- Open access
- Published: 12 March 2020
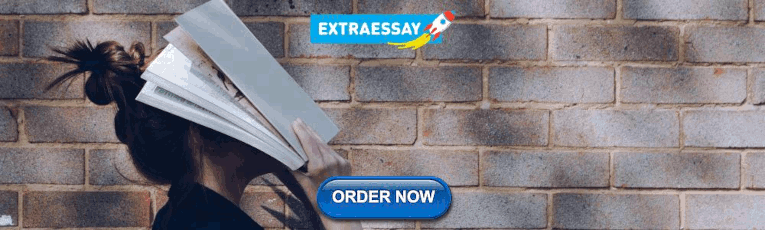
Current landscape and influence of big data on finance
- Md. Morshadul Hasan ORCID: orcid.org/0000-0001-9857-9265 1 ,
- József Popp ORCID: orcid.org/0000-0003-0848-4591 2 &
- Judit Oláh ORCID: orcid.org/0000-0003-2247-1711 2
Journal of Big Data volume 7 , Article number: 21 ( 2020 ) Cite this article
57k Accesses
86 Citations
32 Altmetric
Metrics details
Big data is one of the most recent business and technical issues in the age of technology. Hundreds of millions of events occur every day. The financial field is deeply involved in the calculation of big data events. As a result, hundreds of millions of financial transactions occur in the financial world each day. Therefore, financial practitioners and analysts consider it an emerging issue of the data management and analytics of different financial products and services. Also, big data has significant impacts on financial products and services. Therefore, identifying the financial issues where big data has a significant influence is also an important issue to explore with the influences. Based on these concepts, the objective of this paper was to show the current landscape of finance dealing with big data, and also to show how big data influences different financial sectors, more specifically, its impact on financial markets, financial institutions, and the relationship with internet finance, financial management, internet credit service companies, fraud detection, risk analysis, financial application management, and so on. The connection between big data and financial-related components will be revealed in an exploratory literature review of secondary data sources. Since big data in the financial field is an extremely new concept, future research directions will be pointed out at the end of this study.
Introduction
In the age of technological innovation, various types of data are available with the advance of information technologies, and data is seen as one of the most valuable commodities in managing automation systems [ 13 , 68 ]. In this sense, financial markets and technological evolution have become related to every human activity in the past few decades. Big data technology has become an integral part of the financial services industry and will continue to drive future innovation [ 12 ]. Financial innovations are also considered the fastest emerging issues in financial services. More specifically, they cover a variety of financial businesses such as online peer-to-peer lending, crowd-funding platforms, SME finance, wealth management and asset management platforms, trading management, crypto-currency, money/remittance transfer, mobile payments platforms, and so on. All of these services create thousands of pieces of data every day. Therefore, managing this data is also considered the most important factor in these services. Any damage to the data can cause serious problems for that specific financial industry. Nowadays, financial analysts use external and alternative data to make better investment decisions. In addition, financial industries use big data through different predictive analyses and monitor various spending patterns to develop large decision-making models. In this way, the industries can decide which financial products to offer [ 29 , 48 ]. Millions of data are transmitted among financial companies. That is why big data is receiving more attention in the financial services arena, where information affects important success and production factors. It has been playing increasingly important roles in consolidating our understanding of financial markets [ 71 ]. In any case, the financial industry is using trillions of pieces of data constantly in everyday decisions [ 22 ]. It plays an important role in changing the financial services sector, particularly in trade and investment, tax reform, fraud detection and investigation, risk analysis, and automation [ 37 ]. In addition, it has changed the financial industry by overcoming different challenges and gaining valuable insights to improve customer satisfaction and the overall banking experience [ 45 ]. Razin [ 65 ] pointed out that big data is also changing finance in five ways: creating transparency, analyzing risk, algorithmic trading, leveraging consumer data and transforming culture. Also, big data has a significant influence in economic analysis and economic modeling [ 16 , 21 ].
In this study, the views of different researchers, academics, and others related to big data and finance activities have been collected and analysed. This study not only attempts to test the existing theory but also to gain an in-depth understanding of the research from the qualitative data. However, research on big data in financial services is not as extensive as other financial areas. Few studies have precisely addressed big data in different financial research contexts. Though some studies have done these for some particular topics, the extensive views of big data in financial services haven’t done before with proper explanation of the influence and opportunity of big data on finance. Therefore, the need to identify the finance areas where big data has a significant influence is addressed. Also, the research related to big data and financial issues is extremely new. Therefore, this study presents the emerging issues of finance where big data has a significant influence, which has never been published yet by other researchers. That is why this research explores the influence of big data on financial services and this is the novelty of this study.
This paper seeks to explore the current landscape of big data in financial services. Particularly this study highlights the influence of big data on internet banking, financial markets, and financial service management. This study also presents a framework, which will facilitate the way how big data influence on finance. Some other services relating to finance are also highlighted here to specify the extended area of big data in financial services. These are the contribution of this study in the existing literatures.
This result of the study contribute to the existing literature which will help readers and researchers who are working on this topic and all target readers will obtain an integrated concept of big data in finance from this study. Furthermore, this research is also important for researchers who are working on this topic. The issue of big data has been explored here from different financing perspectives to provide a clear understanding for readers. Therefore, this study aims to outline the current state of big data technology in financial services. More importantly, an attempt has been made to focus on big data finance activities by concentrating on its impact on the finance sector from different dimensions.
Literature review
The concept of big data in finance has taken from the previous literatures, where some studies have been published by some good academic journals. At present, most of the areas of business are linked to big data. It has significant influence on various perspectives of business such as business process management, human resources management, R&D management [ 8 , 63 ], business analytics [ 19 , 26 , 42 , 59 , 63 ], B2B business process, marketing, and sales [ 30 , 39 , 53 , 58 ], industrial manufacturing process [ 7 , 15 , 40 ], enterprise’s operational performance measurement [ 20 , 69 , 81 ], policy making [ 2 ], supply chain management, decision, and performance [ 4 , 38 , 64 ], and so other business arenas.
Particularly, Rabhi et al. [ 63 ] mentioned big data as a significant factor of business process management& HR process to support the decision making. This study also talked about three sophisticated types of analytics techniques such as descriptive analytics, predictive analytics, and prescriptive analytics in order to improve the traditional data analytics process. Duan and Xiong [ 19 ], Grover and Kar [ 26 ], Ji et al. [ 42 ], and Pappas et al. [ 59 ] also explored the significance of big data in business analytics. Big data helps to solve business problems and data management through system infrastructure, which includes any technique to capture, store, transfer, and process data. Duan and Xiong [ 19 ] found that top-performing organizations use analytics as opposed to intuition almost five times more than do the lower performers. Business analytics and business strategy must be closely linked together to gain better analytics-driven insights. Grover and Kar [ 26 ] mentioned about firms, like Apple, Facebook, Google, Amazon, and eBay, that regularly use digitized transaction data such as storing the transaction time, purchase quantities, product prices, and customer credentials on regular basis to estimate the condition of their market for improving their business operations [ 61 , 76 ]. Holland et al. [ 39 ] showed the theoretical and empirical contributions of big data in business. This study inferred that B2B relationships from consumer search patterns, which used to evaluate and measure the online performance of competitors in the US airline market. Moreover, big data also help to foster B2B sales with customer data analytics. The use of customer’s big datasets significantly improve sales growth (monetary performance outcomes), and enhances the customer relationship performance (non-monetary performance outcomes) [ 30 ]. It also relates to market innovation with diversified opportunities.
Big data and its analytics and applications work as indicators of organizations’ ability to innovate to respond to market opportunities [ 78 ]. Also, big data impact on industrial manufacturing process to gain competitive advantages. After analyzing a case study of two company, Belhadi et al. [ 7 ] stated ‘NAPC aims for a qualitative leap with digital and big - data analytics to enable industrial teams to develop or even duplicate models of turnkey factories in Africa’. This study also identified an Overall framework of BDA capabilities in manufacturing process , and mentioned some values of Big Data Analytics for manufacturing process, such as enhancing transparency, improving performance, supporting decision-making and increasing knowledge. Also, Cui et al. [ 15 ] mentioned four most frequently big data applications (Monitoring, prediction, ICT framework, and data analytics) used in manufacturing. These are essential to realize the smart manufacturing process. Shamim et al. [ 69 ] argued that employee ambidexterity is important because employees’ big data management capabilities and ambidexterity are crucial for EMMNEs to manage the demands of global users. Also big data appeared as a frontier of the opportunity in improving firm performance. Yadegaridehkordi et al. [ 81 ] hypothesized that big data adoption has positive effect on firm performance. That study also mentioned that the policy makers, governments, and businesses can take well-informed decisions in adopting big data. According to Hofmann [ 38 ], velocity, variety, and volume significantly influence on supply chain management. For example, at first, velocity offers the biggest opportunity to intensification the efficiency of the processes in the supply chain. Next to this, variety supports different types of data volume in the supply chains is mostly new. After that, the volume is also a bigger interest for the multistage supply chains than to two-staged supply chains. Raman et al. [ 64 ] provided a new model, Supply Chain Operations Reference (SCOR), by incorporating SCM with big data. This model exposes the adoption of big data technology adds significant value as well as creates financial gain for the industry. This model is apt for the evaluation of the financial performance of supply chains. Also it works as a practical decision support means for examining competing decision alternatives along the chain as well as environmental assessment. Lamba and Singh [ 50 ] focused on decision making aspect of supply chain process and mentioned that data-driven decision-making is gaining noteworthy importance in managing logistics activities, process improvement, cost optimization, and better inventory management. Sahal et al. [ 67 ] and Xu and Duan [ 80 ] showed the relation of cyber physical systems and stream processing platform for Industry 4.0. Big data and IoT are considering as much influential forces for the era of Industry 4.0. These are also helping to achieve the two most important goals of Industry 4.0 applications (to increase productivity while reducing production cost & to maximum uptime throughout the production chain). Belhadi et al. [ 7 ] identified manufacturing process challenges, such as quality & process control (Q&PC), energy & environment efficiency (E&EE), proactive diagnosis and maintenance (PD&M), and safety & risk analysis (S&RA). Hofmann [ 38 ] also mentioned that one of the greatest challenges in the field of big data is to find new ways for storing and processing the different types of data. In addition, Duan and Xiong [ 19 ] mentioned that big data encompass more unstructured data such as text, graph, and time-series data compared to structured data for both data storage techniques and data analytics techniques. Zhao et al. [ 86 ] identified two major challenges for integrating both internal and external data for big data analytics. These are connecting datasets across the data sources, and selecting relevant data for analysis. Huang et al. [ 40 ] raised four challenges, first, the accuracy and applicability of the small data-based PSM paradigms is one kind of challenge; second, the traditional static-oriented PSM paradigms difficult to adapt to the dynamic changes of complex production systems; third, it is urgent to carry out research that focuses on forecasting-based PSM paradigms; and fourth, the determining the causal relationship quickly, economically and effectively is difficult, which affects safety predictions and safety decision-making.
The above discussion based on different area of business. Whatever, some studies (such as [ 6 , 11 , 14 , 22 , 23 , 41 , 45 , 54 , 68 , 71 , 73 , 75 , 83 , 85 ] focused different perspectives of financial services. Still, the contribution on this area is not expanded. Based on those researches, the current trends of big data in finance have specified in finding section.
Methodology
The purpose of this study is to locate academic research focusing on the related studies of big data and finance. To accomplish this research, secondary data sources were used to collect related data [ 31 , 32 , 34 ]. To collect secondary data, the study used the electronic database Scopus, the web of science, and Google scholar [ 33 ]. The keywords of this study are big data finance, finance and big data, big data and the stock market, big data in banking, big data management, and big data and FinTech. The search mainly focused only on academic and peer-reviewed journals, but in some cases, the researcher studied some articles on the Internet which were not published in academic and peer-reviewed journals. Sometimes, information from search engines helps understand the topic. The research area of big data has already been explored but data on big data in finance is not so extensive; this is why we did not limit the search to a certain time period because a time limitation may reduce the scope of the area of this research. Here, a structured and systematic data collection process was followed. Figure 1 presents the structured and systematic data collection process of this study. Certain renowned publishers, for example, Elsevier, Springer, Taylor & Francis, Wiley, Emerald, and Sage, among others, were prioritized when collecting the data for this study [ 35 , 36 ].
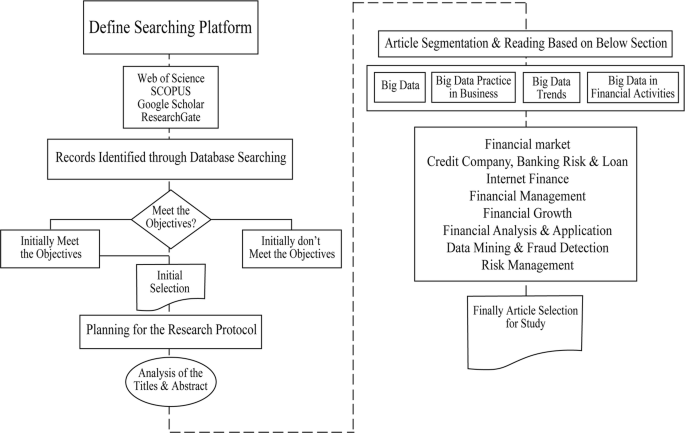
Systematic framework of the research structure. (Source: Author’s illustration)
The number of related articles collected from those databases is only 180. Following this, the collected articles were screened and a shortlist was created, featuring only 100 articles. Finally, data was used from 86 articles, of which 34 articles were directly related to ‘ Big data in Finance’ . Table 1 presents the list of those journals which will help to contribute to future research.
This literature study suggests that some major factors are related to big data and finance. In this context, it has been found that these specific factors also have a deep relationship with big data, such as financial markets, banking risk and lending, internet finance, financial management, financial growth, financial analysis and application, data mining and fraud detection, risk management, and other financial practices. Table 2 describes the focuses within the literature on the financial sector relating to big data.
Theoretical framework
After studying the literature, this study has found that big data is mostly linked to financial market, Internet finance. Credit Service Company, financial service management, financial applications and so forth. Mainly data relates with four types of financial industry such as financial market, online marketplace, lending company, and bank. These companies produce billions of data each day from their daily transaction, user account, data updating, accounts modification, and so other activities. Those companies process the billions of data and take the help to predict the preference of each consumer given his/her previous activities, and the level of credit risk for each user. Based on those data, financial institutions help in taking decisions [ 84 ]. However, different financial companies processing big data and getting help for verification and collection, credit risk prediction, and fraud detection. As the billions of data are producing from heterogeneous sources, missing data is a big concern as well as data quality and data reliability is also significant matter. Whatever, the concept of role of financial big data has taken form [ 71 ], where that study mention the sources of financial market information include the information assembled from stock market data (e.g., stock prices, stock trading volume, interest rates, and so on), social media (e.g., Facebook, twitter, newspapers, advertising, television, and so on). These data has significant roles in financial market such as predicting the market return, forecasting market volatility, valuing market position, identifying excess trading volume, analyzing the market risk, movement of the stock, option pricing, algorithmic trading, idiosyncratic volatility, and so on. Based on these discussions, a theoretical framework is illustrated in Fig. 2 .
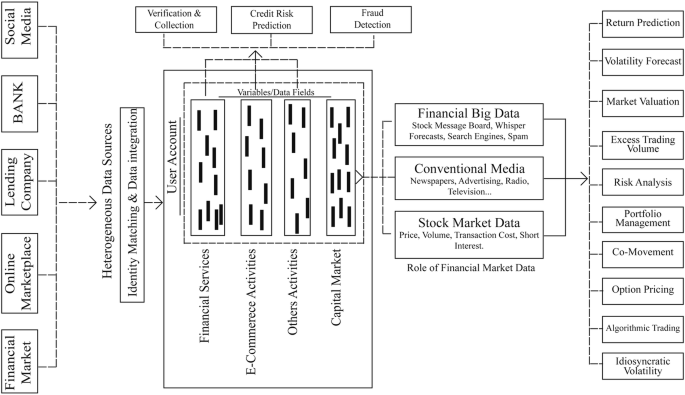
Theoretical framework of big data in financial services. Source: Author’s explanation. (This concept of this framework has been taken from Shen and Chen [ 71 ] and Zhang et al. [ 85 ])
Results and discussion
Massive data and increasingly sophisticated technologies are changing the way industries operate and compete. The financial world is also operating with these big data sets. It has not only influenced many fields of science and society, but has had an important impact on the finance industry [ 6 , 13 , 23 , 41 , 45 , 54 , 62 , 68 , 71 , 72 , 73 , 82 , 85 ]. After reviewing the literature, this study found some financial areas directly linked to big data, such as financial markets, internet credit service-companies and internet finance, financial management, analysis, and applications, credit banking risk analysis, risk management, and so forth. These areas are divided here into three groups; first, big data implications for financial markets and the financial growth of companies; second, big data implications for internet finance and value creation in internet credit-service companies; and third, big data in financial management, risk management, financial analysis, and applications. The discussion of big data in these specified financial areas is the contribution made by this study. Also, these are regarded as emerging landscape of big data in finance in this study.
Big data implications on financial markets
Financial markets always seek technological innovation for different activities, especially technological innovations that are always positively accepted, and which have a great impact on financial markets, and which have truly transforming effects on them. Shen and Chen [ 71 ] explain that the efficiency of financial markets is mostly attributed to the amount of information and its diffusion process. In this sense, social media undoubtedly plays a crucial role in financial markets. In this sense, it is considered one of the most influential forces acting on them. It generates millions of pieces of information every day in financial markets globally [ 9 ]. Big data mainly influences financial markets through return predictions, volatility forecasts, market valuations, excess trading volumes, risk analyses, portfolio management, index performance, co-movement, option pricing, idiosyncratic volatility, and algorithmic trading.
Shen and Chen [ 71 ] focus on the medium effect of big data on the financial market. This effect has two elements, effects on the efficient market hypothesis, and effects on market dynamics. The effect on the efficient market hypothesis refers to the number of times certain stock names are mentioned, the extracted sentiment from the content, and the search frequency of different keywords. Yahoo Finance is a common example of the effect on the efficient market hypothesis. On the other hand, the effect of financial big data usually relies on certain financial theories. Bollen et al. [ 9 ] emphasize that it also helps in sentiment analysis in financial markets, which represents the familiar machine learning technique with big datasets.
In another prospect, Begenau et al. [ 6 ] explore the assumption that big data strangely benefits big firms because of their extended economic activity and longer firm history. Even large firms typically produce more data compared to small firms. Big data also relates corporate finance in different ways such as attracting more financial analysis, as well as reducing equity uncertainty, cutting a firm’s cost of capital, and the costs of investors forecasting related to a financial decision. It cuts the cost of capital as investors process more data to enable large firms to grow larger. In pervasive and transformative information technology, financial markets can process more data, earnings statements, macro announcements, export market demand data, competitors’ performance metrics, and predictions of future returns. By predicting future returns, investors can reduce uncertainty about investment outcomes. In this sense Begenau et al. [ 6 ] stated that “More data processing lowers uncertainty, which reduces risk premia and the cost of capital, making investments more attractive.”.
Big data implications on internet finance and value creation at an internet credit service company
Technological advancements have caused a revolutionary transformation in financial services; especially the way banks and FinTech enterprises provide their services. Thinking about the influence of big data on the financial sector and its services, the process can be highlighted as a modern upgrade to financial access. In particular, online transactions, banking applications, and internet banking produce millions of pieces of data in a single day. Therefore, managing these millions of data is a subject to important [ 46 ]. Because managing these internet financing services has major impacts on financial markets [ 57 ]. Here, Zhang et al. [ 85 ] and Xie et al. [ 79 ] focus on data volume, service variety, information protection, and predictive correctness to show the relationship between information technologies and e-commerce and finance. Big data improves the efficiency of risk-based pricing and risk management while significantly alleviating information asymmetry problems. Also, it helps to verify and collect the data, predict credit risk status, and detect fraud [ 24 , 25 , 56 ]. Jin et al. [ 44 ], [ 47 ], Peji [ 60 ], and Hajizadeh et al. [ 28 ] identified that data mining technology plays vital roles in risk managing and fraud detection.
Big data also has a significant impact on Internet credit service companies. The first impact is to be able to assess more borrowers, even those without a good financial status. Big data also plays a vital role in credit rating bureaus. For example, the two public credit bureaus in China only have 0.3 billion individual’s ‘financial records. For other people, they at most have identity and demographic information (such as ID, name, age, marriage status, and education level), and it is not plausible to obtain reliable credit risk predictions using traditional models. This situation significantly limits financial institutions from approaching new consumers [ 85 ]. In this case, big data benefits by giving the opportunity for unlimited data access. In order to deal with credit risk effectively, financial systems take advantage of transparent information mechanisms. Big data can influence the market-based credit system of both enterprises and individuals by integrating the advantages of cloud computing and information technology. Cloud computing is another motivating factor; by using this cloud computing and big data services, mobile internet technology has opened a crystal price formation process in non-internet-based traditional financial transactions. Besides providing information to both the lenders and borrowers, it creates a positive relationship between the regulatory bodies of both banking and securities sectors. If a company has a large data set from different sources, it leads to multi-dimensional variables. However, managing these big datasets is difficult; sometimes if these datasets are not managed appropriately they may even seem a burden rather than an advantage. In this sense, the concept of data mining technology described in Hajizadeh et al. [ 28 ] to manage a huge volume of data regarding financial markets can contribute to reducing these difficulties. Managing the huge sets of data, the FinTech companies can process their information reliably, efficiently, effectively, and at a comparatively lower cost than the traditional financial institutions. They can analyze and provide services to more customers at greater depth. In addition, they can benefit from the analysis and prediction of systemic financial risks [ 82 ]. However, one critical issue is that individuals or small companies may not be able to afford to access big data directly. In this case, they can take advantage of big data through different information companies such as professional consulting companies, relevant government agencies, relevant private agencies, and so forth.
Big data in managing financial services
Big data is an emerging issue in almost all areas of business. Especially in finance, it effects with a variety of facility, such as financial management, risk management, financial analysis, and managing the data of financial applications. Big data is expressively changing the business models of financial companies and financial management. Also, it is considered a fascinating area nowadays. In this fascinating area, scientists and experts are trying to propose novel finance business models by considering big data methods, particularly, methods for risk control, financial market analysis, creating new finance sentiment indexes from social networks, and setting up information-based tools in different creative ways [ 58 ]. Sun et al. [ 73 ] mentioned the 4 V features of big data. These are volume (large data scale), variety (different data formats), velocity (real-time data streaming), and veracity (data uncertainty). These characteristics comprise different challenges for management, analytics, finance, and different applications. These challenges consist of organizing and managing the financial sector in effective and efficient ways, finding novel business models and handling traditional financial issues. The traditional financial issues are defined as high-frequency trading, credit risk, sentiments, financial analysis, financial regulation, risk management, and so on [ 73 ].
Every financial company receives billions of pieces of data every day but they do not use all of them in one moment. The data helps firms analyze their risk, which is considered the most influential factor affecting their profit maximization. Cerchiello and Giudici [ 11 ] specified systemic risk modelling as one of the most important areas of financial risk management. It mainly, emphasizes the estimation of the interrelationships between financial institutions. It also helps to control both the operational and integrated risk. Choi and Lambert [ 13 ] stated that ‘Big data are becoming more important for risk analysis’. It influences risk management by enhancing the quality of models, especially using the application and behavior scorecards. It also elaborates and interprets the risk analysis information comparatively faster than traditional systems. In addition, it also helps in detecting fraud [ 25 , 56 ] by reducing manual efforts by relating internal as well as external data in issues such as money laundering, credit card fraud, and so on. It also helps in enhancing computational efficiency, handling data storage, creating a visualization toolbox, and developing a sanity-check toolbox by enabling risk analysts to make initial data checks and develop a market-risk-specific remediation plan. Campbell-verduyn et al. [ 10 ] state “Finance is a technology of control, a point illustrated by the use of financial documents, data, models and measures in management, ownership claims, planning, accountability, and resource allocation” .
Moreover, big data techniques help to measure credit banking risk in home equity loans. Every day millions of financial operations lead to growth in companies’ databases. Managing these big databases sometimes creates problems. To resolve those problems, an automatic evaluation of credit status and risk measurements is necessary within a reasonable period of time [ 62 ]. Nowadays, bankers are facing problems in measuring the risks of credit and managing their financial databases. Big data practices are applied to manage financial databases in order to segment different risk groups. Also big data is very helpful for banks to comply with both the legal and the regulatory requirements in the credit risk and integrity risk domains [ 12 ]. A large dataset always needs to be managed with big data techniques to provide faster and unbiased estimators. Financial institutions benefit from improved and accurate credit risk evaluation. This helps to reduce the risks for financial companies in predicting a client’s loan repayment ability. In this way, more and more people get access to credit loans and at the same time banks reduce their credit risks [ 62 ].
Big data and other financial issues
One of the largest data platforms is the Internet, which is clearly playing ever-increasing roles in both the financial markets and personal finance. Information from the Internet always matters. Tumarkin and Whitelaw [ 77 ] examine the relationship between Internet message board activity and abnormal stock returns and trading volume. The study found that abnormal message activity of the stock of the Internet sector changes investors’ opinions in correlation with abnormal industry-adjusted returns, as well as causing trading volume to become abnormally high, since the Internet is the most common channel for information dissemination to investors. As a result, investors are always seeking information from the Internet and other sources. This information is mostly obtained by searching on different search engines. Drake et al. [ 18 ] found that abnormal information searches on search engines increase about two weeks prior to the earnings announcement. This study also suggests that information diffusion is not instantaneous with the release of the earnings information, but rather is spread over a period surrounding the announcement. One more significant correlation identified in this study is that information demand is positively associated with media attention and news, but negatively associated with investor distraction. Dimpfl and Jank [ 17 ] specified that search queries help predict future volatility, and their volatility will exceed the information contained in the lag volatility itself, and the volatility of the search volume will have an impact on volatility, which will last a considerable period of time. Jin et al. [ 43 ] identified that micro blogging also has a significant influence on changing the information environment, which in turn influences changes in stock market behavior.
Conclusions
Big data, machine learning, AI, and the cloud computing are fueling the finance industry toward digitalization. Large companies are embracing these technologies to implement digital transformation, bolster profit and loss, and meet consumer demand. While most companies are storing new and valuable data, the question is the implication and influence of these stored data in finance industry. In this prospect, every financial service is technologically innovative and treats data as blood circulation. Therefore, the findings of this study are reasonable to conclude that big data has revolutionized finance industry mainly with the real time stock market insights by changing trade and investments, fraud detection and prevention, and accurate risk analysis by machine learning process. These services are influencing by increasing revenue and customer satisfaction, speeding up manual processes, improving path to purchase, streamlined workflow and reliable system processing, analyze financial performance, and control growth. Despite these revolutionary service transmissions, several critical issues of big data exist in the finance world. Privacy and protection of data is one the biggest critical issue of big data services. As well as data quality of data and regulatory requirements also considered as significant issues. Even though every financial products and services are fully dependent on data and producing data in every second, still the research on big data and finance hasn’t reached its peak stage. In this perspectives, the discussion of this study reasonable to settle the future research directions. In future, varied research efforts will be important for financial data management systems to address technical challenges in order to realize the promised benefits of big data; in particular, the challenges of managing large data sets should be explored by researchers and financial analysts in order to drive transformative solutions. The common problem is that the larger the industry, the larger the database; therefore, it is important to emphasize the importance of managing large data sets for large companies compared to small firms. Managing such large data sets is expensive, and in some cases very difficult to access. In most cases, individuals or small companies do not have direct access to big data. Therefore, future research may focus on the creation of smooth access for small firms to large data sets. Also, the focus should be on exploring the impact of big data on financial products and services, and financial markets. Research is also essential into the security risks of big data in financial services. In addition, there is a need to expand the formal and integrated process of implementing big data strategies in financial institutions. In particular, the impact of big data on the stock market should continue to be explored. Finally, the emerging issues of big data in finance discussed in this study should be empirically emphasized in future research.
Availability of data and materials
Our data will be available on request.
Abbreviations
Small and medium enterprise
Research & Development
Human resource
Business to Business
Big data analytics
Supply chain management
Internet of things
Production safety management
Financial Technology
Andreasen MM, Christensen JHE, Rudebusch GD. Term structure analysis with big data: one-step estimation using bond prices. J Econom. 2019;212(1):26–46. https://doi.org/10.1016/j.jeconom.2019.04.019 .
Article MathSciNet MATH Google Scholar
Aragona B, Rosa R De. Big data in policy making. Math Popul Stud. 2018;00(00):1–7. https://doi.org/10.1080/08898480.2017.1418113 .
Article Google Scholar
Baak MA, van Hensbergen S. How big data can strengthen banking risk surveillance. Compact, 15–19. https://www.compact.nl/en/articles/how-big-data-can-strengthen-banking-risk-surveillance/ (2015).
Bag S, Wood LC, Xu L, Dhamija P, Kayikci Y. Big data analytics as an operational excellence approach to enhance sustainable supply chain performance. Resour Conserv Recycl. 2020;153:104559. https://doi.org/10.1016/j.resconrec.2019.104559 .
Barr MS, Koziara B, Flood MD, Hero A, Jagadish HV. Big data in finance: highlights from the big data in finance conference hosted at the University of Michigan October 27–28, 2016. SSRN Electron J. 2018. https://doi.org/10.2139/ssrn.3131226 .
Begenau J, Farboodi M, Veldkamp L. Big data in finance and the growth of large firms. J Monet Econ. 2018;97:71–87. https://doi.org/10.1016/j.jmoneco.2018.05.013 .
Belhadi A, Zkik K, Cherrafi A, Yusof SM, El fezazi S. Understanding big data analytics for manufacturing processes: insights from literature review and multiple case studies. Comput Ind Eng. 2019;137:106099. https://doi.org/10.1016/j.cie.2019.106099 .
Blackburn M, Alexander J, Legan JD, Klabjan D. Big data and the future of R&D management: the rise of big data and big data analytics will have significant implications for R&D and innovation management in the next decade. Res Technol Manag. 2017;60(5):43–51. https://doi.org/10.1080/08956308.2017.1348135 .
Bollen J, Mao H, Zeng X. Twitter mood predicts the stock market. J Comput Sci. 2011;2(1):1–8. https://doi.org/10.1016/j.jocs.2010.12.007 .
Campbell-verduyn M, Goguen M, Porter T. Big data and algorithmic governance: the case of financial practices. New Polit Econ. 2017;22(2):1–18. https://doi.org/10.1080/13563467.2016.1216533 .
Cerchiello P, Giudici P. Big data analysis for financial risk management. J Big Data. 2016;3(1):18. https://doi.org/10.1186/s40537-016-0053-4 .
Chen M. How the financial services industry is winning with big data. https://mapr.com/blog/how-financial-services-industry-is-winning-with-big-data/ (2018).
Choi T, Lambert JH. Advances in risk analysis with big data. Risk Anal 2017; 37(8). https://doi.org/10.1111/risa.12859 .
Corporation O. Big data in financial services and banking (Oracle Enterprise Architecture White Paper, Issue February). http://www.oracle.com/us/technologies/big-data/big-data-in-financial-services-wp-2415760.pdf (2015).
Cui Y, Kara S, Chan KC. Manufacturing big data ecosystem: a systematic literature review. Robot Comput Integr Manuf. 2020;62:101861. https://doi.org/10.1016/j.rcim.2019.101861 .
Diebold FX, Ghysels E, Mykland P, Zhang L. Big data in dynamic predictive econometric modeling. J Econ. 2019;212:1–3. https://doi.org/10.1016/j.jeconom.2019.04.017 .
Dimpfl T, Jank S. Can internet search queries help to predict stock market volatility? Eur Financ Manag. 2016;22(2):171–92. https://doi.org/10.1111/eufm.12058 .
Drake MS, Roulstone DT, Thornock JR. Investor information demand: evidence from Google Searches around earnings announcements. J Account Res. 2012;50(4):1001–40. https://doi.org/10.1111/j.1475-679X.2012.00443.x .
Duan L, Xiong Y. Big data analytics and business analytics. J Manag Anal. 2015;2(1):1–21. https://doi.org/10.1080/23270012.2015.1020891 .
Dubey R, Gunasekaran A, Childe SJ, Bryde DJ, Giannakis M, Foropon C, Roubaud D, Hazen BT. Big data analytics and artificial intelligence pathway to operational performance under the effects of entrepreneurial orientation and environmental dynamism: a study of manufacturing organisations. Int J Prod Econ. 2019. https://doi.org/10.1016/j.ijpe.2019.107599 .
Einav L, Levin J. The data revolution and economic analysis. Innov Policy Econ. 2014;14(1):1–24. https://doi.org/10.1086/674019 .
Ewen J. How big data is changing the finance industry. https://www.tamoco.com/blog/big-data-finance-industry-analytics/ (2019).
Fanning K, Grant R. Big data: implications for financial managers. J Corp Account Finance. 2013. https://doi.org/10.1002/jcaf.21872 .
Glancy FH, Yadav SB. A computational model for fi nancial reporting fraud detection. Decis Support Syst. 2011;50(3):595–601. https://doi.org/10.1016/j.dss.2010.08.010 .
Gray GL, Debreceny RS. A taxonomy to guide research on the application of data mining to fraud detection in financial statement audits. Int J Account Inform Sys. 2014. https://doi.org/10.1016/j.accinf.2014.05.006 .
Grover P, Kar AK. Big data analytics: a review on theoretical contributions and tools used in literature. Global J Flex Sys Manag. 2017;18(3):203–29. https://doi.org/10.1007/s40171-017-0159-3 .
Hagenau M, Liebmann M, Neumann D. Automated news reading: stock price prediction based on financial news using context-capturing features. Decis Support Syst. 2013;55(3):685–97. https://doi.org/10.1016/j.dss.2013.02.006 .
Hajizadeh E, Ardakani HD, Shahrabi J. Application of data mining techniques in stock markets: a survey. J Econ Int Finance. 2010;2(7):109–18.
Google Scholar
Hale G, Lopez JA. Monitoring banking system connectedness with big data. J Econ. 2019;212(1):203–20. https://doi.org/10.1016/j.jeconom.2019.04.027 .
Article MATH Google Scholar
Hallikainen H, Savimäki E, Laukkanen T. Fostering B2B sales with customer big data analytics. Ind Mark Manage. 2019. https://doi.org/10.1016/j.indmarman.2019.12.005 .
Hasan MM, Mahmud A. Risks management of ready-made garments industry in Bangladesh. Int Res J Bus Stud. 2017;10(1):1–13. https://doi.org/10.21632/irjbs.10.1.1-13 .
Hasan MM, Mahmud A, Islam MS. Deadly incidents in Bangladeshi apparel industry and illustrating the causes and effects of these incidents. J Finance Account. 2017;5(5):193–9. https://doi.org/10.11648/j.jfa.20170505.13 .
Hasan MM, Nekmahmud M, Yajuan L, Patwary MA. Green business value chain: a systematic review. Sustain Prod Consum. 2019;20:326–39. https://doi.org/10.1016/J.SPC.2019.08.003 .
Hasan MM, Parven T, Khan S, Mahmud A, Yajuan L. Trends and impacts of different barriers on Bangladeshi RMG Industry’s sustainable development. Int Res J Bus Stud. 2018;11(3):245–60. https://doi.org/10.21632/irjbs.11.3.245-260 .
Hasan MM, Yajuan L, Khan S. Promoting China’s inclusive finance through digital financial services. Global Bus Rev. 2020. https://doi.org/10.1177/0972150919895348 .
Hasan MM, Yajuan L, Mahmud A. Regional development of China’s inclusive finance through financial technology. SAGE Open. 2020. https://doi.org/10.1177/2158244019901252 .
Hill C. Where big data is taking the financial industry: trends in 2018. Big data made simple. https://bigdata-madesimple.com/where-big-data-is-taking-the-financial-industry-trends-in-2018/ (2018).
Hofmann E. Big data and supply chain decisions: the impact of volume, variety and velocity properties on the bullwhip effect. Int J Prod Res. 2017;55(17):5108–26. https://doi.org/10.1080/00207543.2015.1061222 .
Holland CP, Thornton SC, Naudé P. B2B analytics in the airline market: harnessing the power of consumer big data. Ind Mark Manage. 2019. https://doi.org/10.1016/j.indmarman.2019.11.002 .
Huang L, Wu C, Wang B. Challenges, opportunities and paradigm of applying big data to production safety management: from a theoretical perspective. J Clean Prod. 2019;231:592–9. https://doi.org/10.1016/j.jclepro.2019.05.245 .
Hussain K, Prieto E. Big data in the finance and insurance sectors. In: Cavanillas JM, Curry E, Wahlster W, editors. New horizons for a data-driven economy: a roadmap for usage and exploitation of big data in Europe. SpringerOpen: Cham; 2016. p. 2019–223. https://doi.org/10.1007/978-3-319-21569-3 .
Chapter Google Scholar
Ji W, Yin S, Wang L. A big data analytics based machining optimisation approach. J Intell Manuf. 2019;30(3):1483–95. https://doi.org/10.1007/s10845-018-1440-9 .
Jin X, Shen D, Zhang W. Has microblogging changed stock market behavior? Evidence from China. Physica A. 2016;452:151–6. https://doi.org/10.1016/j.physa.2016.02.052 .
Jin M, Wang Y, Zeng Y. Application of data mining technology in financial risk. Wireless Pers Commun. 2018. https://doi.org/10.1007/s11277-018-5402-5 .
Joshi N. How big data can transform the finance industry. BBN Times. https://www.bbntimes.com/en/technology/big-data-is-transforming-the-finance-industry .
