- Reference Manager
- Simple TEXT file
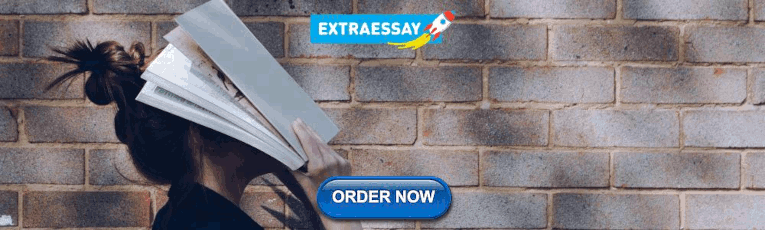
People also looked at
Systematic review article, a systematic review of the effectiveness of online learning in higher education during the covid-19 pandemic period.
- 1 Department of Basic Education, Beihai Campus, Guilin University of Electronic Technology Beihai, Beihai, Guangxi, China
- 2 School of Sports and Arts, Harbin Sport University, Harbin, Heilongjiang, China
- 3 School of Music, Harbin Normal University, Harbin, Heilongjiang, China
- 4 School of General Education, Beihai Vocational College, Beihai, Guangxi, China
- 5 School of Economics and Management, Beihai Campus, Guilin University of Electronic Technology, Guilin, Guangxi, China
Background: The effectiveness of online learning in higher education during the COVID-19 pandemic period is a debated topic but a systematic review on this topic is absent.
Methods: The present study implemented a systematic review of 25 selected articles to comprehensively evaluate online learning effectiveness during the pandemic period and identify factors that influence such effectiveness.
Results: It was concluded that past studies failed to achieve a consensus over online learning effectiveness and research results are largely by how learning effectiveness was assessed, e.g., self-reported online learning effectiveness, longitudinal comparison, and RCT. Meanwhile, a set of factors that positively or negatively influence the effectiveness of online learning were identified, including infrastructure factors, instructional factors, the lack of social interaction, negative emotions, flexibility, and convenience.
Discussion: Although it is debated over the effectiveness of online learning during the pandemic period, it is generally believed that the pandemic brings a lot of challenges and difficulties to higher education and these challenges and difficulties are more prominent in developing countries. In addition, this review critically assesses limitations in past research, develops pedagogical implications, and proposes recommendations for future research.
1 Introduction
1.1 research background.
The COVID-19 pandemic first out broken in early 2020 has considerably shaped the higher education landscape globally. To restrain viral transmission, universities globally locked down, and teaching and learning activities were transferred to online platforms. Although online learning is a relatively mature learning model and is increasingly integrated into higher education, the sudden and unprepared transition to wholly online learning caused by the pandemic posed formidable challenges to higher education stakeholders, e.g., policymakers, instructors, and students, especially at the early stage of the pandemic ( García-Morales et al., 2021 ; Grafton-Clarke et al., 2022 ). Correspondingly, the effectiveness of online learning during the pandemic period is still questionable as online learning during this period has some unique characteristics, e.g., the lack of preparation, sudden and unprepared transition, the huge scale of implementation, and social distancing policies ( Sharma et al., 2020 ; Rahman, 2021 ; Tsang et al., 2021 ; Hollister et al., 2022 ; Zhang and Chen, 2023 ). This question is more prominent in developing or undeveloped countries because of insufficient Internet access, network problems, the lack of electronic devices, and poor network infrastructure ( Adnan and Anwar, 2020 ; Muthuprasad et al., 2021 ; Rahman, 2021 ; Chandrasiri and Weerakoon, 2022 ).
Learning effectiveness is a key consideration of education as it reflects the extent to which learning and teaching objectives are achieved and learners’ needs are satisfied ( Joy and Garcia, 2000 ; Swan, 2003 ). Online learning was generally proven to be effective within a higher education context ( Kebritchi et al., 2017 ) prior to the pandemic. ICTs have fundamentally shaped the process of learning as they allow learners to learn anywhere and anytime, interact with others efficiently and conveniently, and freely acquire a large volume of learning materials online ( Kebritchi et al., 2017 ; Choudhury and Pattnaik, 2020 ). Such benefits may be offset by the challenges brought about by the pandemic. A lot of empirical studies globally have investigated the effectiveness of online learning but there is currently a scarcity of a systematic review of these studies to comprehensively evaluate online learning effectiveness and identify factors that influence effectiveness.
At present, although the vast majority of countries have implemented opening policies to deal with the pandemic and higher education institutes have recovered offline teaching and learning, assessing the effectiveness of online learning during the pandemic period via a systematic review is still essential. First, it is necessary to summarize, learn from, and reflect on the lessons and experiences of online learning practices during the pandemic period to offer implications for future practices and research. Second, the review of online learning research carried out during the pandemic period is likely to generate interesting knowledge because of the unique research context. Third, higher education institutes still need a contingency plan for emergency online learning to deal with potential crises in the future, e.g., wars, pandemics, and natural disasters. A systematic review of research on the effectiveness of online learning during the pandemic period offers valuable knowledge for designing a contingency plan for the future.
1.2 Related concepts
1.2.1 online learning.
Online learning should not be simply understood as learning on the Internet or the integration of ICTs with learning because it is a systematic framework consisting of a set of pedagogies, technologies, implementations, and processes ( Kebritchi et al., 2017 ; Choudhury and Pattnaik, 2020). Choudhury and Pattnaik (2020; p.2) summarized prior definitions of online learning and provided a comprehensive and up-to-date definition, i.e., online learning refers to “ the transfer of knowledge and skills, in a well-designed course content that has established accreditations, through an electronic media like the Internet, Web 4.0, intranets and extranets .” Online learning differs from traditional learning because of not only technological differences, but also differences in social development and pedagogies ( Camargo et al., 2020 ). Online learning has also considerably shaped the patterns by which knowledge is stored, shared, and transferred, skills are practiced, as well as the way by which stakeholders (e.g., teachers and teachers) interact ( Desai et al., 2008 ; Anderson and Hajhashemi, 2013 ). In addition, online learning has altered educational objectives and learning requirements. Memorizing knowledge was traditionally viewed as vital to learning but it is now less important since required knowledge can be conveniently searched and acquired on the Internet while the reflection and application of knowledge becomes more important ( Gamage et al., 2023 ). Online learning also entails learners’ self-regulated learning ability more than traditional learning because the online learning environment inflicts less external regulation and provides more autonomy and flexibility ( Barnard-Brak et al., 2010 ; Wong et al., 2019 ). The above differences imply that traditional pedagogies may not apply to online learning.
There are a variety of online learning models according to the differences in learning methods, processes, outcomes, and the application of technologies ( Zeitoun, 2008 ). As ICTs can be used as either the foundation of learning or auxiliary means, online learning can be classified into assistant, blended, and wholly online models. Here, assistant online learning refers to the scenario where online learning technologies are used to supplement and support traditional learning; blended online learning refers to the integration/ mixture of online and offline methods, and; wholly online learning refers to the exclusive use of the Internet for learning ( Arkorful and Abaidoo, 2015 ). The present review focuses on wholly online learning because the review is interested in the COVID-19 pandemic context where learning activities are fully switched to online platforms.
1.2.2 Learning effectiveness
Learning effectiveness can be broadly defined as the extent to which learning and teaching objectives have been effectively and efficiently achieved via educational activities ( Swan, 2003 ) or the extent to which learners’ needs are satisfied by learning activities ( Joy and Garcia, 2000 ). It is a multi-dimensional construct because learning objectives and needs are always diversified ( Joy and Garcia, 2000 ; Swan, 2003 ). Assessing learning effectiveness is a key challenge in educational research and researchers generally use a set of subjective and objective indicators to assess learning effectiveness, e.g., examination scores, assignment performance, perceived effectiveness, student satisfaction, learning motivation, engagement in learning, and learning experience ( Rajaram and Collins, 2013 ; Noesgaard and Ørngreen, 2015 ). Prior research related to the effectiveness of online learning was diversified in terms of learning outcomes, e.g., satisfaction, perceived effectiveness, motivation, and learning engagement, and there is no consensus over which outcomes are valid indicators of learning effectiveness. The present study adopts a broad definition of learning effectiveness and considers various learning outcomes that are closely associated with learning objectives and needs.
1.3 Previous review research
Up to now, online learning during the COVID-19 pandemic period has attracted considerable attention from academia and there is a lot of related review research. Some review research analyzed the trends and major topics in related research. Pratama et al. (2020) tracked the trend of using online meeting applications in online learning during the pandemic period based on a systematic review of 12 articles. It was reported that the use of these applications kept a rising trend and this use helps promote learning and teaching processes. However, this review was descriptive and failed to identify problems related to these applications as well as the limitations of these applications. Zhang et al. (2022) implemented a bibliometric review to provide a holistic view of research on online learning in higher education during the COVID-19 pandemic period. They concluded that the majority of research focused on identifying the use of strategies and technologies, psychological impacts brought by the pandemic, and student perceptions. Meanwhile, collaborative learning, hands-on learning, discovery learning, and inquiry-based learning were the most frequently discussed instructional approaches. In addition, chemical and medical education were found to be the most investigated disciplines. This review hence offered a relatively comprehensive landscape of related research in the field. However, since it was a bibliometric review, it merely analyzed the superficial characteristics of past articles in the field without a detailed analysis of their research contributions. Bughrara et al. (2023) categorized the major research topics in the field of online medical education during the pandemic period via a scoping review. A total of 174 articles were included in the review and it was found there were seven major topics, including students’ mental health, stigma, student vaccination, use of telehealth, students’ physical health, online modifications and educational adaptations, and students’ attitudes and knowledge. Overall, the review comprehensively reveals major topics in the focused field.
Some scholars believed that online learning during the pandemic period has brought about a lot of problems while both students and teachers encounter many challenges. García-Morales et al. (2021) implemented a systematic review to identify the challenges encountered by higher education in an online learning scenario during the pandemic period. A total of seven studies were included and it was found that higher education suddenly transferred to online learning and a lot of technologies and platforms were used to support online learning. However, this transition was hasty and forced by the extreme situation. Thus, various stakeholders in learning and teaching (e.g., students, universities, and teachers) encountered difficulties in adapting to this sudden change. To deal with these challenges, universities need to utilize the potential of technologies, improve learning experience, and meet students’ expectations. The major limitation of García-Morales et al. (2021) review of the small-sized sample. Meanwhile, García-Morales et al. (2021) also failed to systematically categorize various types of challenges. Stojan et al. (2022) investigated the changes to medical education brought about by the shift to online learning in the COVID-19 pandemic context as well as the lessons and impacts of these changes via a systematic review. A total of 56 articles were included in the analysis, it was reported that small groups and didactics were the most prevalent instructional methods. Although learning engagement was always interactive, teachers majorly integrated technologies to amplify and replace, rather than transform learning. Based on this, they argued that the use of asynchronous and synchronous formats promoted online learning engagement and offered self-directed and flexible learning. The major limitation of this review is that the article is somewhat descriptive and lacks the crucial evaluation of problems of online learning.
Review research has also focused on the changes and impacts brought by online learning during the pandemic period. Camargo et al. (2020) implemented a meta-analysis on seven empirical studies regarding online learning methods during the pandemic period to evaluate feasible online learning platforms, effective online learning models, and the optimal duration of online lectures, as well as the perceptions of teachers and students in the online learning process. Overall, it was concluded that the shift from offline to online learning is feasible, and; effective online learning needs a well-trained and integrated team to identify students’ and teachers’ needs, timely respond, and support them via digital tools. In addition, the pandemic has brought more or less difficulties to online learning. An obvious limitation of this review is the overly small-sized sample ( N = 7), which offers very limited information, but the review tries to answer too many questions (four questions). Grafton-Clarke et al. (2022) investigated the innovation/adaptations implemented, their impacts, and the reasons for their selections in the shift to online learning in medical education during the pandemic period via a systematic review of 55 articles. The major adaptations implemented include the rapid shift to the virtual space, pre-recorded videos or live streaming of surgical procedures, remote adaptations for clinical visits, and multidisciplinary ward rounds and team meetings. Major challenges encountered by students and teachers include the need for technical resources, faculty time, and devices, the shortage of standardized telemedicine curricula, and the lack of personal interactions. Based on this, they criticized the quality of online medical education. Tang (2023) explored the impact of the pandemic on primary, secondary, and tertiary education in the pandemic context via a systematic review of 41 articles. It was reported that the majority of these impacts are negative, e.g., learning loss among learners, assessment and experiential learning in the virtual environment, limitations in instructions, technology-related constraints, the lack of learning materials and resources, and deteriorated psychosocial well-being. These negative impacts are amplified by the unequal distribution of resources, unfair socioeconomic status, ethnicity, gender, physical conditions, and learning ability. Overall, this review comprehensively criticizes the problems brought about by online learning during the pandemic period.
Very little review research evaluated students’ responses to online learning during the pandemic period. For instance, Salas-Pilco et al. (2022) evaluated the engagement in online learning in Latin American higher education during the COVID-19 pandemic period via a systematic review of 23 studies. They considered three dimensions of engagement, including affective, cognitive, and behavioral engagement. They described the characteristics of learning engagement and proposed suggestions for enhancing engagement, including improving Internet connectivity, providing professional training, transforming higher education, ensuring quality, and offering emotional support. A key limitation of the review is that these authors focused on describing the characteristics of engagement without identifying factors that influence engagement.
A synthesis of previous review research offers some implications. First, although learning effectiveness is an important consideration in educational research, review research is scarce on this topic and hence there is a lack of comprehensive knowledge regarding the extent to which online learning is effective during the COVID-19 pandemic period. Second, according to past review research that summarized the major topics of related research, e.g., Bughrara et al. (2023) and Zhang et al. (2022) , the effectiveness of online learning is not a major topic in prior empirical research and hence the author of this article argues that this topic has not received due attention from researchers. Third, some review research has identified a lot of problems in online learning during the pandemic period, e.g., García-Morales et al. (2021) and Stojan et al. (2022) . Many of these problems are caused by the sudden and rapid shift to online learning as well as the unique context of the pandemic. These problems may undermine the effectiveness of online learning. However, the extent to which these problems influence online learning effectiveness is still under-investigated.
1.4 Purpose of the review research
The research is carried out based on a systematic review of past empirical research to answer the following two research questions:
Q1: To what extent online learning in higher education is effective during the COVID-19 pandemic period?
Q2: What factors shape the effectiveness of online learning in higher education during the COVID-19 pandemic period?
2 Research methodology
2.1 literature review as a research methodology.
Regardless of discipline, all academic research activities should be related to and based on existing knowledge. As a result, scholars must identify related research on the topic of interest, critically assess the quality and content of existing research, and synthesize available results ( Linnenluecke et al., 2020 ). However, this task is increasingly challenging for scholars because of the exponential growth of academic knowledge, which makes it difficult to be at the forefront and keep up with state-of-the-art research ( Snyder, 2019 ). Correspondingly, literature review, as a research methodology is more relevant than previously ( Snyder, 2019 ; Linnenluecke et al., 2020 ). A well-implemented review provides a solid foundation for facilitating theory development and advancing knowledge ( Webster and Watson, 2002 ). Here, a literature review is broadly defined as a more or less systematic way of collecting and synthesizing past studies ( Tranfield et al., 2003 ). It allows researchers to integrate perspectives and results from a lot of past research and is able to address research questions unanswered by a single study ( Snyder, 2019 ).
There are generally three types of literature review, including meta-analysis, bibliometric review, and systematic review ( Snyder, 2019 ). A meta-analysis refers to a statistical technique for integrating results from a large volume of empirical research (majorly quantitative research) to compare, identify, and evaluate patterns, relationships, agreements, and disagreements generated by research on the same topic ( Davis et al., 2014 ). This study does not adopt a meta-analysis for two reasons. First, the research on the effectiveness of online learning in the context of the COVID-19 pandemic was published since 2020 and currently, there is a limited volume of empirical evidence. If the study adopts a meta-analysis, the sample size will be small, resulting in limited statistical power. Second, as mentioned above, there are a variety of indicators, e.g., motivation, satisfaction, experience, test score, and perceived effectiveness ( Rajaram and Collins, 2013 ; Noesgaard and Ørngreen, 2015 ), that reflect different aspects of online learning effectiveness. The use of diversified effectiveness indicators increases the difficulty of carrying out meta-analysis.
A bibliometric review refers to the analysis of a large volume of empirical research in terms of publication characteristics (e.g., year, journal, and citation), theories, methods, research questions, countries, and authors ( Donthu et al., 2021 ) and it is useful in tracing the trend, distribution, relationship, and general patterns of research published in a focused topic ( Wallin, 2005 ). A bibliometric review does not fit the present study for two reasons. First, at present, there are less than 4 years of history of research on online learning effectiveness. Hence the volume of relevant research is limited and the public trend is currently unclear. Second, this study is interested in the inner content and results of articles published, rather than their external characteristics.
A systematic review is a method and process of critically identifying and appraising research in a specific field based on predefined inclusion and exclusion criteria to test a hypothesis, answer a research question, evaluate problems in past research, identify research gaps, and/or point out the avenue for future research ( Liberati et al., 2009 ; Moher et al., 2009 ). This type of review is particularly suitable to the present study as there are still a lot of unanswered questions regarding the effectiveness of online learning in the pandemic context, a need for indicating future research direction, a lack of summary of relevant research in this field, and a scarcity of critical appraisal of problems in past research.
Adopting a systematic review methodology brings multiple benefits to the present study. First, it is helpful for distinguishing what needs to be done from what has been done, identifying major contributions made by past research, finding out gaps in past research, avoiding fruitless research, and providing insights for future research in the focused field ( Linnenluecke et al., 2020 ). Second, it is also beneficial for finding out new research directions, needs for theory development, and potential solutions for limitations in past research ( Snyder, 2019 ). Third, this methodology helps scholars to efficiently gain an overview of valuable research results and theories generated by past research, which inspires their research design, ideas, and perspectives ( Callahan, 2014 ).
Commonly, a systematic review can be either author-centric or theme-centric ( Webster and Watson, 2002 ) and the present review is theme-centric. Specifically, an author-centric review focuses on works published by a certain author or a group of authors and summarizes the major contributions made by the author(s; ( Webster and Watson, 2002 ). This type of review is problematic in terms of its incompleteness of research conclusions in a specific field and descriptive nature ( Linnenluecke et al., 2020 ). A theme-centric review is more common where a researcher guides readers through reviewing themes, concepts, and interesting phenomena according to a certain logic ( Callahan, 2014 ). A theme in this review can be further structured into several related sub-themes and this type of review helps researchers to gain a comprehensive understanding of relevant academic knowledge ( Papaioannou et al., 2016 ).
2.2 Research procedures
This study follows the Preferred Reporting Items for Systematic Reviews and Meta-Analyses (PRISMA) guideline ( Liberati et al., 2009 ) to implement a systematic review. The guideline indicates four phases of performing a systematic review, including (1) identifying possible research, (2) abstract screening, (3) assessing full-text for eligibility, and (4) qualitatively synthesizing included research. Figure 1 provides a flowchart of the process and the number of articles excluded and included in each phase.
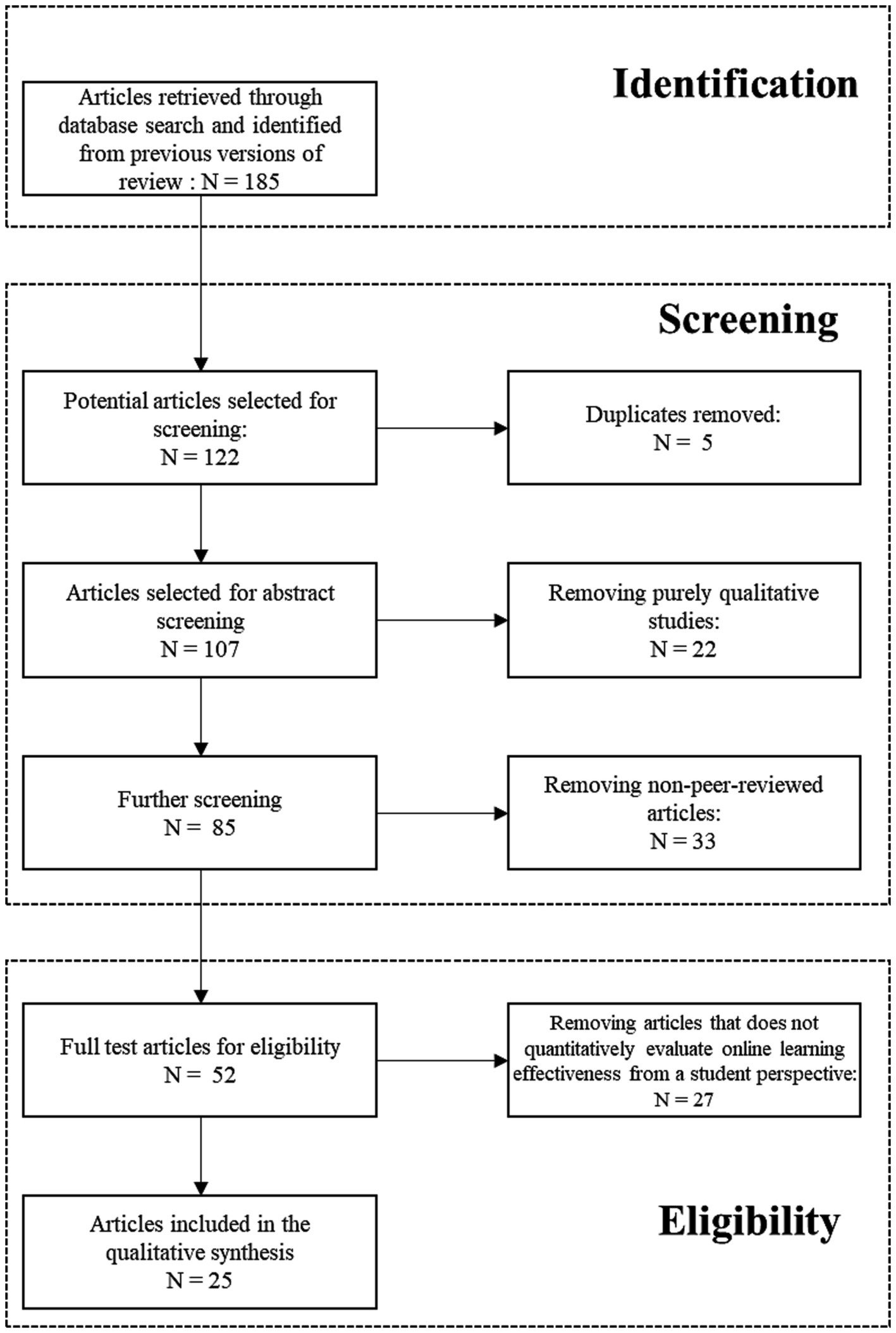
Figure 1 . PRISMA flowchart concerning the selection of articles.
This study uses multiple academic databases to identify possible research, e.g., Academic Search Complete, IGI Global, ACM Digital Library, Elsevier (SCOPUS), Emerald, IEEE Xplore, Web of Science, Science Direct, ProQuest, Wiley Online Library, Taylor and Francis, and EBSCO. Since the COVID-19 pandemic broke out in January 2020, this study limits the literature search to articles published from January 2020 to August 2023. During this period, online learning was highly prevalent in schools globally and a considerable volume of articles were published to investigate various aspects of online learning in this period. Keywords used for searching possible research include pandemic, COVID, SARS-CoV-2, 2019-nCoV, coronavirus, online learning, e-learning, electronic learning, higher education, tertiary education, universities, learning effectiveness, learning satisfaction, learning engagement, and learning motivation. Aside from searching from databases, this study also manually checks the reference lists of relevant articles and uses Google Scholar to find out other articles that have cited these articles.
2.3 Inclusion and exclusion criteria
Articles included in the review must meet the following criteria. First, articles have to be written in English and published on peer-reviewed journals. The academic language being English was chosen because it is in the Q zone of the specified search engines. Second, the research must be carried out in an online learning context. Third, the research must have collected and analyzed empirical data. Fourth, the research should be implemented in a higher education context and during the pandemic period. Fifth, the outcome variable must be factors related to learning effectiveness, and included studies must have reported the quantitative results for online learning effectiveness. The outcome variable should be measured by data collected from students, rather than other individuals (e.g., instructors). For instance, the research of Rahayu and Wirza (2020) used teacher perception as a measurement of online learning effectiveness and was hence excluded from the sample. According to the above criteria, a total of 25 articles were included in the review.
2.4 Data extraction and analysis
Content analysis is performed on included articles and an inductive approach is used to answer the two research questions. First, to understand the basic characteristics of the 25 articles/studies, the researcher summarizes their types, research designs, and samples and categorizes them into several groups. The researcher carefully reads the full-text of these articles and codes valuable pieces of content. In this process, an inductive approach is used, and key themes in these studies have been extracted and summarized. Second, the researcher further categorizes these studies into different groups according to their similarities and differences in research findings. In this way, these studies are broadly categorized into three groups, i.e., (1) ineffective (2) neutral, and (3) effective. Based on this, the research answers the research question and indicates the percentage of studies that evidenced online learning as effective in a COVID-19 pandemic context. The researcher also discusses how online learning is effective by analyzing the learning outcomes brought by online learning. Third, the researcher analyzes and compares the characteristics of the three groups of studies and extracts key themes that are relevant to the conditional effectiveness of online learning from these studies. Based on this, the researcher identifies factors that influence the effectiveness of online learning in a pandemic context. In this way, the two research questions have been adequately answered.
3 Research results and discussion
3.1 study characteristics.
Table 1 shows the statistics of the 25 studies while Table 2 shows a summary of these studies. Overall, these studies varied greatly in terms of research design, research subjects, contexts, measurements of learning effectiveness, and eventually research findings. Approximately half of the studies were published in 2021 and the number of studies reduced in 2022 and 2023, which may be attributed to the fact that universities gradually implemented opening-up policies after 2020. China received the largest number of studies ( N = 5), followed by India ( N = 4) and the United States ( N = 3). The sample sizes of the majority of studies (88.0%) ranged between 101 and 500. As this review excluded qualitative studies, all studies included adopted a purely quantitative design (88.0%) or a mixed design (12.0%). The majority of the studies were cross-sectional (72%) and a few studies (2%) were experimental.
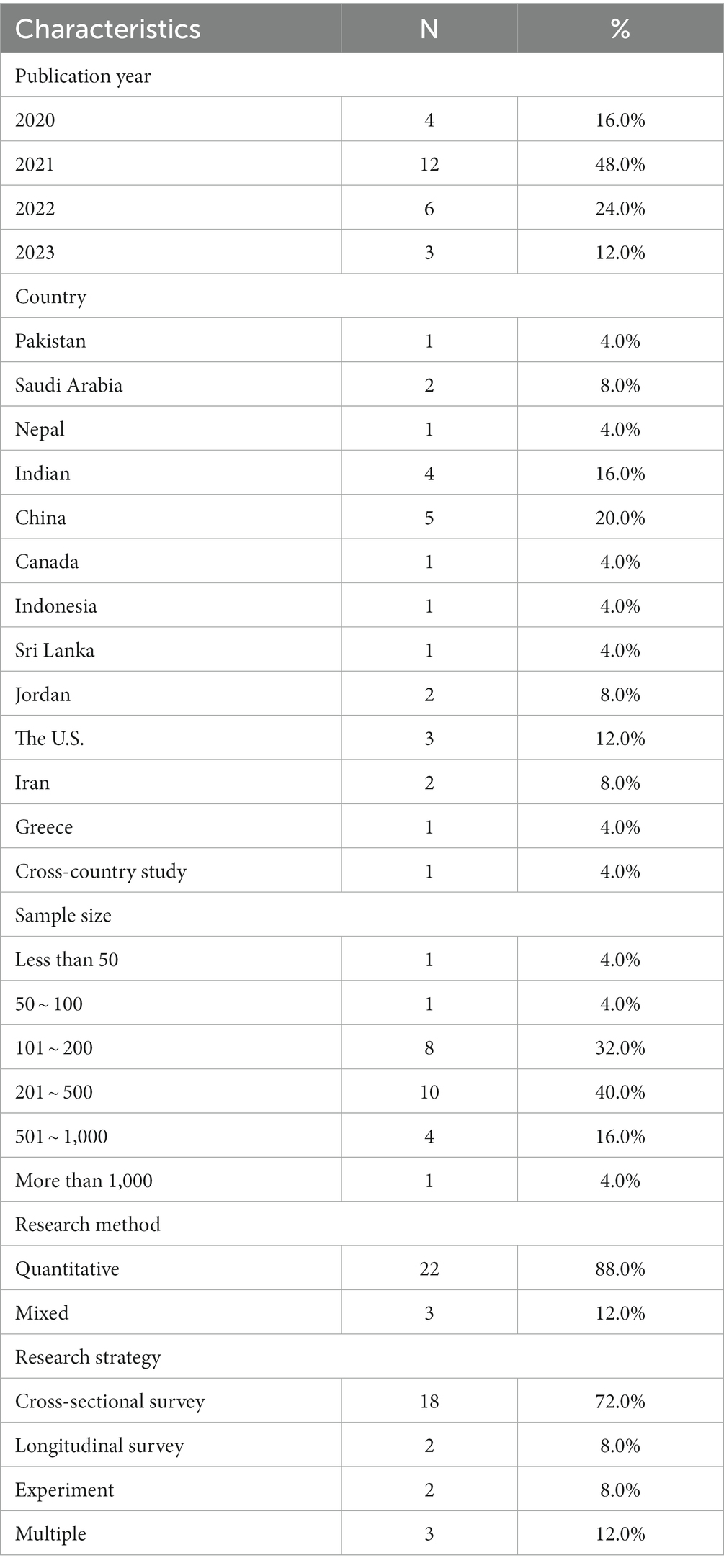
Table 1 . Statistics of studies included in the review.
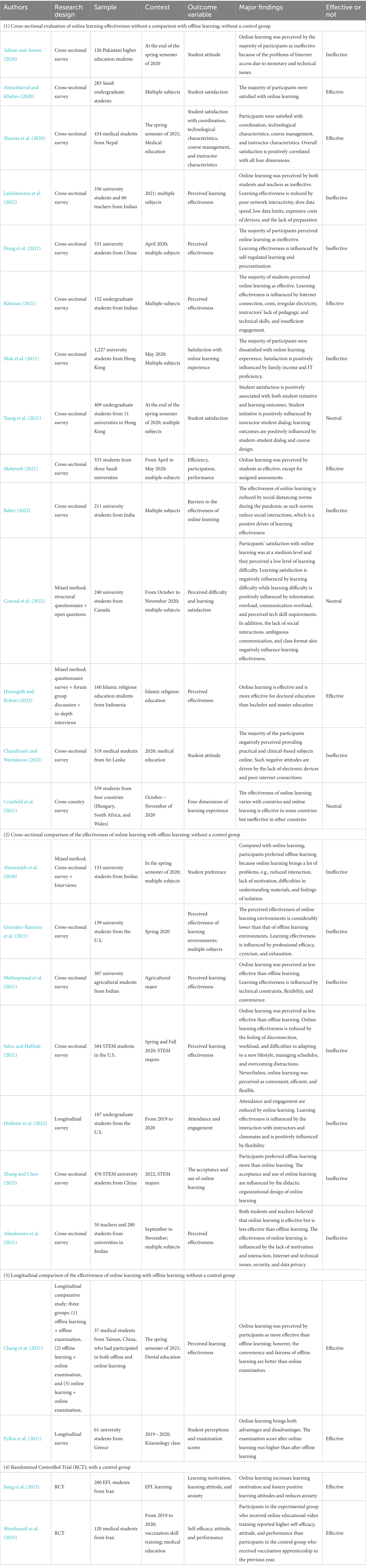
Table 2 . A summary of studies reviewed.
3.2 The effectiveness of online learning
Overall, the 25 studies generated mixed results regarding the effectiveness of online learning during the pandemic period. 9 (36%) studies reported online learning as effective; 13 (52%) studies reported online learning as ineffective, and the rest 3 (12%) studies produced neutral results. However, it should be noted that the results generated by these studies are not comparable as they used different approaches to evaluate the effectiveness of online learning. According to the approach of evaluating online learning effectiveness, these studies are categorized into four groups, including (1) Cross-sectional evaluation of online learning effectiveness without a comparison with offline learning; without a control group ( N = 14; 56%), (2) Cross-sectional comparison of the effectiveness of online learning with offline learning; without control group (7; 28%), (3) Longitudinal comparison of the effectiveness of online learning with offline learning, without a control group ( N = 2; 8%), and (4) Randomized Controlled Trial (RCT); with a control group ( N = 2; 8%).
The first group of studies asked students to report the extent to which they perceived online learning as effective, they had achieved expected learning outcomes through online learning, or they were satisfied with online learning experience or outcomes, without a comparison with offline learning. Six out of 14 studies reported online learning as ineffective, including Adnan and Anwar (2020) , Hong et al. (2021) , Mok et al. (2021) , Baber (2022) , Chandrasiri and Weerakoon (2022) , and Lalduhawma et al. (2022) . Five out of 14 studies reported online learning as effective, including Almusharraf and Khahro (2020) , Sharma et al. (2020) , Mahyoob (2021) , Rahman (2021) , and Haningsih and Rohmi (2022) . In addition, 3 out of 14 studies reported neutral results, including Cranfield et al. (2021) , Tsang et al. (2021) , and Conrad et al. (2022) . It should be noted that this measurement approach is problematic in three aspects. First, researchers used various survey instruments to measure learning effectiveness without reaching a consensus over a widely accepted instrument. As a result, these studies measured different aspects of learning effectiveness and hence their results may be incomparable. Second, these studies relied on students’ self-reports to evaluate learning effectiveness, which is subjective and inaccurate. Third, even though students perceived online learning as effective, it does not imply that online learning is more effective than offline learning because of the absence of comparables.
