- Open access
- Published: 15 September 2016
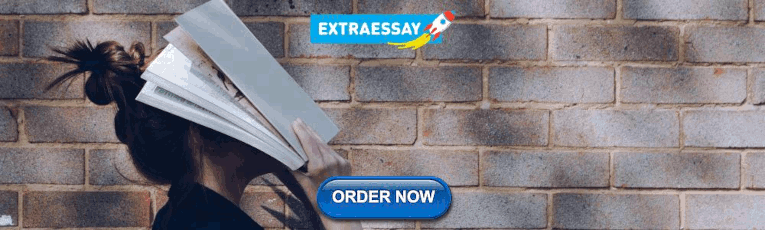
An empirical research on customer satisfaction study: a consideration of different levels of performance
- Yu-Cheng Lee 1 ,
- Yu-Che Wang 2 ,
- Shu-Chiung Lu 3 , 4 ,
- Yi-Fang Hsieh 6 ,
- Chih-Hung Chien 3 , 5 ,
- Sang-Bing Tsai 7 , 8 , 9 , 10 , 11 , 12 &
- Weiwei Dong 13
SpringerPlus volume 5 , Article number: 1577 ( 2016 ) Cite this article
22k Accesses
31 Citations
Metrics details
Customer satisfaction is the key factor for successful and depends highly on the behaviors of frontline service providers. Customers should be managed as assets, and that customers vary in their needs, preferences, and buying behavior. This study applied the Taiwan Customer Satisfaction Index model to a tourism factory to analyze customer satisfaction and loyalty. We surveyed 242 customers served by one tourism factory organizations in Taiwan. A partial least squares was performed to analyze and test the theoretical model. The results show that perceived quality had the greatest influence on the customer satisfaction for satisfied and dissatisfied customers. In addition, in terms of customer loyalty, the customer satisfaction is more important than image for satisfied and dissatisfied customers. The contribution of this paper is to propose two satisfaction levels of CSI models for analyzing customer satisfaction and loyalty, thereby helping tourism factory managers improve customer satisfaction effectively. Compared with traditional techniques, we believe that our method is more appropriate for making decisions about allocating resources and for assisting managers in establishing appropriate priorities in customer satisfaction management.
Traditional manufacturing factories converted for tourism purposes, have become a popular leisure industry in Taiwan. The tourism factories has experienced significant growth in recent years, and more and more tourism factories emphasized service quality improvement, and customized service that contributes to a tourism factory’s image and competitiveness in Taiwan (Wu and Zheng 2014 ). Therefore, tourism factories has become of greater economic importance in Taiwan. By becoming a tourism factory, companies can establish a connection between consumers and the brand, generate additional income from entrance tickets and on-site sales, and eventually add value to service innovations (Tsai et al. 2012 ). Because of these incentives, the Taiwanese tourism factory industry has become highly competitive. Customer satisfaction is seen as very important in this case.
Numerous empirical studies have indicated that service quality and customer satisfaction lead to the profitability of a firm (Anderson et al. 1994 ; Eklof et al. 1999 ; Ittner and Larcker 1996 ; Fornell 1992 ; Anderson and Sullivan 1993 ; Zeithaml 2000 ). Anderson and Sullivan ( 1993 ) stated that a firm’s future profitability depends on satisfying current customers. Anderson et al. ( 1994 ) found a significant relationship between customer satisfaction and return on assets. High quality leads to high levels of customer retention, increase loyalty, and positive word of mouth, which in turn are strongly related to profitability (Reichheld and Sasser 1990 ). In a tourism factory setting, customer satisfaction is the key factor for successful and depends highly on the behaviors of frontline service providers. Kutner and Cripps ( 1997 ) indicated that customers should be managed as assets, and that customers vary in their needs, preferences, buying behavior, and price sensitivity. A tourism factory remains competitive by increasing its service quality relative to that of competitors. Delivering superior customer value and satisfaction is crucial to firm competitiveness (Kotler and Armstrong 1997 ; Weitz and Jap 1995 ; Deng et al. 2013 ). It is crucial to know what customers value most and helps firms allocating resource utilization for continuously improvement based on their needs and wants. The findings of Customer Satisfaction Index (CSI) studies can serve as predictors of a company’s profitability and market value (Anderson et al. 1994 ; Eklof et al. 1999 ; Chiu et al. 2011 ). Such findings provide useful information regarding customer behavior based on a uniform method of customer satisfaction, and offer a unique opportunity to test hypotheses (Anderson et al. 1997 ).
The basic structure of the CSI model has been developed over a number of years and is based on well-established theories and approaches to consumer behavior, customer satisfaction, and product and service quality in the fields of brands, trade, industry, and business (Fornell 1992 ; Fornell et al. 1996 ). In addition, the CSI model leads to superior reliability and validity for interpreting repurchase behavior according to customer satisfaction changes (Fornell 1992 ). These CSIs are fundamentally similar in measurement model (i.e. causal model), they have some obvious distinctions in model’s structure and variable’s selection. Take full advantages of other nations’ experiences can establish the Taiwan CSI Model which is suited for Taiwan’s characters. Thus, the ACSI and ECSI have been used as a foundation for developing the Taiwan Customer Satisfaction Index (TCSI). The TCSI was developed by Chung Hua University and the Chinese Society for Quality in Taiwan. The TCSI provides Taiwan with a fair and objective index for producing vital information that can help the country, industries, and companies improve competitiveness. Every aspect of the TCSI that influences overall customer satisfaction can be measured through surveys, and every construct has a cause–effect relationship with the other five constructs (Fig. 1 ). The relationships among the different aspects of the TCSI are different from those of the ACSI, but are the same as those of the ECSI (Lee et al. 2005 , 2006 ).
The Taiwan Customer Satisfaction Index model
The traditional CSI model for measuring customer satisfaction and loyalty is restricted and does not consider the performance of firms. Moreover, as theoretical and empirical research has shown, the relationship between attribute-level performance and overall satisfaction is asymmetric. If the asymmetries are not considered, the impact of the different attributes on overall satisfaction is not correctly evaluated (Anderson and Mittal 2000 ; Matzler and Sauerwein 2002 ; Mittal et al. 1998 ; Matzler et al. 2003 , 2004 ). Few studies have investigated CSI models that contain different levels of performance (satisfaction), especially in relation to satisfaction levels of a tourism factory. To evaluate overall satisfaction accurately, the impact of the different levels of performance should be considered (Matzler et al. 2004 ). The purpose of this study is to apply the TCSI model that contains different levels of performance to improve and ensure the understanding of firm operational efficiency by managers in the tourism factory. A partial least squares (PLS) was performed to test the theoretical model due to having been successfully applied to customer satisfaction analysis. The PLS is well suited for predictive applications (Barclay et al. 1995 ) and using path coefficients that regard the reasons for customer satisfaction or dissatisfaction and providing latent variable scores that could be used to report customer satisfaction scores. Our findings provide support for the application of TCSI model to derive tourist satisfaction information.
Literature review
National customer satisfaction index (csi).
The CSI model includes a structural equation with estimated parameters of hidden categories and category relationships. The CSI can clearly define the relationships between different categories and provide predictions. The basic CSI model is a structural equation model with latent variables which are calculated as weighted averages of their measurement variables, and the PLS estimation method calculates the weights and provide maximum predictive power of the ultimate dependent variable (Kristensen et al. 2001 ). Many scholars have identified the characteristics of the CSI (Karatepe et al. 2005 ; Malhotra et al. 1994 ).
Although the core of the models are in most respects standard, they have some obvious distinctions in model’s structure and variable’s selection so that their results cannot be compared with each other and some variations between the SCSB (Swedish), the ACSI (American), the ECSI (European), the NCSB (Norwegian) and other indices. For example, the image factor is not employed in the ACSI model (Johnson et al. 2001 ); the NCSB eliminated customer expectation and replaced with corporate image; the ECSI model does not include the customer complaint as a consequence of satisfaction. Many scholars have identified the characteristics of the CSI (Karatepe et al. 2005 ; Malhotra et al. 1994 ). The ECSI model distinguishes service quality from product quality (Kristensen et al. 2001 ) and the NCSB model applies SERVQUAL instrument to evaluate service quality (Johnson et al. 2001 ). A quality measure of a single customer satisfaction index is typically developed according to a certain type of culture or the culture of a certain country. When developing a system for measuring or evaluating a certain country or district’s customer satisfaction level, a specialized customer satisfaction index should be developed.
As such, the ACSI and ECSI were used as a foundation to develop the TCSI. The TCSI was developed by Chung Hua University and the Chinese Society for Quality. Every aspect of the TCSI that influences overall customer satisfaction can be measured through surveys, and every construct has a cause–effect relationship with the other five constructs. The TCSI assumes that currently: (1) Taiwan corporations have ability of dealing with customer complaints; customer complaints have already changed from a factor that influences customer satisfaction results to a factor that affects quality perception; (2) The expectations, satisfaction and loyalty of customers are affected by the image of the corporation. The concept that customer complaints are not calculated into the TCSI model is that they were removed based on the ECSI model (Lee et al. 2005 , 2006 , 2014a , b ; Guo and Tsai 2015 ; Tsai et al. 2015a , b ; 2016a ).
TCSI model and service quality
Service quality is frequently used by both researchers and practitioners to evaluate customer satisfaction. It is generally accepted that customer satisfaction depends on the quality of the product or service offered (Anderson and Sullivan 1993 ). Numerous researchers have emphasized the importance of service quality perceptions and their relationship with customer satisfaction by applying the NCSI model (e.g., Ryzin et al. 2004 ; Hsu 2008 ; Yazdanpanah et al. 2013 ; Chiu et al. 2011 ; Temizer and Turkyilmaz 2012 ; Mutua et al. 2012 ; Dutta and Singh 2014 ). Ryzin et al. ( 2004 ) applied the ACSI to U.S. local government services and indicated that the perceived quality of public schools, police, road conditions, and subway service were the most salient drivers of satisfaction, but that the significance of each service varied among income, race, and geography. Hsu ( 2008 ) proposed an index for online customer satisfaction based on the ACSI and found that e-service quality was more determinative than other factors (e.g., trust and perceived value) for customer satisfaction. To deliver superior service quality, an online business must first understand how customers perceive and evaluate its service quality. This study developed a basic model for using the TCSI to analyze Taiwan’s tourism factory services. The theoretical model comprised 14 observation variables and the following six constructs: image, customer expectations, perceived quality, perceived value, customer satisfaction, and loyalty.
Research methods
The measurement scale items for this study were primarily designed using the questionnaire from the TCSI model. In designing the questionnaire, a 10-point Likert scale (with anchors ranging from strongly disagree to strongly agree) was used to reduce the statistical problem of extreme skewness (Fornell et al. 1996 ; Qu et al. 2015 ; Tsai 2016 ; Tsai et al. 2016b ; Zhou et al. 2016 ). A total of 14 items, organized into six constructs, were included in the questionnaire. The primary questionnaire was pretested on 30 customers who had visited a tourism factory. Because the TCSI model is preliminary research in the tourism factory, this study convened a focus group to decide final attributes of model. The focus group was composed of one manager of tourism factory, one professor in Hospitality Management, and two customers with experience of tourism factory.
We used the TCSI model (Fig. 1 ) to structure our research. From this structure and the basic theories of the ACSI and ECSI, we established the following hypotheses:
Image has a strong influence on tourist expectations.
Image has a strong influence on tourist satisfaction.
Image has a strong influence on tourist loyalty.
Tourist expectations have a strong influence on perceived quality.
Tourist expectations have a strong influence on perceived values.
Tourist expectations have a strong influence on tourist satisfaction.
Perceived quality has a strong influence on perceived value.
Perceived quality has a strong influence on tourist satisfaction.
Perceived value has a strong influence on tourist satisfaction.
Customer satisfaction has a strong influence on tourist loyalty.
The content of our surveys were separated into two parts; customer satisfaction and personal information. The definitions and processing of above categories are listed below:
Part 1 of the survey assessed customer satisfaction by measuring customer levels of tourism factory image, expectations, quality perceptions, value perceptions, satisfaction, and loyalty toward their experience, and used these constructs to indirectly survey the customer’s overall evaluation of the services provided by the tourism factory.
Part 2 of the survey collected personal information: gender, age, family situation, education, income, profession, and residence.
The six constructs are defined as follows:
Image reflects the levels of overall impression of the tourism factory as measured by two items: (1) word-of-mouth reputation, (2) responsibility toward concerned parties that the tourist had toward the tourism factory before traveling.
Customer expectations refer to the levels of overall expectations as measured by two items: (1) expectations regarding the service of employees, (2) expectations regarding reliability that the tourist had before the experience at the tourism factory.
Perceived quality was measured using three survey measures: (1) the overall evaluation, (2) perceptions of reliability, (3) perceptions of customization that the tourist had after the experience at the tourism factory.
Perceived value was measured using two items: (1) the cost in terms of money and time (2) a comparison with other tourism factories.
Customer satisfaction represents the levels of overall satisfaction was captured by two items: (1) meeting of expectations, (2) closeness to the ideal tourism factory.
Loyalty was measured using three survey measures: (1) the probabilities of visiting the tourism factory again (2) attending another activity held by the tourism factory, (3) recommending the tourism factory to others.
Data collection and analysis
The survey sites selected for this study was the parking lots of one food tourism factory in Taipei, Taiwan. A domestic group package and individual tourists were a major source of respondents who were willing to participate in the survey and completed the questionnaires themselves based on their perceptions of their factory tour experience. Four research assistants were trained to conduct the survey regarding to questionnaire distribution and sampling.
To minimize prospective biases of visiting patterns, the survey was conducted at different times of day and days of week—Tuesday, Thursday, Saturday for the first week; Monday, Wednesday, Friday and Sunday for the next week. The afternoon time period was used first then the morning time period in the following weeks. The data were collected over 1 month period.
Of 300 tourists invited to complete the questionnaire, 242 effective responses were obtained (usable response rate of 80.6 %). The sample of tourists contained more females (55.7 %) than males (44.35 %). More than half of the respondents had a college degree or higher, 28 % were students, and 36.8 % had an annual household income of US $10,000–$20,000. The majority of the respondents (63.7 %) were aged 20–40 years.
Comparison of the TCSI models for satisfied and dissatisfied customers
Researchers have claimed that satisfaction levels differ according to gender, age, socioeconomic status, and residence (Bryant and Cha 1996 ). Moreover, the needs, preferences, buying behavior, and price sensitivity of customers vary (Kutner and Cripps 1997 ). Previous studies have demonstrated that it is crucial to measure the relative impact of each attribute for high and low performance (satisfaction) (Matzler et al. 2003 , 2004 ). To determine the reasons for differences, a satisfaction scale was used to group the sample into satisfied (8–10) and dissatisfied (1–7) customers.
The research model was tested using SmartPLS 3.0 software, which is suited for highly complex predictive models (Wold 1985 ; Barclay et al. 1995 ). In particular, it has been successfully applied to customer satisfaction analysis. The PLS method is a useful tool for obtaining indicator weights and predicting latent variables and includes estimating path coefficients and R 2 values. The path coefficients indicate the strengths of the relationships between the dependent and independent variables, and the R 2 values represent the amount of variance explained by the independent variables. Using Smart PLS, we determined the path coefficients. Figures 2 and 3 show ten path estimates corresponding to the ten research hypothesis of TCSI model for satisfied and dissatisfied customers. Every path coefficient was obtained by bootstrapping the computation of R 2 and performing a t test for each hypothesis. Fornell et al. ( 1996 ) demonstrated that the ability to explain the influential latent variables in a model is an indicator of model performance, in particular the customer satisfaction and customer loyalty variables. From the results shown, the R 2 values for the customer satisfaction were 0.53 vs. 0.50, respectively; and the R 2 value for customer loyalty were 0.64 vs. 0.60, respectively. Thus, the TCSI model explained 53 vs. 50 % of the variance in customer satisfaction; 64 vs. 60 % of that in customer loyalty as well.
Path estimate of the TCSI model for satisfied customers. *p < 0.05; **p < 0.01; ***p < 0.001
Path estimate of the TCSI model for dissatisfied customers. *p < 0.05; **p < 0.01; ***p < 0.001
According to the path coefficients shown in Figs. 2 and 3 , image positively affected customer expectations (β = 0.58 vs. 0.37), the customer satisfaction (β = 0.16 vs. 0.11), and customer loyalty (β = 0.47 vs. 0.16). Therefore, H1–H3 were accepted. Customer expectations were significantly related to perceived quality (β = 0.94 vs. 0.83). However, customer expectations were not significantly related to perceived value shown as dotted line (β = −0.01 vs. −0.20) or the customer satisfaction, shown as dotted line (β = −0.21 vs. −0.32). Thus, H4 was accepted but H5 and H6 were not accepted. Perceived value positively affected the customer satisfaction (β = 0.27 vs. 0.14), supporting H7. Accordingly, the analysis showed that each of the antecedent constructs had a reasonable power to explain the overall customer satisfaction. Furthermore, perceived quality positively affected the customer satisfaction (β = 0.70 vs. 0.62), as did perceived value (β = 0.83 vs. 0.74). These results confirm H8 and H9. The path coefficient between the customer satisfaction and customer loyalty was positive and significant (β = 0.63 vs. 0.53). This study tested the suitability of two TCSI models by analyzing the tourism factories in Taiwan. The results showed that the TCSI models were all close fit for this type of research. This study provides empirical evidence of the causal relationships among perceived quality, image, perceived value, perceived expectations, customer satisfaction, and customer loyalty.