Kh R. How big data can play an essential role in Fintech Evolutionno title. Smart Dala Collective. https://www.smartdatacollective.com/fintech-big-data-play-role-financial-evolution/ (2018).
Khadjeh Nassirtoussi A, Aghabozorgi S, Ying Wah T, Ngo DCL. Text mining for market prediction: a systematic review. Expert Syst Appl. 2014;41(16):7653–70. https://doi.org/10.1016/j.eswa.2014.06.009 .
Khan F. Big data in financial services. https://medium.com/datadriveninvestor/big-data-in-financial-services-d62fd130d1f6 (2018).
Kshetri N. Big data’s role in expanding access to financial services in China. Int J Inf Manage. 2016;36(3):297–308. https://doi.org/10.1016/j.ijinfomgt.2015.11.014 .
Lamba K, Singh SP. Big data in operations and supply chain management: current trends and future perspectives. Prod Plan Control. 2017;28(11–12):877–90. https://doi.org/10.1080/09537287.2017.1336787 .
Lien D. Business Finance and Enterprise Management in the era of big data: an introduction. North Am J Econ Finance. 2017;39:143–4. https://doi.org/10.1016/j.najef.2016.10.002 .
Liu S, Shao B, Gao Y, Hu S, Li Y, Zhou W. Game theoretic approach of a novel decision policy for customers based on big data. Electron Commer Res. 2018;18(2):225–40. https://doi.org/10.1007/s10660-017-9259-6 .
Liu Y, Soroka A, Han L, Jian J, Tang M. Cloud-based big data analytics for customer insight-driven design innovation in SMEs. Int J Inf Manage. 2019. https://doi.org/10.1016/j.ijinfomgt.2019.11.002 .
Mohamed TS. How big data does impact finance. Aksaray: Aksaray University; 2019.
Mulla J, Van Vliet B. FinQL: a query language for big data in finance. SSRN Electron J. 2015. https://doi.org/10.2139/ssrn.2685769 .
Ngai EWT, Hu Y, Wong YH, Chen Y, Sun X. The application of data mining techniques in financial fraud detection: a classification framework and an academic review of literature. Decis Support Syst. 2011;50(3):559–69. https://doi.org/10.1016/j.dss.2010.08.006 .
Niu S. Prevention and supervision of internet financial risk in the context of big data. Revista de La Facultad de Ingeniería. 2017;32(11):721–6.
Oracle. (2012) Financial services data management: big Data technology in financial services (Issue June).
Pappas IO, Mikalef P, Giannakos MN, Krogstie J, Lekakos G. Big data and business analytics ecosystems: paving the way towards digital transformation and sustainable societies. IseB. 2018;16(3):479–91. https://doi.org/10.1007/s10257-018-0377-z .
Peji M. Text mining for big data analysis in financial sector: a literature review. Sustainability. 2019. https://doi.org/10.3390/su11051277 .
Pousttchi K, Hufenbach Y. Engineering the value network of the customer interface and marketing in the data-Rich retail environment. Int J Electron Commer. 2015. https://doi.org/10.2753/JEC1086-4415180401 .
Pérez-Martín A, Pérez-Torregrosa A, Vaca M. Big Data techniques to measure credit banking risk in home equity loans. J Bus Res. 2018. https://doi.org/10.1016/j.jbusres.2018.02.008 .
Rabhi L, Falih N, Afraites A, Bouikhalene B. Big data approach and its applications in various fields: review. Proc Comput Sci. 2019;155(2018):599–605. https://doi.org/10.1016/j.procs.2019.08.084 .
Raman S, Patwa N, Niranjan I, Ranjan U, Moorthy K, Mehta A. Impact of big data on supply chain management. Int J Logist Res App. 2018;21(6):579–96. https://doi.org/10.1080/13675567.2018.1459523 .
Razin E. Big buzz about big data: 5 ways big data is changing finance. Forbes. https://www.forbes.com/sites/elyrazin/2015/12/03/big-buzz-about-big-data-5-ways-big-data-is-changing-finance/#1d055654376a (2019).
Retail banks and big data: big data as the key to better risk management. In: The Economist Intelligence Unit. https://eiuperspectives.economist.com/sites/default/files/RetailBanksandBigData.pdf (2014).
Sahal R, Breslin JG, Ali MI. Big data and stream processing platforms for Industry 4.0 requirements mapping for a predictive maintenance use case. J Manuf Sys. 2020;54:138–51. https://doi.org/10.1016/j.jmsy.2019.11.004 .
Schiff A, McCaffrey M. Redesigning digital finance for big data. SSRN Electron J. 2017. https://doi.org/10.2139/ssrn.2967122 .
Shamim S, Zeng J, Shafi Choksy U, Shariq SM. Connecting big data management capabilities with employee ambidexterity in Chinese multinational enterprises through the mediation of big data value creation at the employee level. Int Bus Rev. 2019. https://doi.org/10.1016/j.ibusrev.2019.101604 .
Shen Y (n.d.). Study on internet financial risk early warning based on big data analysis. 1919–1922.
Shen D, Chen S. Big data finance and financial markets. In: Computational social sciences (pp. 235–248). https://doi.org/10.1007/978-3-319-95465-3_12235 (2018).
Shen Y, Shen M, Chen Q. Measurement of the new economy in China: big data approach. China Econ J. 2016;9(3):304–16. https://doi.org/10.1080/17538963.2016.1211384 .
Sun Y, Shi Y, Zhang Z. Finance big data: management, analysis, and applications. Int J Electron Commer. 2019;23(1):9–11. https://doi.org/10.1080/10864415.2018.1512270 .
Sun W, Zhao Y, Sun L. Big data analytics for venture capital application: towards innovation performance improvement. Int J Inf Manage. 2018. https://doi.org/10.1016/j.ijinfomgt.2018.11.017 .
Tang Y, Xiong JJ, Luo Y, Zhang Y, Tang Y. How do the global stock markets Influence one another? Evidence from finance big data and granger causality directed network. Int J Electron Commer. 2019;23(1):85–109. https://doi.org/10.1080/10864415.2018.1512283 .
Thackeray R, Neiger BL, Hanson CL, Mckenzie JF. Enhancing promotional strategies within social marketing programs: use of Web 2.0 social media. Health Promot Pract. 2008. https://doi.org/10.1177/1524839908325335 .
Tumarkin R, Whitelaw RF. News or noise? Internet postings and stock prices. Financ Anal J. 2001;57(3):41–51. https://doi.org/10.2469/faj.v57.n3.2449 .
Wright LT, Robin R, Stone M, Aravopoulou DE. Adoption of big data technology for innovation in B2B marketing. J Business-to-Business Mark. 2019;00(00):1–13. https://doi.org/10.1080/1051712X.2019.1611082 .
Xie P, Zou C, Liu H. The fundamentals of internet finance and its policy implications in China. China Econ J. 2016;9(3):240–52. https://doi.org/10.1080/17538963.2016.1210366 .
Xu L Da, Duan L. Big data for cyber physical systems in industry 4.0: a survey. Enterp Inf Syst. 2019;13(2):148–69. https://doi.org/10.1080/17517575.2018.1442934 .
Article MathSciNet Google Scholar
Yadegaridehkordi E, Nilashi M, Shuib L, Nasir MH, Asadi M, Samad S, Awang NF. The impact of big data on firm performance in hotel industry. Electron Commer Res Appl. 2020;40:100921. https://doi.org/10.1016/j.elerap.2019.100921 .
Yang D, Chen P, Shi F, Wen C. Internet finance: its uncertain legal foundations and the role of big data in its development. Emerg Mark Finance Trade. 2017. https://doi.org/10.1080/1540496X.2016.1278528 .
Yu S, Guo S. Big data in finance. Big data concepts, theories, and application. Cham: Springer International Publishing; 2016. p. 391–412. https://doi.org/10.1007/978-3-319-27763-9 .
Yu ZH, Zhao CL, Guo SX(2017). Research on enterprise credit system under the background of big data. In: 3rd International conference on education and social development (ICESD 2017), ICESD, 903–906. https://doi.org/10.2991/wrarm-17.2017.77 .
Zhang S, Xiong W, Ni W, Li X. Value of big data to finance: observations on an internet credit Service Company in China. Financial Innov. 2015. https://doi.org/10.1186/s40854-015-0017-2 .
Zhao JL, Fan S, Hu D. Business challenges and research directions of management analytics in the big data era. J Manag Anal. 2014;1(3):169–74. https://doi.org/10.1080/23270012.2014.968643 .
Download references
Acknowledgements
All the authors are acknowledged to the reviewers who made significant comments on the review stage.
The project is funded under the program of the Minister of Science and Higher Education titled “Regional Initiative of Excellence in 2019-2022, project number 018/RID/2018/19, the amount of funding PLN 10 788 423 16”.
Author information
Authors and affiliations.
School of Finance, Nanjing Audit University, Nanjing, 211815, China
Md. Morshadul Hasan
WSB University, Cieplaka 1c, 41-300, Dabrowa Górnicza, Poland
József Popp & Judit Oláh
You can also search for this author in PubMed Google Scholar
Contributions
All the authors have the equal contribution on this paper. All authors read and approved the final manuscript.
Corresponding author
Correspondence to József Popp .
Ethics declarations
Competing interests.
There is no competing interests.
Additional information
Publisher's note.
Springer Nature remains neutral with regard to jurisdictional claims in published maps and institutional affiliations.
Rights and permissions
Open Access This article is licensed under a Creative Commons Attribution 4.0 International License, which permits use, sharing, adaptation, distribution and reproduction in any medium or format, as long as you give appropriate credit to the original author(s) and the source, provide a link to the Creative Commons licence, and indicate if changes were made. The images or other third party material in this article are included in the article's Creative Commons licence, unless indicated otherwise in a credit line to the material. If material is not included in the article's Creative Commons licence and your intended use is not permitted by statutory regulation or exceeds the permitted use, you will need to obtain permission directly from the copyright holder. To view a copy of this licence, visit http://creativecommons.org/licenses/by/4.0/ .
Reprints and permissions
About this article
Cite this article.
Hasan, M.M., Popp, J. & Oláh, J. Current landscape and influence of big data on finance. J Big Data 7 , 21 (2020). https://doi.org/10.1186/s40537-020-00291-z
Download citation
Received : 31 August 2019
Accepted : 17 February 2020
Published : 12 March 2020
DOI : https://doi.org/10.1186/s40537-020-00291-z
Share this article
Anyone you share the following link with will be able to read this content:
Sorry, a shareable link is not currently available for this article.
Provided by the Springer Nature SharedIt content-sharing initiative
- Big data finance
- Big data in financial services
- Big data in risk management
- Data management
- Search Menu
- Browse content in A - General Economics and Teaching
- Browse content in A1 - General Economics
- A11 - Role of Economics; Role of Economists; Market for Economists
- A13 - Relation of Economics to Social Values
- A14 - Sociology of Economics
- Browse content in C - Mathematical and Quantitative Methods
- Browse content in C0 - General
- C02 - Mathematical Methods
- Browse content in C1 - Econometric and Statistical Methods and Methodology: General
- C10 - General
- C11 - Bayesian Analysis: General
- C12 - Hypothesis Testing: General
- C13 - Estimation: General
- C14 - Semiparametric and Nonparametric Methods: General
- C15 - Statistical Simulation Methods: General
- C18 - Methodological Issues: General
- Browse content in C2 - Single Equation Models; Single Variables
- C21 - Cross-Sectional Models; Spatial Models; Treatment Effect Models; Quantile Regressions
- C22 - Time-Series Models; Dynamic Quantile Regressions; Dynamic Treatment Effect Models; Diffusion Processes
- C23 - Panel Data Models; Spatio-temporal Models
- Browse content in C3 - Multiple or Simultaneous Equation Models; Multiple Variables
- C32 - Time-Series Models; Dynamic Quantile Regressions; Dynamic Treatment Effect Models; Diffusion Processes; State Space Models
- C38 - Classification Methods; Cluster Analysis; Principal Components; Factor Models
- Browse content in C4 - Econometric and Statistical Methods: Special Topics
- C45 - Neural Networks and Related Topics
- Browse content in C5 - Econometric Modeling
- C50 - General
- C51 - Model Construction and Estimation
- C52 - Model Evaluation, Validation, and Selection
- C53 - Forecasting and Prediction Methods; Simulation Methods
- C55 - Large Data Sets: Modeling and Analysis
- C58 - Financial Econometrics
- Browse content in C6 - Mathematical Methods; Programming Models; Mathematical and Simulation Modeling
- C61 - Optimization Techniques; Programming Models; Dynamic Analysis
- C62 - Existence and Stability Conditions of Equilibrium
- C65 - Miscellaneous Mathematical Tools
- Browse content in C7 - Game Theory and Bargaining Theory
- C70 - General
- C72 - Noncooperative Games
- C73 - Stochastic and Dynamic Games; Evolutionary Games; Repeated Games
- C78 - Bargaining Theory; Matching Theory
- Browse content in C8 - Data Collection and Data Estimation Methodology; Computer Programs
- C81 - Methodology for Collecting, Estimating, and Organizing Microeconomic Data; Data Access
- Browse content in C9 - Design of Experiments
- C91 - Laboratory, Individual Behavior
- C92 - Laboratory, Group Behavior
- C93 - Field Experiments
- Browse content in D - Microeconomics
- Browse content in D0 - General
- D03 - Behavioral Microeconomics: Underlying Principles
- Browse content in D1 - Household Behavior and Family Economics
- D10 - General
- D11 - Consumer Economics: Theory
- D12 - Consumer Economics: Empirical Analysis
- D14 - Household Saving; Personal Finance
- D15 - Intertemporal Household Choice: Life Cycle Models and Saving
- D18 - Consumer Protection
- Browse content in D2 - Production and Organizations
- D20 - General
- D21 - Firm Behavior: Theory
- D22 - Firm Behavior: Empirical Analysis
- D23 - Organizational Behavior; Transaction Costs; Property Rights
- D24 - Production; Cost; Capital; Capital, Total Factor, and Multifactor Productivity; Capacity
- D25 - Intertemporal Firm Choice: Investment, Capacity, and Financing
- Browse content in D3 - Distribution
- D30 - General
- D31 - Personal Income, Wealth, and Their Distributions
- Browse content in D4 - Market Structure, Pricing, and Design
- D40 - General
- D43 - Oligopoly and Other Forms of Market Imperfection
- D44 - Auctions
- D47 - Market Design
- D49 - Other
- Browse content in D5 - General Equilibrium and Disequilibrium
- D50 - General
- D51 - Exchange and Production Economies
- D52 - Incomplete Markets
- D53 - Financial Markets
- Browse content in D6 - Welfare Economics
- D60 - General
- D61 - Allocative Efficiency; Cost-Benefit Analysis
- D62 - Externalities
- Browse content in D7 - Analysis of Collective Decision-Making
- D70 - General
- D71 - Social Choice; Clubs; Committees; Associations
- D72 - Political Processes: Rent-seeking, Lobbying, Elections, Legislatures, and Voting Behavior
- D73 - Bureaucracy; Administrative Processes in Public Organizations; Corruption
- D74 - Conflict; Conflict Resolution; Alliances; Revolutions
- D78 - Positive Analysis of Policy Formulation and Implementation
- Browse content in D8 - Information, Knowledge, and Uncertainty
- D80 - General
- D81 - Criteria for Decision-Making under Risk and Uncertainty
- D82 - Asymmetric and Private Information; Mechanism Design
- D83 - Search; Learning; Information and Knowledge; Communication; Belief; Unawareness
- D84 - Expectations; Speculations
- D85 - Network Formation and Analysis: Theory
- D86 - Economics of Contract: Theory
- D87 - Neuroeconomics
- Browse content in D9 - Micro-Based Behavioral Economics
- D90 - General
- D91 - Role and Effects of Psychological, Emotional, Social, and Cognitive Factors on Decision Making
- D92 - Intertemporal Firm Choice, Investment, Capacity, and Financing
- Browse content in E - Macroeconomics and Monetary Economics
- Browse content in E0 - General
- E00 - General
- E03 - Behavioral Macroeconomics
- Browse content in E1 - General Aggregative Models
- E17 - Forecasting and Simulation: Models and Applications
- Browse content in E2 - Consumption, Saving, Production, Investment, Labor Markets, and Informal Economy
- E20 - General
- E21 - Consumption; Saving; Wealth
- E22 - Investment; Capital; Intangible Capital; Capacity
- E23 - Production
- E24 - Employment; Unemployment; Wages; Intergenerational Income Distribution; Aggregate Human Capital; Aggregate Labor Productivity
- Browse content in E3 - Prices, Business Fluctuations, and Cycles
- E30 - General
- E31 - Price Level; Inflation; Deflation
- E32 - Business Fluctuations; Cycles
- E37 - Forecasting and Simulation: Models and Applications
- Browse content in E4 - Money and Interest Rates
- E40 - General
- E41 - Demand for Money
- E42 - Monetary Systems; Standards; Regimes; Government and the Monetary System; Payment Systems
- E43 - Interest Rates: Determination, Term Structure, and Effects
- E44 - Financial Markets and the Macroeconomy
- E47 - Forecasting and Simulation: Models and Applications
- Browse content in E5 - Monetary Policy, Central Banking, and the Supply of Money and Credit
- E50 - General
- E51 - Money Supply; Credit; Money Multipliers
- E52 - Monetary Policy
- E58 - Central Banks and Their Policies
- Browse content in E6 - Macroeconomic Policy, Macroeconomic Aspects of Public Finance, and General Outlook
- E60 - General
- E61 - Policy Objectives; Policy Designs and Consistency; Policy Coordination
- E62 - Fiscal Policy
- E63 - Comparative or Joint Analysis of Fiscal and Monetary Policy; Stabilization; Treasury Policy
- E64 - Incomes Policy; Price Policy
- E65 - Studies of Particular Policy Episodes
- E66 - General Outlook and Conditions
- Browse content in E7 - Macro-Based Behavioral Economics
- E71 - Role and Effects of Psychological, Emotional, Social, and Cognitive Factors on the Macro Economy
- Browse content in F - International Economics
- Browse content in F0 - General
- F02 - International Economic Order and Integration
- Browse content in F1 - Trade
- F14 - Empirical Studies of Trade
- Browse content in F2 - International Factor Movements and International Business
- F21 - International Investment; Long-Term Capital Movements
- F22 - International Migration
- F23 - Multinational Firms; International Business
- Browse content in F3 - International Finance
- F30 - General
- F31 - Foreign Exchange
- F32 - Current Account Adjustment; Short-Term Capital Movements
- F33 - International Monetary Arrangements and Institutions
- F34 - International Lending and Debt Problems
- F36 - Financial Aspects of Economic Integration
- F37 - International Finance Forecasting and Simulation: Models and Applications
- F38 - International Financial Policy: Financial Transactions Tax; Capital Controls
- Browse content in F4 - Macroeconomic Aspects of International Trade and Finance
- F40 - General
- F41 - Open Economy Macroeconomics
- F42 - International Policy Coordination and Transmission
- F43 - Economic Growth of Open Economies
- F44 - International Business Cycles
- F47 - Forecasting and Simulation: Models and Applications
- Browse content in F5 - International Relations, National Security, and International Political Economy
- F51 - International Conflicts; Negotiations; Sanctions
- Browse content in F6 - Economic Impacts of Globalization
- F63 - Economic Development
- F65 - Finance
- Browse content in G - Financial Economics
- Browse content in G0 - General
- G00 - General
- G01 - Financial Crises
- G02 - Behavioral Finance: Underlying Principles
- Browse content in G1 - General Financial Markets
- G10 - General
- G11 - Portfolio Choice; Investment Decisions
- G12 - Asset Pricing; Trading volume; Bond Interest Rates
- G13 - Contingent Pricing; Futures Pricing
- G14 - Information and Market Efficiency; Event Studies; Insider Trading
- G15 - International Financial Markets
- G17 - Financial Forecasting and Simulation
- G18 - Government Policy and Regulation
- G19 - Other
- Browse content in G2 - Financial Institutions and Services
- G20 - General
- G21 - Banks; Depository Institutions; Micro Finance Institutions; Mortgages
- G22 - Insurance; Insurance Companies; Actuarial Studies
- G23 - Non-bank Financial Institutions; Financial Instruments; Institutional Investors
- G24 - Investment Banking; Venture Capital; Brokerage; Ratings and Ratings Agencies
- G28 - Government Policy and Regulation
- G29 - Other
- Browse content in G3 - Corporate Finance and Governance
- G30 - General
- G31 - Capital Budgeting; Fixed Investment and Inventory Studies; Capacity
- G32 - Financing Policy; Financial Risk and Risk Management; Capital and Ownership Structure; Value of Firms; Goodwill
- G33 - Bankruptcy; Liquidation
- G34 - Mergers; Acquisitions; Restructuring; Corporate Governance
- G35 - Payout Policy
- G38 - Government Policy and Regulation
- G39 - Other
- Browse content in G4 - Behavioral Finance
- G40 - General
- G41 - Role and Effects of Psychological, Emotional, Social, and Cognitive Factors on Decision Making in Financial Markets
- Browse content in G5 - Household Finance
- G50 - General
- G51 - Household Saving, Borrowing, Debt, and Wealth
- G52 - Insurance
- G53 - Financial Literacy
- Browse content in H - Public Economics
- H0 - General
- Browse content in H1 - Structure and Scope of Government
- H11 - Structure, Scope, and Performance of Government
- H19 - Other
- Browse content in H2 - Taxation, Subsidies, and Revenue
- H22 - Incidence
- H24 - Personal Income and Other Nonbusiness Taxes and Subsidies; includes inheritance and gift taxes
- H25 - Business Taxes and Subsidies
- H26 - Tax Evasion and Avoidance
- Browse content in H3 - Fiscal Policies and Behavior of Economic Agents
- H31 - Household
- Browse content in H4 - Publicly Provided Goods
- H40 - General
- H41 - Public Goods
- Browse content in H5 - National Government Expenditures and Related Policies
- H50 - General
- H52 - Government Expenditures and Education
- H53 - Government Expenditures and Welfare Programs
- H54 - Infrastructures; Other Public Investment and Capital Stock
- H55 - Social Security and Public Pensions
- H56 - National Security and War
- H57 - Procurement
- Browse content in H6 - National Budget, Deficit, and Debt
- H63 - Debt; Debt Management; Sovereign Debt
- Browse content in H7 - State and Local Government; Intergovernmental Relations
- H70 - General
- H72 - State and Local Budget and Expenditures
- H74 - State and Local Borrowing
- H75 - State and Local Government: Health; Education; Welfare; Public Pensions
- Browse content in H8 - Miscellaneous Issues
- H81 - Governmental Loans; Loan Guarantees; Credits; Grants; Bailouts
- Browse content in I - Health, Education, and Welfare
- Browse content in I1 - Health
- I11 - Analysis of Health Care Markets
- I12 - Health Behavior
- I13 - Health Insurance, Public and Private
- I18 - Government Policy; Regulation; Public Health
- Browse content in I2 - Education and Research Institutions
- I22 - Educational Finance; Financial Aid
- I23 - Higher Education; Research Institutions
- I28 - Government Policy
- Browse content in I3 - Welfare, Well-Being, and Poverty
- I30 - General
- I38 - Government Policy; Provision and Effects of Welfare Programs
- Browse content in J - Labor and Demographic Economics
- Browse content in J0 - General
- J00 - General
- Browse content in J1 - Demographic Economics
- J11 - Demographic Trends, Macroeconomic Effects, and Forecasts
- J12 - Marriage; Marital Dissolution; Family Structure; Domestic Abuse
- J13 - Fertility; Family Planning; Child Care; Children; Youth
- J15 - Economics of Minorities, Races, Indigenous Peoples, and Immigrants; Non-labor Discrimination
- J16 - Economics of Gender; Non-labor Discrimination
- J18 - Public Policy
- Browse content in J2 - Demand and Supply of Labor
- J20 - General
- J21 - Labor Force and Employment, Size, and Structure
- J22 - Time Allocation and Labor Supply
- J23 - Labor Demand
- J24 - Human Capital; Skills; Occupational Choice; Labor Productivity
- J26 - Retirement; Retirement Policies
- J28 - Safety; Job Satisfaction; Related Public Policy
- Browse content in J3 - Wages, Compensation, and Labor Costs
- J30 - General
- J31 - Wage Level and Structure; Wage Differentials
- J32 - Nonwage Labor Costs and Benefits; Retirement Plans; Private Pensions
- J33 - Compensation Packages; Payment Methods
- J38 - Public Policy
- Browse content in J4 - Particular Labor Markets
- J41 - Labor Contracts
- J44 - Professional Labor Markets; Occupational Licensing
- J45 - Public Sector Labor Markets
- J46 - Informal Labor Markets
- J49 - Other
- Browse content in J5 - Labor-Management Relations, Trade Unions, and Collective Bargaining
- J51 - Trade Unions: Objectives, Structure, and Effects
- J52 - Dispute Resolution: Strikes, Arbitration, and Mediation; Collective Bargaining
- Browse content in J6 - Mobility, Unemployment, Vacancies, and Immigrant Workers
- J61 - Geographic Labor Mobility; Immigrant Workers
- J62 - Job, Occupational, and Intergenerational Mobility
- J63 - Turnover; Vacancies; Layoffs
- J64 - Unemployment: Models, Duration, Incidence, and Job Search
- J65 - Unemployment Insurance; Severance Pay; Plant Closings
- J68 - Public Policy
- Browse content in J7 - Labor Discrimination
- J71 - Discrimination
- Browse content in J8 - Labor Standards: National and International
- J88 - Public Policy
- Browse content in K - Law and Economics
- Browse content in K1 - Basic Areas of Law
- K12 - Contract Law
- Browse content in K2 - Regulation and Business Law
- K22 - Business and Securities Law
- K23 - Regulated Industries and Administrative Law
- Browse content in K3 - Other Substantive Areas of Law
- K31 - Labor Law
- K32 - Environmental, Health, and Safety Law
- K34 - Tax Law
- K35 - Personal Bankruptcy Law
- Browse content in K4 - Legal Procedure, the Legal System, and Illegal Behavior
- K42 - Illegal Behavior and the Enforcement of Law
- Browse content in L - Industrial Organization
- Browse content in L1 - Market Structure, Firm Strategy, and Market Performance
- L10 - General
- L11 - Production, Pricing, and Market Structure; Size Distribution of Firms
- L13 - Oligopoly and Other Imperfect Markets
- L14 - Transactional Relationships; Contracts and Reputation; Networks
- L15 - Information and Product Quality; Standardization and Compatibility
- Browse content in L2 - Firm Objectives, Organization, and Behavior
- L21 - Business Objectives of the Firm
- L22 - Firm Organization and Market Structure
- L23 - Organization of Production
- L24 - Contracting Out; Joint Ventures; Technology Licensing
- L25 - Firm Performance: Size, Diversification, and Scope
- L26 - Entrepreneurship
- L29 - Other
- Browse content in L3 - Nonprofit Organizations and Public Enterprise
- L33 - Comparison of Public and Private Enterprises and Nonprofit Institutions; Privatization; Contracting Out
- Browse content in L4 - Antitrust Issues and Policies
- L43 - Legal Monopolies and Regulation or Deregulation
- L44 - Antitrust Policy and Public Enterprises, Nonprofit Institutions, and Professional Organizations
- Browse content in L5 - Regulation and Industrial Policy
- L51 - Economics of Regulation
- Browse content in L6 - Industry Studies: Manufacturing
- L66 - Food; Beverages; Cosmetics; Tobacco; Wine and Spirits
- Browse content in L8 - Industry Studies: Services
- L81 - Retail and Wholesale Trade; e-Commerce
- L85 - Real Estate Services
- L86 - Information and Internet Services; Computer Software
- Browse content in L9 - Industry Studies: Transportation and Utilities
- L92 - Railroads and Other Surface Transportation
- L94 - Electric Utilities
- Browse content in M - Business Administration and Business Economics; Marketing; Accounting; Personnel Economics
- Browse content in M0 - General
- M00 - General
- Browse content in M1 - Business Administration
- M12 - Personnel Management; Executives; Executive Compensation
- M13 - New Firms; Startups
- M14 - Corporate Culture; Social Responsibility
- M16 - International Business Administration
- Browse content in M2 - Business Economics
- M20 - General
- M21 - Business Economics
- Browse content in M3 - Marketing and Advertising
- M30 - General
- M31 - Marketing
- M37 - Advertising
- Browse content in M4 - Accounting and Auditing
- M40 - General
- M41 - Accounting
- M42 - Auditing
- M48 - Government Policy and Regulation
- Browse content in M5 - Personnel Economics
- M51 - Firm Employment Decisions; Promotions
- M52 - Compensation and Compensation Methods and Their Effects
- M54 - Labor Management
- Browse content in N - Economic History
- Browse content in N1 - Macroeconomics and Monetary Economics; Industrial Structure; Growth; Fluctuations
- N10 - General, International, or Comparative
- N12 - U.S.; Canada: 1913-
- Browse content in N2 - Financial Markets and Institutions
- N20 - General, International, or Comparative
- N21 - U.S.; Canada: Pre-1913
- N22 - U.S.; Canada: 1913-
- N23 - Europe: Pre-1913
- N24 - Europe: 1913-
- N25 - Asia including Middle East
- N27 - Africa; Oceania
- Browse content in N3 - Labor and Consumers, Demography, Education, Health, Welfare, Income, Wealth, Religion, and Philanthropy
- N32 - U.S.; Canada: 1913-
- Browse content in N4 - Government, War, Law, International Relations, and Regulation
- N43 - Europe: Pre-1913
- Browse content in N7 - Transport, Trade, Energy, Technology, and Other Services
- N71 - U.S.; Canada: Pre-1913
- Browse content in N8 - Micro-Business History
- N80 - General, International, or Comparative
- N82 - U.S.; Canada: 1913-
- Browse content in O - Economic Development, Innovation, Technological Change, and Growth
- Browse content in O1 - Economic Development
- O11 - Macroeconomic Analyses of Economic Development
- O12 - Microeconomic Analyses of Economic Development
- O13 - Agriculture; Natural Resources; Energy; Environment; Other Primary Products
- O16 - Financial Markets; Saving and Capital Investment; Corporate Finance and Governance
- O17 - Formal and Informal Sectors; Shadow Economy; Institutional Arrangements
- Browse content in O2 - Development Planning and Policy
- O23 - Fiscal and Monetary Policy in Development
- Browse content in O3 - Innovation; Research and Development; Technological Change; Intellectual Property Rights
- O30 - General
- O31 - Innovation and Invention: Processes and Incentives
- O32 - Management of Technological Innovation and R&D
- O33 - Technological Change: Choices and Consequences; Diffusion Processes
- O34 - Intellectual Property and Intellectual Capital
- O35 - Social Innovation
- O38 - Government Policy
- Browse content in O4 - Economic Growth and Aggregate Productivity
- O40 - General
- O43 - Institutions and Growth
- Browse content in O5 - Economywide Country Studies
- O53 - Asia including Middle East
- Browse content in P - Economic Systems
- Browse content in P1 - Capitalist Systems
- P16 - Political Economy
- P18 - Energy: Environment
- Browse content in P2 - Socialist Systems and Transitional Economies
- P26 - Political Economy; Property Rights
- Browse content in P3 - Socialist Institutions and Their Transitions
- P31 - Socialist Enterprises and Their Transitions
- P34 - Financial Economics
- P39 - Other
- Browse content in P4 - Other Economic Systems
- P43 - Public Economics; Financial Economics
- P48 - Political Economy; Legal Institutions; Property Rights; Natural Resources; Energy; Environment; Regional Studies
- Browse content in Q - Agricultural and Natural Resource Economics; Environmental and Ecological Economics
- Browse content in Q0 - General
- Q02 - Commodity Markets
- Browse content in Q3 - Nonrenewable Resources and Conservation
- Q31 - Demand and Supply; Prices
- Q32 - Exhaustible Resources and Economic Development
- Browse content in Q4 - Energy
- Q40 - General
- Q41 - Demand and Supply; Prices
- Q42 - Alternative Energy Sources
- Q43 - Energy and the Macroeconomy
- Browse content in Q5 - Environmental Economics
- Q50 - General
- Q51 - Valuation of Environmental Effects
- Q53 - Air Pollution; Water Pollution; Noise; Hazardous Waste; Solid Waste; Recycling
- Q54 - Climate; Natural Disasters; Global Warming
- Q56 - Environment and Development; Environment and Trade; Sustainability; Environmental Accounts and Accounting; Environmental Equity; Population Growth
- Browse content in R - Urban, Rural, Regional, Real Estate, and Transportation Economics
- Browse content in R0 - General
- R00 - General
- Browse content in R1 - General Regional Economics
- R10 - General
- R11 - Regional Economic Activity: Growth, Development, Environmental Issues, and Changes
- R12 - Size and Spatial Distributions of Regional Economic Activity
- Browse content in R2 - Household Analysis
- R20 - General
- R21 - Housing Demand
- R23 - Regional Migration; Regional Labor Markets; Population; Neighborhood Characteristics
- Browse content in R3 - Real Estate Markets, Spatial Production Analysis, and Firm Location
- R30 - General
- R31 - Housing Supply and Markets
- R32 - Other Spatial Production and Pricing Analysis
- R33 - Nonagricultural and Nonresidential Real Estate Markets
- R38 - Government Policy
- Browse content in R4 - Transportation Economics
- R41 - Transportation: Demand, Supply, and Congestion; Travel Time; Safety and Accidents; Transportation Noise
- Browse content in R5 - Regional Government Analysis
- R51 - Finance in Urban and Rural Economies
- Browse content in Z - Other Special Topics
- Browse content in Z1 - Cultural Economics; Economic Sociology; Economic Anthropology
- Z11 - Economics of the Arts and Literature
- Z13 - Economic Sociology; Economic Anthropology; Social and Economic Stratification
- Advance articles
- Editor's Choice
- Author Guidelines
- Submission Site
- Open Access
- About The Review of Financial Studies
- Editorial Board
- Advertising and Corporate Services
- Journals Career Network
- Self-Archiving Policy
- Dispatch Dates
- Terms and Conditions
- Journals on Oxford Academic
- Books on Oxford Academic
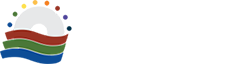
Article Contents
1. the “big data” revolution, 2. what is big data in finance research, 3. what is included in this special issue, 4. where does big data research go from here, acknowledgement, big data in finance.
- Article contents
- Figures & tables
- Supplementary Data
Itay Goldstein, Chester S Spatt, Mao Ye, Big Data in Finance, The Review of Financial Studies , Volume 34, Issue 7, July 2021, Pages 3213–3225, https://doi.org/10.1093/rfs/hhab038
- Permissions Icon Permissions
Big data is revolutionizing the finance industry and has the potential to significantly shape future research in finance. This special issue contains papers following the 2019 NBER-RFS Conference on Big Data. In this introduction to the special issue, we define the “big data” phenomenon as a combination of three features: large size, high dimension, and complex structure. Using the papers in the special issue, we discuss how new research builds on these features to push the frontier on fundamental questions across areas in finance—including corporate finance, market microstructure, and asset pricing. Finally, we offer some thoughts for future research directions.
The digital age has created mountains of data that continue to grow exponentially. The International Data Corporation estimates that the world generates more data every two days than all of humanity generated from the dawn of time to the year 2003. This “big data” revolution is reshaping the financial industry. As the Wall Street Journal wrote, “Today, the ultimate Wall Street status symbol is a trading floor comprising Carnegie Mellon Ph.D.s, not Wharton M.B.A.s.” 1 This industry transition has already started to affect the way we teach students. Along with the drop in the number of Master of Business Administration (MBA) programs, as well as the decline in applications and enrollment in MBA programs, 2 we see a surge of new programs such as Master of Business Analytics (also MBA).
The impact of big data on academic research in finance is also starting to reveal itself, but with it many questions emerge. The classical definition of big data as encompassing three V’s (volume, velocity, and variety) has a strong relation to engineering and computer science, but it does not fully reflect the opportunities and challenges that big data poses to research and practice in finance. What does big data in finance actually mean? How can financial economists benefit from the big data revolution? Does big data open new research topics for financial economists or allow us to answer traditional questions in novel and more revealing ways? Is this really a revolution for finance research or just a continuation of a gradual change? After all, large datasets always have been a feature of research in finance.
In October 2018, the National Science Foundation (NSF) provided a joint grant to the National Bureau of Economic Research (NBER) and the National Center for Supercomputing Application (NCSA) at the University of Illinois at Urbana–Champaign that aimed to explore answers to these questions. Part of the grant is dedicated to education and outreach and support a series of NBER conferences to explore the future of big data research in finance. The summer conferences, organized by Toni Whited and Mao Ye, focus on tutorial sessions on big data techniques and presentations of early ideas on big data. The winter conferences, organized by Itay Goldstein, Chester Spatt, and Mao Ye, focus on completed papers using big data and related methodologies.
This special issue of the Review of Financial Studies (RFS) on big data in finance includes four papers from the first NBER-RFS Winter Conference on Big Data held on March 8, 2019, and two other papers that are closely related to this theme. The RFS has the tradition of encouraging scholars to pursue risky projects that have the potential to push the frontiers of research in finance. The NBER-RFS Conferences on Big Data and this special issue reflect the RFS’s efforts to encourage the use of big data in finance studies and provide a natural complement to the RFS FinTech initiative that was featured in the May 2019 special issue (see Goldstein, Jiang, and Karolyi 2019 for an introduction).
In this introduction, we try to define what “big data” encompasses in the context of finance research. We then review the six papers included in the special issue, discussing how they are related to each other and to the general theme. Finally, we provide some thoughts for future research directions.