The second group of studies asked students to compare online learning with offline learning to evaluate learning effectiveness. Interestingly, all 7 studies, including Alawamleh et al. (2020) , Almahasees et al. (2021) , Gonzalez-Ramirez et al. (2021) , Muthuprasad et al. (2021) , Selco and Habbak (2021) , Hollister et al. (2022) , and Zhang and Chen (2023) , reported that online learning was perceived by participants as less effective than offline learning. It should be noted that these results were specific to the COVID-19 pandemic context where strict social distancing policies were implemented. Consequently, these results should be interpreted as online learning during the school lockdown period was perceived by participants as less effective than offline learning during the pre-pandemic period. A key problem of the measurement of learning effectiveness in these studies is subjectivity, i.e., students’ self-reported online learning effectiveness relative to offline learning may be subjective and influenced by a lot of factors caused by the pandemic, e.g., negative emotions (e.g., fear, loneliness, and anxiety).
Only two studies implemented a longitudinal comparison of the effectiveness of online learning with offline learning, i.e., Chang et al. (2021) and Fyllos et al. (2021) . Interestingly, both studies reported that participants perceived online learning as more effective than offline learning, which is contradicted with the second group of studies. In the two studies, the same group of students participated in offline learning and online learning successively and rated the effectiveness of the two learning approaches, respectively. The two studies were implemented by time coincidence, i.e., researchers unexpectedly encountered the pandemic and subsequently, school lockdown when they were investigating learning effectiveness. Such time coincidence enabled them to compare the effectiveness of offline and online learning. However, this research design has three key problems. First, the content of learning in the online and offline learning periods was different and hence the evaluations of learning effectiveness of the two periods are not comparable. Second, self-reported learning effectiveness is subjective. Third, students are likely to obtain better examination scores in online examinations than in offline examinations because online examinations bring a lot of cheating behaviors and are less fair than offline examinations. As reported by Fyllos et al. (2021) , the examination score after online learning was significantly higher than after offline learning. Chang et al. (2021) reported that participants generally believed that offline examinations are fairer than online examinations.
Lastly, only two studies, i.e., Jiang et al. (2023) and Shirahmadi et al. (2023) , implemented an RCT design, which is more persuasive, objective, and accurate than the above-reviewed studies. Indeed, implementing an RCT to evaluate the effectiveness of online learning was a formidable challenge during the pandemic period because of viral transmission and social distancing policies. Both studies reported that online learning is more effective than offline learning during the pandemic period. However, it is questionable about the extent to which such results are affected by health/safety-related issues. It is reasonable to infer that online learning was perceived by students as safer than offline learning during the pandemic period and such perceptions may affect learning effectiveness.
Overall, it is difficult to conclude whether online learning is effective during the pandemic period. Nevertheless, it is possible to identify factors that shape the effectiveness of online learning, which is discussed in the next section.
3.3 Factors that shape online learning effectiveness
Infrastructure factors were reported as the most salient factors that determine online learning effectiveness. It seems that research from developed countries generated more positive results for online learning than research from less developed countries. This view was confirmed by the cross-country comparative study of Cranfield et al. (2021) . Indeed, online learning entails the support of ICT infrastructure, and hence ICT related factors, e.g., Internet connectivity, technical issues, network speed, accessibility of digital devices, and digital devices, considerably influence the effectiveness of online learning ( García-Morales et al., 2021 ; Grafton-Clarke et al., 2022 ). Prior review research, e.g., Tang (2023) also suggested that the unequal distribution of resources and unfair socioeconomic status intensified the problems brought about by online learning during the pandemic period. Salas-Pilco et al. (2022) recommended that improving Internet connectivity would increase students’ engagement in online learning during the pandemic period.
Adnan and Anwar (2020) study is one of the most cited works in the focused field. They reported that online learning is ineffective in Pakistan because of the problems of Internet access due to monetary and technical issues. The above problems hinder students from implementing online learning activities, making online learning ineffective. Likewise, Lalduhawma et al. (2022) research from India indicated that online learning is ineffective because of poor network interactivity, slow data speed, low data limits, and expensive costs of devices. As a result, online learning during the COVID-19 pandemic may have expanded the education gap between developed and developing countries because of developing countries’ infrastructure disadvantages. More attention to online learning infrastructure problems in developing countries is needed.
Instructional factors, e.g., course management and design, instructor characteristics, instructor-student interaction, assignments, and assessments were found to affect online learning effectiveness ( Sharma et al., 2020 ; Rahman, 2021 ; Tsang et al., 2021 ; Hollister et al., 2022 ; Zhang and Chen, 2023 ). Although these instructional factors have been well-documented as significant drivers of learning effectiveness in traditional learning literature, these factors in the pandemic period have some unique characteristics. Both students and teachers were not well prepared for wholly online instruction and learning in 2020 and hence they encountered a lot of problems in course management and design, learning interactions, assignments, and assessments ( Stojan et al., 2022 ; Tang, 2023 ). García-Morales et al. (2021) review also suggested that various stakeholders in learning and teaching encountered difficulties in adapting to the sudden, hasty, and forced transition of offline to online learning. Consequently, these instructional factors become salient in terms of affecting online learning effectiveness.
The negative role of the lack of social interaction caused by social distancing in affecting online learning effectiveness was highlighted by a lot of studies ( Almahasees et al., 2021 ; Baber, 2022 ; Conrad et al., 2022 ; Hollister et al., 2022 ). Baber (2022) argued that people give more importance to saving lives than socializing in the online environment and hence social interactions in learning are considerably reduced by social distancing norms. The negative impact of the lack of social interaction on online learning effectiveness is reflected in two aspects. First, according to a constructivist view, interaction is an indispensable element of learning because knowledge is actively constructed by learners in social interactions ( Woo and Reeves, 2007 ). Consequently, online learning effectiveness during the pandemic period is reduced by the lack of social interaction. Second, the lack of social interaction brings a lot of negative emotions, e.g., feelings of isolation, loneliness, anxiety, and depression ( Alawamleh et al., 2020 ; Gonzalez-Ramirez et al., 2021 ; Selco and Habbak, 2021 ). Such negative emotions undermine online learning effectiveness.
Negative emotions caused by the pandemic and school lockdown were also found to be detrimental to online learning effectiveness. In this context, it was reported that many students experience a lot of negative emotions, e.g., feelings of isolation, exhaustion, loneliness, and distraction ( Alawamleh et al., 2020 ; Gonzalez-Ramirez et al., 2021 ; Selco and Habbak, 2021 ). Such negative emotions, as mentioned above, reduce online learning effectiveness.
Several factors were also found to increase online learning effectiveness during the pandemic period, e.g., convenience and flexibility ( Hong et al., 2021 ; Muthuprasad et al., 2021 ; Selco and Habbak, 2021 ). Students with strong self-regulated learning abilities gain more benefits from convenience and flexibility in online learning ( Hong et al., 2021 ).
Overall, although it is debated over the effectiveness of online learning during the pandemic period, it is generally believed that the pandemic brings a lot of challenges and difficulties to higher education. Meanwhile, the majority of students prefer offline learning to online learning. The above challenges and difficulties are more prominent in developing countries than in developed countries.
3.4 Pedagogical implications
The results generated by the systematic review offer a lot of pedagogical implications. First, online learning entails the support of ICT infrastructure, and infrastructure defects strongly undermine learning effectiveness ( García-Morales et al., 2021 ; Grafton-Clarke et al., 2022 ). Given the fact online learning is increasingly integrated into higher education ( Kebritchi et al., 2017 ) regardless of the presence of the pandemic, governments globally should increase the investment in learning-related ICT infrastructure in higher education institutes. Meanwhile, schools should consider students’ affordability of digital devices and network fees when implementing online learning activities. It is important to offer material support for those students with poor economic status. Infrastructure issues are more prominent in developing countries because of the lack of monetary resources and poor infrastructure base. Thus, international collaboration and aid are recommended to address these issues.
Second, since the lack of social interaction is a key factor that reduces online learning effectiveness, it is important to increase social interactions during the implementation of online learning activities. On the one hand, both students and instructors are encouraged to utilize network technologies to promote inter-individual interactions. On the other hand, the two parties are also encouraged to engage in offline interaction activities if the risk is acceptable.
Third, special attention should be paid to students’ emotions during the online learning process as online learning may bring a lot of negative emotions to students, which undermine learning effectiveness ( Alawamleh et al., 2020 ; Gonzalez-Ramirez et al., 2021 ; Selco and Habbak, 2021 ). In addition, higher education institutes should prepare a contingency plan for emergency online learning to deal with potential crises in the future, e.g., wars, pandemics, and natural disasters.
3.5 Limitations and suggestions for future research
There are several limitations in past research regarding online learning effectiveness during the pandemic period. The first is the lack of rigor in assessing learning effectiveness. Evidently, there is a scarcity of empirical research with an RCT design, which is considered to be accurate, objective, and rigorous in assessing pedagogical models ( Torgerson and Torgerson, 2001 ). The scarcity of ICT research leads to the difficulty in accurately assessing the effectiveness of online learning and comparing it with offline learning. Second, the widely accepted criteria for assessing learning effectiveness are absent, and past empirical studies used diversified procedures, techniques, instruments, and criteria for measuring online learning effectiveness, resulting in difficulty in comparing research results. Third, learning effectiveness is a multi-dimensional construct but its multidimensionality was largely ignored by past research. Therefore, it is difficult to evaluate which dimensions of learning effectiveness are promoted or undermined by online learning and it is also difficult to compare the results of different studies. Finally, there is very limited knowledge about the difference in online learning effectiveness between different subjects. It is likely that the subjects that depend on lab-based work (e.g., experimental physics, organic chemistry, and cell biology) are less appropriate for online learning than the subjects that depend on desk-based work (e.g., economics, psychology, and literature).
To deal with the above limitations, there are several recommendations for future research on online learning effectiveness. First, future research is encouraged to adopt an RCT design and collect a large-sized sample to objectively, rigorously, and accurately quantify the effectiveness of online learning. Second, scholars are also encouraged to develop a new framework to assess learning effectiveness comprehensively. This framework should cover multiple dimensions of learning effectiveness and have strong generalizability. Finally, it is recommended that future research could compare the effectiveness of online learning between different subjects.
4 Conclusion
This study carried out a systematic review of 25 empirical studies published between 2020 and 2023 to evaluate the effectiveness of online learning during the COVID-19 pandemic period. According to how online learning effectiveness was assessed, these 25 studies were categorized into four groups. The first group of studies employed a cross-sectional design and assessed online learning based on students’ perceptions without a control group. Less than half of these studies reported online learning as effective. The second group of studies also employed a cross-sectional design and asked students to compare the effectiveness of online learning with offline learning. All these studies reported that online learning is less effective than offline learning. The third group of studies employed a longitudinal design and compared the effectiveness of online learning with offline learning but without a control group and this group includes only 2 studies. It was reported that online learning is more effective than offline learning. The fourth group of studies employed an RCT design and this group includes only 2 studies. Both studies reported online learning as more effective than offline learning.
Overall, it is difficult to conclude whether online learning is effective during the pandemic period because of the diversified research contexts, methods, and approaches in past research. Nevertheless, the review identifies a set of factors that positively or negatively influence the effectiveness of online learning, including infrastructure factors, instructional factors, the lack of social interaction, negative emotions, flexibility, and convenience. Although it is debated over the effectiveness of online learning during the pandemic period, it is generally believed that the pandemic brings a lot of challenges and difficulties to higher education. Meanwhile, the majority of students prefer offline learning to online learning. In addition, developing countries face more challenges and difficulties in online learning because of monetary and infrastructure issues.
The findings of this review offer significant pedagogical implications for online learning in higher education institutes, including enhancing the development of ICT infrastructure, providing material support for students with poor economic status, enhancing social interactions, paying attention to students’ emotional status, and preparing a contingency plan of emergency online learning.
The review also identifies several limitations in past research regarding online learning effectiveness during the pandemic period, including the lack of rigor in assessing learning effectiveness, the absence of accepted criteria for assessing learning effectiveness, the neglect of the multidimensionality of learning effectiveness, and limited knowledge about the difference in online learning effectiveness between different subjects.
To deal with the above limitations, there are several recommendations for future research on online learning effectiveness. First, future research is encouraged to adopt an RCT design and collect a large-sized sample to objectively, rigorously, and accurately quantify the effectiveness of online learning. Second, scholars are also encouraged to develop a new framework to assess learning effectiveness comprehensively. This framework should cover multiple dimensions of learning effectiveness and have strong generalizability. Finally, it is recommended that future research could compare the effectiveness of online learning between different subjects. To fix these limitations in future research, recommendations are made.
It should be noted that this review is not free of problems. First, only studies that quantitatively measured online learning effectiveness were included in the review and hence a lot of other studies (e.g., qualitative studies) that investigated factors that influence online learning effectiveness were excluded, resulting in a relatively small-sized sample and incomplete synthesis of past research contributions. Second, since this review was qualitative, it was difficult to accurately quantify the level of online learning effectiveness.
Data availability statement
The original contributions presented in the study are included in the article/supplementary material, further inquiries can be directed to the corresponding author.
Author contributions
WM: Writing – original draft, Writing – review & editing. LY: Writing – original draft, Writing – review & editing. CL: Writing – review & editing. NP: Writing – review & editing. XP: Writing – review & editing. YZ: Writing – review & editing.
The author(s) declare that no financial support was received for the research, authorship, and/or publication of this article.
Conflict of interest
The authors declare that the research was conducted in the absence of any commercial or financial relationships that could be construed as a potential conflict of interest.
Publisher’s note
All claims expressed in this article are solely those of the authors and do not necessarily represent those of their affiliated organizations, or those of the publisher, the editors and the reviewers. Any product that may be evaluated in this article, or claim that may be made by its manufacturer, is not guaranteed or endorsed by the publisher.
Adnan, M., and Anwar, K. (2020). Online learning amid the COVID-19 pandemic: Students' perspectives. J. Pedagogical Sociol. Psychol. 1, 45–51. doi: 10.33902/JPSP.2020261309
Crossref Full Text | Google Scholar
Alawamleh, M., Al-Twait, L. M., and Al-Saht, G. R. (2020). The effect of online learning on communication between instructors and students during Covid-19 pandemic. Asian Educ. Develop. Stud. 11, 380–400. doi: 10.1108/AEDS-06-2020-0131
Almahasees, Z., Mohsen, K., and Amin, M. O. (2021). Faculty’s and students’ perceptions of online learning during COVID-19. Front. Educ. 6:638470. doi: 10.3389/feduc.2021.638470
Almusharraf, N., and Khahro, S. (2020). Students satisfaction with online learning experiences during the COVID-19 pandemic. Int. J. Emerg. Technol. Learn. (iJET) 15, 246–267. doi: 10.3991/ijet.v15i21.15647
Anderson, N., and Hajhashemi, K. (2013). Online learning: from a specialized distance education paradigm to a ubiquitous element of contemporary education. In 4th international conference on e-learning and e-teaching (ICELET 2013) (pp. 91–94). IEEE.
Google Scholar
Arkorful, V., and Abaidoo, N. (2015). The role of e-learning, advantages and disadvantages of its adoption in higher education. Int. J. Instructional Technol. Distance Learn. 12, 29–42.
Baber, H. (2022). Social interaction and effectiveness of the online learning–a moderating role of maintaining social distance during the pandemic COVID-19. Asian Educ. Develop. Stud. 11, 159–171. doi: 10.1108/AEDS-09-2020-0209
Barnard-Brak, L., Paton, V. O., and Lan, W. Y. (2010). Profiles in self-regulated learning in the online learning environment. Int. Rev. Res. Open Dist. Learn. 11, 61–80. doi: 10.19173/irrodl.v11i1.769
Bughrara, M. S., Swanberg, S. M., Lucia, V. C., Schmitz, K., Jung, D., and Wunderlich-Barillas, T. (2023). Beyond COVID-19: the impact of recent pandemics on medical students and their education: a scoping review. Med. Educ. Online 28:2139657. doi: 10.1080/10872981.2022.2139657
PubMed Abstract | Crossref Full Text | Google Scholar
Callahan, J. L. (2014). Writing literature reviews: a reprise and update. Hum. Resour. Dev. Rev. 13, 271–275. doi: 10.1177/1534484314536705
Camargo, C. P., Tempski, P. Z., Busnardo, F. F., Martins, M. D. A., and Gemperli, R. (2020). Online learning and COVID-19: a meta-synthesis analysis. Clinics 75:e2286. doi: 10.6061/clinics/2020/e2286
Choudhury, S., and Pattnaik, S. (2020). Emerging themes in e-learning: A review from the stakeholders’ perspective. Computers and Education 144, 103657. doi: 10.1016/j.compedu.2019.103657
Chandrasiri, N. R., and Weerakoon, B. S. (2022). Online learning during the COVID-19 pandemic: perceptions of allied health sciences undergraduates. Radiography 28, 545–549. doi: 10.1016/j.radi.2021.11.008
Chang, J. Y. F., Wang, L. H., Lin, T. C., Cheng, F. C., and Chiang, C. P. (2021). Comparison of learning effectiveness between physical classroom and online learning for dental education during the COVID-19 pandemic. J. Dental Sci. 16, 1281–1289. doi: 10.1016/j.jds.2021.07.016
Conrad, C., Deng, Q., Caron, I., Shkurska, O., Skerrett, P., and Sundararajan, B. (2022). How student perceptions about online learning difficulty influenced their satisfaction during Canada's Covid-19 response. Br. J. Educ. Technol. 53, 534–557. doi: 10.1111/bjet.13206
Cranfield, D. J., Tick, A., Venter, I. M., Blignaut, R. J., and Renaud, K. (2021). Higher education students’ perceptions of online learning during COVID-19—a comparative study. Educ. Sci. 11, 403–420. doi: 10.3390/educsci11080403
Desai, M. S., Hart, J., and Richards, T. C. (2008). E-learning: paradigm shift in education. Education 129, 1–20.
Davis, J., Mengersen, K., Bennett, S., and Mazerolle, L. (2014). Viewing systematic reviews and meta-analysis in social research through different lenses. SpringerPlus 3, 1–9. doi: 10.1186/2193-1801-3-511
Donthu, N., Kumar, S., Mukherjee, D., Pandey, N., and Lim, W. M. (2021). How to conduct a bibliometric analysis: An overview and guidelines. Journal of business research 133, 264–269. doi: 10.1016/j.jbusres.2021.04.070
Fyllos, A., Kanellopoulos, A., Kitixis, P., Cojocari, D. V., Markou, A., Raoulis, V., et al. (2021). University students perception of online education: is engagement enough? Acta Informatica Medica 29, 4–9. doi: 10.5455/aim.2021.29.4-9
Gamage, D., Ruipérez-Valiente, J. A., and Reich, J. (2023). A paradigm shift in designing education technology for online learning: opportunities and challenges. Front. Educ. 8:1194979. doi: 10.3389/feduc.2023.1194979
García-Morales, V. J., Garrido-Moreno, A., and Martín-Rojas, R. (2021). The transformation of higher education after the COVID disruption: emerging challenges in an online learning scenario. Front. Psychol. 12:616059. doi: 10.3389/fpsyg.2021.616059
Gonzalez-Ramirez, J., Mulqueen, K., Zealand, R., Silverstein, S., Mulqueen, C., and BuShell, S. (2021). Emergency online learning: college students' perceptions during the COVID-19 pandemic. Coll. Stud. J. 55, 29–46.
Grafton-Clarke, C., Uraiby, H., Gordon, M., Clarke, N., Rees, E., Park, S., et al. (2022). Pivot to online learning for adapting or continuing workplace-based clinical learning in medical education following the COVID-19 pandemic: a BEME systematic review: BEME guide no. 70. Med. Teach. 44, 227–243. doi: 10.1080/0142159X.2021.1992372
Haningsih, S., and Rohmi, P. (2022). The pattern of hybrid learning to maintain learning effectiveness at the higher education level post-COVID-19 pandemic. Eurasian J. Educ. Res. 11, 243–257. doi: 10.12973/eu-jer.11.1.243
Hollister, B., Nair, P., Hill-Lindsay, S., and Chukoskie, L. (2022). Engagement in online learning: student attitudes and behavior during COVID-19. Front. Educ. 7:851019. doi: 10.3389/feduc.2022.851019
Hong, J. C., Lee, Y. F., and Ye, J. H. (2021). Procrastination predicts online self-regulated learning and online learning ineffectiveness during the coronavirus lockdown. Personal. Individ. Differ. 174:110673. doi: 10.1016/j.paid.2021.110673
Jiang, P., Namaziandost, E., Azizi, Z., and Razmi, M. H. (2023). Exploring the effects of online learning on EFL learners’ motivation, anxiety, and attitudes during the COVID-19 pandemic: a focus on Iran. Curr. Psychol. 42, 2310–2324. doi: 10.1007/s12144-022-04013-x
Joy, E. H., and Garcia, F. E. (2000). Measuring learning effectiveness: a new look at no-significant-difference findings. JALN 4, 33–39.
Kebritchi, M., Lipschuetz, A., and Santiague, L. (2017). Issues and challenges for teaching successful online courses in higher education: a literature review. J. Educ. Technol. Syst. 46, 4–29. doi: 10.1177/0047239516661713
Lalduhawma, L. P., Thangmawia, L., and Hussain, J. (2022). Effectiveness of online learning during the COVID-19 pandemic in Mizoram. J. Educ. e-Learning Res. 9, 175–183. doi: 10.20448/jeelr.v9i3.4162
Liberati, A., Altman, D. G., Tetzlaff, J., Mulrow, C., Gotzsche, P. C., Ioannidis, J. P., et al. (2009). The PRISMA statement for reporting systematic reviews and meta-analyses of studies that evaluate health care interventions: explanation and elaboration. Annals of internal medicine , 151, W-65. doi: 10.7326/0003-4819-151-4-200908180-00136
Linnenluecke, M. K., Marrone, M., and Singh, A. K. (2020). Conducting systematic literature reviews and bibliometric analyses. Aust. J. Manag. 45, 175–194. doi: 10.1177/0312896219877678
Mahyoob, M. (2021). Online learning effectiveness during the COVID-19 pandemic: a case study of Saudi universities. Int. J. Info. Commun. Technol. Educ. (IJICTE) 17, 1–14. doi: 10.4018/IJICTE.20211001.oa7
Moher, D., Liberati, A., Tetzlaff, D., and Altman, G. and PRISMA Group (2009). Preferred reporting items for systematic reviews and meta-analyses: the PRISMA statement. Annals of internal medicine , 151, 264–269. doi: 10.3736/jcim20090918
Mok, K. H., Xiong, W., and Bin Aedy Rahman, H. N. (2021). COVID-19 pandemic’s disruption on university teaching and learning and competence cultivation: student evaluation of online learning experiences in Hong Kong. Int. J. Chinese Educ. 10:221258682110070. doi: 10.1177/22125868211007011
Muthuprasad, T., Aiswarya, S., Aditya, K. S., and Jha, G. K. (2021). Students’ perception and preference for online education in India during COVID-19 pandemic. Soc. Sci. Humanities open 3:100101. doi: 10.1016/j.ssaho.2020.100101
Noesgaard, S. S., and Ørngreen, R. (2015). The effectiveness of e-learning: an explorative and integrative review of the definitions, methodologies and factors that promote e-learning effectiveness. Electronic J. E-learning 13, 278–290.
Papaioannou, D., Sutton, A., and Booth, A. (2016). Systematic approaches to a successful literature review. London: Sage.
Pratama, H., Azman, M. N. A., Kassymova, G. K., and Duisenbayeva, S. S. (2020). The trend in using online meeting applications for learning during the period of pandemic COVID-19: a literature review. J. Innovation in Educ. Cultural Res. 1, 58–68. doi: 10.46843/jiecr.v1i2.15
Rahayu, R. P., and Wirza, Y. (2020). Teachers’ perception of online learning during pandemic covid-19. Jurnal penelitian pendidikan 20, 392–406. doi: 10.17509/jpp.v20i3.29226
Rahman, A. (2021). Using students’ experience to derive effectiveness of COVID-19-lockdown-induced emergency online learning at undergraduate level: evidence from Assam. India. Higher Education for the Future 8, 71–89. doi: 10.1177/2347631120980549
Rajaram, K., and Collins, B. (2013). Qualitative identification of learning effectiveness indicators among mainland Chinese students in culturally dislocated study environments. J. Int. Educ. Bus. 6, 179–199. doi: 10.1108/JIEB-03-2013-0010
Salas-Pilco, S. Z., Yang, Y., and Zhang, Z. (2022). Student engagement in online learning in Latin American higher education during the COVID-19 pandemic: a systematic review. Br. J. Educ. Technol. 53, 593–619. doi: 10.1111/bjet.13190
Selco, J. I., and Habbak, M. (2021). Stem students’ perceptions on emergency online learning during the covid-19 pandemic: challenges and successes. Educ. Sci. 11:799. doi: 10.3390/educsci11120799
Sharma, K., Deo, G., Timalsina, S., Joshi, A., Shrestha, N., and Neupane, H. C. (2020). Online learning in the face of COVID-19 pandemic: assessment of students’ satisfaction at Chitwan medical college of Nepal. Kathmandu Univ. Med. J. 18, 40–47. doi: 10.3126/kumj.v18i2.32943
Shirahmadi, S., Hazavehei, S. M. M., Abbasi, H., Otogara, M., Etesamifard, T., Roshanaei, G., et al. (2023). Effectiveness of online practical education on vaccination training in the students of bachelor programs during the Covid-19 pandemic. PLoS One 18:e0280312. doi: 10.1371/journal.pone.0280312
Snyder, H. (2019). Literature review as a research methodology: an overview and guidelines. J. Bus. Res. 104, 333–339. doi: 10.1016/j.jbusres.2019.07.039
Stojan, J., Haas, M., Thammasitboon, S., Lander, L., Evans, S., Pawlik, C., et al. (2022). Online learning developments in undergraduate medical education in response to the COVID-19 pandemic: a BEME systematic review: BEME guide no. 69. Med. Teach. 44, 109–129. doi: 10.1080/0142159X.2021.1992373
Swan, K. (2003). Learning effectiveness online: what the research tells us. Elements of quality online education, practice and direction 4, 13–47.
Tang, K. H. D. (2023). Impacts of COVID-19 on primary, secondary and tertiary education: a comprehensive review and recommendations for educational practices. Educ. Res. Policy Prac. 22, 23–61. doi: 10.1007/s10671-022-09319-y
Torgerson, C. J., and Torgerson, D. J. (2001). The need for randomised controlled trials in educational research. Br. J. Educ. Stud. 49, 316–328. doi: 10.1111/1467-8527.t01-1-00178
Tranfield, D., Denyer, D., and Smart, P. (2003). Towards a methodology for developing evidence‐informed management knowledge by means of systematic review. British journal of management , 14, 207–222. doi: 10.1111/1467-8551.00375
Tsang, J. T., So, M. K., Chong, A. C., Lam, B. S., and Chu, A. M. (2021). Higher education during the pandemic: the predictive factors of learning effectiveness in COVID-19 online learning. Educ. Sci. 11:446. doi: 10.3390/educsci11080446
Wallin, J. A. (2005). Bibliometric methods: pitfalls and possibilities. Basic Clin. Pharmacol. Toxicol. 97, 261–275. doi: 10.1111/j.1742-7843.2005.pto_139.x
Webster, J., and Watson, R. T. (2002). Analyzing the past to prepare for the future: Writing a literature review. MIS quarterly , 26, 13–23.
Wong, J., Baars, M., Davis, D., Van Der Zee, T., Houben, G. J., and Paas, F. (2019). Supporting self-regulated learning in online learning environments and MOOCs: a systematic review. Int. J. Human–Computer Interaction 35, 356–373. doi: 10.1080/10447318.2018.1543084
Woo, Y., and Reeves, T. C. (2007). Meaningful interaction in web-based learning: a social constructivist interpretation. Internet High. Educ. 10, 15–25. doi: 10.1016/j.iheduc.2006.10.005