To observe the effects of antecedent constructs of perceived value (e.g., customer expectation and perceived quality), customer expectations were not significantly related to perceived value for either satisfied or dissatisfied customers. Furthermore, satisfied customers were affected more by perceived quality (β = 0.83 vs. 0.74), as shown in Table 1 . Regarding the effect of the antecedents of customer satisfaction (e.g., image, customer expectations, perceived value and perceived quality), the total effects of perceived quality on the customer satisfaction of satisfied and dissatisfied customers were 0.92 and 0.72. The total effects of image on the customer satisfaction of satisfied and dissatisfied customers were 0.45 and 0.19. Thus, the satisfaction level of satisfied customers was affected more by perceived quality. Consequently, regarding customer satisfaction, perceived quality is more important than image for satisfied and dissatisfied customers. Numerous researchers have emphasized the importance of service quality perceptions and their relationship with customer satisfaction by applying the CSI model (e.g., Ryzin et al. 2004 ; Hsu 2008 ; Yazdanpanah et al. 2013 ; Chiu et al. 2011 ; Temizer and Turkyilmaz 2012 ; Mutua et al. 2012 ; Dutta and Singh 2014 ). This is consistent with the results of previous research ( O’Loughlin and Coenders 2002 ; Yazdanpanah et al. 2013 ; Chiu et al. 2011 ; Chin and Liu 2015 ; Chin et al. 2016 ).
With respect to the effect of the antecedents of customer loyalty (e.g., image and customer satisfaction), the total effects of image on customer loyalty for satisfied and dissatisfied customers were 0.57 and 0.21. In other words, the customer loyalty of satisfied customers was affected more by customer satisfaction. Customer satisfaction was significantly related to the customer loyalty of both satisfied and dissatisfied customers, and satisfied customers were affected more by customer satisfaction ( β = 0.63 vs. 0.14). Consequently, regarding customer loyalty, customer satisfaction is more important than image for both satisfied and dissatisfied customers. Numerous studies have shown that customer satisfaction is a crucial factor for ensuring customer loyalty (Barsky 1992 ; Smith and Bolton 1998 ; Hallowell 1996 ; Grønholdt et al. 2000 ). This study empirically supports the notion that customer satisfaction is positively related to customer loyalty.
The TCSI model has a predictive capability that can help tourism factory managers improve customer satisfaction based on different performance levels. Our model enables managers to determine the specific factors that significantly affect overall customer satisfaction and loyalty within a tourism factory. This study also helps managers to address different customer segments (e.g., satisfied vs. dissatisfied); because the purchase behaviors of customers differ, they must be treated differently. The contribution of this paper is to propose two satisfaction levels of CSI models for analyzing customer satisfaction and loyalty, thereby helping tourism factory managers improve customer satisfaction effectively.
Fornell et al. ( 1996 ) demonstrated that the ability to explain influential latent variables in a model, particularly customer satisfaction and customer loyalty variables, is an indicator of model performance. However, the results of this study indicate that customer expectations were not significantly related to perceived value for either satisfied or dissatisfied customers. Moreover, they were affected more by perceived quality of customer satisfaction. Numerous researchers have found that the construct of customer expectations used in the ACSI model does not significantly affect the level of customer satisfaction (Johnson et al. 1996 , 2001 ; Martensen et al. 2000 ; Anderson and Sullivan 1993 ).
Through the overall effects, this study derived several theoretical findings. First, the factors with the largest influence on customer satisfaction were perceived quality and perceived expectations, despite the results showing that customer expectations were not significantly related to perceived value or customer satisfaction. Hence, customer expectations indirectly affected customer satisfaction through perceived quality. Accordingly, perceived quality had the greatest influence on customer satisfaction. Likewise, our results also show that satisfied customers were affected more by perceived quality than dissatisfied customers. This study determined that perceived quality, whether directly or indirectly, positively influenced customer satisfaction. This result is consistent with those of Cronin and Taylor ( 1992 ), Cronin et al. ( 2000 ), Hsu ( 2008 ), Ladhari ( 2009 ), Terblanche and Boshoff ( 2010 ), Deng et al. ( 2013 ), and Yazdanpanah et al. ( 2013 ).
Second, the factors with the most influence on customer loyalty were image and customer satisfaction. The results of this study demonstrate that the customer loyalty of satisfied customers was affected more by customer satisfaction. Consequently, regarding customer loyalty, customer satisfaction is more important than image for satisfied customers. Lee ( 2015 ) found that higher overall satisfaction increased the possibility that visitors will recommend and reattend tourism factory activities. Moreover, numerous studies have shown that customer satisfaction is a crucial factor for ensuring customer loyalty (Barsky 1992 ; Smith and Bolton 1998 ; Hallowell 1996 ; Su 2004; Deng et al. 2013 ). In initial experiments on ECSI, corporate image was assumed to have direct influences on customer expectation, satisfaction, and loyalty. Subsequent experiments in Denmark proved that image affected only expectation and satisfaction and had no relationship with loyalty (Martensen et al. 2000 ). In early attempts to build the ECSI model, image was defined as a variable involving not only a company’s overall image but products or brand awareness; thus image is readily connected with customer expectation and perception. Therefore, this study contributes to relevant research by providing empirical support for the notion that customer satisfaction is positively related to customer loyalty.
In addition to theoretical implications, this study has several managerial implications. First, the TCSI model has a satisfactory predictive capability that can help tourism factory managers to examine customer satisfaction more closely and to understand explicit influences on customer satisfaction for different customer segments by assessing the accurate causal relationships involved. In contrast to general customer satisfaction surveys, the TCSI model cannot obtain information on post-purchase customer behavior to improve customer satisfaction and achieve competitive advantage.
Second, this study not only indicated that each of the antecedent constructs had reasonable power to explain customer satisfaction and loyalty but also showed that perceived quality exerts the largest influence on the customer satisfaction of Taiwan’s tourism factory industry. Therefore, continually, Taiwan’s tourism factories must endeavor to enhance their customer satisfaction, ideally by improving service quality. Managers of Taiwan’s tourism factories must ensure that service providers deliver consistently high service quality.
Third, this research determined that the factors having the most influence on customer loyalty were image and customer satisfaction. Therefore, managers of Taiwan’s tourism factories should allow customer expectations to be fulfilled through experiences, thereby raising their overall level of satisfaction. Regarding image, which refers to a brand name and its related associations, when tourists regard a tourism factory as having a positive image, they tend to perceive higher value of its products and services. This leads to a higher level of customer satisfaction and increased chances of customers’ reattending tourism factory activities.
Different performance levels exist in how tourists express their opinions about various aspects of service quality and satisfaction with tourism factories. Customer segments can have different preferences depending on their needs and purchase behavior. Our findings indicate that tourists belonging to different customer segments (e.g., satisfied vs. dissatisfied) expressed differences toward service quality and customer satisfaction. Thus, the management of Taiwan’s tourism factories must notice the needs of different market segments to meet their individual expectations. This study proposes two satisfaction levels of CSI models for analyzing customer satisfaction and loyalty, thereby helping tourism factory managers improve customer satisfaction effectively. Compared with traditional techniques, we believe that our method is more appropriate for making decisions about allocating resources and for assisting managers in establishing appropriate priorities in customer satisfaction management.
Limitations and suggestions for future research
This study has some limitations. First, the tourism factory surveyed in this study was a food tourism factory operating in Taipei, Taiwan, and the present findings cannot be generalized to the all tourism factory industries. Second, the sample size was quite small for tourists (N = 242). Future research should collect a greater number of samples and include a more diverse range of tourists. Third, this study was preliminary research on tourism factories, and domestic group package tourists were a major source of the respondents. Future studies should collect data from international tourists as well.
Anderson EW, Sullivan M (1993) The antecedents and consequences of customer satisfaction for firms. Mark Sci 12:125–143
Article Google Scholar
Anderson EW, Mittal V (2000) Strengthening the satisfaction-profit chain. J Serv Res 3(2):107–120
Anderson EW, Fornell C, Lehmann DR (1994) Customer satisfaction, market share, and profitability: findings from Sweden. J Mark 58:53–66
Anderson EW, Fornell C, Rust RT (1997) Customer satisfaction, productivity, and profitability: differences between goods and services. Mark Sci 16(2):129–145
Barclay DW, Higgins C, Thompson R (1995) Interdepartmental conflict in organizational buying: the impact of the organizational context. J Mark Res 28:145–159
Barsky JD (1992) Customer satisfaction in the hotel industry: meaning and measurement. J Hosp Tour Res 16(1):51–73
Google Scholar
Bryant BE, Cha J (1996) Crossing the threshold. Mark Res 8(4):20
Chin T, Liu RH (2015) Understanding labor conflicts in Chinese manufacturing: A Yin-Yang harmony perspective. Int J Confl Manag 26(3):288–315
Chin T, Liu RH, Yang X (2016) Reverse internationalization in Chinese firms: A study of how global startup OEMs seek to compete domestically. Asia Pac Bus Rev 22(2):201–219. doi: 10.1080/13602381.2015.1055087
Chiu SI, Cheng CC, Yen TM, Hu HY (2011) Preliminary research on customer satisfaction models in Taiwan: a case study from the automobile industry. Expert Syst Appl 38(8):9780–9787
Cronin Jr JJ, Taylor SA (1992) Measuring service quality: a reexamination and extension. J Mark 56(3):55–68
Cronin JJ, Brady MK, Hult GTM (2000) Assessing the effects of quality, value, and customer satisfaction on consumer behavioral intentions in service environments. J Retail 76(2):193–218
Deng WJ, Yeh ML, Sung ML (2013) A customer satisfaction index model for international tourist hotels: integrating consumption emotions into the American customer satisfaction index. Int J Hosp Manag 35:133–140
Dutta K, Singh S (2014). Deriving Customer Satisfaction and Loyalty from Organized Retailer’s Sales Promotion Activities in India. ISSN 2045-810X, 21
Eklof JA, Hackl P, Westlund A (1999) On measuring interactions between customer satisfaction and financial results. Total Qual Manag 10(4–5):514–522
Fornell C (1992) A national customer satisfaction barometer: the swedish experience. J Mark 56 (1):6
Fornell C, Johnson MD, Anderson EW, Cha J, Bryant BE (1996) The American customer satisfaction index: nature, purpose, and findings. J Mark 60:7–18
Grønholdt L, Martensen A, Kristensen K (2000) The relationship between customer satisfaction and loyalty: cross-industry differences. Total Qual Manag 11(4–6):509–514
Guo JJ, Tsai SB (2015) Discussing and evaluating green supply chain suppliers: a case study of the printed circuit board industry in China. S Afr J Ind Eng 26(2):56–67
Hallowell R (1996) The relationships of customer satisfaction, customer loyalty, and profitability: an empirical study. Int J Serv Ind Manag 7(4):27–42
Hsu SH (2008) Developing an index for online customer satisfaction: adaptation of American Customer Satisfaction Index. Expert Syst Appl 34(4):3033–3042
Ittner CD, Larcker DF (1996) Measuring the impact of quality initiatives on firm financial performance. Adv Manag Organ Qual 1(1):1–37
Johnson MD, Nader G, Fornell C (1996) Expectations, perceived performance, and customer satisfaction for a complex service: the case of bank loans. J Econ Psychol 17(2):163–182
Johnson MD et al (2001) The evolution and future of national customer satisfaction index models. J Econ Psychol 22(2):217–245
Karatepe OM, Yavas U, Babakus E (2005) Measuring service quality of banks: scales development and validation. J Retail Consum Serv 12(5):373–383
Kotler P, Armstrong G (1997) Marketing: an introduction. Prentice-Hall, New York
Kristensen K, Juhl HJ, Østergaard P (2001) Customer satisfaction: some results for European retailing. Total Qual Manag 12(7–8):890–897
Kutner S, Cripps J (1997) Managing the customer portfolio of healthcare enterprises. Healthc Forum J 40(5):52–54
CAS PubMed Google Scholar
Ladhari R (2009) Service quality, emotional satisfaction, and behavioural intentions. Manag Serv Qual Int J 19(3):308–331
Lee C-F (2015) Tourist satisfaction with factory tour experience. Int J Cult Tourism Hosp Res 9:3
Lee YC, He LY, Jiang JS, Lian QY (2005) Foundation of the Taiwan customer satisfaction index model. Qual Mag 41(12):52–56
Lee YC, He LY, Jiang JS, Lian QY (2006) The Taiwan customer satisfaction index model in related to sample decision. Qual Mag 42(4):74–77
CAS Google Scholar
Lee YC, Chen CY, Tsai SB, Wang CT (2014a) Discussing green environmental performance and competitive strategies. Pensee 76(7):190–198
Lee YC, Wu CH, Tsai SB (2014b) Grey system theory and fuzzy time series forecasting for the growth of green electronic materials. Int J Prod Res 299(8):1395–1406
Malhotra NK, Ulgado FM, Agarwal J, Baalbaki IB (1994) International service marketing: a comparative evaluation of the dimensions of service quality between developed and developing countries. Int Mark Rev 11(2):5–15
Martensen A, Gronholdt L, Kristensen K (2000) The drivers of customer satisfaction and loyalty: cross-industry findings from Denmark. Total Qual Manag 11(4–6):544–553
Matzler K, Sauerwein E (2002) The factor structure of customer satisfaction: an empirical test of the importance grid and the penalty-reward-contrast analysis. Int J Serv Ind Manag 13(4):314–332
Matzler K, Sauerwein E, Heischmidt K (2003) Importance-performance analysis revisited: the role of the factor structure of customer satisfaction. Serv Ind J 23(2):112–129
Matzler K, Bailom, F, Hinterhuber HH, Renzl B, Pichler J (2004) The asymmetric relationship between attribute-level performance and overall customer satisfaction: a reconsideration of the importance–performance analysis. Ind Mark Manag 33(4):271–277
Mittal V, Ross WT, Baldasare PM (1998) The asymmetric impact of negative and positive attribute-level performance on overall satisfaction and repurchase intentions. J Mark 62:33–47
Mutua J, Ngui D, Osiolo H, Aligula E, Gachanja J (2012) Consumers satisfaction in the energy sector in Kenya. Energy Policy 48:702–710
O’Loughlin C, Coenders G (2002) Application of the european customer satisfaction index to postal services. Structural equation models versus partial least squares, No. 4. Working Papers of the Department of Economics, University of Girona. Department of Economics, University of Girona
Qu Q, Chen KY, Wei YM et al (2015) Using hybrid model to evaluate performance of innovation and technology professionals in marine logistics industry mathematical problems in engineering. Article ID 361275. doi: 10.1155/2015/361275
Reichheld FF, Sasser WE (1990) Zero defections: quality comes to services. Harvard Bus Rev 68(5):105–111
Ryzin GG, Muzzio D, Immerwahr S, Gulick L, Martinez E (2004) Drivers and consequences of citizen satisfaction: an application of the American customer satisfaction index model to New York City. Public Adm Rev 64(3):331–341
Smith AK, Bolton RN (1998) An experimental investigation of customer reactions to service failure and recovery encounters paradox or peril? J Serv Research 1(1):65–81
Temizer L, Turkyilmaz A (2012) Implementation of student satisfaction index model in higher education institutions. Procedia Soc Behav Sci 46:3802–3806
Terblanche NS, Boshoff C (2010) Quality, value, satisfaction and loyalty amongst race groups: A study of customers in the South African fast food industry. S Afr J Bus Manag 41(1):1–9
Tsai SB (2016) Using grey models for forecasting China’s growth trends in renewable energy consumption. Clean Technol Environ Policy 18:563–571
Tsai CH, Peng YJ, Wu HH (2012, October). Evaluating service process satisfaction of a tourism factory—using Brands’ Health Museum as an example. In: 2012 6th international conference on new trends in information science and service science and data mining (ISSDM), pp 244–247
Tsai SB, Chien MF, Xue Y, Li L et al (2015a) Using the fuzzy DEMATEL to determine environmental performance: a case of printed circuit board industry in Taiwan. PLoS ONE 10(6):e0129153. doi: 10.1371/journal.pone.0129153
Article PubMed PubMed Central Google Scholar
Tsai SB, Saito R, Lin YC, Chen Q et al (2015b) Discussing measurement criteria and competitive strategies of green suppliers from a Green law Perspective. Proc Inst Mech Eng B J Eng Manuf 229(S1):135–145
Tsai SB, Huang CY, Wang CK, Chen Q et al (2016a) Using a mixed model to evaluate job satisfaction in high-tech industries. PLoS ONE 11(5):e0154071. doi: 10.1371/journal.pone.0154071
Tsai SB, Xue Y, Zhang J, Chen Q et al (2016b) Models for forecasting growth trends in renewable energy. Renew Sustain Energy Rev. doi: 10.1016/j.rser.2016.06.001
Weitz BA, Jap SD (1995) Relationship marketing and distribution channels. J Acad Mark Sci 23:305–320
Wold H (1985) Partial least squares. In: Kotz S, Johnson N (eds) Encyclopedia of statistical sciences. Wiley, New York, pp 581–591
Wu SI, Zheng YH (2014) The influence of tourism image and activities appeal on tourist loalty–a study of Tainan City in Taiwan. J Manag Strategy, 5(4):121
Yazdanpanah M, Zamani GH, Hochrainer-Stigler S, Monfared N, Yaghoubi J (2013) Measuring satisfaction of crop insurance a modified American customer satisfaction model approach applied to Iranian Farmers. Int J Disaster Risk Reduct 5:19–27
Zeithaml VA (2000) Service quality, profitability, and the economic worth of customers: what we know and what we need to learn. J Acad Mark Sci 28(1):67–85
Zhou J, Wang Q, Tsai SB et al (2016) How to evaluate the job satisfaction of development personnel. IEEE Trans Syst Man Cybern Syst. doi: 10.1109/TSMC.2016.2519860
Download references
Authors’ contributions
Writing: S-CL; providing case and idea: Y-CL, Y-CW, Y-FH, C-HC; providing revised advice: S-BT, WD. All authors read and approved the final manuscript.
