
An official website of the United States government
The .gov means it’s official. Federal government websites often end in .gov or .mil. Before sharing sensitive information, make sure you’re on a federal government site.
The site is secure. The https:// ensures that you are connecting to the official website and that any information you provide is encrypted and transmitted securely.
- Publications
- Account settings
Preview improvements coming to the PMC website in October 2024. Learn More or Try it out now .
- Advanced Search
- Journal List
- Int J Environ Res Public Health

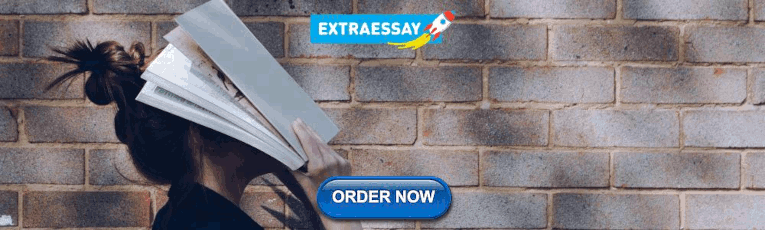
Power Distance Belief and Workplace Communication: The Mediating Role of Fear of Authority
1 Beijing Key Laboratory of Behavior and Mental Health, School of Psychological and Cognitive Sciences, Peking University, Beijing 100871, China; nc.ude.ukp@nawuyiad
2 Plateau Brain Science Research Center, Tibet University, Lhasa 850001, China
Wenting Xie
3 School of Education, Tibet University, Lhasa 850001, China; moc.361@twxyzsg
Tianyi Deng
4 Health Science Center, Peking University, Beijing 100871, China; nc.ude.ukp@9110210171
Associated Data
The data presented in this study are available on request from the corresponding author.
Power distance is the degree of acceptance of unequal distribution of power in societies. In a high power distance context, the acceptance of inequality conflicts with the operation of modern organizations, which causes obstacles to workplace communication or even triggers workplace accidents due to ineffective communication. We conducted four studies ( N = 1063) to explore the relations between and mechanisms of power distance belief and workplace communication. In Study 1, the participants with high power distance belief had ineffective workplace communication—specifically ineffective communication with superiors—but no difference in communication with subordinates and colleagues. We further focused on the mechanism underlying the relationship between power distance belief and communication with superiors. A questionnaire study (Study 2) was conducted in three stages over a three-month period, and an experimental study (Study 3) indicated that fear of authority mediated the negative effect of high power distance on communication with superiors. A cross-culture study (Study 4) re-tested the hypotheses among Chinese and U.S. participants. This research provides insight into the mechanisms that explain the relationship between power distance belief and workplace communication, indicating that fear of authority is significant. Organizations should pay attention to power distance belief and fear of authority, as they may lead to workplace accidents due to communication disasters.
1. Introduction
Workplace communication plays a crucial role in industrial organization with the development of economic globalization as well as the greater complexity of organizational structures. Cultural factors play an important role in workplace communication [ 1 , 2 , 3 ]. Individualism–collectivism affects communication styles directly and also has an indirect effect that is mediated through self-constructs and values [ 4 , 5 ]. As another important cultural factor, power distance belief has also been found to influence communication between teachers and students [ 6 ]. In our current research, we aimed to explore the relationship between power distance belief and workplace communication. Moreover, we proposed that fear of authority could account for the effect of power distance belief on workplace communication.
1.1. High Power Distance Leads to Inefficient Communication
Power distance belief refers to the degree to which individuals, groups, or societies accept the unequal distribution of power, state, or wealth in society or organizations [ 7 ]. Power distance belief is originally a homogeneous national value [ 7 ]; it is also a dispositional trait at the individual level [ 8 , 9 , 10 ]. Daniels and Greguras [ 11 ] indicated that low power distance countries embraces both high power distance belief individuals and low power distance belief individuals. The central characteristic of power distance is acceptance of inequality [ 7 , 11 ]. Specifically, in terms of attitude, individuals with high power distance belief are more likely to accept power inequalities in their organizations [ 12 ]. Accordingly, they would approve of hierarchy and hold the opinion that subordinates should trust and obey the superiors [ 13 , 14 ]. In terms of behavior, individuals with high power distance belief hold weaker trust in others [ 15 ], more feedback seeking from peers, less feedback seeking from superiors [ 16 ], and weaker innovation [ 17 ].
Effective communication is essential to the survival of all organizations [ 18 , 19 ]. Communication refers to a transaction whereby individuals generate meaning through the exchange of symbols [ 20 ]. Two central characteristics of communication are interaction and achieving consistency in the meaning of symbols [ 21 ]. Communication occurs every day in organizations. Effective communication contributes to the daily running, promotes organization benefits, and avoids workplace accidents [ 22 , 23 , 24 ]. Previous studies focused on improving personal communication skills (e.g., listening well, speaking clearly, inquiring skillfully) to enhance workplace communication [ 25 ]. Recent studies indicated that cultural factors play an important role in workplace communication [ 2 , 3 ].
Psychologists have a theoretical speculation on the link between power distance belief and effective communication at work [ 18 ]. Suter et al. [ 26 ] further conducted qualitative research and found that participants emphasize effective communication as a core competency for collaborative practice in interviews. Previous research provides pieces of experimental evidence for this link. On the one hand, individuals with high power distance in organizations interact less in the organization. Ji et al. [ 15 ] found that high power distance individuals are less likely to seek help. Varela and Premeaux [ 27 ] found that subordinates with high power distance tend to keep silent and are less willing to provide feedback. On the other hand, individuals with high power hold low communication openness, which hinders the exchange of information. Superiors with higher power distance belief tend to have an egocentric decision-making style [ 7 ], distrusting subordinates’ opinions [ 10 ]. Meanwhile, subordinates with high power distance belief tend to rely on superiors’ opinions for decision-making [ 7 ], tolerate supervisory mistreatment [ 28 ], and perform more upward ingratiatory behavior to please leaders even if it is against objective facts [ 29 ]. Hence, we proposed that high power distance belief is likely to inhibit people from communicating effectively. This is our first Hypothesis (H1):
High power distance belief is positively associated with more ineffective communication in the workplace.
1.2. Fear of Authority Can Result in Inefficient Communication
Fear of authority is an emotional state in which individuals feel fearful of authority and the evaluation of authority [ 30 ]. Opposite to positive emotions such as admiration and respect, fear of authority is a negative emotion in valence and holds an avoidance motivation [ 31 , 32 ]. Emotionally, in addition to fear and anxiety, individuals may experience self-doubt, disgust, disappointment, and anger [ 33 , 34 ]. Behaviorally, fear elicits alienation, avoiding authority [ 35 ], obedience, conflict avoidance [ 36 ], and expectation of approval [ 37 ]. Fear of authority is elicited not only by authorities and experts but also by high social status, elderly people, superiors, and other people of higher social classes [ 38 ], and even people who dress as if “higher class” can cause those with low self-confidence to experience a fear of authority [ 39 ].
Fear of authority can decrease the effectiveness of communication. Emotional experiences such as fear and anxiety elicit avoidance behavior rather than approach behavior [ 40 , 41 ]. An avoidance behavior that seems ubiquitous in this regard is remaining silent [ 42 , 43 ]. Keeping silent results in less communication, discrete information sharing, and poor feedback. Furthermore, as in the well-known Milgram studies of obedience to authority, it is prevalent to obey authority even when doing so is problematic [ 44 ]. Fear of authority results in compliance with the authority’s orders without consideration. Fear of authority allows superiors to exert strong social pressure, which puts the individual in a situation of conformity, as in peer-pressure situations [ 45 ]. As a result, the individual tends to avoid responsibility and tends to follow orders without question. In addition, individuals with a high fear of authority tend to believe that authority naturally means correct and professional, which can result in cognitive bias. Accordingly, challenging the views of authority would be incorrect, and agreeing is a wise choice. Fear of authority can also lead individuals to change their own opinions to be consistent with the authority in terms of their thoughts, attitudes, and behaviors. The most frequently mentioned reason for remaining silent is the fear of being viewed or labeled negatively, and thus, damaging valued relationships [ 46 ]. In high fear of authority situations, individuals often have increased fear of negative evaluations and social anxiety, which weaken their self-esteem [ 47 , 48 ]. Seeking consistency with authority is a means of obtaining connections and improving self-esteem [ 49 ]. Even if individuals have doubts about their superiors’ decisions, they may be unwilling to express this doubt and even persuade themselves that the decision is correct to address the cognitive dissonance. Therefore, we proposed our second Hypothesis (H2):
Fear of authority is positively associated with more ineffective communication in the workplace.
Fear of authority is an emotional experience that is influenced by personality [ 50 ], environmental context [ 35 ], and culture [ 10 ]. Power distance is an important cultural factor, and previous studies have found that power distance is associated with emotional expression and emotional experience [ 51 ]. For example, under high power distance conditions, people are more eager to be accepted by others [ 52 ]. In addition, Mondillon et al. [ 53 ] indicated that people with power tend to feel more anger and pride, and people without power feel more fear and sadness. People with high power distance belief are more likely to perceive their superiors as authoritarian [ 11 , 51 ] and feel less powerful, which may generate a higher sense of fear of authority. Therefore, we proposed our third Hypothesis (H3) and fourth Hypothesis (H4):
Power distance belief is positively associated with fear of authority.
Fear of authority mediates the effect of power distance belief on workplace communication.
To summarize, empirical research is needed to explore the effect of power distance belief on workplace communication. How power distance belief impacts workplace communication requires further investigation to buffer the negative impacts. We hypothesize that individuals with higher power distance belief experience more fear of authority, which negatively affects communication ( Figure 1 ). We will test these hypotheses through four studies.

A mediation model of power distance belief, fear of authority, and workplace communication.
1.3. The Current Research
We focused, in four studies, on the correlational and causal relation between power distance belief and workplace communication as well as the mechanisms under this relation. In Study 1, we examined the association between individual differences in power distance belief and workplace communication. In Study 2, we tested whether fear of authority mediates the relationship between power distance belief and workplace communication via a questionnaire study in three stages over a three-month period. In Study 3, we conducted an empirical experiment to re-test the mechanisms and provided causal evidence. We manipulated power distance belief and assessed fear of authority as well as workplace communication. In Study 4, we conducted a cross-cultural study, recruiting Chinese participants and American participants, to further test the hypothesis and improve the robustness. We recruited participants from high power distance belief culture (i.e., China) and low power distance belief culture (i.e., the United States) and assessed fear of authority as well as workplace communication. Due to COVID-19, we recruited participants on online recruitment platforms (i.e., Credamo, Turkprime) which are reliable and have been widely used [ 54 , 55 ]. Meanwhile, we used questions to exclude participants who do not have workplace communication experience. In addition, we recruited different samples for the four studies to improve the robustness. We expected that people with high power distance belief would have lower communication efficiency in the workplace and that fear of authority would account for the inefficient communication. The study was conducted according to the guidelines of the Declaration of Helsinki and approved by the Ethics Committee of Peking University.
In Study 1, we conducted a correlational investigation and tested H1 at dispositional level: power distance belief is negatively associated with workplace communication. To attain a nuanced understanding of power distance belief’s effect on communication, we examined three attitude outcomes—communication with superiors, communication with colleagues, and communication with subordinates.
2.1. Method
2.1.1. participants.
Based on Monte Carlo simulations, Schönbrodt and Perugini [ 56 ] recommended using a sample approaching 250 for stable estimates of bivariate correlations. We recruited 250 Chinese participants on the online platform Credamo [ 57 ]. Credamo, the Chinese equivalent of Mturk, is a reliable Chinese recruitment platform [ 54 , 55 ]. We excluded 4 for failing an attention check, leaving 246 participants (246 Han Chinese, 0 other; 127 women, 119 men; M age = 25.88, SD age = 5.37) in the sample. Of them, 8.5% had a high school or college degree, 79.7% had a bachelor’s degree, and 11.8% had a master’s or PhD degree.
2.1.2. Procedure and Materials
We used a question to excluded participants who had never experienced workplace communication before: I have had experience communicating with superiors, subordinates, and colleagues in the workplace. Only participants who answered yes would participate in the survey. This question was shown first in this study and the following studies. We used Brislin’s [ 58 ] procedures to translate and back translate all materials and measures from English to Chinese. We measured dispositional power distance belief with the six-item Power Distance Scale [ 59 ]. Sample items include “Managers should not delegate important tasks to employees” and “Managers should avoid off-the-job social contacts with employees” (1 = strongly disagree, 5 = strongly agree; M = 2.85, SD = 0.87, α = 0.80).
We measured workplace communication in three aspects with fifteen self-composed items: communication with superiors, communication with colleagues, and communication with subordinates. We designed the measurement by modifying the communication openness scale [ 14 , 60 ]. We measured information sending and information receiving behaviors, the communication atmosphere, and the contribution to the work task. We measured communication with superiors using five self-composed items. Items are “At work, I can communicate effectively with leaders”, “At work, when I report to the leaders on a project, the leader can clearly understand the project”, “At work, the content of my expression is understandable for the superiors”, “I feel anxiety when I communicate with my leaders”, and “At work, I can accurately understand the meaning of my leaders’ words.” We reversed scored, as appropriate, and averaged responses to create an index of communication with superiors (1 = not at all, 5 = extremely; M = 3.71, SD = 0.86, α = 0.84). We measured communication with subordinates using five self-composed items. Sample items are “When I communicate with subordinates, she/he can clearly understand what I tell them to do” and “At work, I have misunderstandings with my subordinates because of poor communication.” We reversed scored, as appropriate, and averaged responses to create an index of communication with subordinates (1 = not at all, 5 = extremely; M = 3.86, SD = 0.58, α = 0.73). (Specific items of communication with subordinates can be found in the Supplementary Materials ). We measured communication with colleagues using five self-composed items. Sample items are “At work, I can communicate effectively with colleagues” and “At work, the content of my expression is understandable for the colleagues.” We reversed scored, as appropriate, and averaged responses to create an index of communication with colleagues (1 = not at all, 5 = extremely; M = 2.64, SD = 0.99, α = 0.76). (Specific items of communication with colleagues could be found in the Supplementary Materials ). Workplace communication was measured using 15 self-composed items, including communication with superiors, communication with colleagues, and communication with subordinates. We reversed scored, as appropriate, and averaged responses to create an index of workplace communication (1 = not at all, 5 = extremely; M = 3.40, SD = 0.49, α = 0.79). Answers to demographic questions concluded the session.
2.2. Results and Discussion
As hypothesized, dispositional power distance belief was negatively associated with workplace communication, r (246) = −0.31, p < 0.001; dispositional power distance belief was negatively associated with communication with superiors, r (246) = −0.32, p < 0.001; dispositional power distance belief was close to being statistically associated with communication with colleagues, r (246) = −0.12, p = 0.059; and dispositional power distance was not associated with communication with subordinates, r (246) = −0.11, p = 0.09.
Consistent with H1, individuals with higher power distance belief exhibit lower communication efficiency in the workplace. Specifically, individuals with high power distance belief communicate with their superiors less often. Meanwhile, power distance belief is not significantly related to communication with subordinates and colleagues. In conclusion, power distance belief hindered only communication with superiors.
Study 1 found a negative relationship between power distance and workplace communication with superiors. In Study 2, we extended our thinking to a mechanism and first tested H2, H3, as well as H4 at a dispositional level. We aimed to test whether fear of authority could account for the negative effect of power distance belief on workplace communication. Here, and in all further studies, we retested H1–H4.
3.1. Method
3.1.1. participants.
We used Monte Carlo power analysis for indirect effects [ 61 ] ( https://schoemanna.shinyapps.io/mc_power_med/ , accessed on 5 August 2021) to determine the sample size for our proposed mediational model. We needed at least 163 participants to reach a power of 0.80, assuming correlations of r = 0.30 (SD = 1.00) among the predictor (independent variable), the mediator, and the dependent variable. In anticipation of attrition, we recruited 220 Chinese participants on the reliable online platform Credamo [ 57 ]. We excluded 9 for failing an attention check, leaving 211 participants (211 Han Chinese, 0 other; 107 women, 104 men; M age = 36.44, SD age = 5.26) in the sample. Of them, 8.1% had a high school or college degree, 75.8% had a bachelor’s degree, and 16.1% had a master’s or PhD degree.
3.1.2. Procedure and Materials
We collected data in three stages over a three-month period, using email addresses as the basis for matching and tracking. We measured dispositional power distance belief with the six-item Power Distance Scale [ 59 ], as in Study 1 (1 = strongly disagree, 5 = strongly agree; M = 2.85, SD = 0.88, α = 0.80). After six weeks, fear of authority was measured by the eight-item Fear of Authority Scale [ 30 ]. Sample items are “I feel fear in front of people with higher ranks” and “I feel uncomfortable when I interact with people of high status” (1 = not at all, 5 = extremely; M = 3.41, SD = 0.76, α = 0.84). Six weeks later, we measured workplace communication with superiors, as in Study 1 (1 = not at all, 5 = extremely; M = 3.79, SD = 0.65, α = 0.75). Answers to demographic questions concluded the session.
3.2. Results and Discussion
3.2.1. correlations.
As in Study 1 and consistent with H1, dispositional power distance belief was negatively associated with workplace communication with superiors, r (211) = −0.21, p = 0.003. Consistent with H2, fear of authority was negatively associated with communication with superiors, r (211) = −0.34, p < 0.001. Consistent with H3, dispositional power distance belief was positively associated with fear of authority, r (211) = 0.56, p < 0.001.
3.2.2. Mediational Analysis
We carried out a bootstrapping mediational analysis [ 62 ] (PROCESS, model 4) with 5000 iterations. We entered dispositional power distance belief as an independent variable, fear of authority as a mediator, and workplace communication with superiors as a dependent variable. The indirect effect was significant, b = −0.18, SE = 0.05, 95% (−0.2840, −0.0794) ( Figure 2 ). Controlling for age and education, the indirect effect remained significant, b = −0.20, SE = 0.06, 95% (−0.3120, −0.0920). Fear of authority mediated the relation of dispositional power distance belief on workplace communication with superiors, in support of H4.

Fear of authority mediates the relationship between power distance belief and communication with superiors in Study 2. Unstandardized coefficients displayed. ** p < 0.01, *** p < 0.001.
In Study 3, we aimed to extend Study 2 in two ways. First, Study 1 and Study 2 provided correlational evidence for the hypotheses. In Study 3, we conducted an experimental study and provided further causal evidence. We manipulated power distance belief with the sentence-scrambling task [ 63 ], as in former studies [ 64 , 65 ]. In the sentence-scrambling task, participants construct sentences by only using provided words. The completed sentences are related to social hierarchy or equality. When participants complete ten sentences and focus on sentences associated with the power-distance belief, the power-distance belief should become momentarily accessible [ 63 ]. Second, we retested the hypothesis with a different measure of workplace communication, scenario-based decision making. We designed a scenario related to workplace communication and participants needed to make a specific choice [ 64 , 66 ]. We used the choice index to indicate the communication effectivity with their supervisors. Study 4 could further provide robust evidence for the hypotheses.