Zeitoun, H. (2008). E-learning: Concept, Issues, Application, Evaluation . Riyadh: Dar Alsolateah Publication.
Zhang, L., Carter, R. A. Jr., Qian, X., Yang, S., Rujimora, J., and Wen, S. (2022). Academia's responses to crisis: a bibliometric analysis of literature on online learning in higher education during COVID-19. Br. J. Educ. Technol. 53, 620–646. doi: 10.1111/bjet.13191
Zhang, Y., and Chen, X. (2023). Students’ perceptions of online learning in the post-COVID era: a focused case from the universities of applied sciences in China. Sustain. For. 15:946. doi: 10.3390/su15020946
Keywords: COVID-19 pandemic, higher education, online learning, learning effectiveness, systematic review
Citation: Meng W, Yu L, Liu C, Pan N, Pang X and Zhu Y (2024) A systematic review of the effectiveness of online learning in higher education during the COVID-19 pandemic period. Front. Educ . 8:1334153. doi: 10.3389/feduc.2023.1334153
Received: 06 November 2023; Accepted: 27 December 2023; Published: 17 January 2024.
Reviewed by:
Copyright © 2024 Meng, Yu, Liu, Pan, Pang and Zhu. This is an open-access article distributed under the terms of the Creative Commons Attribution License (CC BY) . The use, distribution or reproduction in other forums is permitted, provided the original author(s) and the copyright owner(s) are credited and that the original publication in this journal is cited, in accordance with accepted academic practice. No use, distribution or reproduction is permitted which does not comply with these terms.
*Correspondence: Lei Yu, [email protected]
Advertisement
A Survey on the Effectiveness of Online Teaching–Learning Methods for University and College Students
- Article of professional interests
- Published: 05 April 2021
- Volume 102 , pages 1325–1334, ( 2021 )
Cite this article
- Preethi Sheba Hepsiba Darius ORCID: orcid.org/0000-0003-0882-6213 1 ,
- Edison Gundabattini ORCID: orcid.org/0000-0003-4217-2321 2 &
- Darius Gnanaraj Solomon ORCID: orcid.org/0000-0001-5321-5775 2
131k Accesses
32 Citations
Explore all metrics
Online teaching–learning methods have been followed by world-class universities for more than a decade to cater to the needs of students who stay far away from universities/colleges. But during the COVID-19 pandemic period, online teaching–learning helped almost all universities, colleges, and affiliated students. An attempt is made to find the effectiveness of online teaching–learning methods for university and college students by conducting an online survey. A questionnaire has been specially designed and deployed among university and college students. About 450 students from various universities, engineering colleges, medical colleges in South India have taken part in the survey and submitted responses. It was found that the following methods promote effective online learning: animations, digital collaborations with peers, video lectures delivered by faculty handling the subject, online quiz having multiple-choice questions, availability of student version software, a conducive environment at home, interactions by the faculty during lectures and online materials provided by the faculty. Moreover, online classes are more effective because they provide PPTs in front of every student, lectures are heard by all students at the sound level of their choice, and walking/travel to reach classes is eliminated.
Avoid common mistakes on your manuscript.
Introduction
Critical thinking and creativity of students increase with innovative educational methods according to the world declaration on higher education in the twenty-first century [ 1 ]. Innovative educational strategies and educational innovations are required to make the students learn. There are three vertices in the teaching–learning process viz., teaching, communication technology through digital tools, and innovative practices in teaching. In the first vertex, the teacher is a facilitator and provides resources and tools to students and helps them to develop new knowledge and skills. Project-based learning helps teachers and students to promote collaborative learning by discussing specific topics. Cognitive independence is developed among students. To promote global learning, teachers are required to innovate permanently. It is possible when university professors and researchers are given space to new educational forms in different areas of specializations. Virtual classrooms, unlike traditional classrooms, give unlimited scope for introducing teaching innovation strategies. The second vertex refers to the use of Information and Communication Technology (ICT) tools for promoting innovative education. Learning management systems (LMS) help in teaching, learning, educational administration, testing, and evaluation. The use of ICT tools promotes technological innovations and advances in learning and knowledge management. The third vertex deals with innovations in teaching/learning to solve problems faced by teachers and students. Creative use of new elements related to curriculum, production of something new, and transformations emerge in classrooms resulting in educational innovations. Evaluations are necessary to improve the innovations so that successful methods can be implemented in all teaching and learning community in an institution [ 2 ]. The pandemic has forced digital learning and job portal Naukri.com reports a fourfold growth for teaching professionals in the e-learning medium [ 3 ]. The initiatives are taken by the government also focus on online mode as an option in a post-covid world [ 4 ]. A notable learning experience design consultant pointed out that, educators are entrusted to lead the way as the world changes and are actively involved in the transformation [ 5 ]. Weiss notes that an educator needs to make the lectures more interesting [ 6 ].
This paper presents the online teaching–learning tools, methods, and a survey on the innovative practices in teaching and learning. Advantages and obstacles in online teaching, various components on the effective use of online tools, team-based collaborative learning, simulation, and animation-based learning are discussed in detail. The outcome of a survey on the effectiveness of online teaching and learning is included. The following sections present the online teaching–learning tools, the details of the questionnaire used for the survey, and the outcome of the survey.
Online Teaching and Learning Tools
The four essential parts of online teaching [ 7 ] are virtual classrooms, individual activities, assessments in real-time, and collaborative group work. Online teaching tools are used to facilitate faculty-student interaction as well as student–student collaborations [ 8 ]. The ease of use, the satisfaction level, the usefulness, and the confidence level of the instructor is crucial [ 9 ] in motivating the instructor to use online teaching tools. Higher education institutes recognize the need to accommodate wide diverse learners and Hilliard [ 10 ] points out that technical support and awareness to both faculty and student is essential in the age of blended learning. Data analytics tool coupled with the LMS is essential to enhance [ 11 ] the quality of teaching and improve the course design. The effective usage of online tools is depicted in Fig. 1 comprising of an instructor to student delivery, collaboration among students, training for the tools, and data analytics for constant improvement of course and assessment methods.
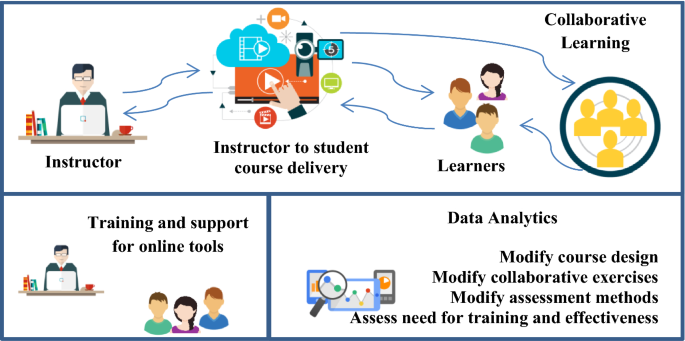
The various components of effective usage of online tools
Online Teaching Tools
A plethora of online teaching tools are available and this poses a challenge for decision-makers to choose the tools that best suits the needs of the course. The need for the tools, the cost, usability, and features determine which tools are adopted by various learners and institutions. Many universities have offered online classes for students. These are taken up by students opting for part-time courses. This offers them flexibility in timing and eliminates the need for travel to campus. The pandemic situation in 2019 has forced many if not all institutions to completely shift classes online. LMS tools are packaged as Software as a Service (SaaS) and the pricing generally falls into 4 categories: (i) per learner, per month (ii) per learner, per use (iii) per course (iv) licensing fee for on-premise installation [ 12 ].
Online Learning Tools
Online teaching/learning as part of the ongoing semester is typically part of a classroom management tool. GSuite for education [ 13 ] and Microsoft Teams [ 14 ] are both widely adopted by schools and colleges during the COVID-19 pandemic to effectively shift regular classes online. Other popular learning management systems that have been adopted as part of blended learning are Edmodo [ 15 ], Blackboard [ 16 ], and MoodleCloud [ 17 ]. Davis et al. [ 18 ] point out advantages and obstacles for both students and instructors about online teaching shown in Table 1 .
The effectiveness of course delivery depends on using the appropriate tools in the course design. This involves engaging the learners and modifying the course design to cater to various learning styles.
A Survey on Innovative Practices in Teaching and Learning
The questionnaire aims to identify the effectiveness of various online tools and technologies, the preferred learning methods of students, and other factors that might influence the teaching–learning process. The parameters were based on different types of learners, advantages, and obstacles to online learning [ 10 , 18 ]. Questions 1–4 are used to comprehend the learning style of the student. Questions 5–7 are posed to find out the effectiveness of the medium used for teaching and evaluation. Questions 8–12 are framed to identify the various barriers to online learning faced by students.
This methodology is adopted as most of the students are attending online courses from home and polls of this kind will go well with the students from various universities. Students participated in the survey and answered most of the questionnaire enthusiastically. The only challenge was a suitable environment and free time for them to answer the questionnaire, as they are already loaded with lots of online work. Students from various universities pursuing professional courses like engineering and medicine took part in this survey. They are from various branches of sciences and technologies. Students are from private universities, colleges, and government institutions. Figure 2 shows the institution-wise respondents. Microsoft Teams and Google meet platforms were used for this survey among university, medical college, and engineering college students. About 450 students responded to this survey. 52% of the respondents are from VIT University Vellore, Tamil Nadu, 23% of the respondents are from CMR Institute of Technology (CMRIT), Bangalore, 15% of the respondents are from medical colleges and 10% are from other engineering colleges. During this pandemic period, VIT students are staying with parents who are living in different states of India like Andhra, Telangana, Kerala, Karnataka, MP, Haryana, Punjab, Maharashtra, Andaman, and so on. Only a few students are living in Tamil Nadu. Some of the students are staying with parents in other countries like Dubai, Oman, South Africa, and so on. Some of the students of CMRIT Bangalore are living in Bangalore and others in towns and villages of Karnataka state. Students of medical colleges are living in different parts of Tamil Nadu and students of engineering colleges are living in different parts of Andhra Pradesh. Hence, the survey is done in a wider geographical region.
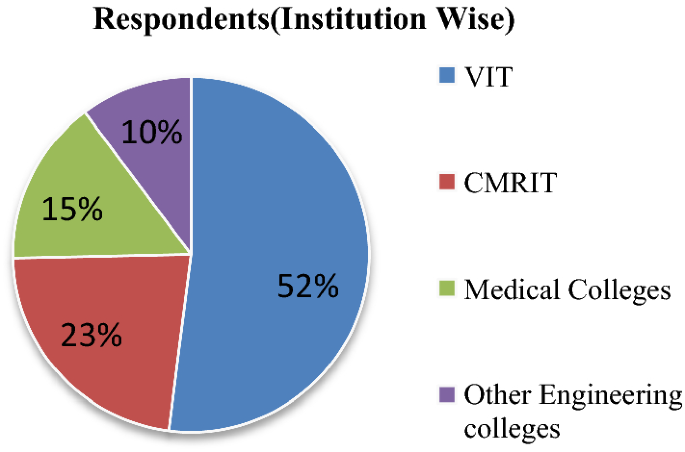
Institution-wise respondents
Figure 3 shows the branch-wise respondents. It is shown that 158 students belong to mechanical/civil engineering. 108 respondents belong to computer science and engineering, 68 students belong to medicine, 58 students belong to electrical & electronics engineering, and electronics & communication engineering. 58 students belong to other disciplines.
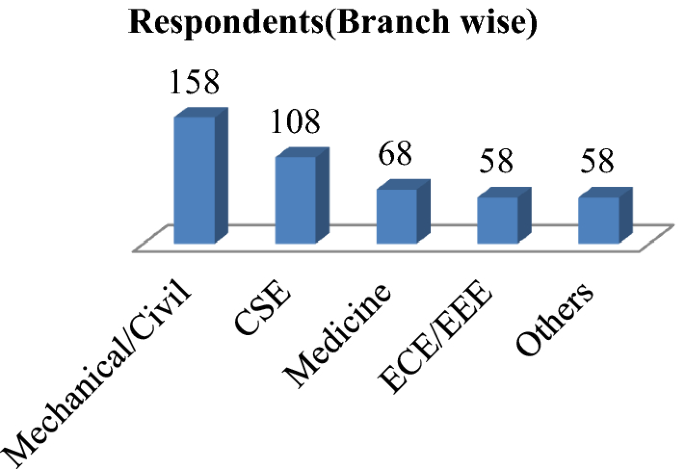
Branch-wise respondents
Questionnaire Used
Students were assured of their confidentiality and were promised that their names would not appear in the document. A list of the questions asked as part of the survey is given below.
Questionnaire:
Sample group: B Tech students from different branches of sciences across various engineering institutions and MBBS medical students.
Which of the methods engage you personally to learn digitally ?
Individual assignment
Small group (No. 5 students) work
Large group (No. 10 students and more) work
Project-based learning
Which of the digital collaborations enables you to work on a specific task at ease
Two by two (2 member team)
Small group workgroup (No. 5 students) work
Which of the digital approaches motivate you to learn
Whiteboard and pen
PowerPoint presentation
Digital pen and slate
My experience with online learning from home digitally
I am learning at my own pace comfortably
My situational challenges are not suitable
I can learn better with uninterrupted network connectivity
I am distracted with various activities at home, viz. TV, chatting, etc.
Which type of recorded video lecture is more effective for learning ?
delivered by my faculty
delivered by NPTEL
delivered by reputed Overseas Universities
delivered by unknown experts
Which type of quiz is more effective for testing the understanding?
Traditional—pen and paper—MCQ
Traditional—pen and paper—short answers
Online quiz—MCQ
Online quiz—short answers
Student version software downloaded from the internet is useful for learning
Unable to decide
Online teaching – learning takes place effectively because:
Every student can hear the lecture clearly
PPTs are available right in front of every student
Students can ask doubts without much reservation
Students need not walk long distances before reaching the class
Which of the following statements is true of online learning off-campus ?
No one disturbs me during my online learning.
My friend/family member/roommate/neighbor occasionally disturb me
My friend/family member/roommate/neighbor constantly disturb me
At home/place of residence, how many responsibilities do you have?
I don’t have many responsibilities.
I have a moderate amount of responsibilities, but I have sufficient time for online learning.
I have many responsibilities; I don’t have any time left for online learning.
What is your most preferred method for clearing doubts in online learning?
Ask the professor during/after an online lecture
Post the query in a discussion forum of your class and get help from your peers
Go through online material providing an additional explanation.
Which of the following devices do you use for your online learning?
A laptop/desktop computer
A smartphone
Other devices
Outcome of the survey
Students would prefer to work in a group of 5 students to engage personally in digital learning as seen from Fig. 4 .
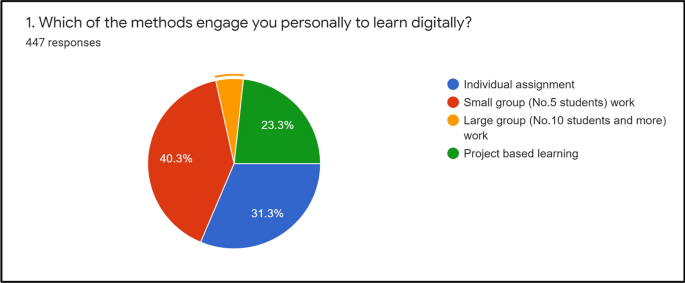
Personal engagement in digital learning
Digital collaboration to enable students to work at ease on a specific task is to allow them to work in small groups of 5 students as seen in Fig. 5 .
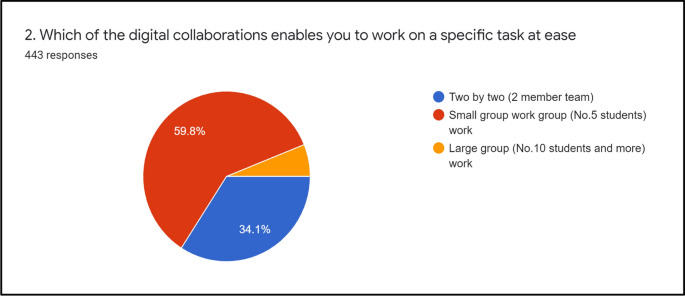
Digital collaboration to enable students to work at ease
Animations are found to be the best digital approach motivating many students to learn as seen in Fig. 6 .
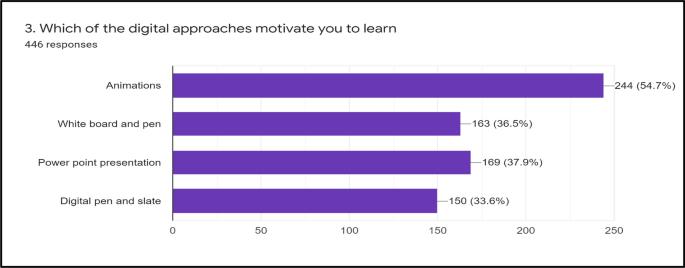
Digital approaches that motivate students to learn
The online learning experience of students is shown in Fig. 7 . The majority of students have said that they can learn at their own pace comfortably through online learning.
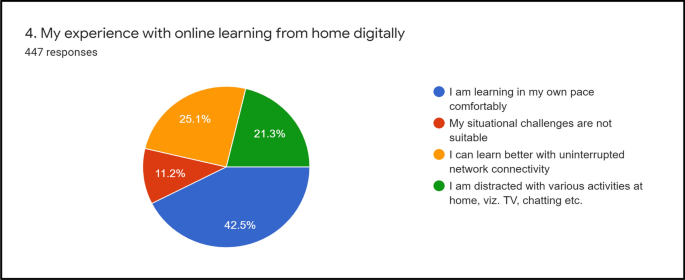
The online learning experience of students
The effectiveness of the recorded video lecture is shown in Fig. 8 . The majority of students agree that the video lectures delivered by his/her faculty teaching the subject help students to learn effectively.
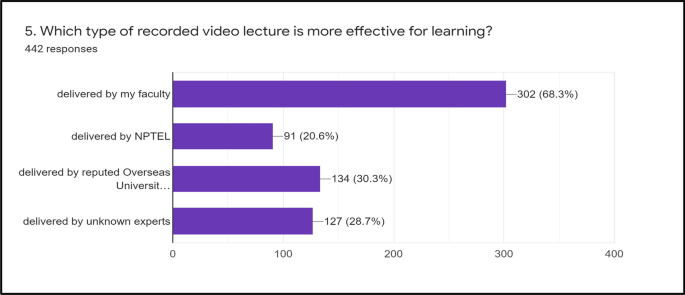
More effective recorded video lecture
Online quiz having multiple-choice questions (MCQ) is preferred by most of the students for testing their understanding of the subject as seen in Fig. 9 .
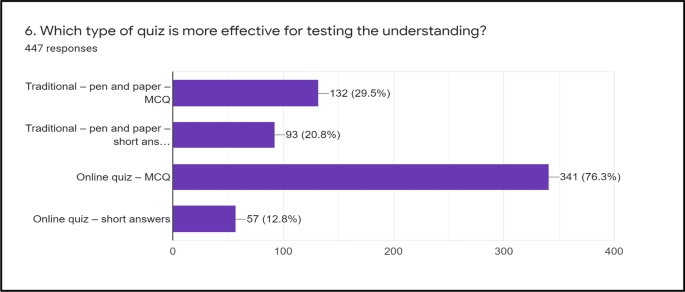
More effective quiz for testing the understanding
The usefulness of the student version of the software downloaded from the internet is shown in Fig. 10 . 45.7% of the students agree that it is useful for learning whereas 45.2% of them are unable to decide. The rest of the students feel that the student version of the software is not useful.
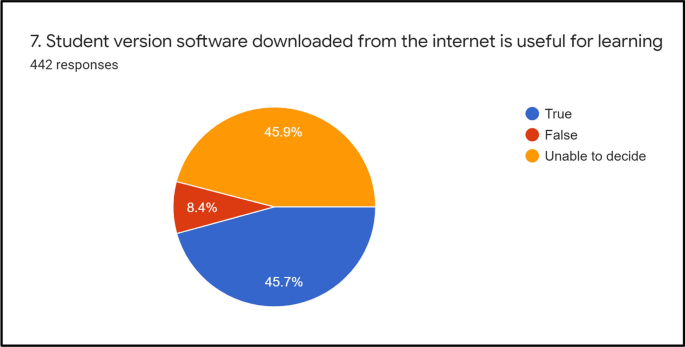
The usefulness of the student version of the software
The reasons for the effectiveness of online teaching–learning are shown in Fig. 11 . The majority of the students, feel that the PPTs are available right in front of every student so that following the lecture makes the learning effective. In universities where a fully flexible credit system (FFCS) is followed, students need to walk long distances for reaching their classrooms. Day Scholars in universities as well as engineering colleges are required to travel a considerable distance before reaching the first-hour class. According to many students, online learning is more effective since walking/traveling is completed eliminated. If the voice of the faculty member is feeble, students sitting in the last few rows of the class would not hear the lecture completely. Some students feel that online learning is more effective since the lecture is reaching every student irrespective of the number of students in a virtual classroom.
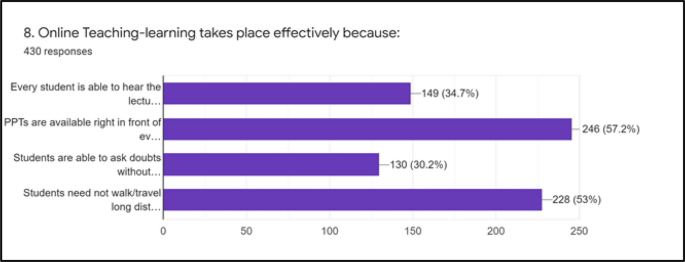
Reasons for the effectiveness of online teaching–learning
50.3% of students agree that they do not have any disturbance during online learning and it is more effective. Many of them feel that occasionally their friends or relatives disturb students during their online learning as shown in Fig. 12 .
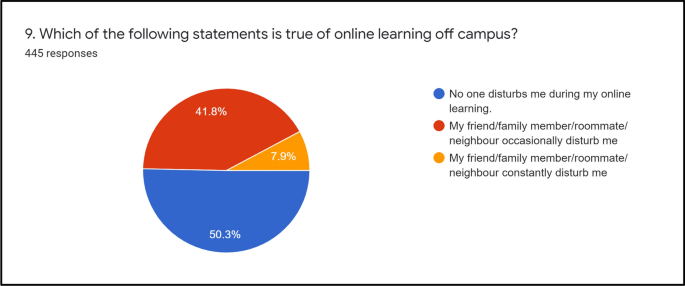
Disturbances during online learning
Figure 13 shows the environment at home for online learning. 76.9% of the respondents stated that they have a moderate amount of responsibilities at home but they have sufficient time for online learning. 16.1% of them have said that they do not have many responsibilities whereas 7% of them claimed that they have many responsibilities at home and they do not have any time left for online learning.
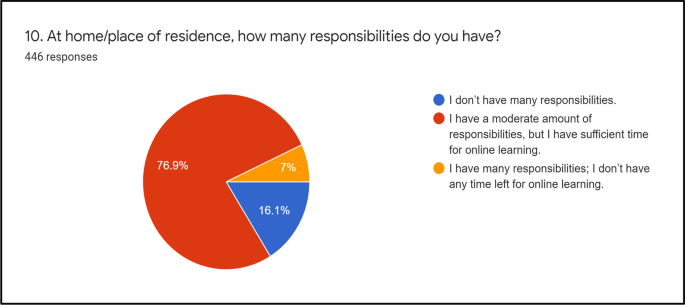
The environment at home for online learning
Figure 14 shows the methods adopted for clearing doubts in online learning. 43.2% of the respondents ask the Professor and get their doubts clarified during online lectures. 25.5% of them post queries in the discussion forum and help from peers. 31.3% of them go through the online materials providing additional explanation and get their doubts clarified.
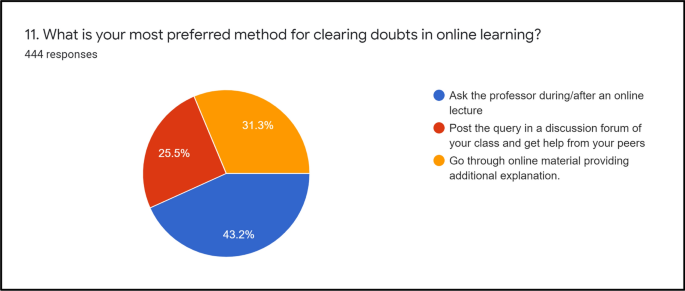
Methods adopted for clearing doubts in online learning
Figure 15 shows the devices used by students for online learning. Most of the students use laptop/desktop computers, many of them use smartphones and very few students use tablets.
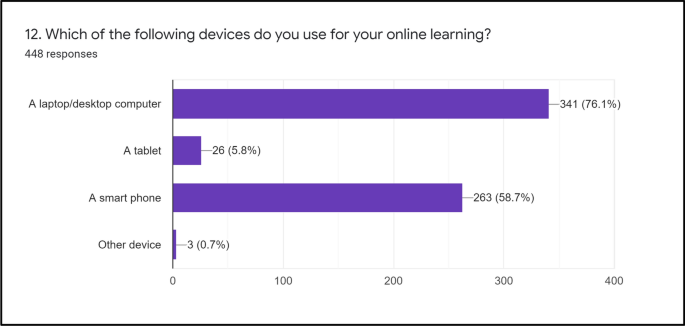
Devices used for online learning
The association between responses 1 and 2 is tested using the chi-square test. The results are presented in Table 2 which shows the observed cell totals, expected cell values, and chi-square statistic for each cell. It is seen that association exists between several responses between questions.
The observed cell values indicate that the highest association is found between responses 1b and 2b since both these responses are related to a small working group having 5 members. The lowest association is found between the responses of 1c and 2a having the lowest observed cell value and expected cell value. The reason for this is response 1c shows the work done by a 10 member team and the response 2a shows a two-member team. The chi-square statistic is 65.6025. The p value is < 0.00001. The result is significant at p < 0.05.
The outcome of a survey on the effectiveness of innovations in online teaching–learning methods for university and college students is presented. About 450 students belonging to VIT Vellore, CMRIT Bangalore, Medical College, Pudukkottai, and engineering colleges have responded to the survey. A questionnaire designed for taking is survey is presented. The chi-square statistic is 65.6025. The p value is < 0.00001. The result is significant at p < 0.05. Associations between several responses of questions exist. The survey undertaken provides an estimate of the effectiveness and pitfalls of online teaching during the online teaching that has been taking place during the pandemic. The study done paves the way for educators to understand the effectiveness of online teaching. It is important to redesign the course delivery in an online mode to make students engaged and the outcome of the survey supports these aforementioned observations.
The outcome of the survey is given below:
A small group of 5 students would help students to have digital collaboration and engage personally in digital learning.
Animations are found to be the best digital approach for effective learning.
Online learning helps students to learn at their own pace comfortably.
Students prefer to learn from video lectures delivered by his/her faculty handling the subject.
Online quiz having multiple-choice questions (MCQ) preferred by students.
Student version software is useful for learning.
Online classes are more effective because they provide PPTs in front of every student, lectures are heard by all students at the sound level of their choice, and walking/travel to reach classes is eliminated.
Students do not have any disturbances or distractions which make learning more effective.
But for a few students, most of the students have no or limited responsibilities at home which provides a good ambiance and a nice environment for effective online learning.
Students can get their doubts clarified during lectures, by posting queries in discussion forums and by referring to online materials provided by the faculty.
World Declaration on Higher Education for the Twenty-first Century: Vision and Action (1998) https://unesdoc.unesco.org/ark:/48223/pf0000141952 . Accessed on 10 December 2020.
S. Cadena-Vela, J.O. Herrera, G. Torres, G. Mejía-Madrid, Innovation in the university, in: Proceedings of the Sixth International Conference on Technological Ecosystems for Enhancing Multiculturality-TEEM’18 (2018), pp. 799–805. https://doi.org/10.1145/3284179.3284308
Demand for online tutors soars, pay increases 28%. Times of India (2020) https://timesofindia.indiatimes.com/city/chennai/demand-for-online-tutors-soars-pay-increases-28/articleshow/77939414.cms . Accessed on 7 December 2020
Can 100 top universities expand e-learning opportunities for 3.7 crore students. Times of India (2020) https://timesofindia.indiatimes.com/home/education/news/can-100-top-universities-expand-e-learning-opportunities-for-3-7-crore-students/articleshow/76032068.cms . Accessed on 9 December 2020.
C. Malemed, Retooling instructional design (2019). https://theelearningcoach.com/elearning2-0/retooling-instructional-design/ accessed on 8 December 2020
C. Wiess, COVID-19 and its impact on learning (2020). https://elearninfo247.com/2020/03/16/covid-19-and-its-impact-on-learning/ . Accessed on 10 December 2020
E. Alqurashi, Technology tools for teaching and learning in real-time, in Educational Technology and Resources for Synchronous Learning in Higher Education (IGI Global, 2019), pp. 255–278
J.M. Mbuva, Examining the effectiveness of online educational technological tools for teaching and learning and the challenges ahead. J. Higher Educ. Theory Pract. 15 (2), 113 (2015)
Google Scholar
S.N.M. Mohamad, M.A.M. Salleh, S. Salam, Factors affecting lecturer’s motivation in using online teaching tools. Procedia Soc. Behav. Sci. 195 , 1778–1784 (2015)
Article Google Scholar
A.T. Hilliard, Global blended learning practices for teaching and learning, leadership and professional development. J. Int. Educ. Res. 11 (3), 179–188 (2015)
M. Moussavi, Y. Amannejad, M. Moshirpour, E. Marasco, L. Behjat, Importance of data analytics for improving teaching and learning methods, in Data Management and Analysis (Springer, Cham, 2020), pp. 91–101
P. Berking, S. Gallagher, Choosing a learning management system, in Advanced Distributed Learning (ADL) Co-Laboratories (2013), pp 40–62
R.J.M. Ventayen, K.L.A. Estira, M.J. De Guzman, C.M. Cabaluna, N.N. Espinosa, Usability evaluation of google classroom: basis for the adaptation of gsuite e-learning platform. Asia Pac. J. Educ. Arts Sci. 5 (1), 47–51 (2018)
B.N. Ilag, Introduction: microsoft teams, in Introducing Microsoft Teams (Apress, Berkeley, CA, 2018), pp. 1–42
A.S. Alqahtani, The use of Edmodo: its impact on learning and students’ attitudes towards it. J. Inf. Technol. Educ. 18 , 319–330 (2019)
J. Uziak, M.T. Oladiran, E. Lorencowicz, K. Becker, Students’ and instructor’s perspectives on the use of Blackboard Platform for delivering an engineering course. Electron. J. E-Learn. 16 (1), 1 (2018)
T. Makarchuk, V. Trofimov, S. Demchenko, Modeling the life cycle of the e-learning course using Moodle Cloud LMS, in Conferences of the Department Informatics , No. 1 (Publishing house Science and Economics Varna, 2019), pp. 62–71
N.L. Davis, M. Gough, L.L. Taylor, Online teaching: advantages, obstacles, and tools for getting it right. J. Teach. Travel Tour. 19 (3), 256–263 (2019)
Download references
Author information
Authors and affiliations.
Department of Computer Science and Engineering, CMR Institute of Technology, Bangalore, 560037, India
Preethi Sheba Hepsiba Darius
School of Mechanical Engineering, Vellore Institute of Technology, Vellore, 632014, India
Edison Gundabattini & Darius Gnanaraj Solomon
You can also search for this author in PubMed Google Scholar
Corresponding author
Correspondence to Darius Gnanaraj Solomon .
Additional information
Publisher's note.
Springer Nature remains neutral with regard to jurisdictional claims in published maps and institutional affiliations.
Rights and permissions
Reprints and permissions
About this article
Darius, P.S.H., Gundabattini, E. & Solomon, D.G. A Survey on the Effectiveness of Online Teaching–Learning Methods for University and College Students. J. Inst. Eng. India Ser. B 102 , 1325–1334 (2021). https://doi.org/10.1007/s40031-021-00581-x
Download citation
Received : 10 August 2020
Accepted : 18 March 2021
Published : 05 April 2021
Issue Date : December 2021
DOI : https://doi.org/10.1007/s40031-021-00581-x
Share this article
Anyone you share the following link with will be able to read this content:
Sorry, a shareable link is not currently available for this article.
Provided by the Springer Nature SharedIt content-sharing initiative
- Learning management
- Learning environment
- Teaching and learning
- Digital learning
- Collaborative learning
- Online learning
- Find a journal
- Publish with us
- Track your research
Systematic research of e-learning platforms for solving challenges faced by Indian engineering students
Asian Association of Open Universities Journal
ISSN : 2414-6994
Article publication date: 2 December 2020
Issue publication date: 21 May 2021
- Supplementary Material
As educational institutes began to address the challenges posed by COVID-19, e-learning came to the foreground as the best bet left. This study is in quest of revealing engineering student's perceptions of the available e-learning platforms, thus surfacing the underlying bottlenecks. Further, it aims at providing solutions that would help enhance the e-learning experience not only in pandemic times but also in the long run.
Design/methodology/approach
This holistic research begins with a comprehensive comparative study about the available e-learning platforms, followed by a primary data analysis through an online survey of 364 engineering students from various colleges and branches. The collected data was analyzed to detect bottlenecks in online learning and suggestions are given for solving some challenges.
On a five-point Likert scale, the available e-learning platforms garnered ratings ranging from 2.81 to 3.46. Google meet was the most preferred platform. However, with a net promoter score (NPS) of 30.36, Microsoft Teams emerged as the most satisfying platform. Technical shortcomings clubbed with psychological and biological factors were found to be taking a toll on e-learning.
Research limitations/implications
This innovative research is based on the perceptions of engineering students hailing majorly from Indian cities, and hence, it may be having educational stream bias and geographical bias. The research could be further extended to cover rural areas and global trends in e-learning.
Originality/value
The research offers a thorough analysis of e-learning platforms, as seen through the lens of engineering students. Furthermore, the analysis does not constrain itself to the technicalities and thus proves to be an all-encompassing one, potent enough to surface critical issues marring the e-learning experience.
- Online teaching
- E-learning platform
- Engineering education
- Open and Distance education
Thakker, S.V. , Parab, J. and Kaisare, S. (2021), "Systematic research of e-learning platforms for solving challenges faced by Indian engineering students", Asian Association of Open Universities Journal , Vol. 16 No. 1, pp. 1-19. https://doi.org/10.1108/AAOUJ-09-2020-0078
Emerald Publishing Limited
Copyright © 2020, Shivangi Viral Thakker, Jayesh Parab and Shubhankar Kaisare
Published in the Asian Association of Open Universities Journal . Published by Emerald Publishing Limited. This article is published under the Creative Commons Attribution (CC BY 4.0) licence. Anyone may reproduce, distribute, translate and create derivative works of this article (for both commercial and non-commercial purposes), subject to full attribution to the original publication and authors. The full terms of this licence may be seen at http://creativecommons.org/licences/by/4.0/legalcode
1. Introduction
Acting as an interface between science and society, engineering surpasses the boundaries of knowledge, analysis and practices the sublime art of organizing forces of technological change. An effective transfer of engineering education stands on the pillars of remembering, understanding, applying, analyzing, evaluating and creating ( Barak, 2013 ).
With the advent of neoliberal market policies, the private sector in engineering and technical education has shifted the focus from philanthropy to profit thereby resulting in a poor quality of education and a mere 25% employability rate ( Choudhury, 2019 ; Gambhir et al. , 2016 ). To add to the existing troubles, COVID-19 has caused education to blow up in the air like an unprecedented display of fireworks. Education systems have no choice but to accept the digital checkmate imposed, ranging from major shutdowns of classroom teaching to spontaneous virtualization ( Xiao, 2018 ). Lack of access to remote learning tools and materials has pushed an alarming number of students not just out of colleges but also out of the system ( Azorín, 2020 ).
COVID-19 has left the post-pandemic education system with two possibilities: a return to traditional education or a transformation towards enhanced education. The key to transformational change will be for systems to focus on their professional capital and find ways to develop teachers' knowledge and skills, support effective collaborative networks that include parents ( McPhee and Söderström, 2012 ). Including educators in the decision- making and communication process ensures easy transformation ( Hollweck and Doucet, 2020 ).