Acknowledgements
Department of Technology Management, Chung-Hua University, Hsinchu, Taiwan. This work was supported by University of Electronic Science Technology of China, Zhongshan Institute (414YKQ01 and 415YKQ08).
Competing interests
The authors declare that they have no competing interests.
Author information
Authors and affiliations.
Department of Technology Management, Chung-Hua University, Hsinchu, 300, Taiwan
Yu-Cheng Lee
Department of Business Administration, Chung-Hua University, Hsinchu, 300, Taiwan
Yu-Che Wang
PhD Program of Technology Management, Chung-Hua University, Hsinchu, 300, Taiwan
Shu-Chiung Lu & Chih-Hung Chien
Department of Food and Beverage Management, Lee-Ming Institute of Technology, New Taipei City, 243, Taiwan
Shu-Chiung Lu
Department of Business Administration, Lee-Ming Institute of Technology, New Taipei City, 243, Taiwan
Chih-Hung Chien
Department of Food and Beverage Management, Taipei College of Maritime Technology, New Taipei City, 251, Taiwan
Yi-Fang Hsieh
Zhongshan Institute, University of Electronic Science and Technology of China, Dongguan, 528402, Guangdong, China
Sang-Bing Tsai
School of Economics and Management, Shanghai Maritime University, Shanghai, 201306, China
Law School, Nankai University, Tianjin, 300071, China
School of Business, Dalian University of Technology, Panjin, 124221, China
College of Business Administration, Dongguan University of Technology, Dongguan, 523808, Guangdong, China
Department of Psychology, Universidad Santo Tomas de Oriente y Medio Día, Granada, Nicaragua
School of Economics and Management, Shanghai Institute of Technology, Shanghai, 201418, China
Weiwei Dong
You can also search for this author in PubMed Google Scholar
Corresponding authors
Correspondence to Sang-Bing Tsai or Weiwei Dong .
Rights and permissions
Open Access This article is distributed under the terms of the Creative Commons Attribution 4.0 International License ( http://creativecommons.org/licenses/by/4.0/ ), which permits unrestricted use, distribution, and reproduction in any medium, provided you give appropriate credit to the original author(s) and the source, provide a link to the Creative Commons license, and indicate if changes were made.
Reprints and permissions
About this article
Cite this article.
Lee, YC., Wang, YC., Lu, SC. et al. An empirical research on customer satisfaction study: a consideration of different levels of performance. SpringerPlus 5 , 1577 (2016). https://doi.org/10.1186/s40064-016-3208-z
Download citation
Received : 25 April 2016
Accepted : 02 September 2016
Published : 15 September 2016
DOI : https://doi.org/10.1186/s40064-016-3208-z
Share this article
Anyone you share the following link with will be able to read this content:
Sorry, a shareable link is not currently available for this article.
Provided by the Springer Nature SharedIt content-sharing initiative
- Customer satisfaction
- Tourism factory industry
- Partial least squares
- Business management
- Service management

An official website of the United States government
The .gov means it’s official. Federal government websites often end in .gov or .mil. Before sharing sensitive information, make sure you’re on a federal government site.
The site is secure. The https:// ensures that you are connecting to the official website and that any information you provide is encrypted and transmitted securely.
- Publications
- Account settings
Preview improvements coming to the PMC website in October 2024. Learn More or Try it out now .
- Advanced Search
- Journal List
- Springerplus

An empirical research on customer satisfaction study: a consideration of different levels of performance
Yu-cheng lee.
1 Department of Technology Management, Chung-Hua University, Hsinchu, 300 Taiwan
Yu-Che Wang
2 Department of Business Administration, Chung-Hua University, Hsinchu, 300 Taiwan
Shu-Chiung Lu
3 PhD Program of Technology Management, Chung-Hua University, Hsinchu, 300 Taiwan
4 Department of Food and Beverage Management, Lee-Ming Institute of Technology, New Taipei City, 243 Taiwan
Yi-Fang Hsieh
6 Department of Food and Beverage Management, Taipei College of Maritime Technology, New Taipei City, 251 Taiwan
Chih-Hung Chien
5 Department of Business Administration, Lee-Ming Institute of Technology, New Taipei City, 243 Taiwan
Sang-Bing Tsai
7 Zhongshan Institute, University of Electronic Science and Technology of China, Dongguan, 528402 Guangdong China
8 School of Economics and Management, Shanghai Maritime University, Shanghai, 201306 China
9 Law School, Nankai University, Tianjin, 300071 China
10 School of Business, Dalian University of Technology, Panjin, 124221 China
11 College of Business Administration, Dongguan University of Technology, Dongguan, 523808 Guangdong China
12 Department of Psychology, Universidad Santo Tomas de Oriente y Medio Día, Granada, Nicaragua
Weiwei Dong
13 School of Economics and Management, Shanghai Institute of Technology, Shanghai, 201418 China
Customer satisfaction is the key factor for successful and depends highly on the behaviors of frontline service providers. Customers should be managed as assets, and that customers vary in their needs, preferences, and buying behavior. This study applied the Taiwan Customer Satisfaction Index model to a tourism factory to analyze customer satisfaction and loyalty. We surveyed 242 customers served by one tourism factory organizations in Taiwan. A partial least squares was performed to analyze and test the theoretical model. The results show that perceived quality had the greatest influence on the customer satisfaction for satisfied and dissatisfied customers. In addition, in terms of customer loyalty, the customer satisfaction is more important than image for satisfied and dissatisfied customers. The contribution of this paper is to propose two satisfaction levels of CSI models for analyzing customer satisfaction and loyalty, thereby helping tourism factory managers improve customer satisfaction effectively. Compared with traditional techniques, we believe that our method is more appropriate for making decisions about allocating resources and for assisting managers in establishing appropriate priorities in customer satisfaction management.
Traditional manufacturing factories converted for tourism purposes, have become a popular leisure industry in Taiwan. The tourism factories has experienced significant growth in recent years, and more and more tourism factories emphasized service quality improvement, and customized service that contributes to a tourism factory’s image and competitiveness in Taiwan (Wu and Zheng 2014 ). Therefore, tourism factories has become of greater economic importance in Taiwan. By becoming a tourism factory, companies can establish a connection between consumers and the brand, generate additional income from entrance tickets and on-site sales, and eventually add value to service innovations (Tsai et al. 2012 ). Because of these incentives, the Taiwanese tourism factory industry has become highly competitive. Customer satisfaction is seen as very important in this case.
Numerous empirical studies have indicated that service quality and customer satisfaction lead to the profitability of a firm (Anderson et al. 1994 ; Eklof et al. 1999 ; Ittner and Larcker 1996 ; Fornell 1992 ; Anderson and Sullivan 1993 ; Zeithaml 2000 ). Anderson and Sullivan ( 1993 ) stated that a firm’s future profitability depends on satisfying current customers. Anderson et al. ( 1994 ) found a significant relationship between customer satisfaction and return on assets. High quality leads to high levels of customer retention, increase loyalty, and positive word of mouth, which in turn are strongly related to profitability (Reichheld and Sasser 1990 ). In a tourism factory setting, customer satisfaction is the key factor for successful and depends highly on the behaviors of frontline service providers. Kutner and Cripps ( 1997 ) indicated that customers should be managed as assets, and that customers vary in their needs, preferences, buying behavior, and price sensitivity. A tourism factory remains competitive by increasing its service quality relative to that of competitors. Delivering superior customer value and satisfaction is crucial to firm competitiveness (Kotler and Armstrong 1997 ; Weitz and Jap 1995 ; Deng et al. 2013 ). It is crucial to know what customers value most and helps firms allocating resource utilization for continuously improvement based on their needs and wants. The findings of Customer Satisfaction Index (CSI) studies can serve as predictors of a company’s profitability and market value (Anderson et al. 1994 ; Eklof et al. 1999 ; Chiu et al. 2011 ). Such findings provide useful information regarding customer behavior based on a uniform method of customer satisfaction, and offer a unique opportunity to test hypotheses (Anderson et al. 1997 ).
The basic structure of the CSI model has been developed over a number of years and is based on well-established theories and approaches to consumer behavior, customer satisfaction, and product and service quality in the fields of brands, trade, industry, and business (Fornell 1992 ; Fornell et al. 1996 ). In addition, the CSI model leads to superior reliability and validity for interpreting repurchase behavior according to customer satisfaction changes (Fornell 1992 ). These CSIs are fundamentally similar in measurement model (i.e. causal model), they have some obvious distinctions in model’s structure and variable’s selection. Take full advantages of other nations’ experiences can establish the Taiwan CSI Model which is suited for Taiwan’s characters. Thus, the ACSI and ECSI have been used as a foundation for developing the Taiwan Customer Satisfaction Index (TCSI). The TCSI was developed by Chung Hua University and the Chinese Society for Quality in Taiwan. The TCSI provides Taiwan with a fair and objective index for producing vital information that can help the country, industries, and companies improve competitiveness. Every aspect of the TCSI that influences overall customer satisfaction can be measured through surveys, and every construct has a cause–effect relationship with the other five constructs (Fig. 1 ). The relationships among the different aspects of the TCSI are different from those of the ACSI, but are the same as those of the ECSI (Lee et al. 2005 , 2006 ).

The Taiwan Customer Satisfaction Index model
The traditional CSI model for measuring customer satisfaction and loyalty is restricted and does not consider the performance of firms. Moreover, as theoretical and empirical research has shown, the relationship between attribute-level performance and overall satisfaction is asymmetric. If the asymmetries are not considered, the impact of the different attributes on overall satisfaction is not correctly evaluated (Anderson and Mittal 2000 ; Matzler and Sauerwein 2002 ; Mittal et al. 1998 ; Matzler et al. 2003 , 2004 ). Few studies have investigated CSI models that contain different levels of performance (satisfaction), especially in relation to satisfaction levels of a tourism factory. To evaluate overall satisfaction accurately, the impact of the different levels of performance should be considered (Matzler et al. 2004 ). The purpose of this study is to apply the TCSI model that contains different levels of performance to improve and ensure the understanding of firm operational efficiency by managers in the tourism factory. A partial least squares (PLS) was performed to test the theoretical model due to having been successfully applied to customer satisfaction analysis. The PLS is well suited for predictive applications (Barclay et al. 1995 ) and using path coefficients that regard the reasons for customer satisfaction or dissatisfaction and providing latent variable scores that could be used to report customer satisfaction scores. Our findings provide support for the application of TCSI model to derive tourist satisfaction information.
Literature review
National customer satisfaction index (csi).
The CSI model includes a structural equation with estimated parameters of hidden categories and category relationships. The CSI can clearly define the relationships between different categories and provide predictions. The basic CSI model is a structural equation model with latent variables which are calculated as weighted averages of their measurement variables, and the PLS estimation method calculates the weights and provide maximum predictive power of the ultimate dependent variable (Kristensen et al. 2001 ). Many scholars have identified the characteristics of the CSI (Karatepe et al. 2005 ; Malhotra et al. 1994 ).
Although the core of the models are in most respects standard, they have some obvious distinctions in model’s structure and variable’s selection so that their results cannot be compared with each other and some variations between the SCSB (Swedish), the ACSI (American), the ECSI (European), the NCSB (Norwegian) and other indices. For example, the image factor is not employed in the ACSI model (Johnson et al. 2001 ); the NCSB eliminated customer expectation and replaced with corporate image; the ECSI model does not include the customer complaint as a consequence of satisfaction. Many scholars have identified the characteristics of the CSI (Karatepe et al. 2005 ; Malhotra et al. 1994 ). The ECSI model distinguishes service quality from product quality (Kristensen et al. 2001 ) and the NCSB model applies SERVQUAL instrument to evaluate service quality (Johnson et al. 2001 ). A quality measure of a single customer satisfaction index is typically developed according to a certain type of culture or the culture of a certain country. When developing a system for measuring or evaluating a certain country or district’s customer satisfaction level, a specialized customer satisfaction index should be developed.
As such, the ACSI and ECSI were used as a foundation to develop the TCSI. The TCSI was developed by Chung Hua University and the Chinese Society for Quality. Every aspect of the TCSI that influences overall customer satisfaction can be measured through surveys, and every construct has a cause–effect relationship with the other five constructs. The TCSI assumes that currently: (1) Taiwan corporations have ability of dealing with customer complaints; customer complaints have already changed from a factor that influences customer satisfaction results to a factor that affects quality perception; (2) The expectations, satisfaction and loyalty of customers are affected by the image of the corporation. The concept that customer complaints are not calculated into the TCSI model is that they were removed based on the ECSI model (Lee et al. 2005 , 2006 , 2014a , b ; Guo and Tsai 2015 ; Tsai et al. 2015a , b ; 2016a ).
TCSI model and service quality
Service quality is frequently used by both researchers and practitioners to evaluate customer satisfaction. It is generally accepted that customer satisfaction depends on the quality of the product or service offered (Anderson and Sullivan 1993 ). Numerous researchers have emphasized the importance of service quality perceptions and their relationship with customer satisfaction by applying the NCSI model (e.g., Ryzin et al. 2004 ; Hsu 2008 ; Yazdanpanah et al. 2013 ; Chiu et al. 2011 ; Temizer and Turkyilmaz 2012 ; Mutua et al. 2012 ; Dutta and Singh 2014 ). Ryzin et al. ( 2004 ) applied the ACSI to U.S. local government services and indicated that the perceived quality of public schools, police, road conditions, and subway service were the most salient drivers of satisfaction, but that the significance of each service varied among income, race, and geography. Hsu ( 2008 ) proposed an index for online customer satisfaction based on the ACSI and found that e-service quality was more determinative than other factors (e.g., trust and perceived value) for customer satisfaction. To deliver superior service quality, an online business must first understand how customers perceive and evaluate its service quality. This study developed a basic model for using the TCSI to analyze Taiwan’s tourism factory services. The theoretical model comprised 14 observation variables and the following six constructs: image, customer expectations, perceived quality, perceived value, customer satisfaction, and loyalty.
Research methods
The measurement scale items for this study were primarily designed using the questionnaire from the TCSI model. In designing the questionnaire, a 10-point Likert scale (with anchors ranging from strongly disagree to strongly agree) was used to reduce the statistical problem of extreme skewness (Fornell et al. 1996 ; Qu et al. 2015 ; Tsai 2016 ; Tsai et al. 2016b ; Zhou et al. 2016 ). A total of 14 items, organized into six constructs, were included in the questionnaire. The primary questionnaire was pretested on 30 customers who had visited a tourism factory. Because the TCSI model is preliminary research in the tourism factory, this study convened a focus group to decide final attributes of model. The focus group was composed of one manager of tourism factory, one professor in Hospitality Management, and two customers with experience of tourism factory.
We used the TCSI model (Fig. 1 ) to structure our research. From this structure and the basic theories of the ACSI and ECSI, we established the following hypotheses:
Image has a strong influence on tourist expectations.
Image has a strong influence on tourist satisfaction.
Image has a strong influence on tourist loyalty.
Tourist expectations have a strong influence on perceived quality.
Tourist expectations have a strong influence on perceived values.
Tourist expectations have a strong influence on tourist satisfaction.
Perceived quality has a strong influence on perceived value.
Perceived quality has a strong influence on tourist satisfaction.
Perceived value has a strong influence on tourist satisfaction.
Customer satisfaction has a strong influence on tourist loyalty.
The content of our surveys were separated into two parts; customer satisfaction and personal information. The definitions and processing of above categories are listed below:
- Part 1 of the survey assessed customer satisfaction by measuring customer levels of tourism factory image, expectations, quality perceptions, value perceptions, satisfaction, and loyalty toward their experience, and used these constructs to indirectly survey the customer’s overall evaluation of the services provided by the tourism factory.
- Part 2 of the survey collected personal information: gender, age, family situation, education, income, profession, and residence.
The six constructs are defined as follows:
- Image reflects the levels of overall impression of the tourism factory as measured by two items: (1) word-of-mouth reputation, (2) responsibility toward concerned parties that the tourist had toward the tourism factory before traveling.