4.1. Method
4.1.1. participants.
According to G*Power analysis [ 67 ], at least 172 participants were needed to detect a medium effect size ( f = 0.25) for a between-subjects design with power 0.90 (α = 0.05).
Additionally, we put an item at the beginning, “Do you have subordinates in workplace’’, and only people who have subordinates in the workplace were allowed to participate in this questionnaire. In anticipation of attrition, we recruited 195 Chinese participants on the reliable online platform Credamo [ 57 ]. We excluded 10 for failing an attention check, leaving 185 participants (185 Han Chinese, 0 other; 127 women, 58 men; M age = 29.05, SD age = 6.58) in the sample. Of them, 15.1% had a high school or college degree, 72.4% a bachelor’s degree, and 12.4% a master’s or PhD degree. We randomly assigned participants to the high power distance ( n = 93) or low power distance ( n = 92) condition.
4.1.2. Procedure and Materials
We manipulated power distance belief by the sentence-scrambling task [ 63 , 64 ]. Participants formed meaningful sentences from sets of scrambled words and only used the words being offered. These sentences were related to social hierarchy in the high power distance condition or related to equality in the low power distance condition. Specifically, we presented participants in the high power distance belief condition with ten tasks that express high power distance belief. A sample item is “Necessary superiors our social order obedience from is subordinates to for.” Participants should complete the task by forming these words into “Obedience from subordinates to superiors is necessary for our social order.” We presented participants in the low power distance condition with ten tasks that express low power distance belief. A sample item is “an organization is position in Everyone’s equal.” Participants should complete the task by forming these words into “Everyone’s position in an organization is equal.” We measured state dispositional power distance with the six-item Culture Attitudes Inventor as a manipulation check [ 59 ] as in Study 1(1 = strongly disagree, 5 = strongly agree; M = 2.30, SD = 0.67, α = 0.75).
Fear of authority was measured by the eight-item Fear of Authority Scale [ 30 ] as in Study 2 (1 = not at all, 5 = extremely; M = 3.34, SD = 0.78, α = 0.85).
Subsequently, participants were presented with an imaginary workplace situation that read as follows: “You are an engineer in charge of architectural design. Your supervisor asks you to modify the construction design of a building. His reason is that he wants to compress the cost of construction. And your analysis showed that the current design is the best balance of cost and safety. According to your professional knowledge and analysis, the modified design plan would reduce safety and security but not so much as to cause a safety accident.” Participants had to imagine they were the protagonist of the situation and indicate which of the communication decisions they would make. Participants indicated the extent to which they would make a case for the former plan and the extent to which they would follow the supervisor without communication (reversed item) on a seven-point Likert scale (1 = strongly disagree; 7 = strongly agree; r = 0.65, p < 0.001). The second item was reversed and the average of their scores reflected the workplace communication ( M = 5.44, SD = 1.22). Higher scores indicated more effective workplace communication. Answers to demographic questions concluded the session.
4.2. Results and Discussion
4.2.1. power distance manipulation check.
Participants in the high power distance condition ( M = 2.49, SD = 0.70) felt more power distance belief than those in the low power distance condition ( M = 2.11, SD = 0.58), F (1, 183) = 16.08, p < 0.001, η p 2 = 0.081. The manipulation was effective.
4.2.2. Fear of Authority
As we expected, participants in high power distance condition ( M = 3.51, SD = 0.71) felt more fear of authority than those in the low power distance condition ( M = 3.17, SD = 0.82), F (1, 183) = 8.69, p = 0.004, η p 2 = 0.045, indicating that power distance belief increases fear of authority.
4.2.3. Workplace Communication with Superiors
Participants in high power distance condition ( M = 5.17, SD = 1.40) reported less communication than those in the low power distance condition ( M = 5.71, SD = 0.93), F (1, 183) = 9.69, p = 0.002, η p 2 = 0.050. High power distance belief led to less workplace communication.
4.2.4. Mediational Analysis
We carried out a bootstrapping mediational analysis [ 62 ] (PROCESS, model 4) with 5000 iterations. We entered the power distance as independent variable (0 = low power distance, 1 = high power distance), fear of authority as mediator, and workplace communication with superiors as dependent variable. The indirect effect was significant b = −0.23, SE = 0.09, 95% (−0.4168, −0.0740) ( Figure 3 ). Fear of authority mediated the effect of power distance on workplace communication with superiors.

Fear of authority mediates the effect of power distance belief on communication with superiors in Study 3. Unstandardized coefficients displayed. † p < 0.08, ** p < 0.01, *** p < 0.001.
To further test the hypotheses of whether fear of authority mediates the relationship between power distance belief and workplace communication, we conducted a cross-cultural study in Study 4. We manipulated power distance belief by collecting participants with an American or Chinese cultural background, as these cultures exhibit significant differences in power distance belief [ 7 ]. According to the hypotheses, Chinese participants with high authority fear would be less effective in communicating with their superiors in the workplace than the American participants.
5.1. Method
5.1.1. participants.
A G*Power analysis [ 67 ] showed that at least 256 participants were needed to detect a medium effect size ( η p 2 = 0.03) for a between-subject design with a power of 0.80 (α = 0.05).
In case of any necessary data exclusion, we aimed to recruit 220 American participants (recruitment criteria: residency in the United States, human intelligence test approval rate > 90%) via the Cloud Research Service of Turkprime and 220 Chinese participants on the reliable online platform Credamo [ 57 ]. A total of 440 participants participated in Study 4. We excluded 3 participants for noncompletion and 16 for failing attention check questions, resulting in 421 total participants (186 men, 235 women, M age = 34.54, SD age = 7.91), 211 American participants (89 men, 122 women, M age = 38.27, SD age = 8.46) and 210 Chinese participants (97 men, 113 women, M age = 30.70, SD age = 4.99). Among the American participants, 72.5% were White/Caucasian, 10.9% were African American, 5.7% were Hispanic, 8.5% were Asian, 0.9% were Native American, and 1.4% were of other races. For the highest level of education, 7.1% held a senior high school diploma, 28.4% held a college degree, 41.7% held a bachelor degree, 16.6% held a master’s degree, 3.8% held a PhD, and 2.4% held other education certifications. Among the Chinese participants, 100% were Han Chinese. For the highest level of education, 13.9% had a high school or associate’s degree, 75.7% had a bachelor’s degree, and 10.5% had a master’s or PhD degree.
5.1.2. Procedure and Materials
We measured dispositional power distance belief with the six-item Power Distance Scale [ 59 ] as in Study 1 (1 = strongly disagree, 5 = strongly agree; M = 2.88, SD = 0.89, α = 0.80). Fear of authority was measured by the eight-item Fear of Authority Scale [ 30 ] as in Study 2 (1 = not at all, 5 = extremely; M = 3.06, SD = 0.76, α = 0.82). We measured workplace communication with superiors as in Study 1 (1 = not at all, 5 = extremely; M = 2.99, SD = 1.09, α = 0.79). Answers to demographic questions concluded the session.
5.2. Results and Discussion
5.2.1. power distance check.
As intended, the Chinese participants ( M = 3.42, SD = 0.73) reported higher power distance scores than the American participants ( M = 2.35, SD = 0.70), F (1, 419) = 237.99, p < 0.001, η p 2 = 0.362, and the Chinese participants were more likely to agree with a higher power distance.
5.2.2. Fear of Authority
As we expected, the Chinese participants ( M = 3.37, SD = 0.71) felt more fear of authority than the American participants ( M = 2.74, SD = 0.66), F (1, 419) = 90.38, p < 0.001, η p 2 = 0.177, indicating that power distance increases fear of authority.
5.2.3. Workplace Communication with Superiors
The Chinese participants ( M = 2.12, SD = 0.76) reported less communication than the American participants ( M = 3.85, SD = 0.59), F (1, 419) = 702.42, p < 0.001, η p 2 = 0.626. Individuals with high power distance belief communicated less in the workplace.
5.2.4. Mediational Analysis
We carried out a bootstrapping mediational analysis [ 62 ] (PROCESS, model 4) with 5000 iterations. We entered cross-cultural power distance belief as an independent variable (1 = Chinese participants, 0 = American participants), fear of authority as a mediator, and workplace communication with superiors as a dependent variable. The indirect effect was significant b = −0.17, SE = 0.03, 95% (−0.2416, −0.1135) ( Figure 4 ). Fear of authority mediated the effect of power distance in different cultures on workplace communication with superiors.

Fear of authority mediates the effect of power distance belief on communication with superiors in Study 4. Unstandardized coefficients displayed. *** p < 0.001.
6. Discussion
We conducted four studies and verified that high power distance belief negatively impacts workplace communication. We conducted two correlation studies, an experimental study, and a cross-cultural study, providing correlational and causal evidence. Specifically, our findings indicated that high power distance belief hinders workplace communication via less communication with superiors and no variation in communication with colleagues as well as subordinates. Meanwhile, we further uncovered that fear of authority mediated the negative effects of high power distance belief on inefficient communication with superiors.
6.1. Research Implications
People with high power distance belief are more inclined to view superiors as authorities. Both subordinates and superiors in high power distance societies are more likely to rationalize inequalities [ 7 , 68 ] of ability, merit, or resources. When people tend to believe that their superiors are better than themselves, they naturally regard them as authorities, which evokes a fear of authority in high power distance societies.
Individuals with higher levels of authority fear communicate with superiors ineffectively, keeping silence and expressing less defiance. Consistent with our findings, studies on emotions found that fear could induce compliance with unreasonable suggestions and elicit stress [ 69 ]. This indicates another perspective, that people may tend to be silent in the face of superiors out of self-protection. Previous studies indicated that defensive silence is a fear based form of self-protection, involving withholding relevant ideas, information, or opinions [ 70 ]. Adherence to appropriate social norms in communicating with authorities is a sign of social adaptability, which allows these subordinates to better survive in the workplace and avoid workplace bullying [ 71 ]. Fear of authority exacerbates silent communication.
It is important to buffer the negative effect of high power distance belief on communication in organizations and societies. Our findings indicated the negative impact of high power distance on workplace communication. Previous studies proposed that fear and power distance belief also changed the original rational intentions of team members [ 72 ]. Based on our findings, we proposed three suggestions to reduce the negative effects of high power distance belief on workplace communication. First, reducing the power distance between the superiors and subordinates could contribute to workplace communication. Specifically, the shortened physical distance could decrease the sense of power distance [ 73 ]. Similarly, students with high power distance belief improved communication when their teachers maintained a low power distance relationship with them [ 6 ]. Policies reducing actual interpersonal distance may improve the inefficient communication associated with high power distance belief. Second, superiors could reduce subordinates’ fear of authority to enhance effective communication. Specifically, more impartial behavior by supervisors could reduce subordinates’ stress and eliminate subordinates’ nervousness when confronted with their leaders [ 74 ]. A servant leadership style reduces subordinates’ fear of their direct supervisors [ 75 ]. In contrast, abusive supervision increases subordinates’ fear [ 76 ]. Supervisors’ behavior and leadership style contribute to better communication by eliminating subordinates’ fear. Third, subordinates could reduce the fear of authority actively. People with a critical thinking style are more likely to take a holistic view of issues and buffer the fear of authority [ 77 ]. In addition, social connectedness increases the sense of perceived social support and thus decreases fear and improves communication with others [ 78 ]. Our research reveals the relationships and mechanisms between power distance belief and workplace communication. Effective communication further enhances productivity [ 79 ] and prevents workplace accidents [ 80 , 81 ].
6.2. Limitations and Future Directions
First, in Studies 1, 2 and 4, we measured workplace communication through a self-developed scale. Although we addressed this limitation by testing the reliability and validity of the scale, we should be cautious about our findings regarding workplace communication. Future research should further explore diverse and valid measures of workplace communication.
Second, in current research, we found that fear of authority mediated the effect of power distance belief on workplace communication. As discussed, we proposed shortened power distance, supervisor’ behavior, and subordinates’ thinking style as potential factors that could be used to buffer the undesirable resultant effects. Future research could also test the inhibitory solutions and provide experimental evidence.
Third, in the current study, we recruited participants via an online platform. Although online recruitment platforms (i.e., Credamo; Turkprime) are reliable and have been widely used [ 54 , 55 ] and we also used questions to exclude participants who do not have workplace communication experience, nevertheless, longitudinal design and field study would be needed to complement our experimental and cross-sectional work. Future research could further conduct field studies (i.e., data collection in companies) and explore diverse measures (i.e., evaluate communication by other-reported) to further provide more cogent evidence.
7. Conclusions
In conclusion, we found that high power distance belief hinders workplace communication via less communication with superiors due to fear of authority. In addition to objective conditions and technology, subjective factors such as power distance belief are worthy of attention. Fear of authority is another important aspect for organization managers to consider when improving workplace communication.
Supplementary Materials
The following are available online at https://www.mdpi.com/article/10.3390/ijerph19052932/s1 .
Author Contributions
Y.D. and H.L. conceptualized the idea. Y.D. and H.L. designed the studies. Y.D. prepared the materials, conducted the studies, and collected the data. Y.D. and H.L. conducted data analysis. Y.D. and H.L. wrote the initial manuscript. Y.D., W.X., and T.D. revised the manuscript. H.L. supervised and provided funds for this project. All authors have read and agreed to the published version of the manuscript.
This research was funded by the Natural Science Foundation of Tibet, grant number XZ2018ZR G-04.
Institutional Review Board Statement
The study was conducted according to the guidelines of the Declaration of Helsinki and approved by the Ethics Committee of Peking University (20210609) on 21 June 2021.
Informed Consent Statement
Informed consent was obtained from all subjects involved in the study.
Data Availability Statement
Conflicts of interest.
The authors declare no conflict of interest.
Publisher’s Note: MDPI stays neutral with regard to jurisdictional claims in published maps and institutional affiliations.
Hofstede’s Cultural Dimensions Theory & Examples
Charlotte Nickerson
Research Assistant at Harvard University
Undergraduate at Harvard University
Charlotte Nickerson is a student at Harvard University obsessed with the intersection of mental health, productivity, and design.
Learn about our Editorial Process
Saul Mcleod, PhD
Editor-in-Chief for Simply Psychology
BSc (Hons) Psychology, MRes, PhD, University of Manchester
Saul Mcleod, PhD., is a qualified psychology teacher with over 18 years of experience in further and higher education. He has been published in peer-reviewed journals, including the Journal of Clinical Psychology.
On This Page:
- Hofstede’s Cultural Dimensions Theory, developed by Geert Hofstede, is a framework used to understand the differences in culture across countries.
- Hofstede’s initial six key dimensions include power distance, uncertainty avoidance, individualism-collectivism, masculinity-femininity, and short vs. long-term orientation. Later, researchers added restraint vs. indulgence to this list.
- The extent to which individual countries share key dimensions depends on a number of factors, such as shared language and geographical location.
- Hofstede’s cultural dimensions are widely used to understand etiquette and facilitate communication across cultures in areas ranging from business to diplomacy.
History and Overview
Hofstede’s cultural values or dimensions provide a framework through which sociologists can describe the effects of culture on the values of its members and how these values relate to the behavior of people who live within a culture.
Outside of sociology, Hofstede’s work is also applicable to fields such as cross-cultural psychology, international management, and cross-cultural communication.
The Dutch management researcher Geert Hofstede created the cultural dimensions theory in 1980 (Hofstede, 1980).
Hofstede’s cultural dimensions originate from a large survey that he conducted from the 1960s to 1970s that examined value differences among different divisions of IBM, a multinational computer manufacturing company.
This study encompassed over 100,000 employees from 50 countries across three regions. Hoftstede, using a specific statistical method called factor analysis, initially identified four value dimensions: individualism and collectivism, power distance, uncertainty avoidance, and masculinity and femininity.
Later research from Chinese sociologists identified a fifty-dimension, long-term, or short-term orientation (Bond, 1991).
Finally, a replication of Hofstede’s study, conducted across 93 separate countries, confirmed the existence of the five dimensions and identified a sixth known as indulgence and restraint (Hofstede & Minkov, 2010).
Cultural Dimensions
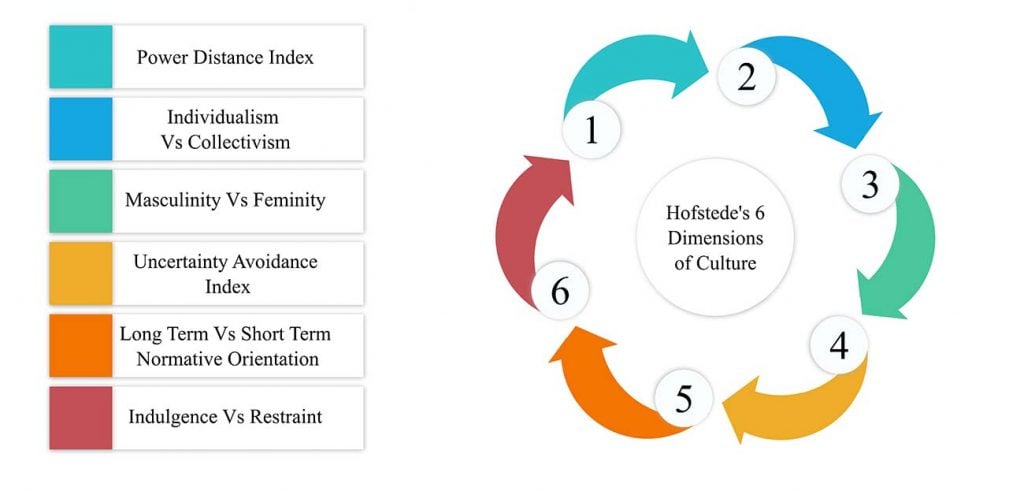
Geert Hofstede’s cultural dimensions theory (1980) examined people’s values in the workplace and created differentiation along three dimensions: small/large power distance, strong/weak uncertainty avoidance, masculinity/femininity, and individualism/collectivism.
Power-Distance Index
The power distance index describes the extent to which the less powerful members of an organization or institution — such as a family — accept and expect that power is distributed unequally.
Although there is a certain degree of inequality in all societies, Hofstede notes that there is relatively more equality in some societies than in others.
Individuals in societies that have a high degree of power distance accept hierarchies where everyone has a place in a ranking without the need for justification.
Meanwhile, societies with low power distance seek to have an equal distribution of power. The implication of this is that cultures endorse and expect relations that are more consultative, democratic, or egalitarian.