This paper identifies the current perception of available e-learning platforms among engineering students. A comprehensive internal study followed by a thorough analysis helps detect the underlying problems. Further, the paper proposes solutions for these problems to ensure that the transition to e-learning is a smooth endeavor.
2. Literature review
There are recent studies on the demands and needs of engineering education and the exact process of distance learning in the Internet environment. The current effect of the COVID-19 pandemic on the education sector globally and countrywide is studied by few researchers recently. There is a dire need to search for online learning tools available currently and their impact on future e-learning and classroom learning aspects ( Hillier, 2018 ). The following sub-sections give a detailed literature review done on Engineering education requirements, the effect of the pandemic on the education system, various e-learning platforms, and a comparison of online survey methods.
2.1 Engineering education
Fuentes-Del-Burgo and Navarro-Astor (2016) explained in brief how the concepts of “episteme,” “techne” and “phronesis” given by Aristotle are associated with engineering education. Though mainly concerned with Spanish building engineers, it almost gives a worldwide perspective on how various educational factors play an important role in building good engineers and various suggestions to improve them. Barak (2013) discusses how the United States has implemented the three principles of K-12 education and how it can be utilized in other countries to have an overall development of engineering students. The difference between technology and engineering; integration of Bloom's Taxonomy and K-12 education; and the importance of cognitive education on the improvement of engineering students have also been explained.
Choudhury (2019) , surveyed 1178 undergraduate engineering students in Delhi to understand student's perceptions of various criteria of teaching methods used, skills acquired by the students, and involvement of students. This does provide a brief overview of the current situation of engineering education in India and how the current teaching methods can be improved. Upadhayay and Vrat (2017) , have analyzed the growth of India's technical education from the system's dynamic perspective followed by a comparison with the Gartner Hype cycle. The concept of the Boomerang effect has been introduced in this paper to compare it with the current movement of Indian technical education along with concerns over the quality of technical education currently in India. Gambhir et al. (2016) echo the same concerns and have developed a methodology to build a quality model for the integration of various factors in a technical institute.
2.2 COVID-19 pandemic's effect on education
UNESCO report (2020) gives some interesting numbers on how much the pandemic has affected the education system globally. The study indicates that 60.9% of the enrolled student population has been affected with over 1 billion student learners affected and 107 nationwide closures (as of July 2020). The study shows that this pandemic has crippled the education system all over the world further emphasizing the need for new measures to be taken to handle this inopportune time.
Carter et al. (2020) have discussed the effect of COVID-19 on classroom education and how e-learning would need to come to the forefront. The concept of self-regulated learning has been introduced along with its components and ways to integrate it with online learning. Hollweck and Doucet (2020) have also discussed the effects of COVID-19 on education, but they have created an interesting analogy with supernova. They have compared COVID-19 with a supernova in terms that after a supernova event everything changes for the better and the status quo are broken which was unraveling before. Similarly, the paper takes this pandemic as an opportunity to disrupt the status quo and build the education system in a much better way.
Further, Fullan (2020) reiterates that evolution could have wonderful things in store for us - but only if we do our part to shape it and thus hints to take this pandemic positively. Izumi et al. (2020) have similarly gone about discussing the issue of COVID-19 on the transition from classroom learning to online learning and the financial strains of the same. They have surveyed to understand the preparedness for such a transition and the available infrastructure. This has given great insight into the current capacity of the world to transform itself from classroom teaching to online learning.
Lall and Singh (2020) have discussed the impact of COVID-19 on India, emphasizing the importance of a smooth transition towards online learning. A survey to gauge the current perception towards online learning, drawbacks of it, and also the preferred mode of learning was done, which gives a great insight into what factors affect the success of online learning. Similar survey-based research has been done by Basilaia and Kvavadze (2020) with great emphasis on the transition to online learning in Georgia. A brief discussion on the social impact of this immediate transition from classroom learning to online learning has been done by Kufi et al. (2020) along with the importance of free online courses and how resource use should be done in schools to tackle this situation.
2.3 E-learning
Harper et al. (2004) explain distance learning, the advancement of the same along with the impact of government involvement on distance learning. The authors embellish the current details with information on the role of participants in the success of distance learning, change in the organizational structure required for the success of distance learning, and the pros and cons of it in long term perspectives. Along similar lines, Au et al. (2018) discuss the success factors for students learning online such as time management, online examination conduction and flexibility. Jones et al. (2014) discuss how the introduction of technology affects the temporal experience of the learner and states the importance of time flexibility which must be introduced in online learning. This, in a direct sense, gives an understanding of how synchronous and asynchronous ways of teaching can affect the learning capacity of a student. Though Fang et al. (2019) discuss the evolution of MOOCs from 2009 to 2018 in language learning through literature study, they also reiterate similar points and their results show that online learning courses and platforms have raised the time and space for learning, which has made it flexible.
Veletsianos and Houlden (2019) have discussed various themes associated with distance learning. These themes have been closely associated with flexibility and further discuss various approaches towards it from a pedagogical, liberal, temporal and cultural point of view for the past 40 years of distance learning. A similar analysis of the flexibility of transactional education has been done by Paul et al. (2015) . Even Naidu (2019) has given a brief overview of how open learning, flexible learning, and e-learning are very dynamic with narrative changing at any given point of time. Its psychological impact on students also has been reviewed along with various advantages and disadvantages of it. A similar yet a very unique study also has been done by Estacio and Raga (2017) , where they have used machine learning models and correlated the quantitative data available from Moodle to the online learning behavior of students, where the grades obtained are used as a determining medium.
Major et al. (2014) explained the various pedagogical approaches which can be used in distance learning like constructivist, problem-based learning, holistic approach, teamwork. They further provided an overview of how the transition to the online setting must be done along with the technological challenges associated with it. Joanna Rabiega-Wiśniewska (2020) conducted a case study on the current perception of e-learning at Maria Grzegorzewska University, Poland. The study does indicate a neutral stance over liking of the immediate change in learning method, but with 91% of students having a stable Internet connection; it's a good sign nonetheless. A brief understanding of the type of scaling system to be used in such surveys has been explained, which shall be imbibed in this paper to enhance and avoid response bias and to evaluate NPS. Marengo and Marengo (2005) have discussed in brief the actual organizational structure and proper education requirements through Kirkpatrick's taxonomy which needs to be imbibed in e-learning. The concept of blended learning also has been introduced in this paper with its pros and cons in economic terms. The study has effectively discussed various costs involved in e-learning along with the benefits gained. These costs have played an important role in deciding parametric questions to be asked to the students for correct evaluation of the current perception of e-learning tools among engineering students.
2.3.1 Comparison of online platforms for e-learning
A comprehensive comparative study becomes crucial to determine the publicly known best available tools as floating a survey on the unpopular tools may hamper the survey outcome significantly. To aid this comparison, identification of parameters to be compared must be identified. Agrawal et al. (2016) discuss in brief how a parametric survey needs to be conducted and the importance of information quality, service quality, system quality in the success of e-learning. Further, James-Gordon et al. (2003) explain the importance of security features required for e-learning to not be a hindrance for people and an understanding of how market demand or the popularity of a learning platform affects its overall success. Wong (2015) mentions the importance of flexibility of the platforms provided for MOOCs and this flexibility will play a big role in these e-learning platforms as well. Keeping these factors in mind, various parameters such as features provided, platforms which the tool supports, typical customers the tool attracts, customer support provision, price of the tool, overall customer perception about the tool, third party integration, the scope of the tool have been devised for comparison.
2.3.2 Comparison of survey methods
Surveys can be conducted in two ways: online and offline. Offline surveys are generally avoided as they have a localized outreach and getting timely responses is a big task. Online surveys break the barriers of distance and have a hassle-free response collection process. Online survey forms have an easy build coupled with cost-effectiveness. There are various online survey platforms available and a proper comparison must be done among them to find out an apt option for the survey.
To accurately garner student perceptions, the online survey tool should be selected with keen consideration. Along with cost-effectiveness, this tool should bring the magical combination of accuracy and customization. To narrow down on the best survey tool, it was necessary to adopt a comprehensive approach that compared these tools based on the parameters like permissible number of questions, permissible number of responses, data export availability and options, number of free surveys allowed, customization and its scale. Table 1 displays the permissible values with the free version of the tool along with the cost to upgrade to the premium version.
3. Methodology
3.1 data collection tool.
A comprehensive study of seven e-learning platforms (Zoom, Google Meet, Microsoft Teams, GoToWebinar, Zoho Meeting, Adobe Connect and GoToMeeting) was performed to gauge the consistency and performance of platforms based on features, security, customer support and third-party integrations. This study acted as predictive analysis to understand what could be the student's standpoint and then understand how much it varies. Further, a comparative analysis was adopted to find the most suitable online survey platform. A survey-based approach was adopted to gauge the perception of engineering students on the available e-learning tools. Through the review done above, Google Forms was finalized as the survey platform.
3.2 Questionnaire for survey
For drawing valuable insights, it is vital to analyze the most critical parameters. A respondent friendly survey was constructed on Google Forms wherein the respondents had to rate the e-learning platforms based on the parameters like video quality, audio quality, privacy/security, multi-device support, user-friendliness of the interface, screen sharing, chat features, host's control and quality of meeting recording. Figure 1 shows the flow of the questionnaire.
The questionnaire ratings were taken on a five-point Likert scale developed by Rensis Likert ( Reichheld, 2003 ) as this type of scale is used in attitude research projects ( Joanna Rabiega-Wiśniewska, 2020 ). An odd-numbered Likert scale was used to avoid emotion bias and to provide an option for indecision, negativity, and positivity ( Croasmunand Ostrom, 2011 ).
3.3 Distribution channel
A robust distribution channel ensures a greater number of responses from students, spread across various engineering colleges and branches. To achieve the same, the survey form was circulated through platforms like WhatsApp, Gmail, Instagram, LinkedIn and personal calling.
These tools and methods helped in collecting responses from students spread across 12 branches and 49 colleges. The responses generated from surveys generally depict a bell curve. In such cases, if the sample size or the number of respondents is very large, the confidence interval narrows down and errors decrease. Error reduction is good, but the confidence interval should not decrease to a point where it starts showing that negligible people have positive responses. Now, with a decrease in sample size, the confidence interval increases but the error also increases. Thus, selecting the number of respondents is a double-edged sword as a perfect balance has to be struck among confidence interval and error. Hence, an optimal range of 350–400 responses was chosen and the survey form was closed on receiving 364 responses.
4. Data analysis
4.1 respondents profiles.
A total of 364 responses were collected from 49 colleges across India. It was ensured that all the respondents have extensively used the platforms voted by them for at least a month. This data needs to be sorted into various categories to identify trends and gain insights from them. These responses were analyzed branch–wise and year-wise to check whether there is slight response bias, to identify trends, and to draw insights based on the same (see Figures 2 and 3 ).
The Mechanical branch accounted for 44.23% of responses and had the maximum number of responses. Computer Science and Engineering (CSE) branch was second to the Mechanical branch and held 23.07% of responses. Information Technology (IT) branch and Electronics and Telecommunication branch (EXTC) had an almost similar number of respondents and contributed 9.89 and 9.07% of responses respectively. This does indicate that the perception generated was slightly biased towards the requirements of Mechanical Engineering students, but on a closer look at the data, the platforms selected and the ratings given by other branches were on similar lines as the Mechanical branch. Last year students of engineering submitted the maximum responses indicating that maximum awareness, for now, has been limited to certain students only with the further scope for improvement. As first-year students had just been admitted to their respective colleges when the survey was conducted, they were not exposed to the e-learning environment thus resulting in a fewer number of responses from the first year.
4.2 Net promoter score of platforms
The data, collected from the survey responses of 364 students, was analyzed firstly by segregating and making a college wise distribution of responses to check the demographic reach of the survey. A wider demographic reach ensures a varied perspective thereby eliminating regional bias. Branch wise distribution of responses was also plotted to check for singular branch bias for a particular online learning tool. A similar approach was used to check singular year bias by plotting the year-wise distribution of responses. This data was crucial in understanding how the perception is influenced by branch and year of study.
Awareness of platforms was analyzed to check the popularity or reach of each platform irrespective of its liking or disliking. The average ratings of each platform based on the nine parameterized survey outcomes provided insights as to which platform has been consistent in providing all the features satisfactorily to its target audience.
Further, an NPS for each online learning platform was evaluated. NPS is a loyalty index introduced by Frederick F. Reichheld in 2003, primarily used to evaluate how much a product has been liked by the customers and can be used for further product referrals. Promoters are individuals who strongly recommend the product and are convinced of the parameter, thus rating it 4 or 5. Detractors are individuals who are unsatisfied with the product or some parameter of it, thus rating it 1 or 2. Individuals, who give a rating of 3, lie between these two categories and are called passives. NPS for a particular platform, on a 5-point Likert scale, is evaluated as: NPS = ( Number of promoters − Number of detractors ) * 100 Number of respondents who have used that platform
(−100 to 0): Needs improvement
(0–30): Good
(30–70): Very good
(70–100): Excellent
The above ranges helped to boil down the overall user sentiment into a single quantifiable value and classify the platform on the same. Finally, the preference percentage was plotted for each platform to understand the current perception and to recognize which platform currently is ruling the roost in the online learning world among engineering students.
5. Results and interpretations
5.1 internal study outcomes.
The internal study focused on performing a comparative analysis of the available e-learning platforms. By comparing these platforms based on the offered features, integrations, reviews, and pricing, the study aimed at finding a platform that provided a complete package to its users at a reasonable subscription cost. Table 2 provides an overview of the internal study outcome.
From Table 2 , it is evident that Zoom and Microsoft Teams are the best platforms with 44 and 67 features available respectively. A closer introspection does reveal a shortcoming of Microsoft Teams over Zoom that is the absence of an attendance management system. In terms of security aspects, Google Meet, GoToMeeting, and GoToWebinar do not have access control and an activity dashboard thereby making these platforms weak. The only salvation for Google Meet is that it has a better API. A bird's eye view indicates that Google Meets supports all the platforms available to people, whereas Zoom and Microsoft Teams do not support the Windows phone app. Microsoft Teams does not attract freelancers and does not provide customer support over the phone. Other platforms satisfactorily provide this, thereby leaving Microsoft Teams with a massive scope of improvement in this aspect. Google Meet is the best in this aspect followed closely by Zoom.
As visible from both Table 3 , Microsoft Teams is the most feasible platform whereas GoToWebinar is the highest priced platform. Zoom and Google Meet are also priced affordably but Microsoft Teams wins the battle in pricing. Table 4 shows that the rankings of all the platforms are not too bad, all crossing 4 stars, but the number of reviews given for Zoom and Google Meet shows that they are the most popular platforms among the others. Zoho Meeting though not as popular, has been highly ranked by those who have used it. Zoom and Google Meet are closely followed by Microsoft Teams which ranks third in popularity. The pricing of GoToWebinar and Adobe Connect does surely reflect their lack of popularity amongst general people. Table 4 does show that Zoom is the platform with the highest number of Third-Party Integrations amounting to whopping 170 integrations. It is closely followed by Microsoft Teams with 154 Third-Party integrations. Other platforms need improvements in this aspect with 86 integrations from GoToWebinar and then an equally shocking drop to 15 integrations from Google Meet. This does show that Third-Party Integrations are surely a challenge for these platforms, Zoom and Microsoft Teams being the only exceptions.
These results show that Zoom has the best balance among features, overview, pricing, popularity, third-party integrations as compared to other platforms. Though just by score value, Microsoft Teams should have followed as the next best; the graph shows high inconsistencies in these parameters. This indicates that due notice over certain parameters has not been given in Microsoft Teams. This makes Google Meet slightly more favorable over Microsoft Teams. Adobe Connect and Zoho Meeting do not make a case to prove their chance in the education sector with even GoToWebinar becoming a rare case of use due to its high price (see Figures 4–6 ).
5.2 Awareness of platforms
Zoom and Google Meets are the most publicly known platforms with an astounding awareness percentage of 86 and 81.6% respectively. Adobe connect and Zoho Meeting is the least known ones and the perception matches the internal study where the higher pricing and fewer features value seen in the graph of these tools had made them possibly least known ones. Thus, there are increased chances of Google Meets and Zoom ruling the roost in the online education industry as these are the platforms mostly used (see Table 5 ).
5.3 Comparison of platforms based on survey results
These ratings indicate that Microsoft Teams is the best platform followed by Google Meets, Zoom, GoToWebinar, GoToMeeting, Zoho Meeting and Adobe Connect. Microsoft Teams had a maximum rating of 3.46 closely followed by Google Meets with a rating of 3.45. No platform had an average rating beyond 4. These passive ratings indicate an even greater perspective over the audience being a low tolerant one with a keen eye towards perfection. Considering this it can be predicted that the NPS would not be very high and in a rare case, it would breach the barrier of 30 (see Table 6 ).
5.4 Net promoter score
Microsoft Teams has the maximum NPS of 30.36, overcoming the Good band and entering the Very Good band. Adobe Connect has the worst NPS of −50 indicating that it needs improvement. Also, the NPS of almost all platforms is closely ranged showing that the competition is very stiff (see Figures 7 and 8 ).
5.5 Preference of platforms
A majority of the audience has given preference to Google Meets followed by Zoom and Microsoft Teams respectively. Here, though the quality of Microsoft Teams is much higher than that of both Zoom and Google Meets, lack of awareness of Microsoft Teams has resulted in it being ruled out of favor. There is also a curious case of Zoom, but a closer introspection shows that concerns over privacy and security of the platform have caused it to be not as favorable as Google Meet, though being superior in other features. Zoho Meetings and Adobe Connect have not made it through as far as students' preference is concerned.
6. Challenges and solutions
The survey respondents highlighted several shortcomings which were barring them from having an effective e-learning experience. Along with these shortcomings, the respondents expressed their desire for certain additional features which would greatly boost the e-learning experience.
6.1 Security concerns
A high number of students are attending digital classrooms and it has become easier for cybercriminals to hijack meetings. Events of video hijacking by uninvited parties to disrupt the usual proceedings have been on the rise since the global quarantine began. Spreading hateful comments, racist and obscene content on these platforms has given rise to a new kind of Internet trolling. Further, unwarranted logins to the enterprise cloud architecture have resulted in immense data breaches.
To greatly reduce such malpractices, the responsibility lies on the shoulders of the platform, the host, and the attendees. Platforms have been striving to enhance their security measures and have also created robust privacy policies. Hosts should secure meetings with a passcode and use private distribution channels to invite participants. Also, disabling features like join before host and participant screen sharing would provide a greater immunity against hijacking. Attendees should refrain from sharing the meeting details on public platforms and avoid clicking on any malicious links.
6.2 Online engagement concerns
6.2.1 proctor mode.
After spending huge amounts on these e-learning platforms, educational institutions do not prefer using separate applications designed specifically for proctoring. This leaves them with two broad options which are to either conduct examinations without proctoring or to use the same e-learning platform for proctoring. The former invites a large number of unfair practices and thus is unjust for diligent students ( Nguyen, 2015 ). The latter requires all the participants to switch on their video which consumes a great amount of bandwidth resulting in lags. Even if incoming videos are disabled, the bandwidth problem persists with the host which leads to difficulties in proctoring ( Gillett-Swan, 2017 ; Dhawan, 2020 ). Thus, the introduction of a specialized proctor mode on these platforms is a desire of many students.
6.2.2 Lecture mode
Survey respondents reported that mischiefs by certain students (e.g.: disturbing annotations on the screen, muting the instructor, etc.) disrupt the flow of the lectures. Though the platforms have provided certain host-specific features, the spontaneous virtualization of education resulted in the instructors getting insufficient time to adapt themselves to these features. This issue has also been highlighted by Moradimokhles and Hwang (2020) as a limitation of online learning. As a result, a majority of them are not aware of or are unable to use all the features they have at their disposal. Even before the pandemic hit, this adaptability was an issue that was highlighted by Parkes et al. , (2014) . As a result, a majority of them are not aware of or are unable to use all the features they have at their disposal. Bringing all these features under a single button of lecture mode would thus help in conducting lectures smoothly without mischiefs.
6.3 Introduction of new features
In addition to the existing features, the respondents expressed the need for certain features. A large number of instructors annotate the content to provide a lucid explanation. However, the students can download the file without any annotations. An option to download it with annotations would ensure a quicker grasping of the concept when students revisit that concept. An inbuilt notepad that can be opened along with the lecture content, in a split-screen mode, would make the notes taking process hassle-free. Live polling would facilitate the instructor in a variety of ways. Similarly, live quizzes with leader-boards would not only add an element of fun to the learning but would also be an indicator of how much the students have learned ( Huang et al. (2019a , b) , Seaborn and Fels (2015) ). The platforms should further be compatible with augmented reality and virtual reality as these would greatly increase the level of understanding ( Bower, 2017 ; El Kabtane et al. , 2019 ; Uhomoibhi et al. , 2019 ). The presence of a virtual user guide along with a chat box would help in resolving the basic issues faced by a great number of users. The ability to rewind live lectures, like YouTube Live, would help students who have missed out on certain important parts of a lecture.
7. Conclusion
Almost all the platforms are sufficient for learning for the time being but have shortcomings that need to be improved to adapt to this fast-changing education sector. There is a large amount of concern over the video and audio quality of all the platforms and the students feel that the platforms are not updated as per current requirements. As per this research study, Google Meet is the best platform among students followed by Zoom and Microsoft Teams respectively, even though NPS indicates Microsoft Teams is the best. If Microsoft teams can improve its social presence, it can prove to be a strong competitor for both Zoom and Google Meet.
The available online learning tools are not the best means to study a holistic curriculum of theory and practical combined. These tools will have to be more adaptable, more technically friendly for the audience to achieve high effectiveness. Along with online learning, methods to improve motivation to study through these means need to be developed ( Roberts et al. , 2018 ). Available e-learning tools serve the basic purpose but integrations of these platforms with other platforms must be improved to give a wider, more enriching experience. Keeping these points in mind, it would not be wrong to conclude that currently, online learning is the best bet left to counter this unprecedented situation in India, but infrastructure development for such platforms needs to be enhanced to consider this method of learning completely fruitful.
8. Limitations and future scope
This research is based on the perceptions of engineering students hailing majorly from Indian cities and is thus subject to educational stream bias and geographical bias. Curbing the educational stream bias by incorporating respondents from other streams could help in understanding the shortcomings on a broader level. Expanding the respondent base by breaking the geographical barriers would help in further understanding the overall access to technology and its implications on the e-learning experience. Furthermore, the overall experience, perceptions, and awareness of students about these platforms are subject to the instructor's ICT proficiency along with the availability and compatibility with the existing infrastructure in the institutions. Considering the unequal penetration of technology across the various socioeconomic classes, an equal amount of focus should be laid on bridging these gaps ( Zhao, 2016 ). As the COVID-19 pandemic is heralding the end of a largely obsolete educational system, developing solutions on a global level while keeping in mind the issues on local levels would bolster the possibility of redesigning a better education system on the bedrocks of equity, excellence and student well-being.
Questionnaire flow chart
Respondents' profiles
Overall aspect comparison of platforms (Individual platform level comparison is given in Appendix )
Platform awareness among respondents
Average survey ratings (For individual platform level comparison, see Appendix )
Platform-based average survey ratings
Comparison of platforms based on NPS
Survey respondents' preference for platforms
Comparison of survey platforms based on features
Overall comparison of platforms
Feature-based comparison of platforms
Overall survey ratings
The appendix file are available online for this article.
Agrawal , V. , Agrawal , A. and Agarwal , S. ( 2016 ), “ Assessment of factors for e-learning: an empirical investigation ”, Industrial and Commercial Training , Vol. 48 No. 8 , pp. 409 - 415 .
Au , O.T.S. , Li , K. and Wong , T.M. ( 2018 ), “ Student persistence in open and distance learning: success factors and challenges ”, Asian Association of Open Universities Journal , Vol. 13 No. 2 , pp. 191 - 202 .
Azorín , C. ( 2020 ), “ Beyond COVID-19 supernova. Is another education coming? ”, Journal of Professional Capital and Community . doi: 10.1108/JPCC-05-2020-0019 .