- Customer expectations refer to the levels of overall expectations as measured by two items: (1) expectations regarding the service of employees, (2) expectations regarding reliability that the tourist had before the experience at the tourism factory.
- Perceived quality was measured using three survey measures: (1) the overall evaluation, (2) perceptions of reliability, (3) perceptions of customization that the tourist had after the experience at the tourism factory.
- Perceived value was measured using two items: (1) the cost in terms of money and time (2) a comparison with other tourism factories.
- Customer satisfaction represents the levels of overall satisfaction was captured by two items: (1) meeting of expectations, (2) closeness to the ideal tourism factory.
- Loyalty was measured using three survey measures: (1) the probabilities of visiting the tourism factory again (2) attending another activity held by the tourism factory, (3) recommending the tourism factory to others.
Data collection and analysis
The survey sites selected for this study was the parking lots of one food tourism factory in Taipei, Taiwan. A domestic group package and individual tourists were a major source of respondents who were willing to participate in the survey and completed the questionnaires themselves based on their perceptions of their factory tour experience. Four research assistants were trained to conduct the survey regarding to questionnaire distribution and sampling.
To minimize prospective biases of visiting patterns, the survey was conducted at different times of day and days of week—Tuesday, Thursday, Saturday for the first week; Monday, Wednesday, Friday and Sunday for the next week. The afternoon time period was used first then the morning time period in the following weeks. The data were collected over 1 month period.
Of 300 tourists invited to complete the questionnaire, 242 effective responses were obtained (usable response rate of 80.6 %). The sample of tourists contained more females (55.7 %) than males (44.35 %). More than half of the respondents had a college degree or higher, 28 % were students, and 36.8 % had an annual household income of US $10,000–$20,000. The majority of the respondents (63.7 %) were aged 20–40 years.
Comparison of the TCSI models for satisfied and dissatisfied customers
Researchers have claimed that satisfaction levels differ according to gender, age, socioeconomic status, and residence (Bryant and Cha 1996 ). Moreover, the needs, preferences, buying behavior, and price sensitivity of customers vary (Kutner and Cripps 1997 ). Previous studies have demonstrated that it is crucial to measure the relative impact of each attribute for high and low performance (satisfaction) (Matzler et al. 2003 , 2004 ). To determine the reasons for differences, a satisfaction scale was used to group the sample into satisfied (8–10) and dissatisfied (1–7) customers.
The research model was tested using SmartPLS 3.0 software, which is suited for highly complex predictive models (Wold 1985 ; Barclay et al. 1995 ). In particular, it has been successfully applied to customer satisfaction analysis. The PLS method is a useful tool for obtaining indicator weights and predicting latent variables and includes estimating path coefficients and R 2 values. The path coefficients indicate the strengths of the relationships between the dependent and independent variables, and the R 2 values represent the amount of variance explained by the independent variables. Using Smart PLS, we determined the path coefficients. Figures 2 and and3 3 show ten path estimates corresponding to the ten research hypothesis of TCSI model for satisfied and dissatisfied customers. Every path coefficient was obtained by bootstrapping the computation of R 2 and performing a t test for each hypothesis. Fornell et al. ( 1996 ) demonstrated that the ability to explain the influential latent variables in a model is an indicator of model performance, in particular the customer satisfaction and customer loyalty variables. From the results shown, the R 2 values for the customer satisfaction were 0.53 vs. 0.50, respectively; and the R 2 value for customer loyalty were 0.64 vs. 0.60, respectively. Thus, the TCSI model explained 53 vs. 50 % of the variance in customer satisfaction; 64 vs. 60 % of that in customer loyalty as well.

Path estimate of the TCSI model for satisfied customers. *p < 0.05; **p < 0.01; ***p < 0.001

Path estimate of the TCSI model for dissatisfied customers. *p < 0.05; **p < 0.01; ***p < 0.001
According to the path coefficients shown in Figs. 2 and and3, 3 , image positively affected customer expectations (β = 0.58 vs. 0.37), the customer satisfaction (β = 0.16 vs. 0.11), and customer loyalty (β = 0.47 vs. 0.16). Therefore, H1–H3 were accepted. Customer expectations were significantly related to perceived quality (β = 0.94 vs. 0.83). However, customer expectations were not significantly related to perceived value shown as dotted line (β = −0.01 vs. −0.20) or the customer satisfaction, shown as dotted line (β = −0.21 vs. −0.32). Thus, H4 was accepted but H5 and H6 were not accepted. Perceived value positively affected the customer satisfaction (β = 0.27 vs. 0.14), supporting H7. Accordingly, the analysis showed that each of the antecedent constructs had a reasonable power to explain the overall customer satisfaction. Furthermore, perceived quality positively affected the customer satisfaction (β = 0.70 vs. 0.62), as did perceived value (β = 0.83 vs. 0.74). These results confirm H8 and H9. The path coefficient between the customer satisfaction and customer loyalty was positive and significant (β = 0.63 vs. 0.53). This study tested the suitability of two TCSI models by analyzing the tourism factories in Taiwan. The results showed that the TCSI models were all close fit for this type of research. This study provides empirical evidence of the causal relationships among perceived quality, image, perceived value, perceived expectations, customer satisfaction, and customer loyalty.
To observe the effects of antecedent constructs of perceived value (e.g., customer expectation and perceived quality), customer expectations were not significantly related to perceived value for either satisfied or dissatisfied customers. Furthermore, satisfied customers were affected more by perceived quality (β = 0.83 vs. 0.74), as shown in Table 1 . Regarding the effect of the antecedents of customer satisfaction (e.g., image, customer expectations, perceived value and perceived quality), the total effects of perceived quality on the customer satisfaction of satisfied and dissatisfied customers were 0.92 and 0.72. The total effects of image on the customer satisfaction of satisfied and dissatisfied customers were 0.45 and 0.19. Thus, the satisfaction level of satisfied customers was affected more by perceived quality. Consequently, regarding customer satisfaction, perceived quality is more important than image for satisfied and dissatisfied customers. Numerous researchers have emphasized the importance of service quality perceptions and their relationship with customer satisfaction by applying the CSI model (e.g., Ryzin et al. 2004 ; Hsu 2008 ; Yazdanpanah et al. 2013 ; Chiu et al. 2011 ; Temizer and Turkyilmaz 2012 ; Mutua et al. 2012 ; Dutta and Singh 2014 ). This is consistent with the results of previous research ( O’Loughlin and Coenders 2002 ; Yazdanpanah et al. 2013 ; Chiu et al. 2011 ; Chin and Liu 2015 ; Chin et al. 2016 ).
Table 1
Path estimates of the satisfied and dissatisfied customer CSI model
CS customer satisfaction
* p < 0.05; ** p < 0.01; *** p < 0.001
With respect to the effect of the antecedents of customer loyalty (e.g., image and customer satisfaction), the total effects of image on customer loyalty for satisfied and dissatisfied customers were 0.57 and 0.21. In other words, the customer loyalty of satisfied customers was affected more by customer satisfaction. Customer satisfaction was significantly related to the customer loyalty of both satisfied and dissatisfied customers, and satisfied customers were affected more by customer satisfaction ( β = 0.63 vs. 0.14). Consequently, regarding customer loyalty, customer satisfaction is more important than image for both satisfied and dissatisfied customers. Numerous studies have shown that customer satisfaction is a crucial factor for ensuring customer loyalty (Barsky 1992 ; Smith and Bolton 1998 ; Hallowell 1996 ; Grønholdt et al. 2000 ). This study empirically supports the notion that customer satisfaction is positively related to customer loyalty.
The TCSI model has a predictive capability that can help tourism factory managers improve customer satisfaction based on different performance levels. Our model enables managers to determine the specific factors that significantly affect overall customer satisfaction and loyalty within a tourism factory. This study also helps managers to address different customer segments (e.g., satisfied vs. dissatisfied); because the purchase behaviors of customers differ, they must be treated differently. The contribution of this paper is to propose two satisfaction levels of CSI models for analyzing customer satisfaction and loyalty, thereby helping tourism factory managers improve customer satisfaction effectively.
Fornell et al. ( 1996 ) demonstrated that the ability to explain influential latent variables in a model, particularly customer satisfaction and customer loyalty variables, is an indicator of model performance. However, the results of this study indicate that customer expectations were not significantly related to perceived value for either satisfied or dissatisfied customers. Moreover, they were affected more by perceived quality of customer satisfaction. Numerous researchers have found that the construct of customer expectations used in the ACSI model does not significantly affect the level of customer satisfaction (Johnson et al. 1996 , 2001 ; Martensen et al. 2000 ; Anderson and Sullivan 1993 ).
Through the overall effects, this study derived several theoretical findings. First, the factors with the largest influence on customer satisfaction were perceived quality and perceived expectations, despite the results showing that customer expectations were not significantly related to perceived value or customer satisfaction. Hence, customer expectations indirectly affected customer satisfaction through perceived quality. Accordingly, perceived quality had the greatest influence on customer satisfaction. Likewise, our results also show that satisfied customers were affected more by perceived quality than dissatisfied customers. This study determined that perceived quality, whether directly or indirectly, positively influenced customer satisfaction. This result is consistent with those of Cronin and Taylor ( 1992 ), Cronin et al. ( 2000 ), Hsu ( 2008 ), Ladhari ( 2009 ), Terblanche and Boshoff ( 2010 ), Deng et al. ( 2013 ), and Yazdanpanah et al. ( 2013 ).
Second, the factors with the most influence on customer loyalty were image and customer satisfaction. The results of this study demonstrate that the customer loyalty of satisfied customers was affected more by customer satisfaction. Consequently, regarding customer loyalty, customer satisfaction is more important than image for satisfied customers. Lee ( 2015 ) found that higher overall satisfaction increased the possibility that visitors will recommend and reattend tourism factory activities. Moreover, numerous studies have shown that customer satisfaction is a crucial factor for ensuring customer loyalty (Barsky 1992 ; Smith and Bolton 1998 ; Hallowell 1996 ; Su 2004; Deng et al. 2013 ). In initial experiments on ECSI, corporate image was assumed to have direct influences on customer expectation, satisfaction, and loyalty. Subsequent experiments in Denmark proved that image affected only expectation and satisfaction and had no relationship with loyalty (Martensen et al. 2000 ). In early attempts to build the ECSI model, image was defined as a variable involving not only a company’s overall image but products or brand awareness; thus image is readily connected with customer expectation and perception. Therefore, this study contributes to relevant research by providing empirical support for the notion that customer satisfaction is positively related to customer loyalty.
In addition to theoretical implications, this study has several managerial implications. First, the TCSI model has a satisfactory predictive capability that can help tourism factory managers to examine customer satisfaction more closely and to understand explicit influences on customer satisfaction for different customer segments by assessing the accurate causal relationships involved. In contrast to general customer satisfaction surveys, the TCSI model cannot obtain information on post-purchase customer behavior to improve customer satisfaction and achieve competitive advantage.
Second, this study not only indicated that each of the antecedent constructs had reasonable power to explain customer satisfaction and loyalty but also showed that perceived quality exerts the largest influence on the customer satisfaction of Taiwan’s tourism factory industry. Therefore, continually, Taiwan’s tourism factories must endeavor to enhance their customer satisfaction, ideally by improving service quality. Managers of Taiwan’s tourism factories must ensure that service providers deliver consistently high service quality.
Third, this research determined that the factors having the most influence on customer loyalty were image and customer satisfaction. Therefore, managers of Taiwan’s tourism factories should allow customer expectations to be fulfilled through experiences, thereby raising their overall level of satisfaction. Regarding image, which refers to a brand name and its related associations, when tourists regard a tourism factory as having a positive image, they tend to perceive higher value of its products and services. This leads to a higher level of customer satisfaction and increased chances of customers’ reattending tourism factory activities.
Different performance levels exist in how tourists express their opinions about various aspects of service quality and satisfaction with tourism factories. Customer segments can have different preferences depending on their needs and purchase behavior. Our findings indicate that tourists belonging to different customer segments (e.g., satisfied vs. dissatisfied) expressed differences toward service quality and customer satisfaction. Thus, the management of Taiwan’s tourism factories must notice the needs of different market segments to meet their individual expectations. This study proposes two satisfaction levels of CSI models for analyzing customer satisfaction and loyalty, thereby helping tourism factory managers improve customer satisfaction effectively. Compared with traditional techniques, we believe that our method is more appropriate for making decisions about allocating resources and for assisting managers in establishing appropriate priorities in customer satisfaction management.
Limitations and suggestions for future research
This study has some limitations. First, the tourism factory surveyed in this study was a food tourism factory operating in Taipei, Taiwan, and the present findings cannot be generalized to the all tourism factory industries. Second, the sample size was quite small for tourists (N = 242). Future research should collect a greater number of samples and include a more diverse range of tourists. Third, this study was preliminary research on tourism factories, and domestic group package tourists were a major source of the respondents. Future studies should collect data from international tourists as well.
Authors’ contributions
Writing: S-CL; providing case and idea: Y-CL, Y-CW, Y-FH, C-HC; providing revised advice: S-BT, WD. All authors read and approved the final manuscript.
Acknowledgements
Department of Technology Management, Chung-Hua University, Hsinchu, Taiwan. This work was supported by University of Electronic Science Technology of China, Zhongshan Institute (414YKQ01 and 415YKQ08).
Competing interests
The authors declare that they have no competing interests.
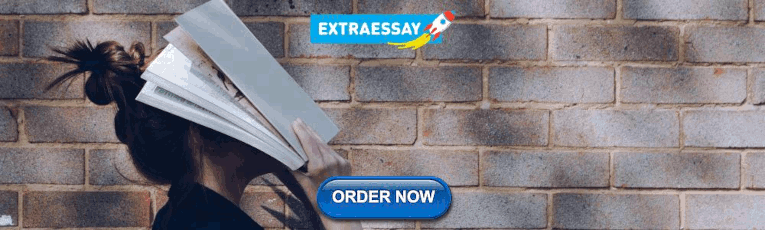
Contributor Information
Yu-Cheng Lee, Email: moc.liamg@861eelrd .
Yu-Che Wang, Email: wt.ude.uhc@gnawyrrej .
Shu-Chiung Lu, Email: moc.liamg@56ulecarg .
Yi-Fang Hsieh, Email: moc.liamg@gnafiyheish .
Chih-Hung Chien, Email: moc.liamtoh@neihctsirhc .
Sang-Bing Tsai, Phone: +86-22-2350-8785, Email: moc.liamtoh@gnibgnas .
Weiwei Dong, Email: moc.361@4949gnodiewiew .