In countries with low power distance index values, there tends to be more equality between parents and children, with parents more likely to accept it if children argue or “talk back” to authority.
In low power distance index workplaces, employers and managers are more likely to ask employees for input; in fact, those at the lower ends of the hierarchy expect to be asked for their input (Hofstede, 1980).
Meanwhile, in countries with high power distance, parents may expect children to obey without questioning their authority. Those of higher status may also regularly experience obvious displays of subordination and respect from subordinates.
Superiors and subordinates are unlikely to see each other as equals in the workplace, and employees assume that higher-ups will make decisions without asking them for input.
These major differences in how institutions operate make status more important in high power distance countries than low power distance ones (Hofstede, 1980).
Collectivism vs. Individualism
Individualism and collectivism, respectively, refer to the integration of individuals into groups.
Individualistic societies stress achievement and individual rights, focusing on the needs of oneself and one’s immediate family.
A person’s self-image in this category is defined as “I.”
In contrast, collectivist societies place greater importance on the goals and well-being of the group, with a person’s self-image in this category being more similar to a “We.”
Those from collectivist cultures tend to emphasize relationships and loyalty more than those from individualistic cultures.
They tend to belong to fewer groups but are defined more by their membership in them. Lastly, communication tends to be more direct in individualistic societies but more indirect in collectivistic ones (Hofstede, 1980).
Uncertainty Avoidance Index
The uncertainty avoidance dimension of Hofstede’s cultural dimensions addresses a society’s tolerance for uncertainty and ambiguity.
This dimension reflects the extent to which members of a society attempt to cope with their anxiety by minimizing uncertainty. In its most simplified form, uncertainty avoidance refers to how threatening change is to a culture (Hofstede, 1980).
A high uncertainty avoidance index indicates a low tolerance for uncertainty, ambiguity, and risk-taking. Both the institutions and individuals within these societies seek to minimize the unknown through strict rules, regulations, and so forth.
People within these cultures also tend to be more emotional.
In contrast, those in low uncertainty avoidance cultures accept and feel comfortable in unstructured situations or changeable environments and try to have as few rules as possible. This means that people within these cultures tend to be more tolerant of change.
The unknown is more openly accepted, and less strict rules and regulations may ensue.
For example, a student may be more accepting of a teacher saying they do not know the answer to a question in a low uncertainty avoidance culture than in a high uncertainty avoidance one (Hofstede, 1980).
Femininity vs. Masculinity
Femininity vs. masculinity, also known as gender role differentiation, is yet another one of Hofstede’s six dimensions of national culture. This dimension looks at how much a society values traditional masculine and feminine roles.
A masculine society values assertiveness, courage, strength, and competition; a feminine society values cooperation, nurturing, and quality of life (Hofstede, 1980).
A high femininity score indicates that traditionally feminine gender roles are more important in that society; a low femininity score indicates that those roles are less important.
For example, a country with a high femininity score is likely to have better maternity leave policies and more affordable child care.
Meanwhile, a country with a low femininity score is likely to have more women in leadership positions and higher rates of female entrepreneurship (Hofstede, 1980).
Short-Term vs. Long-Term Orientation
The long-term and short-term orientation dimension refers to the degree to which cultures encourage delaying gratification or the material, social, and emotional needs of their members (Hofstede, 1980).
Societies with long-term orientations tend to focus on the future in a way that delays short-term success in favor of success in the long term.
These societies emphasize traits such as persistence, perseverance, thrift, saving, long-term growth, and the capacity for adaptation.
Short-term orientation in a society, in contrast, indicates a focus on the near future, involves delivering short-term success or gratification, and places a stronger emphasis on the present than the future.
The end result of this is an emphasis on quick results and respect for tradition. The values of a short-term society are related to the past and the present and can result in unrestrained spending, often in response to social or ecological pressure (Hofstede, 1980).
Restraint vs. Indulgence
Finally, the restraint and indulgence dimension considers the extent and tendency of a society to fulfill its desires.
That is to say, this dimension is a measure of societal impulse and desire control. High levels of indulgence indicate that society allows relatively free gratification and high levels of bon de vivre.
Meanwhile, restraint indicates that society tends to suppress the gratification of needs and regulate them through social norms.
For example, in a highly indulgent society, people may tend to spend more money on luxuries and enjoy more freedom when it comes to leisure time activities. In a restrained society, people are more likely to save money and focus on practical needs (Hofstede, 2011).
Correlations With Other Country’s Differences
Hofstede’s dimensions have been found to correlate with a variety of other country difference variables, including:
- geographical proximity,
- shared language,
- related historical background,
- similar religious beliefs and practices,
- common philosophical influences,
- and identical political systems (Hofstede, 2011).
For example, countries that share a border tend to have more similarities in culture than those that are further apart.
This is because people who live close to each other are more likely to interact with each other on a regular basis, which leads to a greater understanding and appreciation of each other’s cultures.
Similarly, countries that share a common language tend to have more similarities in culture than those that do not.
Those who speak the same language can communicate more easily with each other, which leads to a greater understanding and appreciation of each other’s cultures (Hofstede, 2011).
Finally, countries that have similar historical backgrounds tend to have more similarities in culture than those that do not.
People who share a common history are more likely to have similar values and beliefs, which leads, it has generally been theorized, to a greater understanding and appreciation of each other’s cultures.
Applications
Cultural difference awareness.
Geert Hofstede shed light on how cultural differences are still significant today in a world that is becoming more and more diverse.
Hofstede’s cultural dimensions can be used to help explain why certain behaviors are more or less common in different cultures.
For example, individualism vs. collectivism can help explain why some cultures place more emphasis on personal achievement than others. Masculinity vs. feminism could help explain why some cultures are more competitive than others.
And long-term vs. short-term orientation can help explain why some cultures focus more on the future than the present (Hofstede, 2011).
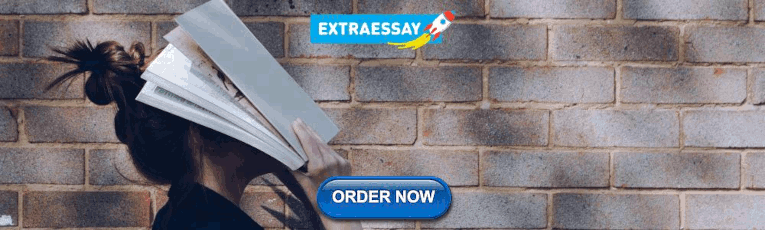
International communication and negotiation
Hofstede’s cultural dimensions can also be used to predict how people from different cultures will interact with each other.
For example, if two people from cultures with high levels of power distance meet, they may have difficulty communicating because they have different expectations about who should be in charge (Hofstede, 2011).
In Business
Finally, Hofstede’s cultural dimensions can be used to help businesses adapt their products and marketing to different cultures.
For example, if a company wants to sell its products in a country with a high collectivism score, it may need to design its packaging and advertising to appeal to groups rather than individuals.
Within a business, Hofstede’s framework can also help managers to understand why their employees behave the way they do.
For example, if a manager is having difficulty getting her employees to work together as a team, she may need to take into account that her employees come from cultures with different levels of collectivism (Hofstede, 2011).
Although the cultural value dimensions identified by Hofstede and others are useful ways to think about culture and study cultural psychology, the theory has been chronically questioned and critiqued.
Most of this criticism has been directed at the methodology of Hofstede’s original study.
Orr and Hauser (2008) note Hofstede’s questionnaire was not originally designed to measure culture but workplace satisfaction. Indeed, many of the conclusions are based on a small number of responses.
Although Hofstede administered 117,000 questionnaires, he used the results from 40 countries, only six of which had more than 1000 respondents.
This has led critics to question the representativeness of the original sample.
Furthermore, Hofstede conducted this study using the employees of a multinational corporation, who — especially when the study was conducted in the 1960s and 1970s — were overwhelmingly highly educated, mostly male, and performed so-called “white collar” work (McSweeney, 2002).
Hofstede’s theory has also been criticized for promoting a static view of culture that does not respond to the influences or changes of other cultures.
For example, as Hamden-Turner and Trompenaars (1997) have envisioned, the cultural influence of Western powers such as the United States has likely influenced a tide of individualism in the notoriously collectivist Japanese culture.
Nonetheless, Hofstede’s theory still has a few enduring strengths. As McSweeney (2002) notes, Hofstede’s work has “stimulated a great deal of cross-cultural research and provided a useful framework for the comparative study of cultures” (p. 83).
Additionally, as Orr and Hauser (2008) point out, Hofstede’s dimensions have been found to be correlated with actual behavior in cross-cultural studies, suggesting that it does hold some validity.
All in all, as McSweeney (2002) points out, Hofstede’s theory is a useful starting point for cultural analysis, but there have been many additional and more methodologically rigorous advances made in the last several decades.
Bond, M. H. (1991). Beyond the Chinese face: Insights from psychology . Oxford University Press, USA.
Hampden-Turner, C., & Trompenaars, F. (1997). Response to geert hofstede. International Journal of Intercultural Relations, 21 (1), 149.
Hofstede, G. (1980). Culture and organizations. International studies of management & organization, 10 (4), 15-41.
Hofstede, G. (2011). Dimensionalizing cultures: The Hofstede model in context. Online readings in psychology and culture, 2 (1), 2307-0919.
Hofstede, G., & Minkov, M. (2010). Long-versus short-term orientation: new perspectives. Asia Pacific Business Review, 16(4), 493-504.
Hofstede, G. (1980). Culture’s Consequences (Vol. Sage): Beverly Hills, CA.
Hofstede, G. (1991). Cultures and Organizations: Software of the mind . London, England: McGraw-Hill.
McSweeney, B. (2002). The essentials of scholarship: A reply to Geert Hofstede. Human Relations, 55( 11), 1363-1372.
Orr, L. M., & Hauser, W. J. (2008). A re-inquiry of Hofstede’s cultural dimensions: A call for 21st century cross-cultural research. Marketing Management Journal, 18 (2), 1-19.
Further Information
- Markus, H. R., & Kitayama, S. (1991). Culture and the self: Implications for cognition, emotion, and motivation. Psychological review, 98(2), 224.
- Triandis, H. C. (1989). The self and social behavior in differing cultural contexts. Psychological review, 96(3), 506.
- Oyserman, D., Coon, H. M., & Kemmelmeier, M. (2002). Rethinking individualism and collectivism: evaluation of theoretical assumptions and meta-analyses. Psychological bulletin, 128(1), 3.
- Brewer, M. B., & Chen, Y. R. (2007). Where (who) are collectives in collectivism? Toward conceptual clarification of individualism and collectivism. Psychological review, 114(1), 133.
- Grossmann, I., & Santos, H. (2017). Individualistic culture.

Related Articles
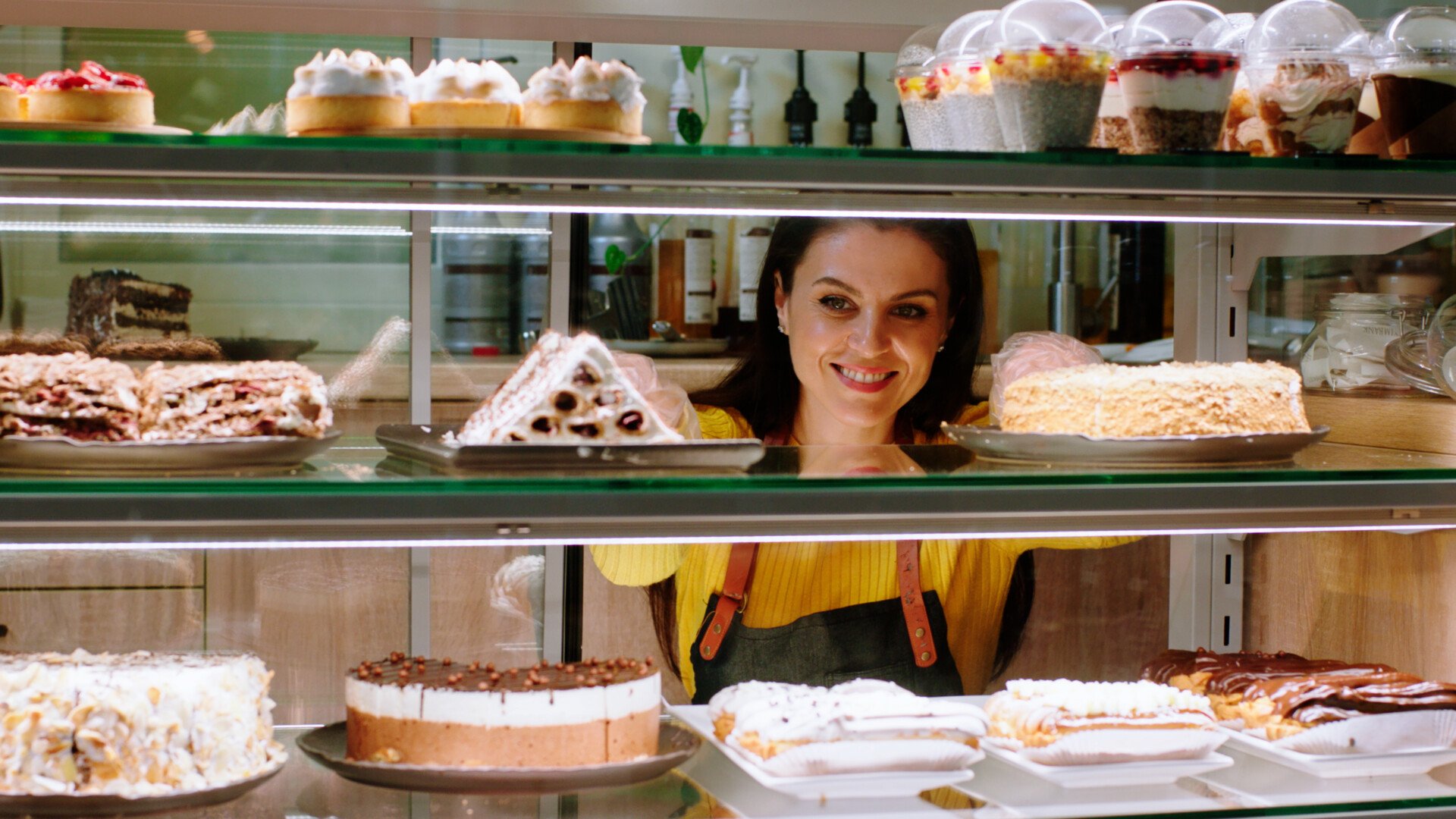
Social Science
Hard Determinism: Philosophy & Examples (Does Free Will Exist?)