Barak , M. ( 2013 ), “ Teaching engineering and technology: cognitive, knowledge and problem-solving taxonomies ”, Journal of Engineering, Design and Technology , Vol. 11 No. 3 , pp. 316 - 333 .
Basilaia , G. and Kvavadze , D. ( 2020 ), “ Transition to online education in schools during a SARS-CoV-2 coronavirus (COVID-19) pandemic in Georgia ”, Pedagogical Research , Vol. 5 No. 4 , pp. 1 - 9 .
Bower , M. ( 2017 ), “ Designing for learning using virtual worlds ”, Design of Technology-Enhanced Learning , Emerald Publishing Limited , pp. 305 - 364 .
Carter , R.A. Jr , Rice , M. , Yang , S. and Jackson , H.A. ( 2020 ), “ Self-regulated learning in online learning environments: strategies for remote learning ”, Information and Learning Sciences , Vol. 121 No. 5 , pp. 321 - 329 .
Choudhury , P.K. ( 2019 ), “ Student assessment of the quality of engineering education in India: evidence from a field survey ”, Quality Assurance in Education , Vol. 27 No. 1 , pp. 103 - 126 .
Croasmun , J.T. and Ostrom , L. ( 2011 ), “ Using likert-type scales in the social sciences ”, Journal of Adult Education , Vol. 40 No. 1 , pp. 19 - 22 .
Dhawan , S. ( 2020 ), “ Online learning: a panacea in the time of COVID-19 crisis ”, Journal of Education Technology Systems , Vol. 49 No. 1 , pp. 5 - 22 .
El Kabtane , H. , El Adnani , M. , Sadgal , M. and Mourdi , Y. ( 2019 ), “ Augmented reality-based approach for interactivity in MOOCs ”, International Journal of Web Information Systems , Vol. 15 No. 2 , pp. 134 - 154 .
Estacio , R.R. and Raga , R.C. Jr ( 2017 ), “ Analyzing students online learning behavior in blended courses using Moodle ”, Asian Association of Open Universities Journal , Vol. 12 No. 1 , pp. 52 - 68 .
Fang , J.W. , Hwang , G.J. and Chang , C.Y. ( 2019 ), “ Advancement and the foci of investigation of MOOCs and open online courses for language learning: a review of journal publications from 2009 to 2018 ”, Interactive Learning Environments , pp. 1 - 19 , doi: 10.1080/10494820.2019.1703011 .
Fuentes-Del-Burgo , J. and Navarro-Astor , E. ( 2016 ), “ What is engineering education for? Listening to the voices of some Spanish building engineers ”, Journal of Engineering, Design and Technology , Vol. 14 No. 4 , pp. 897 - 919 .
Fullan , M. ( 2020 ), “ The battle of the century: catastrophe versus evolutionary nirvana ”, Australian Education Leader , Vol. 42 No. 1 , pp. 8 - 10 .
Gambhir , V. , Wadhwa , N.C. and Grover , S. ( 2016 ), “ Quality concerns in technical education in India ”, Quality Assurance in Education , Vol. 24 No. 1 , pp. 2 - 25 .
Gillett-Swan , J. ( 2017 ), “ The challenges of online learning: supporting and engaging the isolated learner ”, Journal of Learning Design , Vol. 10 No. 1 , pp. 20 - 30 .
Harper , K.C. , Chen , K. and Yen , D.C. ( 2004 ), “ Distance learning, virtual classrooms, and teaching pedagogy in the Internet environment ”, Technology in Society , Vol. 26 No. 4 , pp. 585 - 598 .
Hillier , M. ( 2018 ), “ Bridging the digital divide with off-line e-learning ”, Distance Education , Vol. 39 No. 1 , pp. 110 - 12 .
Hollweck , T. and Doucet , A. ( 2020 ), “ Pracademics in the pandemic: pedagogies and professionalism ”, Journal of Professional Capital and Community , pp. 1 - 11 .
Huang , B. , Hew , K.F. and Lo , C.K. ( 2019a ), “ Investigating the effects of gamification-enhanced flipped learning on undergraduate students' behavioral and cognitive engagement ”, Interactive Learning Environments , Vol. 27 No. 8 , pp. 1106 - 1126 .
Huang , B. , Hwang , G.J. , Hew , K.F. and Warning , P. ( 2019b ), “ Effects of gamification on students' online interactive patterns and peer-feedback ”, Distance Education , Vol. 40 No. 3 , pp. 350 - 379 .
Izumi , T. , Sukhwani , V. , Surjan , A. and Shaw , R. ( 2020 ), “ Managing and responding to pandemics in higher educational institutions: initial learning from COVID-19 ”, International Journal of Disaster Resilience in the Built Environment , pp. 1 - 16 .
James-Gordon , Y. , Young , A. and Bal , J. ( 2003 ), “ External environmental forces affecting e-learning providers ”, Marketing Intelligence and Planning , Vol. 21 No. 3 , pp. 168 - 172 .
Joanna Rabiega-Wiśniewska ( 2020 ), “ When students faced online learning in coronavirus times: a case study ”, The International Virtual Conference on Education , Teaching and Learning , pp. 1 - 11 .
Jones , P. , Skinner , H. and Leeds , B. ( 2014 ), “ Temporal experiences of e-learning by distance learners ”, Education + Training , Vol. 56 No. 3 , pp. 179 - 189 .
Kufi , E.F. , Negassa , T. , Melaku , R. and Mergo , R. ( 2020 ), “ Impact of corona pandemic on educational undertakings and possible breakthrough mechanisms ”, BizEcons Quarterly , Vol. 11 No. 1 , pp. 3 - 14 .
Lall , S. and Singh , N. ( 2020 ), “ COVID-19: unmasking the new face of education ”, International Journal of Research in Pharmaceutical Sciences (SPL) , Vol. 11 SPL1 , pp. 48 - 53 .
Major , A.E. , Chaudhury , S.R. , Gilbertson , B.M. and King , D.T. Jr ( 2014 ), “ An integrated science course moves online: four concurrent perspectives ”, Journal of Applied Research in Higher Education , Vol. 6 No. 2 , pp. 248 - 268 .
Marengo , A. and Marengo , V. ( 2005 ), “ Measuring the economic benefits of e-learning: a proposal for a new index for academic environments ”, Journal of Information Technology Education , Vol. 4 No. 1 , pp. 329 - 346 .
McPhee , I. and Söderström , T. ( 2012 ), “ Distance, online and campus higher education: reflections on learning outcomes ”, Campus-Wide Information Systems , Vol. 29 No. 3 , pp. 144 - 155 .
Moradimokhles , H. and Hwang , G.J. ( 2020 ), “ The effect of online vs. blended learning in developing English language skills by nursing student: an experimental study ”, Interactive Learning Environments , Vol. 28 , pp. 1 - 10 .
Naidu , S. ( 2019 ), “ The changing narratives of open, flexible and online learning ”, Distance Education , Vol. 40 No. 2 , pp. 149 - 152 .
Nguyen , T. ( 2015 ), “ The effectiveness of online learning: beyond No significant difference and future horizons ”, MERLOT Journal of Online Learning and Teaching , Vol. 11 No. 2 , pp. 309 - 319 .
Parkes , M. , Stein , S. and Reading , C. ( 2014 ), “ Student preparedness for university e-learning environments ”, The Internet and Higher Education , Vol. 25 , pp. 1 - 10 .
Paul , R.C. , Swart , W. , Zhang , A.M. and MacLeod , K.R. ( 2015 ), “ Revisiting Zhang's scale of transactional distance: refinement and validation using structural equation modeling ”, Distance Education , Vol. 36 No. 3 , pp. 364 - 382 .
Reichheld , F.F. ( 2003 ), “ The one number you need to grow ”, Harvard Business Review , Vol. 81 No. 12 , pp. 46 - 55 .
Roberts , J. , Kigotho , M. and Stagg , A. ( 2018 ), “ Expanding horizons in open and distance learning ”, Distance Education , Vol. 39 No. 1 , pp. 1 - 3 .
Seaborn , K. and Fels , D.I. ( 2015 ), “ Gamification in theory and action: a survey ”, International Journal of Human-Computer Studies , Vol. 74 , pp. 14 - 31 .
Uhomoibhi , J. , Onime , C. and Wang , H. ( 2019 ), “ A study of developments and applications of mixed reality cubicles and their impact on learning ”, International Journal of Information and Learning Technology , Vol. 37 , pp. 15 - 31 .
UNESCO ( 2020 ), COVID-19 Impact on Education , UNESCO , available at: https://en.unesco.org/covid19/educationresponse .
Upadhayay , L. and Vrat , P. ( 2017 ), “ Policy boomerang in technical education: a system dynamics perspective ”, Journal of Advances in Management Research , Vol. 14 No. 2 , pp. 143 - 161 .
Veletsianos , G. and Houlden , S. ( 2019 ), “ An analysis of flexible learning and flexibility over the last 40 years of distance education ”, Distance Education , Vol. 40 No. 4 , pp. 454 - 468 .
Wong , B.T.M. ( 2015 ), “ Pedagogic orientations of MOOC platforms: influence on course delivery ”, Asian Association of Open Universities Journal , Vol. 10 No. 2 , pp. 49 - 66 .
Xiao , J. ( 2018 ), “ On the margins or at the center? Distance education in higher education ”, Distance Education , Vol. 39 No. 2 , pp. 259 - 274 .
Zhao , Y. ( 2016 ), “ From deficiency to strength: shifting the mindset about education inequality ”, Journal of Social Issues , Vol. 72 No. 4 , pp. 716 - 735 .
Acknowledgements
The authors are grateful to all the students of various engineering colleges for responding to the survey and using the online platforms for a month as a prerequisite for the survey.
Corresponding author
Supplementary materials.
AAOUJ-09-2020-0078_suppl2.docx (44 KB) AAOUJ-09-2020-0078_suppl1.docx (42 KB) AAOUJ-09-2020-0078_suppl3.docx (27 KB)
Related articles
We’re listening — tell us what you think, something didn’t work….
Report bugs here
All feedback is valuable
Please share your general feedback
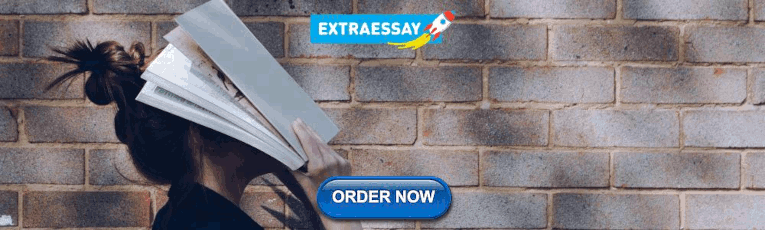
Join us on our journey
Platform update page.
Visit emeraldpublishing.com/platformupdate to discover the latest news and updates
Questions & More Information
Answers to the most commonly asked questions here
Thank you for visiting nature.com. You are using a browser version with limited support for CSS. To obtain the best experience, we recommend you use a more up to date browser (or turn off compatibility mode in Internet Explorer). In the meantime, to ensure continued support, we are displaying the site without styles and JavaScript.
- View all journals
- My Account Login
- Explore content
- About the journal
- Publish with us
- Sign up for alerts
- Open access
- Published: 11 March 2024
Twitter users perceptions of AI-based e-learning technologies
- Luisa Stracqualursi 1 &
- Patrizia Agati 1
Scientific Reports volume 14 , Article number: 5927 ( 2024 ) Cite this article
1374 Accesses
15 Altmetric
Metrics details
- Human behaviour
- Machine learning
- Psychology and behaviour
Today, teaching and learning paths increasingly intersect with technologies powered by emerging artificial intelligence (AI).This work analyses public opinions and sentiments about AI applications that affect e-learning, such as ChatGPT, virtual and augmented reality, microlearning, mobile learning, adaptive learning, and gamification. The way people perceive technologies fuelled by artificial intelligence can be tracked in real time in microblog messages promptly shared by Twitter users, who currently constitute a large and ever-increasing number of individuals. The observation period was from November 30, 2022, the date on which ChatGPT was launched, to March 31, 2023. A two-step sentiment analysis was performed on the collected English-language tweets to determine the overall sentiments and emotions. A latent Dirichlet allocation model was built to identify commonly discussed topics in tweets. The results show that the majority of opinions are positive. Among the eight emotions of the Syuzhet package, ‘trust’ and ‘joy’ are the most common positive emotions observed in the tweets, while ‘fear’ is the most common negative emotion. Among the most discussed topics with a negative outlook, two particular aspects of fear are identified: an ‘apocalyptic-fear’ that artificial intelligence could lead the end of humankind, and a fear for the ‘future of artistic and intellectual jobs’ as AI could not only destroy human art and creativity but also make the individual contributions of students and researchers not assessable. On the other hand, among the topics with a positive outlook, trust and hope in AI tools for improving efficiency in jobs and the educational world are identified. Overall, the results suggest that AI will play a significant role in the future of the world and education, but it is important to consider the potential ethical and social implications of this technology. By leveraging the positive aspects of AI while addressing these concerns, the education system can unlock the full potential of this emerging technology and provide a better learning experience for students.
Similar content being viewed by others
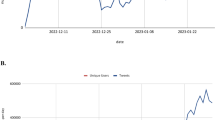
ChatGPT in education: global reactions to AI innovations
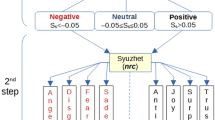
Tweet topics and sentiments relating to distance learning among Italian Twitter users
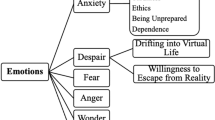
A perspective from Turkey on construction of the new digital world: analysis of emotions and future expectations regarding Metaverse on Twitter
Introduction, ai-powered e-learning technologies.
Current technology continues to advance, continuously transforming the way we live and the way we learn. Over the past few years, e-learning has become increasingly popular, as more people turn to online platforms for education and training. The COVID-19 pandemic has further accelerated this trend, as traditional classroom-based learning has become difficult, if not impossible, in many parts of the world.
Some of the key trends that we are also likely to see in the future of e-learning are as follows:
Adaptive learning. This method uses AI technology to personalize the learning experience for each student. This method adjusts the presentation of educational material according to an individual learner’s needs, preferences, and progress. By analysing students’ performance, it is possible to track their progress, and identify areas where they need more support or challenge. 1 , 2 , 3
Immersive learning. This refers to an educational approach that involves deeply engaging learners in a simulated or interactive environment. It seeks to create a sense of immersion, in which individuals feel fully involved in the learning process through various sensory experiences. The primary goal is to enhance the understanding, retention, and application of knowledge or skills. The key components of immersive learning include virtual and augmented reality (VR/AR). These technologies are becoming increasingly advanced and accessible, and we are likely to see more e-learning platforms using these technologies. 4 , 5 For example, a medical student could use VR to practice surgical techniques in a simulated operating room, 6 or an engineering student could use AR to visualize complex machinery and processes. 7
Microlearning. This refers to short, bite-sized learning modules that are designed to be completed in a few minutes or less. These modules are ideal for learners who have limited time or attention spans, and they can be easily accessed on mobile devices. In the future, we are likely to see more e-learning platforms using microlearning to deliver targeted, on-demand learning experiences. 8 , 9
Gamification. This refers to the use of game-like elements, such as badges, points, and leaderboards, to increase engagement and motivation among learners. Through the addition of game-like features to e-learning courses, learners can be incentivized to complete assignments and reach learning goals; thus, the learning experience becomes more engaging and enjoyable. 10 , 11 , 12
Mobile learning. With the widespread use of smartphones and tablets, e-learning is becoming more mobile-friendly, allowing learners to access course materials and complete assignments on the go. This makes learning more convenient and accessible, as learners can fit their learning into their busy schedules and on-the-go lifestyles. 13 , 14
Social learning. Social media and collaborative tools can enable learners to connect and learn from each other in online communities. 15 Learners can share their experiences, ask questions, and receive feedback from their peers, creating a sense of community and collaboration that can enhance the learning experience. 16
Generative AI. This refers to a subset of artificial intelligence focused on creating new content or information. It involves algorithms and models that are capable of generating novel content that can resemble human-generaed data. In February, 2022, an AI generative system named AlphaCode was launched. This system has been trained to ‘understand’ natural language, design algorithms to solve problems, and then implement them in code. At the end of November 2022, a new generative artificial intelligence chatbot that was developed by OpenAI and named ChatGPT was launched. ChatGPT has a wide range of potential applications due to its ability to generate human-like responses to natural language input. Some of its potentialities include text generation, summarization of long texts into shorter summaries, language translation, question answering, text completion, text correction, programming code generation, equation solutions and so on. ChatGPT evolved into OpenAI’s most widely used product to date, leading to the launch of ChatGPT Plus, a pilot paid subscription, in March 2023. Overall, the potential of AlphaCode and ChatGPT are vast, and these tools are likely to be used in many applications in the future of e-learning, as their capabilities will continue to improve through further research and development. ChatGPT can be integrated as a conversational AI within e-learning platforms. It can provide real-time responses to queries, clarify doubts, offer explanations, and guide learners through course materials. It is a promising tool for language lessons since it can translate text from one language to another. 17 To assist students and improve their writing abilities, ChatGPT may check for grammatical and structural problems in their work and provide valuable comments. 18 Students can explore many things with the help of ChatGPT, such as developing a computer program, writing an essay and solving a mathematical problem. 19 AlphaCode can aid e-learning platforms focused on programming or coding courses. It can provide code suggestions, explanations, and debugging assistance, helping learners better understand coding concepts. 20
How people perceive AI-based technologies: general and e-learning-focused literature overview
In the literature, people’s perceptions of technologies fuelled by artificial intelligence (AI) can vary depending on various factors such as their personal experiences and cultural backgrounds, and the way in which AI is portrayed in the media. The following are some common perceptions of AI technologies:
Fear of Job Loss. One of the most common fears associated with AI technologies is that they will take over jobs previously performed by humans. This fear is especially prominent in industries such as manufacturing, customer service, translation 21 , 22 , 23 and teaching 24 .
Improved Efficiency. Many people view AI technologies as a way to improve efficiency and accuracy in various fields. For example, AI-powered tools can improve students’ performance, 25 AI-powered software can help doctors diagnose diseases, 26 and chatbots can help students 27 and customer service representatives answer queries more quickly. 28
Ethical Concerns. There are concerns about the ethical implications of AI, such as bias in decision-making, 29 invasion of privacy, 30 and the potential for the development of AI-powered weapons. 31 For example, schools and institutions use AI-powered technologies to analyse student academic performance and collect a large amount of personal identity data; if these data are leaked or misused, this will seriously affect students’ personal privacy and security. In addition, students have difficulty controlling their own data and understanding how it is being used and shared, which may also lead to concerns and mistrust regarding personal privacy. 32 , 33
Excitement for Innovation. Some people are excited about the potential of AI to bring about new and innovative solutions to long-standing problems. For example, AI is being used to develop autonomous vehicles, which could revolutionize transportation, 34 and new methods for teaching and learning music. 35
Lack of Trust. Many people are still sceptical about the reliability and safety of AI technologies, especially given recent high-profile incidents of AI systems making mistakes 36 or being manipulated. 37 The lack of trust in the current application of generative AI in education mainly involves two aspects: opacity and reliability. When AI gives a result, it is difficult to explain the decision-making process, which makes it difficult for students to understand why they obtain a particular answer and how to improve their mistakes (opacity). Moreover, generative AI needs to be trained on a large dataset to ensure its effectiveness and reliability. For example, to train an effective automatic grading model, a large dataset of student essays and high-quality labelled data, such as scores for grammar, spelling, and logic, is needed. Insufficient datasets or low-quality labelled data may cause an automatic grading model to make mistakes and miss important aspects and affect its accuracy and application effectiveness. 38
Overall, people’s perceptions of AI technologies are complex and multifaceted, are influenced by a range of factors and are likely to continue evolving as AI becomes more integrated into our lives.
Purpose and outline of the paper
Considering what has been said thus far, it could be interesting to explore sentiments and major topics in the tweets about the new AI-based technologies.
Social media are indeed a major and rich data source for research in many domains due to their 4.8 billion active users 39 across the globe. For instance, researchers analyse user comments extracted from social media platforms (such as Facebook, 40 Twitter, 40 and Instagram 41 ) to uncover insights into social issues such as health, politics and business. Among these platforms, Twitter is one of the most immediate; tweets flow nonstop on the bulletin boards of users. Twitter allows users to express and spread opinions, thoughts and emotions as concisely and quickly as possible. Therefore, researchers have often preferred to analyse user comments on Twitter to immediately uncover insights into social issues during the COVID-19 pandemic (e.g., conspiracy theories, 42 why people oppose wearing a mask, 43 experiences in health care 44 and vaccinations 45 ) or distance learning. 46 , 47 , 48
Furthermore, we chose Twitter for its ability to immediately capture and spread people’s opinions and emotions on any topic, as well as for its ability to provide plentiful data, even in a short amount of time. Moreover, the people who have more direct experience with e-learning and AI technologies are students, teachers and researchers, i.e., persons of school or working age; that is, people who, by age, make up approximately 83% of Twitter users. 49
The text content of a tweet is a short microblog message containing at most 280 characters. This feature makes tweets particularly suitable for natural language processing (NLP) techniques, which are widely used to extract insights from unstructured texts and can then be used to explore sentiments and major topics of tweets. Unlike traditional methods, which use surveys and samples to evaluate these frameworks and are expensive and time-consuming, NLP techniques are economical and fast and provide immediate results.
In this paper, we aim to answer three main questions related to the first months following the launch of ChatGPT:
What has been the dominant sentiment towards AI-powered technologies? We responded through a sentiment analysis of related tweets. We used VADER as a sentiment analysis tool. 50
Which emotions about AI-powered technologies are prevalent? In this regard, we explored the emotions to the tweets using the Syuzhet package. 51
What are the most discussed topics among those who have positive feelings and those who have negative feelings? With respect to this problem, we used the latent Dirichlet allocation (LDA) model. 52
The findings from this study could aid in reimagining education in the postpandemic era to exploit technology and emerging strategies as benefits for educational institutions rather than as preparation for a new possible increase in infections. To this end, we decided to use only the technologies listed in “ AI-powered e-learning technologies ” as keywords for extracting tweets.
Methodology
Twitter was chosen as the data source. It is one of the world’s major social media platforms, with 237.8 million active users in July 2022, 53 and it is also a common source of text for sentiment analyses. 54 , 55 , 56
To collect AI-related tweets, we used ‘Academic Account for Twitter API V2’, which provides historical data and allows for the data to be filtered by language and geolocation. 57
For our study, we chose geolocated English-tweets only, posted from November 30, 2022 - March 31, 2023, with one or more of the following keywords: ‘ChatGPT’, ‘AlphaCode’, ‘virtual reality’, ‘augmented reality’, ‘micro-learning’, ‘mobile learning’, ‘adaptive learning’, ‘social leaning’, ‘AI’, ‘AI learning’ and ‘gamification’. A total of 31,147 tweets were collected.
Data preprocessing
In order to prepare the data for sentiment analysis, we employed various preprocessing techniques using NLP tools in Python. The steps we followed are as follows:
Eliminated mentions, URLs, and hashtags from the text,
Substituted HTML characters with their respective Unicode equivalents (e.g., replacing ‘ &’ with ‘ &’),
Removed HTML tags such as<br>,<p>, and others,
Eliminated unnecessary line breaks,
Removed special characters and punctuation except for exclamation points (the exclamation point is the only punctuation marks to which the used VADER lexicon is sensitive),
Excluded words that consist of only numbers.
For the second part, a high-quality dataset was required for the topic model. To achieve this, we removed duplicate tweets. In addition to the general data cleaning methods, we employed tokenization and lemmatization techniques to enhance the model’s performance.
We used the Gensim library 58 to tokenize the text, converting all the content to lowercase to ensure uniformity in word representation. Next, we pruned the vocabulary by removing stop words and terms unrelated to the topic. Additionally, we created a ‘ bigrams ' model to capture meaningful word combinations.
Finally, we employed the ‘ spaCy ' library from NLTK 59 to carry out lemmatization, which helped simplify words to their base form.
Sentiment and emotions analysis
To conduct sentiment analysis, we utilized the Valence Aware Dictionary for Sentiment Reasoning (VADER) algorithm, developed by Hutto et al. 50 VADER is a sentiment analysis tool that uses a sentiment lexicon, a dictionary specifically designed for sentiment analysis, to determine the emotion intensity of sentiment expressed in a text. The lexicon consists of words or phrases with their accompanying sentiment ratings. It allows for efficient sentiment analysis of social media content and exhibits remarkable accuracy comparable to that of humans.
Using VADER, we assigned sentiment scores to the preprocessed text data of each tweet. We followed the classification method recommended by the authors, categorizing the sentiment scores into three main categories: positive, negative, and neutral (see Fig. 1 —1st Step).
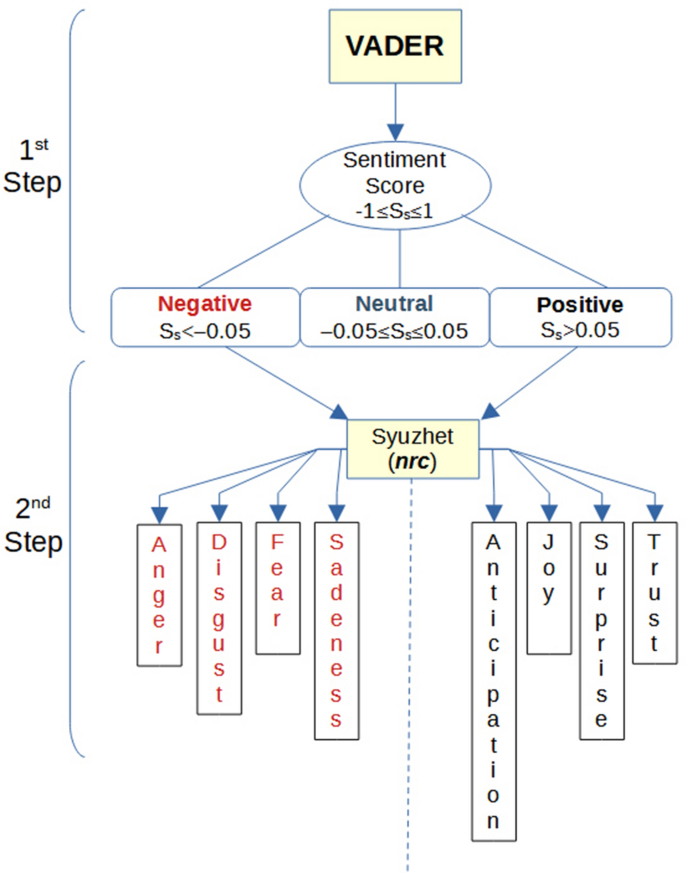
Steps in determining sentiment and emotions analysis.
VADER has demonstrated outstanding performance in analysing social media text. Comprehensive rules to consider various lexical features, including punctuation, capitalization, degree modifiers, the contrastive conjunction ’but’ and negation flipping trigrams.
Next, we employed the ‘ nrc ' algorithm, a component of the R library Syuzhet package, 51 to explore the underlying emotions associated with the tweet categories. In the ‘ nrc ' algorithm, an emotion dictionary is utilized to evaluate each tweet based on two sentiments (positive or negative) and eight emotions (anger, fear, anticipation, trust, surprise, sadness, joy, and disgust). Its purpose is to recognize the emotions conveyed within a tweet.
Whenever a tweet is connected to a specific emotion or sentiment, it receives points indicating the degree of valence in relation to that category. For instance, if a tweet includes three words associated with the ‘fear’ emotion in the word list, the tweet will receive a score of 3 in the fear category. Conversely, if a tweet does not contain any words related to a particular emotion, it will not receive a score for that specific emotion category.
When employing the ‘ nrc ’ lexicon, each tweet is assigned a score for each emotion category instead of a single algebraic score based on positive and negative words. However, this algorithm has limitations in accounting for negators and relies on a bag-of-words approach, disregarding the influence of syntax and grammar. Consequently, the VADER and ‘ nrc ’ methods are not directly comparable in terms of tweet volume and polarity categories.
Therefore, we utilized VADER for sentiment analysis and subsequently employed the ‘ nrc ' algorithm specifically for identifying positive and negative emotions. The sentiment analysis process follows a two-step procedure, as illustrated in Fig. 1 . While VADER’s neutral tweets play a valuable role in classification, they are not particularly informative for emotion analysis. Therefore we focused on tweets exhibiting positive and negative sentiments. This methodology was the original source of our previous paper. 60
The topic model
The topic model is an unsupervised machine learning method; that is, it is a text mining procedure that can be used to identify the topics or themes of documents in a large document corpus. 61 The latent Dirichlet allocation (LDA) model is one of the most popular topic modelling methods; it is a probabilistic model for expressing a corpus based on a three-level hierarchical Bayesian model. Latent Dirichlet allocation (LDA) is a generative probabilistic model of a corpus. The basic idea is that documents are represented as random mixtures over latent topics, where each topic is characterized by a distribution over words. 62 Particularly in LDA models, the generation of documents within a corpus follows the following process:
A mixture of k topics, \(\theta\) , is sampled from a Dirichlet prior, which is parameterized by \(\alpha\) ;
A topic \(z_n\) is sampled from the multinomial distribution, \(p(\theta \mid \alpha )\) that is the document topic distribution which models \(p(z_{n}=i\mid \theta )\) ;
Fixed the number of topics \(k=1...,K\) , the distribution of words for k topics is denoted by \(\phi\) ,which is also a multinomial distribution whose hyper-parameter \(\beta\) follows the Dirichlet distribution;
Given the topic \(z_n\) , a word, \(w_n\) , is then sampled via the multinomial distribution \(p(w \mid z_{n};\beta )\) .
Overall, the probability of a document (or tweet, in our case) “ \(\textbf{w}\) ” containing words can be described as:
Finally, the probability of the corpus of M documents \(D=\{\textbf{ w}_{\textbf{1}},...,{\textbf{w}}_{\textbf{M}}\}\) can be expressed as the product of the marginal probabilities of each single document \(D_m\) , as shown in ( 2 ).
An essential challenge in LDA is deterining an appropriate number of topics. Roder et al. 63 proposed coherence scores to evaluate the quality of each topic model. In particular, topic coherence is the metric used to evaluate the coherence between topics inferred by a model. As coherence measures, we used \(C_v\) which is a measure based on a sliding window that uses normalized pointwise mutual information (NPMI) and cosine similarity. 63 This value is used to emulate the relative score that a human is likely to assign to a topic and indicate how much the topic words ‘make sense’. This score is used to infer cohesiveness between ‘top’ words within a given topic. For topic visualization we used PyLDAvis, a web-based interactive visualization package that facilitates the display of the topics that were identified using the LDA approach. 52 In this package, each topic is visualized as a circle in a two-dimensional plane determined by principal components between topics and used Multidimensional scaling is used to project all the interrelated topic distances to two dimensions. 64 In the best hypothetic situation, the circles have similar dimensions and are well spaced from each other, covering the entire space made up of the 4 quadrants of the graph. An LDA model is evaluated the better the more the coherence is and the closer the pyLDAvis visualization is to the hypothetical situation.