- Anderson EW, Sullivan M. The antecedents and consequences of customer satisfaction for firms. Mark Sci. 1993; 12 :125–143. doi: 10.1287/mksc.12.2.125. [ CrossRef ] [ Google Scholar ]
- Anderson EW, Mittal V. Strengthening the satisfaction-profit chain. J Serv Res. 2000; 3 (2):107–120. doi: 10.1177/109467050032001. [ CrossRef ] [ Google Scholar ]
- Anderson EW, Fornell C, Lehmann DR. Customer satisfaction, market share, and profitability: findings from Sweden. J Mark. 1994; 58 :53–66. doi: 10.2307/1252310. [ CrossRef ] [ Google Scholar ]
- Anderson EW, Fornell C, Rust RT. Customer satisfaction, productivity, and profitability: differences between goods and services. Mark Sci. 1997; 16 (2):129–145. doi: 10.1287/mksc.16.2.129. [ CrossRef ] [ Google Scholar ]
- Barclay DW, Higgins C, Thompson R. Interdepartmental conflict in organizational buying: the impact of the organizational context. J Mark Res. 1995; 28 :145–159. doi: 10.2307/3172804. [ CrossRef ] [ Google Scholar ]
- Barsky JD. Customer satisfaction in the hotel industry: meaning and measurement. J Hosp Tour Res. 1992; 16 (1):51–73. [ Google Scholar ]
- Bryant BE, Cha J. Crossing the threshold. Mark Res. 1996; 8 (4):20. [ Google Scholar ]
- Chin T, Liu RH. Understanding labor conflicts in Chinese manufacturing: A Yin-Yang harmony perspective. Int J Confl Manag. 2015; 26 (3):288–315. doi: 10.1108/IJCMA-09-2014-0074. [ CrossRef ] [ Google Scholar ]
- Chin T, Liu RH, Yang X. Reverse internationalization in Chinese firms: A study of how global startup OEMs seek to compete domestically. Asia Pac Bus Rev. 2016; 22 (2):201–219. doi: 10.1080/13602381.2015.1055087. [ CrossRef ] [ Google Scholar ]
- Chiu SI, Cheng CC, Yen TM, Hu HY. Preliminary research on customer satisfaction models in Taiwan: a case study from the automobile industry. Expert Syst Appl. 2011; 38 (8):9780–9787. doi: 10.1016/j.eswa.2011.01.172. [ CrossRef ] [ Google Scholar ]
- Cronin JJ, Jr, Taylor SA. Measuring service quality: a reexamination and extension. J Mark. 1992; 56 (3):55–68. doi: 10.2307/1252296. [ CrossRef ] [ Google Scholar ]
- Cronin JJ, Brady MK, Hult GTM. Assessing the effects of quality, value, and customer satisfaction on consumer behavioral intentions in service environments. J Retail. 2000; 76 (2):193–218. doi: 10.1016/S0022-4359(00)00028-2. [ CrossRef ] [ Google Scholar ]
- Deng WJ, Yeh ML, Sung ML. A customer satisfaction index model for international tourist hotels: integrating consumption emotions into the American customer satisfaction index. Int J Hosp Manag. 2013; 35 :133–140. doi: 10.1016/j.ijhm.2013.05.010. [ CrossRef ] [ Google Scholar ]
- Dutta K, Singh S (2014). Deriving Customer Satisfaction and Loyalty from Organized Retailer’s Sales Promotion Activities in India. ISSN 2045-810X, 21
- Eklof JA, Hackl P, Westlund A. On measuring interactions between customer satisfaction and financial results. Total Qual Manag. 1999; 10 (4–5):514–522. doi: 10.1080/0954412997479. [ CrossRef ] [ Google Scholar ]
- Fornell C. A national customer satisfaction barometer: the swedish experience. J Mark. 1992; 56 (1):6. doi: 10.2307/1252129. [ CrossRef ] [ Google Scholar ]
- Fornell C, Johnson MD, Anderson EW, Cha J, Bryant BE. The American customer satisfaction index: nature, purpose, and findings. J Mark. 1996; 60 :7–18. doi: 10.2307/1251898. [ CrossRef ] [ Google Scholar ]
- Grønholdt L, Martensen A, Kristensen K. The relationship between customer satisfaction and loyalty: cross-industry differences. Total Qual Manag. 2000; 11 (4–6):509–514. doi: 10.1080/09544120050007823. [ CrossRef ] [ Google Scholar ]
- Guo JJ, Tsai SB. Discussing and evaluating green supply chain suppliers: a case study of the printed circuit board industry in China. S Afr J Ind Eng. 2015; 26 (2):56–67. [ Google Scholar ]
- Hallowell R. The relationships of customer satisfaction, customer loyalty, and profitability: an empirical study. Int J Serv Ind Manag. 1996; 7 (4):27–42. doi: 10.1108/09564239610129931. [ CrossRef ] [ Google Scholar ]
- Hsu SH. Developing an index for online customer satisfaction: adaptation of American Customer Satisfaction Index. Expert Syst Appl. 2008; 34 (4):3033–3042. doi: 10.1016/j.eswa.2007.06.036. [ CrossRef ] [ Google Scholar ]
- Ittner CD, Larcker DF. Measuring the impact of quality initiatives on firm financial performance. Adv Manag Organ Qual. 1996; 1 (1):1–37. [ Google Scholar ]
- Johnson MD, Nader G, Fornell C. Expectations, perceived performance, and customer satisfaction for a complex service: the case of bank loans. J Econ Psychol. 1996; 17 (2):163–182. doi: 10.1016/0167-4870(96)00002-5. [ CrossRef ] [ Google Scholar ]
- Johnson MD, et al. The evolution and future of national customer satisfaction index models. J Econ Psychol. 2001; 22 (2):217–245. doi: 10.1016/S0167-4870(01)00030-7. [ CrossRef ] [ Google Scholar ]
- Karatepe OM, Yavas U, Babakus E. Measuring service quality of banks: scales development and validation. J Retail Consum Serv. 2005; 12 (5):373–383. doi: 10.1016/j.jretconser.2005.01.001. [ CrossRef ] [ Google Scholar ]
- Kotler P, Armstrong G. Marketing: an introduction. New York: Prentice-Hall; 1997. [ Google Scholar ]
- Kristensen K, Juhl HJ, Østergaard P. Customer satisfaction: some results for European retailing. Total Qual Manag. 2001; 12 (7–8):890–897. doi: 10.1080/09544120100000012. [ CrossRef ] [ Google Scholar ]
- Kutner S, Cripps J. Managing the customer portfolio of healthcare enterprises. Healthc Forum J. 1997; 40 (5):52–54. [ PubMed ] [ Google Scholar ]
- Ladhari R. Service quality, emotional satisfaction, and behavioural intentions. Manag Serv Qual Int J. 2009; 19 (3):308–331. doi: 10.1108/09604520910955320. [ CrossRef ] [ Google Scholar ]
- Lee C-F. Tourist satisfaction with factory tour experience. Int J Cult Tourism Hosp Res. 2015; 9 :3. doi: 10.1108/IJCTHR-02-2015-0005. [ CrossRef ] [ Google Scholar ]
- Lee YC, He LY, Jiang JS, Lian QY. Foundation of the Taiwan customer satisfaction index model. Qual Mag. 2005; 41 (12):52–56. [ Google Scholar ]
- Lee YC, He LY, Jiang JS, Lian QY. The Taiwan customer satisfaction index model in related to sample decision. Qual Mag. 2006; 42 (4):74–77. [ Google Scholar ]
- Lee YC, Chen CY, Tsai SB, Wang CT. Discussing green environmental performance and competitive strategies. Pensee. 2014; 76 (7):190–198. [ Google Scholar ]
- Lee YC, Wu CH, Tsai SB. Grey system theory and fuzzy time series forecasting for the growth of green electronic materials. Int J Prod Res. 2014; 299 (8):1395–1406. [ Google Scholar ]
- Malhotra NK, Ulgado FM, Agarwal J, Baalbaki IB. International service marketing: a comparative evaluation of the dimensions of service quality between developed and developing countries. Int Mark Rev. 1994; 11 (2):5–15. doi: 10.1108/02651339410061937. [ CrossRef ] [ Google Scholar ]
- Martensen A, Gronholdt L, Kristensen K. The drivers of customer satisfaction and loyalty: cross-industry findings from Denmark. Total Qual Manag. 2000; 11 (4–6):544–553. doi: 10.1080/09544120050007878. [ CrossRef ] [ Google Scholar ]
- Matzler K, Sauerwein E. The factor structure of customer satisfaction: an empirical test of the importance grid and the penalty-reward-contrast analysis. Int J Serv Ind Manag. 2002; 13 (4):314–332. doi: 10.1108/09564230210445078. [ CrossRef ] [ Google Scholar ]
- Matzler K, Sauerwein E, Heischmidt K. Importance-performance analysis revisited: the role of the factor structure of customer satisfaction. Serv Ind J. 2003; 23 (2):112–129. doi: 10.1080/02642060412331300912. [ CrossRef ] [ Google Scholar ]
- Matzler K, Bailom F, Hinterhuber HH, Renzl B, Pichler J. The asymmetric relationship between attribute-level performance and overall customer satisfaction: a reconsideration of the importance–performance analysis. Ind Mark Manag. 2004; 33 (4):271–277. doi: 10.1016/S0019-8501(03)00055-5. [ CrossRef ] [ Google Scholar ]
- Mittal V, Ross WT, Baldasare PM. The asymmetric impact of negative and positive attribute-level performance on overall satisfaction and repurchase intentions. J Mark. 1998; 62 :33–47. doi: 10.2307/1251801. [ CrossRef ] [ Google Scholar ]
- Mutua J, Ngui D, Osiolo H, Aligula E, Gachanja J. Consumers satisfaction in the energy sector in Kenya. Energy Policy. 2012; 48 :702–710. doi: 10.1016/j.enpol.2012.06.004. [ CrossRef ] [ Google Scholar ]
- O’Loughlin C, Coenders G (2002) Application of the european customer satisfaction index to postal services. Structural equation models versus partial least squares, No. 4. Working Papers of the Department of Economics, University of Girona. Department of Economics, University of Girona
- Qu Q, Chen KY, Wei YM et al (2015) Using hybrid model to evaluate performance of innovation and technology professionals in marine logistics industry mathematical problems in engineering. Article ID 361275. doi:10.1155/2015/361275
- Reichheld FF, Sasser WE. Zero defections: qualiiy comes to services. Harvard Bus Rev. 1990; 68 (5):105–111. [ PubMed ] [ Google Scholar ]
- Ryzin GG, Muzzio D, Immerwahr S, Gulick L, Martinez E. Drivers and consequences of citizen satisfaction: an application of the American customer satisfaction index model to New York City. Public Adm Rev. 2004; 64 (3):331–341. doi: 10.1111/j.1540-6210.2004.00377.x. [ CrossRef ] [ Google Scholar ]
- Smith AK, Bolton RN. An experimental investigation of customer reactions to service failure and recovery encounters paradox or peril? J Serv Research. 1998; 1 (1):65–81. doi: 10.1177/109467059800100106. [ CrossRef ] [ Google Scholar ]
- Temizer L, Turkyilmaz A. Implementation of student satisfaction index model in higher education institutions. Procedia Soc Behav Sci. 2012; 46 :3802–3806. doi: 10.1016/j.sbspro.2012.06.150. [ CrossRef ] [ Google Scholar ]
- NS NS, Boshoff C. Quality, value, satisfaction and loyalty amongst race groups: A study of customers in the South African fast food industry. S Afr J Bus Manag. 2010; 41 (1):1–9. [ Google Scholar ]
- Tsai SB. Using grey models for forecasting China’s growth trends in renewable energy consumption. Clean Technol Environ Policy. 2016; 18 :563–571. doi: 10.1007/s10098-015-1017-7. [ CrossRef ] [ Google Scholar ]
- Tsai CH, Peng YJ, Wu HH (2012, October). Evaluating service process satisfaction of a tourism factory—using Brands’ Health Museum as an example. In: 2012 6th international conference on new trends in information science and service science and data mining (ISSDM), pp 244–247
- Tsai SB, Chien MF, Xue Y, Li L, et al. Using the fuzzy DEMATEL to determine environmental performance: a case of printed circuit board industry in Taiwan. PLoS ONE. 2015; 10 (6):e0129153. doi: 10.1371/journal.pone.0129153. [ PMC free article ] [ PubMed ] [ CrossRef ] [ Google Scholar ]
- Tsai SB, Saito R, Lin YC, Chen Q, et al. Discussing measurement criteria and competitive strategies of green suppliers from a Green law Perspective. Proc Inst Mech Eng B J Eng Manuf. 2015; 229 (S1):135–145. doi: 10.1177/0954405414558740. [ CrossRef ] [ Google Scholar ]
- Tsai SB, Huang CY, Wang CK, Chen Q, et al. Using a mixed model to evaluate job satisfaction in high-tech industries. PLoS ONE. 2016; 11 (5):e0154071. doi: 10.1371/journal.pone.0154071. [ PMC free article ] [ PubMed ] [ CrossRef ] [ Google Scholar ]
- Tsai SB, Xue Y, Zhang J, Chen Q, et al. Models for forecasting growth trends in renewable energy. Renew Sustain Energy Rev. 2016 [ Google Scholar ]
- Weitz BA, Jap SD. Relationship marketing and distribution channels. J Acad Mark Sci. 1995; 23 :305–320. doi: 10.1177/009207039502300411. [ CrossRef ] [ Google Scholar ]
- Wold H. Partial least squares. In: Kotz S, Johnson N, editors. Encyclopedia of statistical sciences. New York: Wiley; 1985. pp. 581–591. [ Google Scholar ]
- Wu SI, Zheng YH. The influence of tourism image and activities appeal on tourist loalty–a study of Tainan City in Taiwan. J Manag Strategy. 2014; 5 (4):121. [ Google Scholar ]
- Yazdanpanah M, Zamani GH, Hochrainer-Stigler S, Monfared N, Yaghoubi J. Measuring satisfaction of crop insurance a modified American customer satisfaction model approach applied to Iranian Farmers. Int J Disaster Risk Reduct. 2013; 5 :19–27. doi: 10.1016/j.ijdrr.2013.04.003. [ CrossRef ] [ Google Scholar ]
- Zeithaml VA. Service quality, profitability, and the economic worth of customers: what we know and what we need to learn. J Acad Mark Sci. 2000; 28 (1):67–85. doi: 10.1177/0092070300281007. [ CrossRef ] [ Google Scholar ]
- Zhou J, Wang Q, Tsai SB, et al. How to evaluate the job satisfaction of development personnel. IEEE Trans Syst Man Cybern Syst. 2016 [ Google Scholar ]

How To Write An A-Grade Literature Review
3 straightforward steps (with examples) + free template.
By: Derek Jansen (MBA) | Expert Reviewed By: Dr. Eunice Rautenbach | October 2019
Quality research is about building onto the existing work of others , “standing on the shoulders of giants”, as Newton put it. The literature review chapter of your dissertation, thesis or research project is where you synthesise this prior work and lay the theoretical foundation for your own research.
Long story short, this chapter is a pretty big deal, which is why you want to make sure you get it right . In this post, I’ll show you exactly how to write a literature review in three straightforward steps, so you can conquer this vital chapter (the smart way).
Overview: The Literature Review Process
- Understanding the “ why “
- Finding the relevant literature
- Cataloguing and synthesising the information
- Outlining & writing up your literature review
- Example of a literature review
But first, the “why”…
Before we unpack how to write the literature review chapter, we’ve got to look at the why . To put it bluntly, if you don’t understand the function and purpose of the literature review process, there’s no way you can pull it off well. So, what exactly is the purpose of the literature review?
Well, there are (at least) four core functions:
- For you to gain an understanding (and demonstrate this understanding) of where the research is at currently, what the key arguments and disagreements are.
- For you to identify the gap(s) in the literature and then use this as justification for your own research topic.
- To help you build a conceptual framework for empirical testing (if applicable to your research topic).
- To inform your methodological choices and help you source tried and tested questionnaires (for interviews ) and measurement instruments (for surveys ).
Most students understand the first point but don’t give any thought to the rest. To get the most from the literature review process, you must keep all four points front of mind as you review the literature (more on this shortly), or you’ll land up with a wonky foundation.
Okay – with the why out the way, let’s move on to the how . As mentioned above, writing your literature review is a process, which I’ll break down into three steps:
- Finding the most suitable literature
- Understanding , distilling and organising the literature
- Planning and writing up your literature review chapter
Importantly, you must complete steps one and two before you start writing up your chapter. I know it’s very tempting, but don’t try to kill two birds with one stone and write as you read. You’ll invariably end up wasting huge amounts of time re-writing and re-shaping, or you’ll just land up with a disjointed, hard-to-digest mess . Instead, you need to read first and distil the information, then plan and execute the writing.
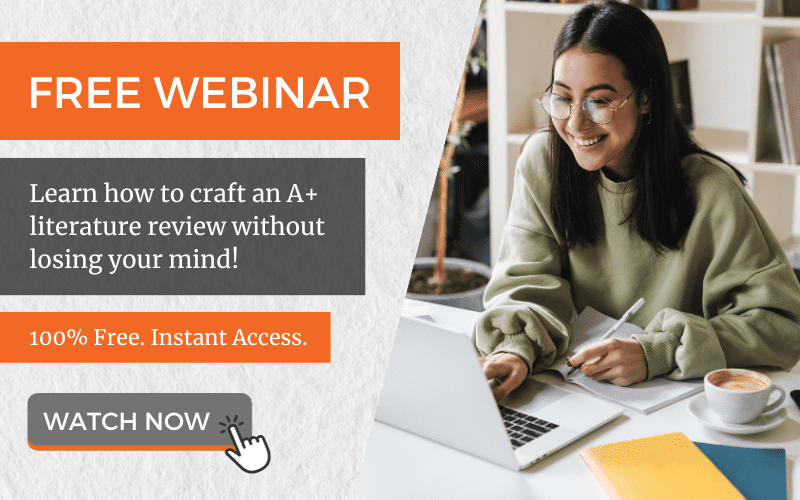
Step 1: Find the relevant literature
Naturally, the first step in the literature review journey is to hunt down the existing research that’s relevant to your topic. While you probably already have a decent base of this from your research proposal , you need to expand on this substantially in the dissertation or thesis itself.
Essentially, you need to be looking for any existing literature that potentially helps you answer your research question (or develop it, if that’s not yet pinned down). There are numerous ways to find relevant literature, but I’ll cover my top four tactics here. I’d suggest combining all four methods to ensure that nothing slips past you:
Method 1 – Google Scholar Scrubbing
Google’s academic search engine, Google Scholar , is a great starting point as it provides a good high-level view of the relevant journal articles for whatever keyword you throw at it. Most valuably, it tells you how many times each article has been cited, which gives you an idea of how credible (or at least, popular) it is. Some articles will be free to access, while others will require an account, which brings us to the next method.
Method 2 – University Database Scrounging
Generally, universities provide students with access to an online library, which provides access to many (but not all) of the major journals.
So, if you find an article using Google Scholar that requires paid access (which is quite likely), search for that article in your university’s database – if it’s listed there, you’ll have access. Note that, generally, the search engine capabilities of these databases are poor, so make sure you search for the exact article name, or you might not find it.
Method 3 – Journal Article Snowballing
At the end of every academic journal article, you’ll find a list of references. As with any academic writing, these references are the building blocks of the article, so if the article is relevant to your topic, there’s a good chance a portion of the referenced works will be too. Do a quick scan of the titles and see what seems relevant, then search for the relevant ones in your university’s database.