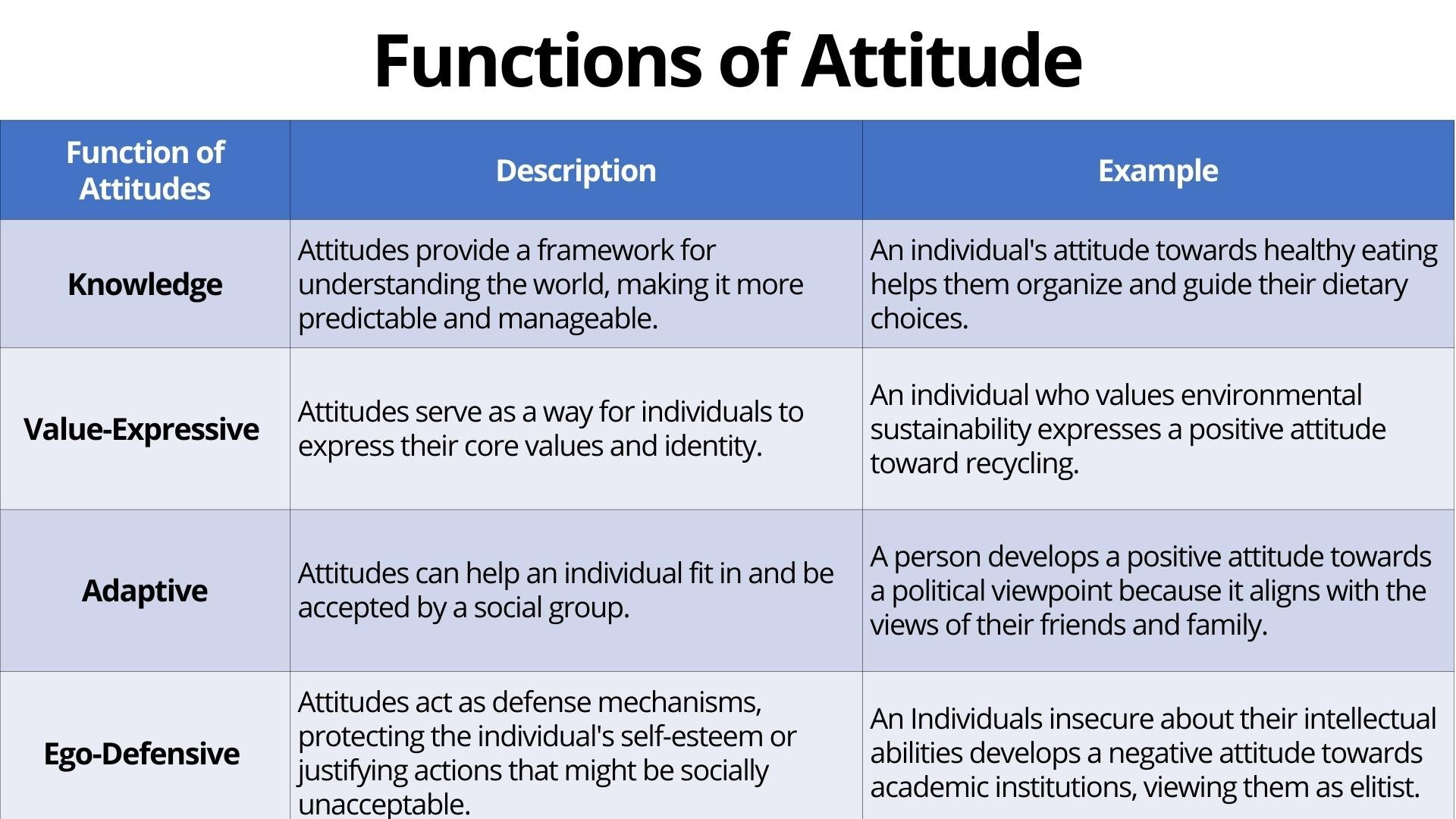
Functions of Attitude Theory
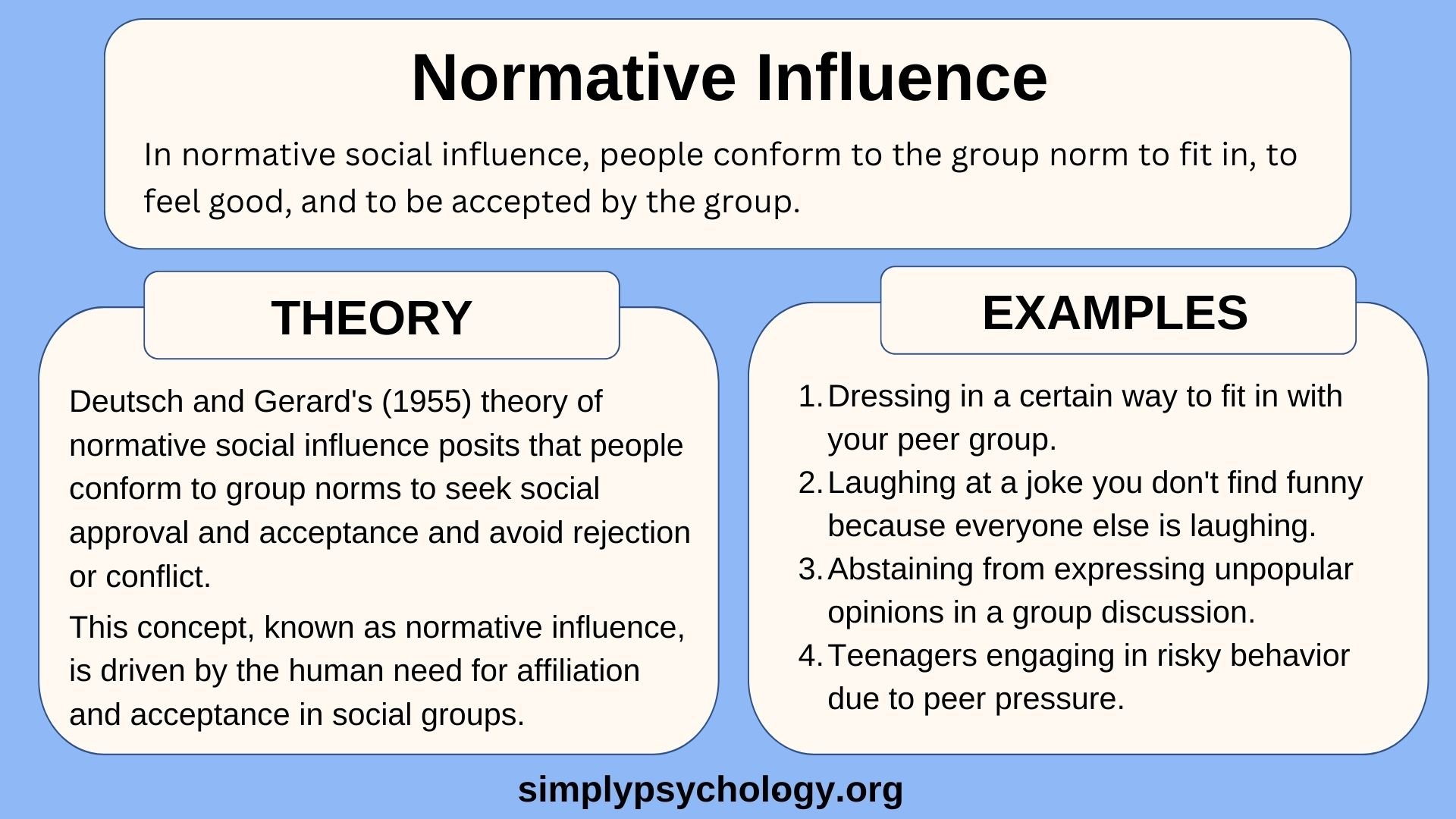
Understanding Conformity: Normative vs. Informational Social Influence
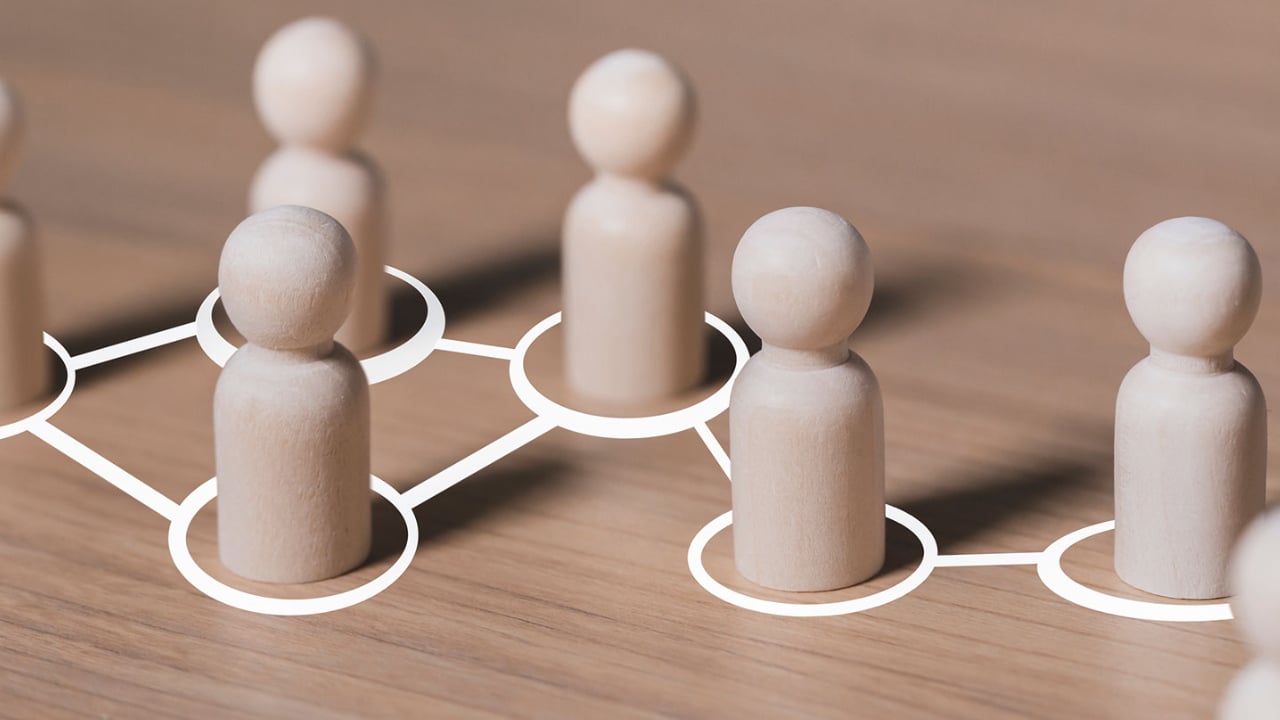
Social Control Theory of Crime
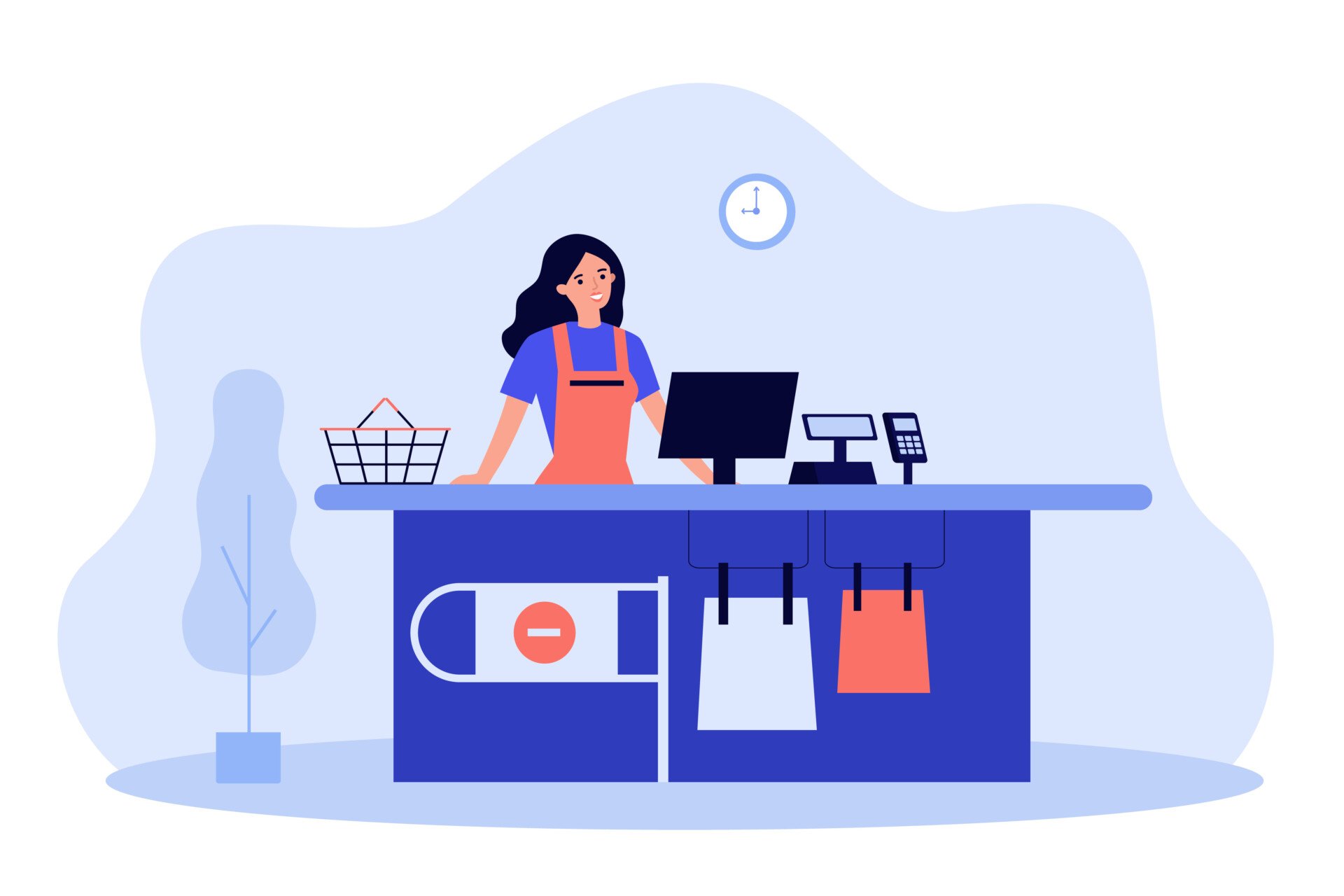
Emotions , Mood , Social Science
Emotional Labor: Definition, Examples, Types, and Consequences
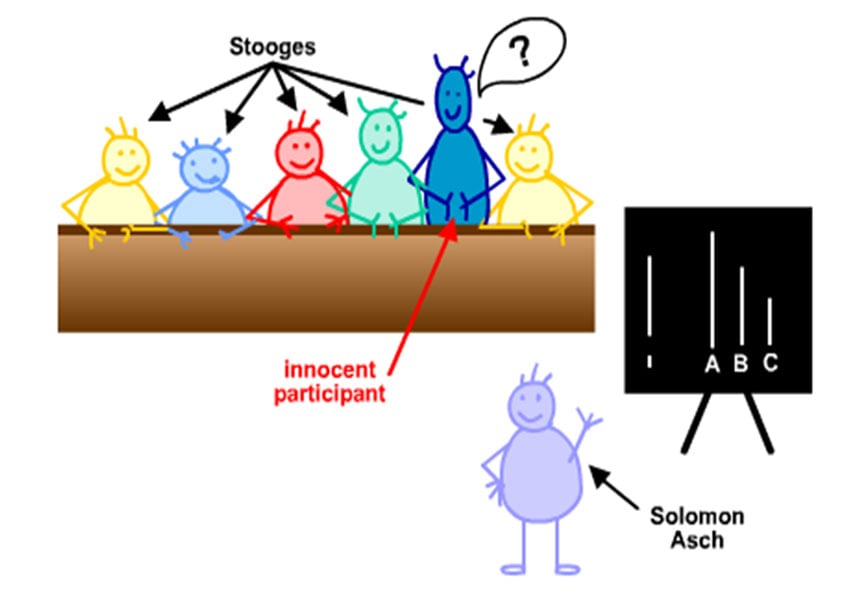
Famous Experiments , Social Science
Solomon Asch Conformity Line Experiment Study
Sustainable Global Human Resource: The Impact of Power Distance on Work–Family Balance
- Conference paper
- First Online: 20 July 2018
- Cite this conference paper
- Hooi Ching Khor 6 &
- Azura Abdullah Effendi 7
460 Accesses
The aim of this conceptual paper is to draw the attention of researchers, employers, and human resource policy makers to one of Hofstede’s cultural dimensions, power distance, from a communication perspective. Power distance is believed to facilitate the ability of employees in balancing their work and family life. It is possible to view this factor as either a barrier or a catalyst for the accessibility and utilization of an organization’s human resource family-supportive policies to achieve the desired and satisfied work and family life integration. The level of power distance may either facilitates ones’ resource gain to experience positive work–family spillover or resource depletion which leads to negative work–family spillover. Power distance is proposed to have a buffering effect on the relationship between family-supportive policy and work–family balance (WFB) to unveil the possible reasons behind inconsistent findings in prior research. Besides this, the level of power distance is also proposed to have a predictive effect on WFB. The theoretical and practical importance and implications of power distance are addressed.
This is a preview of subscription content, log in via an institution to check access.
Access this chapter
- Available as PDF
- Read on any device
- Instant download
- Own it forever
- Available as EPUB and PDF
- Compact, lightweight edition
- Dispatched in 3 to 5 business days
- Free shipping worldwide - see info
- Durable hardcover edition
Tax calculation will be finalised at checkout
Purchases are for personal use only
Institutional subscriptions
Allen TD, Johnson RC, Shockley KM, Kiburz KM (2013) Work-family conflict and flexible work arrangements: deconstructing flexibility. Pers Psychol 66(2):345–376
Article Google Scholar
Aryee S, Fields D, Luk V (1999) A cross-cultural test of a model of the work-family interface. J Manag 25(4):491–511
Google Scholar
Baron RM, Kenny DA (1986) The moderator-mediator variable distinction in social psychological research: conceptual, strategic, and statistical considerations. J Pers Soc Psychol 51(6):1173–1182
Botero IC, Van Dyne L (2009) Employee voice behavior interactive effects of LMX and power distance in the United States and Colombia. Manag Commun Q 23(1):84–104
Brockner J, Ackerman G, Greenberg J, Gelfand MJ, Francesco AM, Chen ZX, Leung K, Bierbrauer G, Gomez C, Kirkman BL, Shapiro D (2001) Culture and procedural justice: the influence of power distance on reactions to voice. J Exp Soc Psychol 37(4):300–315
Chen IH, Brown R, Bowers BJ, Chang WY (2015) Work-to-family conflict as a mediator of the relationship between job satisfaction and turnover intention. J Adv Nurs 71(10):2350–2363
Chudnovskaya EV, O’Hara LS (2016) Experiences of Danish business expatriates in Russia: power distance in organizational communication. J Intercult Commun Res 45(4):261–281
Fischlmayr IC, Kollinger I (2010) Work-life balance—a neglected issue among Austrian female expatriates. Int J Human Resour Manag 21(4):455–487
Greenhaus JH, Beutell NJ (1985) Sources of conflict between work and family roles. Acad Manag Rev 10(1):76–88
Hassan Z, Dollard MF, Winefield AH (2010) WFC in East versus Western countries. Cross Cult Manag Int J 17(1):30–49
Hill EJ, Yang C, Hawkins AJ, Ferris M (2004) A cross-cultural test of the work-family interface in 48 countries. J Marriage Fam 66(5):1300–1316
Hodgetts RM, Luthans F, Doh JP (2006) International management: culture, strategy, and behavior, 6th edn. McGraw-Hill Companies
Hofstede G (1983) The cultural relativity of organizational practices and theories. J Int Bus Stud 14(2):75–89
Ji Y, Zhou E, Li C, Yan Y (2015) Power distance orientation and employee help seeking: trust in supervisor as a mediator. Soc Behav Pers Int J 43(6):1043–1054
Kinman G, Jones F (2008) A life beyond work? Job demands, work-life balance, and wellbeing in UK academics. J Human Behav Soc Environ 17(1):41–60
Kreiner GE, Hollensbe EC, Sheep ML (2009) Balancing borders and bridges: negotiating the work-home interface via boundary work tactics. Acad Manag J 52(4):704–730
Luk DM, Shaffer MA (2005) Work and family domain stressors and support: within- and cross-domain influences on WFC. J Occup Organ Psychol 78(4):489–508
McNamara TK, Pitt-Catsouphes M, Matz-Costa C, Brown M, Valcour M (2013) Across the continuum of satisfaction with work-family balance: work hours, flexibility-fit, and work-family culture. Soc Sci Res 42(2):283–298
Netemeyer RG, Boles JS, McMurrian R (1996) Development and validation of work-family conflict and family-work conflict scales. J Appl Psychol 81(4):400–410
O’Brien KM, Ganginis Del Pino HV, Yoo SK, Cinamon RG, Han YJ (2014) Work, family, support, and depression: employed mothers in Israel, Korea, and the United States. J Couns Psychol 61(3):461–472
O’Driscoll MP, Poelmans S, Spector PE, Kalliath T, Allen TD, Cooper CL, Sanchez JI (2003) Family-responsive interventions, perceived organizational and supervisor support, work-family conflict, and psychological strain. Int J Stress Manag 10(4):326–344
Odle-Dusseau HN, Britt TW, Bobko P (2012) Work-family balance, well-being, and organizational outcomes: investigating actual versus desired work/family time discrepancies. J Bus Psychol 27(3):331–343
Parakandi M, Behery M (2016) Sustainable human resources: examining the status of organizational work-life balance practices in the United Arab Emirates. Renew Sustain Energy Rev 55(1):1370–1379
Pinto LH, Cabral-Cardoso C, Werther WB (2012) Adjustment elusiveness: an empirical investigation of the effects of cross-cultural adjustment on general assignment satisfaction and withdrawal intentions. Int J Intercult Relat 36(2):188–199
Raghuram S, London M, Larsen HH (2001) Flexible employment practices in Europe: country versus culture. Int J Human Resour Manag 12(5):738–753
Rothbard NP, Phillips KW, Dumas TL (2005) Managing multiple roles: work-family policies and individuals’ desires for segmentation. Organ Sci 16(3):243–258
Schütter H, Boerner S (2013) Illuminating the work-family interface on international assignments: an exploratory approach. J Glob Mobility Home Expatriate Manag Res 1(1):46–71
Shaffer MA, Harrison DA, Gilley KM, Luk DM (2001) Struggling for balance amid turbulence on international assignments: work-family conflict, support and commitment. J Manag 27(1):99–121
Shockley KM, Allen TD (2007) When flexibility helps: another look at the availability of flexible work arrangements and work-family conflict. J Vocat Behav 71(3):479–493
Shockley KM, Allen TD (2010) Investigating the missing link in flexible work arrangement utilization: an individual difference perspective. J Vocat Behav 76(1):131–142
Tausig M, Fenwick R (2001) Unbinding time: alternate work schedules and work-life balance. J Fam Econ Issues 22(2):101–119
Thompson CA, Beauvais LL, Lyness KS (1999) When work-family benefits are not enough: the influence of work-family culture on benefit utilization, organizational attachment, and work-family conflict. J Vocat Behav 54(3):392–415
Voydanoff P (2005) Consequences of boundary-spanning demands and resources for work-to-family conflict and perceived stress. J Occup Health Psychol 10(4):491–503
Watanabe M, Falci CD (2016) A demands and resources approach to understanding faculty turnover intentions due to work-family balance. J Fam Issues 37(3):393–415
Download references
Author information
Authors and affiliations.