It is fairly clear that a definition of big data in finance research should be different from ones that are used in engineering and statistics. Researchers in these disciplines focus on providing facilities and tools to capture, curate, manage, and process data. Financial economists, on the other hand, focus on applying these tools to address interesting economic questions. While it is risky to give a broad-based definition at this stage, we think it is important to try. The definition may be imprecise or incomplete, but it will provide a starting point for future iterations and corrections.
We thus propose three properties that together can potentially define big data in finance research: large size, high dimension, and complex structure. This definition combines the characteristics of the data with possible new research questions that cannot be addressed using “small data.” We used this definition in our call for papers for the 2019 NBER-RFS Winter Conference. “Big data” papers can feature different combinations of these three properties. We now elaborate on what each of these properties captures.
Large size: As the term “big data” suggests, it would be impossible to avoid a reference to size. This feature means that data are large in an absolute or relative sense. A natural example for absolute size is transaction-level market microstructure data. 3 In a relative sense, big data is defined relative to the best existing “small data.” Many datasets are small simply because they are a subset of a larger dataset. By subsampling or aggregating observations into categories or taking snapshots of activities in time series, large datasets are made smaller. Using the underlying larger dataset is important if it overcomes the sample selection bias in the small dataset, or if it captures important economic activities not depicted in the small dataset.
High dimension: “Big data” is not just about size. The second feature means that the data have many variables relative to the sample size. Machine learning, which is often thought of as a hallmark of big data research, is a common solution to the dimension challenge, and it is increasingly used in finance research. Machine learning techniques become economically meaningful if they satisfy, but are not limited to, the following criteria: (i) the actual economic problem involves lots of variables; (ii) the impact of the variables is highly nonlinear or involves interaction terms among the variables (high dimensionality of function class); and (iii) prediction is more important economically than statistical inference. The most natural research questions occur when the decision-makers are machines, such as algorithmic traders or robo-advisors.
Complex structure: Finally, another important feature is that data are not in the traditional row-column format. Unstructured data include text, pictures, videos, audio, and voice. Unstructured data create value if they can measure economic activities that cannot be captured using structured data. Unstructured data are often high-dimensional by nature. The first step to analyze the data is usually to extract features from the unstructured data, often with help from deep learning and computer science. For example, researchers may extract semantic information from text using natural language processing (NLP), identify tone information from voice and audio using speech recognition, and recognize geographic or facial information from images and videos using computer vision (CV).
Overall, as these features reveal, big data is not only about the size of the data, but also about other characteristics. Developments in all three categories—increased availability and capability of handling large datasets, developments in methodologies to deal with high dimensionality, and the emergence of complex datasets with new methods for processing them—have led to the increased prominence of big data in finance research.
Each of the six papers in this special issue fits into one or more of these three categories. Anand et al. (2021) analyze the agency conflicts between brokers and their customers using a particularly large dataset established by the Financial Industry Regulatory Authority (FINRA) called the Order Audit Trail System (OATS). The dataset is big also in the relative sense because the OATS data include publicly unavailable information on broker identity and do not suffer from attrition and sample selection bias from self-reported data. Easley et al. (2021) also analyze large market microstructure data and, due to high dimensionality, apply machine-learning techniques to evaluate the effectiveness of traditional market microstructure measures after machines started dominating trading. The dataset in Giglio, Liao, and Xiu (2021) is distinctive not for its size, but for its high dimensionality. They also use machine-learning techniques to develop a new framework to deal with data snooping, a major concern in empirical asset pricing. Unlike the study by Giglio, Liao, and Xiu (2021) , where high dimensionality comes from a large number of hypothesis tests that may lead to false positives in multiple testing, the high dimensionality in the paper by Erel et al. (2021) comes from the interaction terms and nonlinearity. Erel et al. (2021) show that machines can dominate humans in choosing directors, perhaps because machines suffer less from biases or agency conflicts. Papers by Benamar, Foucault, and Vega (2021) and Li et al. (2021) both use unstructured data. Benamar, Foucault, and Vega (2021) measure information demand and uncertainty using clickstream data provided by a vendor that transforms unstructured data into structured data. Li et al. (2021) transform unstructured data themselves and develop a measure of corporate culture from textual data based on earnings calls.
These six papers cover topics in asset pricing, corporate finance, and market microstructure, demonstrating the broad scope of big data techniques in finance research. We now turn to describe these papers in more detail, their relation to one another, and to the broader theme.
In the first paper in the special issue, Erel et al. (2021) show that machine learning can outperform the actual selection of new board members, currently done by humans. They demonstrate that directors who algorithms predict will perform poorly indeed do, compared to a realistic pool of candidates in out-of-sample tests. 4 Relative to algorithm-selected directors, management-selected directors who later receive predictably low shareholder approval are more likely to be male, have larger networks, and sit on more boards. One possibility is that firms that nominate predictably unpopular directors tend to be subject to homophily, while the algorithm selects a more diverse board. The authors also find that firms that nominate predictably poor directors suffer from worse corporate governance structures, which suggests that agency conflicts could be a driver for the distortion in selecting directors.
The analysis in this paper is among the first applications of machine-learning methods in corporate finance, demonstrating the broad appeal of these methods across areas of finance. The authors demonstrate the usefulness of these methods by showing that traditional OLS results are unable to adequately predict director performance. They attribute these findings to nonlinearity and interactions among variables being key in predicting future performance. These results raise interesting questions for future research, trying to understand why the interaction among variables and/or the nonlinearity in the effects of different variables are so important.
Machine learning in a corporate finance context is a key characteristic of the second paper in the special issue, written by Li et al. (2021) . The authors try to quantify the notion of corporate culture and understand its implications across firms. Corporate culture is important because it is perceived to be a key factor behind many business successes and failures ( Graham et al. 2018 ), and it is thought to be able to solve problems that cannot be regulated properly ex ante ( Guiso, Sapienza, and Zingales 2015 ). Data challenges have always made studying corporate culture a formidable task. Despite the boom in empirical studies since the mid-1980s, 5 variables of economic interest may not be measured perfectly with structured data. Indeed, in the interview evidence by Graham et al. (2018) , corporate executives suggest 11 sources of data to measure corporate culture, most of which are unstructured data.
Li et al. (2021) make progress by using NLP models to extract key features of corporate culture from earnings call transcripts, which is one source of data suggested by corporate executives. They use a semi-supervised machine-learning approach with word embedding for textual analysis instead of the traditional “bag of words” approach ( Loughran and McDonald 2011 ). The “bag of words” approach is good at predicting the tone of a document by counting positive or negative words, but it is hard to capture important semantic information in an earnings call. The authors provide a method to decompose corporate culture onto a five-dimensional space of innovation, integrity, quality, respect, and teamwork, which are the five most-often mentioned values by the S&P 500 firms (see Guiso, Sapienza, and Zingales 2015 ). Guiso, Sapienza, and Zingales (2015) find that the culture-performance link is more significant during periods of distress, and that corporate culture is shaped by major corporate events, such as mergers and acquisitions. They show that firms scoring high on the cultural values of innovation and respect are more likely to be acquirers, and firms closer in cultural value are more likely to merge.
Another area where machine-learning methods have much unexploited potential is market microstructure. The third paper in the special issue, by Easley et al. (2021) , explores an application for analyzing whether machine-based trading affects the efficacy of market microstructure measures that were developed before machines dominated trading volume. Specifically, Easley et al. (2021) examine whether six extant market microstructure measures—the Roll measure, the Roll impact, 6 volatility (VIX), Kyle’s |$\lambda$| , the Amihud measure, and the volume-synchronized probability of informed trading (VPIN)—can still predict the future values of price and liquidity.
The authors find that the answer is still positive after the rise of high-frequency and machine-based trading. The functional form to make such predictions, however, depends on the application. For example, for making predictions within the same asset, a simple logistic regression performs almost as well as complex machine-learning techniques. One explanation is that there is already a deep understanding of the market structure for a single asset. For making predictions across assets, however, machine learning strictly dominates simple logistic regression. 7 Although the rise of high-frequency and machine-based trading has made cross-asset trading more the norm, few market microstructure theories show how these cross-asset effects should, or even could, occur. Easley et al. (2021) provide strong evidence that the interactions among assets can predict market outcomes and that machine learning helps address challenges from high dimensions in cross-asset market microstructure.
Thinking about big data in the context of market microstructure research more broadly, it is often noted that large datasets were the norm in this literature for a long time. Yet, the fourth article in the special issue, by Anand et al. (2021) , pushes the boundary in this sense, analyzing a particularly large dataset to identify agency conflicts between institutional traders and their brokers. To find such agency conflicts, it is very instructive to know the brokers’ identities, which are missing in the publicly available TAQ data. Self-reported data would suffer from attrition or sample selection bias issues. Anand et al. (2021) use OATS data to surmount these two challenges, as it is comprehensive regulatory data from FINRA.
The authors find that brokers, who route more orders to affiliated alternative trading systems (ATSs), offer lower execution quality (lower fill rates and higher implementation shortfall costs) for their customers. Therefore, these brokers take the private benefit by increasing the market share and fee revenues of their own ATSs, but do not necessarily satisfy their fiduciary responsibilities to achieve the best execution for their customers. As Anand et al. (2021) use a large and comprehensive dataset, a subsample of the dataset can still generate enough statistical power, which allows the authors to establish causality using a unique controlled experiment that overlaps with their sample period: the SEC Tick Size Pilot (TSP). Based on a triple-difference analysis, the authors find that execution quality improves for TSP-treated stocks for orders handled by brokers who prefer affiliated ATSs since the TSP imposes constraints on brokers to route orders to ATS venues.
The fifth paper in the special issue, written by Benamar, Foucault, and Vega (2021) , also analyzes a large dataset in the context of trading in financial markets. Another important feature of this paper is the processing of unstructured data. Here, unlike in Li et al. (2021) , who process such data themselves, Benamar, Foucault, and Vega rely on commercial data vendors that preprocessed the raw and unstructured data into structured data. This is part of the trend in the era of big data: along with the boom of data availability, the data vending industry has grown as well. J. P. Morgan’s Big Data and AI Strategies report provides a 78-page summary of available data vendors. 8 Benamar, Foucault, and Vega (2021) measure information demand with webpage clickstream statistics from Bitly, a URL-shortening service provider. 9 They use this to understand the role of uncertainty in financial market trading, a topic that has long occupied academics studying financial markets.
Benamar, Foucault, and Vega (2021) show that information demand is a good proxy for uncertainty because, based on their theory, an exogenous increase in an asset’s uncertainty motivates investors to search for more information on it. The search for information, however, cannot fully neutralize the increase in uncertainty. Thus, a stronger information demand about future interest rates ahead of macroeconomic and monetary policy announcements (MMPAs) implies that U.S. Treasury yields exhibit both higher uncertainty and stronger sensitivity to MMPAs. They find that a one-standard-deviation increase in the number of Bitly clicks on the news related to nonfarm payroll (NFP) in the two hours preceding NFP announcements raises the sensitivity of U.S. Treasury note yields by 4 to 6 basis points (bps), depending on maturity. The increase is economically significant because the unconditional sensitivity of U.S. Treasury note yields to NFP announcements varies between 3. 5 bps and 7 bps (depending on maturity) during their sample period. They also find that such predictability mostly comes from clicks within two hours before the announcement, which highlights the usefulness of high-frequency data for measuring information demand and uncertainty.
Finally, closing the special issue is the paper by Giglio, Liao, and Xiu (2021) . This paper belongs to the asset pricing literature, in which machine-learning methods have already been explored in some depth. A recent special issue of the Review of Financial Studies featured some of this research in the context of new methods for the cross-section of returns (see Karolyi and Van Nieuwerburgh 2020 for an introduction). Giglio, Liao, and Xiu (2021) show how machine learning can be applied by proposing a new framework to rigorously perform multiple hypothesis testing in linear asset pricing models, with a focus on addressing data snooping.
The dimension challenge in Giglio, Liao, and Xiu (2021) comes from multiple testing—that is, when trying to identify which factors in the “factor zoo” add explanatory power for the cross-section of returns or to identify which funds among thousands of funds can produce positive alpha. If the number of tests is high due to a large number of factors or funds, a potentially large fraction of the tests will be positive purely by chance and lead to a high false discovery rate. Giglio, Liao, and Xiu (2021) solve data snooping and false positives using a combination of matrix completion, wild bootstrap, screening, and false discovery control. Matrix completion, a machine-learning technique, helps them to interpolate missing data and latent factors. The latent factors constructed from machine learning correct correlation among alpha test statistics. Bootstrap and screening improve the robustness of multiple testing in a finite and skewed sample. The authors illustrate their framework using a hedge fund dataset, but their toolbox can be applied in other asset pricing research as well.
The six papers in this special issue can provide a starting point for discussing big data in finance. As a burgeoning field, big data and machine learning raise many new questions. We discuss several promising lines of research. We believe the list will continue to grow and be refined over time.
4.1 Machine learning and learning machines
To date, most research using machine learning, including papers in this special issue, use machine learning to understand human behavior. One promising area of machine learning in finance is when the decision-makers are machines. For example, most existing machine-learning research in asset pricing uses monthly return data from CRSP or quarterly holding data from 13F filings. Yet traders who apply machine learning techniques often operate at a horizon that is much less than a month. Hedge funds such as Renaissance, Two Sigma Investments, D. E. Shaw Group, PDT Partners, and TGS Management Company make thousands of trades and manage tens of billions of dollars in investor assets. 10 These firms, which are faster than most traditional funds but slower than high-frequency traders, are largely outside the radar of the academic finance literature. One exception is Chinco, Clark-Joseph, and Ye (2019) , who find that machine learning aims to predict news at the minute-by-minute horizon. A promising new line of research is to bridge the gap between studies that focus on the monthly horizon or above and the studies on high-frequency traders, which focus on horizons below a second. In this underexplored territory, applying machine learning is not only natural but also necessary. Just as insights into human behavior from the psychology literature spawned the field of behavioral finance, so can insights into algorithmic behavior (or the psychology of machines) spawn an analogous blossoming of research in algorithmic behavioral finance.
4.2 Feedback effects of the big data revolution
Once machines become decision-makers, will corporations change their behavior? The widespread application of machine learning in the investment community and the feedback effects between the secondary market and corporate decisions ( Bond, Edmans, and Goldstein 2012 ) imply that firms should respond to the big data revolution. While no papers in this special issue examine feedback effects, we saw related studies at the 2020 NBER-RFS Winter Conference on Big Data. Cao et al. (2020) find that firms adjust their 10-Ks and 10-Qs to cater to machine readers. The next step following their research is perhaps to examine whether firms react to the big data revolution when making real decisions. For example, as investors increasingly become machines, will firms increasingly pursue shorter-term projects? Does the advent of “big data” reduce managers’ incentives to learn from market prices because firms now have more information sources, or does it increase incentives because prices aggregate more information from the “big data” collected by investors?
4.3 Heterogeneous impact of the big data revolution
Although big data provides more information for sophisticated players such as institutional investors and firms, the impact of big data may not always be positive. Chawla et al. (2019) show that social media, which allows enthusiasm for the market to spread much more widely than it would have otherwise ( Shiller (2015) ), can push price away from fundamentals. In Chawla et al. (2019) , the price pressures led by retail traders quickly revert, probably because sophisticated arbitragers rapidly jump in and trade against retail behavioral bias. We witnessed a much more significant impact of social media during the GameStop episode in January 2021. Retail traders coordinated using social media, resulting in the hedge fund Melvin Capital losing 53%. 11 The interaction between retail and sophisticated investors leads to extreme market volatility. The impact of big data on different types of agents and its aggregated effect on society will be an interesting new direction to explore.
4.4 More complex data
Big data in finance starts from analyzing large-size data such as trades and quotes. More recent development allows researchers to use natural language processing (NLP) to extract information from unstructured data such as text ( Gentzkow, Kelly, and Taddy 2019 ). A promising research line is to analyze data of more complex structures, such as audio, video, and images if these more complex data provide additional insights. For example, Li et al. (2021) use the transcripts of earnings call as input for their analysis in this special issue. The earnings call transcripts are small data when we compare them with the audio file that generates the transcripts. Mayew and Venkatachalam (2012) show that managerial vocal cues contain information about a firm’s fundamentals, incremental to information conveyed by linguistic content. As the NBER-RFS Big Data Conference evolves, we see submissions using more complex datasets, such as satellite images ( Gerken and Painter 2020 ). More complex datasets create value for finance researchers if they measure economic activities that cannot be captured using simpler data.
4.5 Regulations
As machines start to be major players in many areas such as trading ( Angel, Harris, and Spatt 2015 ), it will be interesting to examine whether existing regulations, which are designed mostly for humans, need to be adapted to an environment with machines. O’Hara, Yao, and Ye (2014) provide one example for such need. Regulators used to consider trades of less than 100 shares to come from retail traders, and would exempt these odd lots from the reporting requirement. Yet informed traders later became major sources of odd lots by using algorithms to slice and dice their orders to less than 100 shares to escape the reporting requirement. While much of our financial regulatory system focuses on actual realized transactions, assessing problematic aspects of the underlying algorithms is arguably more fundamental and cuts to the heart of such issues as the possibility of front running by market markers, whether brokers have satisfied their best execution responsibilities, and whether insiders are exploiting informational advantages. Spatt (2020) discusses how regulations designed years ago need to be adapted to modern reality. The traditional focus of regulators has not emphasized biases in specific algorithms.
The other promising line of research on big data will be on privacy regulations and the fairness of algorithms and data (e. g., Kearns and Roth 2020 ). The question becomes extremely important because algorithms and data increasingly became a major resource for the economy, particularly for finance. Back in 2017, the Economist published a story titled “The World’s Most Valuable Resource Is No Longer Oil, but Data,” which called for new regulations for the data economy. 12 Who owns the data, what is the price of the data, and what is the impact of unfair access to data? Easley, O’Hara, and Yang (2016) provide a theoretical analysis of the issue. It would be interesting to explore this topic empirically.
The papers in this special issue are predominantly empirical, but theoretical work is also important for big data in finance. Although high-dimensional data are often defined as when the number of variables is larger than the number of observations ( Martin and Nagel 2019 ), the dataset frequently used in finance research is typically large enough to cover the number of variables. The success of machine learning often comes from high-order interaction terms between variables ( Mullainathan and Spiess 2017 ). Indeed, the success of machine learning for the papers in this issue also comes from nonlinear terms and interactions between variables. Such high-order interactions are a natural place to develop new theoretical models to explain why one economic variable’s impact depends on its interaction with another variable. The nonlinearity also motivates theory models to explain why a variable’s impact depends largely on its value. Machine learning is one way to describe the world, and we also need theory to explain the world.
Theory may become more important in the era of machine learning and artificial intelligence for one simple reason. Human judgment can be inconsistent, whereas machines tend to make consistent decisions based on their model. Li and Ye (2020) find that their theory model can generate quantitatively accurate predictions for market liquidity in cross-section and after corporate events such as stock splits, probably because liquidity providers are now algorithms, and these algorithms probably make decisions using similar models to the theoretical models in Li and Ye (2020) .
4.7 Interdisciplinary collaborations
Future work on big data in finance may involve more scholars from other fields. We believe such collaborations will expand the tools and scope of research in finance and economics and help researchers overcome big data challenges.
Researchers can overcome the large-size challenge by collaborating with supercomputing centers. The NSF’s Extreme Science and Engineering Discovery Environment Project (XSEDE) provides computing resources and staff support to manage and store large datasets free of charge. NBER has posted videotaped lectures for researchers in economics and finance on the application process for such free resources on the webpage for the 2018 Summer Conference on Big Data. 13
Researchers can overcome the high-dimension challenge and the complex-structure challenge by collaborating with scholars from the fields of math, statistics, and computer science. The recent development in deep-learning models like natural language processing (NLP), speech recognition, and computer vision (CV) helps researchers parse textual, verbal, and visual data. Researchers can also choose to work with data vendors. J. P. Morgan’s Big Data and AI Strategies report provides a list of vendors for alternative data, such as satellite photos, sentiment measures, and credit card usages.
The NSF lists big data as one of its 10 big ideas and provides funding to support innovative, interdisciplinary research in data science. We hope this special issue is only a starting point, and that we will see more research at the intersection of big data, finance, and public policy for many years.
This introduction is written for a special issue of the Review of Financial Studies focused on big data in finance. The authors thank Ken French, Harrison Hong, Wei Jiang, Andrew Karolyi, and Jim Poterba for comments. We thank Jim Poterba and Carl Beck for help with the NBER Workshops on Big Data. Ye acknowledges support from National Science Foundation grant 1838183 and the Extreme Science and Engineering Discovery Environment (XSEDE).
1 G. Rogow, “Meet the New Kings of Wall Street,” Wall Street Journal , May 21, 2017, https://www.wsj.com/articles/the-quants-meet-the-new-kings-of-wall-street-1495389163 .
2 C. Cutter, “Elite MBA Programs Report Steep Drop in Applications,” Wall Street Journal , October 15, 2019, https://www.wsj.com/articles/elite-m-b-a-programs-report-steep-drop-in-applications-11571130001 .
3 One day of current option trading data alone is roughly two terabytes. In the 2019 NBER-RFS Summer Conference on Big Data supported by the same NSF grant, the chief economist of the U.S. Securities and Exchange Commission (SEC), S. P. Kothari, pointed out that one of the biggest data collection efforts in finance is the Consolidated Audit Trial (CAT), which provides a single, comprehensive database enabling regulators to track more efficiently and thoroughly all trading activity in equities and options throughout the U.S. markets. https://www.sec.gov/news/speech/policy-challenges-research-opportunities-era-big-data .
4 The task of measuring the performance of an individual director is challenging because directors generally act collectively on the board. The authors’ main measure of director performance is the level of shareholder support in annual director reelections, because Hart and Zingales (2017) emphasize that directors’ fiduciary duty is to represent the interests of the firm’s shareholders.
5 See Einav and Levin (2014) .
6 Roll impact is the Roll measure divided by the dollar value traded over a certain period.
7 The cross-asset effects in their paper mean using market microstructure measures in one asset, such as equity futures, to predict price and liquidity dynamics of another asset, such as fixed-income futures.
8 Kolanovic and Krishnamachari (2017) .
9 A shortened URL is a compressed link to certain webpages. For example, https://academic.oup.com/rfs/advance-articles can be shortened to https://bit.ly/3mS7yDv .
10 G. Zuckerman and B. Hope, “The Quants Run Wall Street Now,” Wall Street Journal , May 21, 2017, https://www.wsj.com/articles/the-quants-run-wall-street-now-1495389108 .
11 J. Chung, “Melvin Capital Lost 53% in January, Hurt by GameStop and Other Bets, ” Wall Street Journal, January 31, 2021, https://www.wsj.com/articles/melvin-capital-lost-53-in-january-hurt-by-gamestop-and-other-bets-11612103117 .
12 “The World’s Most Valuable Resource Is No Longer Oil, but Data,” Economist , May 6, 2017, https://www.economist.com/leaders/2017/05/06/the-worlds-most-valuable-resource-is-no-longer-oil-but-data .
13 http://www2.nber.org/si2018_video/bigdatafinancialecon/ .
Anand, A. , Samadi M. , Sokobin J. , and Venkataraman K. . 2021 . Institutional order handling and broker-affiliated trading venues . Review of Financial Studies 34:3364–402.
Google Scholar
Angel, J. J. , Harris L. E. , and Spatt C. S. . 2015 . Equity trading in the 21st century: An update . Quarterly Journal of Finance 5 : 1 – 39 .
Benamar, H. , Foucault T. , and Vega C. . 2021 . Demand for information, uncertainty, and the response of US Treasury securities to news . Review of Financial Studies 34:3403–55.
Bond, P. , Edmans A. , and Goldstein I. . 2012 . The real effects of financial markets . Annual Review of Financial Economics 4 : 339 – 60 .
Cao, S. , Jiang W. , Yang B. , and Zhang A. L. . 2020 . How to talk when a machine is listening: Corporate disclosure in the age of AI . NBER Working Paper 27950 .
Google Preview
Chawla, N. , Da Z. , Xu J. , and Ye M. . 2019 . Information diffusion on social media: Does it affect trading, return, and liquidity? Working Paper .
Chinco, A. , Clark-Joseph A. D. , and Ye M. . 2019 . Sparse signals in the cross-section of returns . Journal of Finance 74 : 449 – 92 .
Easley, D. , Lopez de Prado M. , O’Hara M. , and Zhang Z. . 2021 . Microstructure in the machine age . Review of Financial Studies 34:3316–63.
Easley, D. , O’Hara M. , and Yang L. . 2016 . Differential access to price information in financial markets . Journal of Financial and Quantitative Analysis 51 : 1071 – 1110 .
Einav, L. , and Levin J. . 2014 . Economics in the age of big data . Science 346 ( 6210 ): 715 .
Erel, I. , Stern L. , Tan C. , and Weisbach M. S. . 2021 . Selecting directors using machine learning . Review of Financial Studies 34:3226–64.
Gentzkow, M. , Kelly B. , and Taddy M. . 2019 . Text as data . Journal of Economic Literature 57 : 535 – 74 .
Gerken, W. C. , and Painter M. . 2020 . The value of differing points of view: Evidence from Financial Analysts’ Geographic Diversity . Working Paper .
Giglio, S. , Liao Y. , and Xiu D. . 2021 . Thousands of alpha tests . Review of Financial Studies 34:3456–96.
Goldstein, I. , Jiang W. , and Karolyi G. A. . 2019 . To FinTech and beyond . Review of Financial Studies 32 : 1647 – 61 .
Graham, J. R. , Grennan J. , Harvey C. R. , and Rajgopal S. . 2018 . Corporate culture: The interview evidence . Working Paper .
Guiso, L. , Sapienza P. , and Zingales L. . 2015 . The value of corporate culture . Journal of Financial Economics 117 : 60 – 76 .
Hart, O. , and Zingales L. . 2017 . Companies should maximize shareholder welfare not market value . Journal of Law, Finance, and Accounting 2 : 247 – 74 .
Karolyi, G. A. , and Van Nieuwerburgh S. . 2020 . New methods for the cross-section of returns . Review of Financial Studies 33 : 1879 – 90 .
Kearns, M. , and Roth A. . 2020 . The ethical algorithm: The science of socially aware algorithm design . Oxford : Oxford University Press .
Kolanovic, M. , and Krishnamachari R. . 2017 . Big data and AI strategies: Machine learning and alternative data approach to investing. J. P. Morgan. Available at https://www.cfasociety.org/cleveland/Lists/Events
Li, K. , Mai F. , Shen R. , and Yan X. . 2021 . Measuring corporate culture using machine learning . Review of Financial Studies 34:3265–315.
Li, S. , and Ye M. . 2020 . The share price that maximizes liquidity: A tale of two discretenesses . Working Paper .
Loughran, T. , and McDonald B. . 2011 . When is a liability not a liability? Textual analysis, dictionaries, and 10-Ks . Journal of Finance 66 : 35 – 65 .
Martin, I. , and Nagel S. . 2019 . Market efficiency in the age of big data . NBER Working Paper 26586 .
Mayew, W. J. , and Venkatachalam M. . 2012 . The power of voice: Managerial affective states and future firm performance . Journal of Finance 67 : 1 – 43 .
Mullainathan, S. , and Spiess J. . 2017 . Machine learning: An applied econometric approach . Journal of Economic Perspectives 31 : 87 – 106 .
O’Hara, M. , Yao C. , and Ye M. . 2014 . What’s not there: Odd lots and market data . Journal of Finance 69 : 2199 – 236 .
Shiller, R. J. 2015 . Irrational exuberance . Princeton, NJ : Princeton University Press .
Spatt, C. S. 2020 . Is equity market exchange structure anti-competitive? Working Paper .
Email alerts
Citing articles via.
- Recommend to your Library
Affiliations
- Online ISSN 1465-7368
- Print ISSN 0893-9454
- Copyright © 2024 Society for Financial Studies
- About Oxford Academic
- Publish journals with us
- University press partners
- What we publish
- New features
- Open access
- Institutional account management
- Rights and permissions
- Get help with access
- Accessibility
- Advertising
- Media enquiries
- Oxford University Press
- Oxford Languages
- University of Oxford
Oxford University Press is a department of the University of Oxford. It furthers the University's objective of excellence in research, scholarship, and education by publishing worldwide
- Copyright © 2024 Oxford University Press
- Cookie settings
- Cookie policy
- Privacy policy
- Legal notice
This Feature Is Available To Subscribers Only
Sign In or Create an Account
This PDF is available to Subscribers Only
For full access to this pdf, sign in to an existing account, or purchase an annual subscription.
- Open access
- Published: 06 October 2021
Insights into financial technology (FinTech): a bibliometric and visual study
- Bo Li ORCID: orcid.org/0000-0003-0721-0601 1 &
- Zeshui Xu 2
Financial Innovation volume 7 , Article number: 69 ( 2021 ) Cite this article
22k Accesses
48 Citations
Metrics details
This paper conducted a comprehensive analysis based on bibliometrics and science mapping analysis. First, 848 publications were obtained from Web of Science. Their fundamental characteristics were analyzed, including the types, annual publications, hot research directions, and foci (by theme analysis, co-occurrence analysis, and timeline analysis of author keywords). Next, the prolific objects (at the level of countries/regions, institutions, journals, and authors) and corresponding pivotal cooperative relationship networks were used to highlight who pays attention to FinTech. Furthermore, the citation structures of authors and journals were investigated, including citation and co-citation. Additionally, this paper presents the burst detection analysis of cited authors, journals, and references. Finally, combining the analysis results with the current financial environment, the challenges and future development opportunities are discussed further. Accordingly, a comprehensive study of the FinTech documents not only reviews the current research characteristics and trajectories but also helps scholars find the appropriate research entry point and conduct in-depth research.
Introduction
FinTech (abbreviation for financial technology, as an emerging technical term) is driven by a variety of emerging frontier technologies. It is a series of new business models, new technology applications, and new products and services that have a significant impact on the financial market and supply of financial services. It has attracted wide attention because of the following advantages: improving the efficiency of operations, reducing operating costs effectively, disrupting the existing industry structures, blurring industry boundaries, facilitating strategic disintermediation, providing new gateways for entrepreneurship, and democratizing access to financial services (Agarwal and Zhang 2020 ; Cao et al. 2020 ; Admati and Hellwig 2013 ; Loubere 2017 ; Pinochet et al. 2019 ; Philippon 2016 ; Yang et al. 2020 ; Suryono et al. 2020 ). The key technologies of FinTech include internet technology (including Internet and Web of Things) (Ruan et al. 2019 ), big data (Chen et al. 2017 ; Gai et al. 2018a ), artificial intelligence (Belanche et al. 2019 ), distributed technology (blockchain and cloud computing) (Belanche et al. 2019 ; Gomber et al. 2018 ; Chen et al. 2019 ; Wamba et al. 2020 ; Miau and Yang 2018 ), and security technology (biometric technology) (Gai et al. 2018a , b ; Wamba et al. 2020 ). Under the influence of these technologies, the traditional development model of the financial industry has changed.
Furthermore, scholars have done studies involving theories and applications. To examine FinTech adoption and use from the technology acceptance perspective, Singh et al. ( 2020 ) proposed a research framework by adding substructures of the technology acceptance model. The FinTech ecosystem consisting of FinTech startups, technology developers, government, financial customers, and traditional financial institutions was presented by Lee and Shin ( 2018 ). Accordingly, the application of FinTech has been involved in many areas, such as mobile payment (Gomber et al. 2018 ), mobile networks (Gai et al. 2016 ; Wen et al. 2013 ; Zhang et al. 2013 ; Zhang and Soong 2004 ), big data (Yin and Gai 2015 ), blockchain (Wamba et al. 2020 ; Iman 2018 ), P2P lending (Gomber et al. 2018 ; Ge et al. 2017 ; Suryono et al. 2021 ; Wang et al. 2020a , b ), cloud computing (Castiglione et al. 2015 ; Gai et al. 2018a , b ), banking service, investment funds, retail groups, and telecom operators (Singh et al. 2020 ), image processing (Castiglione et al. 2007 ), and data analysis techniques (Qiu et al. 2015 ).
FinTech promotes the development of the financial industry. Specifically, it will be easier to collect and analyze data in the financial market to reduce information asymmetry. Trading and investment strategies based on artificial intelligence and big data can redefine the price discovery mechanism of the financial market and improve transaction speed, promoting the liquidity of the financial market and enhancing the efficiency and stability of the financial market. Regulators analyze, warn, and prevent systemic risks in the financial market more efficiently. Additionally, the smart FinTech helps save labor costs and reduce staff duplication by combining big data with artificial intelligence. Next, the development and application of FinTech help more people, especially the poor, obtain financial services at a lower cost and more conveniently, and share more reform results. Moreover, because of the “Belt and Road”, many countries share the achievements of FinTech. For example, our country’s mobile payment helps the economic and financial development of countries along the “Belt and Road”.
To explore the boundaries and research paradigms of the financial disciplines that have been broken and reconstructed, this paper analyzed the current research characteristics and development trends according to the publications in the field of FinTech. 95.28% of all publications were published after 2015 (according to Web of Science (WoS)). The explosive growth and the advantages of bringing great convenience to economic management activities have prompted us to conduct a comprehensive analysis and explore the current challenges and opportunities facing the field of FinTech. It is essential for scholars who are interested in this field to conduct better and more in-depth research. Additionally, a comprehensive analysis helps investigate the development track characteristics and disclose statistical patterns through bibliometric analysis (Borgman and Furner 2002 ; Wang et al. 2018 ). Furthermore, this paper investigated the current research hot topics, identified the challenges, and predicted the future development trends.
Bibliometrics, as a statistical and quantitative analysis of academic literature, has access to visualizing the analysis results using science mapping analysis tools, such as CiteSpace and Vosviewer (Chen 2006 ; Stopar and Bartol 2019 ; Van Eck and Waltman 2010 ), thereby improving the readability of analysis results. Bibliometric analysis has been widely applied in different research areas, such as bitcoin (Merediz-Solà and Bariviera 2019 ), blockchain (Miau and Yang 2018 ), fuzzy decision making (Liu and Liao 2017 ), deep learning (Li et al. 2020 ), social sciences (Nasir et al. 2020 ), business and economics (Merigo et al. 2016 ), COVID-19 (Lou et al. 2020 ), financial innovation (Li and Xu 2021 ), poverty cycles (Qin et al. 2021 ), blockchain and cryptocurrency (Nasir et al. 2021 ), and journals ( European Journal of Operational Research (Laengle et al. 2017 ), Information Sciences (Yu et al. 2017 ), IEEE Transaction on Fuzzy Systems (Yu et al. 2018 ), Environmental Impact Assessment Review (Nita 2019 ), and International Journal of Systems Science (Wang et al. 2021 )). The two visualization tools, i.e., CiteSpace and Vosviewer, can assist the bibliometric method in revealing the static and dynamic characteristics of FinTech publications from various aspects. For example, the co-occurrence network of author keywords demonstrates the main research topics; the citation and co-citation analysis highlight the top influential objects; the burst detection analysis and timeline view can exhibit changes in a certain period. These processes are called science mapping analysis (Van Eck and Waltman 2010 ; Cobo et al. 2011 ).
The contributions of this paper can be summarized as follows: (1) Illustrate the basic features of FinTech publications, including the types, annual publications, main research directions by co-occurrence analysis of keywords, and dynamic changes of research focus by timeline analysis; (2) Explore popular countries/regions, institutions, journals, and authors and the collaboration relationship networks, and present the citation and co-citation networks to highlight the influential authors and journals; (3) Furthermore, detect the dynamic changes of cited authors, cited journals and cited references based on burst detection analysis, and more intuitively show the citation process of all FinTech publications based on overlay analysis; (4) With the current special environment, discuss the challenges FinTech faced and future possible development directions.
The rest of this paper is organized as follows: “ Data and methods ” section briefly describes the data and methods used in this paper. “ Fundamental characteristics of FinTech publications ” section presents the foundation characteristics of all FinTech publications, in terms of types, annual publications, current research directions and themes, co-occurrence, and timeline analysis of author keywords. The top productive countries/regions, institutions, and journals are presented in “ Productive object analysis and cooperation relationship analysis ” section. Additionally, the cooperation relationship is demonstrated. “ Citation structure analysis ” section investigates the citation structure, including citation and co-citation of authors and journals, respectively. Meanwhile, a burst detection analysis of cited journals, cited authors, and cited references is conducted. Furthermore, the current challenges and future possible research directions are discussed in “ Discussion ” section. “ Conclusions ” section ends this paper with some conclusions.
Data and methods
The literature data used in this paper are obtained from WoS (Falagas et al. 2008 ), one of the most widely used databases in academics, owned by Thomson Reuters Corporation. In this paper, we derived data through the search function in WoS by selecting as Database = Web of Science ™ Core Collection database; Topic search = FinTech or “Financial technology” or “Financial technologies”; Timespan = 1900–2020 (The data were derived on September 23, 2020. We searched the documents from the earliest time of WoS). As a result, 848 documents were retrieved and exported in plain text file format for software (CiteSpace and Vosviewer) bibliometric analysis. The contents in the derived documents are representative, including title, abstract, keywords, citations and references.
As presented in the Introduction, bibliometrics is used to highlight the development trajectory and characteristics of a particular research field (Mourao and Martinho 2020 ). This paper used the bibliometric analysis method to evaluate the development of FinTech documents from the following aspects: (1) start with the types and annual publications with significant indexes (such as numbers and rates), the distribution among different countries/regions, and important branches. Moreover, the productive countries/regions, institutions, journals, and authors are assessed by several recognized indicators, including the total number of publications (TP), the total number of citations (TC), the average citations per publications (AC), and H-index. (2) With statistics and visualization tools, the science mapping analysis is conducted to deeply master the characteristics of FinTech documents, such as the dynamic development trend. CiteSpace and Vosviewer, as two mature visualization tools, effectively illustrate the inner relationship of documents and visualize them in different ways, such as clustering and dynamic timeline (Chen 2006 ; Stopar and Bartol 2019 ; Kou et al. 2014 ). Through several bibliometric methods, including co-occurrence analysis, timeline analysis, burst detection and co-authorship analysis, this paper presented the keyword situation, citations, and cooperation networks of countries/regions and institutions on FinTech research. The whole process of bibliometric analysis in the field of FinTech can be illustrated in Fig. 1 .