Method 4 – Dissertation Scavenging
Similar to Method 3 above, you can leverage other students’ dissertations. All you have to do is skim through literature review chapters of existing dissertations related to your topic and you’ll find a gold mine of potential literature. Usually, your university will provide you with access to previous students’ dissertations, but you can also find a much larger selection in the following databases:
- Open Access Theses & Dissertations
- Stanford SearchWorks
Keep in mind that dissertations and theses are not as academically sound as published, peer-reviewed journal articles (because they’re written by students, not professionals), so be sure to check the credibility of any sources you find using this method. You can do this by assessing the citation count of any given article in Google Scholar. If you need help with assessing the credibility of any article, or with finding relevant research in general, you can chat with one of our Research Specialists .
Alright – with a good base of literature firmly under your belt, it’s time to move onto the next step.
Need a helping hand?
Step 2: Log, catalogue and synthesise
Once you’ve built a little treasure trove of articles, it’s time to get reading and start digesting the information – what does it all mean?
While I present steps one and two (hunting and digesting) as sequential, in reality, it’s more of a back-and-forth tango – you’ll read a little , then have an idea, spot a new citation, or a new potential variable, and then go back to searching for articles. This is perfectly natural – through the reading process, your thoughts will develop , new avenues might crop up, and directional adjustments might arise. This is, after all, one of the main purposes of the literature review process (i.e. to familiarise yourself with the current state of research in your field).
As you’re working through your treasure chest, it’s essential that you simultaneously start organising the information. There are three aspects to this:
- Logging reference information
- Building an organised catalogue
- Distilling and synthesising the information
I’ll discuss each of these below:
2.1 – Log the reference information
As you read each article, you should add it to your reference management software. I usually recommend Mendeley for this purpose (see the Mendeley 101 video below), but you can use whichever software you’re comfortable with. Most importantly, make sure you load EVERY article you read into your reference manager, even if it doesn’t seem very relevant at the time.
2.2 – Build an organised catalogue
In the beginning, you might feel confident that you can remember who said what, where, and what their main arguments were. Trust me, you won’t. If you do a thorough review of the relevant literature (as you must!), you’re going to read many, many articles, and it’s simply impossible to remember who said what, when, and in what context . Also, without the bird’s eye view that a catalogue provides, you’ll miss connections between various articles, and have no view of how the research developed over time. Simply put, it’s essential to build your own catalogue of the literature.
I would suggest using Excel to build your catalogue, as it allows you to run filters, colour code and sort – all very useful when your list grows large (which it will). How you lay your spreadsheet out is up to you, but I’d suggest you have the following columns (at minimum):
- Author, date, title – Start with three columns containing this core information. This will make it easy for you to search for titles with certain words, order research by date, or group by author.
- Categories or keywords – You can either create multiple columns, one for each category/theme and then tick the relevant categories, or you can have one column with keywords.
- Key arguments/points – Use this column to succinctly convey the essence of the article, the key arguments and implications thereof for your research.
- Context – Note the socioeconomic context in which the research was undertaken. For example, US-based, respondents aged 25-35, lower- income, etc. This will be useful for making an argument about gaps in the research.
- Methodology – Note which methodology was used and why. Also, note any issues you feel arise due to the methodology. Again, you can use this to make an argument about gaps in the research.
- Quotations – Note down any quoteworthy lines you feel might be useful later.
- Notes – Make notes about anything not already covered. For example, linkages to or disagreements with other theories, questions raised but unanswered, shortcomings or limitations, and so forth.
If you’d like, you can try out our free catalog template here (see screenshot below).
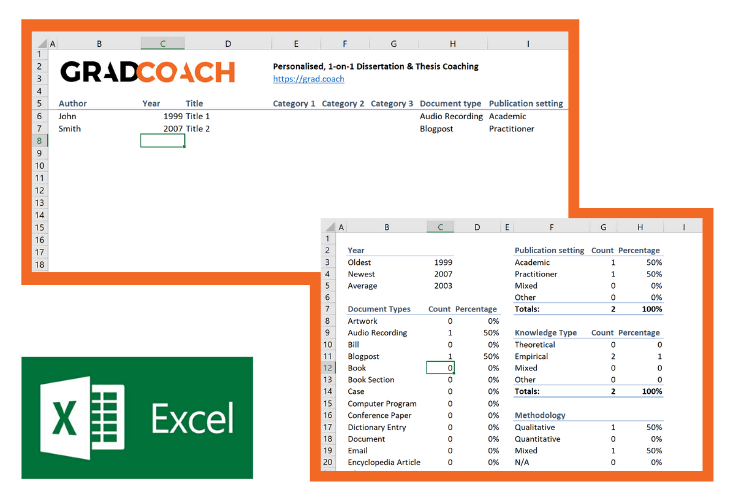
2.3 – Digest and synthesise
Most importantly, as you work through the literature and build your catalogue, you need to synthesise all the information in your own mind – how does it all fit together? Look for links between the various articles and try to develop a bigger picture view of the state of the research. Some important questions to ask yourself are:
- What answers does the existing research provide to my own research questions ?
- Which points do the researchers agree (and disagree) on?
- How has the research developed over time?
- Where do the gaps in the current research lie?
To help you develop a big-picture view and synthesise all the information, you might find mind mapping software such as Freemind useful. Alternatively, if you’re a fan of physical note-taking, investing in a large whiteboard might work for you.
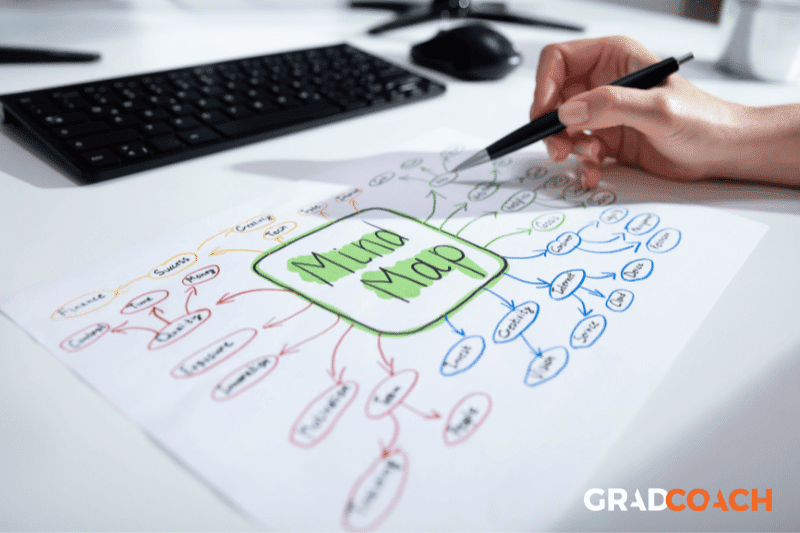
Step 3: Outline and write it up!
Once you’re satisfied that you have digested and distilled all the relevant literature in your mind, it’s time to put pen to paper (or rather, fingers to keyboard). There are two steps here – outlining and writing:
3.1 – Draw up your outline
Having spent so much time reading, it might be tempting to just start writing up without a clear structure in mind. However, it’s critically important to decide on your structure and develop a detailed outline before you write anything. Your literature review chapter needs to present a clear, logical and an easy to follow narrative – and that requires some planning. Don’t try to wing it!
Naturally, you won’t always follow the plan to the letter, but without a detailed outline, you’re more than likely going to end up with a disjointed pile of waffle , and then you’re going to spend a far greater amount of time re-writing, hacking and patching. The adage, “measure twice, cut once” is very suitable here.
In terms of structure, the first decision you’ll have to make is whether you’ll lay out your review thematically (into themes) or chronologically (by date/period). The right choice depends on your topic, research objectives and research questions, which we discuss in this article .
Once that’s decided, you need to draw up an outline of your entire chapter in bullet point format. Try to get as detailed as possible, so that you know exactly what you’ll cover where, how each section will connect to the next, and how your entire argument will develop throughout the chapter. Also, at this stage, it’s a good idea to allocate rough word count limits for each section, so that you can identify word count problems before you’ve spent weeks or months writing!
PS – check out our free literature review chapter template…
3.2 – Get writing
With a detailed outline at your side, it’s time to start writing up (finally!). At this stage, it’s common to feel a bit of writer’s block and find yourself procrastinating under the pressure of finally having to put something on paper. To help with this, remember that the objective of the first draft is not perfection – it’s simply to get your thoughts out of your head and onto paper, after which you can refine them. The structure might change a little, the word count allocations might shift and shuffle, and you might add or remove a section – that’s all okay. Don’t worry about all this on your first draft – just get your thoughts down on paper.
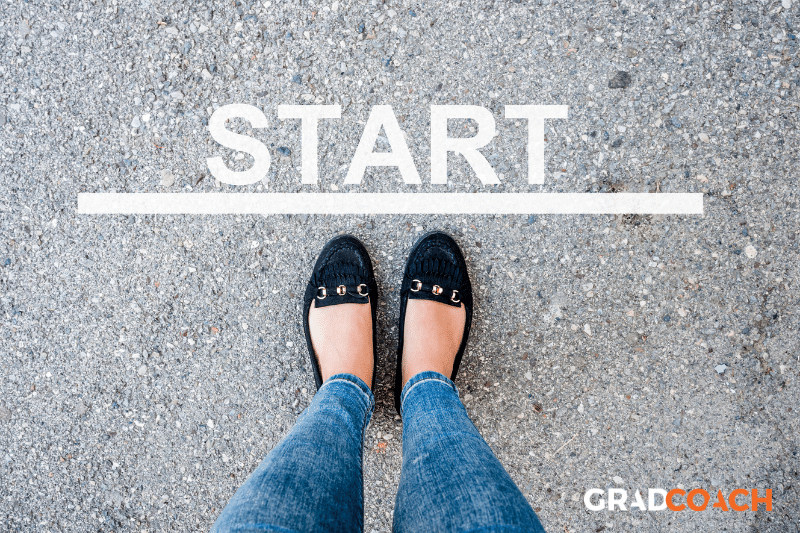
Once you’ve got a full first draft (however rough it may be), step away from it for a day or two (longer if you can) and then come back at it with fresh eyes. Pay particular attention to the flow and narrative – does it fall fit together and flow from one section to another smoothly? Now’s the time to try to improve the linkage from each section to the next, tighten up the writing to be more concise, trim down word count and sand it down into a more digestible read.
Once you’ve done that, give your writing to a friend or colleague who is not a subject matter expert and ask them if they understand the overall discussion. The best way to assess this is to ask them to explain the chapter back to you. This technique will give you a strong indication of which points were clearly communicated and which weren’t. If you’re working with Grad Coach, this is a good time to have your Research Specialist review your chapter.
Finally, tighten it up and send it off to your supervisor for comment. Some might argue that you should be sending your work to your supervisor sooner than this (indeed your university might formally require this), but in my experience, supervisors are extremely short on time (and often patience), so, the more refined your chapter is, the less time they’ll waste on addressing basic issues (which you know about already) and the more time they’ll spend on valuable feedback that will increase your mark-earning potential.
Literature Review Example
In the video below, we unpack an actual literature review so that you can see how all the core components come together in reality.
Let’s Recap
In this post, we’ve covered how to research and write up a high-quality literature review chapter. Let’s do a quick recap of the key takeaways:
- It is essential to understand the WHY of the literature review before you read or write anything. Make sure you understand the 4 core functions of the process.
- The first step is to hunt down the relevant literature . You can do this using Google Scholar, your university database, the snowballing technique and by reviewing other dissertations and theses.
- Next, you need to log all the articles in your reference manager , build your own catalogue of literature and synthesise all the research.
- Following that, you need to develop a detailed outline of your entire chapter – the more detail the better. Don’t start writing without a clear outline (on paper, not in your head!)
- Write up your first draft in rough form – don’t aim for perfection. Remember, done beats perfect.
- Refine your second draft and get a layman’s perspective on it . Then tighten it up and submit it to your supervisor.
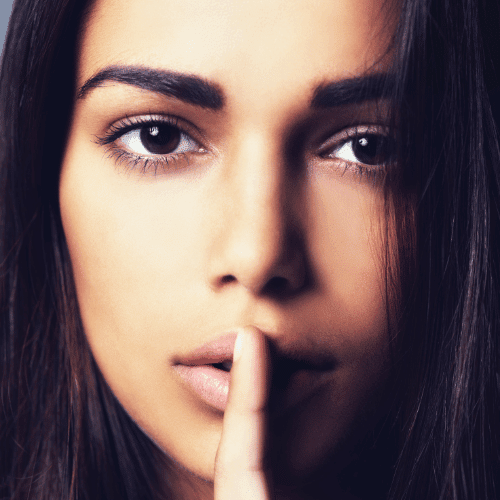
Psst… there’s more!
This post is an extract from our bestselling short course, Literature Review Bootcamp . If you want to work smart, you don't want to miss this .
You Might Also Like:
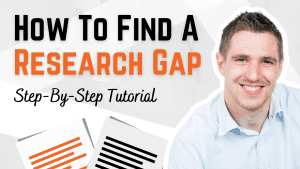
38 Comments
Thank you very much. This page is an eye opener and easy to comprehend.
This is awesome!
I wish I come across GradCoach earlier enough.
But all the same I’ll make use of this opportunity to the fullest.
Thank you for this good job.
Keep it up!
You’re welcome, Yinka. Thank you for the kind words. All the best writing your literature review.
Thank you for a very useful literature review session. Although I am doing most of the steps…it being my first masters an Mphil is a self study and one not sure you are on the right track. I have an amazing supervisor but one also knows they are super busy. So not wanting to bother on the minutae. Thank you.
You’re most welcome, Renee. Good luck with your literature review 🙂
This has been really helpful. Will make full use of it. 🙂
Thank you Gradcoach.
Really agreed. Admirable effort
thank you for this beautiful well explained recap.
Thank you so much for your guide of video and other instructions for the dissertation writing.
It is instrumental. It encouraged me to write a dissertation now.
Thank you the video was great – from someone that knows nothing thankyou
an amazing and very constructive way of presetting a topic, very useful, thanks for the effort,
It is timely
It is very good video of guidance for writing a research proposal and a dissertation. Since I have been watching and reading instructions, I have started my research proposal to write. I appreciate to Mr Jansen hugely.
I learn a lot from your videos. Very comprehensive and detailed.
Thank you for sharing your knowledge. As a research student, you learn better with your learning tips in research
I was really stuck in reading and gathering information but after watching these things are cleared thanks, it is so helpful.
Really helpful, Thank you for the effort in showing such information
This is super helpful thank you very much.
Thank you for this whole literature writing review.You have simplified the process.
I’m so glad I found GradCoach. Excellent information, Clear explanation, and Easy to follow, Many thanks Derek!
You’re welcome, Maithe. Good luck writing your literature review 🙂
Thank you Coach, you have greatly enriched and improved my knowledge
Great piece, so enriching and it is going to help me a great lot in my project and thesis, thanks so much
This is THE BEST site for ANYONE doing a masters or doctorate! Thank you for the sound advice and templates. You rock!
Thanks, Stephanie 🙂
This is mind blowing, the detailed explanation and simplicity is perfect.
I am doing two papers on my final year thesis, and I must stay I feel very confident to face both headlong after reading this article.
thank you so much.
if anyone is to get a paper done on time and in the best way possible, GRADCOACH is certainly the go to area!
This is very good video which is well explained with detailed explanation
Thank you excellent piece of work and great mentoring
Thanks, it was useful
Thank you very much. the video and the information were very helpful.
Good morning scholar. I’m delighted coming to know you even before the commencement of my dissertation which hopefully is expected in not more than six months from now. I would love to engage my study under your guidance from the beginning to the end. I love to know how to do good job
Thank you so much Derek for such useful information on writing up a good literature review. I am at a stage where I need to start writing my one. My proposal was accepted late last year but I honestly did not know where to start
Like the name of your YouTube implies you are GRAD (great,resource person, about dissertation). In short you are smart enough in coaching research work.
This is a very well thought out webpage. Very informative and a great read.
Very timely.
I appreciate.
Very comprehensive and eye opener for me as beginner in postgraduate study. Well explained and easy to understand. Appreciate and good reference in guiding me in my research journey. Thank you
Thank you. I requested to download the free literature review template, however, your website wouldn’t allow me to complete the request or complete a download. May I request that you email me the free template? Thank you.
Submit a Comment Cancel reply
Your email address will not be published. Required fields are marked *
Save my name, email, and website in this browser for the next time I comment.
- Print Friendly
Literature Review
- First Online: 01 January 2013
Cite this chapter
- Alireza Faed 2
Part of the book series: Springer Theses ((Springer Theses))
1407 Accesses
Early in Chapter 1, we introduced and discussed various terms and concepts regarding customer relationship management, its components and the differences between CRM systems and strategies. We also explained the significance of complaints and their importance in any organization.
This is a preview of subscription content, log in via an institution to check access.
Access this chapter
- Available as PDF
- Read on any device
- Instant download
- Own it forever
- Available as EPUB and PDF
- Compact, lightweight edition
- Dispatched in 3 to 5 business days
- Free shipping worldwide - see info
- Durable hardcover edition
Tax calculation will be finalised at checkout
Purchases are for personal use only
Institutional subscriptions
Chen, I. J., & Popovich, K. (2003). Understanding customer relationship management (CRM). Business Process Management Journal, 9 , 672–688.
Article Google Scholar
Frow, P., Payne, A., Wilkinson, I. F., & Young, L. (2011). Customer management and CRM: Addressing the dark side. Journal of Services Marketing, 25 , 79–89.