Faculty of Business and Finance, Universiti Tunku Abdul Rahman, Perak, Malaysia
Hooi Ching Khor
School of Management, Universiti Sains Malaysia, Penang, Malaysia
Azura Abdullah Effendi
You can also search for this author in PubMed Google Scholar
Corresponding author
Correspondence to Hooi Ching Khor .
Editor information
Editors and affiliations.
Universiti Teknologi MARA, Bandar Tun Abdul Razak Jengka, Pahang, Malaysia
Mohd Yusri Mohamad Noor
Badli Esham Ahmad
Mohd Rozaidi Ismail
Universiti Teknologi MARA, Raub, Pahang, Malaysia
Hasnizawati Hashim
Mohd Amli Abdullah Baharum
Rights and permissions
Reprints and permissions
Copyright information
© 2019 Springer Nature Singapore Pte Ltd.
About this paper
Cite this paper.
Khor, H.C., Abdullah Effendi, A. (2019). Sustainable Global Human Resource: The Impact of Power Distance on Work–Family Balance. In: Mohamad Noor, M., Ahmad, B., Ismail, M., Hashim, H., Abdullah Baharum, M. (eds) Proceedings of the Regional Conference on Science, Technology and Social Sciences (RCSTSS 2016) . Springer, Singapore. https://doi.org/10.1007/978-981-13-0203-9_33
Download citation
DOI : https://doi.org/10.1007/978-981-13-0203-9_33
Published : 20 July 2018
Publisher Name : Springer, Singapore
Print ISBN : 978-981-13-0202-2
Online ISBN : 978-981-13-0203-9
eBook Packages : Social Sciences Social Sciences (R0)
Share this paper
Anyone you share the following link with will be able to read this content:
Sorry, a shareable link is not currently available for this article.
Provided by the Springer Nature SharedIt content-sharing initiative
- Publish with us
Policies and ethics
- Find a journal
- Track your research
- Open access
- Published: 12 May 2024
Distance plus attention for binding affinity prediction
- Julia Rahman 1 na1 ,
- M. A. Hakim Newton 2 , 3 na1 ,
- Mohammed Eunus Ali 4 &
- Abdul Sattar 2
Journal of Cheminformatics volume 16 , Article number: 52 ( 2024 ) Cite this article
117 Accesses
Metrics details
Protein-ligand binding affinity plays a pivotal role in drug development, particularly in identifying potential ligands for target disease-related proteins. Accurate affinity predictions can significantly reduce both the time and cost involved in drug development. However, highly precise affinity prediction remains a research challenge. A key to improve affinity prediction is to capture interactions between proteins and ligands effectively. Existing deep-learning-based computational approaches use 3D grids, 4D tensors, molecular graphs, or proximity-based adjacency matrices, which are either resource-intensive or do not directly represent potential interactions. In this paper, we propose atomic-level distance features and attention mechanisms to capture better specific protein-ligand interactions based on donor-acceptor relations, hydrophobicity, and \(\pi \) -stacking atoms. We argue that distances encompass both short-range direct and long-range indirect interaction effects while attention mechanisms capture levels of interaction effects. On the very well-known CASF-2016 dataset, our proposed method, named Distance plus Attention for Affinity Prediction (DAAP), significantly outperforms existing methods by achieving Correlation Coefficient (R) 0.909, Root Mean Squared Error (RMSE) 0.987, Mean Absolute Error (MAE) 0.745, Standard Deviation (SD) 0.988, and Concordance Index (CI) 0.876. The proposed method also shows substantial improvement, around 2% to 37%, on five other benchmark datasets. The program and data are publicly available on the website https://gitlab.com/mahnewton/daap.
Scientific Contribution Statement
This study innovatively introduces distance-based features to predict protein-ligand binding affinity, capitalizing on unique molecular interactions. Furthermore, the incorporation of protein sequence features of specific residues enhances the model’s proficiency in capturing intricate binding patterns. The predictive capabilities are further strengthened through the use of a deep learning architecture with attention mechanisms, and an ensemble approach, averaging the outputs of five models, is implemented to ensure robust and reliable predictions.
Introduction
Conventional drug discovery, as noted by a recent study [ 1 ], is a resource-intensive and time-consuming process that typically lasts for about 10 to 15 years and costs approximately 2.558 billion USD to bring each new drug successfully to the market. Computational approaches can expedite the drug discovery process by identifying drug molecules or ligands that have high binding affinities towards disease-related proteins and would thus form strong transient bonds to inhibit protein functions [ 2 , 3 , 4 ]. In a typical drug development pipeline, a pool of potential ligands is usually given, and the ligands exhibiting strong binding affinities are identified as the most promising drug candidates against a target protein. In essence, protein-ligand binding affinity values serve as a scoring method to narrow the search space for virtual screening [ 5 ].
Existing computational methods for protein-ligand binding affinity prediction include both traditional machine learning and deep learning-based approaches. Early methods used Kernel Partial Least Squares [ 6 ], Support Vector Regression (SVR) [ 7 ], Random Forest (RF) Regression [ 8 ], and Gradient Boosting [ 9 ]. However, just like various other domains [ 10 , 11 , 12 , 13 , 14 ], drug discovery has also seen significant recent advancements [ 15 , 16 , 17 , 18 ] from the computational power and extensive datasets used in deep learning. Deep learning models for protein-ligand binding affinity prediction take protein-ligand docked complexes as input and give binding affinity values as output. Moreover, these models use various input features to capture the global characteristics of the proteins and the ligands and their local interactions in the pocket areas where the ligands get docked into the proteins.
Recent deep learning models for protein-ligand binding affinity prediction include DeepDTA [ 19 ], Pafnucy [ 20 ], \(K_\text {DEEP}\) [ 21 ], DeepAtom [ 22 ], DeepDTAF [ 23 ], BAPA [ 5 ], SFCNN [ 24 ], DLSSAffinity [ 4 ] EGNA [ 25 ], CAPLA [ 26 ] and ResBiGAAT [ 27 ]. DeepDTA [ 19 ] introduced a Convolutional Neural Network (CNN) model with input features Simplified Molecular Input Line Entry System (SMILES) sequences for ligands and full-length protein sequences. Pafnucy and \(K_{DEEP}\) used a 3D-CNN with 4D tensor representations of the protein-ligand complexes as input features. DeepAtom employed a 3D-CNN to automatically extract binding-related atomic interaction patterns from voxelized complex structures. DeepDTAF combined global contextual features and local binding area-related features with dilated convolution to capture multiscale long-range interactions. BAPA introduced a deep neural network model for affinity prediction, featuring descriptor embeddings and an attention mechanism to capture local structural details. SFCNN employed a 3D-CNN with simplified 4D tensor features having only basic atomic type information. DLSSAffinity employed 1D-CNN with pocket-ligand structural pairs as local features and ligand SMILES and protein sequences as global features. EGNA introduced an empirical graph neural network (GNN) that utilizes graphs to represent proteins, ligands, and their interactions in the pocket areas. CAPLA [ 26 ] utilized a cross-attention mechanism within a CNN along with sequence-level input features for proteins and ligands and structural features for secondary structural elements. ResBiGAAT [ 27 ] integrates a deep Residual Bidirectional Gated Recurrent Unit (Bi-GRU) with two-sided self-attention mechanisms, utilizing both protein and ligand sequence-level features along with their physicochemical properties for efficient prediction of protein-ligand binding affinity.
In this work, we consider the effective capturing of protein-ligand interaction as a key to making further progress in binding affinity prediction. However, as we see from the literature, a sequential feature-based model such as DeepDTA was designed mainly to capture long-range interactions between proteins and ligands, not considering local interactions. CAPLA incorporates cross-attention mechanisms along with sequence-based features to indirectly encompass short-range interactions to some extent. ResBiGAAT employs a residual Bi-GRU architecture and two-sided self-attention mechanisms to capture long-term dependencies between protein and ligand molecules, utilizing SMILES representations, protein sequences, and diverse physicochemical properties for improved binding affinity prediction. On the other hand, structural feature-based models such as Pafnucy, \(K_{DEEP}\) and SFCNN use 3D grids, 4D tensors, or molecular graph representations. These features provide valuable insights into the pocket region of the protein-ligand complexes but incur significant computational costs in terms of memory and processing time. Additionally, these features have limitations in capturing long-range indirect interactions among protein-ligand pairs. DLSSAffinity aims to bridge the gap between short- and long-range interactions by considering both sequential and structural features. Moreover, DLSSAffinity uses 4D tensors for Cartesian coordinates and atom-level features to represent interactions between heavy atoms in the pocket areas of the protein-ligand complexes. These representations of interactions are still indirect, considering the importance of protein-ligand interaction in binding affinity. EGNA tried to use graphs and Boolean-valued adjacency matrices to capture protein-ligand interactions to some extent. However, EGNA’s interaction graph considers only edges between each pair of a \(C_\beta \) atom in the pocket areas of the protein and a heavy atom in the ligand when their distance is below a threshold of \(10\mathring{A}\) .
Inspired by the use of distance measures in protein structure prediction [ 14 , 28 , 29 ], in this work, we employ distance-based input features in protein-ligand binding affinity prediction. To be more specific, we use distances between donor-acceptor [ 30 ], hydrophobic [ 31 , 32 ], and \(\pi \) -stacking [ 31 , 32 ] atoms as interactions between such atoms play crucial roles in protein-ligand binding. These distance measures between various types of atoms could essentially capture more direct and more precise information about protein-ligand interactions than using sequence-based features or various other features representing the pocket areas of the protein-ligand complexes. Moreover, the distance values could more directly capture both short- and long-range interactions than adjacency-based interaction graphs of EGNA or tensor-based pocket area representations of DLSSAffinity. Besides capturing protein-ligand interactions, we also consider only those protein residues with donor, hydrophobic, and \(\pi \) -stacking atoms in this work. Considering only these selective residues is also in contrast with all other methods that use all the protein residues. For ligand representation, we use SMILES strings. After concatenating all input features, we use an attention mechanism to effectively weigh the significance of various input features. Lastly, we enhance the predictive performance of our model by adopting an ensembling approach, averaging the outputs of several trained models.
We name our proposed method as Distance plus Attention for Affinity Prediction (DAAP). On the very well-known CASF-2016 dataset, DAAP significantly outperforms existing methods by achieving the Correlation Coefficient (R) 0.909, Root Mean Squared Error (RMSE) 0.987, Mean Absolute Error (MAE) 0.745, Standard Deviation (SD) 0.988, and Concordance Index (CI) 0.876. DAAP also shows substantial improvement, ranging from 2% to 37%, on five other benchmark datasets. The program and data are publicly available on the website https://gitlab.com/mahnewton/daap .
In our study, we first demonstrate the robustness of our deep architecture through five-fold cross-validation. Subsequently, the learning curve, as depicted in Fig. 1 , illustrates the dynamics of training and validation loss, providing insights into the stability and reliability of the learning process. Furthermore, we provide a comprehensive performance comparison of our proposed model with current state-of-the-art predictors. We also provide an in-depth analysis of the experimental results. The effectiveness of our proposed features is substantiated through an ablation study and a detailed analysis of input features.
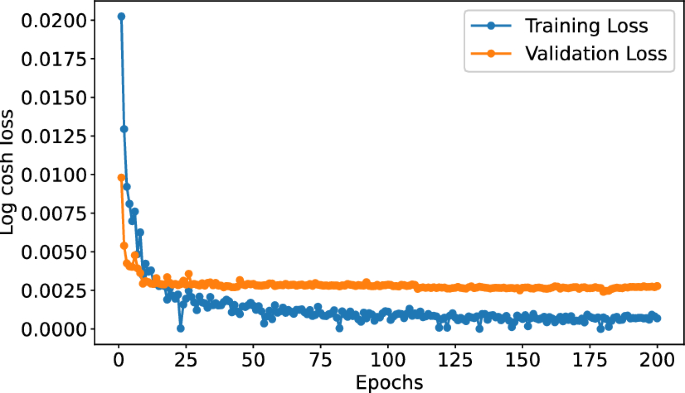
Training and validation loss curve of DAAP
Five-fold cross-validation
This study employs a five-fold cross-validation approach to evaluate the performance of the proposed model thoroughly, demonstrating the robustness of the deep architecture. Table 1 provides the average performance metrics (R, RMSE, MAE, SD, and CI) along with their corresponding standard deviations derived from the 5-fold cross-validation on the CASF \(-\) 2016.290 test set when the model is trained with PDBbind2016 and PDBbind2020 datasets. This presentation highlights the predictor’s predictive accuracy and reliability, emphasising the proposed model’s effectiveness.
Average ensemble
Our proposed approach leverages an attention-based deep learning architecture to predict binding affinity. The input feature set comprises distance matrices, sequence-based features for specific protein residues, and SMILES sequences. To enhance the robustness and mitigate the effects of variability and overfitting, we train five models and employ arithmetic averaging for ensembling. Average ensembling is more suitable than max voting ensembling when dealing with real values.
Table 2 shows the results of five models and their averages when all models have the identical setting of their training parameters and the training datasets. We see that the ensemble results are better than the results of the individual models in both the PDBbind2016 and PDBbind2020 training datasets. To check that the proposed approach is robust over the variability in the training datasets, we also train five models but each with a different training subset. These training subsets were obtained by using sampling with replacement. Table 3 shows the results of these five models and their averages.
Tables 2 and 3 depict that the ensemble results are better than the results of the individual results in both training sets. It might seem counterintuitive to see the average results are better than all the individual results, but note that these are not simple average of averages. When the ensemble results are compared across Tables 2 and 3 , the best results are observed in Table 2 for the PDBbind2020 training set. All evaluation metrics R, RMSE, SD, MAE, and CI display improved performance when using the same training data (Table 2 ) compared to different varying training data (Table 3 ) in PDBbind2020 data set. Accordingly, we choose the ensemble with the same training data for PDBbind2020 (Table 2 ) as our final binding affinity prediction model. Conversely, for PDBbind2016, superior outcomes are obtained from the varied training subsets in Table 3 . Henceforth, the best-performing models using PDBbind2016 and PDBbind2020 will be referred to as DAAP16 and DAAP20, respectively, in subsequent discussions.
Comparison with state-of-the-art methods
In our comparative analysis, we assess the performance of our proposed affinity predictor, DAAP, on the CASF-2016 test set, compared to nine recent state-of-the-art predictors: Pafnucy [ 20 ], DeepDTA [ 19 ], OnionNet [ 3 ], DeepDTAF [ 23 ], SFCNN [ 24 ] DLSSAffinity [ 4 ], EGNA [ 25 ], CAPLA [ 26 ] and ResBiGAAT [ 27 ]. Notably, the most recent predictors have surpassed the performance of the initial four, prompting us to focus our comparison on their reported results. For the latter five predictors, we detail the methodology of obtaining their results as follows:
DLSSAffinity We rely on the results available on DLSSAffinity’s GitHub repository, as direct prediction for specific target proteins is not possible due to the unavailability of its trained model.
SFCNN Utilizing the provided weights and prediction code from SFCNN, we replicate their results, except for CASF-2013. The ambiguity regarding the inclusion of CASF-2013 data in their training set (sourced from the PDBbind database version 2019) leads us to omit these from our comparison.
EGNA We have adopted EGNA’s published results for the CASF-2016 test set with 285 protein-ligand complexes due to differing Uniclust30 database versions for HHM feature construction. We applied EGNA’s code with our HHM features for the other five test sets to ensure a consistent evaluation framework.
CAPLA Predictions are made based on the features given in CAPLA’s GitHub, except for the ADS.74 dataset, where we can’t predict results due to the unavailability of feature sets. Their results are the same as their reported results.
ResBiGAAT We included ResBiGAAT’s published results in our analysis after encountering discrepancies with their online server using the same SMILES sequences and protein sequences from test PDB files as us. Variations in results, particularly for PDB files with multiple chains, led us to rely on their reported data, as it yielded more consistent and higher accuracies than our attempts.
In Table 4 , the first 8 methods, namely Pafnucy, DeepDTA, OnionNet, DeepDTAF, DLSSAffinity, SFCNN, \(EGNA^*\) and CAPLA reported on 290 CASF-2016 protein-ligand complexes. To make a fair comparison with these 8 methods, we compared our proposed method DAAP16 and DAAP20 on those 290 protein-ligand complexes. From the data presented in the Table 4 , it is clear that our DAAP20 approach outperforms all the 8 predictors, achieving the highest R-value of 0.909, the highest CI value of 0.876, the lowest RMSE of 0.987, the lowest MAE of 0.745, and the lowest SD of 0.988. Specifically, compared to the closest state-of-the-art predictor, CAPLA, our approach demonstrated significant improvements, with approximately 5% improvement in R, 12% in RMSE, 14% in MAE, 11% in SD, and 4% in CI metrics, showcasing its superior predictive capabilities. As 3 of the recent predictors, namely SFCNN, EGNA, and ResBiGAAT, reported their result for the 285 protein-ligand complexes on the CASF-2016 dataset, to make a fair comparison with them as well, we assess our predictor, DAAP, on these 285 proteins as well. From the data presented in Table 4 , the results revealed that, across all metrics, DAAP20 outperformed these three predictors on 285 proteins as well. Particularly, compared to the recent predictor ResBiGAAT, our approach demonstrated notable improvements, with around 6% improvement in R, 19% in RMSE, 20% in MAE, and 5% in CI metrics, highlighting its superior predictive capabilities.