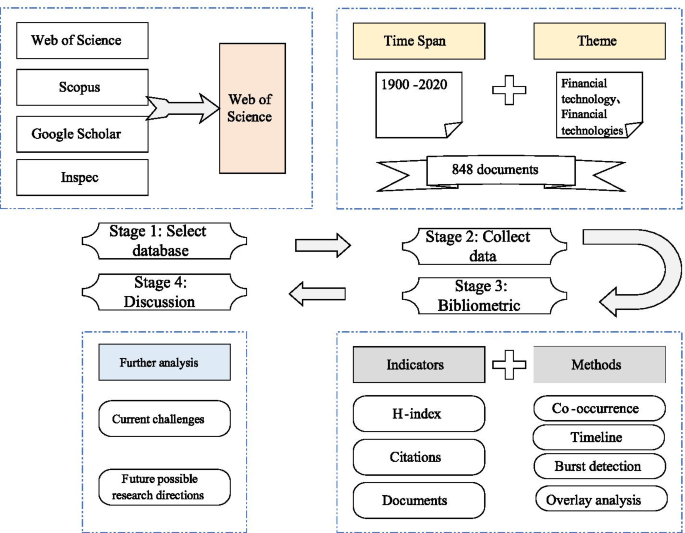
The research framework and process of this study
Fundamental characteristics of FinTech publications
Types and annual publications.
For the 848 obtained publications in the field of FinTech, the first document was written by Ronner and Trappeniers ( 1996 ), that is, Currency exposure management within Philips , a proceedings paper. The average number of publications is 33, which is low because a lot of literature has exploded in the past five years. The types of the 848 documents and annual publications are presented in Table 1 and Fig. 2 , respectively.
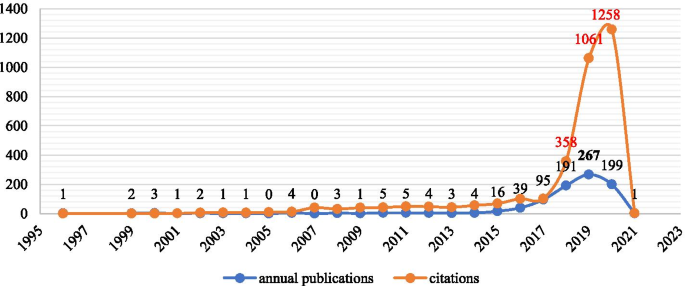
The annual publications and citations
From Table 2 , most publications are articles with 531, accounting for 63.139%. Followed, 236 are proceedings papers, accounting for 28.062%; 51 (6.064%) are early access. Besides, there are 35 reviews, 33 editorial materials, 10 book reviews, four book chapters, two corrections, one data paper, and one meeting abstract. Articles and proceedings papers dominated the FinTech publications and accounted for 91.201%. Figure 1 illustrates the annual publications and the number of citations per year. 2019 has the greatest number of publications with 267 and ranks second for the numbers of citations with 1,061. 2020 and 2018 have 199 and 191 publications, respectively. 2020 has the greatest number of citations with 1,258. Furthermore, the H-index (Wang et al. 2020a , b ) of all documents is 27 and total citations are 3,338 (remove self-citations: 2,423). All the above phenomena reflect that FinTech is a relatively new field and has attracted wide attention recently. In contrast, it reflects that there is still room for development.
Figure 3 demonstrates the countries/regions with more than 20 publications. Taiwan, a part of China, is studied as a region in this paper. As shown in Fig. 3 , the United States is the most productive country with 159. Together with Taiwan, China ranks first with 187. Then, the third to the tenth are England (96), Australia (50), Russia (50), Indonesia (44), South Korea (44), Taiwan (41), Germany (40), and Switzerland (26), respectively. The top 10 countries/regions account for 82.076% of the 848 publications. The United States and China, as the top two most productive countries, have 305 publications, accounting for 35.967%.
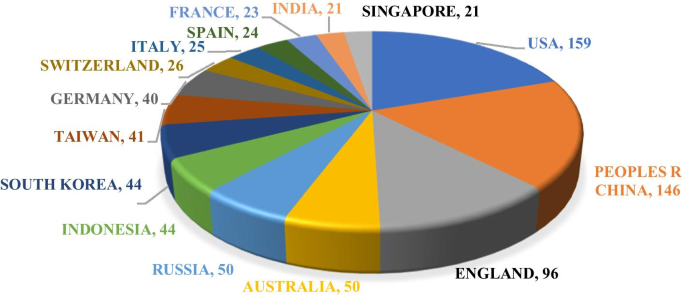
The countries/regions with more than 20 publications
Research directions and themes
The top 25 research directions of the publications are shown in Fig. 4 . Business economics and computer science are the most popular research directions. The number of publications for business economics is 408, and this accounts for 48.11%. The number of publications for computer science is 213, which accounts for 25.12%. Furthermore, research is widespread in government law (86), engineering (82), telecommunications (32), science technology and other topics (30), and environmental sciences ecology (29). FinTech covers many areas and promotes the development of numerous research directions. Based on Vosviewer, the visualization of the theme of the 848 publications is shown in Fig. 5 . We can see that, except for FinTech, some terms (e.g., data, market, model, system, bank, and neural network) have a high frequency. Moreover, Fig. 6 a presents the co-occurrence view of author keywords in this field. The co-occurrence method was first provided in the 1980s, which has been widely applied in bibliometrics or other fields and helps scholars grasp the study hotspots (Ding et al. 2001 ). We obtained 389 author keywords by setting the minimum number of occurrences of a keyword to 2 and merging financial technology and financial technologies into FinTech. The high-frequency keywords with the close co-occurrence relationship in the field of FinTech are shown in Fig. 6 b by setting the minimum number of occurrences of a keyword to 10.

The top 25 hot research directions (generated using WoS on data)
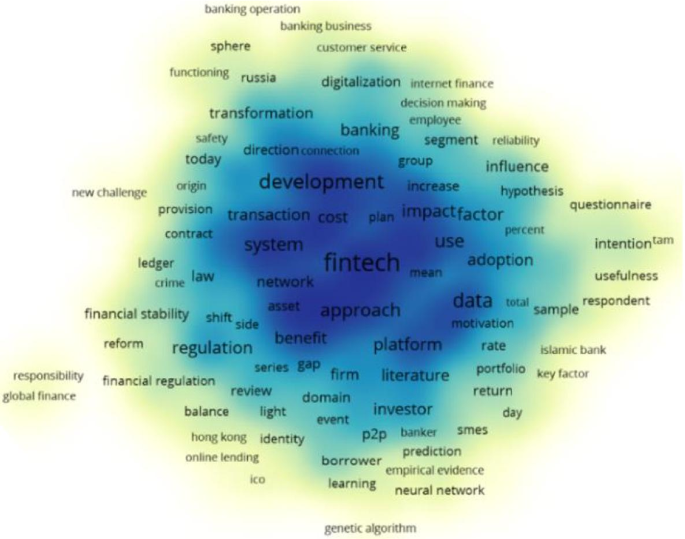
The theme of all 848 publications (generated using Vosviewer on data)
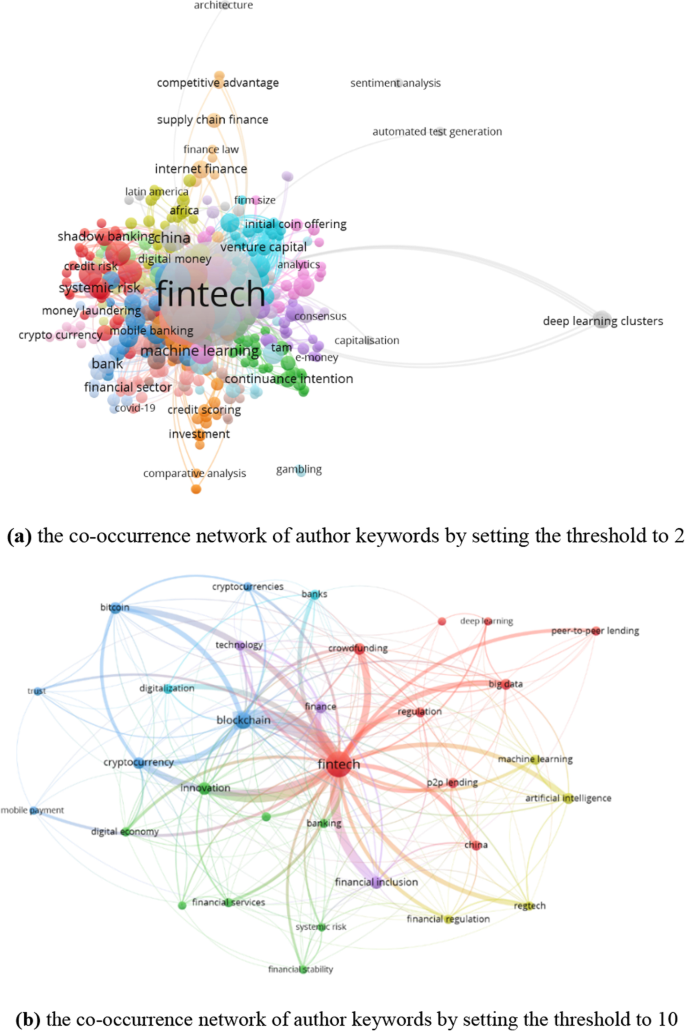
The co-occurrence network of author keywords (generated using Vosviewer on data)
In Fig. 6 a, the nodes denote the author keywords, and the sizes mean the occurrences. The link between two keywords reflects that the two keywords appear in one paper simultaneously. The thickness of the lines between nodes means the number of co-occurrences of the two keywords. Specifically, the frequency of “FinTech” is 385 with 338 links (338 keywords appear with it) and 1,106 total link strength (a total of 1,106 times). “blockchain” has 98 occurrences and ranks second, followed by “financial inclusion” with 36, “innovation” with 36, “cryptocurrency” with 34, and “bitcoin” with 29. For “FinTech”, “blockchain” has the strongest connection with it (link strength is 57). Also, keywords that are closely related to “FinTech” include financial inclusion (link strength is 29), innovation (27), crowdfunding (22), and big data (18). In Fig. 6 b, we obtained 32 keywords. Popular topics include digitalization (the frequency is 21), machine learning (17), deep learning (11), and Internet finance (8).
Timeline view of author keywords
As shown in Fig. 6 , various keywords belong to different subareas. To understand the dynamic development trend further, this subsection illustrates their timeline view (Fig. 7 ). From this view, all keywords are classified into 5 clusters, i.e., P2P lending, finance law, lending, cryptocurrency, and technology acceptance model. Cluster 3 is the category with the longest time. The vast majority of keywords broke out after 2014. From 2014 to 2019, research topics, such as banking, mobile payment, P2P lending, financial regulation, e-payment, and big data emerged and continued. Digital economy, household finance, and financial stability were proposed in 2019. Mobile money appeared in 2020. The phenomena verify that FinTech is a new and hot field in recent years once again. With the rapid development of science and technology, much research will explode in a short time. Thus, it is necessary to summarize the research results on time, which highlights the significance of this paper.
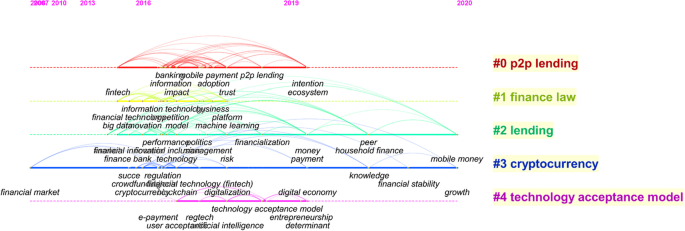
The timeline view of keywords (generated using CiteSpace on data)
Productive object analysis and cooperation relationship analysis
This section presents the most productive countries/regions, institutions, journals, and authors. Knowing their foci and situations help scholars locate authoritative academics and journals accurately. Moreover, as one important indicator, cooperation relationship analysis is another research point in this section.
The most productive countries/regions
Table 2 lists the top 5 most productive countries/regions. Some bibliometric indicators, such as TP, TC, AC, the number of publications that are cited equal to or more than 100/50 (≥ 100/ ≥ 50), and H-index are used to demonstrate the citation impact of the productive countries/regions.
The United States has the greatest number of citations with 1,235 and H-index with 18. The most cited US paper is the work of AN Berger The economic effects of technological progress: Evidence from the banking industry , a review, published in the Journal of Money Credit and Banking (its impact factor is 1.355, belongs to Q3). The paper examined technological progress and its effects on the banking industry (Berger 2003 ). China has 566 citations. The most cited Chinese paper is the work of KK Gai et al. A survey on FinTech , published in the Journal of Network and Computer Applications (its impact factor is 5.57, belongs to Q1). It produced a survey of FinTech by collecting and reviewing contemporary achievements and then proposed a theoretical data-driven FinTech framework (Gai et al. 2018a , b ). Table 3 lists the top 10 most frequent author keywords of the top 3 most productive countries (i.e., the United States, China, and England). Blockchain is one of the most common research topics, and the frequencies for each country are 13, 17, and 7, respectively. Furthermore, scholars in the United States and China pay more attention to P2P lending, financial inclusion, and cryptocurrency. Regulatory technology (RegTech) is another key topic for Chinese and British scholars. In summary, current hot research directions include blockchain, P2P lending, big data, financial inclusion, and regulation.
The cooperation relationship among countries/regions is illustrated in Fig. 8 . There are a total of 86 countries/regions, and we selected 62 terms by setting the minimum number of documents of a country to 2 and presented the closest cooperation network, including 52 terms. They are divided into 11 clusters, and different colors represent different categories. In Fig. 8 , the nodes represent the countries/regions, and the sizes of the nodes denote the number of documents. The links between two nodes denote that they have a cooperative relationship with each other. The thicker the link is, the greater their collaboration. The most cooperative countries/regions in each category are the United States, China, England, Russia, Australia, Canada, and South Korea, respectively. The United States is the most cooperative and often cooperates with China, which can be reflected by the links between them. From the perspective of TP and TC of the United States and China, the importance of cooperation is clear.
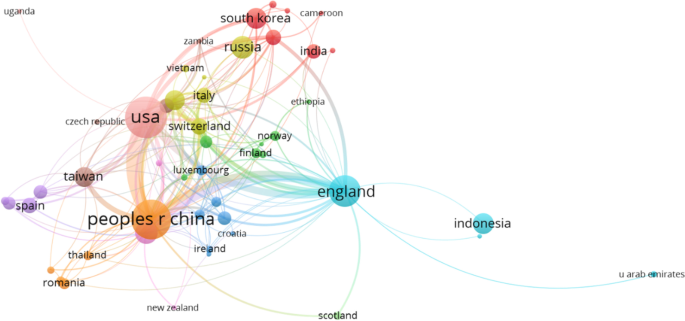
The collaboration network of countries/regions (generated using Vosviewer on data)
Productive institutions
Table 4 exhibits the top 5 most productive institutions. In the list, the University of London has the greatest number of TP, and it is the only institution that has published more than 50 citations. The Ministry of Education Science of Ukraine, University of New South Wales Sydney, University of Hong Kong, and Massachusetts Institute of Technology follow. In terms of TC, the University of New South Wales Sydney ranks first with 148. The second to the fifth institutions are the University of London (111), University of Hong Kong (77), Massachusetts Institute of Technology (72), and Ministry of Education Science of Ukraine (6). The University of New South Wales Sydney and the University of Hong Kong jointly published an article, FinTech, RegTech, and the Reconceptualization of Financial Regulation (Arner et al. 2017 ). It is the most cited publication in the field of FinTech. The phenomenon drives us to explore the cooperative relationship among institutions.
There are 1,048 institutions in the field of FinTech. Figure 9 presents the cooperative relationship networks of all institutions and the closest network of 333 institutions. In Fig. 9 , the sizes of the nodes present the number of documents. The gray nodes indicate that the articles published by these institutions in the field of FinTech were not done in cooperation with other institutions. To improve the TP and TC of publications in this area, cooperation urgently needs to enhance. In the closest cooperation network, the size of the node represents the number of total link strengths. As a result, Singapore Management University is the most cooperative institution, and its total link strength is 38 related to 8 documents with 81 citations. The University of Minnesota System (its total link strength, related documents, and citations are 32, 6, and 81, respectively), New York University (32, 6, and 77), City University of Hong Kong (32, 7, and 56), and the City University of New York (30, 4, and 61) follow. Even though they are not in the top 5 most productive institutions list, their influence is at a higher level.
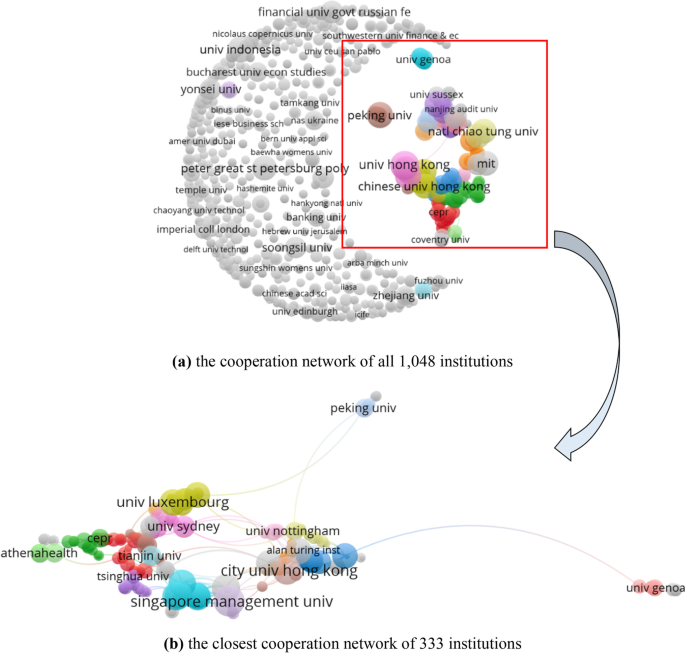
The cooperation networks of institutions (generated using Vosviewer on data)
Productive journals
Table 5 lists the top 10 most productive journals and their corresponding important indicators, such as TP, TC, and impact factor (IF), ≥ 100 and ≥ 50. Considering that FinTech is a new field, the number of TP and TC is relatively low. For the top 10 list, three are proceedings papers/books. Four belong to Q1 journals, including Electronic Commerce Research and Applications , Journal of Management Information Systems , Financial Innovation and IEEE Access . Additionally, 5 journals have IF greater than 2. Electronic Commerce Research and Applications (its TP is 15) is the most popular journal for scholars in the field of FinTech, and it has the greatest number of TC with 248. Financial Innovation , as a new journal launched recently, has 8 publications related to the keywords FinTech. It has a relatively high level of TC with 80.
Moreover, only one journal, i.e., Electronic Commerce Research and Applications , has published a paper cited more than 100. The paper is, The economics of mobile payments: Understanding stakeholder issues for an emerging financial technology application . It examined a new technology application, in association with the revolution in wireless connectivity, i.e., mobile payments (Au and Kauffman 2008 ). Similarly, the number of articles posted by authors is scattered. The authors who have published more than 5 papers are DW Arner, RP Buckley, RJ Kauffman, SH Huang, D Wojcik, and J Zhang. Among them, the work of RJ Kauffman has the most citation with 151, i.e., The economics of mobile payments: Understanding stakeholder issues for an emerging financial technology application . FinTech, RegTech, and the Reconceptualization of Financial Regulation (Arner et al. 2017 ), the work of DW Arner and RP Buckley has the most citation (38) for the documents published by them in the field of FinTech, which once again confirms the importance of cooperation. The cooperation network is shown in Fig. 10 . Setting the threshold to 2 means the minimum number of documents of an author. We obtained the closest network (Fig. 9 a) from the complete cooperation network (Fig. 10 b). According to the above analysis, the spirit of cooperation is worth promoting to enhance the influence of publications and authors.
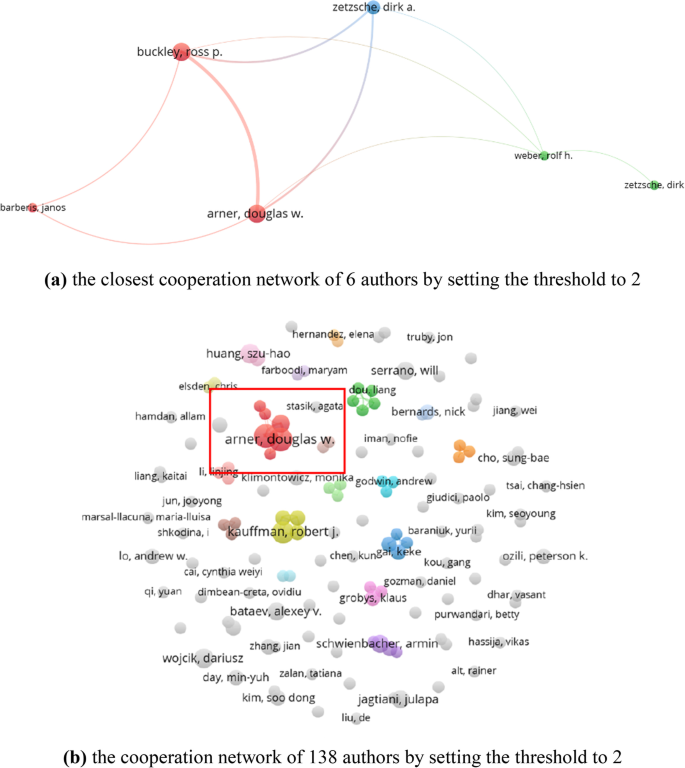
The cooperation network of authors (generated using Vosviewer on data)
Citation structure analysis
To investigate the influence of the cited authors and journals further, this section conducts citation structure analysis, including citation analysis and co-citation analysis in terms of authors and journals. Thus, scholars who are interested in this field can locate authoritative academics and journals accurately.
Citation explains the number of times the author has been cited, and co-citation reflects that two authors/journals/references/sources are cited in one paper simutaneously. According to Vosviewer, we obtained 138 authors out of 2,025 based on the minimum number of documents of an author (i.e., the threshold is 2), and 90 cited authors out of 20,877 based on the minimum number of citations of an author (i.e., the threshold is 20). The citation network and co-citation network are presented in Fig. 11 . 79 of 138 cited authors constitute the closest citation network. They are divided into 7 clusters. The nodes and their sizes denote the authors and the citation degree, respectively. The greater the node, the more times the author is cited. 89 of the 90 cited authors constitute the closest co-citation network and are divided into 6 clusters. The connection between the two cited authors indicates that they appeared in one paper. The thicker the line, the more frequently the two authors appeared together.
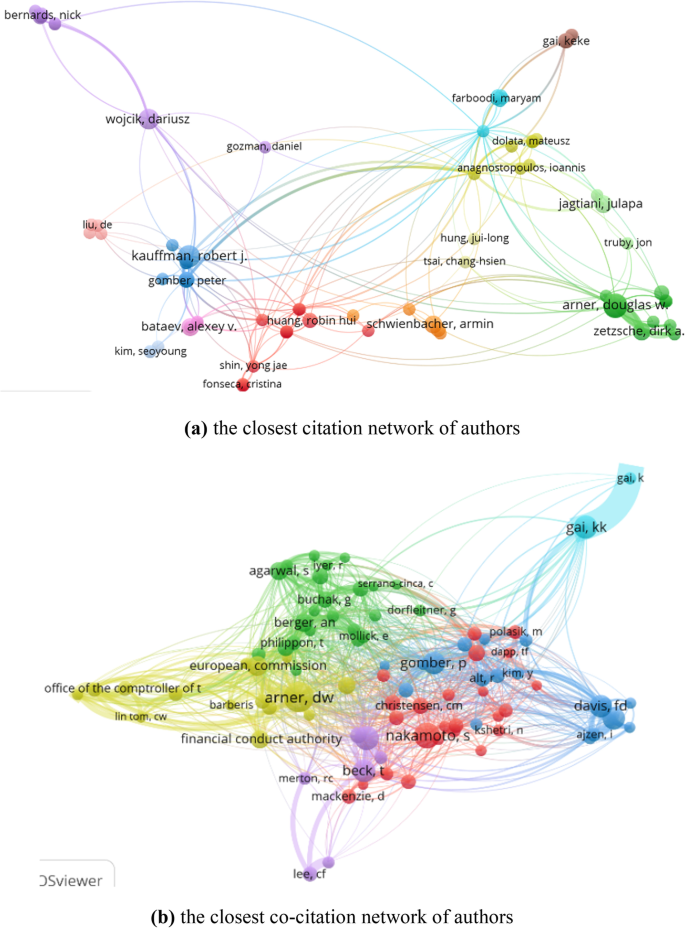
The citation and co-citation network of authors (generated using Vosviewer on data)
For more details, Tables 6 and 7 presents the top 10 most cited authors and most co-cited authors based on some important indicators, including TP, TC, links (the number of the authors cited together), the total link strength (the weights of links), and cluster.
The most cited paper with 158 citations is not included in Table 6 because AN Berger has only published one document in the field of FinTech. According to the results, among the authors who have published more than 2 papers, RJ Kauffman is the most influential with 6 publications and 675 citations, which is obvious in Fig. 11 a. Kauffman focused on researching the FinTech revolution including mobile payment and cards, evaluating changes and transformations in different areas of financial services (Au and Kauffman 2008 ; Gomber et al. 2018 ; Kauffman et al. 2017 ). Additionally, DW Arner is the most co-cited author in the co-citation network (the number of citations is 104). 79 authors have been cited with him. Arner focuses on digital financial service and its regulation, sustainability features, and RegTech (Arner et al 2017 , 2020 ; Zhou et al. 2015 ). Furthermore, the citation network and co-citation network of journals are illustrated. The corresponding indexes are listed in Table 8 . The meanings of the nodes, sizes of the nodes, the links, and their thickness are similar to that of the authors.
From Fig. 12 , the top cited journals are Electronic Commerce Research and Applications , Journal of Money Credit and Banking , Social Studies of Science , Accounting Organizations and Society , Journal of Economics and Business , Business Horizons , and Financial Innovation . The top co-cited journals include Journal of Finance , Management Science , MIS Quarterly , Review of Financial Studies , Journal of Financial Economics , Journal of Banking & Finance , Electronic Commerce Research and Applications , American Economic Review , and Strategic Management Journal . Next, combining the top citation journals with the top prolific journals (Table 5 ), we selected three important journals belonging to Q1 ( Electronic Commerce Research and Applications , Journal of Management Information Systems , and Financial Innovation ) to represent their research focus and help scholars conduct targeted research based on the co-occurrence analysis of author keywords, as shown in Fig. 13 . We obtained 84, 50, and 33 author keywords of the three journals, respectively. Except for the general keyword, i.e., FinTech, the foci of Electronic Commerce Research and Applications include cryptocurrency, blockchain, digital economy, mobile payment, and bitcoin. The top hot topics of the Journal of Management Information Systems are business models, crowdfunding, P2P lending and bitcoin. For Financial Innovation , big data, blockchain, and digital banking are popular research topics. Combining with Fig. 5 and Table 3 , the main research subfields are obvious.
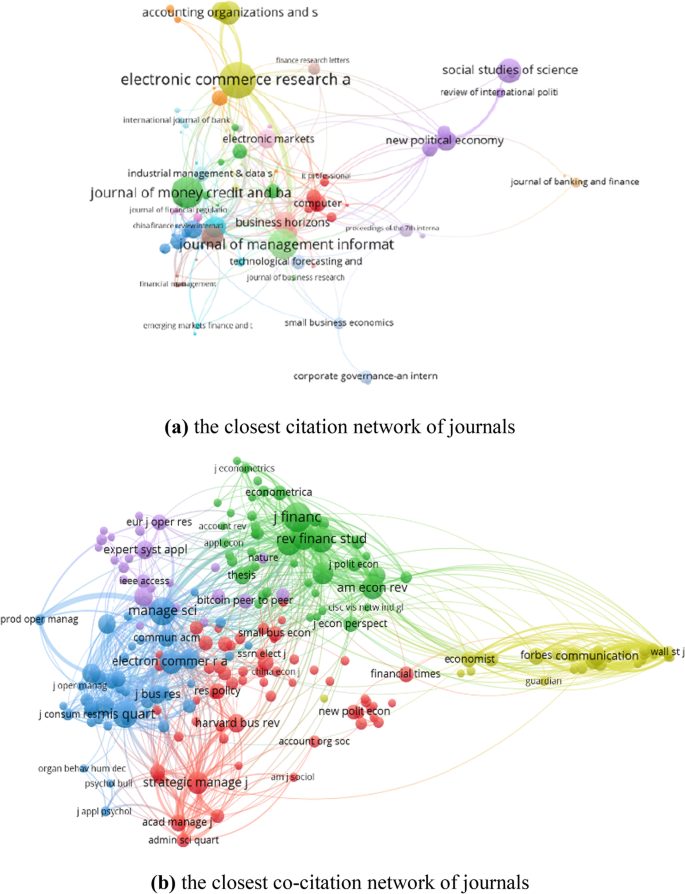
The citation and co-citation network of journals (generated using Vosviewer on data)
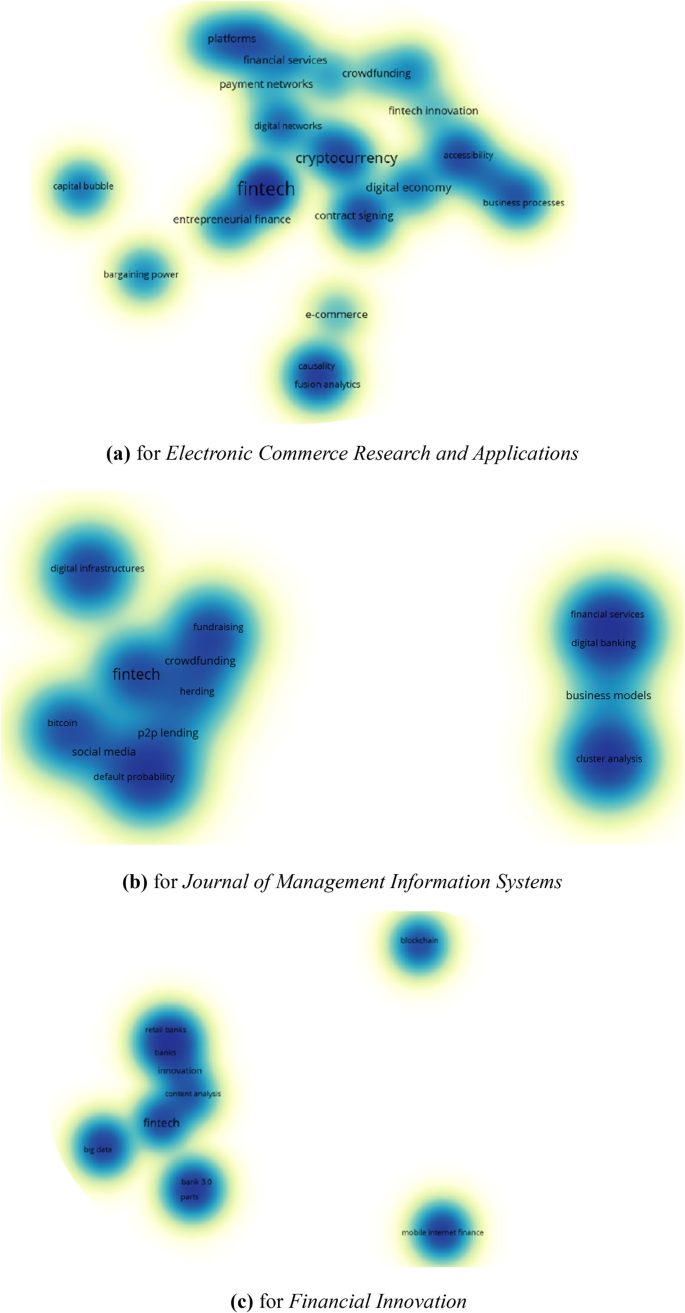
The research topics of top important journals (generated using Vosviewer on data)
Then, this paper conducted a the burst detection analysis (a popular method that can reflect the explosive data attracted attention by the academic in a certain period) (Xu et al. 2019 ; Kleinberg 2003 ) for the cited authors, journals, and references. It can be used to reflect the dynamic changes of publications in the field of FinTech.
The visualization of the cited authors is shown in Fig. 14 . The node and its size denote the cited authors and citation, respectively. The red nodes represent the authors with the strongest citation bursts. As a result, we obtained 6 terms with the strongest citation bursts; one of them is the name of a forum, the World Economic Forum. Thus, Table 8 lists the other 5 cited authors with the strongest citation bursts.
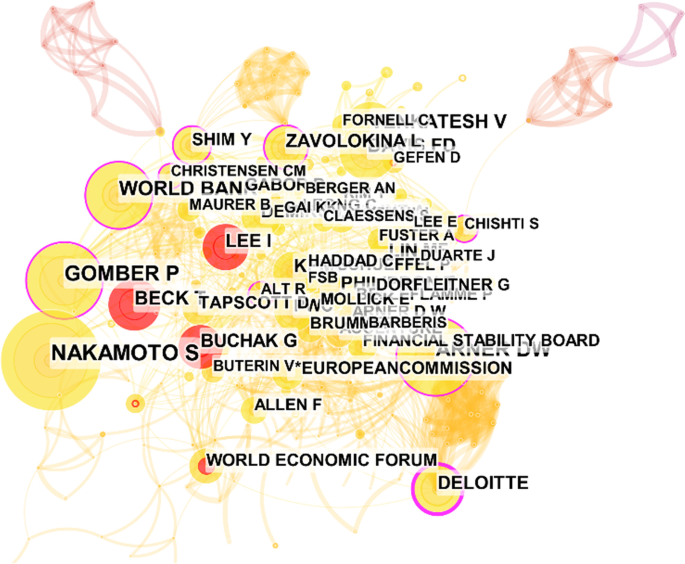
The visualization of the cited authors (generated using CiteSpace on data)
From Table 8 , we have noticed that most of the research was done recently. Not surprisingly, only a few authors have the strongest citation bursts and are all close to the present. I Lee, G Buchak, and T Beck have been closed to 2021. Of the 848 publications, I Lee published only one paper, FinTech: Ecosystem, business models, investment decisions, and challenges . It introduced a historical view of FinTech; discussed the ecosystem of the FinTech sector, various FinTech business models, and investment types; and illustrated real options for FinTech investment decisions (Lee and Shin 2018 ). The paper, FinTech, regulatory arbitrage, and the rise of shadow banks , published by G Buchak, studied the contributions of regulatory differences and technological advantages to the growth of the shadow bank market (Buchak et al. 2018 ). FinTech has widespread applications in various fields and is still expanding.
According to CiteSpace, 14 journals have been cited frequently in a certain period (Table 9 ). The citation frequency for most of the journals has increased since 2016. The strength of the Financial Times is the strongest with a value of 8.2555. Financial Times is a newspaper edited in London and has a strong influence on the financial policies of the British government. For academic journals, the strength of the Harvard Business Review is the strongest with 5.2399. It focuses on leadership, organizational change, negotiation, strategy, operations, marketing, finance, and managing people. The citation burst of the cited journal of Economic and Social Review has the longest duration of 12 years from 2006 to 2017. Moreover, only one has continued until 2021, i.e., Business Horizons .
Next, we studied the relationship between references related to FinTech. Based on CiteSpace, the reference network is constructed as shown in Fig. 15 . Similarly, the red node is the reference with the strongest citation burst. There is only one reference with the strongest citation burst (its strength is 3.6071), i.e., Social media analytics for enterprise: typology, methods and processes . It provided an overview of social media analytics for managers (Lee 2018 ), and was published on Business Horizons in 2018. As listed in Table 10 , the duration result begins in 2019 and continues to 2021, which reflects that its influence is continuing in the field of FinTech.
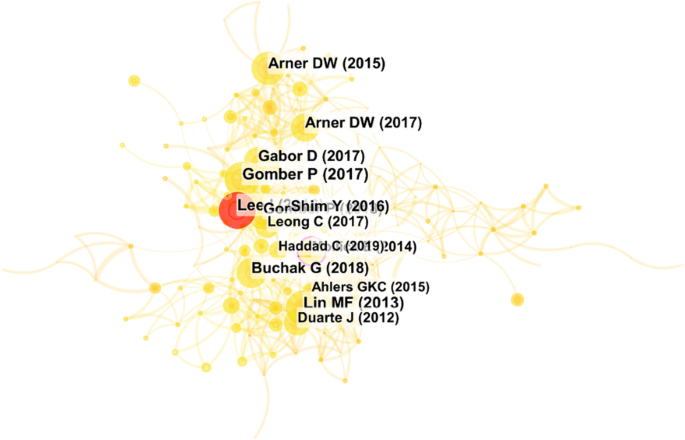
The visualization of the reference network (generated using CiteSpace on data)
To show the trends of the documents more intuitively, Fig. 16 presents the overlay analysis (Nita 2019 ). It is divided into two parts, i.e., citing (left part) and cited (right part). The curves denote the citation connections. For the oval in Fig. 15 , the horizontal axis and the vertical axis reflect the numbers of authors and documents, respectively. The more papers published in journals in specific fields, the longer the vertical axis. The greater the number of authors, the longer the horizontal axis. On the left, we can see that the journals mainly belong to cluster 1 (mathematics, systems, mathematical), cluster 6 (psychology, education, health) and cluster 10 (economics, economic, political). Correspondingly, the number of authors is large. For the cited part, the references are involved in many areas, for instance, chemistry, materials, physics (cluster 4), environment, toxicology, nutrition (cluster 2), molecular, biology and genetics (cluster 8). Of course, they are mainly concentrated in the same three areas as the left. In comparison, the research in the field of FinTech has a widespread impact on many fields and is still expanding.