Exploring the tweets
The word frequency of the most frequent 25 words terms are counted and visualized in Fig. 2 . The words are related to new AI tools and have positive attributes such as ‘good’ or ‘great’.
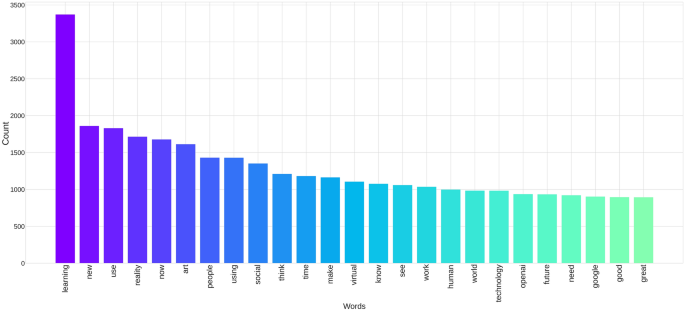
The total text word frequency. After removing irrelevant words, we counted and visualized the 25 most frequent words in our dataset.
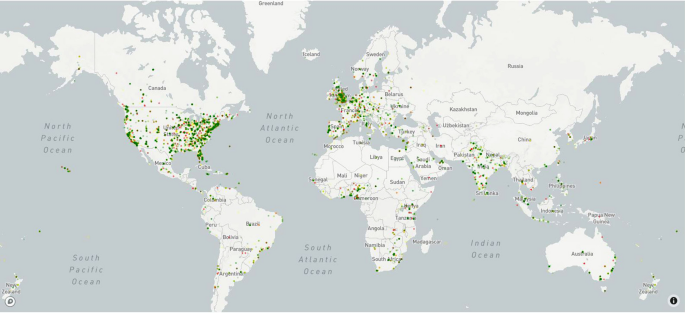
Tweets according to polarity by country.
All the tweets extracted were geolocated, but the ‘user location’ was detected in only approximately 85% of the tweets, highlighting the number of tweets that came from different counties around the world. The countries with the highest number of tweets are the United States and Great Britain (Fig. 3 ) but this result should be read considering that we have extracted tweets in the English language; therefore, it is normal that in countries where English is spoken, the number of tweets is very high. Notably, India and Europe also have a many Twitter users. In the United States, most Twitter users are located near the East and West Coasts. This figure also shows the polarity of the tweets: the colours red, yellow and green indicate negative, neutral and positive tweets, respectively.
Sentiment analysis
The sentiment score of a sentence is calculated by summing up the lexicon rates of each VADER-dictionary-listed word in the sentence. After proper normalization is applied, VADER returns a ‘compound’ sentiment score ( \(S_s\) ) in the range of \(-1\) to 1, from the most negative to the most positive. Once the score \(S_s\) is known, threshold values can be used to categorize tweets as positive, negative, or neutral (see Fig. 1 —1st Step). According to our analysis, the output of the VADER model shows a great predominance of positive public opinion (Table 1 ). As an example, three tweets with their own polarity are shown in Table 2 . Table 3 shows the number of total tweets with the related percentages and the percentages of positive, negative and neutral tweets of the various AI applications examined.
Regarding the timeline, the results showed that the number of tweets slowly increased during the observation period (Fig. 4 ). Clearly, as shown in the chart, there was a single weekly decline in the third week of March, likely due to the St. Patrick’s Day holiday, which fell close to the weekend (March 17).
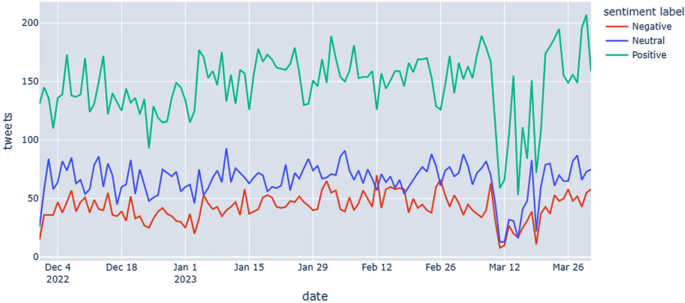
Timeline showing the sentiment of tweets.
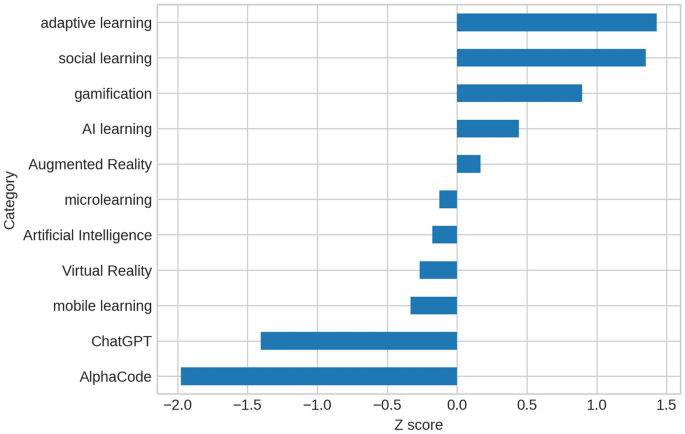
z scores of relative frequencies for positive sentiments.
The graph in Fig. 5 includes only the tweets with positive sentiment towards the 11 AI-based tools (categories). It returns the z scores of the relative frequencies \(Fr_{i}\) of positive tweets of the i -tool ( \(i=1,...,11\) ), where the z score is computed as follows:
A z score describes the position of a score (in our case \(Fr_i\) ) in terms of standard deviation units from the average. This score is grater then 0 if the relative frequency of \(Fr_i\) lies above the mean, while it is less than 0 if \(Fr_i\) lies below the mean.
We preferred z scores to relative frequencies because the different categories have different averages: the z scores highlight the position of each \(Fr_i\) with respect to the average of all the relative frequencies.
In Fig. 5 , we can see that the new generative AI ‘AlphaCode’ and ‘ChatGPT’ have scores that are much lower than and far from the average of positive sentiments. This could be due to concerns about the possible errors of such AI-based tools. Instead, ‘adaptive learning’, ‘social learning’ and ‘gamification’ lie above the mean of positive sentiments. This clearly attests to the more positive sentiment towards these tools, in our opinion largely due to their immediate feedback and to their attitude towards keeping learners engaged and motivated.
The second step of the analysis focused on identifying emotions in non-neutral tweets (see Fig. 1 —2nd Step). Among the eight basic emotions, ‘trust’ was the most common positive emotion observed in the tweets, followed by ‘joy’, while ‘fear’ was the most common negative emotion (Fig. 6 ). These results need to be interpreted in light of recent literature on the psychological dimensions of AI-based e-learning technologies (see section " How people perceive AI-based technologies: general and e-learning-focused literature overview "). In the literature, the dimension of fear includes the fear of job loss for teachers but also for the entire working world, 21 , 22 , 23 , 65 as well as concerns about AI systems making mistakes 36 or being manipulated. 37 The ‘trust’ dimension could be interpreted as the expectation that such technologies can improve the performance of students and people in general, 26 while ‘joy’ could be associated with enthusiasm for the potential of artificial intelligence in creating new and innovative solutions. 34
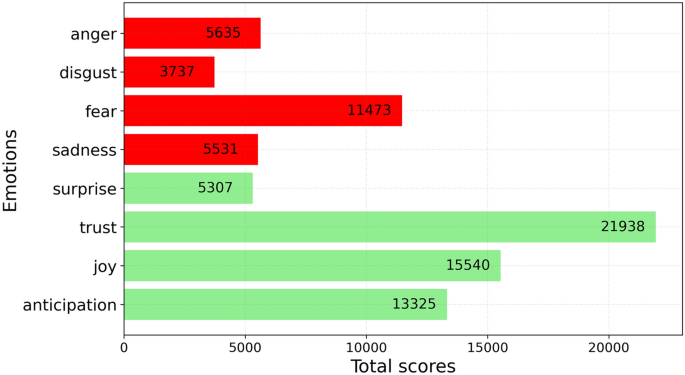
Emotion analysis of non-neutral tweets performed by Syuzhet .
To explore what concerns about AI-based tools Twitter users have, we applied the LDA model to our clean corpus of 28,259 words, which included only the following tagger components: nouns, adjectives, verbs and adverbs. Our goal was not to discover the topics discussed in the whole set of tweets but to detect the topics discussed in the positive sentiment tweets and the topics discussed in the negative sentiment tweets. Due to the large difference between the number of tweets with positive and negative sentiment polarity (57.58% vs. 16.65%), the application of the LDA model to the whole dataset would lead to not seeing the topics discussed in tweets with negative sentiment. Therefore, we chose to create two LDA models: one for tweets with positive polarity and one for those with negative polarity. For a better representation of the entire content, in each model, it is necessary to find an appropriate number of topics. By using topic numbers k ranging from 2 to 10, we initialized the LDA models and calculated the model coherence.
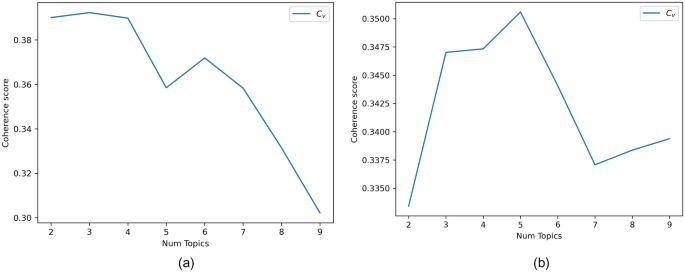
Coherence values of the LDA models.
We used \(C_v\) coherence for both models as a first reference. This value indicates the degree of ‘sense’ and ‘cohesiveness’ of the main words within a topic. 63
According to Fig. 7 a, the coherence score peaked at 3 for the negative tweet model. In contrast, in the positive tweet model, the coherence score (Fig. 7 b) peaked at 3 and 5 topics. The choice of 5 topic numbers would lead to a nonuniform distribution on Principal Component (PC) axes displayed by pyLDAvis, which means that there is not a high degree of independence for each topic (see the LDAvis map in Supplementary Information ‘ S2 ’ and ‘ S3 ’). A good model is judeged by a higher coherence and an average distribution on the principal component analysis displayed by pyLDAvis. 52 Therefore, we chose 3 as the topic number: the model has no intersections among topics, summarizes the whole word space well, and retains relatively independent topics.
The LDA analysis for negative and positive polarity tweets is shown in Table 4 .
In the negative tweets, the first theme accounts for 37.5% of the total tokens and includes tokens such as ‘chatgpt’, ‘write’,‘art’, ‘need’, ‘thing’, ‘stop’ and ‘generate’. It is rather immediate to think that this negative opinion refers to tools such as ChatGPT and expresses concerns about its use in art and writing. People think that human creativity can generate art and literature rather than generative AI; for this reason, the use of generative tools in these contexts should be stopped. The second theme accounts for 33.3% of the total tokens and includes the words ‘bad’, ‘job’, ‘technology’, ‘scary’, ‘change’ and ‘learn’. We infer people’s fear of the changes that technology will bring in the world of job and learning. Several words, such as ‘kill’, ‘war’, ‘worry’ ,‘fear’, ‘fight’, ‘robot’ and ‘dangerous’, are mentioned in the third topic. This may indicate a sort of ‘apocalyptic fear’ that artificial intelligence could lead us to a new war and the end of humankind. For a map representation of the three topics, see the Supplementary Information ‘ S1 ’.
In the positive tweets, the first theme accounts for 36.3% of the total tokens and includes tokens such as ‘learn’, ‘help’, ‘technology’, ‘student’ and ‘job’. Based on this, we inferred that people think that AI technologies have the potential to improve the job world and educational system. The second theme accounts for 34.7% of the total tokens, including the words ‘chatgpt’, ‘well’, ‘write’, ‘create’ and ‘ask’, showing people’s positive perception of AIs such as ChatGPT writing, asking questions and creating new solutions. After all, several words, such as ‘love’, ‘chatgpt’, ‘good’ ,‘answer’ and ‘believe’, are mentioned in the third topic. This indicates that people ‘believe in AI’ and trust tha AI, particularly ChatGPT provides good answers and solutions. For a map representation of the three topics, see the Supplementary Information ‘ S2 ’.
Based on the LDA outputs, the following six topics were identified:
For ‘negative polarity’ tweets:
Topic 1: Concerns about ChatGPT use in art and writing
Topic 2: Fear of changes in the world of job and learning
Topic 3: Apocalyptic-fear.
For ‘positive polarity’ tweets:
Topic 1: AI technologies can improve job and learning
Topic 2: Useful ChatGPT features
Topic 3: Belief in the ability of ChatGPT.
Limitations
This study has several limitations, which can be summarized as follows.
Limitations related to the use of keywords to extract tweets. Sometimes, keywords can be ambiguous, leading to noise-affected results. Due to the dynamic nature of social media, trends and topics change rapidly. Keywords might quickly lose relevance as new terms emerge.
Limitations related to emotion analysis . A first limitation is that the number of emotion categories was limited to 8; 51 , 66 however, emotion is a broad concept and, according to Cowen and Keltner 67 , may involve up to 27 categories. A second limitation is that misspelled words could not be identified or analysed in the algorithm. Further limitations involve the dictionary of sentiments (“lexicon”) developed by Mohammad and Turney for emotion analysis. 51 This dictionary maps a list of language features to emotion intensities, where:
Only 5 individuals were recruited to annotate a term against each of the 8 primary emotions.
The emotions associated with a term were annotated without considering the possible term context.
Although the percentages of agreement were apparently high, interrater reliability statistics were not reported.
Limitations of topic analysis. Considering that LDA is an unsupervised learning technique, the main limitation is the degree of subjectivity in defining the topic created. 45
Limitations of Twitter-based studies. Twitter data generally underestimate the opinions of people aged 50 and over, because approximately 83% of Twitter users worldwide are indeed under age 50. 49 However, in the present study bias has an almost negligible impact: the future of AI-powered e-learning technologies indeed has a greater impact on younger people than on older people.
Conclusions and future perspectives
With the aim of studying the opinions and emotions related to AI-powered e-learning technologies, we collected tweets on this issue and carried out a sentiment analysis using the VADER and Syuzhet packages in combination with a topic analysis.
There is no doubt that artificial intelligence has the potential to transform the whole education system. The results showed a predominance of positive attitudes: topics with a positive outlook indicate trust and hope in AI tools that can improve efficiency in jobs and the educational world. Indeed, among the eight emotions of the Syuzhet package, ‘trust’ and ’joy’ were the most positive emotions observed in the tweets, while ‘fear’ was the most common negative emotion. Based on the analysis, two particular aspects of fear were identified: an ‘apocalyptic fear’ that artificial intelligence could lead to the end of humankind and a fear of the ‘future of artistic and intellectual jobs’, as AI could not only destroy human art and creativity but also make individual contributions of students and researchers not assessable.
In our analysis, people with positive sentiments were directed towards ‘adaptive learning’, ‘social learning’ and ‘gamification’. Therefore, from a future perspective, we can expect an ever-increasing implementation of these aspects in e-learning. AI could help educators ‘adapt learning’ techniques to tailor them to the individual student, with his or her own interests, strengths and preferences.
By analysing data about interactions between students, AI can identify opportunities for collaboration between students and thus transform ‘social learning’ into ‘collaborative learning’. AI could help educators create more effective group work assignments, provide targeted support to struggling students, and promote positive social interactions among them.
In class, instead of administering boring tests, AI-powered ‘games and simulations’ could increasingly provide engaging and interactive learning experiences to help students develop skills and knowledge in a fun and engaging way. Moreover, gamification could be increasingly useful for providing immediate feedback and monitoring student progress over time.
Despite our analysis highlighting the great potential of and people’s expectations for AI-based technologies, there is an aspect that cannot be elucidated by examining tweets.
Algorithms such as ChatGPT disrupt traditional text-based assessments, as students can query the program to research a topic that results in documents authored by an algorithm and ready to submit as a graded assignment. Therefore, we need to reimagine student assessment in new ways. The current debate is whether educators should ban artificial intelligence platforms through school internet filters 38 or embrace algorithms as teaching and research tools. 68
In March, 2023, Italy was the first government to ban ChatGPT as a result of privacy concerns. The Italian data-protection authority said there were privacy concerns relating to the model and said it would investigate immediately. However, in late April, the ChatGPT chatbot was reactivated in Italy after its maker OpenAI addressed issues raised by Italy’s data protection authority.
Regardless of privacy concerns, possible data manipulations or the right answers to AI-based tools, we believe that the future cannot be stopped.
Data availability
All data generated or analyzed during this study are included in the Supplementary Information Files of this published article.
Zahabi, M. & Abdul Razak, A. M. Adaptive virtual reality-based training: A systematic literature review and framework. Virtual Real. 24 , 725–752. https://doi.org/10.1007/s10055-020-00434-w (2020).
Article Google Scholar
Raj, N. S. & Renumol, V. G. A systematic literature review on adaptive content recommenders in personalized learning environments from 2015 to 2020. J. Comput. Educ. 9 , 113–148. https://doi.org/10.1007/s40692-021-00199-4 (2022).
Al-Badi, A., Khan, A. & Eid-Alotaibi,. Perceptions of learners and instructors towards artificial intelligence in personalized learning. Proced. Comput. Sci. 201 , 445–451. https://doi.org/10.1016/j.procs.2022.03.058 (2022).
Bizami, N. A., Tasir, Z. & Kew, S. N. Innovative pedagogical principles and technological tools capabilities for immersive blended learning: A systematic literature review. Educ. Inf. Technol. 28 , 1373–1425. https://doi.org/10.1007/s10639-022-11243-w (2023).
Won, M. et al. Diverse approaches to learning with immersive virtual reality identified from a systematic review. Comput. Educ. 195 , 104701. https://doi.org/10.1016/j.compedu.2022.104701 (2023).
Tang, Y. M., Chau, K. Y., Kwok, A. P. K., Zhu, T. & Ma, X. A systematic review of immersive technology applications for medical practice and education—trends, application areas, recipients, teaching contents, evaluation methods, and performance. Educ. Res. Rev. 35 , 100429. https://doi.org/10.1016/j.edurev.2021.100429 (2022).
Wilkerson, M., Maldonado, V., Sivaraman, S., Rao, R. R. & Elsaadany, M. Incorporating immersive learning into biomedical engineering laboratories using virtual reality. J. Biol. Eng. 16 , 20. https://doi.org/10.1186/s13036-022-00300-0 (2022).
Article CAS PubMed PubMed Central Google Scholar
Taylor, A.-D. & Hung, W. The effects of microlearning: A scoping review. Educ. Technol. Res. Dev. 70 , 363–395. https://doi.org/10.1007/s11423-022-10084-1 (2022).
Wang, C., Bakhet, M., Roberts, D., Gnani, S. & El-Osta, A. The efficacy of microlearning in improving self-care capability: A systematic review of the literature. Public Health 186 , 286–296. https://doi.org/10.1016/j.puhe.2020.07.007 (2020).
Article CAS PubMed Google Scholar
Oliveira, W. et al. Tailored gamification in education: A literature review and future agenda. Educ. Inf. Technol. 28 , 373–406. https://doi.org/10.1007/s10639-022-11122-4 (2023).
Indriasari, T. D., Luxton-Reilly, A. & Denny, P. Gamification of student peer review in education: A systematic literature review. Educ. Inf. Technol. 25 , 5205–5234. https://doi.org/10.1007/s10639-020-10228-x (2020).
Liu, T., Oubibi, M., Zhou, Y. & Fute, A. Research on online teachers’ training based on the gamification design: A survey analysis of primary and secondary school teachers. Heliyon 9 , e15053. https://doi.org/10.1016/j.heliyon.2023.e15053 (2023).
Article PubMed PubMed Central Google Scholar
Widiastuti, N. L. A systematic literature review of mobile learning applications in environmental education from 2011–2021. J. Educ. Technol. Inst. 1 , 89–98 (2022).
Google Scholar
Criollo-C, S., Guerrero-Arias, A., Jaramillo-Alcázar, A. & Luján-Mora, S. Mobile learning technologies for education: Benefits and pending issues. Appl. Sci. https://doi.org/10.3390/app11094111 (2021).
Chelarescu, P. Deception in social learning: a multi-agent reinforcement learning perspective. arxiv: 2106.05402 (2021)
Gweon, H. Inferential social learning: Cognitive foundations of human social learning and teaching. Trends Cogn. Sci. 25 , 896–910. https://doi.org/10.1016/j.tics.2021.07.008 (2021).
Article PubMed Google Scholar
Javaid, M., Haleem, A., Singh, R. P., Khan, S. & Khan, I. H. Unlocking the opportunities through chatgpt tool towards ameliorating the education system. BenchCouncil Trans. Benchmarks Stand. Eval. 3 , 100115. https://doi.org/10.1016/j.tbench.2023.100115 (2023).
Sok, S. & Heng, K. ChatGPT for education and research: A review of benefits and risks. SSRN Electron. J. https://doi.org/10.2139/ssrn.4378735 (2023).
Yilmaz, R. & Karaoglan Yilmaz, F. G. Augmented intelligence in programming learning: Examining student views on the use of chatgpt for programming learning. Comput. Hum. Behav. Artif. Hum. 1 , 100005. https://doi.org/10.1016/j.chbah.2023.100005 (2023).
Becker, B. A. et al. Programming is hard—or at least it used to be: Educational opportunities and challenges of AI code generation. In Proceedings of the 54th ACM Technical Symposium on Computer Science Education V. 1 , SIGCSE 2023, 500–506, https://doi.org/10.1145/3545945.3569759 (Association for Computing Machinery, New York, 2023).
Ernst, E., Merola, R. & Samaan, D. Economics of artificial intelligence: Implications for the future of work. IZA J. Labor Policy 9 , 55. https://doi.org/10.2478/izajolp-2019-0004 (2019).
Jaiswal, A., Arun, C. J. & Varma, A. Rebooting employees: Upskilling for artificial intelligence in multinational corporations. Int. J. Hum. Resour. Manag. 33 , 1179–1208. https://doi.org/10.1080/09585192.2021.1891114 (2022).
Kirov, V. & Malamin, B. Are translators afraid of artificial intelligence?. Societies https://doi.org/10.3390/soc12020070 (2022).
Selwyn, N. Should Robots Replace Teachers?: AI and the Future of Education (John Wiley & Sons, 2019).
Baidoo-Anu, D. & Owusu Ansah, L. Education in the era of generative artificial intelligence (AI): Understanding the potential benefits of chatgpt in promoting teaching and learning. J. AI 7 , 52–62. https://doi.org/10.61969/jai.1337500 (2023).
van Leeuwen, K. G., de Rooij, M., Schalekamp, S., van Ginneken, B. & Rutten, M. J. C. M. How does artificial intelligence in radiology improve efficiency and health outcomes?. Pediatr. Radiol. 52 , 2087–2093. https://doi.org/10.1007/s00247-021-05114-8 (2022).
Shingte, K., Chaudhari, A., Patil, A., Chaudhari, A. & Desai, S. Chatbot development for educational institute. SSRN Electron. J. https://doi.org/10.2139/ssrn.3861241 (2021).
Wang, X., Lin, X. & Shao, B. How does artificial intelligence create business agility? Evidence from chatbots. Int. J. Inf. Manage. 66 , 102535. https://doi.org/10.1016/j.ijinfomgt.2022.102535 (2022).
Parikh, R. B., Teeple, S. & Navathe, A. S. Addressing bias in artificial intelligence in health care. JAMA 322 , 2377–2378. https://doi.org/10.1001/jama.2019.18058 (2019).
Mazurek, G. & Małagocka, K. Perception of privacy and data protection in the context of the development of artificial intelligence. J. Manag. Anal. 6 , 344–364 (2019).
David, W. E. A. Ai-powered lethal autonomous weapon systems in defence transformation. Impact and challenges. In Modelling and Simulation for Autonomous Systems (eds Mazal, J. et al. ) 337–350 (Springer International Publishing, 2020).
Chapter Google Scholar
May, M. & George, S. Privacy concerns in e-learning: Is UsingTracking system a threat?. Int. J. Inf. Educ. Technol. 1 , 1–8 (2011).
ADS Google Scholar
Ashman, H. et al. The ethical and social implications of personalization technologies for e-learning. Inf. Manag. 51 , 819–832. https://doi.org/10.1016/j.im.2014.04.003 (2014).
Ma, Y., Wang, Z., Yang, H. & Yang, L. Artificial intelligence applications in the development of autonomous vehicles: A survey. IEEE/CAA J. Autom. Sin. 7 , 315–329. https://doi.org/10.1109/JAS.2020.1003021 (2020).
Wei, J., Karuppiah, M. & Prathik, A. College music education and teaching based on AI techniques. Comput. Electr. Eng. 100 , 107851. https://doi.org/10.1016/j.compeleceng.2022.107851 (2022).
Mahmood, A., Fung, J. W., Won, I. & Huang, C.-M. Owning mistakes sincerely: Strategies for mitigating AI errors. In Proceedings of the 2022 CHI Conference on Human Factors in Computing Systems , CHI ’22, https://doi.org/10.1145/3491102.3517565 (Association for Computing Machinery, New York, 2022).
Carroll, M., Chan, A., Ashton, H. & Krueger, D. Characterizing manipulation from AI systems. arxiv: 2303.09387 (2023).
Yu, H. & Guo, Y. Generative artificial intelligence empowers educational reform: Current status, issues, and prospects. Front. Educ. https://doi.org/10.3389/feduc.2023.1183162 (2023).
Kemp, S. Digital 2023: Global digital overview. (Accessed April 2023); Online https://datareportal.com/reports/digital-2023-april-global-statshot (2023).
Zhan, Y., Etter, J.-F., Leischow, S. & Zeng, D. Electronic cigarette usage patterns: A case study combining survey and social media data. J. Am. Med. Inform. Assoc. 26 , 9–18. https://doi.org/10.1093/jamia/ocy140 (2019).
Hassanpour, S., Tomita, N., DeLise, T., Crosier, B. & Marsch, L. A. Identifying substance use risk based on deep neural networks and instagram social media data. Neuropsychopharmacology 44 , 487–494. https://doi.org/10.1038/s41386-018-0247-x (2019).
Rains, S. A., Leroy, G., Warner, E. L. & Harber, P. Psycholinguistic markers of COVID-19 conspiracy tweets and predictors of tweet dissemination. Health Commun. https://doi.org/10.1080/10410236.2021.1929691 (2021).
He, L. et al. Why do people oppose mask wearing? a comprehensive analysis of U.S. tweets during the COVID-19 pandemic. J. Am. Med. Inform. Assoc. 28 , 1564–1573. https://doi.org/10.1093/jamia/ocab047 (2021).
Ainley, E., Witwicki, C., Tallett, A. & Graham, C. Using twitter comments to understand people’s experiences of UK health care during the COVID-19 pandemic: Thematic and sentiment analysis. J. Med. Internet Res. https://doi.org/10.2196/31101 (2021).
Kwok, S. W. H., Vadde, S. K. & Wang, G. Tweet topics and sentiments relating to COVID-19 vaccination among Australian twitter users: Machine learning analysis. J. Med. Internet Res. 23 , e26953. https://doi.org/10.2196/26953 (2021).
Aljabri, M. et al. Sentiment analysis of Arabic tweets regarding distance learning in Saudi Arabia during the COVID-19 pandemic. Sensors (Basel) 21 , 5431. https://doi.org/10.3390/s21165431 (2021).
Article ADS CAS PubMed Google Scholar
Mujahid, M. et al. Sentiment analysis and topic modeling on tweets about online education during COVID-19. Appl. Sci. (Basel) 11 , 8438. https://doi.org/10.3390/app11188438 (2021).
Article CAS Google Scholar
Asare, A. O., Yap, R., Truong, N. & Sarpong, E. O. The pandemic semesters: Examining public opinion regarding online learning amidst COVID-19. J. Comput. Assist. Learn. 37 , 1591–1605. https://doi.org/10.1111/jcal.12574 (2021).
Statista. Distribution of twitter users worldwide as of april 2021, by age group. Statista. https://www.statista.com/statistics/283119/age-distribution-of-global-twitter-users/ (2021).
Hutto, C. & Gilbert, E. Vader: A parsimonious rule-based model for sentiment analysis of social media text. In Proceedings of the 8th International Conference on Weblogs and Social Media, ICWSM 2014 (2015).
Mohammad, S. & Turney, P. Emotions evoked by common words and phrases: Using mechanical turk to create an emotion lexicon. In Proceedings of the NAACL HLT 2010 Workshop on Computational Approaches to Analysis and Generation of Emotion in Text (LA, California, 2010).
Sievert, C. & Shirley, K. LDAvis: A method for visualizing and interpreting topics. In Proceedings of the Workshop on Interactive Language Learning, Visualization, and Interfaces , 63–70, https://doi.org/10.3115/v1/W14-3110 (Association for Computational Linguistics, https://aclanthology.org/W14-3110 , Baltimore, Maryland, 2014).
Kemp, S. Digital 2022: Twitter report (Accessed July 2022). Online https://datareportal.com/reports/digital-2023-deep-dive-the-state-of-twitter-in-april-2023 (2022).
Tumasjan, A., Sprenger, T., Sandner, P. & Welpe, I. Predicting elections with twitter: What 140 characters reveal about political sentiment. In Proc. Fourth Int. AAAI Conf. Weblogs Soc. Media Predict. , vol. 10 (2010).
Oyebode, O., Orji, R. Social. & media and sentiment analysis: The Nigeria presidential election,. In 2019 IEEE 10th Annual Information Technology. Electronics and Mobile Communication Conference (IEMCON) 2019 , https://doi.org/10.1109/IEMCON.2019.8936139 (IEEE 2019).
Budiharto, W. & Meiliana, M. Prediction and analysis of Indonesia presidential election from twitter using sentiment analysis. J. Big Data https://doi.org/10.1186/s40537-018-0164-1 (2018).
Twitter API V2 . Academic Account for Twitter API V2. https://developer.twitter.com/en/products/twitter-api/academic-research (2022).
Řehuřek, R. & Sojka, P. Software framework for topic modelling with large corpora. In Proceedings of LREC 2010 workshop New Challenges for NLP Frameworks , 46–50 (Univerity of Malta, 2010).
Bird, S., Klein, E. & Loper, E. Natural Language Processing with Python (O’Reilly Media, 2009).
Stracqualursi, L. & Agati, P. Tweet topics and sentiments relating to distance learning among Italian twitter users. Sci. Rep. 12 , 9163 (2022).
Article ADS CAS PubMed PubMed Central Google Scholar
Blei, D. M., Ng, A. Y., Jordan, M. I. & Lafferty, J. Latent dirichlet allocation. J. Mach. Learn. Res. 3 , 993–1022 (2003).
Lee, J. et al. Ensemble modeling for sustainable technology transfer. Sustainability 10 , 22–78. https://doi.org/10.3390/su10072278 (2018).
Röder, M., Both, A. & Hinneburg, A. Exploring the space of topic coherence measures. In Proceedings of the Eighth ACM International Conference on Web Search and Data Mining - WSDM ’15 (ACM Press, 2015).
Sievert, C. & Shirley, K. Package ldavis (Online) https://cran.r-project.org/web/packages/LDAvis/LDAvis.pdf (2022).
Edwards, B. I. & Cheok, A. D. Why not robot teachers: Artificial intelligence for addressing teacher shortage. Appl. Artif. Intell. 32 , 345–360. https://doi.org/10.1080/08839514.2018.1464286 (2018).
Plutchik, R. A general psychoevolutionary theory of emotion. In Theories of Emotion , 3–33, https://doi.org/10.1016/b978-0-12-558701-3.50007-7 (Elsevier, 1980).
Cowen, A. S. & Keltner, D. Self-report captures 27 distinct categories of emotion bridged by continuous gradients. Proc. Natl. Acad. Sci. U. S. A. 114 , E7900–E7909. https://doi.org/10.1073/pnas.1702247114 (2017).
Reyna, J. The potential of artificial intelligence (AI) and chatgpt for teaching, learning and research. In EdMedia+ Innovate Learning , 1509–1519 (Association for the Advancement of Computing in Education (AACE), 2023).
Download references
Author information
Authors and affiliations.
Department of Statistics, University of Bologna, 40126, Bologna, Italy
Luisa Stracqualursi & Patrizia Agati
You can also search for this author in PubMed Google Scholar
Contributions
L.S. and P.A. contributed equally to this work. Particularly, L.S. wrote the main text of the manuscript, contributed to the formal analysis, conceptualization, investigation and creation of the software in Python. P.A. contributed to the formalization of the applied methodology, to the data management, to the preparation of the figures, to the supervision and to the revision and modification of the manuscript.
Corresponding author
Correspondence to Luisa Stracqualursi .
Ethics declarations
Competing interests.
The authors declare no competing interests.
Additional information
Publisher's note.
Springer Nature remains neutral with regard to jurisdictional claims in published maps and institutional affiliations.
Supplementary Information
Supplementary figure s1., supplementary figure s2., supplementary figure s3., supplementary table s1., rights and permissions.
Open Access This article is licensed under a Creative Commons Attribution 4.0 International License, which permits use, sharing, adaptation, distribution and reproduction in any medium or format, as long as you give appropriate credit to the original author(s) and the source, provide a link to the Creative Commons licence, and indicate if changes were made. The images or other third party material in this article are included in the article’s Creative Commons licence, unless indicated otherwise in a credit line to the material. If material is not included in the article’s Creative Commons licence and your intended use is not permitted by statutory regulation or exceeds the permitted use, you will need to obtain permission directly from the copyright holder. To view a copy of this licence, visit http://creativecommons.org/licenses/by/4.0/ .
Reprints and permissions
About this article
Cite this article.
Stracqualursi, L., Agati, P. Twitter users perceptions of AI-based e-learning technologies. Sci Rep 14 , 5927 (2024). https://doi.org/10.1038/s41598-024-56284-y
Download citation
Received : 06 May 2023
Accepted : 05 March 2024
Published : 11 March 2024
DOI : https://doi.org/10.1038/s41598-024-56284-y
Share this article
Anyone you share the following link with will be able to read this content:
Sorry, a shareable link is not currently available for this article.
Provided by the Springer Nature SharedIt content-sharing initiative
By submitting a comment you agree to abide by our Terms and Community Guidelines . If you find something abusive or that does not comply with our terms or guidelines please flag it as inappropriate.
Quick links
- Explore articles by subject
- Guide to authors
- Editorial policies
Sign up for the Nature Briefing newsletter — what matters in science, free to your inbox daily.