Table 5 presents a comprehensive evaluation of the prediction performance of our proposed DAAP approach on five other well-known test sets CASF \(-\) 2013.87, CASF \(-\) 2013.195 ADS.74, CSAR-HiQ.51 and CSAR-HiQ.36. Across these test sets, our DAAP approaches demonstrate superior predictive performance in protein-ligand binding affinity. On the CASF \(-\) 2013.87 dataset, EGNA surpasses CAPLA with higher R-value and CI-value of 0.752 and 0.767, respectively, while CAPLA records lower RMSE, MAE and SD values of 1.512, 1.197, and 1.521. In contrast, our DAAP20 surpasses both, excelling in all metrics with an R of 0.811, RMSE of 1.324, MAE of 1.043, SD of 1.332, and CI of 0.813, with DAAP16 also delivering robust performance. For the CASF \(-\) 2013.195 test set, a similar trend is observed with our DAAP20 approach outperforming the nearest state-of-the-art predictor by a significant margin of 8%-20% across all evaluation metrics. The DAAP16 approach, not DAAP20, stands out on the ADS.74 dataset by surpassing predictors like Pafnucy, SFCNN and EGNA, showcasing substantial improvements of approximately 12%-37% in various metrics. When evaluating the CSAR-HiQ.51 and CSAR-HiQ.36 datasets against six state-of-the-art predictors, DAAP20 consistently outperforms all, indicating enhancements of 2%-20% and 3%-31%, respectively. Although DAAP16 does not surpass ResBiGAAT in CSAR-HiQ.51, it notably excels in the CSAR-HiQ.36 dataset, outperforming ResBiGAAT in all metrics except MAE. These results underscore the exceptional predictive capabilities of our DAAP approach across diverse datasets and evaluation criteria, consistently surpassing existing state-of-the-art predictors.
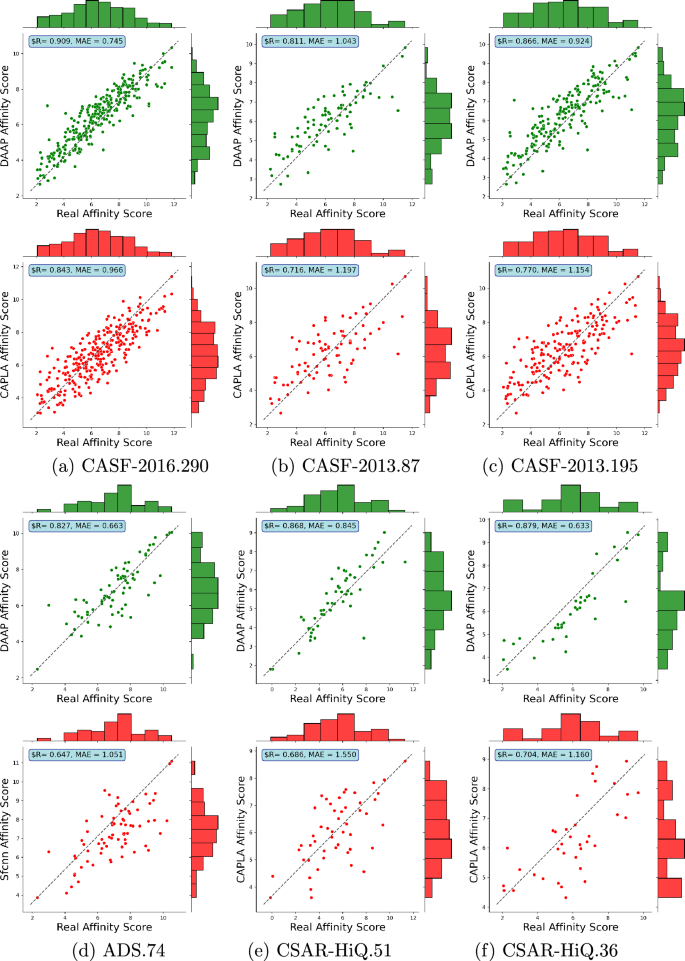
The distributions of real and predicted binding affinity values by our predictor (green) and the closest state-of-the-art predictor (red) across the six test sets
Figure 2 presents the distributions of actual and predicted binding affinities for our best DAAP approach and the closest state-of-the-art predictor. In all six test sets, a clear linear correlation and low mean absolute error (MAE) between predicted and actual binding affinity values can be observed for our DAAP model, demonstrating the strong performance of our model across these test sets. The other predictors show scattering over larger areas. In our analysis, we could not consider ResBiGAAT in the CSAR-HiQ.51 and CSAR-HiQ.36 datasets due to the unavailability of their results.
Ablation study and explainability
A significant contribution of this work is utilising distance matrix input features to capture critical information about the protein-ligand relationship. Specifically, we employ a concatenation of three distance maps, representing donor-acceptor, hydrophobic, and \(\pi \) -stacking interactions, as input features, effectively conveying essential protein-ligand bonding details. Following finalising our prediction architecture by incorporating two additional features derived from protein and SMILES sequences, we conduct an in-depth analysis of the impact of various combinations of these distance matrices as features. In the case of protein features, residues are selected based on which distance maps are considered.
Table 6 illustrates the outcomes obtained from experimenting with different combinations of distance maps and selected protein residue and ligand SMILES features on the CASF \(-\) 2016.290 test set. We devise four unique combinations, employing three distinct distance maps for both the PDBbind2016 and PDBbind2020 training datasets. Additionally, we explore a combination that integrates donor-acceptor, hydrophobic, and \(\pi \) -stacking distance maps with features from all protein residues, denoted as DA + \(\pi \) S + HP + FP, to evaluate the impact of using all residues versus selected ones.
From the information presented in Table 6 , it is evident that utilizing the donor-acceptor (DA) solely distance maps yields the lowest performance across both training sets, particularly when different combinations of distance maps are paired with selective protein residues. However, as expected, the combination of the three distance maps, namely DA, \(\pi \) S ( \(\pi \) -stacking), and HP (Hydrophobicity), demonstrates superior performance compared to other combinations. Notably, the combination of DA and HP outperforms the other two combinations but falls short of our best-performing feature set. The ensemble of DA, \(\pi \) S, HP and all protein residues exhibit the least favourable outcomes among the tested combinations. This result aligns with our expectations, as Hydrophobic interactions are the most prevalent in protein-ligand binding, underscoring their significance in feature analysis.
Integrating an attention mechanism into our model is crucial in achieving improved results. After consolidating the outputs of three 1D-CNN blocks, we employ attention, each receiving inputs from distance maps, protein sequences, and ligand sequences. The dimension of the feature is 384. As depicted in Fig. 3 , the heatmap visualization highlights the differential attention weights assigned to various features, with brighter and darker regions indicating higher weights to certain features, thus improving binding affinity predictions. This process underscores the mechanism’s ability to discern and elevate critical features, showing that not all features are equally important. Further emphasizing the significance of attention, a comparative analysis using the same model architecture without the attention mechanism on the same features-shown in the last row of Table 6 demonstrates its vital role in boosting predictive accuracy. This comparison not only reinforces the value of the attention mechanism in detecting intricate patterns within the feature space but also significantly enhances the model’s predictive capabilities.
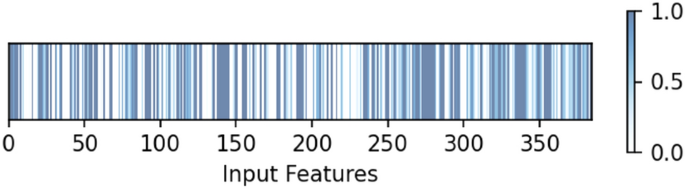
Visualization of attention maps for concatenated features in the 1o0h protein-ligand complex of the CASF \(-\) 2016.290 dataset
Statistical analysis
In assessing the statistical significance of performance differences between DAAP and its closest competitors, Wilcoxon Signed Ranked Tests at a 95% confidence level were conducted. Comparisons included DAAP against CAPLA for CASF \(-\) 2016.290, CASF \(-\) 2013.87, CASF \(-\) 2013.195, CSAR-HiQ.36, and CSAR-HiQ.51 datasets and between DAAP and SFCNN for the ADS.74 test set. Unfortunately, ResBiGAAT’s results were unavailable for inclusion in the analysis. Table 7 depicts that DAAP demonstrated statistical significance compared to the closest state-of-the-art predictor across various test sets, as indicated by p-values ranging from 0.000 to 0.047. The consistently negative mean Z-values, ranging from \(-\) 14.71 to \(-\) 5.086, suggest a systematic improvement in predictive performance. Moreover, higher mean rankings, ranging from 19.5 to 144.5, further emphasize the overall superiority of DAAP. Notably, the superior performance is observed across diverse datasets, including CASF \(-\) 2016.290, CASF \(-\) 2013.87, CASF \(-\) 2013.195, ADS.74, CSAR-HiQ.51, and CSAR-HiQ.36. These findings underscore the robustness and effectiveness of DAAP in predicting protein-ligand binding affinity.
Screening results
In this section, we scrutinize the effectiveness of our predicted affinity scores to accurately differentiate between active binders (actives) and non-binders (decoys) throughout the screening procedure. To this end, we have carefully curated a subset of seven hand-verified targets from the Database of Useful Decoys: Enhanced (DUD-E), accessible via https://dude.docking.org , to serve as our evaluative benchmark. The details about seven targets are given in Table 8 . This table underscores the diversity and challenges inherent in the dataset, reflecting a wide range of D/A ratios that present a comprehensive framework for evaluating the discriminatory power of our predicted affinity scores.
To construct protein-ligand complexes for these targets, we employed AutoDock Vina, configuring the docking grid to a \(20\mathring{A} \times 20\mathring{A} \times 20\mathring{A}\) cube centred on the ligand’s position. This setup and 32 consecutive Monte-Carlo sampling iterations identified the optimal pose for each molecule pair. Our evaluation of the screening performance utilizes two pivotal metrics: the Receiver Operating Characteristic (ROC) curve [ 33 ] and the Enrichment Factor (EF) [ 34 ]. Figure 4 shows the ROC curve and the EF graph for a detailed examination of a predictive model’s efficacy in virtual screening. The ROC curve’s analysis, with AUC values spanning from 0.63 to 0.76 for the seven targets, illustrates our model’s proficient capability in differentiating between actives and decoys. These values, closely approaching the top-left corner of the graph, denote a high true positive rate alongside a low false positive rate, underscoring our model’s efficacy.
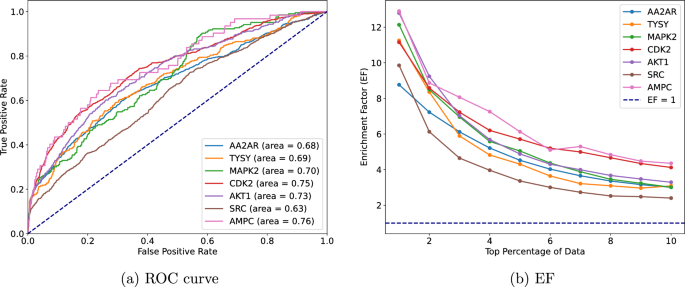
Screening Performance of the Predictive Model: Roc curve (left) and EF (right)
Furthermore, the EF graph of Fig. 4 provides a quantitative assessment of the model’s success in prioritizing active compounds within the top fractions of the dataset, notably the top 1% to 10%. Initial EF values ranging from 12.3 to 9.9 for the top 1% underscore our model’s exceptional ability to enrich active compounds beyond random chance significantly. This pronounced enrichment highlights the model’s utility in the early identification of promising candidates. However, the observed gradual decline in EF values with increasing dataset fractions aligns with expectations, reflecting the challenge of sustaining high enrichment levels across broader selections.
Conclusions
In our protein-ligand binding affinity prediction, we introduce atomic-level distance map features encompassing donor-acceptor, hydrophobic, and \(\pi \) -stacking interactions, providing deeper insights into interactions for precise predictions, both for short and long-range. We enhance our model further with specific protein sequence features of specific residues and ligand SMILES information. These features are integrated into an attention-based 1D-CNN architecture that is used a number of times for ensemble-based performance enhancement, resulting in superior results compared to existing methods across six benchmark datasets. Remarkably, on the CASF-2016 dataset, our model achieves a Correlation Coefficient (R) of 0.909, Root Mean Squared Error (RMSE) of 0.987, Mean Absolute Error (MAE) of 0.745, Standard Deviation (SD) of 0.988, and Concordance Index (CI) of 0.876, signifying its potential to advance drug discovery binding affinity prediction. The program and data are publicly available on the website https://gitlab.com/mahnewton/daap .
We describe the protein-ligand dataset used in our work. We also describe our proposed method in terms of its input features, output representations, and deep learning architectures.
Protein-ligand datasets
In the domain of protein-ligand binding affinity research, one of the primary sources for training, validation, and test sets is the widely recognized PDBbind database [ 35 ]. This database is meticulously curated. It comprises experimentally verified protein-ligand complexes. Each complex encompasses the three-dimensional structures of a protein-ligand pair alongside its corresponding binding affinities expressed as \(pK_d\) values. The PDBbind database ( http://www.pdbbind.org.cn/ ) is subdivided into two primary subsets: the general set and the refinement set . The PDBbind version 2016 dataset (named PDBbind2016) contains 9221 and 3685 unique protein-ligand complexes, while the PDBbind version 2020 dataset (named PDBbind2020) includes 14127 and 5316 protein-ligand complexes in the general and refinement sets, respectively.
Similar to the most recent state-of-the-art affinity predictors such as Pafnucy [ 20 ], DeepDTAF [ 23 ], OnionNet [ 3 ], DLSSAffinity [ 4 ], LuEtAl [ 36 ], EGNA [ 25 ] and CAPLA [ 26 ], our DAAP16 method is trained using the 9221 + 3685 = 12906 protein-ligand complexes in the general and refinement subsets of the PDBbind dataset version 2016 . Following the same training-validation set formation approach of the recent predictors such as Pafnucy, OnionNet, DeepDTAF, DLSSAffinity and CAPLA, we put 1000 randomly selected protein-ligand complexes in the validation set and the remaining 11906 distinct protein-ligand pairs in the training set. Another version of DAAP, named DAAP20, was generated using the PDBbind database version 2020 , which aligns with the training set of ResBiGAAT [ 27 ]. To avoid overlap, we filtered out protein-ligand complexes common between the PDBbind2020 training set and the six independent test sets. After this filtering process, 19027 unique protein-ligand complexes were retained for training from the initial pool of 19443 in PDBbind2020.
To ensure a rigorous and impartial assessment of the effectiveness of our proposed approach, we employ six well-established, independent blind test datasets. There is no overlap of protein-ligand complexes between the training sets and these six independent test sets.
CASF-2016.290 The 290 protein-ligand complexes, commonly referred to as CASF-2016, are selected from the PDBbind version 2016 core set ( http://www.pdbbind.org.cn/casf.php ) and have become the gold standard test set for recent affinity predictors such as DLSSAffinity [ 4 ], LuEtAl [ 36 ], EGNA [ 25 ] and CAPLA [ 26 ].
CASF-2013.87 and CASF-2013.195 Similar to the approach taken by DLSSAffinity [ 4 ], we carefully curated 87 unique protein-ligand complexes from the CASF-2013 dataset, which originally consists of 195 complexes ( http://www.pdbbind.org.cn/casf.php ). These 87 complexes were chosen to ensure no overlap with our training set or the CASF-2016 test set. Additionally, we use the entire set of 195 complexes as another test set, named CASF \(-\) 2013.195.
ADS.74 This test set from SFCNN [ 24 ] comprises 74 protein-ligand complexes sourced from the Astex diverse set [ 37 ].
CSAR-HiQ.51 and CSAR-HiQ.36 These two test datasets contain 51 and 36 protein-ligand complexes from the well-known CSAR [ 38 ] dataset. Recent affinity predictors such as EGNA [ 25 ], CAPLA and ResBiGAAT [ 26 , 27 ] have employed CSAR as a benchmark dataset. To get our two test datasets, we have followed the procedure of CAPLA and filtered out protein-ligand complexes with duplicate PDB IDs from two distinct CSAR subsets containing 176 and 167 protein-ligand complexes, respectively.
Input features
Given protein-ligand complexes in the datasets, we extract three distinctive features from proteins, ligands, and protein-ligand binding pockets. We describe these below.
Protein representation
We employ three distinct features for encoding protein sequences: one-hot encoding of amino acids, a Hidden Markov model based on multiple sequence alignment features (HHM), and seven physicochemical properties.
In the one-hot encoding scheme for the 20 standard amino acids and non-standard amino acids, each amino acid is represented by a 21-dimensional vector. This vector contains twenty “0 s” and one “1”, where the position of the “1” corresponds to the amino acid index in the protein sequence.
To construct the HHM features, we have run an iterative searching tool named HHblits [ 39 ] against the Uniclust30 database ( http://wwwuser.gwdg.de/~compbiol/uniclust/2020_06/ ) as of June 2020. This process allows us to generate HHM sequence profile features for the proteins in our analysis. Each resulting .hhm feature file contains 30 columns corresponding to various parameters such as emission frequencies, transition frequencies, and Multiple Sequence Alignment (MSA) diversities for each residue. Like EGNA, for columns 1 to 27, the numbers are transformed into frequencies using the formula \(f = 2^{-0.001*p}\) , where f represents the frequency, and p is the pseudo-count. This transformation allows the conversion of these parameters into frequency values. Columns 28 to 30 are normalized using the equation: \(f = \frac{0.001*p}{20}\) . This normalization process ensures that these columns are appropriately scaled for further analysis and interpretation.
The seven physicochemical properties [ 14 , 29 ] for each amino acid residue are steric parameter (graph shape index), hydrophobicity, volume, polarisability, isoelectric point, helix probability, and sheet probability. When extracting these three features for protein residues, we focused exclusively on the 20 standard amino acid residues. If a residue is non-standard, we assigned a feature value of 0.0.
In our approach, we initially concatenate all three features sequentially for the entire protein sequence. Subsequently, to enhance the specificity of our model, we employ a filtering strategy where residues lacking donor [ 40 ], hydrophobic [ 31 ], and \(\pi \) -stacking [ 32 ] atoms within their amino acid side chains are excluded from the analysis. Additionally, to prevent overlap, we select unique residues after identification based on donor, hydrophobic, or \(\pi \) -stacking atoms for each protein sequence. The rationale behind this filtering is to focus on residues that are actively involved in critical interactions relevant to protein-ligand binding. The resulting feature dimension for each retained protein residue is 58. This feature set includes one-hot encoding of amino acids, a Hidden Markov model based on multiple sequence alignment features (HHM), and seven physicochemical properties. These features are comprehensively summarised in Table 9 for clarity.
Considering the variable numbers of residues that proteins can possess, we have considered a standardized protein sequence length to align with the fixed-size requirements of deep learning algorithms. In our initial experiments exploring various sequence lengths in the datasets, we found that a maximum length of 500 yields better performance in terms of pearson correlation coefficient (R) and mean absolute error (MAE). If the number of selected residues falls below 500, we pad the sequence with zeros; conversely, if it exceeds 500, we truncate it to 500 from the initial position of the sequence. The final dimension of each protein is \(500\times 58\) .
