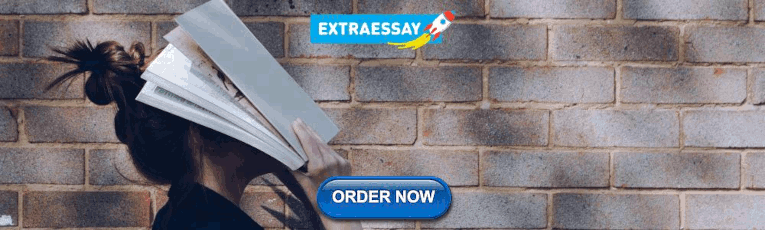
IMAGES
VIDEO
COMMENTS
Null Hypothesis H 0: The correlation in the population is zero: ρ = 0. Alternative Hypothesis H A: The correlation in the population is not zero: ρ ≠ 0. For all these cases, the analysts define the hypotheses before the study. After collecting the data, they perform a hypothesis test to determine whether they can reject the null hypothesis.
When the research question asks "Does the independent variable affect the dependent variable?": The null hypothesis ( H0) answers "No, there's no effect in the population.". The alternative hypothesis ( Ha) answers "Yes, there is an effect in the population.". The null and alternative are always claims about the population.
Basic definitions. The null hypothesis and the alternative hypothesis are types of conjectures used in statistical tests to make statistical inferences, which are formal methods of reaching conclusions and separating scientific claims from statistical noise.. The statement being tested in a test of statistical significance is called the null hypothesis. . The test of significance is designed ...
Null Hypothesis Examples. "Hyperactivity is unrelated to eating sugar " is an example of a null hypothesis. If the hypothesis is tested and found to be false, using statistics, then a connection between hyperactivity and sugar ingestion may be indicated. A significance test is the most common statistical test used to establish confidence in a ...
A crucial step in null hypothesis testing is finding the likelihood of the sample result if the null hypothesis were true. This probability is called the p value. A low p value means that the sample result would be unlikely if the null hypothesis were true and leads to the rejection of the null hypothesis. A high p value means that the sample ...
The null and alternative hypotheses offer competing answers to your research question. When the research question asks "Does the independent variable affect the dependent variable?", the null hypothesis (H 0) answers "No, there's no effect in the population.". On the other hand, the alternative hypothesis (H A) answers "Yes, there ...
6. Write a null hypothesis. If your research involves statistical hypothesis testing, you will also have to write a null hypothesis. The null hypothesis is the default position that there is no association between the variables. The null hypothesis is written as H 0, while the alternative hypothesis is H 1 or H a.
Simple hypothesis. A simple hypothesis is a statement made to reflect the relation between exactly two variables. One independent and one dependent. Consider the example, "Smoking is a prominent cause of lung cancer." The dependent variable, lung cancer, is dependent on the independent variable, smoking. 4.
Definition. In formal hypothesis testing, the null hypothesis ( H0) is the hypothesis assumed to be true in the population and which gives rise to the sampling distribution of the test statistic in question (Hays 1994 ). The critical feature of the null hypothesis across hypothesis testing frameworks is that it is stated with enough precision ...
There are 5 main steps in hypothesis testing: State your research hypothesis as a null hypothesis and alternate hypothesis (H o) and (H a or H 1). Collect data in a way designed to test the hypothesis. Perform an appropriate statistical test. Decide whether to reject or fail to reject your null hypothesis.
It seeks to explore and understand a particular aspect of the research subject. In contrast, a research hypothesis is a specific statement or prediction that suggests an expected relationship between variables. It is formulated based on existing knowledge or theories and guides the research design and data analysis. 7.
Definition: Hypothesis is an educated guess or proposed explanation for a phenomenon, based on some initial observations or data. It is a tentative statement that can be tested and potentially proven or disproven through further investigation and experimentation. Hypothesis is often used in scientific research to guide the design of experiments ...
A research hypothesis, in its plural form "hypotheses," is a specific, testable prediction about the anticipated results of a study, established at its outset. It is a key component of the scientific method. Hypotheses connect theory to data and guide the research process towards expanding scientific understanding.