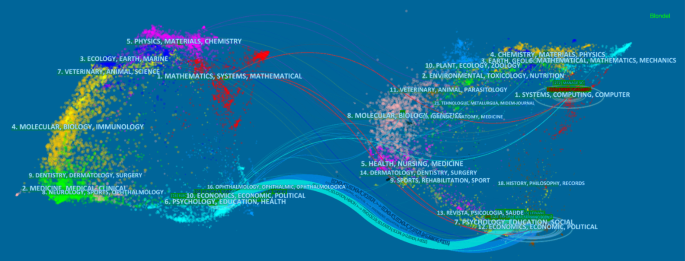
Overlay analysis of all 848 publications (generated using CiteSpace on data)
FinTech is still at an early stage. Combining with the theme view, co-occurrence networks, and a timeline view of author keywords, the related research areas mainly involve lending, blockchain, machine learning, big data, financial regulation, and financial inclusion. The research not only promotes scientific and technological progress but also plays a key role in economic development. To clarify the challenges and possible opportunities reasonably in the future, this section combines the derived documents and the characteristics of the current economic environment. Subsequently, the challenges and development prospects are discussed, especially from the perspective of the impact of big data and COVID-19 on FinTech.
As a critical technology and one of the research topics closely related to FinTech (see Fig. 6 ), big data has a great influence on reshaping the market by introducing new algorithmic technologies and is the key production element of the digital economy and digitalization (Gruin 2020 ). It is the best technical support for financial innovation. The integration of technologies such as big data and cloud computing has promoted the rapid development of the Internet of Things, which has realized the interconnection and intercommunication of people, people and things, and things and things, leading to explosive growth in the amount of data.
As the core means of production and production factors of Digital Economy 2.0, the value of data needs to be realized by the technology cluster of the supporting layer, including artificial intelligence, blockchain, and AR/VR. Data intelligence is the core of future financial (Gai et al. 2018a , b ).
With the development of the social economy, progressively more financial companies are beginning to build their big data platforms, from banks to P2P to insurance and securities. Ensuring data security and improving data usage efficiency, distinguishing and filtering the interfering elements, and obtaining more effective models or financial products will play a vital role (Hung et al. 2020 ). For example, (1) banking will analyze behavior data of clients, including deposits, withdrawals, and electronic transfers, and then conduct marketing, financial product innovation, and satisfaction degree survey to send the targeted advertising information; (2) for machine learning, good data help improve the capability to predict future situations based on known variables in the learning process (Yeh and Chen 2020 ). The important content of big data security and a simple big data platform is shown in Fig. 17 . Footnote 1
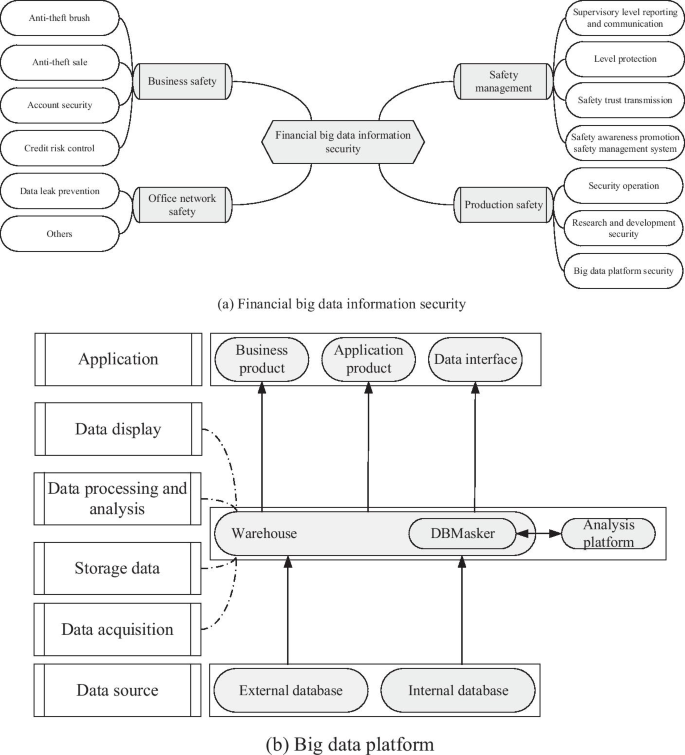
Big data security
Influence of COVID-19 on FinTech
For various fields of research, it is important to identify the intrinsic features of complex data and use them, not limited to financial big data (Huang et al. 2021 ). At present, because of the impact of the pandemic, FinTech products and services face many uncertainties and unpredictable risks because many banks and financial institutions had offered online loan application services based on remote data during the COVID-19 pandemic. Najaf et al. ( 2021 ) proposed that the COVID-19 had brought a drastic change in the key determinants of P2P lending. Chen et al. ( 2021 ) investigated the impact of FinTech products on commercial banks’ performance in China. The impact of COVID-19 on financial constraints and the moderating effect of financial technology are examined by Ling et al. ( 2021 ). The development of FinTech can alleviate the negative impact of COVID-19 on corporate financial constraints.
On one hand, the explosion of the pandemic encourages the company to review its products and progress. The importance of financial regulation is self-evident (Yi et al. 2020 ). On the other hand, enterprises and banks should expand current products and create new service lines to accelerate the transition to e-commerce. The transition pushes enterprises and banks to reexamine and reconstruct the digital strategies aimed to master new opportunities and digital customers.
Taking lending as an example, because of online operations, it is more difficult to obtain complete client information compared with traditional face-to-face work, which can result in many malicious fraudulent loans. This will not only affect the development of enterprises but also cause a decline in the post-loan management ability. Furthermore, from the top 5 most cited authors with the strongest citation bursts by 2021, Buchak was ranked second with research on how two forces, regulatory differences and technological advantages, work online. From the top 10 most cited authors, Arner was ranked second and presented that the financial system requires increasing the use and reliance on RegTech (Arner et al. 2017 ). We confirmed the importance of financial regulation in the field of FinTech. Additionally, here is a challenge for financial regulators to achieve network security and decrease current online microfinance. That is, from the perspective of financial risk, how to effectively use technology, improve supervision tools, and optimize supervision paths is another challenge.
Research feature and development prospects
The research hotspots in the field of FinTech mainly focus on specific technologies in practice and emphasize the role of the finance field. This causes insufficient theoretical discussions and neglects the innovation of technology itself. FinTech is the integration of finance and technology, the latter pushes the development of the former. Thus, in the next stage, how to jointly promote the innovation of finance and technology and achieve deep combination is the third challenge proposed in this paper.
However, with the advancement of big data, cloud computing, artificial computing, and blockchain, FinTech still has many opportunities and broad prospects for development. The core is to introduce new elements and combine them with multiple disciplines to promote the technology level. Specifically, (1) improving the modern regulation system and highlighting risk management methods help accelerate the realization of effective financial regulation and promote the stable operation of financial institutions legally. For example, under the mobile payment and artificial intelligence environment, ensuring consumer safety and avoiding information leakage is the main task of enterprises and banks at present and in the future (Tritto et al. 2020 ); (2) as analyzed above, the transformation triggered by emerging technologies in the FinTech has been mainly manifested in the technology applications; however, it should dig deeper into more basic theories (Mao et al. 2019 ); (3) with the assistance of artificial intelligence and machine learning, studying the predictive procedures with high accuracy, stability and robustness will benefit the financial market, like predicting capital markets (Alam et al. 2020 ) and the stock market. Additionally, the corresponding fuzzy decision-making theories and methods are helpful (Liang et al. 2017 ; Xu and Wang 2016 ). Similarly, strengthening further integration with big data will make it easier to quantify subjective and objective indicators, such as sentiment indicators.
Conclusions
In this paper, we have presented an overall analysis of publications in the field of FinTech up to 2020. Based on WoS, we obtained 848 publications; the first document was published in 1996. Even though this topic appeared early, with the advancement of the economy and technology, the real explosions of research occurred in 2015 (see Fig. 2 ). From various aspects, this paper investigated the characteristics of all publications in the field of FinTech based on visualization tools.
First, the development of FinTech benefits from common progress in many fields, such as blockchain, big data, machine learning, artificial intelligence, and digital economy (see Fig. 6 ). Moreover, mobile money is currently a hot topic and will continue to be one (see Fig. 7 ). In light of countries/regions, China has the greatest number of publications, which can be illustrated by the advanced technological environment of China, such as the convenient mobile payment and intelligent life. Meanwhile, it is expected to achieve the progress of citations (see Fig. 3 and Table 2 ). The research hotspots of this field are clear. It can be reflected from different angles: (1) from the top productive countries/regions (see Table 3 ), their research topics concentrate on the blockchain, P2P lending, financial inclusion and regulation; (2) from the popular journals (see Fig. 13 ), Electronic Commerce Research and Applications , Journal of Management Information Systems , and Financial Innovation are the top influential journals. Their research focuses on e-commerce, digital economy, blockchain, big data, and banking; (3) from the timeline view of author keywords (see Fig. 7 ), and it not only presents the popular topics but also illustrates their time. Furthermore, burst detection analysis and overlay analysis give impetus to the scholars who are interested in FinTech to grasp the dynamic changes more intuitively. With the overall results, the current challenges (lending, risk management, and financial regulators) and future possible research directions and extensions (introducing uncertain decision making and speeding up the connection with machine learning and big data) are discussed.
In general, the findings in this paper play a key role in the next stage and encourage scholars to conduct further studies. However, because the publications presented in this paper are limited to the WoS score database and the search keywords are related to FinTech and financial technology, the content needs to be enriched in the future. We will pay more attention to the innovation research of FinTech and its dynamic development.
Availability of data and materials
Data used in this paper were collected from Web of Science Core Collection.
https://www.sohu.com/a/163585024_621613
Admati AR, Hellwig M (2013) The Bankers’ New Clothes. Princeton University Press
Google Scholar
Agarwal S, Zhang J (2020) FinTech, lending and pay innovation: a review. Asia-Pac J Financ St 49:353–367
Article Google Scholar
Alam TM, Shaukat K, Mushtaq M, Ali Y, Khushi M, Luo S, Wahab A (2020) Corporate bankruptcy prediction: an approach towards better corporate world. Comput J. https://doi.org/10.1093/comjnl/bxaa056
Arner DW, Barberis J, Buckley RP (2017) FinTech, RegTech, and the reconceptualization of financial regulation. NW J Int Law Bus 37(3):371–413
Arner DW, Douglas W, Buckley RP, Zetzsche DA (2020) Sustainability, fintech and financial inclusion. Eur Buw Organ Law Re 21(1):7–35
Au YA, Kauffman RJ (2008) The economics of mobile payments: understanding stakeholder issues for an emerging financial technology application. Electron Comme R A 7(2):141–164
Belanche D, Casalo LV, Flavian C (2019) Artificial intelligence in FinTech: understanding robo-advisors adoption among customers. Ind Manage Data Syst 119(7):1411–1430
Berger AN (2003) The economic effects of technological progress: evidence from the banking industry. J Money Credit Bank 35(2):141–176
Borgman CL, Furner J (2002) Scholarly communication and bibliometrics. Annu Rev Inform Sci 36:3–72
Buchak G, Matvos G, Piskorski T, Seru A (2018) FinTech, regulatory arbitrage, and the rise of shadow banks. J Financ Econ 130(3):453–483
Cao LB, Yuan G, Leung T, Zhang W (2020) Special issue on AI and FinTech: the challenge ahead. IEEE Intell Syst 35(3):3–6
Castiglione A, Pizzolante R, De Santis A, Carpentieri B, Castiglione A, Palmieri F (2015) Cloud-based adaptive compression and secure management services for 3D healthcare data. Future Gener Comput Syst 43:120–134
Castiglione A, De Santis A, Soriente C (2007) Taking advantages of a disadvantage: digital forensics and steganography using document metadata. J Syst Softw 80(5):750–764
Chen ZM, Li YS, Wu YW, Luo JJ (2017) The transition from traditional banking to mobile internet finance: an organizational innovation perspective—a comparative study of Citibank and ICBC. Financ Innov 3(1):1–16
Chen MA, Wu QX, Yang BZ (2019) How valuable is FinTech innovation? Rev Financ Stud 32(5):2062–2106
Chen CM (2006) CiteSpace II: detecting and visualizing emerging trends and transient patterns in scientific literature. J Am Soc Inf Sci Tec 57(3):359–377
Chen XH, You XY, Chang V (2021) FinTech and commerical banks? Performance in China: a leap forward or survival of the fittest? Technol Forecast Soc. https://doi.org/10.1016/j.techfore.2021.120645
Cobo MJ, Lopez-Herrera AG, Herrera-Viedma E, Herrera F (2011) Science mapping software tools: review, analysis, and cooperative study among tools. J Am Soc Inf Sci Tec 62(7):1382–1402
Ding Y, Chowdhury GG, Foo S (2001) Bibliometric cartography of information retrieval research by using co-word analysis. Inform Process Manag 37(6):817–842
Falagas ME, Pitsouni EI, Malietzis GA, Pappas G (2008) Comparison of PubMed, Scopus, Web of Science, and Google Scholar: strengths and weaknesses. FASEB J 22(2):338–342
Gai KK, Qiu M, Tao L, Zhu Y (2016) Intrusion detection techniques for mobile cloud computing in heterogeneous 5G. Secur Commun Netw 9(16):3049–3058
Gai KK, Qiu MK, Sun XT (2018a) A survey on FinTech. J Netw Comput Appl 103:262–273
Gai KK, Qiu MK, Zhao H (2018b) Energy-aware task assignment for mobile cyber-enabled applications in heterogeneous cloud computing. J Parallel Distrib Comput 111:126–135
Ge RY, Feng J, Gu B, Zhang PZ (2017) Predicting and deterring default with social media information in peer-to-peer lending. J Manage Inform Syst 34(2):401–424
Gomber P, Kauffman RJ, Parker C, Weber BW (2018) On the FinTech revolution: interpreting the forces of innovation, disruption, and transformation in financial services. J Manag Inf Syst 35(1):220–265
Gruin J (2020) The epistemic evolution of market authority: Big data, blockchain and China’s neostatist challenge to neoliberalism. Compet Change. https://doi.org/10.1177/1024529420965524
Huang SC, Wu CF, Chiou CC, Lin MC (2021) Intelligent FinTech data mining by advanced deep learning approaches. Comput Econ. https://doi.org/10.1007/s10614-021-10118-5
Hung JL, He W, Shen JC (2020) Big data analytics for supply chain relationship in banking. Ind Market Manag. https://doi.org/10.1016/j.indmarman.2019.11.001
Iman N (2018) Is mobile payment still relevant in the fintech era? Electron Commer R A 30:72–82
Kauffman RJ, Kim K, Lee SYT, Hoang AP, Ren J (2017) Combining machine-based and econometrics methods for policy aalytics insights. Electron Commer R A 25:115–140
Kleinberg J (2003) Bursty and hierarchical structure in streams. Data Min Knowl Discov 7(4):373–397
Kou G, Peng L, Wang GX (2014) Evaluation of clustering algorithms for financial risk analysis using MCDM methods. Inform Sci 275:1–12
Laengle S, Merigo JM, Miranda J, Slowinski R, Bomze I, Borgonovo E, Dyson RG, Oliveira JF, Teunter R (2017) Forty years of the European Journal of Operational Research: a bibliometric overview. Eur J Oper Res 262(3):803–816
Liang DC, Xu ZS, Liu D, Wu Y (2017) Method for three-way decisions using ideal TOPSIS solutions at Pythagorean fuzzy information. Inform Sciences 435:282–295
Ling SX, Per TY, Li ZH, Zhang ZP (2021) Impact of COVID-19 on financial constraints and the moderating effect of financial technology. Emerg Mark Financ Tr 57(6):1675–1688
Lee I, Shin YJ (2018) FinTech: ecosystem, business models, investment decisions, and challenges. Bus Horizons 61(1):35–46
Lee I (2018) Social media analytics for enterprise: typology, methods and processes. Bus Horizons 61(2):199–210
Li B, Xu ZS (2021) A comprehensive bibliometric analysis of financial innovation. Econ Res-Ekon Istraz. https://doi.org/10.1080/1331677X.2021.1893203
Li Y, Xu ZS, Wang XX, Wang XZ (2020) A bibliometric analysis on deep learning during 2007–2019. Int J Mach Learn Cyb. https://doi.org/10.1007/s13042-020-01152-0
Liu WS, Liao HC (2017) A bibliometric analysis of fuzzy decision research during 1970–2015. Int J Fuzzy Syst 19(1):1–14
Lou J, Tian SJ, Niu SM, Kang XQ, Lian HX, Zhang LX, Zhang JJ (2020) Coronavirus disease 2019: a bibliometric analysis and review. Eur Rev Med Pharmacol 24(6):3411–3421
Loubere N (2017) China’s internet finance boom and tyrannies of inclusion. China Perspect 4:9–18
Mao XB, Wu M, Dong JY, Wan SP, Jin Z (2019) A new method for probabilistic linguistic multi-attribute group decision making: application to the selection of financial technologies. Appl Soft Comput 77:155–175
Merigo J, Rocafort A, Juan PA (2016) Bibliometric overview of business & economics research. J Bus Econ Manag 17(3):397–413
Merediz-Solà I, Bariviera AF (2019) A bibliometric analysis of Bitcoin scientific production. Res Int Bus Financ 50:294–305
Miau S, Yang JM (2018) Bibliometrics-based evaluation of the Blockchain research trend: 2008-March 2017. Technol Anal Strateg 30(9):1029–1045
Mourao PR, Martinho VD (2020) Choosing the best socioeconomic nutrients for the best trees: a discussion about the distribution of Portuguese Trees of Public Interest. Environ Dev Sustain. https://doi.org/10.1007/s10668-020-00858-z
Najaf K, Subramaniam RK, Atayah OF (2021) Understanding the implications of FinTech peer-to-peer (P2P) lending during the COVID-19 pandemic. J Sustain Finance Invest. https://doi.org/10.1080/20430795.2021.1917225
Nasir A, Shaukat K, Khan KI, Hameed IA, Alam TM, Suhuai L (2021) What is core and what future holds for bolckchain technologies and cryptocurrencies: a bibliometric analysis. IEEE Access 9:989–1004
Nasir A, Shaukat K, Hameed IA, Luo SH, Mahboob T, Iqbal F (2020) A bibliometric analysis of corona pandemic in social sciences: a review of influential aspects and conceptual structure. IEEE Access 8:133377–133402
Nita A (2019) Empowering impact assessments knowledge and international research collaboration—a bibliometric analysis of Environmental Impact Assessment Review journal. Environ Impact Asses 78:1–10
Pinochet LHC, Diogo GT, Lopes EL, Herrero E, Bueno RLP (2019) Propensity of contracting loans services from FinTech’s in Brazil. Int J Bank Mark 37(5):1190–1214
Philippon T (2016) The Fintech opportunity. NBER Working Paper
Qin Y, Wang XX, Xu ZS, Share M (2021) The impact of poverty cycles on economic research: evidence from econometric analysis. Econ Res-Ekon Istraz 34(1):152–171
Qiu M, Cao D, Su H, Gai K (2015) Data transfer minimization for financial derivative pricing using Monte Carlo simulation with GPU in 5G. Int J Commun Syst 11(16):2364–2374
Ronner AE, Trappeniers DAM (1996) Currency exposure management within Philips. Financial and Monetary Policy Studies 32:171-+
Ruan JH, Wang YX, Chan FTS, Hu XP, Zhao MJ, Zhu FW, Shi BF, Shi Y, Lin F (2019) A life-cycle framework of green IoT-Based agriculture and its finance, operation and management issues. IEEE Commun Mag 57(3):90–96
Stopar K, Bartol T (2019) Digital competences, computer skills and information literacy in secondary education: mapping and visualization of trends and concepts. Scientometrics 118(2):479–498
Singh S, Sahni MM, Kovid RK (2020) What drives FinTech adoption? A multi-method evaluation using an adapted technology acceptance model. Manag Decision. https://doi.org/10.1108/MD-09-2019-1318
Suryono RR, Budi I, Purwandari B (2021) Detection of Fintech P2P lending issues in Indonesia. Heliyon 7(4):e06782
Suryono RR, Budi I, Purwandari B (2020) Challenges and trends of financial technology (Fintech): a systematic literature review. Information 11(12):590
Tritto A, He YJ, Junaedi VA (2020) Governing the gold rush into emerging markets: a case study of Indonesia’s regulatory responses to the expansion of Chinese-backed online P2P lending. Financ Innov. https://doi.org/10.1186/s40854-020-00202-4
Van Eck NJ, Waltman L (2010) Software survey: VOSviewer, a computer program for bibliometric mapping. Scientometrics 84(2):523–538
Wamba SF, Kamdjoug JRK, Bawack RE, Keogh JG (2020) Bitcoin, blockchain and FinTech: a systematic review and case studies in the supply chain. Prod Plan Control 31(2–3):115–142
Wang H, Xu ZS, Zeng XJ (2018) Modeling complex linguistic expressions in qualitative decision making: an overview. Knowl-Based Syst 144:174–187
Wang HM, Kou G, Peng Y (2020a) Multi-class misclassification cost matrix for credit rating in peer-to-peer lending. J Oper Res Soc 72(2):1–12
Wang XX, Xu ZS, Share M (2020b) A bibliometric analysis of Economic Research-Ekonomska Istrazivanja (2007–2019). Ekono Istraz 33(1):865–886
Wang XX, Chang YR, Xu ZS, Wang ZD, Kadirkamanathan V (2021) 50 Years of international journal of systems science: a review of the past and trends for the future. Int J Syst Sci 52(8):1515–1538
Wen S, Zhou W, Zhang J, Xiang Y, Zhou W, Jia W (2013) Modeling propagation dynamics of social network worms. IEEE Trans Parallel Distrib Syst 24(8):1633–1643
Xu M, Chen XT, Kou G (2019) A systematic review of blockchain. Financial Innov 5(1):1–14
Xu ZS, Wang H (2016) On the syntax and semantics of virtual linguistic terms for information fusion in decision making. Informaiton Fusion 34:43–48
Yang W, Sui XP, Qi Z (2020) Can fintech improve the efficiency of commercial banks? An analysis based on big data. Res Int Bus Financ. https://doi.org/10.1016/j.ribaf.2020.101338
Yeh JY, Chen CH (2020) A machine learning approach to predict the success of crowdfunding fintech project. J Enterp Inf Manag. https://doi.org/10.1108/JEIM-01-2019-0017
Yin H, Gai KK (2015) An empirical study on preprocessing high-dimensional class-imbalanced data for classification. In: Proceedings of the IEEE international symposium on big data security on cloud, New York, USA, pp 1314–1319
Yi XJ, Sheng K, Yu T, Wang YY, Wang SH (2020) R&D investment and financing efficiency in Chinese environmental protection enterprises: perspectives of COVID-19 and supply chain financial regulation. Int J Logist-Res App. https://doi.org/10.1080/13675567.2020.1856800
Yu DJ, Xu ZS, Pedrycz W, Wang WR (2017) Information sciences 1968–2016: a retrospective analysis with text mining and bibliometric. Inform Sciences 418:619–634
Yu DJ, Xu ZS, Kao YS, Lin CT (2018) The structure and citation landscape of IEEE Transactions on Fuzzy Systems (1994–2015). IEEE Trans Fuzzy Syst 26(2):430–442
Zhang J, Chen C, Xiang Y, Zhou W, Xiang Y (2013) Internet traffic classification by aggregating correlated naive Bayes predictions. IEEE Trans Inf Forensics Secur 8(1):5–15
Zhang Y, Soong B (2004) Performance evaluation of GSM/GPRS networks with channel re-allocation scheme. IEEE Commun Lett 8(5):280–282
Zhou WH, Arner DW, Buckley RP (2015) Regulation of digital financial services in china: lase mover advantage? Tsinghua China Law Rev 8(1):26–61
Download references
Acknowledgements
Not applicable.
This work was funded by the National Natural Science Foundation of China under Grant 71771155.
Author information
Authors and affiliations.
College of Statistics and Mathematics, Zhejiang Gongshang University, Hangzhou, 610064, China
Business School, Sichuan University, Chengdu, 610064, China
You can also search for this author in PubMed Google Scholar
Contributions
BL contributed by retrieving literature, conducting data analysis and writing the paper. ZX contributed by writing the paper and checking the language. Both authors read and approved the final manuscript.
Corresponding author
Correspondence to Zeshui Xu .
Ethics declarations
Competing interests.
The authors declare that they have no competing interests.
Additional information
Publisher's note.
Springer Nature remains neutral with regard to jurisdictional claims in published maps and institutional affiliations.
Rights and permissions
Open Access This article is licensed under a Creative Commons Attribution 4.0 International License, which permits use, sharing, adaptation, distribution and reproduction in any medium or format, as long as you give appropriate credit to the original author(s) and the source, provide a link to the Creative Commons licence, and indicate if changes were made. The images or other third party material in this article are included in the article's Creative Commons licence, unless indicated otherwise in a credit line to the material. If material is not included in the article's Creative Commons licence and your intended use is not permitted by statutory regulation or exceeds the permitted use, you will need to obtain permission directly from the copyright holder. To view a copy of this licence, visit http://creativecommons.org/licenses/by/4.0/ .
Reprints and permissions
About this article
Cite this article.
Li, B., Xu, Z. Insights into financial technology (FinTech): a bibliometric and visual study. Financ Innov 7 , 69 (2021). https://doi.org/10.1186/s40854-021-00285-7
Download citation
Received : 13 October 2020
Accepted : 13 August 2021
Published : 06 October 2021
DOI : https://doi.org/10.1186/s40854-021-00285-7
Share this article
Anyone you share the following link with will be able to read this content:
Sorry, a shareable link is not currently available for this article.
Provided by the Springer Nature SharedIt content-sharing initiative
- Bibliometric analysis
- Citation structure
- Development trends
- Visualization networks
Financial data unbound: The value of open data for individuals and institutions
As countries around the world look to ensure rapid recovery once the COVID-19 crisis abates, improved financial services are emerging as a key element to boost growth, raise economic efficiency, and lift productivity. Robust digital financial infrastructure proved its worth during the crisis, helping governments cushion people and businesses from the economic shock of the pandemic. The next frontier is to create an open-data ecosystem for finance.
Already, technological, regulatory, and competitive forces are moving markets toward easier and safer financial data sharing . Open-data initiatives are springing up globally, including the United Kingdom’s Open Banking Implementation Entity, the European Union’s second payment services directive , Australia’s new consumer protection laws, Brazil’s drafting of open data guidelines, and Nigeria’s new Open Technology Foundation (Open Banking Nigeria). In the United States, the Consumer Financial Protection Bureau aims to facilitate a consumer-authorized data-sharing market , while the Financial Data Exchange consortium attempts to promote common, interoperable standards for secure access to financial data. Yet, even as many countries put in place stronger digital financial infrastructure and data-sharing mechanisms, COVID-19 has exposed limitations and gaps in their reach, a theme we explored in earlier research .
This discussion paper from the McKinsey Global Institute (download full text in 36-page PDF) looks at the potential value that could be created—and the key issues that will need to be addressed—by the adoption of open data for finance. We focus on four regions: the European Union, India, the United Kingdom, and the United States.
By open data, we mean the ability to share financial data through a digital ecosystem in a manner that requires limited effort or manipulation. Advantages include more accurate credit risk evaluation and risk-based pricing, improved workforce allocation, better product delivery and customer service, and stronger fraud protection.
Our analysis suggests that the boost to the economy from broad adoption of open-data ecosystems could range from about 1 to 1.5 percent of GDP in 2030 in the European Union, the United Kingdom, and the United States, to as much as 4 to 5 percent in India. All market participants benefit, be they institutions or consumers—either individuals or micro-, small-, and medium-sized enterprises (MSMEs)—albeit to varying degrees.
Capturing the full value requires both a level of data standardization and a breadth of data sharing that are not yet current in many economies. Indeed, our research suggests that more than half the potential value remains inaccessible, particularly the value that financial institutions could gain directly through greater efficiency and reduced fraud costs. The use of open data raises questions about user consent, data protection, and cybersecurity. But if these issues are addressed, the innovation such ecosystems could enable would be a spur to economic recovery and broader-based prosperity.
Open financial data create economic value across a wide range of interactions between consumers and financial institutions
Financial data are created or used throughout the life cycle of financial services. They accompany every step of the consumer journey and are used in every action taken by financial institutions as they engage with customers.
Our research identifies seven broad mechanisms through which open financial data can create economic value. Three directly benefit individual and MSME customers: increased access to financial services, greater user convenience, and improved product options. The other four mechanisms directly benefit financial institutions: increased operational efficiency, better fraud protection, improved workforce allocation, and reduced friction in data intermediation.
To size the overall economic value that open financial data can create, we quantify the potential of 24 use cases in banking and payments. We then scale up from these use cases to develop a broader view of the macroeconomic gains.
For consumers in the financial services life cycle, benefits include the following:
- Increased access to financial services. Data sharing enables customers to buy and use financial services to which they might not otherwise have access. For example, where limited data from traditional documentary sources may disqualify consumers from accessing loans, open financial data can help assess the creditworthiness of borrowers by sourcing rent, phone, and utility bills. Individuals and MSMEs with thin files or no formal records can gain access to formal credit, often for the first time. One Experian study showed that including utility data allowed 20 percent of customers with scant documentation in support of their credit application to move on to become “thick-file” customers. Scaling such gains to an economy-wide level, we find that increased access to credit using alternative data could raise an economy’s credit-to-GDP ratio by 20 basis points in the United States and the European Union. In India, the lift could be as much as 130 basis points, the equivalent of about $80 billion to $90 billion in GDP by 2030.
- Greater user convenience. Data sharing saves time for customers in their interactions with their financial services provider. MSMEs can provide documentation faster during customer onboarding, for example. Open access to data on available mortgage products, with applications automatically prefilled, allows consumers to apply for loans without needing to use mortgage brokers. This not only eases the process but also enables customers to benefit from the best rates. In the United Kingdom, which introduced its Open Banking system in 2018, startups use open-banking data to enable quick and easy mortgage applications to all participating providers for free, unlike traditional mortgage brokers who charge arrangement fees.
- Improved product options. Open financial data can broaden and improve the range of product options available to customers, saving them money. For example, an open-data ecosystem makes it easier to switch accounts from one institution to another, helping retail and MSME customers achieve the best yield.
For financial institutions, benefits span the entire life cycle and include the following:
- Increased operational efficiency. Since most data are still found in physical documents or disparate digitized sources, open financial data could cut costs and make it easier to adopt automation technologies, with the associated efficiency boost. This can improve experience for customers by promoting faster and more transparent interactions with providers. In India, for example, the use of the national digital identification system, Aadhaar, for “know your customer” (KYC) verification of retail consumers reportedly reduced costs for financial institutions from about $5 per customer to $0.70. For mortgage underwriting, sharing borrowers’ data allows standard mortgages to go through automated underwriting. Financial data sharing also helps avoid multiple manual data handoffs that lead to errors, rework, and less efficient outcomes. It significantly reduces the costs associated with remediating bad customer relationship management data, currently estimated at 20 percent of a typical financial institution’s income .
- Better fraud prediction. The Association of Certified Fraud Examiners estimates total fraud (including but also extending beyond financial services) at more than $4.5 trillion annually , or the equivalent of about 5 percent of global corporate revenue. Fraud in financial services takes multiple guises, including synthetic and traditional ID fraud, payments fraud, and credit application fraud. Real-time access to customer data can support advanced techniques to identify and reduce costs related to these and other types of fraud. Sharing data provides more evidence and clues with which to flag suspicious activity. This helps institutions build out their predictive modeling of fraud and catch cases earlier. Cifas, a not-for-profit fraud prevention organization in the United Kingdom, says that its members reported more than 350,000 cases of fraud in 2019, preventing fraud totaling £1.5 billion.
- Improved workforce allocation. Companies can use open data to better allocate and target their workforce, assigning staff to the highest-value activities. This helps them better focus their calls on high-risk customers, reduce the time spent monitoring credit of low-risk customers, and ultimately recover more debt.
- Reduced friction from data intermediation. This mechanism is most relevant to financial institutions before they have direct knowledge of a prospective customer, such as in lead generation or loan origination, and so look to acquire and use data from third-party providers. The missing details can range from basic identification data to more behavioral information. Open data systems enable direct access to data by using application programming interfaces (APIs) for data intermediation, which reduces friction. In the United States, for example, where nearly half of all mortgage providers rely on third-party data for mortgage origination, such as credit data, KYC data, and property valuation data. These data can cost as much as $80 per mortgage application, but with open data for finance, much of this information is becoming more publicly available.
Exhibit 1 demonstrates the potential economic gains by region from an example use case linked to each of the seven mechanisms for creating value. The bubbles in blue represent the largest potential gain.
The potential value of open financial data ranges from 1 percent to as much as 5 percent of GDP, depending on economic structure and levels of financial access
Aggregating the potential GDP impact across the 24 use cases to the economy level, we find significant value at stake overall and for all market participants. The total potential GDP impact from open financial data in 2030 is highest for India, at 4 to 5 percent. We estimate the impact for the European Union, the United Kingdom, and the United States to be between 1 and 1.5 percent of GDP (Exhibit 2).
The differences are the result of several factors, typically structural features of economies. Notably, emerging economies tend to have lower levels of financial access and less financial depth, which means the lift in value creation they could achieve with open data is large. India has significant unmet need for retail and MSME credit. It thus has higher economic growth potential for every unit of physical capital added, because open financial data improves credit access.
MSMEs also benefit from time saved in opening accounts; this would translate into higher GDP, assuming the time saved was deployed in market-based economic activities rather than, say, leisure. In India, the time saving accruing to MSMEs could make them the largest beneficiary segment, with more than 60 percent of the potential economic value from our use cases accruing to them.
In the United Kingdom and the United States, by comparison, while we estimate that individuals would capture the largest share of value, financial institutions also have sizable value at stake. In the European Union, financial institutions would gain the largest share of value, nearly 45 percent of the total.
For each region, we find differences in the share of GDP impact in each major step in the financial services life cycle and for each of the seven mechanisms.
India stands out, with a greater share of economic value, about 75 percent of the total, coming from the decisioning and onboarding component of the life cycle, particularly linked to increased access to financial services. This is due to the large potential of opening access to credit to currently excluded individuals and MSMEs. Greater user convenience from simplified onboarding processes for new customers, particularly MSMEs, also saves meaningful amounts of time.
In the European Union, the United Kingdom and the United States, the share of economic value from decisioning and onboarding is lower than that in India, while the share from servicing and monitoring is higher, ranging between 35 and 45 percent of the total. In the United States, the value at stake in relation to decisioning and onboarding is higher than in the European Union because of the size of the credit gap for the country’s many MSMEs.
The main difference between the European Union and the United Kingdom is in the breakdown of potential value associated with servicing and monitoring. In the United Kingdom, we estimate nearly half of this value could flow from improved product options, driven by the potential for MSMEs to increase their deposit yields through easier account switching. In the European Union, where the average MSME is less than half the size of those in the United Kingdom and total MSME savings are lower, the relative potential value coming from account switching is smaller.
Capturing the full potential value of open data for finance requires data standardization and breadth of data sharing
The value creation mechanisms require varying levels of data standardization and breadth of data sharing for their potential to be captured (Exhibit 3).
By standardization, we mean the extent to which standardized mechanisms exist for sharing data and the associated cost of access. In some use cases, data sharing occurs only through ad hoc means. For example, consumers wanting to receive automated access to competitive mortgages need to provide the same specific mortgage application data to multiple providers. To operate at scale, other data-sharing use cases require data to be sourced easily through standardized APIs at minimal cost.
Breadth refers to how broadly data are shared and the mechanisms in place that drive that data sharing. Some use cases work when individuals can request specific data to be shared on an ad hoc basis. For example, consumers can benefit from faster mortgage closure when they are able to grant their prospective lender on-off access to the required data. To operate at scale, other use cases require data sharing over time across a wide range of types of financial data, albeit with consumer consent. An example of an ecosystem with broad data sharing is India, where banks must share all consumer data including personal nonfinancial and financial data at the request of consumers via private but highly standardized APIs developed on a publicly built technology ecosystem known as IndiaStack. Consumers can choose to share their data with digital nonbank lenders via an app to secure loans.
From our research, we see that consumers (both individuals and MSMEs) require moderate levels of standardization and breadth of data sharing to reap the benefits of open data. Institutions, by contrast, could only access full benefits when the level of standardization and breadth of data sharing are higher.
Economies vary in their current levels of data standardization and breadth of data sharing
The type of data-sharing ecosystem used in an economy depends on multiple factors including local market conditions, the robustness of existing digital financial infrastructure, and regulation, including consumer protection laws and mechanisms. In the four economic regions on which we focus, we find significant differences in the degree of standardization and breadth of data sharing (Exhibit 4).
In the European Union and the United Kingdom, for example, we find a high level of standardization combined with relatively less breadth of data sharing. In such data ecosystems, a limited subset of financial data is available via highly standardized and regulated APIs. The data are accessible and usable for third-party service providers such as financial technology firms and other banks. For example, in the United Kingdom, APIs are mandatory for most account and direct debit data, such as address, beneficiary, and payment time stamp. Access to data outside of the subset is more restricted and at the discretion of each individual financial services provider.