Ligand representation
We use SMILES to represent ligands. SMILES is a widely adopted one-dimensional representation of chemical structures of ligands [ 41 ]. To convert ligand properties such as atoms, bonds, and rings from ligand SDF files into SMILES strings, we use the Open Babel chemical tool [ 42 ]. The SMILES strings comprise 64 unique characters, each corresponding to a specific numeric digit ranging from 1 to 64. For example, the SMILES string “HC(O=)N” is represented as [12, 42, 1, 48, 40, 31, 14]. In line with our protein representation approach, we set a fixed length of 150 characters for each SMILES string.
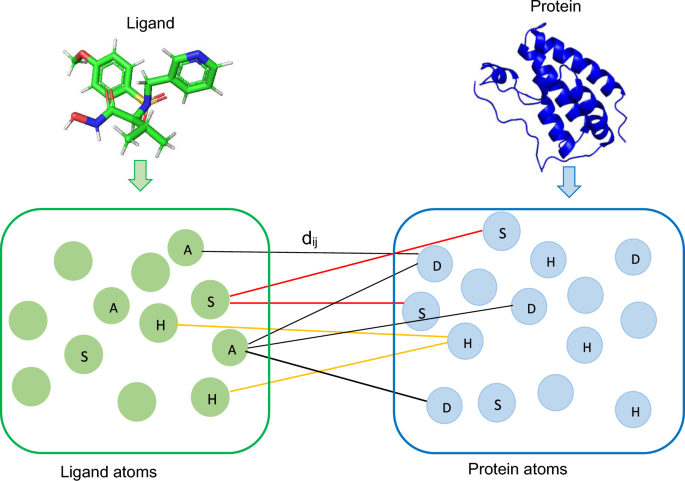
Various distance measures that potentially capture protein-ligand interactions. In the figure, \(d_{ij}\) represents the distance between a donor (D), hydrophobic (H), or \(\pi \) -stacking (S) atom i in the protein and the corresponding acceptor (A), hydrophobic (H), or \(\pi \) -stacking (S) atom j in the ligand. Empty circles represent other atom types. Different colour lines represent different types of interactions
Binding pocket representation
A binding pocket refers to a cavity located either on the surface or within the interior of a protein. A binding pocket possesses specific characteristics that make it suitable for binding a ligand [ 43 ]. Protein residues within the binding pocket region exert a direct influence, while residues outside this binding site can also have a far-reaching impact on affinity prediction. Among various protein-ligand interactions within the binding pocket regions, donor-acceptor atoms [ 30 ], hydrophobic contacts [ 31 , 32 ], and \(\pi \) -stacking [ 31 , 32 ] interactions are the most prevalent, and these interactions could significantly contribute to the enhancement of affinity score prediction. The formation of the protein-ligand complexes involves donor atoms from the proteins and acceptor atoms from the ligands. This process is subject to stringent chemical and geometric constraints associated with protein donor groups and ligand acceptors [ 30 ]. Hydrophobic interactions stand out as the primary driving force in protein-ligand interactions, while \(\pi \) -stacking interactions, particularly involving aromatic rings, play a substantial role in protein-ligand interactions [ 32 ]. However, there are instances where donor-acceptor interactions alone may not suffice, potentially failing to capture other interactions that do not conform to traditional donor-acceptor patterns. In such scenarios, hydrophobic contacts and \(\pi \) -stacking interactions become essential as they could provide valuable insights for accurate affinity prediction.
We employ three types of distance matrices in our work shown in Fig. 5 to capture protein-ligand interactions. The first one is the donor-acceptor distance matrix , which considers distances between protein donor atoms and acceptor ligand atoms, with data sourced from mol2/SDF files. We ensure that all ligand atoms contribute to the distance matrix construction, even in cases where ligands lack explicit acceptor atoms. Furthermore, we calculate the hydrophobic distance matrix by measuring the distance between hydrophobic protein atoms and hydrophobic ligand atoms, ensuring the distance is less than \(4.5\mathring{A}\) [ 31 ]. Similarly, we compute the \(\pi \) - stacking distance matrix by considering protein and ligand \(\pi \) -stacking atoms and applying a distance threshold of \(4.0\mathring{A}\) [ 32 ]. These three types of atoms are selected from the heavy atoms, referring to any atom that is not hydrogen.
We discretize the initially calculated real-valued distance matrices representing the three types of interactions into binned distance matrices. These matrices are constrained within a maximum distance threshold of \(20\mathring{A}\) . The decision to set a maximum distance threshold of \(20\mathring{A}\) for capturing the binding pocket’s spatial context is informed by practices in both affinity prediction and protein structure prediction fields. Notably, methodologies like Pafnucy [ 20 ], DLSSAffinity [ 4 ], and EGNA [ 25 ], as well as advanced protein structure prediction models such as AlphaFold [ 28 ] and trRosetta [ 44 ], utilize a 20Å range to define interaction spaces or predict structures. This consensus on the 20Å threshold reflects its sufficiency in providing valuable spatial information necessary for accurate modeling. The distance values ranging from \(0\mathring{A} - 20\mathring{A}\) are discretized into 40 bins, each with a \(0.5\mathring{A}\) interval. Any distance exceeding \(20\mathring{A}\) is assigned to the \(41^{st}\) bin. In our experimentation, we explored different distance ranges ( \(20\mathring{A}\) , \(25\mathring{A}\) , \(30\mathring{A}\) , \(35\mathring{A}\) , and \(40\mathring{A}\) ) while maintaining a uniform bin interval of \(0.5\mathring{A}\) . Among these ranges, \(20\mathring{A}\) yielded optimal results, and as such, we adopted it for our final analysis. Following this binning process, the original real-valued distances in the matrices are substituted with their corresponding bin numbers. Subsequently, we convert the 2D distance matrix into a 1D feature vector. We concatenate the three 1D vectors representing the three distinct interactions into a single vector to construct the final feature vector. To ensure consistency, the maximum length of the feature vector is set to 1000 for each pocket.
Output representations
This binding affinity is measured in the dissociation constant ( \(K_d\) ). For simplicity in calculations, the actual affinity score \(K_d\) is commonly converted into \(pK_d\) by taking the negative logarithm of \(K_d\) .
Deep learning architectures
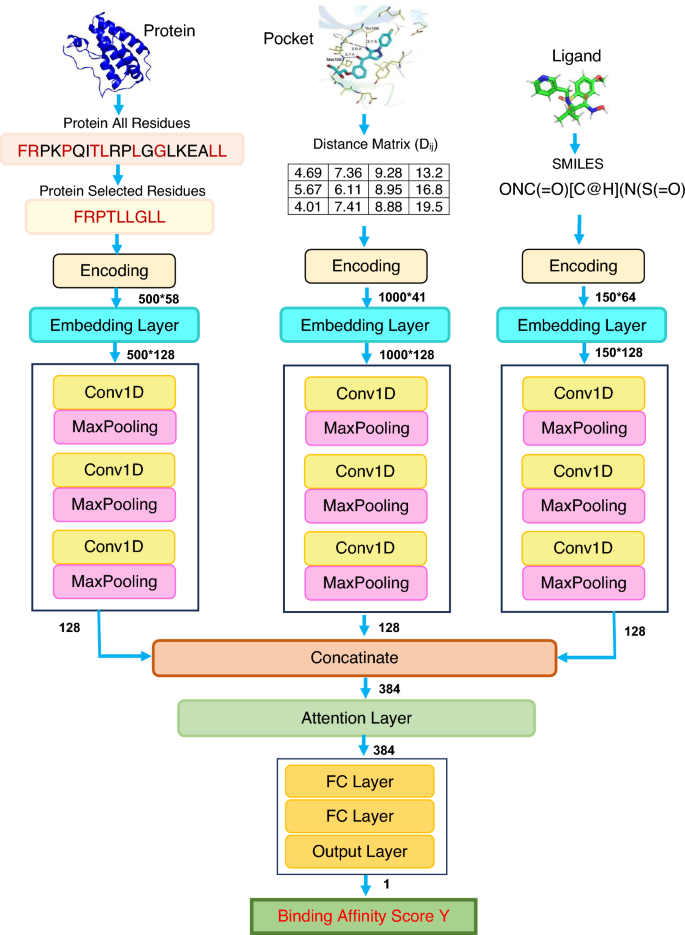
The proposed model architecture
We propose a deep-learning regression model to predict protein-ligand binding affinities, shown in Fig. 6 . Our model comprises three integral components: convolutional neural network (CNN), attention mechanism, and fully connected neural network (FCNN). Before feeding to the CNN block, information from three distinct feature sources (proteins, ligands, and interactions) is encoded and subsequently processed through the embedding layer. The embedding layer transforms the inputs into fixed-length vectors of a predefined size (in this case, 128 dimensions), enabling more effective feature representation with reduced dimensionality. During training, our model operates with a batch size of 16 and is optimized using the Adam optimizer and a learning rate set at 0.001. We adopt the log cosh loss function for this work to optimise the model’s performance. The training regimen consists of 200 epochs, with the best model selected based on the validation loss, and a dropout rate of 0.2 is applied. The explored hyperparameter settings are summarised in Table 10 . We have explored these settings, and after preliminary experiments, we have selected these values which are emboldened.
Convolutional neural network
Much like DLSSAffinity [ 4 ], our model employs three 1D-CNN blocks, each dedicated to processing distinct feature sources: proteins, ligands, and interactions in pockets. Each of these 1D-CNN blocks comprises three convolutional layers paired with three Maxpooling layers. The configuration of the first two 1D-CNN blocks includes 32, 64, and 128 filters, each with corresponding filter lengths of 4, 8, and 12. In contrast, the 1D-CNN block responsible for handling SMILES sequence inputs features filters with 4, 6, and 8 adjusted lengths. Each of the three 1D-CNN blocks in our model generates a 128-dimensional output. Subsequently, before progressing to the next stage, the outputs of these three 1D-CNN blocks are concatenated and condensed into a unified 384-dimensional output.
Attention mechanism
In affinity prediction, attention mechanisms serve as crucial components in neural networks, enabling models to allocate varying levels of focus to distinct facets of input data [ 5 ]. These mechanisms play a critical role in weighing the significance of different features or entities when assessing their interaction strength. The attention mechanism uses the formula below.
We use the Scaled Dot-Product Attention [ 45 ] mechanism to calculate and apply attention scores to the input data. The attention mechanism calculates query ( Q ), key ( K ), and value ( V ) matrices from the input data. In this context, Q is a vector capturing a specific aspect of the input, K represents the context or memory of the model with each key associated with a value, and V signifies the values linked to the keys. It computes attention scores using the dot product of Q and K matrices, scaled by the square root of the dimensionality ( \(d_k\) ). Subsequently, a softmax function normalises the attention scores. Finally, the output is generated as a weighted summation of the value (V) matrix, guided by the computed attention scores.
Notably, the output of the concatenation layer passes through the attention layer. The input to the attention layer originates from the output of the concatenation layer, preserving the same dimensionality as the input data. This design ensures the retention of crucial structural information throughout the attention mechanism.
Fully connected neural network
The output of the attention layer transitions into the subsequent stage within our model architecture, known as the Fully Connected Neural Network (FCNN) block. The FCNN block consists of two fully connected (FC) layers, where the two layers have 256 and 128 nodes respectively. The final stage in our proposed prediction model is the output layer, which follows the last FC layer.
Evaluation metrics
We comprehensively evaluate our affinity prediction model using five well-established performance metrics. The Pearson Correlation Coefficient (R) [ 4 , 24 , 26 , 36 ] measures the linear relationship between predicted and actual values. The Root Mean Square Error (RMSE) [ 4 , 24 , 26 ] and the Mean Absolute Error (MAE) [ 24 , 26 ] assess prediction accuracy and error dispersion. The Standard Deviation (SD) [ 4 , 24 , 26 , 36 ] evaluates prediction consistency, and the Concordance Index (CI) [ 26 , 36 ] determines the model’s ability to rank protein-ligand complexes accurately. Higher R and CI values and lower RMSE, MAE, and SD values indicate better prediction accuracy. These metrics are collectively very robust measures for comparison of our model’s performance against that of the state-of-the-art techniques in the field of affinity prediction.
N : the number of protein-ligand complexes
\(Y_{\text {act}}\) : experimentally measured actual binding affinity values for the protein-ligand complexes
\(Y_{\text {pred}}\) : the predicted binding affinity values for the given protein-ligand complexes
\(y_{\text {act}_i}\) and \(y_{\text {pred}_i}\) : respectively the actual and predicted binding affinity value of the \(i^{th}\) protein-ligand complex
a : is slope
b : interpretation of the linear regression line of the predicted and actual values. Z : the normalization constant, i.e. the number of data pairs with different label values.
h ( u ): the step function that returns 1.0, 0.5, and 0.0for \(u>0\) , \(u = 0\) , and \(u<0\) respectively.
Availability of data and materials
The program and corresponding data are publicly available on the website https://gitlab.com/mahnewton/daap .
DiMasi JA, Grabowski HG, Hansen RW (2016) Innovation in the pharmaceutical industry: new estimates of r &d costs. J Health Econ 47:20–33
Article PubMed Google Scholar
Gilson MK, Zhou H-X (2007) Calculation of protein-ligand binding affinities. Ann Rev Biophys Biomol Str 36(1):21–42
Article CAS Google Scholar
Zheng L, Fan J, Mu Y (2019) Onionnet: a multiple-layer intermolecular-contact-based convolutional neural network for protein-ligand binding affinity prediction. ACS Omega 4(14):15956–15965
Article CAS PubMed PubMed Central Google Scholar
Wang H, Liu H, Ning S, Zeng C, Zhao Y (2022) Dlssaffinity: protein-ligand binding affinity prediction via a deep learning model. Phys Chem Chem Phys 24(17):10124–10133
Article CAS PubMed Google Scholar
Seo S, Choi J, Park S, Ahn J (2021) Binding affinity prediction for protein-ligand complex using deep attention mechanism based on intermolecular interactions. BMC Bioinform 22(1):1–15
Article Google Scholar
Deng W, Breneman C, Embrechts MJ (2004) Predicting protein- ligand binding affinities using novel geometrical descriptors and machine-learning methods. J Chem Inf Comput Sci 44(2):699–703
Li L, Wang B, Meroueh SO (2011) Support vector regression scoring of receptor-ligand complexes for rank-ordering and virtual screening of chemical libraries. J Chem Inf Modeling 51(9):2132–2138
Ballester PJ, Mitchell JB (2010) A machine learning approach to predicting protein-ligand binding affinity with applications to molecular docking. Bioinformatics 26(9):1169–1175
Li H, Peng J, Sidorov P, Leung Y, Leung K-S, Wong M-H, Lu G, Ballester PJ (2019) Classical scoring functions for docking are unable to exploit large volumes of structural and interaction data. Bioinformatics 35(20):3989–3995
Deng L, Platt J. Ensemble deep learning for speech recognition. In: Proc. Interspeech. 2014
Chen C, Seff A, Kornhauser A, Xiao J. Deepdriving: learning affordance for direct perception in autonomous driving. In: Proceedings of the IEEE International Conference on Computer Vision, 2015; pp. 2722–2730
Lin T-Y, RoyChowdhury A, Maji S (2017) Bilinear convolutional neural networks for fine-grained visual recognition. IEEE Trans Pattern Anal Mach Intell 40(6):1309–1322
Newton MH, Rahman J, Zaman R, Sattar A. Enhancing protein contact map prediction accuracy via ensembles of inter-residue distance predictors. Computational Biology and Chemistry, 2022; 107700.
Rahman J, Newton MH, Hasan MAM, Sattar A (2022) A stacked meta-ensemble for protein inter-residue distance prediction. Comput Biol Med 148:105824
Isert C, Atz K, Schneider G (2023) Structure-based drug design with geometric deep learning. Curr Opin Struct Biol 79:102548
Krentzel D, Shorte SL, Zimmer C (2023) Deep learning in image-based phenotypic drug discovery. Trend Cell Biol 33(7):538–554
Yang L, Jin C, Yang G, Bing Z, Huang L, Niu Y, Yang L (2023) Transformer-based deep learning method for optimizing admet properties of lead compounds. Phys Chem Chem Phys 25(3):2377–2385
Masters MR, Mahmoud AH, Wei Y, Lill MA (2023) Deep learning model for efficient protein-ligand docking with implicit side-chain flexibility. J Chem Inf Modeling 63(6):1695–1707
Öztürk H, Özgür A, Ozkirimli E (2018) Deepdta: deep drug-target binding affinity prediction. Bioinformatics 34(17):821–829
Stepniewska-Dziubinska MM, Zielenkiewicz P, Siedlecki P (2018) Development and evaluation of a deep learning model for protein-ligand binding affinity prediction. Bioinformatics 34(21):3666–3674
Jiménez J, Skalic M, Martinez-Rosell G, De Fabritiis G (2018) \(k_{deep}\) : protein-ligand absolute binding affinity prediction via 3d-convolutional neural networks. J Chem Inf Modeling 58(2):287–296
Li Y, Rezaei MA, Li C, Li X (2019) Deepatom: a framework for protein-ligand binding affinity prediction. In: 2019 IEEE International Conference on Bioinformatics and Biomedicine (BIBM), pp. 303–310, IEEE
Wang K, Zhou R, Li Y, Li M (2021) Deepdtaf: a deep learning method to predict protein-ligand binding affinity. Brief Bioinf 22(5):072
Wang Y, Wei Z, Xi L (2022) Sfcnn: a novel scoring function based on 3d convolutional neural network for accurate and stable protein-ligand affinity prediction. BMC Bioinform 23(1):1–18
Xia C, Feng S-H, Xia Y, Pan X, Shen H-B (2023) Leveraging scaffold information to predict protein-ligand binding affinity with an empirical graph neural network. Brief Bioinf. https://doi.org/10.1093/bib/bbac603
Jin Z, Wu T, Chen T, Pan D, Wang X, Xie J, Quan L, Lyu Q (2023) Capla: improved prediction of protein-ligand binding affinity by a deep learning approach based on a cross-attention mechanism. Bioinformatics 39(2):049
Abdelkader GA, Njimbouom SN, Oh T-J, Kim J-D (2023) Resbigaat: Residual bi-gru with attention for protein-ligand binding affinity prediction. Computational Biology and Chemistry, 107969
Senior AW, Evans R, Jumper J, Kirkpatrick J, Sifre L, Green T, Qin C, Žídek A, Nelson AW, Bridgland A et al (2020) Improved protein structure prediction using potentials from deep learning. Nature 577(7792):706–710
Rahman J, Newton MH, Islam MKB, Sattar A (2022) Enhancing protein inter-residue real distance prediction by scrutinising deep learning models. Sci Rep 12(1):787
Raschka S, Wolf AJ, Bemister-Buffington J, Kuhn LA (2018) Protein-ligand interfaces are polarized: discovery of a strong trend for intermolecular hydrogen bonds to favor donors on the protein side with implications for predicting and designing ligand complexes. J Computer-aided Mol Design 32:511–528
Jubb HC, Higueruelo AP, Ochoa-Montaño B, Pitt WR, Ascher DB, Blundell TL (2017) Arpeggio: a web server for calculating and visualising interatomic interactions in protein structures. J Mol Biol 429(3):365–371
Freitas RF, Schapira M (2017) A systematic analysis of atomic protein-ligand interactions in the pdb. Medchemcomm 8(10):1970–1981
Empereur-Mot C, Guillemain H, Latouche A, Zagury J-F, Viallon V, Montes M (2015) Predictiveness curves in virtual screening. J Cheminf 7(1):1–17
Li H, Zhang H, Zheng M, Luo J, Kang L, Liu X, Wang X, Jiang H (2009) An effective docking strategy for virtual screening based on multi-objective optimization algorithm. BMC Bioinf 10:1–12
Liu T, Lin Y, Wen X, Jorissen RN, Gilson MK (2007) Bindingdb: a web-accessible database of experimentally determined protein-ligand binding affinities. Nucleic Acids Res 35(suppl–1):198–201
Lu Y, Liu J, Jiang T, Guan S, Wu H. Protein-ligand binding affinity prediction based on deep learning. In: International Conference on Intelligent Computing, 2022; pp. 310–316. Springer.