Chen, K., & Sockel, H. (2004). The impact of interactivity on business website visibility. International Journal of Web Engineering and Technology, 1 , 202–217.
Mendoza, L. E., Marius, A., Pérez, M., & Grimán, A. C. (2007). Critical success factors for a customer relationship management strategy. Information and Software Technology, 49 , 913–945.
Ueno, S. (2006). The impact of Customer Relationship Management. USJP Occasional Paper, pp. 6–13.
Google Scholar
Özgener, S., & Iraz, R. (2006). Customer relationship management in small-medium enterprises: The case of Turkish tourism industry. Tourism Management, 27 , 1356–1363.
Hoots, M. (2005). Customer relationship management for facility managers. Journal of Facilities Management, 3 , 346–361.
Payne, A., & Frow, P. (2005). A strategic framework for customer relationship management. Journal of Marketing, 69 , 167–176.
Kerr, C., & Anderson. K. (2002, 01-may-2011). Customer Relationship Management .New York: McGraw-Hill.
Kim, H.-S., & Kim, Y.-G. (2009). A CRM performance measurement framework: Its development process and application. Industrial Marketing Management, 38 , 477–489.
Faed, A., Ashouri, A., & Wu, C. (2010). The efficient bond among mobile commerce, CRM and E-loyalty to maximise the productivity of companies. In Information sciences and interaction sciences (ICIS), 2010 3rd international conference on , pp. 312–317.
Robinson, L., Jr, Neeley, S. E., & Williamson, K. (2011). Implementing service recovery through customer relationship management: Identifying the antecedents. Journal of Services Marketing, 25 , 90–100.
Chaudhry, P. E. (2007). Developing a process to enhance customer relationship management for small entrepreneurial businesses in the service sector. Journal of Research in Marketing and Entrepreneurship, 9 , 4–23.
Lawson Body, A., & Limayem, M. (2004). The impact of customer relationship management on customer loyalty: The moderating role of web site characteristics. Journal of Computer Mediated Communication, 9 , 00–00.
Limayem, M. (2006). Customer relationship management: Aims and objectives . Tehran: Tarbiat Modares University of Tehran.
Ngai, E. W. T., Xiu, L., & Chau, D. C. K. (2009). Application of data mining techniques in customer relationship management: A literature review and classification. Expert Systems with Applications, 36 , 2592–2602.
Bose, R. (2002). Customer relationship management: Key components for IT success. Industrial Management & Data Systems, 102 , 89–97.
Furuholt, B., & Skutle, N. (2007). Strategic use of customer relationship management (CRM) in Sports: The Rosenborg case. Advances in information systems development, 123–133.
King, S. F., & Burgess, T. F. (2008). Understanding success and failure in customer relationship management. Industrial Marketing Management, 37 , 421–431.
Reinartz, W., Krafft, M., & Hoyer, W. D. (2004). The customer relationship management process: Its measurement and impact on performance. Journal of Marketing Research, 41 , 293–305.
Goldsmith, R. E. (2010). The Goals of Customer Relationship Management. International Journal of Customer Relationship Marketing and Management (IJCRMM), 1 , 16.
Lindgreen, A., Palmer, R., Vanhamme, J., & Wouters, J. (2006). A relationship-management assessment tool: Questioning, identifying, and prioritizing critical aspects of customer relationships. Industrial Marketing Management, 35 , 57–71.
Richards, K. A., & Jones, E. (2008). Customer relationship management: Finding value drivers. Industrial Marketing Management, 37 , 120–130.
Iriana, R., & Buttle, F. (2007). Strategic, operational, and analytical customer relationship management. Journal of Relationship Marketing, 5 , 23–42.
Foss, B., Stone, M., & Ekinci, Y. (2008). What makes for CRM system success—Or failure? Journal of Database Marketing & Customer Strategy Management, 15 , 68–78.
Chan, J. O. (2005). Toward a unified view of customer relationship management. Journal of American Academy of Business, 6 , 32–38.
Cho, Y., Im, I., & Hiltz, R. (2003). The impact of e-services failures and customer complaints on electronic commerce customer relationship management. Journal of Consumer Satisfaction Dissatisfaction and Complaining Behavior, 16 , 106–118.
Reinartz, W., Thomas, J. S., & Kumar, V. (2005). Balancing acquisition and retention resources to maximize customer profitability. Journal of Marketing, 69 , 63–79.
Heung, V., & Lam, T. (2003). Customer complaint behaviour towards hotel restaurant services. International Journal of Contemporary Hospitality Management, 15 , 283–289.
Ndubisi, N. O., & Ling, T. Y. (2006). Complaint behaviour of Malaysian consumers. Management Research News, 29 , 65–76.
Stauss, B., & Seidel, W. (2010). Complaint management . Wiley Online Library.
Cho, Y., Im, I., Hiltz, R., & Fjermestad, J. (2001). Causes and outcomes of online customer complaining behavior: Implications for customer relationship management (CRM). In Proceedings of the 7th americas conference on information systems , pp. 900–907.
Florenthal, B. & Shoham, A. Four-mode channel interactivity concept and channel preferences. Journal of Services Marketing, 24 , 29–41.
Liu, Y., & Shrum, L. (2002). What is interactivity and is it always such a good thing? Implications of definition, person, and situation for the influence of interactivity on advertising effectiveness. Journal of Advertising, 31 , 53–64.
Goodman, J. (2006). Manage complaints to enhance loyalty. Quality Control and Applied Statistics, 51 , 535.
Vos, J. F. J., Huitema, G. B., & de Lange-Ros, E. (2008). How organisations can learn from complaints. TQM Journal, 20 , 8.
Faed, A. (2010). A conceptual model for interactivity, complaint and expectation for CRM,” in Computer Information Systems and Industrial Management Applications (CISIM). International conference on 2010, pp. 314–318.
Becker, J. U., Greve, G., & Albers, S. (2009). The impact of technological and organizational implementation of CRM on customer acquisition, maintenance, and retention. International Journal of Research in Marketing, 26 , 207–215.
Hidalgo, P., Manzur, E., Olavarrieta, S., & Farías, P. (2008). Customer retention and price matching: The AFPs case. Journal of Business Research, 61 , 691–696.
Larivière, B., & Van den Poel, D. (2005). Predicting customer retention and profitability by using random forests and regression forests techniques. Expert Systems with Applications, 29 , 472–484.
Avlonitis, G. J., & Panagopoulos, N. G. (2005). Antecedents and consequences of CRM technology acceptance in the sales force. Industrial Marketing Management, 34 , 355–368.
Sánchez-Fernández, R., & Iniesta-Bonillo, M. Á. (2009). Efficiency and quality as economic dimensions of perceived value: Conceptualization, measurement, and effect on satisfaction. Journal of Retailing and Consumer Services, 16 , 425–433.
Chen, P.-T., & Hu, H.-H. (2010). The effect of relational benefits on perceived value in relation to customer loyalty: An empirical study in the Australian coffee outlets industry. International Journal of Hospitality Management, 29 , 405–412.
Hua, H. H., Kandampullyb, J., & Juwaheer T. D. (2009). Relationships and impacts of service quality, perceived value, customer satisfaction, and image: An empirical study. The Service Industries Journal, 29 (2), 111–125.
Faed, A. (2011). Maximizing productivity using CRM within the context of M-Commerce. International Journal of Information Processing and Management, 2 , 1–9.
Korda, A. P., & Snoj, B. (2010). Development, validity and reliability of perceived service quality in retail banking and its relationship with perceived value and customer satisfaction. Managing Global Transitions, 8 , 187–205.
Hu, H–. H., Kandampully, J., & Juwaheer, T. D. (2009). Relationships and impacts of service quality, perceived value, customer satisfaction, and image: An empirical study. The Service Industries Journal, 29 , 111–125.
Georges, L., Eggert, A., & Goala, G. (2010). The impact of Key Account Managers’ Communication on Customer-Perceived Value and Satisfaction. URL: http://www.cr2m.net/membres/ngoala/travaux/pdfs/D-Gilles%20NGoala-Recherche-Article%20AMS%20EMAC%20KAM-KAMcommunication%20emac.pdf . Quoted, vol. 26.
Mithas, S., Krishnan, M. S., & Fornell, C. (2005). Why do customer relationship management applications affect customer satisfaction? Journal of Marketing, 69 , 201–209.
Flint, D. J., Blocker, C. P., & Boutin, P. J., Jr. (2011). Customer value anticipation, customer satisfaction and loyalty: An empirical examination. Industrial Marketing Management, 40 , 219–230.
Caruana, A. (2002). Service loyalty: The effects of service quality and the mediating role of customer satisfaction. European Journal of Marketing, 36 , 811–828.
Minami, C., & Dawson, J. (2008). The CRM process in retail and service sector firms in Japan: Loyalty development and financial return. Journal of Retailing and Consumer Services, 15 , 375–385.
Wang, M. L., & Yang, F. F. (2010). How does CRM create better customer outcomes for small educational institutions? African Journal of Business Management, 4 , 3541–3549.
Gee, R., Coates, G., & Nicholson, M. (2008). Understanding and profitably managing customer loyalty. Marketing Intelligence & Planning, 26 , 359–374.
Faed, A., & Chang, E. (2012) Adaptive Neuro-Fuzzy inference system based approach to examine customer complaint issues. Presented at the second world conference on soft computing , Baku, Azerbaijan.
Gommans, M., Krishnan, K. S., & Scheffold, K. B. (2001). From brand loyalty to e-loyalty: A conceptual framework. Journal of Economic and Social research, 3 , 43–58.
Kumar, V., & Shah, D. (2004). Building and sustaining profitable customer loyalty for the 21st century. Journal of Retailing, 80 , 317–329.
Anderson, R. E., & Srinivasan, S. S. (2003). E-satisfaction and E-loyalty: A contingency framework. Psychology and Marketing, 20 , 123–138.
Bridson, K., Evans, J., & Hickman, M. (2008). Assessing the relationship between loyalty program attributes, store satisfaction and store loyalty. Journal of Retailing and Consumer Services, 15 , 364–374.
Lee-Kelley, L., Gilbert, D., & Mannicom, R. (2003). How e-CRM can enhance customer loyalty. Marketing Intelligence & Planning, 21 , 239–248.
Reynolds, K. E., & Beatty, S. E. (1999). Customer benefits and company consequences of customer-salesperson relationships in retailing. Journal of Retailing, 75 , 11–32.
Cortiñas, M., Elorz, M., & Múgica, J. M. (2008). The use of loyalty-cards databases: Differences in regular price and discount sensitivity in the brand choice decision between card and non-card holders. Journal of Retailing and Consumer Services, 15 , 52–62.
Rowley, J. (2005). The four Cs of customer loyalty. Marketing Intelligence & Planning, 23 , 574–581.
Sharp, B., & Sharp, A. (1997). Loyalty programs and their impact on repeat-purchase loyalty patterns. International Journal of Research in Marketing, 14 , 473–486.
Bridges, E., & Freytag, P. V. (2009). When do firms invest in offensive and/or defensive marketing? Journal of Business Research, 62 , 745–749.
Thomas, J. S. (2001) A methodology for linking customer acquisition to customer retention. Journal of Marketing Research, 262–268.
Lewis, M. (2006). Customer acquisition promotions and customer asset value. Journal of Marketing Research, 43 , 195–203.
Verhoef, P. C., & Donkers, B. (2005). The effect of acquisition channels on customer loyalty and cross-buying. Journal of Interactive Marketing, 19 , 31–43.
Xu, M., & Walton, J. (2005). Gaining customer knowledge through analytical CRM. Industrial Management & Data Systems, 105 , 955–971.
Azvine, B., Nauck, D., Ho, C., Broszat, K., & Lim, J. (2006). Intelligent process analytics for CRM. BT technology journal, 24 , 60–69.
Öztaysi, B., Kaya, T., & Kahraman, C. (2011). Performance comparison based on customer relationship management using analytic network process. Expert Systems with Applications, 38 , 9788–9798.
Torkzadeh, G., Chang, J. C.-J., & Hansen, G. W. (2006). Identifying issues in customer relationship management at Merck-Medco. Decision Support Systems, 42 , 1116–1130.
Dimitriadis, S., & Stevens, E. (2008). Integrated customer relationship management for service activities: An internal/external gap model. Managing Service Quality, 18 , 496–511.
Phan, D. D., & Vogel, D. R. (2010). A model of customer relationship management and business intelligence systems for catalogue and online retailers. Information & Management, 47 , 69–77.
Eggert, A., & Ulaga, W. (2002). Customer perceived value: A substitute for satisfaction in business markets? Journal of Business & Industrial Marketing, 17 , 107–118.
Yang, Z., & Peterson, R. T. (2004). Customer perceived value, satisfaction, and loyalty: The role of switching costs. Psychology and Marketing, 21 , 799–822.
Roig, J. C. F., Garcia, J. S., Tena, M. A. M., & Monzonis, J. L. (2006). Customer perceived value in banking services. International Journal of Bank Marketing, 24 , 266–283.
Chang, H. H., & Wang, H. W. (2011). The moderating effect of customer perceived value on online shopping behaviour. Online Information Review, 35 , 333–359.
Lai, F., Griffin, M., & Babin, B. J. (2009). How quality, value, image, and satisfaction create loyalty at a Chinese telecom. Journal of Business Research, 62 , 980–986.
Ryu, K., Han, H., & Kim, T. H. (2008). The relationships among overall quick-casual restaurant image, perceived value, customer satisfaction, and behavioral intentions. International Journal of Hospitality Management, 27 , 459–469.
Chang, W. L., & Wu, Y. X. (2011). A framework for CRM E-services: From customer value perspective. Exploring the Grand Challenges for Next Generation E-Business, 52 , 235–242.
Mimouni-Chaabane, A., & Volle, P. (2010). Perceived benefits of loyalty programs: Scale development and implications for relational strategies. Journal of Business Research, 63 , 32–37.
Blocker, C. P., Flint, D. J., Myers, M. B., & Slater, S. F. (2011). Proactive customer orientation and its role for creating customer value in global markets. Journal of the Academy of Marketing Science, 39 , 216–233.
Florenthal, B., & Shoham, A. (2010). Four-mode channel interactivity concept and channel preferences. Journal of Services Marketing, 24 , 29–41.
Yoo, W.-S., Lee, Y., & Park, J. (2010). The role of interactivity in e-tailing: Creating value and increasing satisfaction. Journal of Retailing and Consumer Services, 17 , 89–96.
Bonner, J. M. (2005). The influence of formal controls on customer interactivity in new product development. Industrial Marketing Management, 34 , 63–69.
Kirk, C. P., Chiagouris, L., & Gopalakrishna, P. (2012). Some people just want to read: The roles of age, interactivity, and perceived usefulness of print in the consumption of digital information products. Journal of Retailing and Consumer Services, 19 , 168–178.
Lee, T. M. (2005). The impact of perceptions of interactivity on customer trust and transaction intentions in mobile commerce. Journal of Electronic Commerce Research, 6 (3), 165–180.
Ballantine, P. W. (2005). Effects of interactivity and product information on consumer satisfaction in an online retail setting. International Journal of Retail & Distribution Management, 33 , 461–471.
Liu, Y., & Shrum, L. (2002). What is interactivity and is it always such a good thing? Implications of definition, person, and situation for the influence of interactivity on advertising effectiveness. Journal of Advertising , 53–64.
Roh, T. H., Ahn, C. K., & Han, I. (2005). The priority factor model for customer relationship management system success. Expert Systems with Applications, 28 , 641–654.
Min, H., & Lambert, T. (2002). Truck driver shortage revisited. Transportation journal, 42 , 5–16.
Lin, H. H., & Wang, Y. S. (2006). An examination of the determinants of customer loyalty in mobile commerce contexts. Information & Management, 43 , 271–282.
Sweeney, J., & Swait, J. (2008). The effects of brand credibility on customer loyalty. Journal of Retailing and Consumer Services, 15 , 179–193.
Ball, D., Coelho, P. S., & Vilares, M. J. (2006). Service personalization and loyalty. Journal of Services Marketing, 20 , 391–403.
Gómez, B. G., Arranz, A. G., & Cillan, J. G. (2006). The role of loyalty programs in behavioral and affective loyalty. Journal of Consumer Marketing, 23 , 387–396.
Leenheer, J., Van Heerde, H. J., Bijmolt, T. H. A., & Smidts, A. (2007). Do loyalty programs really enhance behavioral loyalty? An empirical analysis accounting for self-selecting members. International Journal of Research in Marketing, 24 , 31–47.
Wallenburg, C. (2009). Innovation in logistics outsourcing relationships: Proactive improvement by logistics service providers as a driver of customer loyalty. Journal of Supply Chain Management, 45 , 75–93.
Oztaysi, B., Kaya, T., & Kahraman, C. (2011). Performance comparison based on customer relationship management using analytic network process. Expert Systems with Applications, 38 , 9788–9798.
Schweidel, D. A., Fader, P. S., & Bradlow, E. T. (2008). A bivariate timing model of customer acquisition and retention. Marketing Science, 27 , 829–843.