In the European Union, the second payment services directive stipulates that data specific to payments be shared via highly standardized APIs. At the same time, separate data privacy regulations restrict the sharing, harvesting, and use of other financial and nonfinancial data, including, for instance, screen scraping, a prevalent form of data gathering in the United States.
For its part, the United States has a broad range of data sharing, but data standardization is more limited. Financial data aggregators broker data flow between providers and users, with limited consumer control. This is primarily because of a lack of strong federal regulation regarding data privacy and a private-market approach to data sharing. This has made private data aggregators the de facto standard setters for how data are shared.
In India, a relatively broad range of data is shared and there is some degree of standardization. Private APIs in India are built by licensed data aggregators on IndiaStack. Data aggregators broker data sharing across institutions using this standard, and consumers can view the data and share directly with payment systems players.
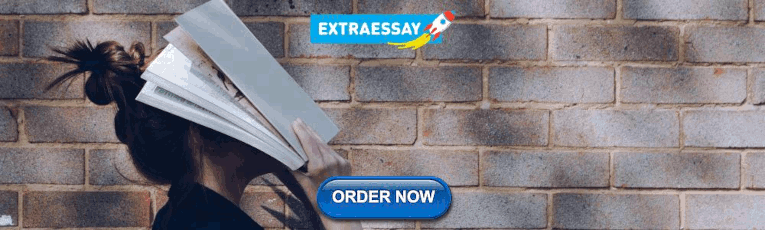
Only low to moderate levels of economic value are accessible with today’s levels of data standardization and data-sharing breadth
A country’s level of data standardization and breadth of data sharing sets the potential value from open financial data it might access today. Exhibit 5 indicates the potential value the European Union, the United Kingdom, the United States, and India could derive from open data, and their current level of standardization and breadth of data sharing. (Click on the country name to change view).
In the European Union, the United Kingdom, and the United States, current data ecosystems leave much of the potential value at stake inaccessible.
Both the United States and the European Union can currently capture only a small fraction of the potential value from open financial data—less than 10 percent, in our estimate. In the United States, the constraint is lack of standardization, while in the European Union it is limited breadth of data sharing. In the United Kingdom, somewhat more value—we estimate between 30 and 40 percent—is currently accessible.
India is better poised to capture value today. Its open data environment positions it to access between 60 and 70 percent of the potential value that open financial data could offer, provided other enablers are in place, as we describe in the next section.
Navigating risk and implementation challenges
Capturing the value from open financial data requires more than sufficient data standardization and breadth of data sharing: users—both consumers and providers—must trust the system, and infrastructure is needed to support it. These factors together provide the groundwork for potential economic gains from open financial data, both those which we size and those that future innovation may make possible.
Well-founded trust. An open-data ecosystem can function effectively only by achieving a level of well-founded trust among all the participants. Without this, market participants—whether individual consumers or businesses—may opt out. Financial data are particularly sensitive, and users are more likely to want to share data if they know what they are sharing and why that sharing is valuable to them. Strong consumer financial protections are also necessary to prevent financial discrimination and foster trust. Absent an automatic or easy mechanism for correction of data errors and updating to reflect changes to life circumstances, problematic data might block an individual or MSME from accessing a needed financial product at a fair price. User trust is also encouraged when threats to cybersecurity are anticipated and mitigated. Breaches can occur during transfer of data, or at any institution involved in the open data ecosystem, such as a bank or fintech. For example, when data transfer is achieved via APIs, a hacker who breaches such an API can hijack any apps that use the interface to collect data. As a result, open APIs require strong customer authentication. Successful data ecosystems tend to have built-in safeguards to ensure privacy and security while giving users access to their personal data, decision rights over who else has access to that data, and transparency about who has accessed it.
Robust financial infrastructure. Financial accounts and digital payment channels, along with digital identification systems with broad population coverage, are critical structural features needed to harness the value of open financial data. High-assurance digital ID facilitates user control of data, privacy protections, security for online interactions, and reduces friction in managing online accounts. Open-data systems without high-assurance digital IDs could make it harder for consumers to keep track of their digital footprint or use their data securely and efficiently. At the same time, digital ID can help provide support strong customer authentication and help control against cyberattacks. For many emerging economies, basic internet access, smartphone penetration, and reliability of electricity are also prerequisites for capturing the full economic value of a data-sharing ecosystem. Shortfalls in these elements limit the value of open financial data that could be captured.
Looking ahead: the role of innovation in open data unbound
Capturing the value accessible today from open financial data will require innovation. At the same time, the more value becomes accessible, through increases in standardization, breadth, or both, the more the potential for innovation will grow, likely beyond use cases we can envisage today.
Market participants will need to develop and scale products and services that address specific use cases, including but not limited to the 24 major ones we profile in this research. That will entail identifying new business opportunities, designing customer value propositions, and scaling new business models across the financial services value chain.
Different types of innovators, from traditional banking incumbents to technology platform-based players and new fintech startups, could all play meaningful roles, focusing on their areas of strength and competitive advantage. The specific types of innovators would vary by market and depend on the structure of the financial data ecosystem. Expanding the boundaries of open-data enablement would make new types of use cases possible, fueling greater innovation and greater value capture.
Olivia White is a McKinsey senior partner in San Francisco, where Tawanda Sibanda is a partner. Anu Madgavkar is a partner in New Jersey for the McKinsey Global Institute, of which James Manyika , based in San Francisco, is co-chair. Tunde Olanrewaju is a senior partner in London. Zac Townsend is an associate partner in Austin, and Scott Kaufman is a consultant in New York.
The authors wish to thank Flourish Ventures, who acted as research collaboration partners, and especially Kabir Kumar, Tilman Ehrbeck, Tina Moran, and Chuck Muckenfuss. They also acknowledge the valuable input and guidance of several individuals and organizations: Nandan Nilekani, co-founder and chairman of Infosys and founding chairman of the Unique Identification Authority of India; Pia Tayag and David Symington at the office of the UN Secretary General’s Special Advocate for Inclusive Finance for Development; Jason Lamb at the Bill and Melinda Gates Foundation; Kimberley Bella and Bob Hedges at Visa; Jo Ann Barefoot at Barefoot Innovation; Jennifer Tescher and Dan Murphy at the Financial Health Network; Aaron Klein at the Brookings Institution; Melissa Koide at FinRegLab; and Nick Cook at the Financial Conduct Authority.
This article was edited by Peter Gumbel, editorial director of the McKinsey Global Institute.
Explore a career with us
Related articles.

COVID-19: Making the case for robust digital financial infrastructure

A new look at how corporations impact the economy and households
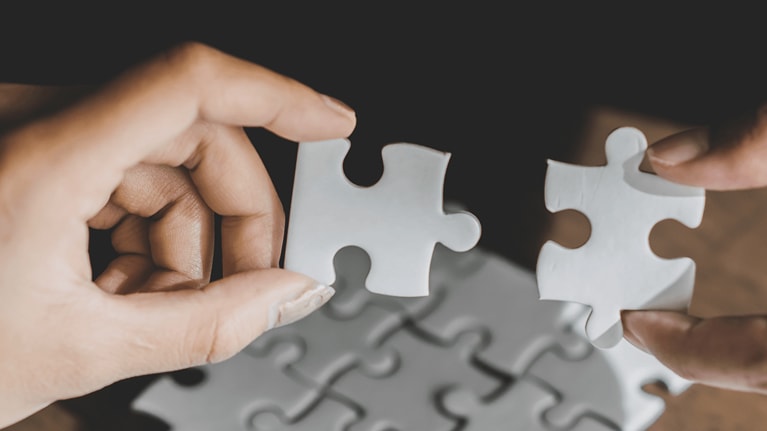
COVID-19 has revived the social contract in advanced economies—for now. What will stick once the crisis abates?
FinancialResearch.gov
Promoting financial stability by delivering high-quality financial data, standards and analysis principally to support the Financial Stability Oversight Council and its member agencies.
Short-term Funding Monitor
This monitor presents a set of curated charts that provide insights into different dimensions of how short-term funding markets are functioning, broken down into four categories: collateral, tenor, volume, and rates.
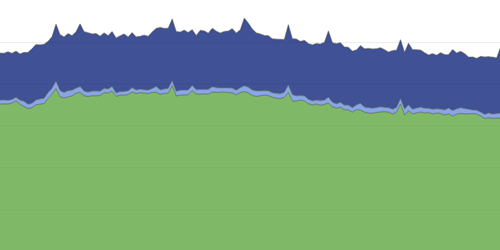
Financial Stress Index
This index is a daily market-based snapshot of stress in global markets. The OFR Financial Stress Index is positive when stress levels are above average, and negative when stress levels are below average.
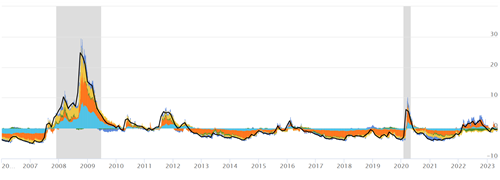
U.S. Money Market Fund Monitor
This monitor tracks the investment portfolios of money market funds by funds' asset types, investments in different countries, counterparties, and other characteristics.
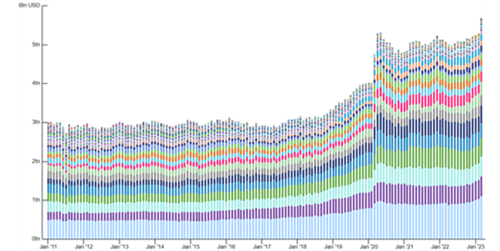
Bank Systemic Risk Monitor
This monitor presents key measures of systemic risks posed by the largest banks, including systemic importance scores for international and U.S. banks, the OFR's Contagion Index, and more.
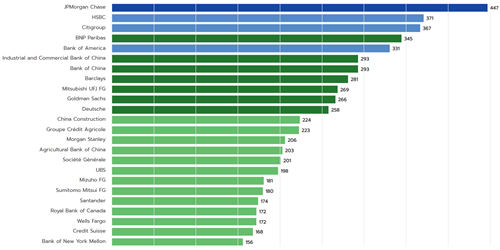
OFR Resources
Ofr highlights.
- Brief: Liquidity Coverage Ratios of Banks During/After COVID-19
- Blog: U.S. Money Market Funds Reach $6.4 Trillion at End of 2023
- Conference: 2024 Rising Scholars Conference: The Future of Financial Stability
- Press Release: 2024 Rising Scholars Conference Announcement
- Blog: OFR Congratulates CFTC and SEC on Initiation of Reporting on UPI for OTC Derivatives
- Blog: Lenders Value Borrower Relationships
- Working Paper: The Value of Lending Relationships
- Brief: The Uneven Distribution of Climate Risks and Discounts
- Blog: Model Shows Network Density Affects Derivatives Trade Costs
- Working Paper: Intermediation Networks and Derivative Market Liquidity
- Blog: Economic Narratives Shape How Investors Perceive Risks
- Working Paper: Crash Narratives
- Brief: Some U.S. Banks May Remain Vulnerable to Losses in Their Securities Portfolios: Introducing Two New Forward-looking Metrics to Assess Future Risk
- Conference: Rising Scholars Call For Papers
- Blog: Wind, Fire, Water, Hail: What Is Going on In the Property Insurance Market and Why Does It Matter?
- Press Release: OFR Finds Elevated Threats to U.S. Financial Stability Due to Inflation, Ongoing Geopolitical Risks, and Global Conflicts
- Report: Office of Financial Research 2023 Annual Report to Congress
- Blog: OFR, University of Auckland Researchers Create Model to Analyze Effects of Trend Inflation on Economic Stability
- Working Paper: Trend Inflation Under Bounded Rationality
- Conference: 2023 Financial Stability Conference
- Public Appearances: Remarks by OFR Acting Director James Martin at the 2023 PhD Symposium
- Blog: Limiting Short Selling During Periods of Individual Stock Stress Reduces Volatility and Raises Prices
- Working Paper: Are Short-selling Restrictions Effective?
- Press Release: OFR Launches LinkedIn Page to Raise Awareness of Financial-Stability Risks
- Blog: Twelve Years of Promoting Financial Stability
- Brief: Work-from-Home and the Future Consolidation of the U.S. Commercial Real Estate Office Sector: The Decline of Regional Malls May Provide Insight
- Press Release: Office of Financial Research Launches Joint Analysis Data Environment for FSOC
- Public Appearances: Remarks by OFR Acting Director James Martin to the Financial Stability Oversight Council
- Joint Analysis Data Environment (JADE)
- Working Paper: The Transition to Alternative Reference Rates in the OFR Financial Stress Index
- Press Release: OFR Updates Financial Stress Index with Alternative Reference Rate Indicators
- Working Paper: Technology Shocks and Predictable Minsky Cycles
- Press release: OFR’s 2023 Interagency Data Inventory Catalogs More Than 500 Data Collections
- Blog: Five Office Sector Metrics to Watch
- From the Director: OFR Plays a Leading Role in Developing Standards for Emerging Technologies
- Blog: OFR Data Analysis Shows High Growth, High Concentration in Digital Asset Market
- Brief: An Early Look into Digital-Assets Regulatory Data
- From the Director: Remarks by James Martin to the Financial Research Advisory Committee
- FRAC: May 18, 2023 meeting of the Financial Research Advisory Committee
- Blog: Threats to Sustainability Stem Particularly from Factors That Stymie Growth and Have High Levels of Risk
- Working Paper: Sustainability with Risky Growth
- From the Director: Update on OFR’s Efforts to Fill Key Gap in Financial Data
- Blog: Why Is So Much Repo Not Centrally Cleared?
- Brief: Why Is So Much Repo Not Centrally Cleared?
- Public Appearances: Remarks of James Martin at the OFR's Rising Scholars Conference
- Blog: OFR Identifies Factors That May Have Contributed to the 2019 Spike in Repo Rates
- Working Paper: Anatomy of the Repo Rate Spikes in September 2019
- Conference: Rising Scholars
- Blog: OFR Creates Model Justifying Long-held View About Inflation Determinants
- Working Paper: Can Supply Shocks be Inflationary with a Flat Phillips Curve?
- Public Appearances: Remarks of James Martin, Acting Director of the Office of Financial Research, at the RegTech 2023 Data Summit
- Press Release: OFR Advances Data-and-Analytics Platform Following Successful Pilot
- Blog: OFR Models One Theory on the Cause of March 2020’s Treasury Market Fragility
- Working Paper: Fragility of Safe Assets
You are now leaving the OFR’s website.
You will be redirected to:
You are now leaving the OFR Website. The website associated with the link you have selected is located on another server and is not subject to Federal information quality, privacy, security, and related guidelines. To remain on the OFR Website, click 'Cancel'. To continue to the other website you selected, click 'Proceed'. The OFR does not endorse this other website, its sponsor, or any of the views, activities, products, or services offered on the website or by any advertiser on the website.
Thank you for visiting www.financialresearch.gov.
Fintech: A content analysis of the finance and information systems literature
- Research Paper
- Published: 03 April 2023
- Volume 33 , article number 2 , ( 2023 )
Cite this article
- Zack Jourdan 1 ,
- J. Ken. Corley 2 ,
- Randall Valentine 3 &
- Arthur M. Tran 3
6306 Accesses
8 Citations
3 Altmetric
Explore all metrics
The amount of research related to financial technologies (fintech) has grown rapidly since these modalities have been implemented. A review of this literature base will help identify the topics that have been explored and identify topics for further research. This research project collects, synthesizes, and analyzes both the research strategies (i.e., methodologies) and content (e.g., topics, focus, categories) of the literature, and then discusses an agenda for future research efforts. We searched for fintech research published in the last 20 years and analyzed 146 articles published in Finance and 70 articles published in Information Systems (IS) during this period in their respective A*, A, and B journals in the 2019 Australian Business Deans Council list. We found an increasing level of activity during the most recent 6-year period and a biased distribution of fintech articles focused on exploratory methodologies. We also found several research strategies that were either underrepresented or absent from the pool of fintech research and identified several subject areas that need further exploration. We also created four fintech topic categories to organize and classify this diverse research stream.
Similar content being viewed by others
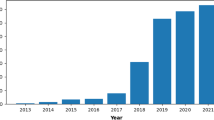
Cryptocurrency trading: a comprehensive survey
Fan Fang, Carmine Ventre, … Lingbo Li
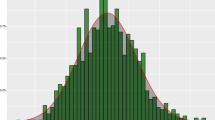
Machine learning for financial forecasting, planning and analysis: recent developments and pitfalls
Helmut Wasserbacher & Martin Spindler
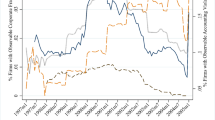
How pervasive is corporate fraud?
Alexander Dyck, Adair Morse & Luigi Zingales
Avoid common mistakes on your manuscript.
Introduction
With the continuous advancements in technology, the current interest in fintech in both academia and in practice is more prevalent than ever. Typically, a portmanteau for “financial technology”, fintech has been referenced for more than 40 years in more than 200 scholarly articles (Schueffel, 2016 ). Throughout the years, different definitions of fintech have been proposed for different contexts and across countries, while the origin of the term “fintech” remains to be a point of contention. Only until recently, Schueffel ( 2016 ) reconciles various existing definitions and defines fintech as “a new financial industry that applies technology to improve financial activities.” As a joint evolution of finance and technology, fintech encompasses cryptocurrencies, Internet banking, mobile payments, crowdfunding, peer-to-peer lending, Robo-Advisory, online identification, and many other important innovations (Lagna & Ravishankar, 2022 ). Nonetheless, fintech is still a relatively undiscovered academic field and expects its definition to continue to evolve. To date, no study has examined neither the methodologies employed nor the content thereof. The purpose of this study is to synthesize the methodologies and content of all fintech article from the past 20 year encompassing all journals on the Australian Business Deans list that have a rating of A*, A, and B. In doing so, we hope to find a synthesis of keywords and methodological advances that can be used in further exploration of fintech research.
Studies which systematically review the literature, such as Farooq and Jibran ( 2018 ), have been shown to be valuable contributions to understanding the scope, measurements, impact size, and determinants of a particular area to synthesize with the area’s future research agenda. In this paper, we performed a meta-analysis of research methods employed in the data stream of fintech research. In the literature stream, there has not been a comprehensive survey of the methodologies employed in fintech literature. In fact, there have been very few studies reviewing the methodologies employed in finance research in the past 15 years, with Kim and Ji ( 2015 ) and Adams et al. ( 2019 ) being the closest examples. Lagna and Ravishankar ( 2022 ) illustrated the growing interest that IS researchers have shown in the fintech research domain. Alt et al. ( 2018 ) called fintech a revolution that had evolved from offline, hierarchical, process-oriented organizations to digital, agile, customer-centric system and stated, “FinTech businesses are more IT companies than financial providers were before.”
The following sections of the paper will examine the current literature to determine what is known about the concept of fintech. The remainder of this paper is organized as follows: a description of the methodology for the analysis of the fintech research is presented. This is followed by the results. Finally, the research is summarized with a discussion of the limitations of this project and suggestions for future research.
- Literature review
One focus in the fintech literature is about how fintech companies provide new and improved financial services. As Thakor ( 1999 ) discusses, the development of information technology enables new financial firms to be highly specialized and provides products and services which are tailored to customer preferences. As new players in the financial market, fintech companies have the potential to reduce financial contracting frictions and increase consumer welfare (Philippon, 2015 ). For example, Fuster et al. ( 2019 ) find evidence that fintechs have improved the productivity of mortgage lending.
These additional values which fintechs may bring to the finance industry come from the fact that these firms are different from traditional financial institutions. Thakor ( 2020 ) discusses that fintech firms bare lower operating costs than traditional banks. For instance, Lending Club, a fintech firm, has operating costs as a percentage of outstanding loans at 2.70% compared to those of banks at almost 7%. According to Benoit et al. ( 2019 ), fintechs also have lower regulatory costs than banks. In the USA, even though peer-to-peer (P2P) lending is subject to the US Securities and Exchange Commission (SEC)’s regulation and state laws, these regulatory burdens are much lighter than that of banks.
Much of the recent fintech research is concerned with how fintechs impact traditional banks. Christensen ( 2016 ) provides the “disruptive theory” in which new entrants effectively compete with traditional players by providing accessible and cost-effective goods and services to customers. Boyd and De Nicolo ( 2005 ) posit that banks become more competitive by providing cheaper loans. In turn, borrowers have less incentive to risk shift which results in banks having less default risk. Similarly, Goetz ( 2018 ) finds that the increased competition forces banks to be more efficient by reducing over-lending and engaging in relationship lending. On the other hand, Bertsch et al. ( 2020 ) find that banks’ increased misconduct is related to the emergence of the US online lending market. Large banks can also choose to acquire fintech firms. For instance, in 2015, Capital One acquired Level Money to strengthen its capabilities in digital banking technologies (Li et al., 2017 ). Hornuf et al. ( 2021 ) find that many banks acknowledge the technical superiority of fintech start-ups and have incorporated these firms’ products and services into their own business models.
Thakor ( 2020 ) and other survey papers review the fintech literature’s research contents of what we currently know about fintech and the research directions that have been taken. On the other hand, this paper focuses on reviewing the research methodologies. Studies which systematically review the literature, such as Farooq and Jibran ( 2018 ), have been shown to be valuable contributions to understanding the scope, measurements, impact size, and determinants of a particular area in order to synthesize with the area’s future research agenda. There has not been a comprehensive survey of the methodologies employed in fintech literature. In fact, there have been very few studies reviewing the methodologies employed in finance research in the past 15 years, with Kim and Ji ( 2015 ) and Adams et al. ( 2019 ) being the closest examples.
For the purpose of reviewing the practice of significance testing, Kim and Ji ( 2015 ) survey recently published articles in four top-ranking finance journals. They find that finance researchers almost exclusively use the conventional significance levels (1%, 5%, and 10%) while paying little attention to the sample size, power of the test, and expected losses. The authors also suggest using more often the Bayesian method or revised standards for evidence (0.1% or 0.5%). Adams et al. ( 2019 ) review the articles published in the same four top-ranking finance journals from 1988 to 2017 in order to investigate whether outliers are treated appropriately in these studies. The authors document that each year, 30–70% of these articles use OLS. To encourage finance researchers to utilize other useful econometric methods, they propose a multivariate outlier identification strategy. As the authors explain, this technique can minimize frictions which hinder the adoption of these methods. Due to their purposes of addressing very specific problems, these two articles provide method surveys that are non-comprehensive. Table 1 summarizes the differences between this paper and the other surveys of fintech methods.
Methodology
The approach to the analysis of the fintech research is to first identify trends in the Finance and Information Systems (IS) literature because fintech is the intersection between financial services and information systems. Specifically, we wished to capture the trends pertaining to (1) the number and distribution of fintech articles published in the leading journals, (2) methodologies employed in fintech research, and (3) the research topics being published in this research. During the analysis of this literature, we attempted to identify gaps and needs in the research and therefore enumerate and discuss a research agenda which allows for the progression of research (Webster & Watson, 2002 ). Systematic literature reviews are a meta-analysis technique designed to collect, organize, analyze, and categorize existing knowledge and concepts in the research literature of a given category (Briner et al., 2009 ). In short, we hope to paint a representative landscape of the current fintech literature base to influence the direction of future research efforts in this important area of study.
To examine the current state of research on fintech in the top Finance and IS journals, the authors conducted a literature review and analysis in three phases. Phase 1 accumulated a representative pool of articles. Phase 2 classified the articles by research method. Phase 3 classified the research by topic. Each of the three phases is discussed in the following paragraphs.
Phase 1: Accumulation of article pool
We used the Web of Science (WoS) citation database, Scopus citation database, and Google Scholar to search for research articles with a focus on fintech. The search parameters were constrained based on (a) a list of top ranked journals, (b) a specific time range, and (c) key search terms. Figure 1 illustrates steps in the content analysis process adapted from Neuendorf ( 2002 ) and successfully employed by several similar research studies in Internet marketing (Corley et al., 2013 ), Business Intelligence (Jourdan et al., 2008 ), and Enterprise Resource Planning systems (Cumbie et al., 2005 ).
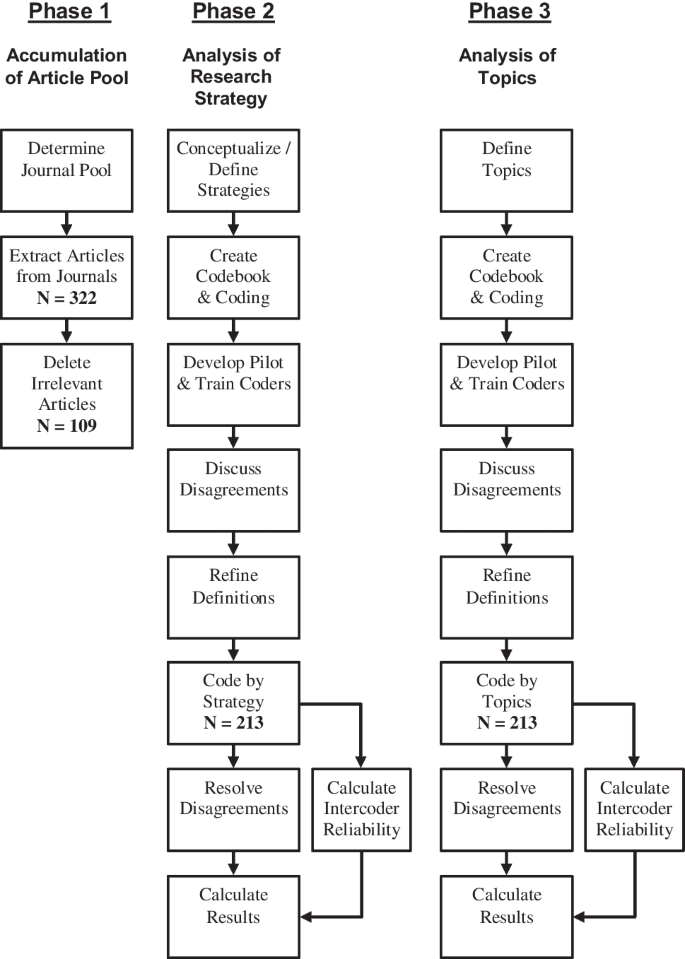
Overview of literature analysis
First, the researchers chose to use the journals from the Australian Business Dean’s Council ABDC list (ABDC, 2019 ). Then, we filtered the ranking of journals to include only Finance (Code 1502) and collected the list of A* (see Table 2 ), A (see Table 3 ), and B (see Table 4 ) journals. Then, we filtered the ranking of journals to include only Information Systems (Code 0806) and collected the list of A* (see Table 5 ), A (see Table 6 ), and B (see Table 7 ) journals. Many of the Finance and IS journals in the sample contained no fintech articles and were deleted from the tables.
The search parameters were further constrained to a specific timeframe. As previously discussed, the term fintech was first coined by Citicorp in 1993 (Schueffel, 2016 ). The search parameters were further constrained based on the historical timeframe in which technologies capable of facilitating the Finance function were first introduced, and the years of publications for articles in our search sample were constrained to the years of 2002 through December of 2021.
The final constraint was based on the key search term “fintech.” In WoS, Scopus, and Google Scholar the search engine scanned for the term “fintech” and close variations of this term found in the title, abstract, and keywords of articles published in the top Finance journals between January of 2002 and December of 2021 when the search was executed. There was a considerable overlap in the pool of articles returned from the three search engines (WoS, SCOPUS, and Google Scholar). Of the 322 (227 Finance and 95 IS) total articles in the initial search, 83 articles (67 Finance and 16 IS) were removed because the articles’ publication year was 2022. This further shows the explosive growth of this research topic’s popularity as the search was conducted in late February of 2022. Once duplicate entries and non-research articles (book reviews, editorials, commentary, etc.) were removed, another 26 (17 Finance and 9 IS) articles were removed. As a result of this process, 216 (146 Finance and 70 IS) articles remained in the composite data pool for analysis. All 216 article files were collected in Adobe Acrobat PDF format and loaded into NVivo 11 to run a word frequency query of the content without numbers and extemporaneous words (i.e. “a,” “and,” “the,” etc.). Figure 2 shows the word cloud that resulted from this query.
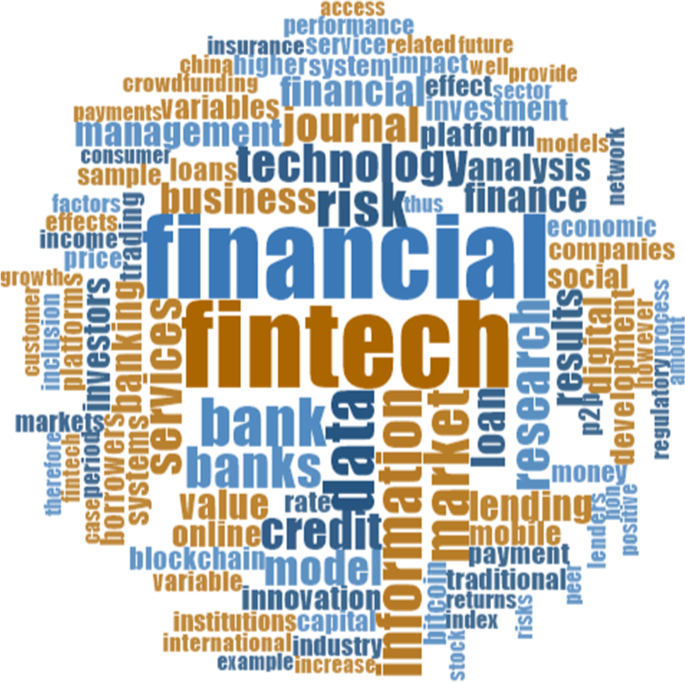
Word cloud of fintech research created in NVivo
Phase 2: Classification by research strategy
Once the researchers identified the articles for the final data pool, each article was examined and categorized according to its research strategy. Due to the subjective nature of research strategy classification, content analysis methods were used for the categorization process (Neuendorf, 2017 ).
First, the research categories were adopted from Scandura and Williams ( 2000 ) (see Table 8 ), who extended the research strategies initially described by McGrath ( 1982 ). Specifically, nine categories of business research strategies were selected including: Formal theory/literature reviews, sample survey, laboratory experiment, experimental simulation, field study (primary data), field study (secondary data), field experiment, judgment task, and computer simulation.
Second, to guard against the threats to reliability (Neuendorf, 2017 ), we performed a pilot test on articles not included in the final data pool for this study. Researchers independently categorized the articles in the pilot test based on the best fit among the nine research strategies. After all articles in the pilot test were categorized, the researchers compared their analyses. In instances where the independent categorizations did not match, the researchers re-evaluated the article collaboratively by reviewing the research category definitions, discussing the disagreement thoroughly, and collaboratively assigning the article to a single category. This process allowed the researchers to develop a collaborative interpretation of the research category definitions. This pilot test served as a training session for accurately categorizing the articles for this study.
Each research strategy is defined by a specific design approach, and each is also associated with certain tradeoffs that researchers must make when designing a study. These tradeoffs are inherent flaws that limit the conclusions that can be drawn from a particular research strategy. These tradeoffs refer to three aspects of a study that can vary depending on the research strategy employed. These variable aspects include generalizability from the sample to the target population (external validity), precision in measurement and control of behavioral variables (internal and construct validity), and the issue of realism of context (Scandura & Williams, 2000 ).
Campbell and Cook ( 1976 ) stated that a study has generalizability when the study has external validity across times, settings, and individuals. Formal theory/literature reviews and sample surveys have a high degree of generalizability by establishing the relationship between two constructs and illustrating that this relationship has external validity. A research strategy that has low external validity, but high internal validity is a benefit of the laboratory experiment. In the laboratory experiment, where the degree of measurement precision is high, cause and effect relationships may be determined, but these relationships may not be generalizable for other times, settings, and populations. While the formal theory/literature reviews and sample surveys have a high degree of generalizability and the laboratory experiment has a high degree of precision of measurement, these strategies have low degree of contextual realism. The only two strategies that maximize degree of contextual realism are field studies that use either primary or secondary data because the data is collected in an organizational setting (Scandura & Williams, 2000 ). The other four strategies maximize neither generalizability, nor degree of precision in measurement, nor degree of contextual realism. This point illustrates the futility of using only one strategy when conducting fintech research. Because no single strategy can maximize all types of validity, it is best for researchers to use a variety of research strategies.
Two coders independently reviewed and classified each article according to research strategy. Only a few articles were reviewed at one sitting to minimize coder fatigue and thus protect intercoder reliability (Neuendorf, 2017 ). Upon completion of the classification process, agreements and disagreements were tabulated. The percent agreement was 87.5% ( N = 216). Then, intercoder reliability ( κ = 0.874) using Cohen’s Kappa (Cohen, 1960 ) and Krippendorf’s Alpha (Krippendorff, 2013 ) for each methodology ( α = 0.859) was calculated. Neuendorf ( 2017 ) suggests that a Cohen’s kappa greater than 0.800 is considered acceptable. Krippendorff ( 2013 ) stated that researchers could use reliability scores greater than 0.800. Therefore, the calculations for intercoder reliability were well within the acceptable ranges. We calculated the reliability measures prior to discussing disagreements as mandated by Weber ( 1990 ). If the original reviewers did not agree on how a particular article was coded, a third reviewer arbitrated the discussion of how the disputed article was to be coded. This process resolved the disputes in all cases.
Phase 3: Categorization by fintech research topic
Typically, the process of categorizing research articles by a specific research topic involves an iterative cycle of brainstorming and discussion sessions among the researchers. This iterative process helps to identify common themes within the data pool of articles. Through the collaborative discussions during this process researchers can synthesize a hierarchical structure within the literature of overarching research topics and more granular level subtopics. The outcome is a better understanding of the current state of a particular stream of research. This iterative process was modified for this specific study on the topic of fintech.
This process resulted in four research topics: Enhance, Impact, Innovate, and Research. The Enhance topic was research that investigates how traditional financial products and services are implemented and improved by using fintech. Examples include using fintech to improve the traditional activities of making personal consumer loans (Di Maggio & Yao, 2021 ; Gerrans et al., 2021 ), analyzing the creditworthiness of borrowers (Jagtiani & Lemieux, 2019 ), and enhancing customer experience in traditional wealth management (Kim et al., 2020 ). The Impact topic analyses fintech’s influence on industries, governments, and economies and includes the impact of technology on banking industry misconduct (Bertsch et al., 2020 ), fragility of financial institutions that use various technologies (Fung et al., 2020 ), how various technologies are affecting the insurance industry (Stoeckli et al., 2018 ), and the new regulatory models necessary from fintech (Jiang et al., 2021 ). The Innovate topic explores financial products and services that were created by or made possible by the implementation of fintech with financial products and services such as blockchain, initial coin offerings (ICOs), and cryptocurrencies (Zhao et al., 2021 ), digital tokens (Benedetti & Nikbakht, 2021 ), peer to peer lending (Fu, Huang, & Singh, 2021), mobile payments (Du, 2018), crowdfunding (Lin & Pursiainen, 2021 ), and the analysis of the new business models created by innovations in fintech (Gomber, Kauffman, Parker, & Weber, 2018). The Research topic illustrates the importance and impact of fintech on individuals and society up to and including research on fintech itself. Research that represents this topic include financial literacy (Philippas & Avdoulas, 2020 ), financial inclusion (Hua & Huang, 2021 ; Kanga et al., 2021 ; Senyo, Osabutey, & Kan, 2021), the use of fintech as a research tool (Bradbury et al., 2019 ), and research on the concept of fintech itself (Bollaert et al., 2021 ). The authors used these four topics to successfully categorize all 216 articles in the research sample.
To guard against the threats to reliability (Neuendorf, 2017 ), we once again performed a pilot test on articles not included in the final data pool for this study. Following the adoption of the four research topics, this second pilot study was used as a training session for categorizing articles by research topic. Researchers independently categorized the articles in the pilot test based on the best fit among the four research topics. After all articles in the pilot test were categorized, the researchers compared their analyses. In instances where the independent categorizations did not match, the researchers re-evaluated the article collaboratively by reviewing the research category definitions, discussing the disagreement thoroughly, and collaboratively assigning the article to a single category. This process allowed the researchers to develop a collaborative interpretation of the research topic definitions (see Table 9 ). Once we established the topic definitions, we independently placed each article in one fintech category. As before, we categorized only a few articles at a time to minimize coder fatigue and thus protect intercoder reliability (Neuendorf, 2017 ).
Upon completion of the classification process, agreements and disagreements were tabulated. The percent agreement was 86.1% ( N = 216). Then, intercoder reliability ( κ = 0.860) using Cohen’s Kappa (Cohen, 1960 ) and Krippendorf’s Alpha (Krippendorff, 2013 ) for each methodology ( α = 0.815) was calculated. Neuendorf ( 2017 ) suggests that a Cohen’s kappa greater than 0.800 is considered acceptable. Krippendorff ( 2013 ) stated that researchers could use reliability scores greater than 0.800. Therefore, the calculations for intercoder reliability were well within the acceptable ranges. We calculated the reliability measures prior to discussing disagreements as mandated by Weber ( 1990 ). If the original reviewers did not agree on how a particular article was coded, a third reviewer arbitrated the discussion of how the disputed article was to be coded. This process resolved the disputes in all cases.
To identify gaps and needs in the research (Webster & Watson, 2002 ), we hope to paint a representative landscape of the current fintech literature base. To examine the current state of this research, the authors conducted a literature review and analysis in three phases. Phase 1 accumulated a representative pool of fintech articles, and the articles were then analyzed with respect to year of publication, journal, and author. Phase 2 briefly discussed the research strategies set forth by Scandura and Williams ( 2000 ) and the results of the classification of the articles by those research strategies. Phase 3 involved the creation and use of four fintech topics, a short discussion of each topic, and the results of the classification of each article within the research topics. These results are discussed in the following paragraphs.