Hartshorn MJ, Verdonk ML, Chessari G, Brewerton SC, Mooij WT, Mortenson PN, Murray CW (2007) Diverse, high-quality test set for the validation of protein- ligand docking performance. J Med Chem 50(4):726–741
Dunbar JB Jr, Smith RD, Yang C-Y, Ung PM-U, Lexa KW, Khazanov NA, Stuckey JA, Wang S, Carlson HA (2011) Csar benchmark exercise of 2010: selection of the protein-ligand complexes. J Chem Inf Modeling 51(9):2036–2046
Remmert M, Biegert A, Hauser A, Söding J (2012) Hhblits: lightning-fast iterative protein sequence searching by hmm-hmm alignment. Nat Methods 9(2):173–175
Hydrogen donor and acceptor atoms of the amino acid. https://www.imgt.org/IMGTeducation/Aide-memoire/_UK/aminoacids/charge/ . Accessed: 13-08-2023
Weininger D (1988) Smiles, a chemical language and information system. 1. introduction to methodology and encoding rules. J Chem Inf Comput Sci 28(1):31–36
O’Boyle NM, Banck M, James CA, Morley C, Vandermeersch T, Hutchison GR (2011) Open babel: An open chemical toolbox. J Cheminf 3(1):1–14
Google Scholar
Stank A, Kokh DB, Fuller JC, Wade RC (2016) Protein binding pocket dynamics. Accounts Chem Res 49(5):809–815
Yang J, Anishchenko I, Park H, Peng Z, Ovchinnikov S, Baker D (2020) Improved protein structure prediction using predicted interresidue orientations. Proc Natl Acad Sci 117(3):1496–1503
Vaswani A, Shazeer N, Parmar N, Uszkoreit J, Jones L, Gomez AN, Kaiser Ł, Polosukhin I (2017) Attention is all you need. Advances in neural information processing systems 30
Download references
Acknowledgements
This research is partially supported by the research seed grant awarded to M.A.H.N. at the University of Newcastle. The research team acknowledges the valuable assistance of the Griffith University eResearch Service & Specialised Platforms team for granting access to their High-Performance Computing Cluster, which played a crucial role in completing this research endeavour.
This research is partially supported by the research seed Grant awarded to M.A.H.N. at the University of Newcastle.
Author information
Julia Rahman and M. A. Hakim Newton are co-first-authors and contributed equally.
Authors and Affiliations
School of Information and Communication Technology, Griffith University, 170 Kessels Rd, Nathan, 4111, QLD, Australia
Julia Rahman
Institute for Integrated and Intelligent Systems (IIIS), Griffith University, 170 Kessels Rd, Nathan, 4111, QLD, Australia
M. A. Hakim Newton & Abdul Sattar
School of Information and Physical Sciences, University of Newcastle, University Dr, Callaghan, 2308, NSW, Australia
M. A. Hakim Newton
Department of Computer Science & Engineering, Bangladesh University of Engineering and Technology, Palashi, 1205, Dhaka, Bangladesh
Mohammed Eunus Ali
You can also search for this author in PubMed Google Scholar
Contributions
The contributions of the authors to this work were as follows: J.R. and M.A.H.N. played equal roles in all aspects of the project, including conceptualization, data curation, formal analysis, methodology, software development, and writing of the initial draft. M.E.A. helped in the concept development, review and editing of the manuscript. A.S. actively engaged in discussions, facilitated funding acquisition, provided supervision, and thoroughly reviewed the manuscript.
Corresponding author
Correspondence to Julia Rahman .
Ethics declarations
Competing interests.
No Conflict of interest is declared.
Additional information
Publisher's note.
Springer Nature remains neutral with regard to jurisdictional claims in published maps and institutional affiliations.
Rights and permissions
Open Access This article is licensed under a Creative Commons Attribution 4.0 International License, which permits use, sharing, adaptation, distribution and reproduction in any medium or format, as long as you give appropriate credit to the original author(s) and the source, provide a link to the Creative Commons licence, and indicate if changes were made. The images or other third party material in this article are included in the article’s Creative Commons licence, unless indicated otherwise in a credit line to the material. If material is not included in the article’s Creative Commons licence and your intended use is not permitted by statutory regulation or exceeds the permitted use, you will need to obtain permission directly from the copyright holder. To view a copy of this licence, visit http://creativecommons.org/licenses/by/4.0/ . The Creative Commons Public Domain Dedication waiver ( http://creativecommons.org/publicdomain/zero/1.0/ ) applies to the data made available in this article, unless otherwise stated in a credit line to the data.
Reprints and permissions
About this article
Cite this article.
Rahman, J., Newton, M.A.H., Ali, M.E. et al. Distance plus attention for binding affinity prediction. J Cheminform 16 , 52 (2024). https://doi.org/10.1186/s13321-024-00844-x
Download citation
Received : 01 December 2023
Accepted : 24 April 2024
Published : 12 May 2024
DOI : https://doi.org/10.1186/s13321-024-00844-x
Share this article
Anyone you share the following link with will be able to read this content:
Sorry, a shareable link is not currently available for this article.
Provided by the Springer Nature SharedIt content-sharing initiative
- Binding affinity
- Distance matrix
- Donor-acceptor
- Hydrophobicity
- \(\pi \) -Stacking
- Deep learning
Journal of Cheminformatics
ISSN: 1758-2946
- Submission enquiries: [email protected]
New research aims to gauge brain power of the T. rex
In December 2022, Vanderbilt University neuroscientist Suzana Herculano-Houzel published a paper that caused an uproar in the dinosaur world.
After analyzing previous research on fossilized dinosaur brain cavities and the neuron counts of birds and other related living animals, Herculano-Houzel extrapolated that the fearsome Tyrannosaurus rex may have had more than 3 billion neurons — more than a baboon.
As a result, she argued, the predators could have been smart enough to make and use tools and to form social cultures akin to those seen in present-day primates.
The original “Jurassic Park” film spooked audiences by imagining velociraptors smart enough to open doors. Herculano-Houzel’s paper described T. rex as essentially wily enough to sharpen their own shivs. The bold claims made headlines, and almost immediately attracted scrutiny and skepticism from paleontologists.
In a paper published Monday in “The Anatomical Record,” an international team of paleontologists, neuroscientists and behavioral scientists argue that Herculano-Houzel’s assumptions about brain cavity size and corresponding neuron counts were off-base.
True T. rex intelligence, the scientists say, was probably much closer to that of modern-day crocodiles than primates — a perfectly respectable amount of smarts for a therapod to have.
“What needs to be emphasized is that reptiles are certainly not as dim-witted as is commonly believed,” said Kai Caspar, a biologist at Heinrich Heine University Düsseldorf and co-author of the paper. “So whereas there is no reason to assume that T. rex had primate-like habits, it was certainly a behaviorally sophisticated animal.”
Brain tissue doesn’t fossilize, and so researchers examine the shape and size of the brain cavity in fossilized dinosaur skulls to deduce what their brains may have been like.
In their analysis, the authors took issue with Herculano-Houzel’s assumption that dinosaur brains filled their skull cavities in a proportion similar to bird brains. Herculano-Houzel’s analysis posited that T. rex brains occupied most of their brain cavity, analogous to that of the modern-day ostrich.
But dinosaur brain cases more closely resemble those of modern-day reptiles like crocodiles, Caspar said. For animals like crocodiles, brain matter occupies only 30% to 50% of the brain cavity. Though brain size isn’t a perfect predictor of neuron numbers, a much smaller organ would have far fewer than the 3 billion neurons Herculano-Houzel projected.
“T. rex does come out as the biggest-brained big dinosaur we studied, and the biggest one not closely related to modern birds, but we couldn’t find the 2 to 3 billion neurons she found, even under our most generous estimates,” said co-author Thomas R. Holtz, Jr., a vertebrate paleontologist at University of Maryland, College Park.
What’s more, the research team argued, neuron counts aren’t an ideal indicator of an animal’s intelligence.
Giraffes have roughly the same number of neurons that crows and baboons have, Holtz pointed out, but they don’t use tools or display complex social behavior in the way those species do.
“Obviously in broad strokes you need more neurons to create more thoughts and memories and to solve problems,” Holtz said, but the sheer number of neurons an animal has can’t tell us how the animal will use them.
“Neuronal counts really are comparable to the storage capacity and active memory on your laptop, but cognition and behavior is more like the operating system,” he said. “Not all animal brains are running the same software.”
Based on CT scan reconstructions, the T. rex brain was probably “ a long tube that has very little in terms of the cortical expansion that you see in a primate or a modern bird,” said paleontologist Luis Chiappe, director of the Dinosaur Institute at the Natural History Museum of Los Angeles County.
“The argument that a T. Rex would have been as intelligent as a primate — no. That makes no sense to me,” said Chiappe, who was not involved in the study.
Like many paleontologists, Chiappe and his colleagues at the Dinosaur Institute were skeptical of Herculano-Houzel’s original conclusions. The new paper is more consistent with previous understandings of dinosaur anatomy and intelligence, he said.
“I am delighted to see that my simple study using solid data published by paleontologists opened the way for new studies,” Herculano-Houzel said in an email. “Readers should analyze the evidence and draw their own conclusions. That’s what science is about!”
When thinking about the inner life of T. rex, the most important takeaway is that reptilian intelligence is in fact more sophisticated than our species often assumes, scientists said.
“These animals engage in play, are capable of being trained, and even show excitement when they see their owners,” Holtz said. “What we found doesn’t mean that T. rex was a mindless automaton; but neither was it going to organize a Triceratops rodeo or pass down stories of the duckbill that was THAT BIG but got away.”
©2024 Los Angeles Times. Visit at latimes.com. Distributed by Tribune Content Agency, LLC.
Related Stories
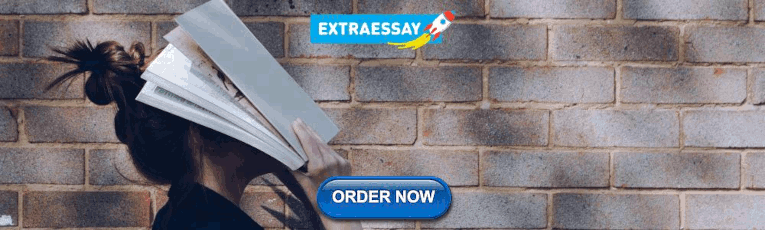
COMMENTS
power distance is the most frequently studied cultural value in organizational research (Erez, 2011). Power distance refers to the degree to which individuals, groups, or societies accept ...
1.1. High Power Distance Leads to Inefficient Communication. Power distance belief refers to the degree to which individuals, groups, or societies accept the unequal distribution of power, state, or wealth in society or organizations [].Power distance belief is originally a homogeneous national value []; it is also a dispositional trait at the individual level [8,9,10].
This research focuses on how power distance in meetings affects emotional labour, including whether leader-member exchange (LMX) serves as a moderator for this relationship. It is hypothesized that power distance in meetings would ... Paper type - Research Paper . REGULATING EMOTIONS IN REPSONSE TO POWER DISTANCE IN MEETINGS 3
The five dimensions of psychological power distance. To provide a more precise understanding of the effects of power distance, we have developed a new psychological power distance conceptualization based on five sub-dimensions: 1) Power, 2) conflict with authority figure, 3) hierarchy, 4) prestige, and 5) social distance ( Fig. 1 ).
SUBMIT PAPER. Journal of Management. Impact Factor: 13.5 / 5-Year Impact Factor: 17.3 . JOURNAL HOMEPAGE. SUBMIT PAPER. Close ... We conclude by identifying areas where additional organizational research on power distance is needed and, in doing so, provide an agenda for future research in this area. Our review highlights power distance as an ...
The aim of this paper is to renew interest and expand the relationship between organizational structure, societal culture, and its impact by focusing on distinct individual and organizational level outcomes. Drawing from the structural contingency theory, we extend previous research and propose that the alignment between power distance and structure variables of formalization and ...
Destructive leadership, a prevalent negative behavior in modern organizations, continues to captivate the interest of scholars and professionals due to its detrimental aftermath. Drawing from social psychological (culture) and conservation of resources theory, we explore the moderating impact of psychological power distance on the link between destructive leadership and emotional exhaustion ...
SUBMIT PAPER. Cross-Cultural Research. Impact Factor ... Research article. First published online October 12, 2011. Hofstede and Shane Revisited: The Role of Power Distance and Individualism in National-Level Innovation ... and interpreting changes in farming and rural society. Additional research activity focuses on research methods, farmer ...
1. Introduction. In this paper, we propose that what we call an active case study approach extends the repertoire of approaches to be used in the exploration of power distance in organizational life. Approaches utilized to date have followed largely from Hofstede's pioneering work [1] using a survey style of research.
Different forms of social distance exist (e.g., similarity and power). In construal level theory (CLT), social power may cause a sense of distance from others (Trope and Liberman 2010). Previous research has found that individuals in high power positions perceive themselves as more distant from others than those individuals with low power (Hogg ...
The mean score of school power distance was 2.26, indicating a low level of teachers' perceived power distance in schools. PLCs were positively related to teacher self-efficacy ( r = 0.64, p < 0.01) and work engagement ( r = 0.51, p < 0.01).
Psychological ownership can reduce the detrimental impacts of power distance on goal-setting participation and task crafting behavior in high-power distance cultures. Originality/Value of paper- This research addresses the need to study how goal setting affects employee task crafting. The paper suggests how organizations might encourage task ...
In this paper, we take a look at the behavioural implications in the organisations of power distance (status differences), which is one of the five cultural dimensions in Hofstede's framework. ... Three of his research papers have received nominations for the Best Paper Award at the Academy of Management Annual Meetings.
1. Introduction. In this paper, we propose that what we call an active case. study approach extends the repertoire of approaches to be. used in the exploration of power distance in organizational ...
expatriates' leadership in China through their power distance with the subordinates. Keywords: Expatriate, Leadership, Power distance, Motivation, Job satisfaction Paper Type: Research paper Introduction In the last decades of internationalization, expatriation has become one of the main topics in cross-cultural management studies. Those
Power Distance. Power distance stands for the extent to which people are content with the unequal distribution of resources, authority, power, and status in a society, organization, or group. ... 1995, 2001) are fundamental to cross-cultural analysis and appear in numerous research papers, many of which focus upon business-related topics, such ...
Hofstede's initial six key dimensions include power distance, uncertainty avoidance, individualism-collectivism, masculinity-femininity, and short vs. long-term orientation. Later, researchers added restraint vs. indulgence to this list. The extent to which individual countries share key dimensions depends on a number of factors, such as ...
The aim of this conceptual paper is to draw the attention of researchers, employers, and human resource policy makers to one of Hofstede's cultural dimensions, power distance, from a communication perspective. Power distance is believed to facilitate the...
It is crucial to research the topology and fault isolation methods applicable to large-scale offshore wind power all-DC systems in deep-sea areas. This paper proposes a novel series-connected all-DC system topology and presents corresponding fault isolation methods for internal faults in wind turbine units and faults in high-voltage DC ...
Although research has shown that the possession of power can change the power holder, the full extent of power's consequences on individuals' decision-making capabilities and social interactions within organizations is not fully understood. The goal of this paper is to review, synthesize, and critique the literature on power with a focus on ...
This paper focuses on the influence of the inducer's wake on the energy characteristics of downstream impellers, and conducts the following research: by adjusting the axial matching dimensions between the upstream inducer and the centrifugal impeller in the initial model, ten sets of axial distance models with matching dimensions of KD are ...
Recently, ZnO-based composites have been widely applied in the field of electric power. To meet the diverse application requirements, it is necessary to figure out the I-V characteristics of ZnO composites whose high-voltage and ground-voltage electrodes are arranged on the opposite sides with a certain horizontal distance. 30 vol%, 40 vol% and 50 vol% ZnO-based silicone rubber composites ...
Drawing upon social cognitive theory, we theorize teacher self-efficacy as a motivational explanation for the relationship between professional learning communities (PLCs) and teacher work engagement, and further posit school power distance as a boundary condition for this mechanism. With a sample of 3417 teachers in 61 schools in China, we tested the multilevel moderated mediation model, and ...
Conventional drug discovery, as noted by a recent study [], is a resource-intensive and time-consuming process that typically lasts for about 10 to 15 years and costs approximately 2.558 billion USD to bring each new drug successfully to the market.Computational approaches can expedite the drug discovery process by identifying drug molecules or ligands that have high binding affinities towards ...
Optical wireless power transmission (OWPT) has emerged as a promising technology for remote power applications due to its long-distance transmission, high directionality, and lack of electromagnetic interference. In practical applications, light-emitting diode (LED) based OWPT systems have advantages over Laser Diode in terms of easy commercialization and reduced safety issues.
Individualism-collectivism, uncertainty avoidance, power distance, masculinity-femininity, long-term orientation, and indulgence are the six dimensions of Hofstede's theory (Agodzo, 2015 ...
In a paper published Monday in "The Anatomical Record," an international team of paleontologists, neuroscientists and behavioral scientists argue that Herculano-Houzel's assumptions about ...