share this!
April 30, 2024
This article has been reviewed according to Science X's editorial process and policies . Editors have highlighted the following attributes while ensuring the content's credibility:
fact-checked
peer-reviewed publication
trusted source
Should online educational platforms offer courses following a schedule or release them on demand?
by Marilyn Stone, American Marketing Association
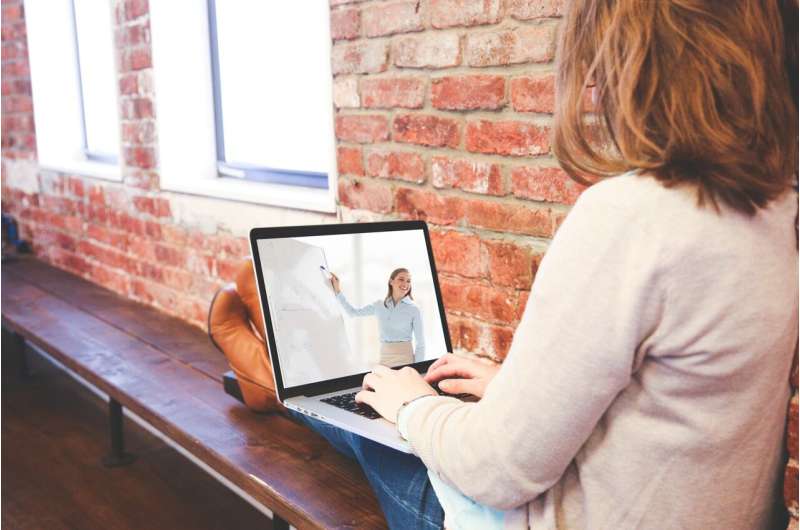
Researchers from Carnegie Mellon University and University of Pennsylvania have published a new Journal of Marketing study that examines online educational platforms and the question of whether they should release content through a scheduled format that resembles a traditional university course or use an on-demand release strategy.
The study is titled "More Likely to Pay but Less Engaged: The Effects of Switching Online Courses from Scheduled to On-Demand Release on User Behavior" and is authored by Joy Lu, Eric T. Bradlow, and J. Wesley Hutchinson.
In 2011, the online education industry catered to around 300,000 consumers. In 2021, it served 220 million, thanks in part to increased enrollment during the COVID-19 pandemic. Traditional universities and institutions are increasingly adopting hybrid course formats. For example, the number of full-time online MBA students surpassed in-person MBA students for the first time in the 2020-21 academic year.
Today, online educational platforms like Coursera and edX offer a range of flexible course content, but these firms are faced with a tricky question: Should they release content through a scheduled format that resembles a traditional university course with a subset of lectures and quizzes available at the start of each week, or should they follow in the footsteps of Netflix and Hulu with an on-demand release strategy where all the material is immediately available upon registration?
This new article finds that the choice of format for content release not only impacts overall user engagement and firm revenue but also user performance and learning outcomes.
The researchers studied over 67,000 users taking an introductory marketing course on Coursera consisting of 32 short lecture videos and four quizzes. The study took advantage of a natural experiment policy change where the platform switched the course from a scheduled format to an on-demand release format while keeping the actual content the same.
The scheduled format closely resembled a traditional university course, with some of the study material available at the beginning of each week for four weeks. In the on-demand format, all four weeks of content was made available upon registration. All users could take the course for free or opt into paying for a completion certificate, either as a one-time fee in the scheduled format or a monthly subscription in the on-demand format.
More users, less engagement
The study's findings show that the switch to on-demand content doubled the percentage of paying users from 14% to 28%. Lu explains that "the on-demand format was successful in increasing short-term firm revenue by bringing in more paying users. On the downside, the switch resulted in significantly lower lecture completion rates and lower quiz performance."
The on-demand format also negatively impacted downstream platform engagement. The marketing course was promoted in a "Business Foundations" set with three other courses on operations, accounting, and finance.
"Compared to users in the scheduled format, those in the on-demand format ended up taking one or two fewer additional courses six months after the focal marketing course," says Bradlow.
Analysis of user activity reveals two new learning patterns:
- A subset (13%) of users in the on-demand format continued to return and take quizzes well beyond the recommended four-week course period. The greater flexibility in the on-demand content release and payment structure likely enabled these users to "stretch out" their consumption.
- The on-demand format increased the practice of binging—with user activity being clumped together (i.e., more binging) as compared to being evenly spaced out (i.e., less binging). In the scheduled format, binging was negatively related to course performance, which is consistent with the intuition that binging reflects procrastination or cramming. However, in the on-demand format, binging was positively related to performance, suggesting that on-demand users may binge as a form of strategic time management by setting aside time to consume in spurts.
Real-world implications
This study offers vital lessons for chief marketing officers in the online education space:
- The switch to the on-demand format attracted a set of users who were more likely to pay, but were less engaged in the course. On-demand content is potentially helpful at bringing in a new user segment or expanding the current user base, similar to universities offering concurrent hybrid MBAs that cater to busy students with full-time jobs. Managers must consider the trade-off between offering structure versus flexibility and may even consider offering different content release options simultaneously but at different price points by emphasizing their unique features.
- Platforms may need to adapt their content to account for users who binge on content and others who space it out over time. For example, firms can include more recaps or reviews to reduce frustration resulting from users forgetting content. It may even be a viable strategy to embrace the prevalence of binging among users by highlighting or designing sets of lectures that are "bingeable" versus more modular.
- Many online platforms offer episodic content that may be released in installments and thus need to make decisions regarding the content release format.
"Our study provides insights that help managers anticipate the potential consequences of such decisions," says Hutchinson. "On-demand content offers clear short-term benefits in terms of increased revenue but potentially long-term costs in terms of decreased engagement and new challenges in maintaining user engagement."
Journal information: Journal of Marketing
Provided by American Marketing Association
Explore further
Feedback to editors