Results of Phase 1
Using the described search criteria within the selected journals, we collected a total of 216 articles. For the complete list of Finance articles in our sample ( N = 146), see Appendix 1 . For the complete list of Information Systems articles in our sample ( N = 70), see Appendix 2 . In phase 1, we further analyzed the articles’ year of publication, journal, and author. Figure 3 shows the number of articles per year in our sample. Although no articles were collected prior to 2016, there is a dramatically increasing trend over the 6-year period of 2016 through 2021. From 2020 to 2021, the number of articles more than doubled, with N = 52 in 2020 and N = 105 in 2021. With fintech issues becoming ever more important to researchers and practitioners, this drastic increase comes as no surprise.
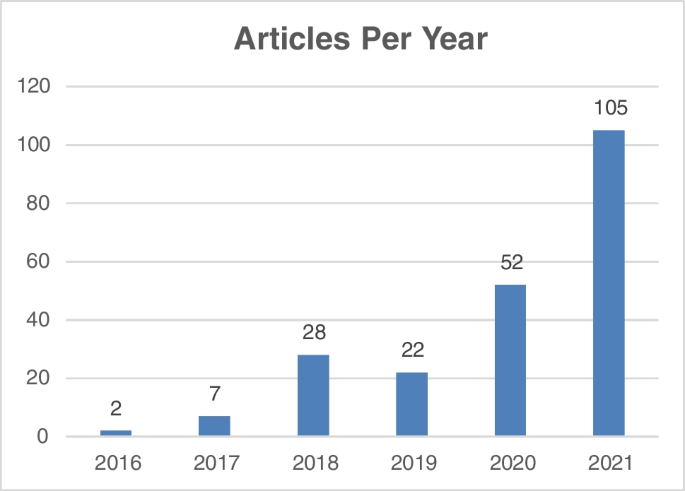
Number of fintech articles published per year
We analyzed the productivity of authors who published in this line of research by assigning scores based on each author’s share of each article. For projects with multiple authors, each co-author was given an equal share of the credit. An author who published an article alone was assigned a score of 1.0. For a two-author article, each author earned a score of 0.500, three authors shared 0.333, and so on. Authorship order was not calculated into this formula. We totaled the scores for each Finance author, then ranked the authors according to their totaled scores in descending order. The results of the top 43 fintech authors in Finance are displayed in Table 10 . This system rewards both quantity of research and ownership of research. The top ranked Finance researcher (Schwienbacher, A.) and the second ranked research (Selim, M.) both had a sole-author paper and co-authorship on another article in the Finance sample. All others who wrote a sole-author research article tied for third place. All of the remaining authors in this list co-authored more than one fintech research article published in Finance, so their scores are above 0.500.
Similarly, the scores for each Information Systems author were totaled, and the authors were sorted from highest to lowest scores. The results of the top 13 fintech authors in the Information Systems sample are displayed in Table 11 . This system rewards both quantity of research and ownership of research. The top ranked researcher (Gozman, D.) had co-authorship on many articles in the Information Systems sample. All the second-ranked authors had a sole author paper (score = 1.0). The remaining authors who had a score greater than 0.500 were also ranked in the sample. A score greater than 0.500 indicates having more than one co-authorship in the sample.
Results of Phase 2
The results of the categorization of the 216 articles according to the nine research strategies described by Scandura and Williams ( 2000 ) are summarized in Table 12 . Of the 216 articles, 104 articles (48.15%) were classified as Field Study—Secondary Data making this category the most used research strategy. With 62 articles (28.70%), Formal Theory/Literature Review was the second most prevalent research strategy. Following were Sample Survey with 23 articles (10.65%) and Field Study—Primary Data with 20 articles (9.26%). The remaining categories had three or fewer articles. These top four research strategies composed of 96.76% of the articles in the sample. No articles were classified as a Judgment Task nor a Field Experiment. These four strategies are exploratory in nature and indicate the beginnings of a body of research (Scandura & Williams, 2000 ). Further categorization and analysis of the articles with respect to fintech topic categories were conducted in the third phase of this research project.
Results of Phase 3
Table 13 shows the number of articles per fintech research topic category. These four categories provided a topic area classification for all the 216 articles in our research sample. Of the 216 articles, 38.43% were classified as “Research,” making it the most prevalent fintech topic category. This result is not surprising because the content analyzed was collected from research publishing outlets. Closely following were “Impact” and “Innovate” (21.76%) tying for second place. “Enhance” was the least popular with 18.06% of the articles. These four research strategies accounted for 100% of the articles in the sample. This illustration of the share of fintech research that is represented by each topic reveals the amount of attention fintech is receiving in Finance journals across a new, yet diverse, research stream.
Fintech research strategies versus topics
By plotting fintech research topics against research strategies (Table 14 ), many of the gaps in fintech research are exposed. In our minds, these gaps exist for two reasons. First, some of these research strategies are not prevalent in Finance and IS research. Because some top research journals do not accept papers that use non-traditional or qualitative research strategies, researchers tend to avoid unorthodox strategies. Second, some of these categories have not been studied because they represent a relatively new phenomenon, of which the research has not caught up with the business reality. The great news for researchers interested in fintech is that this domain should provide research opportunities for years to come.
Almost half (48.15%) of the journal articles in this study use the Field Study—Secondary Data research strategy across all research topics. Therefore, classifying the sources of the secondary data used in this research may be valuable for new researchers by providing them insights and sources for future research. The use of Formal Theory/Literature Review (28.7%) and Sample Survey (10.65%) research strategies indicates the exploratory nature of the current state of fintech research. We speculate four reasons for the top three strategies used to study fintech to be prevalent and appropriate for the early stages of research. First, secondary data is common in Finance research with the common practice of using freely available data from financial markets. This abundance of financial data is augmented by the availability of premium financial information services as a source of data for research projects. Second, in these exploratory years of fintech research, formal theory/literature reviews are appropriate to determine what other strategies are being used in the research and to find reference disciplines that are conducting related research. Third, researchers in business schools tend to be more skilled in administering literature reviews, field studies (with primary and secondary data), and sample surveys than in the strategies of laboratory experiment, field experiments, experimental simulation, judgment task, and computer simulation. Finally, organizations are less likely to commit to certain strategies (e.g., primary field studies and field experiments) because these strategies are more expensive for the organizations. These types of research strategies are very labor intensive to the organization being studied because they require records to be examined, personnel to be interviewed, and senior managers to devote large amounts of their expensive time to help facilitate the research project.
Contributions
To date, no study has examined fintech research topics in words, content, or methodologies. The purpose of this study is to synthesize the methodologies and content of all fintech article from the past 20 year encompassing all journals on the Australian Business Deans list that have a rating of A*, A, and B. This study finds that the majority of fintech research has been conducted over the past 4 years, with the number of articles significantly increasing during that period. The majority of this research is focused on banking, credit, lending, and intermediaries. However, many other subjects are yet to be covered in a robust manner. Despite the proliferation of fintech research, there unfortunately is no standard set of best practices or methodological norms that researchers can use as of yet. Our findings show that fintech research is in its infancy.
Limitations
The current analysis of the fintech literature in this study has limitations and should be enhanced with future research efforts. Future literature reviews could expand article searches to full article text searches, search a broader domain of research outlets (such as adding the C journals in the ABDC journal list), and include other fintech related search terms. Our literature analysis is meant to serve as a representative sample of articles and not a comprehensive and exhaustive analysis of the entire population of articles published on the topic of fintech.
This study provided a content analysis of the current state of the research with respect to research strategy and topic at the journals on the ABDC list. Other publication outlets may be publishing greater quantities of fintech research with similar quality as the journals in our sample.
Directions for future research
For researchers to continue to attempt to answer the important questions in fintech, future studies need to employ a wider variety of research strategies to investigate these important issues. Scandura and Williams ( 2000 ) stated that looking at research strategies employed over time by triangulation in each subject area can provide useful insights into how theories are developing. In addition to the lack of variety in research strategy, very little triangulation has occurred during the timeframe used to conduct this literature review. This absence of coordinated theory development causes the research in fintech to appear haphazard and unfocused. Clearly, future studies should consider the identified gaps and consider the future research role relative to generalizability, precision of measure, and realism of context.
Future efforts should also consider the four research topics with respect to the research strategies. To further investigate this body of research, future studies could explore the fintech topics in depth by creating subtopics within the four topics in the study. For example, fintech will be deployed by organizations to improve their current business processes for future study under the Enhance topic. For the Impact, many of these fintech modalities have not been in place long enough for researchers, practitioners, governments, and other stakeholders to collect analyze data on how industries, governments, and economies are affected on a short or long-time horizon. As previously unknown business models and technologies combine fintech and artificial intelligence, new opportunities for research will be presented for researchers and practitioners alike to explore Innovate topic. As the number and quality of research grows in Enhance, Impact, and Innovate grows, this will give researchers in disciplines as varied as Economics, Engineering, Psychology, Sociology, and others to contribute to the research body of fintech and how this concept is progressing across time and a variety of research streams.
Future studies could take a more in-depth look at the various business models or fintech strategies associated with this research stream. Moreover, much of the research in our sample reports the new technologies and issues in fintech without attempting to explain the fundamental issues of the technology implemented or the effects of these technologies on individuals, organizations, and society. This is to be expected in the exploratory stages of research in a subject area.
This study used the content analysis methodology to create a current, cross-disciplinary image of the current state of fintech research in the top Finance and Information Systems journals across time, research strategy, and topic to classify this concept of financial technologies. Further, this study illustrates the future potential of fintech domain across both research strategy and topic. Despite the efforts of the researchers in the article sample, fintech is in the beginning stages of the research stream. The bad news is that much research needs to take place in this domain using a variety of research strategies over time to develop best practices for practitioners and theory for the research domain. In this sample, most of the research had been published in the previous four years, and the good news for researchers and practitioners alike is that many of the topics and research strategies in this research are open for future research efforts including some research strategy and topic areas that are completely unresearched (Table 14 ). As more practitioners deploy more fintech modalities, researchers will have the opportunity to create even more novel and rigorous research studies. We hope that this content analysis has laid the foundation for such efforts that will enhance the body of knowledge and theoretical progression relative to fintech.
ABDC. (2019). ABDC journal quality list. Retrieved from https://abdc.edu.au/research/abdc-journal-quality-list/
Adams, J., Hayunga, D., Mansi, S., Reeb, D., & Verardi, V. (2019). Identifying and treating outliers in finance. Financial Management, 48 (2), 345–384. https://doi.org/10.1111/fima.12269
Article Google Scholar
Alt, R., Beck, R., & Smits, M. T. (2018). Fintech and the transformation of the financial industry. Electronic Markets, 28 (3), 235–243. https://doi.org/10.1007/s12525-018-0310-9
Benedetti, H., & Nikbakht, E. (2021). Returns and network growth of digital tokens after cross-listings. Journal of Corporate Finance, 66 . https://doi.org/10.1016/j.jcorpfin.2020.101853
Benoit, S., Hurlin, C., & Perignon, C. (2019). Pitfalls in systemic-risk scoring. Journal of Financial Intermediation, 38 , 19–44. https://doi.org/10.1016/j.jfi.2018.05.004
Bertsch, C., Hull, I., Qi, Y. J., & Zhang, X. (2020). Bank misconduct and online lending. Journal of Banking & Finance, 116 . https://doi.org/10.1016/j.jbankfin.2020.105822.
Bollaert, H., Lopez-de-Silanes, F., & Schwienbacher, A. (2021). Fintech and access to finance. Journal of Corporate Finance, 68 . https://doi.org/10.1016/j.jcorpfin.2021.101941.
Boyd, J. H., & De Nicolo, G. (2005). The theory of bank risk taking and competition revisited. Journal of Finance, 60 (3), 1329–1343. https://doi.org/10.1111/j.1540-6261.2005.00763.x
Bradbury, M. A. S., Hens, T., & Zeisberger, S. (2019). How persistent are the effects of experience sampling on investor behavior? Journal of Banking & Finance, 98 , 61–79. https://doi.org/10.1016/j.jbankfin.2018.10.014
Briner, R. B., Denyer, D., & Rousseau, D. M. (2009). Evidence-based management: Concept cleanup time? Academy of Management Perspectives, 23 (4), 19–32. https://doi.org/10.5465/amp.2009.45590138
Campbell, D. T., & Cook, T. D. (1976). The design and conduct of quasi-experiments and true experiments in field settings. In M. D. Dunnette (Ed.), Handbook of industrial and organizational psychology . Chicago, IL: Rand McNally.
Christensen, C. M. A. (2016). The innovator’s dilemma: When new technologies cause great firms to fail / Clayton M. Christensen . Boston: Harvard Business Review Press.
Cohen, J. (1960). A coefficient of agreement for nominal scales. Educational and Psychological Measurement, 20 (1), 37–46. https://doi.org/10.1177/001316446002000104
Corley, J. K., Jourdan, Z., & Ingram, W. R. (2013). Internet marketing: A content analysis of the research. Electronic Markets, 23 (3), 177–204. https://doi.org/10.1007/s12525-012-0118-y
Cumbie, B., Jourdan, Z., Peachey, T., Dugo, T., & Craighead, C. (2005). Enterprise resource planning research: Where are we now and where should we go from here? Journal of Information Technology Theory and Application (JITTA), 7 (2), 21–36.
Google Scholar
Di Maggio, M., & Yao, V. (2021). Fintech borrowers: Lax screening or cream-skimming? Review of Financial Studies, 34 (10), 4565–4618. https://doi.org/10.1093/rfs/hhaa142
Farooq, U., & Jibran, A. Q. (2018). Scope, measurement, impact size and determinants of indirect cost of financial distress: A systematic literature review. Qualitative Research in Financial Markets, 10 (1), 111–129. https://doi.org/10.1108/qrfm-08-2017-0080
Fung, D. W. H., Lee, W. Y., Yeh, J. J. H., & Yuen, F. L. (2020). Friend or foe: The divergent effects of fintech on financial stability. Emerging Markets Review, 45 . https://doi.org/10.1016/j.ememar.2020.100727.
Fuster, A., Plosser, M., Schnabl, P., & Vickery, J. (2019). The role of technology in mortgage lending. Review of Financial Studies, 32 (5), 1854–1899. https://doi.org/10.1093/rfs/hhz018
Gerrans, P., Baur, D. G., & Lavagna-Slater, S. (2021). Fintech and responsibility: Buy-now-pay-later arrangements. Australian Journal of Management . https://doi.org/10.1177/03128962211032448
Goetz, M. R. (2018). Competition and bank stability. Journal of Financial Intermediation, 35 , 57–69. https://doi.org/10.1016/j.jfi.2017.06.001
Hornuf, L., Klus, M. F., Lohwasser, T. S., & Schwienbacher, A. (2021). How do banks interact with fintech startups? Small Business Economics, 57 (3), 1505–1526. https://doi.org/10.1007/s11187-020-00359-3
Hua, X. P., & Huang, Y. P. (2021). Understanding China’s fintech sector: Development, impacts and risks. European Journal of Finance, 27 (4–5), 321–333. https://doi.org/10.1080/1351847x.2020.1811131
Jagtiani, J., & Lemieux, C. (2019). The roles of alternative data and machine learning in fintech lending: Evidence from the LendingClub consumer platform. Financial Management, 48 (4), 1009–1029. https://doi.org/10.1111/fima.12295
Jiang, J. L., Liao, L., Wang, Z. W., & Zhang, X. Y. (2021). Government affiliation and peer-to-peer lending platforms in China. Journal of Empirical Finance, 62 , 87–106. https://doi.org/10.1016/j.jempfin.2021.02.004
Jourdan, Z., Rainer, R. K., & Marshall, T. E. (2008). Business intelligence: An analysis of the literature. Information Systems Management, 25 (2), 121–131. https://doi.org/10.1080/10580530801941512
Kanga, D., Oughton, C., Harris, L., & Murinde, V. (2021). The diffusion of fintech, financial inclusion and income per capita. European Journal of Finance . https://doi.org/10.1080/1351847x.2021.1945646
Kim, J. H., & Ji, P. I. (2015). Significance testing in empirical finance: A critical review and assessment. Journal of Empirical Finance, 34 , 1–14. https://doi.org/10.1016/j.jempfin.2015.08.006
Kim, W. C., Kwon, D. G., Lee, Y., Kim, J. H., & Lin, C. (2020). Personalized goal-based investing via multi-stage stochastic goal programming. Quantitative Finance, 20 (3), 515–526. https://doi.org/10.1080/14697688.2019.1662079
Krippendorff, K. (2013). Content analysis: An introduction to its methodology / Klaus Krippendorff . Los Angeles [etc.]: SAGE Publications.
Lagna, A., & Ravishankar, M. N. (2022). Making the world a better place with fintech research. Information Systems Journal, 32 (1), 61–102. https://doi.org/10.1111/isj.12333
Li, Y. Q., Spigt, R., & Swinkels, L. (2017). The impact of fintech start-ups on incumbent retail banks’ share prices. Financial Innovation, 3 (1). https://doi.org/10.1186/s40854-017-0076-7.
Lin, T. C., & Pursiainen, V. (2021). The round number heuristic and entrepreneur crowdfunding performance. Journal of Corporate Finance, 68 . https://doi.org/10.1016/j.jcorpfin.2021.101894.
McGrath, J. E. (1982). Dilemmatics: The study of research choices and delimmas . Sage Publications.
Neuendorf, K. A. (2002). The content analysis guidebook (1st ed.). Thousand Oaks, Calif. [etc.]: Sage Publications.
Neuendorf, K. A. (2017). The content analysis guidebook (2nd ed.). Thousand Oaks, California: SAGE Publications, Inc.
Philippas, N. D., & Avdoulas, C. (2020). Financial literacy and financial well-being among generation-Z university students: Evidence from Greece. European Journal of Finance, 26 (4–5), 360–381. https://doi.org/10.1080/1351847x.2019.1701512
Philippon, T. (2015). Has the US finance industry become less efficient? On the theory and measurement of financial intermediation. American Economic Review, 105 (4), 1408–1438. https://doi.org/10.1257/aer.20120578
Scandura, T. A., & Williams, E. A. (2000). Research methodology in management: Current practices, trends, and implications for future research. Academy of Management Journal, 43 (6), 1248–1264. https://doi.org/10.2307/1556348
Schueffel, P. (2016). Taming the beast: A scientific definition of fintech. SSRN Electronic Journal ; ISSN 1556–5068 . https://doi.org/10.2139/ssrn.3097312.
Stoeckli, E., Dremel, C., & Uebernickel, F. (2018). Exploring characteristics and transformational capabilities of InsurTech innovations to understand insurance value creation in a digital world. Electronic Markets, 28 (3), 287–305. https://doi.org/10.1007/s12525-018-0304-7
Thakor, A. V. (1999). Information technology and financial services consolidation. Journal of Banking & Finance, 23 (2–4), 697–700. https://doi.org/10.1016/s0378-4266(98)00104-6
Thakor, A. V. (2020). Fintech and banking: What do we know? Journal of Financial Intermediation, 41 . https://doi.org/10.1016/j.jfi.2019.100833
Weber, R. P. (1990). Basic content analysis (2nd ed.). Nowbury Park: Sage Publications.
Webster, J., & Watson, R. T. (2002). Analyzing the past to prepare for the future: Writing a literature review. MIS Quarterly, 26 (2), XIII-XXIII. Retrieved from https://www.jstor.org/stable/4132319
Zhao, X. J., Hou, W. X., An, J. F., Liu, X. D., & Zhang, Y. (2021). Initial coin offerings: What rights do investors have? European Journal of Finance, 27 (4–5), 305–320. https://doi.org/10.1080/1351847x.2020.1858130
Download references
Author information
Authors and affiliations.
College of Business, Auburn University at Montgomery, 7400 East Drive, Montgomery, AL, 36117, USA
Zack Jourdan
Marketing and SCM Department , Appalachian State Universtiy, Boone, NC, United States
J. Ken. Corley
Area of Finance, Texas Tech University, Lubbock, TX, United States
Randall Valentine & Arthur M. Tran
You can also search for this author in PubMed Google Scholar
Corresponding author
Correspondence to Zack Jourdan .
Additional information
Responsible Editor: Younghoon Chang.
Publisher's note
Springer Nature remains neutral with regard to jurisdictional claims in published maps and institutional affiliations.
Appendix 1 Sample of 146 fintech finance articles
Agarwal, S., & Zhang, J. (2020). Fintech, lending and payment innovation: A review. Asia-Pacific Journal of Financial Studies, 49 (3), 353-367. https://doi.org/10.1111/ajfs.12294
Ahmed, S., Grobys, K., & Sapkota, N. (2020). Profitability of technical trading rules among cryptocurrencies with privacy function. Finance Research Letters, 35 . https://doi.org/10.1016/j.frl.2020.101495
Akyildirim, E., Corbet, S., Sensoy, A., & Yarovaya, L. (2020). The impact of blockchain related name changes on corporate performance. Journal of Corporate Finance, 65 . https://doi.org/10.1016/j.jcorpfin.2020.101759
Akyildirim, E., Sensoy, A., Gulay, G., Corbet, S., & Salari, H. N. (2021). Big data analytics, order imbalance and the predictability of stock returns. Journal of Multinational Financial Management, 62 . https://doi.org/10.1016/j.mulfin.2021.100717
Albarrak, M. S., & Alokley, S. A. (2021). Fintech: Ecosystem, opportunities and challenges in Saudi Arabia. Journal of Risk and Financial Management, 14 (10). https://doi.org/10.3390/jrfm14100460
Altamura, C. E., & Daunton, M. (2020). Finance, financiers and financial centres: a special issue in honour of Youssef Cassis Introduction. Financial History Review, 27 (3), 283-302. https://doi.org/10.1017/s0968565020000153
Altwijry, O. I., Mohammed, M. O., Hassan, M. K., & Selim, M. Developing a Shari'ah based fintech money creation free SFMCF model for Islamic banking. International Journal of Islamic and Middle Eastern Finance and Management . https://doi.org/10.1108/imefm-05-2021-0189
Arner, D. W., Barberis, J., & Buckley, R. P. (2016). 150 YEARS OF FINTECH: An evolutionary analysis. Jassa-the Finsia Journal of Applied Finance (3), 22-29. Retrieved from https://search.informit.org/doi/10.3316/ielapa.419780653701585
Ascarya, A. (2021). The role of Islamic social finance during Covid-19 pandemic in Indonesia's economic recovery. International Journal of Islamic and Middle Eastern Finance and Management . https://doi.org/10.1108/imefm-07-2020-0351
Azarenkova, G., Shkodina, I., Samorodov, B., Babenko, M., & Onishchenko, I. (2018). The influence of financial technologies on the global financial system stability. Investment Management and Financial Innovations, 15 (4), 229-238. https://doi.org/10.21511/imfi.15(4).2018.19
Ba, S. S., Wei, W., & Yuan, H. M. (2021). How does active change affect investment efficiency? Evidence from monthly account-level data on Chinese online platform. Emerging Markets Finance and Trade . https://doi.org/10.1080/1540496x.2021.1965984
Baber, H. (2020). Financial inclusion and fintech: A comparative study of countries following Islamic finance and conventional finance. Qualitative Research in Financial Markets, 12 (1), 24-42. https://doi.org/10.1108/qrfm-12-2018-0131
Baek, H. Y., Cho, D. D., Jordan, R. A., & Kuvvet, E. (2021). The differential effect of social disclosure on loan funding and loan repayment: evidence from fixed-rate peer-to-peer lending. Managerial Finance, 47 (3), 394-412. https://doi.org/10.1108/mf-02-2020-0079
Banna, H., Hassan, M. K., Ahmad, R., & Alam, M. R. (2021). Islamic banking stability amidst the COVID-19 pandemic: The role of digital financial inclusion. International Journal of Islamic and Middle Eastern Finance and Management . https://doi.org/10.1108/imefm-08-2020-0389
Banna, H., Hassan, M. K., & Rashid, M. (2021). Fintech-based financial inclusion and bank risk-taking: Evidence from OIC countries. Journal of International Financial Markets Institutions & Money, 75 . https://doi.org/10.1016/j.intfin.2021.101447
Bao, Z. Y., & Huang, D. F. (2021). Shadow banking in a crisis: Evidence from fintech during COVID-19. Journal of Financial and Quantitative Analysis, 56 (7), 2320-2355. https://doi.org/10.1017/s0022109021000430
Bertsch, C., Hull, I., Qi, Y. J., & Zhang, X. (2020). Bank misconduct and online lending. Journal of Banking & Finance, 116 . https://doi.org/10.1016/j.jbankfin.2020.105822
Bhatia, A., Chandani, A., Atiq, R., Mehta, M., & Divekar, R. (2021). Artificial intelligence in financial services: A qualitative research to discover robo-advisory services. Qualitative Research in Financial Markets, 13 (5), 632-654. https://doi.org/10.1108/qrfm-10-2020-0199
Bhatia, A., Chandani, A., & Chhateja, J. (2020). Robo advisory and its potential in addressing the behavioral biases of investors - A qualitative study in Indian context. Journal of Behavioral and Experimental Finance, 25 . https://doi.org/10.1016/j.jbef.2020.100281
Blach, J., & Klimontowicz, M. (2021). The Determinants of PayTech's success in the mobile payment market-The case of BLIK. Journal of Risk and Financial Management, 14 (9). https://doi.org/10.3390/jrfm14090422
Bollaert, H., Lopez-de-Silanes, F., & Schwienbacher, A. (2021). Fintech and access to finance. Journal of Corporate Finance, 68 . https://doi.org/10.1016/j.jcorpfin.2021.101941
Bradbury, M. A. S., Hens, T., & Zeisberger, S. (2019). How persistent are the effects of experience sampling on investor behavior? Journal of Banking & Finance, 98 , 61-79. https://doi.org/10.1016/j.jbankfin.2018.10.014
Brammertz, W., & Mendelowitz, A. I. (2018). From digital currencies to digital finance: The case for a smart financial contract standard. Journal of Risk Finance, 19 (1), 76-92. https://doi.org/10.1108/jrf-02-2017-0025
Brenner, L., & Meyll, T. (2020). Robo-advisors: A substitute for human financial advice? Journal of Behavioral and Experimental Finance, 25 . https://doi.org/10.1016/j.jbef.2020.100275
Buchak, G., Matvos, G., Piskorski, T., & Seru, A. (2018). Fintech, regulatory arbitrage, and the rise of shadow banks. Journal of Financial Economics, 130 (3), 453-483. https://doi.org/10.1016/j.jfineco.2018.03.011
Caglayan, M., Talavera, O., Xiong, L., & Zhang, J. (2020). What does not kill us makes us stronger: The story of repetitive consumer loan applications. European Journal of Finance . https://doi.org/10.1080/1351847x.2020.1793792
Caglayan, M., Talavera, O., & Zhang, W. (2021). Herding behaviour in P2P lending markets. Journal of Empirical Finance, 63 , 27-41. https://doi.org/10.1016/j.jempfin.2021.05.005
Chava, S., Ganduri, R., Paradkar, N., & Zhang, Y. F. (2021). Impact of marketplace lending on consumers' future borrowing capacities and borrowing outcomes. Journal of Financial Economics, 142 (3), 1186-1208. https://doi.org/10.1016/j.jfineco.2021.06.005
Chen, M. A., Wu, Q. X., & Yang, B. Z. (2019). How valuable is fintech innovation? Review of Financial Studies, 32 (5), 2062-2106. https://doi.org/10.1093/rfs/hhy130
Chen, X. E., & Wang, C. (2021). Information disclosure in China's rising securitization market. International Journal of Financial Studies, 9 (4). https://doi.org/10.3390/ijfs9040066
Cheng, M. Y., & Qu, Y. (2020). Does bank fintech reduce credit risk? Evidence from China. Pacific-Basin Finance Journal, 63 . https://doi.org/10.1016/j.pacfin.2020.101398
Chong, F. H. L. (2021). Enhancing trust through digital Islamic finance and blockchain technology. Qualitative Research in Financial Markets, 13 (3), 328-341. https://doi.org/10.1108/qrfm-05-2020-0076
Chuen, D. L. K. (2018). Fintech and alternativeiInvestment. Journal of Alternative Investments, 20 (3), 6-15. https://doi.org/10.3905/jai.2018.20.3.006
Cumming, D. J., Johan, S., & Pant, A. (2019). Regulation of the crypto-economy: Managing risks, challenges, and regulatory uncertainty. Journal of Risk and Financial Management, 12 (3). https://doi.org/10.3390/jrfm12030126
Daluwathumullagamage, D. J., & Sims, A. (2021). Fantastic Beasts: Blockchain based banking. Journal of Risk and Financial Management, 14 (4). https://doi.org/10.3390/jrfm14040170
Danbolt, J., Eshraghi, A., & Lukas, M. (2021). Investment transparency and the disposition effect. European Financial Management . https://doi.org/10.1111/eufm.12329
Daragmeh, A., Lentner, C., & Sagi, J. (2021). Fintech payments in the era of COVID-19: Factors influencing behavioral intentions of "Generation X"in Hungary to use mobile payment. Journal of Behavioral and Experimental Finance, 32 . https://doi.org/10.1016/j.jbef.2021.100574
Das, S. R. (2019). The future of fintech. Financial Management, 48 (4), 981-1007. https://doi.org/10.1111/fima.12297
Demir, A., Pesque-Cela, V., Altunbas, Y., & Murinde, V. (2020). Fintech, financial inclusion and income inequality: a quantile regression approach. European Journal of Finance . https://doi.org/10.1080/1351847x.2020.1772335
Deng, L. R., Lv, Y. B., Liu, Y., & Zhao, Y. W. (2021). Impact of fintech on bank risk-taking: Evidence from China. Risks, 9 (5). https://doi.org/10.3390/risks9050099
Di Maggio, M., & Yao, V. (2021). Fintech borrowers: Lax screening or cream-skimming? Review of Financial Studies, 34 (10), 4565-4618. https://doi.org/10.1093/rfs/hhaa142
Dranev, Y., Frolova, K., & Ochirova, E. (2019). The impact of fintech M&A on stock returns. Research in International Business and Finance, 48 , 353-364. https://doi.org/10.1016/j.ribaf.2019.01.012
Dugast, J., & Foucault, T. (2018). Data abundance and asset price informativeness. Journal of Financial Economics, 130 (2), 367-391. https://doi.org/10.1016/j.jfineco.2018.07.004
Elsaid, H. M. A review of literature directions regarding the impact of fintech firms on the banking industry. Qualitative Research in Financial Markets . https://doi.org/10.1108/qrfm-10-2020-0197
Fahlenbrach, R., & Frattaroli, M. (2021). ICO investors. Financial Markets and Portfolio Management, 35 (1), 1-59. https://doi.org/10.1007/s11408-020-00366-0
Faloon, M., & Scherer, B. (2017). Individualization of robo-advice. Journal of Wealth Management, 20 (1), 30-36. https://doi.org/10.3905/jwm.2017.20.1.030
Fang, H., Chung, C. P., Lu, Y. C., Lee, Y. H., & Wang, W. H. (2021). The impacts of investors' sentiments on stock returns using fintech approaches. International Review of Financial Analysis, 77 . https://doi.org/10.1016/j.irfa.2021.101858
Farag, H., & Johan, S. (2021). How alternative finance informs central themes in corporate finance. Journal of Corporate Finance, 67 . https://doi.org/10.1016/j.jcorpfin.2020.101879
Foglia, M., Recchioni, M. C., & Polinesi, G. (2021). Smart beta allocation and macroeconomic variables: The impact of COVID-19. Risks, 9 (2). https://doi.org/10.3390/risks9020034
Fung, D. W. H., Lee, W. Y., Yeh, J. J. H., & Yuen, F. L. (2020). Friend or foe: The divergent effects of fintech on financial stability. Emerging Markets Review, 45 . https://doi.org/10.1016/j.ememar.2020.100727
Fuster, A., Plosser, M., Schnabl, P., & Vickery, J. (2019). The role of technology in mortgage lending. Review of Financial Studies, 32 (5), 1854-1899. https://doi.org/10.1093/rfs/hhz018
Gachter, I., & Gachter, M. (2021). Success factors in ICOs: Individual firm characteristics or lucky timing? Finance Research Letters, 40 . https://doi.org/10.1016/j.frl.2020.101715
Glavina, S., Aidrus, I., & Trusova, A. (2021). Assessment of the competitiveness of Islamic fintech implementation: A composite indicator for cross-country analysis. Journal of Risk and Financial Management, 14 (12). https://doi.org/10.3390/jrfm14120602
Goldstein, I., Jiang, W., & Karolyi, G. A. (2019). To fintech and beyond. Review of Financial Studies, 32 (5), 1647-1661. https://doi.org/10.1093/rfs/hhz025
Golub, A., Grossmass, L., & Poon, S. H. (2021). Ultra-short tenor yield curve for intraday trading and settlement. European Journal of Finance, 27 (4-5), 441-459. https://doi.org/10.1080/1351847x.2019.1662821
Gong, Q., Liu, C., Peng, Q. N., & Wang, L. Y. (2020). Will CEOs with banking experience lower default risks? Evidence from P2P lending platforms in China. Finance Research Letters, 36 . https://doi.org/10.1016/j.frl.2020.101461
Gonzalez, L. (2020). Blockchain, herding and trust in peer-to-peer lending. Managerial Finance, 46 (6), 815-831. https://doi.org/10.1108/mf-09-2018-0423
Grabowski, M. (2021). Legal aspects of "White-Label" banking in the European, Polish and German law. Journal of Risk and Financial Management, 14 (6). https://doi.org/10.3390/jrfm14060280
Grobys, K., Ahmed, S., & Sapkota, N. (2020). Technical trading rules in the cryptocurrency market. Finance Research Letters, 32 . https://doi.org/10.1016/j.frl.2019.101396
Gupta, M., Verma, S., & Pachare, S. (2021). An analysis of conventional and alternative financing-customers' perspective. International Journal of Finance & Economics . https://doi.org/10.1002/ijfe.2541
Han, J. J., & Kim, H. J. (2021). Stock price prediction using multiple valuation methods based on artificial neural networks for KOSDAQ IPO companies. Investment Analysts Journal, 50 (1), 17-31. https://doi.org/10.1080/10293523.2020.1870860
Harasim, J. (2021). Fintechs, Bigtechs and banks-When cooperation and when competition? Journal of Risk and Financial Management, 14 (12). https://doi.org/10.3390/jrfm14120614
Ho, M.-T., Le, N.-T. B., Tran, H.-L. D., Nguyen, Q.-H., Pham, M.-H., Ly, M. H., Ho, M.-T., Nguyen, M.-H. & Vuong, Q.-H. (2021). A systematic and critical review on the research landscape of finance in Vietnam from 2008 to 2020. Journal of Risk and Financial Management, 14 (5). https://doi.org/10.3390/jrfm14050219
Hua, X. P., & Huang, Y. P. (2021). Understanding China's fintech sector: development, impacts and risks. European Journal of Finance, 27 (4-5), 321-333. https://doi.org/10.1080/1351847x.2020.1811131
Hudaefi, F. A. (2020). How does Islamic fintech promote the SDGs? Qualitative evidence from Indonesia. Qualitative Research in Financial Markets, 12 (4), 353-366. https://doi.org/10.1108/qrfm-05-2019-0058
Huibers, F. (2021). Regulatory response to the rise of fintech credit in The Netherlands. Journal of Risk and Financial Management, 14 (8). https://doi.org/10.3390/jrfm14080368
Iman, N. (2018). Assessing the dynamics of fintech in Indonesia. Investment Management and Financial Innovations, 15 (4), 296-303. https://doi.org/10.21511/imfi.15(4).2018.24
Imerman, M. B., & Fabozzi, F. J. (2020). Cashing in on innovation: A taxonomy of fintech. Journal of Asset Management, 21 (3), 167-177. https://doi.org/10.1057/s41260-020-00163-4
Ishak, M. S. I., & Rahman, M. H. (2021). Equity-based Islamic crowdfunding in Malaysia: A potential application for mudharabah. Qualitative Research in Financial Markets, 13 (2), 183-198. https://doi.org/10.1108/qrfm-03-2020-0024
Jagtiani, J., & Lemieux, C. (2019). The roles of alternative data and machine learning in fintech lending: Evidence from the LendingClub consumer platform. Financial Management, 48 (4), 1009-1029. https://doi.org/10.1111/fima.12295
Jiang, J. L., Liao, L., Lu, X., Wang, Z. W., & Xiang, H. Y. (2021). Deciphering big data in consumer credit evaluation. Journal of Empirical Finance, 62 , 28-45. https://doi.org/10.1016/j.jempfin.2021.01.009