Arnold, T. J., Fang, E., & Palmatier, R. W. (2011). The effects of customer acquisition and retention orientations on a firm’s radical and incremental innovation performance. Journal of the Academy of Marketing Science, 39 , 234–251.
Villanueva, J., Yoo, S., & Hanssens, D. M. (2008). The impact of marketing-induced versus word-of-mouth customer acquisition on customer equity growth. Journal of Marketing Research, 45 , 48–59.
Bougie, R., Pieters, R., & Zeelenberg, M. (2003). Angry customers don’t come back, they get back: The experience and behavioral implications of anger and dissatisfaction in services. Journal of the Academy of Marketing Science, 31 , 377–393.
Richins, M. L. (1983). Negative word-of-mouth by dissatisfied consumers: A pilot study. The Journal of Marketing, 68–78.
M. Jarrar, R. Verlinden, and R. Meersman (2003). Ontology-based customer complaint management. In On the move to meaningful internet systems 2003: OTM 2003 workshops (pp. 594–606). Berlin Heidelberg, Springer.
Hulten, P. (2011). A Lindblomian perspective on customer complaint management policies. Journal of Business Research, 65 , 788–793.
Homburg, C., & Fürst, A. (2005). How organizational complaint handling drives customer loyalty: An analysis of the mechanistic and the organic approach. Journal of Marketing, 69 , 95–114.
Stauss, B. (2002). The dimensions of complaint satisfaction: Process and outcome complaint satisfaction versus cold fact and warm act complaint satisfaction. Managing Service Quality, 12 , 173–183.
Ro, H., & Wong, J. (2012). Customer opportunistic complaints management: A critical incident approach. International Journal of Hospitality Management, 31 , 419–427.
Karatepe, O. M. (2006). Customer complaints and organizational responses: The effects of complainants’ perceptions of justice on satisfaction and loyalty. International Journal of Hospitality Management, 25 , 69–90.
Davidow, M. (2003). Organizational responses to customer complaints: What works and what doesn’t. Journal of Service Research, 5 , 225–250.
Luo, X., & Homburg, C. (2007). Neglected outcomes of customer satisfaction. Journal of Marketing, 71 , 133–149.
Kwong, C. K., Wong, T. C., & Chan, K. Y. (2009). A methodology of generating customer satisfaction models for new product development using a neuro-fuzzy approach. Expert Systems with Applications, 36 , 11262–11270.
Briggs, E., Landry, T. D., & Daugherty, P. J. (2010). Investigating the influence of velocity performance on satisfaction with third party logistics service. Industrial Marketing Management, 39 , 640–649.
Sivadas, E., & Baker-Prewitt, J. L. (2000). An examination of the relationship between service quality, customer satisfaction, and store loyalty. International Journal of Retail & Distribution Management, 28 , 73–82.
Steven, A. B., Dong, Y., & Dresner, M. (2012). Linkages between customer service, customer satisfaction and performance in the airline industry: Investigation of non-linearities and moderating effects. Transportation Research Part E: Logistics and Transportation Review, 48 , 743–754.
Bayraktar, E., Tatoglu, E., Turkyilmaz, A., Delen, D., & Zaim, S. (2012). Measuring the efficiency of customer satisfaction and loyalty for mobile phone brands with DEA. Expert Systems with Applications, 39 , 99–106.
Edvardsson, B., Gustafsson, A., & Roos, L. U. (2010). Improving the prerequisites for customer satisfaction and performance: A study of policy deployment in a global truck company. International Journal of Quality and Service Sciences, 2 , 239–258.
Tahriri, F., Osman, M. R., Ali, A., Yusuff, R. M., & Esfandiary, A. (2008). AHP approach for supplier evaluation and selection in a steel manufacturing company. Journal of Industrial Engineering and Management, 1 , 54–76.
Moshref Javadi, M. H., & Azmoon, Z. (2011). Ranking branches of system group company in Terms of acceptance preparation of electronic customer relationship management using AHP method. Procedia Computer Science, 3 , 1243–1248.
Bayazit, O. (2005). Use of AHP in decision-making for flexible manufacturing systems. Journal of Manufacturing Technology Management, 16 , 808–819.
Wang, Y.-J. (2010). A clustering method based on fuzzy equivalence relation for customer relationship management. Expert Systems with Applications, 37 , 6421–6428.
Lin, J., & Lee, M.-C. (2004). An object-oriented analysis method for customer relationship management information systems. Information and Software Technology, 46 , 433–443.
Anderson, J. L., Jolly, L. D., & Fairhurst, A. E. (2007). Customer relationship management in retailing: A content analysis of retail trade journals. Journal of Retailing and Consumer Services, 14 , 394–399.
Bowen, J. T., & Sparks, B. A. (1998). Hospitality marketing research: A content analysis and implications for future research. International Journal of Hospitality Management, 17 , 125–144.
McDonald, W. J. (1994). Developing international direct marketing strategies with a consumer decision-making content analysis. Journal of Direct Marketing, 8 , 18–27.
Gebauer, J., Tang, Y., & Baimai, C. (2007). User requirements of mobile technology—Results from a content analysis of user reviews , Champaign: University of Illinois at Urbana-Champaign.
McAlister, D. T., & Erffmeyer, R. C. (2003). A content analysis of outcomes and responsibilities for consumer complaints to third-party organizations. Journal of Business Research, 56 , 341–351.
Atkins, C., & Sampson, J. (2002). Critical appraisal guidelines for single case study research. In Proceedings of the European Conference on Information Systems, June (pp. 6–8).
Lemon, K. N., White, T. B., & Winer, R. S. (2002). Dynamic customer relationship management: Incorporating future considerations into the service retention decision. The Journal of Marketing, 1–14.
Nambisan, S., & Baron, R. A. (2007). Interactions in virtual customer environments: Implications for product support and customer relationship management. Journal of Interactive Marketing, 21 , 42–62.
Limayem, M., & Cheung, C. M. K. (2008). Understanding information systems continuance: The case of Internet-based learning technologies. Information & Management, 45 , 227–232.
Ko, E., Kim, S. H., Kim, M., & Woo, J. Y. (2008). Organizational characteristics and the CRM adoption process. Journal of Business Research, 61 , 65–74.
Krasnikov, A., Jayachandran, S., & Kumar, V. (2009). The impact of customer relationship management implementation on cost and profit efficiencies: Evidence from the US commercial banking industry. Journal of Marketing, 73 , 61–76.
Stein, A., & Smith, M. (2009). CRM systems and organizational learning: An exploration of the relationship between CRM effectiveness and the customer information orientation of the firm in industrial markets. Industrial Marketing Management, 38 , 198–206.
Rygielski, C., Wang, J. C., & Yen, D. C. (2002). Data mining techniques for customer relationship management. Technology in Society, 24 , 483–502.
Choy, K. L., Lee, W. B., & Lo, V. (2002). Development of a case based intelligent customer–supplier relationship management system. Expert Systems with Applications, 23 , 281–297.
Business-Software. (2012). TOP 40 CRM Software Vendors. Available: http://www.business-software.com/crm/crm.php .
Business-Software. (2010). TOP 40 CRM Software vendors revealed. 100. Available: http://www.business-software.com/top-40-crm-vendors.php .
Integrity, Q. (2009). Sage SalesLogix vs. SalesForce.com. Available: http://www.qualityintegrity.com/compare_saleslogix_vs_salesforce.asp .
N. R. Inc. (2007). Guidebook, Oracle’s Siebel CRM on demand. Available: http://crmondemand.oracle.com/ocom/groups/public/@crmondemand/documents/webcontent/6071_en.pdf .
M. Software. (2009). Maximizer CRM Central. Available: http://www.maximizercrmcentral.com/forums/p/183/490.aspx .
SiteGround. (2009). What is Vtiger. Available: http://www.siteground.com/tutorials/vtiger/ .
S. Software. (2007). SageCRM Customer Care. Available: http://www.sagecrmsolutions.com/assets/Collateral/SageCRMCustSupprtLo.pdf .
U. S. Inc. (2007). Sage CRM—What it Can and Cannot Do—Part 1. Available: http://blog.unisoft.net/category/sage-crm/ .
M. Dynamics. (2009). Microsoft Dynamics CRM. Available: http://crm.dynamics.com/solutions/crm-solutions-overview.aspx .
Faed, A., Wu, C., & Chang, E. (2010) Intelligent CRM on the Cloud. In Network-Based Information Systems (NBiS), 2010 13th International Conference on 2010 , pp. 216–223.
H. V. LLC. (2009). VTiger Summary. Available: http://www.seekdotnet.com/vtigerhosting.aspx .
D. CRM. (2011). Daffodil. Available: http://crm.daffodilsw.com/ .
Galitsky, B. A., González, M. P., & Chesņevar, C. I. (2009). A novel approach for classifying customer complaints through graphs similarities in argumentative dialogues. Decision Support Systems, 46 , 717–729.
Atalik, Ö. (2007). Customer complaints about airline service: A preliminary study of Turkish frequent flyers. Management Research News, 30 , 409–419.
Ferguson, J. L., & Johnston, W. J. (2011). Customer response to dissatisfaction: A synthesis of literature and conceptual framework. Industrial Marketing Management, 40 , 118–127.
Bennett, R., & Savani, S. (2011). Complaints-handling procedures of human services charities: Prevalence, antecedents, and outcomes of strategic approaches. Managing Service Quality, 21 , 484–510.
Kaltcheva, V. D., Winsor, R. D., & Parasuraman, A. (2013). Do customer relationships mitigate or amplify failure responses? Journal of Business Research, 66 , 525–532.
Coussement, K., & Van den Poel, D. (2008). Improving customer complaint management by automatic email classification using linguistic style features as predictors. Decision Support Systems, 44 , 870–882.
Galitsky, B. A., González, M. P., & Chesñevar, C. I. (2009). A novel approach for classifying customer complaints through graphs similarities in argumentative dialogues. Decision Support Systems, 46 , 717–729.
Download references
Author information
Authors and affiliations.
Curtin Business School, School of Information Systems, EU 4 Technology Park, Curtin University, Kent Street, Perth, WA, 6102, Australia
Alireza Faed
You can also search for this author in PubMed Google Scholar
Corresponding author
Correspondence to Alireza Faed .
Rights and permissions
Reprints and permissions
Copyright information
© 2013 Springer Heidelberg
About this chapter
Faed, A. (2013). Literature Review. In: An Intelligent Customer Complaint Management System with Application to the Transport and Logistics Industry. Springer Theses. Springer, Heidelberg. https://doi.org/10.1007/978-3-319-00324-5_2
Download citation
DOI : https://doi.org/10.1007/978-3-319-00324-5_2
Published : 13 June 2013
Publisher Name : Springer, Heidelberg
Print ISBN : 978-3-319-00323-8
Online ISBN : 978-3-319-00324-5
eBook Packages : Engineering Engineering (R0)
Share this chapter
Anyone you share the following link with will be able to read this content:
Sorry, a shareable link is not currently available for this article.
Provided by the Springer Nature SharedIt content-sharing initiative
- Publish with us
Policies and ethics
- Find a journal
- Track your research
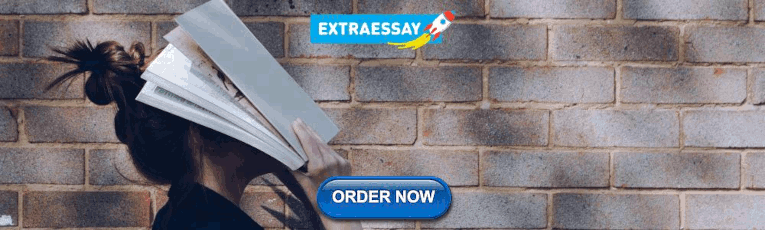
IMAGES
VIDEO
COMMENTS
MEASURING CUSTOMER SATISFACTION: A LITERATURE REVIEW. April 2015; Conference: Finance and Performance of Firms in Science, Education and Practice 2015 ... For example, Stauss and Neuhaus (1997 ...
To explore the field further, the purpose of this paper is twofold. First, it offers a literature review on customer satisfaction taking various papers published into account. Second, it offers a conceptual framework to summarize the research in this field comprising three parts.
Like Sánchez-Rebull, (2018) conducted a systematic literature review on customer satisfaction. A literature review was conducted on 495 articles published in 41 journals from 1988 to 2016 (29 years).
The existing state of customer experience research was assessed by reviewing 99 articles. Table 2 reveals that the customer experience has been studied in four categories; with most of the articles published in the context of experience with a brand (n = 35), followed by the context of experience with a product/service (n = 28), experience with a website or a specific medium (n = 19), and the ...
1. Introduction. Customer satisfaction, loyalty, product knowledge and competitive ability are variables which have been researched extensively across the globe. The relationships which tend to be researched the most are customer satisfaction and loyalty (e.g., Fornell, Johnson, Anderson, Cha, & Bryant, 1996; Türkyilmaz & Özkan, 2007 ).
This study is a review that identifies customer satisfaction as the dependent variable, through a systematic review of online literature sources. Three independent variables were then identified ...
This literature review shows that the spread of knowledge about customer-related improvements is wide, ranging from value chain improvements in the mango industry (e.g., Badar et al., 2015) to improving client-centered care (e.g., Broekhuis et al., 2009 ). However, it has several gaps that can make it difficult for researchers to comprehend and ...
The customer experience (CX) is a concept that has been closely studied by companies in recent years. This is because it is one of the most important factors in maintaining a competitive advantage. In addition, it is customers who seek to create an experience that goes beyond the acquisition of a product. In this article, we present a systematic literature review of the CX in retail. We ...
There is a desperate need for new research that will advance customer satisfaction (CS) and service quality (SO) methodologies in the hospitality industry. This comprehensive review of the theories and methodologies reported in CS and SQ studies cited in the hospitality literature provides suggestions for future CS and SQ research in the ...
Customer satisfaction is the key factor for successful and depends highly on the behaviors of frontline service providers. Customers should be managed as assets, and that customers vary in their needs, preferences, and buying behavior. This study applied the Taiwan Customer Satisfaction Index model to a tourism factory to analyze customer satisfaction and loyalty. We surveyed 242 customers ...
Customer satisfaction (CS) has attracted serious research attention in the recent past year. Customer satisfaction is now for all companies the primary criterion for the assessment of their relationship with the market, a permanent object of their operating policies and an important element for the reinforcement of company reputation, as well as a fundamental guide to direct operational processes.
The main goal of this systematic literature review is to understand whether there are common approaches to establish collaborations with customers, the main challenges, the main factors that affect the customer satisfaction, and whether the chosen software development methodology plays any role.
Examples of literature reviews. Step 1 - Search for relevant literature. Step 2 - Evaluate and select sources. Step 3 - Identify themes, debates, and gaps. Step 4 - Outline your literature review's structure. Step 5 - Write your literature review.
MEASURING CUSTOMER SATISFACTION: A LITER ATURE REVIEW. Nadia Angraini, Rahmi Fahmy, Hafiz Rahm an, Ma'ruf4. 1 Management Science Doctoral Program. Unand Padang, Indonesia. 2,3,4 Management Study ...
Customer satisfaction is the key factor for successful and depends highly on the behaviors of frontline service providers. ... Literature review. ... The Taiwan customer satisfaction index model in related to sample decision. Qual Mag. 2006; 42 (4):74-77. [Google Scholar] Lee YC, Chen CY, Tsai SB, Wang CT. Discussing green environmental ...
Based on the review of available literature, customer satisfaction was considered as a dependent variable ... The literature review consists up concepts and theory regarding the research area. Similarly, it includes review of articles related to the study. ... sample size calculation and sampling, data analysis and data management. Findings are
An extensive review of the literature suggests a lack of bibliometric studies that examine and scientifically map the body of knowledge related to service quality and customer satisfaction. This research aims to examine the trends in service quality and customer satisfaction research, identify the gaps, and propose future research agenda.
Smart services serve customers and their individual, continuously changing needs; information and communications technology enables such services. The interactions between customers and service providers form the basis for co-created value. A growing interest in smart services has been reported in the literature in recent years. However, a categorization of the literature and relevant research ...
LITERATURE REVIEW Customer Satisfaction Customer Satisfaction is afeeling of pleasure or disappointment of someone who appears after comparing the performance (results) of the product thought against the expected performance results (Kotler 2006:177, 2019) ). The dimension or indicator of Customer
Okay - with the why out the way, let's move on to the how. As mentioned above, writing your literature review is a process, which I'll break down into three steps: Finding the most suitable literature. Understanding, distilling and organising the literature. Planning and writing up your literature review chapter.
PDF | On Jan 1, 2018, Sokchan Ok and others published Customer Satisfaction and Service Quality in the Marketing Practice: Study on Literature Review | Find, read and cite all the research you ...
In this chapter, we review the current literature on customer relationship management including its definitions, concepts and the solutions provided by CRM systems and strategies. We also examine the underlying components of these systems including customer satisfaction, customer acquisition, customer loyalty, perceived value and interactivity.
The study examines the literature on Customer Relationship Management (CRM), with a particular emphasis on CRM's effect on client satisfaction and customer loyalty.
the main theme of Cust omer Relationship Management (CRM) (Rababah, Moh d and Ibrahim, 2011). T he. reality is customer str ategy is all a bout using information to gain c ompetitive advantage to ...