New phononics materials may lead to smaller, more powerful wireless devices

New 'forever chemical' cleanup strategy discovered
2 hours ago

TESS discovers a rocky planet that glows with molten lava as it's squeezed by its neighbors

Ultrasound experiment identifies new superconductor
3 hours ago

Quantum breakthrough sheds light on perplexing high-temperature superconductors

How climate change will affect malaria transmission
5 hours ago

Topological phonons: Where vibrations find their twist

Looking for life on Enceladus: What questions should we ask?

Analysis of millions of posts shows that users seek out echo chambers on social media

NASA images help explain eating habits of massive black hole
Relevant physicsforums posts, physics instructor minimum education to teach community college.
May 6, 2024
Studying "Useful" vs. "Useless" Stuff in School
Apr 30, 2024
Why are Physicists so informal with mathematics?
Apr 29, 2024
Plagiarism & ChatGPT: Is Cheating with AI the New Normal?
Apr 28, 2024
Digital oscilloscope for high school use
Apr 25, 2024
Motivating high school Physics students with Popcorn Physics
Apr 3, 2024
More from STEM Educators and Teaching
Related Stories

A mix of in-person and online learning may boost student performance, reduce anxiety
Jun 20, 2018

Facebook boosts 'stories' format, now open to ads
Sep 26, 2018

TikTok rivals Twitter with new text format
Jul 24, 2023

Cross-cultural TikTok study offers insights into user behavior and motivations
Feb 13, 2024

Study highlights benefits of user-generated content to digital platform
Dec 12, 2023

Google takes on Snapchat with its own 'Stories' format
Feb 13, 2018
Recommended for you

Investigation reveals varied impact of preschool programs on long-term school success
May 2, 2024

Training of brain processes makes reading more efficient
Apr 18, 2024

Researchers find lower grades given to students with surnames that come later in alphabetical order
Apr 17, 2024

Earth, the sun and a bike wheel: Why your high-school textbook was wrong about the shape of Earth's orbit
Apr 8, 2024

Touchibo, a robot that fosters inclusion in education through touch
Apr 5, 2024

More than money, family and community bonds prep teens for college success: Study
Let us know if there is a problem with our content.
Use this form if you have come across a typo, inaccuracy or would like to send an edit request for the content on this page. For general inquiries, please use our contact form . For general feedback, use the public comments section below (please adhere to guidelines ).
Please select the most appropriate category to facilitate processing of your request
Thank you for taking time to provide your feedback to the editors.
Your feedback is important to us. However, we do not guarantee individual replies due to the high volume of messages.
E-mail the story
Your email address is used only to let the recipient know who sent the email. Neither your address nor the recipient's address will be used for any other purpose. The information you enter will appear in your e-mail message and is not retained by Phys.org in any form.
Newsletter sign up
Get weekly and/or daily updates delivered to your inbox. You can unsubscribe at any time and we'll never share your details to third parties.
More information Privacy policy
Donate and enjoy an ad-free experience
We keep our content available to everyone. Consider supporting Science X's mission by getting a premium account.
E-mail newsletter
Purdue Online Writing Lab Purdue OWL® College of Liberal Arts
Welcome to the Purdue Online Writing Lab
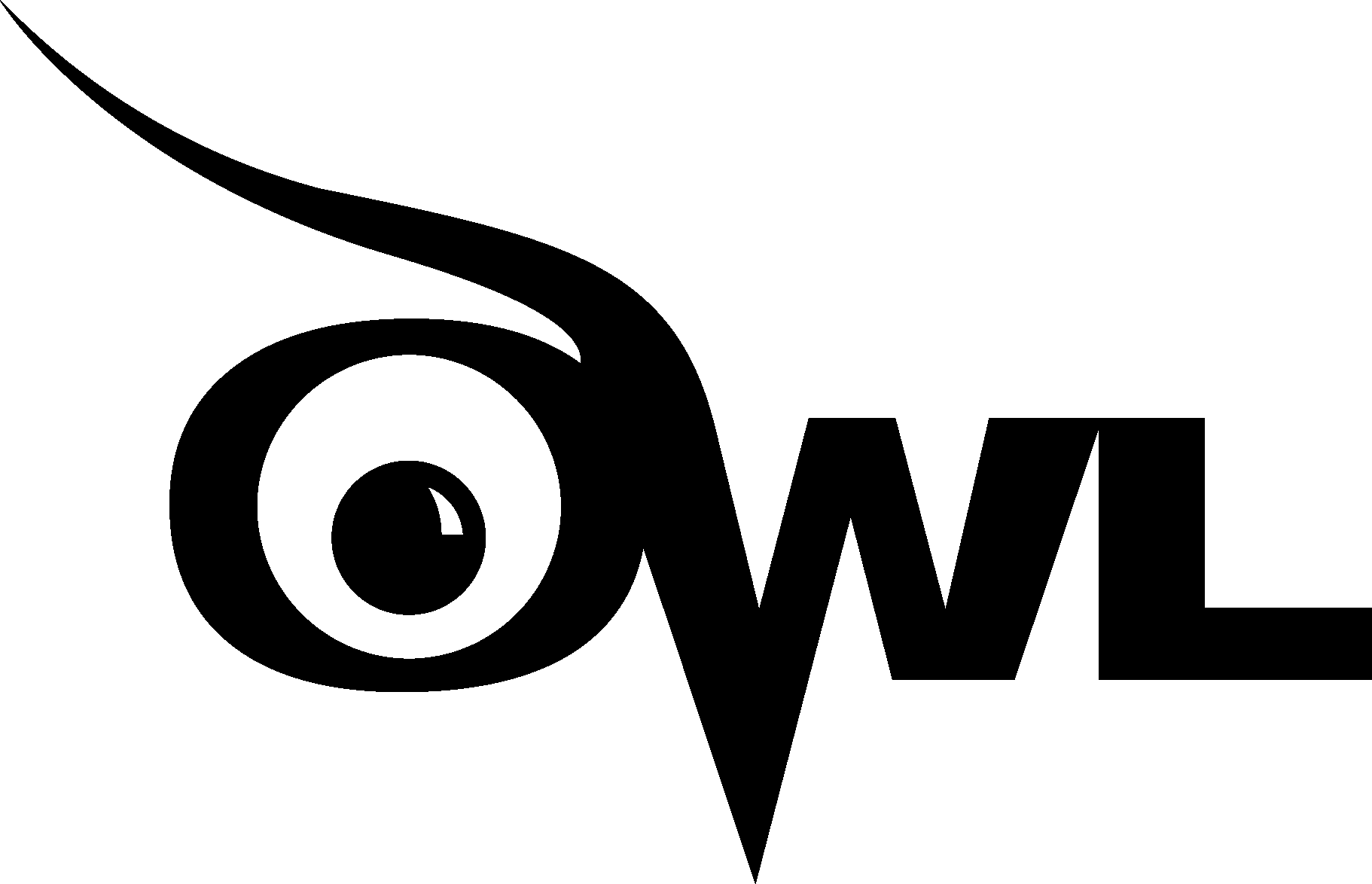
Welcome to the Purdue OWL
This page is brought to you by the OWL at Purdue University. When printing this page, you must include the entire legal notice.
Copyright ©1995-2018 by The Writing Lab & The OWL at Purdue and Purdue University. All rights reserved. This material may not be published, reproduced, broadcast, rewritten, or redistributed without permission. Use of this site constitutes acceptance of our terms and conditions of fair use.
The Online Writing Lab at Purdue University houses writing resources and instructional material, and we provide these as a free service of the Writing Lab at Purdue. Students, members of the community, and users worldwide will find information to assist with many writing projects. Teachers and trainers may use this material for in-class and out-of-class instruction.
The Purdue On-Campus Writing Lab and Purdue Online Writing Lab assist clients in their development as writers—no matter what their skill level—with on-campus consultations, online participation, and community engagement. The Purdue Writing Lab serves the Purdue, West Lafayette, campus and coordinates with local literacy initiatives. The Purdue OWL offers global support through online reference materials and services.
A Message From the Assistant Director of Content Development
The Purdue OWL® is committed to supporting students, instructors, and writers by offering a wide range of resources that are developed and revised with them in mind. To do this, the OWL team is always exploring possibilties for a better design, allowing accessibility and user experience to guide our process. As the OWL undergoes some changes, we welcome your feedback and suggestions by email at any time.
Please don't hesitate to contact us via our contact page if you have any questions or comments.
All the best,
Social Media
Facebook twitter.
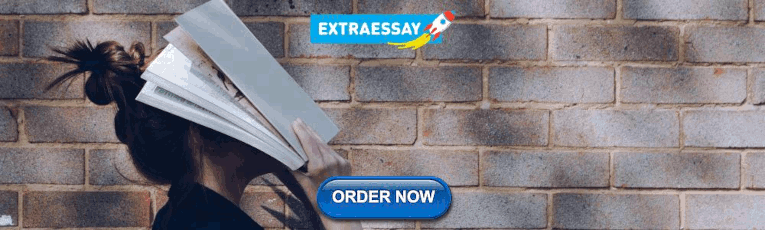
IMAGES
VIDEO
COMMENTS
This research aims to explore and investigate potential factors influencing students' academic achievements and satisfaction with using online learning platforms. This study was constructed based on Transactional Distance Theory (TDT) and Bloom's Taxonomy Theory (BTT). This study was conducted on 243 students using online learning platforms in higher education. This research utilized a ...
The coronavirus pandemic has forced students and educators across all levels of education to rapidly adapt to online learning. The impact of this — and the developments required to make it work ...
The purpose of this study is to analyze the effect of online education, which has been extensively used on student achievement since the beginning of the pandemic. In line with this purpose, a meta-analysis of the related studies focusing on the effect of online education on students' academic achievement in several countries between the years 2010 and 2021 was carried out. Furthermore, this ...
Feature papers represent the most advanced research with significant potential for high impact in the field. A Feature Paper should be a substantial original Article that involves several techniques or approaches, provides an outlook for future research directions and describes possible research applications. ... The online learning platform ...
This paper analyzes the difficulties faced by the students and teachers in online teaching learning process during the COVID-19 pandemic. Online learning is an alternative platform that replaced ...
E-learning behaviour data refers to the data generated by learners in various behavioural activities performed on e-learning platforms or online ... research hotspot in e-learning. This paper ...
This systematic analysis examines effectiveness research on online and blended learning from schools, particularly relevant during the Covid-19 pandemic, and also educational games, computer-supported cooperative learning (CSCL) and computer-assisted instruction (CAI), largely used in schools but with potential for outside school.
BackgroundThe effectiveness of online learning in higher education during the COVID-19 pandemic period is a debated topic but a systematic review on this topic is absent.MethodsThe present study implemented a systematic review of 25 selected articles to comprehensively evaluate online learning effectiveness during the pandemic period and identify factors that influence such effectiveness ...
actions within online learning environments which influence their academic perform-ance and satisfaction. Thus, this developed model must be as a guidance for instructors and decision makers in the online education industry in terms of using online platforms to improve students learning experience through online platforms. Bearing in mind
UNESCO report (2020) gives some interesting numbers on how much the pandemic has affected the education system globally. The study indicates that 60.9% of the enrolled student population has been affected with over 1 billion student learners affected and 107 nationwide closures (as of July 2020).
Online teaching-learning methods have been followed by world-class universities for more than a decade to cater to the needs of students who stay far away from universities/colleges. But during the COVID-19 pandemic period, online teaching-learning helped almost all universities, colleges, and affiliated students. An attempt is made to find the effectiveness of online teaching-learning ...
Research Methodology. The study is descriptive and tries to understand the importance of online learning in the period of a crisis and pandemics such as the Covid-19. The problems associated with online learning and possible solutions were also identified based on previous studies.
Tallent-Runnels et al. (2006) reviewed research late 1990's to early 2000's, Berge and Mrozowski (2001) reviewed research 1990 to 1999, and Zawacki-Richter et al. (2009) reviewed research in 2000-2008 on distance education and online learning. Table 1 shows the research themes from previous systematic reviews on online learning research.
Online learning platforms play an important role in modern education. However, they may not be sufficiently represented in educational institutions. In this regard, the research objectives are set ...
Further, it aims at providing solutions that would help enhance the e-learning experience not only in pandemic times but also in the long run.,This holistic research begins with a comprehensive comparative study about the available e-learning platforms, followed by a primary data analysis through an online survey of 364 engineering students ...
Accordingly, faculty members have been given online learning training on how to implement online teaching by online teaching experts through the university's electronic platforms to teach students remotely through the Internet (Rucker & Frass, 2017). This study aims to analyze the implications of the shift to online learning from a faculty ...
The purpose of this study is to investigate students' intention to continue using online learning platforms during face-to-face traditional classes in a way that is parallel to their usage during online virtual classes (during the pandemic). This investigation of students' intention is based on a conceptual model that uses newly used external factors in addition to the technology ...
1.1. Related literature. Online learning is a form of distance education which mainly involves internet‐based education where courses are offered synchronously (i.e. live sessions online) and/or asynchronously (i.e. students access course materials online in their own time, which is associated with the more traditional distance education).
The COVID-19 pandemic has led to the largest interruption in education around the world, affecting some 1.6 billion students. Classroom education stopped, to be replaced by online e-learning platforms. In higher education, e-learning is made available through recorded lectures, with online platforms becoming a significant part of the overall system. This study aims to identify the quality of ...
The article presents some challenges faced by teachers and learners, supplemented with the recommendations to remove them. JEL Code: A20. The COVID-19 pandemic has led to an expansion in the demand for online teaching and learning across the globe. Online teaching and learning is attracting many students for enhanced learning experiences.
Even though online learning research has been advancing in uncovering student experiences in various settings (i.e., tertiary, adult, and professional education), very little progress has been achieved in understanding the experience of the K‐12 student population, especially when narrowed down to different school‐year segments (i.e ...
online classes could affect the academic performance of students. This paper seeks to study the. impact of online learning on the academic performance of university students and to determine. whether education systems should increase the amount of online learning for traditional in-class. subjects.
The data. Twitter was chosen as the data source. It is one of the world's major social media platforms, with 237.8 million active users in July 2022, 53 and it is also a common source of text ...
1. 90% students have started the online mode of learning and 74% professors have started the. online mode of teaching, during this pandem ic. 2. 84 % of students understand the concepts taught to ...
Researchers from Carnegie Mellon University and University of Pennsylvania have published a new Journal of Marketing study that examines online educational platforms and the question of whether ...
Semantic Scholar extracted view of "Enhancing network intrusion detection by lifelong active online learning" by Po-Jen Chuang et al. Skip to search ... Showing 1 through 3 of 0 Related Papers. 4 References; ... Semantic Scholar is a free, AI-powered research tool for scientific literature, based at the Allen Institute for AI. Learn More. About
The incessant evolution of online platforms has ushered in a multitude of shopping modalities. Within the food industry, however, assessing the delectability of meals can only be tentatively determined based on consumer feedback encompassing aspects such as taste, pricing, packaging, service quality, delivery timeliness, hygiene standards, and environmental considerations. Traditional text ...
From seeking to learning: Understanding contextual factors associated with users' self-regulated learning on medical Q&A platforms. Ye Chen, Xiaoyu Chen, Liyu Li, Shaoxiong Fu. Article 101297 ... Footed in the information deep: Research frameworks for (re)claiming and (re) ...
In order to do that, the IS group helps organizations to: (i) understand the business needs and value propositions and accordingly design the required business and information system architecture; (ii) design, implement, and improve the operational processes and supporting (information) systems that address the business need, and (iii) use advanced data analytics methods and techniques to ...
The Online Writing Lab at Purdue University houses writing resources and instructional material, and we provide these as a free service of the Writing Lab at Purdue. Students, members of the community, and users worldwide will find information to assist with many writing projects.