The Research Hypothesis. A research hypothesis is a mathematical way of stating a research question. A research hypothesis names the groups (we'll start with a sample and a population), what was measured, and which we think will have a higher mean. The last one gives the research hypothesis a direction. In other words, a research hypothesis ...
A research hypothesis (also called a scientific hypothesis) is a statement about the expected outcome of a study (for example, a dissertation or thesis). To constitute a quality hypothesis, the statement needs to have three attributes - specificity, clarity and testability. Let's take a look at these more closely.
Abstract: "null hypothesis significance testing is the statistical method of choice in biological, biomedical and social sciences to investigate if an effect is likely". No, NHST is the method to test the hypothesis of no effect. I agree - yet people use it to investigate (not test) if an effect is likely.
INTRODUCTION. Scientific research is usually initiated by posing evidenced-based research questions which are then explicitly restated as hypotheses.1,2 The hypotheses provide directions to guide the study, solutions, explanations, and expected results.3,4 Both research questions and hypotheses are essentially formulated based on conventional theories and real-world processes, which allow the ...
Null Hypothesis Definition. ... In this method, the sampling distribution is the function of the sample size. ... If this hypothesis is rejected means, that research could be invalid. Many researchers will neglect this hypothesis as it is merely opposite to the alternate hypothesis. It is a better practice to create a hypothesis and test it.
The Logic of Null Hypothesis Testing. Null hypothesis testing (often called null hypothesis significance testing or NHST) is a formal approach to deciding between two interpretations of a statistical relationship in a sample. One interpretation is called the null hypothesis (often symbolized H0 and read as "H-zero").
The null hypothesis states that P (xi > yi) = P (xi < yi) =1/2 while the alternative hypothesis states that P (xi > yi) ≠1/2. Kolmogorov-Smirnov test. The two-sample Kolmogorov-Smirnov (KS) test was designed as a generic method to test whether two random samples are drawn from the same distribution.
The test is a non-parametric method used to determine if there are statistically significant differences between the medians of three or more independent groups of the independent variable. ... (df.head()) # Define X (independent variables) and y (dependent variable) X = df[['Study ... where the null hypothesis on the intercept and the ...
A crucial step in null hypothesis testing is finding the likelihood of the sample result if the null hypothesis were true. This probability is called the p value. A low p value means that the sample result would be unlikely if the null hypothesis were true and leads to the rejection of the null hypothesis. A high p value means that the sample ...
The difference between Research Hypothesis Vs Null Hypothesis is as follows: Research Hypothesis. A Research Hypothesis is a tentative statement that proposes a relationship between two or more variables. It is based on a theoretical or conceptual framework and is typically tested through empirical research. Null Hypothesis
Null Hypothesis Significance Testing (NHST) is the most familiar statistical procedure for making inferences about population effects. ... Generalizability is a topic that is often debated in qualitative research methodology. Many researchers do not consider generalizability the purpose of qualitative research, but rather finding in-depth ...
Define research objectives. Articulate goals and objectives, specify research questions, hypotheses to test ... Formulate null and alternative hypotheses, select appropriate statistical tests, conduct hypothesis testing and interpret results. 5. Regression Analysis ... There are many different learning methods for ML, but we will define three ...
The null hypothesis distribution curve below shows the probabilities of obtaining all possible results if the study were repeated with new samples and the null hypothesis were true in the population. ... methodology, or research bias, make sure to check out some of our other articles with explanations and examples. ... Definition and Examples
The probability that the null hypothesis can be rejected at a predetermined significance level [0.05 or 0.01]. Statistical Tests-- researchers use statistical tests to make quantitative decisions about whether a study's data indicate a significant effect from the intervention and allow the researcher to reject the null hypothesis. That is ...
Statistical significance. In statistical hypothesis testing, [1] [2] a result has statistical significance when a result at least as "extreme" would be very infrequent if the null hypothesis were true. [3] More precisely, a study's defined significance level, denoted by , is the probability of the study rejecting the null hypothesis, given that ...