Jiang, J. L., Liao, L., Wang, Z. W., & Zhang, X. Y. (2021). Government affiliation and peer-to-peer lending platforms in China. Journal of Empirical Finance, 62 , 87-106. https://doi.org/10.1016/j.jempfin.2021.02.004
Jun, J., & Yeo, E. (2016). Entry of fintech firms and competition in the retail payments market. Asia-Pacific Journal of Financial Studies, 45 (2), 159-184. https://doi.org/10.1111/ajfs.12126
Junger, M., & Mietzner, M. (2020). Banking goes digital: The adoption of fintech services by German households. Finance Research Letters, 34 . https://doi.org/10.1016/j.frl.2019.08.008
Kavassalis, P., Stieber, H., Breymann, W., Saxton, K., & Gross, F. J. (2018). An innovative RegTech approach to financial risk monitoring and supervisory reporting. Journal of Risk Finance, 19 (1), 39-55. https://doi.org/10.1108/jrf-07-2017-0111
Kim, W. C., Kwon, D. G., Lee, Y., Kim, J. H., & Lin, C. (2020). Personalized goal-based investing via multi-stage stochastic goal programming. Quantitative Finance, 20 (3), 515-526. https://doi.org/10.1080/14697688.2019.1662079
Kliber, A., Bedowska-Sojka, B., Rutkowska, A., & Swierczynska, K. (2021). Triggers and obstacles to the development of the fintech sector in Poland. Risks, 9 (2). https://doi.org/10.3390/risks9020030
Knewtson, H. S., & Rosenbaum, Z. A. (2020). Toward understanding fintech and its industry. Managerial Finance, 46 (8), 1043-1060. https://doi.org/10.1108/mf-01-2020-0024
Koziuk, V. (2021). Confidence in digital money: Are central banks more trusted than age is matter? Investment Management and Financial Innovations, 18 (1), 12-32. https://doi.org/10.21511/imfi.18(1).2021.02
Lanfranchi, D., & Grassi, L. (2022). Examining insurance companies' use of technology for innovation. Geneva Papers on Risk and Insurance-Issues and Practice . https://doi.org/10.1057/s41288-021-00258-y
Le, L. T., Yarovaya, L., & Nasir, M. A. (2021). Did COVID-19 change spillover patterns between fintech and other asset classes? Research in International Business and Finance, 58 . https://doi.org/10.1016/j.ribaf.2021.101441
Le, T. D. Q., Ho, T. H., Nguyen, D. T., & Ngo, T. (2021). Fintech credit and bank efficiency: International evidence. International Journal of Financial Studies, 9 (3). https://doi.org/10.3390/ijfs9030044
Lee, A. D., Li, M. L., & Zheng, H. H. (2020). Bitcoin: Speculative asset or innovative technology? Journal of International Financial Markets Institutions & Money, 67 . https://doi.org/10.1016/j.intfin.2020.101209
Lee, C. C. A., Li, X. R., Yu, C. H., & Zhao, J. S. (2021). Does fintech innovation improve bank efficiency? Evidence from China's banking industry. International Review of Economics & Finance, 74 , 468-483. https://doi.org/10.1016/j.iref.2021.03.009
Leinweber, D. (2017). Fintech codgers look back 25 years. Journal of Investing, 26 (1), 33-45. https://doi.org/10.3905/joi.2017.26.1.033
Li, J. P., Li, J. Y., Zhu, X. Q., Yao, Y. H., & Casu, B. (2020). Risk spillovers between fintech and traditional financial institutions: Evidence from the U.S. International Review of Financial Analysis, 71 . https://doi.org/10.1016/j.irfa.2020.101544
Li, W. P., & Mei, F. (2020). Asset returns in deep learning methods: An empirical analysis on SSE 50 and CSI 300. Research in International Business and Finance, 54 . https://doi.org/10.1016/j.ribaf.2020.101291
Lin, T. C., & Pursiainen, V. (2021). The round number heuristic and entrepreneur crowdfunding performance. Journal of Corporate Finance, 68 . https://doi.org/10.1016/j.jcorpfin.2021.101894
Ling, S. X., Pei, T. Y., Li, Z. H., & Zhang, Z. P. (2021). Impact of COVID-19 on financial constraints and the moderating effect of financial technology. Emerging Markets Finance and Trade, 57 (6), 1675-1688. https://doi.org/10.1080/1540496x.2021.1904883
Liu, M., Wu, W. F., & Yu, T. (2019). Information, incentives, and effects of risk-sharing on the real economy. Pacific-Basin Finance Journal, 57 . https://doi.org/10.1016/j.pacfin.2018.12.004
Loo, M. K. L. (2019). Enhancing financial inclusion in ASEAN: Identifying the best growth markets for fintech. Journal of Risk and Financial Management, 12 (4). https://doi.org/10.3390/jrfm12040181
Luo, D., Mishra, T., Yarovaya, L., & Zhang, Z. (2021). Investing during a fintech revolution: Ambiguity and return risk in cryptocurrencies. Journal of International Financial Markets Institutions & Money, 73 . https://doi.org/10.1016/j.intfin.2021.101362
Maskara, P. K., Kuvvet, E., & Chen, G. X. (2021). The role of P2P platforms in enhancing financial inclusion in the United States: An analysis of peer-to-peer lending across the rural-urban divide. Financial Management, 50 (3), 747-774. https://doi.org/10.1111/fima.12341
McKillop, D., French, D., Quinn, B., Sobiech, A. L., & Wilson, J. O. S. (2020). Cooperative financial institutions: A review of the literature. International Review of Financial Analysis, 71 . https://doi.org/10.1016/j.irfa.2020.101520
Mhlanga, D. (2020). Industry 4.0 in Finance: The impact of artificial intelligence (AI) on digital financial inclusion. International Journal of Financial Studies, 8 (3). https://doi.org/10.3390/ijfs8030045
Miglo, A. (2021). STO vs. ICO: A theory of token issues under moral hazard and demand uncertainty. Journal of Risk and Financial Management, 14 (6). https://doi.org/10.3390/jrfm14060232
Mishchenko, S., Naumenkova, S., Mishchenko, V., & Dorofeiev, D. (2021). Innovation risk management in financial institutions. Investment Management and Financial Innovations, 18 (1), 191-203. https://doi.org/10.21511/imfi.18(1).2021.16
Najaf, K., Schinckus, C., & Yoong, L. C. (2021). VaR and market value of fintech companies: an analysis and evidence from global data. Managerial Finance, 47 (7), 915-936. https://doi.org/10.1108/mf-04-2020-0169
Nastiti, N. D., & Kasri, R. A. (2019). The role of banking regulation in the development of Islamic banking financing in Indonesia. International Journal of Islamic and Middle Eastern Finance and Management, 12 (5), 643-662. https://doi.org/10.1108/imefm-10-2018-0365
Neale, F. R., Drake, P. P., & Konstantopoulos, T. (2020). InsurTech and the disruption of the insurance industry. Journal of Insurance Issues, 43 (2), 64-96. Retrieved from https://www.jstor.org/stable/26931211
Olsen, R., Battiston, S., Caldarelli, G., Golub, A., Nikulin, M., & Ivliev, S. (2018). Case study of Lykke exchange: Architecture and outlook. Journal of Risk Finance, 19 (1), 26-38. https://doi.org/10.1108/jrf-12-2016-0168
Ozik, G., Sadka, R., & Shen, S. Y. (2021). Flattening the illiquidity curve: Retail trading during the COVID-19 lockdown. Journal of Financial and Quantitative Analysis, 56 (7), 2356-2388. https://doi.org/10.1017/s0022109021000387
Ozili, P. K. (2022). Banking sector earnings management using loan loss provisions in the fintech era. International Journal of Managerial Finance, 18 (1), 75-93. https://doi.org/10.1108/ijmf-07-2020-0369
Petrushenko, Y., Kozarezenko, L., Glinska-Newes, A., Tokarenko, M., & But, M. (2018). The opportunities of engaging fintech companies into the system of crossborder money transfers in Ukraine. Investment Management and Financial Innovations, 15 (4), 332-344. https://doi.org/10.21511/imfi.15(4).2018.27
Petukhina, A. A., Reule, R. C. G., & Hardle, W. K. (2021). Rise of the machines? Intraday high-frequency trading patterns of cryptocurrencies. European Journal of Finance, 27 (1-2), 8-30. https://doi.org/10.1080/1351847x.2020.1789684
Phan, D. H. B., Narayan, P. K., Rahman, R. E., & Hutabarat, A. R. (2020). Do financial technology firms influence bank performance? Pacific-Basin Finance Journal, 62 . https://doi.org/10.1016/j.pacfin.2019.101210
Philippas, N. D., & Avdoulas, C. (2020). Financial literacy and financial well-being among generation-Z university students: Evidence from Greece. European Journal of Finance, 26 (4-5), 360-381. https://doi.org/10.1080/1351847x.2019.1701512
Pu, R. H., Teresiene, D., Pieczulis, I., Kong, J., & Yue, X. G. (2021). The interaction between banking sector and financial technology companies: Qualitative assessment-A case of Lithuania. Risks, 9 (1). https://doi.org/10.3390/risks9010021
Putri, W. H., Nurwiyanta, N., Sungkono, S., & Wahyuningsih, T. (2019). The emerging fintech and financial slack on corporate financial performance. Investment Management and Financial Innovations, 16 (2), 348-354. https://doi.org/10.21511/imfi.16(2).2019.29
Rosavina, M., Rahadi, R. A., Kitri, M. L., Nuraeni, S., & Mayangsari, L. (2019). P2P lending adoption by SMEs in Indonesia. Qualitative Research in Financial Markets, 11 (2), 260-279. https://doi.org/10.1108/qrfm-09-2018-0103
Rupeika-Apoga, R., & Wendt, S. (2021). Fintech in Latvia: Status quo, current developments, and challenges ahead. Risks, 9 (10). https://doi.org/10.3390/risks9100181
Santosa, P. W. (2020). Determinants of price reversal in high-frequency trading: Empirical evidence from Indonesia. Investment Management and Financial Innovations, 17 (1), 175-187. https://doi.org/10.21511/imfi.17(1).2020.16
Savchuk, N., Bludova, T., Leonov, D., Murashko, O., & Shelud'Ko, N. (2021). Innovation imperatives of global financial innovation and development of their matrix models. Investment Management and Financial Innovations, 18 (3), 312-326. https://doi.org/10.21511/imfi.18(3).2021.26
Schulte, P., & Liu, G. (2018). Fintech is merging with IoT and AI to challenge banks: How entrenched interests can prepare. Journal of Alternative Investments, 20 (3), 41-57. https://doi.org/10.3905/jai.2018.20.3.041
Schwienbacher, A. (2019). Equity crowdfunding: Anything to celebrate? Venture Capital, 21 (1), 65-74. https://doi.org/10.1080/13691066.2018.1559010
Seiler, V., & Fanenbruck, K. M. (2021). Acceptance of digital investment solutions: The case of robo advisory in Germany. Research in International Business and Finance, 58 . https://doi.org/10.1016/j.ribaf.2021.101490
Selim, M. (2021). The effects of eliminating Riba in foreign currency transactions by introducing global Fintech network. International Journal of Islamic and Middle Eastern Finance and Management, 14 (3), 506-523. https://doi.org/10.1108/imefm-01-2020-0035
Semko, R. (2019). Machine learning for robo-advisors: Testing for neurons specialization. Investment Management and Financial Innovations, 16 (4), 205-214. https://doi.org/10.21511/imfi.16(4).2019.18
Sharma, Z., & Zhu, Y. (2020). Platform building in initial coin offering market: Empirical evidence. Pacific-Basin Finance Journal, 61 . https://doi.org/10.1016/j.pacfin.2020.101318
Sheng, T. X. (2021). The effect of fintech on banks' credit provision to SMEs: Evidence from China. Finance Research Letters, 39 . https://doi.org/10.1016/j.frl.2020.101558
Shrestha, K. (2021). Multifractal detrended fluctuation analysis of return on bitcoin. International Review of Finance, 21 (1), 312-323. https://doi.org/10.1111/irfi.12256
Stulz, R. M. (2019). Fintech, Bigtech, and the future of banks. Journal of Applied Corporate Finance, 31 (4), 86-97. https://doi.org/10.1111/jacf.12378
Sybirianska, Y., Dyba, M., Britchenko, I., Ivashchenko, A., Vasylyshen, Y., & Polishchuk, Y. (2018). Fintech platforms in sme’s financing: eu experience and ways of their application in Ukraine. Investment Management and Financial Innovations, 15 (3), 83-96. https://doi.org/10.21511/imfi.15(3).2018.07
Takeda, F., Takeda, K., Takemura, T., & Ueda, R. (2021). The impact of information technology investment announcements on the market value of the Japanese regional banks. Finance Research Letters, 41 . https://doi.org/10.1016/j.frl.2020.101811
Tantri, P. (2021). Fintech for the poor: Financial intermediation without discrimination. Review of Finance, 25 (2), 561-593. https://doi.org/10.1093/rof/rfaa039
Tseng, P. L., & Guo, W. C. (2021). Fintech, credit market competition, and bank asset quality. Journal of Financial Services Research . https://doi.org/10.1007/s10693-021-00363-y
Uddin, A., & Yu, D. T. (2020). Latent factor model for asset pricing. Journal of Behavioral and Experimental Finance, 27 . https://doi.org/10.1016/j.jbef.2020.100353
Uddin, M. H., Mollah, S., & Ali, M. H. (2020). Does cyber tech spending matter for bank stability? International Review of Financial Analysis, 72 . https://doi.org/10.1016/j.irfa.2020.101587
Ullah, A., Pinglu, C., Ullah, S., Qian, N. Y., & Zaman, M. (2021). Impact of intellectual capital efficiency on financial stability in banks: Insights from an emerging economy abstract. International Journal of Finance & Economics . https://doi.org/10.1002/ijfe.2512
Vasenska, I., Dimitrov, P., Koyundzhiyska-Davidkova, B., Krastev, V., Durana, P., & Poulaki, I. (2021). Financial transactions using Fintech during the Covid-19 crisis in Bulgaria. Risks, 9 (3). https://doi.org/10.3390/risks9030048
Wang, R., Liu, J. T., & Luo, H. (2021). Fintech development and bank risk taking in China. European Journal of Finance, 27 (4-5), 397-418. https://doi.org/10.1080/1351847x.2020.1805782
Wang, Y., & Drabek, Z. (2021). Adverse selection in P2P lending: Does peer screening work efficiently?-Empirical evidence from a P2P platform. International Journal of Financial Studies, 9 (4). https://doi.org/10.3390/ijfs9040073
Yang, D., & Li, M. (2018). Evolutionary approaches and the construction of technology-driven regulations. Emerging Markets Finance and Trade, 54 (14), 3256-3271. https://doi.org/10.1080/1540496x.2018.1496422
Yang, W., Sui, X. P., & Qi, Z. (2021). Can fintech improve the efficiency of commercial banks?-An analysis based on big data. Research in International Business and Finance, 55 . https://doi.org/10.1016/j.ribaf.2020.101338
Yao, T., & Song, L. R. (2021). Fintech and the economic capital of Chinese commercial bank's risk: Based on theory and evidence. International Journal of Finance & Economics . https://doi.org/10.1002/ijfe.2528
Yao, Y. H., Li, J. P., & Sun, X. L. (2021). Measuring the risk of Chinese Fintech industry: Evidence from the stock index. Finance Research Letters, 39 . https://doi.org/10.1016/j.frl.2020.101564
Yehorycheva, S., Fysun, I., Hudz, T., Palchuk, O., & Boiko, N. (2020). Innovations in the insurance market of a developing country: Case of Ukraine. Investment Management and Financial Innovations, 17 (4), 175-188. https://doi.org/10.21511/imfi.17(4).2020.17
Zhang, A. L., Wang, S. Y., Liu, B., & Liu, P. (2020). How fintech impacts pre- and post-loan risk in Chinese commercial banks. International Journal of Finance & Economics . https://doi.org/10.1002/ijfe.2284
Zhang, X., & Wu, C. (2018). Continuous cash flow payment: Theories and practice framework. Emerging Markets Finance and Trade, 54 (4), 774-782. https://doi.org/10.1080/1540496x.2016.1241706
Zhao, X. J., Hou, W. X., An, J. F., Liu, X. D., & Zhang, Y. (2021). Initial coin offerings: What rights do investors have? European Journal of Finance, 27 (4-5), 305-320. https://doi.org/10.1080/1351847x.2020.1858130
Zhong, W. Q., & Jiang, T. F. (2021). Can internet finance alleviate the exclusiveness of traditional finance? Evidence from Chinese P2P lending markets. Finance Research Letters, 40 . https://doi.org/10.1016/j.frl.2020.101731
Zhou, X., & Chen, S. (2021). Fintech innovation regulation based on reputation theory with the participation of new media. Pacific-Basin Finance Journal, 67 . https://doi.org/10.1016/j.pacfin.2021.101565
Appendix 2 Sample of 70 Fintech IS articles
Alam, M. M., Awawdeh, A. E., & Bin Muhamad, A. I. (2021). Using e-wallet for business process development: Challenges and prospects in Malaysia. Business Process Management Journal, 27 (4), 1142-1162. https://doi.org/10.1108/bpmj-11-2020-0528
Alhassan, M. D., Kolog, E. A., & Boateng, R. (2020). Effect of gratification on user attitude and continuance use of mobile payment services: A developing country context. Journal of Systems and Information Technology, 22 (4), 353-380. https://doi.org/10.1108/JSIT-01-2020-0010
Alt, R., Beck, R., & Smits, M. T. (2018). Fintech and the transformation of the financial industry. Electronic Markets, 28 (3), 235-243. https://doi.org/10.1007/s12525-018-0310-9
Alyakoob, M., Rahman, M. S., & Wei, Z. Y. (2021). Where you live matters: local bank competition, online marketplace lending, and disparity in borrower benefits. Information Systems Research, 32 (4), 1390-1411. https://doi.org/10.1287/isre.2021.1043
Au, C. H., Tan, B. N., & Sun, Y. (2020). Developing a P2P lending platform: Stages, strategies and platform configurations. Internet Research, 30 (4), 1229-1249. https://doi.org/10.1108/intr-03-2019-0099
Barbu, C. M., Florea, D. L., Dabija, D. C., & Barbu, M. C. R. (2021). Customer experience in fintech. Journal of Theoretical and Applied Electronic Commerce Research, 16 (5), 1415-1433. https://doi.org/10.3390/jtaer16050080
Belanche, D., Casalo, L. V., & Flavian, C. (2019). Artificial intelligence in fintech: Understanding robo-advisors adoption among customers. Industrial Management & Data Systems, 119 (7), 1411-1430. https://doi.org/10.1108/imds-08-2018-0368
Bongomin, G. O. C., & Ntayi, J. M. (2020). Mobile money adoption and usage and financial inclusion: Mediating effect of digital consumer protection. Digital Policy Regulation and Governance, 22 (3), 157-176. https://doi.org/10.1108/dprg-01-2019-0005
Bunnell, L., Osei-Bryson, K. M., & Yoon, V. Y. (2020). FinPathlight: Framework for an multiagent recommender system designed to increase consumer financial capability. Decision Support Systems, 134 . https://doi.org/10.1016/j.dss.2020.113306
Burtch, G., Hong, Y. L., & Liu, D. (2018). The Role of provision points in online crowdfunding. Journal of Management Information Systems, 35 (1), 117-144. https://doi.org/10.1080/07421222.2018.1440764
Chatterjee, S., Sarker, S., Lee, M. J., Xiao, X., & Elbanna, A. (2021). A possible conceptualization of the information systems (IS) artifact: A general systems theory perspective(1). Information Systems Journal, 31 (4), 550-578. https://doi.org/10.1111/isj.12320
Chen, X. R., Hu, X. J., & Ben, S. L. (2021). How individual investors react to negative events in the fintech era? Evidence from China's peer-to-peer lending market. Journal of Theoretical and Applied Electronic Commerce Research, 16 (1), 52-70. https://doi.org/10.4067/s0718-18762021000100105
Coffie, C. P. K., Hongjiang, Z., Mensah, I. A., Kiconco, R., & Simon, A. E. O. (2021). Determinants of fintech payment services diffusion by SMEs in Sub-Saharan Africa: evidence from Ghana. Information Technology for Development, 27 (3), 539-560. https://doi.org/10.1080/02681102.2020.1840324
Cui, X., Liu, W., & He, Z. (2021). Attribute reduction algorithm of enterprise credit evaluation and its applications for web-based communities. International Journal of Web Based Communities, 17 (4), 293-304. https://doi.org/10.1504/IJWBC.2021.119473
Currie, W. L., Gozman, D. P., & Seddon, J. J. M. (2018). Dialectic tensions in the financial markets: A longitudinal study of pre- and post-crisis regulatory technology. Journal of Information Technology, 33 (4), 304-325. https://doi.org/10.1057/s41265-017-0047-5
Dehnert, M., & Schumann, J. (2022). Uncovering the digitalization impact on consumer decision-making for checking accounts in banking. Electronic Markets, 32 (3). https://doi.org/10.1007/s12525-022-00524-4
Dhar, V., & Stein, R. M. (2017). Economic and business dimensions fintech platforms and strategy. Communications of the Acm, 60 (10), 32-35. https://doi.org/10.1145/3132726
Du, K. (2018). Complacency, capabilities, and institutional pressure: Understanding financial institutions' participation in the nascent mobile payments ecosystem. Electronic Markets, 28 (3), 307-319. https://doi.org/10.1007/s12525-017-0267-0
Du, W. Y., Pan, S. L., Leidner, D. E., & Ying, W. C. (2019). Affordances, experimentation and actualization of fintech: A blockchain implementation study. Journal of Strategic Information Systems, 28 (1), 50-65. https://doi.org/10.1016/j.jsis.2018.10.002
Fu, R. S., Huang, Y., & Singh, P. V. (2021). Crowds, lending, machine, and bias. Information Systems Research, 32 (1), 72-92. https://doi.org/10.1287/isre.2020.0990
Gao, Z., Guo, Z., & Tang, Q. (2021). How do monetary incentives influence giving? An empirical investigation of matching subsidies on kiva. Information Systems and e-Business Management . https://doi.org/10.1007/s10257-021-00515-6
Ge, R. Y., Feng, J., Gu, B., & Zhang, P. Z. (2017). Predicting and deterring default with social media information in peer-to-peer lending. Journal of Management Information Systems, 34 (2), 401-424. https://doi.org/10.1080/07421222.2017.1334472
Gimpel, H., Rau, D., & Roglinger, M. (2018). Understanding fintech start-ups - A taxonomy of consumer-oriented service offerings. Electronic Markets, 28 (3), 245-264. https://doi.org/10.1007/s12525-017-0275-0
Gomber, P., Kauffman, R. J., Parker, C., & Weber, B. W. (2018). On the fintech revolution: Interpreting the forces of innovation, disruption, and transformation in financial services. Journal of Management Information Systems, 35 (1), 220-265. https://doi.org/10.1080/07421222.2018.1440766
Gozman, D., Liebenau, J., & Mangan, J. (2018). The Innovation mechanisms of fintech start-ups: Insights from SWIFT's Innotribe competition. Journal of Management Information Systems, 35 (1), 145-179. https://doi.org/10.1080/07421222.2018.1440768
Haitao, S. (2020). Big data analysis of e-commerce loan risk of college students in the context of network finance. Information Systems and e-Business Management, 18 (3), 439-454. https://doi.org/10.1007/s10257-019-00424-9
Han, H., Teng, J., Xia, J. R., Wang, Y. H., Guo, Z. H., & Li, D. Q. (2021). Predict high-frequency trading marker via manifold learning. Knowledge-Based Systems, 213 . https://doi.org/10.1016/j.knosys.2020.106662
Hendershott, T., Zhang, X. Q., Zhao, J. L., & Zheng, Z. Q. (2021). Fintech as a game changer: Overview of research frontiers. Information Systems Research, 32 (1), 1-17. https://doi.org/10.1287/isre2021.0997
Hua, X. P., Huang, Y. P., & Zheng, Y. F. (2019). Current practices, new insights, and emerging trends of financial technologies. Industrial Management & Data Systems, 119 (7), 1401-1410. https://doi.org/10.1108/imds-08-2019-0431
Ilk, N., Shang, G. Z., Fan, S. K., & Zhao, J. L. (2021). Stability of transaction fees in BitCoin: a supply and demand perspective. MIS Quarterly, 45 (2), 563-592. https://doi.org/10.25300/misq/2021/15718
Jiang, Y., Ho, Y. C., Yan, X. B., & Tan, Y. (2018). Investor platform choice: Herding, platform attributes, and regulations. Journal of Management Information Systems, 35 (1), 86-116. https://doi.org/10.1080/07421222.2018.1440770
Jin, Y., Ding, C., Duan, Y., & Cheng, H. K. (2020). Click to success? The temporal effects of facebook likes on crowdfunding. Journal of the Association for Information Systems, 21 (5), 1191-1213. https://doi.org/10.17705/1jais.00634
Jinasena, D. N., Spanaki, K., Papadopoulos, T., & Balta, M. E. (2023). Success and failure retrospectives of fintech projects: A case study approach. Information Systems Frontiers . https://doi.org/10.1007/s10796-020-10079-4
Jung, D., Dorner, V., Weinhardt, C., & Pusmaz, H. (2018). Designing a robo-advisor for risk-averse, low-budget consumers. Electronic Markets, 28 (3), 367-380. https://doi.org/10.1007/s12525-017-0279-9
Lagna, A., & Ravishankar, M. N. (2022). Making the world a better place with fintech research. Information Systems Journal, 32 (1), 61-102. https://doi.org/10.1111/isj.12333
Leong, C., Tan, B., Xiao, X., Tan, F. T. C., & Sun, Y. (2017). Nurturing a fintech ecosystem: The case of a youth microloan startup in China. International Journal of Information Management, 37 (2), 92-97. https://doi.org/10.1016/j.ijinfomgt.2016.11.006
Li, M. Y., Qin, Y., Liu, B., & Chu, X. W. (2021). Enhancing the efficiency and scalability of blockchain through probabilistic verification and clustering. Information Processing & Management, 58 (5). https://doi.org/10.1016/j.ipm.2021.102650
Lim, S. H., Kim, D. J., Hur, Y., & Park, K. (2019). An empirical study of the impacts of perceived security and knowledge on continuous intention to use mobile fintech payment services. International Journal of Human-Computer Interaction, 35 (10), 886-898. https://doi.org/10.1080/10447318.2018.1507132
Mai, F., Shan, Z., Bai, Q., Wang, X., & Chiang, R. H. L. (2018). How does social media impact Bitcoin value? A test of the silent majority hypothesis. Journal of Management Information Systems, 35 (1), 19-52. https://doi.org/10.1080/07421222.2018.1440774
Muthukannan, P., Tan, B., Gozman, D., & Johnson, L. (2020). The emergence of a fintech ecosystem: A case study of the Vizag fintech valley in India. Information & Management, 57 (8). https://doi.org/10.1016/j.im.2020.103385
Muthukannan, P., Tan, B., Tan, F. T., & Leong, C. (2021). Novel mechanisms of scalability of financial services in an emerging market context: Insights from Indonesian fintech Ecosystem. International Journal of Information Management, 61 . https://doi.org/10.1016/j.ijinfomgt.2021.102403
Nayak, B., Bhattacharyya, S. S., & Krishnamoorthy, B. (2019). Integrating wearable technology products and big data analytics in business strategy: A study of health insurance firms. Journal of Systems and Information Technology, 21 (2), 255-275. https://doi.org/10.1108/JSIT-08-2018-0109
Odoom, R., & Kosiba, J. P. (2020). Mobile money usage and continuance intention among micro enterprises in an emerging market – The mediating role of agent credibility. Journal of Systems and Information Technology, 22 (4), 97-117. https://doi.org/10.1108/JSIT-03-2019-0062
Ozili, P. K. (2020). Contesting digital finance for the poor. Digital Policy Regulation and Governance, 22 (2), 135-151. https://doi.org/10.1108/dprg-12-2019-0104
Pousttchi, K., & Dehnert, M. (2018). Exploring the digitalization impact on consumer decision-making in retail banking. Electronic Markets, 28 (3), 265-286. https://doi.org/10.1007/s12525-017-0283-0
Puschmann, T. (2017). Fintech. Business & Information Systems Engineering, 59 (1), 69-76. https://doi.org/10.1007/s12599-017-0464-6
Ryu, H. S. (2018). What makes users willing or hesitant to use fintech? The moderating effect of user type. Industrial Management & Data Systems, 118 (3), 541-569. https://doi.org/10.1108/imds-07-2017-0325
Saura, J. R., Reyes-Menendez, A., deMatos, N., & Correia, M. B. (2021). Identifying startups business opportunities from UGC on Twitter chatting: An exploratory analysis. Journal of Theoretical and Applied Electronic Commerce Research, 16 (6), 1929-1944. https://doi.org/10.3390/jtaer16060108
Senyo, P. K., Karanasios, S., Gozman, D., & Baba, M. (2022). Fintech ecosystem practices shaping financial inclusion: The case of mobile money in Ghana. European Journal of Information Systems, 31 (1), 112-127. https://doi.org/10.1080/0960085x.2021.1978342
Senyo, P. K., Osabutey, E. L. C., & Kan, K. A. S. (2021). Pathways to improving financial inclusion through mobile money: A fuzzy set qualitative comparative analysis. Information Technology & People, 34 (7), 1997-2017. https://doi.org/10.1108/itp-06-2020-0418
Shiau, W. L., Yuan, Y., Pu, X. D., Ray, S., & Chen, C. C. (2020). Understanding fintech continuance: Perspectives from self-efficacy and ECT-IS theories. Industrial Management & Data Systems, 120 (9), 1659-1689. https://doi.org/10.1108/imds-02-2020-0069
Stewart, H., & Jurjens, J. (2018). Data security and consumer trust in fintech innovation in Germany. Information and Computer Security, 26 (1), 109-128. https://doi.org/10.1108/ics-06-2017-0039
Stoeckli, E., Dremel, C., & Uebernickel, F. (2018). Exploring characteristics and transformational capabilities of InsurTech innovations to understand insurance value creation in a digital world. Electronic Markets, 28 (3), 287-305. https://doi.org/10.1007/s12525-018-0304-7
Stojanovic, B., Hofer-Schmitz, K., & Kleb, U. (2020). APT datasets and attack modeling for automated detection methods: A review. Computers & Security, 92 . https://doi.org/10.1016/j.cose.2020.101734
Tan, T. H., Zhang, Y., Heng, C. S. A., & Ge, C. M. (2021). Empowerment of grassroots consumers: A revelatory case of a Chinese fintech innovation. Journal of the Association for Information Systems, 22 (1), 179-203. https://doi.org/10.17705/1jais.00658
Tao, Q. Z., Dong, Y. Z., & Lin, Z. M. (2017). Who can get money? Evidence from the Chinese peer-to-peer lending platform. Information Systems Frontiers, 19 (3), 425-441. https://doi.org/10.1007/s10796-017-9751-5
Vo, A., Chapman, T. A., & Lee, Y. S. Examining Bitcoin and economic determinants: an evolutionary perspective. Journal of Computer Information Systems . https://doi.org/10.1080/08874417.2020.1865851
Vucetic, M., Brokesova, Z., Hudec, M., & Pastorakova, E. (2022). Financial literacy and psychological disaster preparedness: Applicability of approach based on fuzzy functional dependencies. Information Processing & Management, 59 (2). https://doi.org/10.1016/j.ipm.2021.102848
Wang, Z., & Ben, S. (2021). Effect of consumers’ online shopping on their investment in money market funds on ecommerce platforms. Information Systems and e-Business Management . https://doi.org/10.1007/s10257-021-00516-5
Wang, Z., Jiang, C. Q., & Zhao, H. M. (2021). Know where to invest: Platform risk evaluation in online lending. Information Systems Research . https://doi.org/10.1287/isre.2021.1083
Wang, Z. N., Guan, Z. Z., Hou, F. F., Li, B. Y., & Zhou, W. Y. (2019). What determines customers' continuance intention of fintech? Evidence from YuEbao. Industrial Management & Data Systems, 119 (8), 1625-1637. https://doi.org/10.1108/imds-01-2019-0011
Wessel, M., Gleasure, R., & Kauffman, R. J. (2021). Sustainability of rewards-based crowdfunding: a quasi-experimental analysis of funding targets and backer satisfaction. Journal of Management Information Systems, 38 (3), 612-646. https://doi.org/10.1080/07421222.2021.1987622
Wingreen, S. C., Kavanagh, D., Ennis, P. J., & Miscione, G. (2020). Sources of cryptocurrency value systems: the case of Bitcoin. International Journal of Electronic Commerce, 24 (4), 474-496. https://doi.org/10.1080/10864415.2020.1806469
Wu, M. E., Syu, J. H., Srivastava, G., & Lin, J. C. W. (2022). Informative index for investment based on Kelly criterion. Enterprise Information Systems . https://doi.org/10.1080/17517575.2021.1939425
Xie, J. L., Ye, L. Y., Huang, W., & Ye, M. (2021). Understanding fintech platform adoption: Impacts of perceived value and perceived risk. Journal of Theoretical and Applied Electronic Commerce Research, 16 (5), 1893-1911. https://doi.org/10.3390/jtaer16050106
Xie, P., Chen, H. L., & Hu, Y. J. (2020). Signal or noise in social media discussions: the role of network cohesion in predicting the Bitcoin market. Journal of Management Information Systems, 37 (4), 933-956. https://doi.org/10.1080/07421222.2020.1831762
Xu, Y. L., Bao, H. J., Zhang, W. Y., & Zhang, S. (2021). Which financial earmarking policy is more effective in promoting fintech innovation and regulation? Industrial Management & Data Systems, 121 (10), 2181-2206. https://doi.org/10.1108/imds-11-2020-0656
Yeh, J. Y., & Chen, C. H. (2022). A machine learning approach to predict the success of crowdfunding fintech project. Journal of Enterprise Information Management . https://doi.org/10.1108/jeim-01-2019-0017
Yun, J. J., Liu, Z., & Zhao, X. F. (2021). Introduction: Ambidextrous open innovation in the 4th industrial revolution. Science Technology and Society, 26 (2), 183-200. https://doi.org/10.1177/09717218211006969
Zhao, Y., & Chen, X. H. (2022). The relationship between the withdrawal of the digital economy's innovators, government interventions, the marketization level and market size based on big data. Journal of Enterprise Information Management . https://doi.org/10.1108/jeim-01-2021-0050
Rights and permissions
Springer Nature or its licensor (e.g. a society or other partner) holds exclusive rights to this article under a publishing agreement with the author(s) or other rightsholder(s); author self-archiving of the accepted manuscript version of this article is solely governed by the terms of such publishing agreement and applicable law.
Reprints and permissions
About this article
Jourdan, Z., Corley, J.K., Valentine, R. et al. Fintech: A content analysis of the finance and information systems literature. Electron Markets 33 , 2 (2023). https://doi.org/10.1007/s12525-023-00624-9
Download citation
Received : 07 April 2022
Accepted : 26 January 2023
Published : 03 April 2023
DOI : https://doi.org/10.1007/s12525-023-00624-9
Share this article
Anyone you share the following link with will be able to read this content:
Sorry, a shareable link is not currently available for this article.
Provided by the Springer Nature SharedIt content-sharing initiative
- Qualitative
- Content analysis
JEL classification
Advertisement
- Find a journal
- Publish with us
- Track your research
Financial Research Paper Topics: Interesting Finance Questions to Uncover
Are you having trouble thinking of a good topic for your finance research paper? Believe it or not, you are not alone. It might be difficult to find the perfect financial research topic time and time again. After all, picking the right subject is crucial to your financial field. Whether you’re putting together a presentation, penning an essay, or doing research papers, your choice of subject is of critical significance.
To aid you in overcoming this obstacle, we have compiled a detailed list of organized finance topics for research papers. If you want to be sure you choose the right subject for your financial management efforts, we’ve provided a concise guide with crucial advice.
How to Choose Topics for a Finance Research Paper?
If you need assistance deciding on a subject for your finance research paper, here are some pointers. But before we get into those pointers, it’s important to keep in mind that custom writing services may be a great resource for choosing finance topics for your research paper. You may save yourself time and effort by relying on their staff of seasoned writers to help you choose a subject that is both interesting and applicable to your assignment. The following are three guidelines for deciding on a subject for a finance research paper:
- Find Unanswered Questions : Try to pinpoint issues that haven’t received enough attention so far in financial research. You may add to the corpus of knowledge already available by identifying information gaps. Investigate financial management, traditional finance, corporate finance, personal finance and similar topics in order to develop a workable solution or to provide novel ideas.
- Review Existing Literature : Gaining familiarity with the state of the art in finance research requires reading theses and academic articles. Doing so will aid you in pinpointing certain niches in which you may excel. Search the literature for broad perspectives or recurring themes that might help you zero in on a particular issue.
- Stay Updated and Seek Input : Conduct internet research to keep up with the latest financial concerns. Investigate pressing concerns in the industry, such as the effects of the global financial crisis or new developments in the financial markets. You should also talk about your topic with others who have written research papers, such as your friends, classmates, or professors. Getting their thoughts might help you hone your subject and provide vital information.
Where to Get Data for Finance Papers?
It is crucial to get accurate and up-to-date information while conducting studies in the financial sector. One efficient method is to pay for papers or to hire a finance researcher and analysts to do the work for you, especially when it comes to personal finance.
- ProQuest is a significant tool since it provides access to scholarly literature from every field of study in the form of periodicals, newspapers, industry reports, dissertations, and profiles of prominent businesses.
- Scopus and Web of Science provide a plethora of resources, including journals, books, and conference proceedings, that provide comprehensive coverage across academic subjects.
- Global Financial Data (GFD) is one such database that caters only to finance research, and its extensive research has a wealth of data on various asset classes, prices, indexes, and currency exchange rates.
- Bloomberg, Thomson Reuters Datastream, and WRDS provide faculty and researchers with institutional access to a plethora of financial data and tools. This includes real-time market data, financial statements, economic indicators, and personal finance topics to write about.
List of Finance Research Topics
This exhaustive list covers everything you need, whether you’re an MBA student, a finance management professional, or a college student. Explore the exciting field of finance research, delving into areas like healthcare financing, the latest developments in the field, corporate finance, and the aftereffects of the global financial crisis. The finance research papers” in this volume will keep you interested and well-informed.
Finance Research Topics for MBA
Investment analysis, financial management, and personal finance are just a few of the many disciplines that fall under the umbrella of finance research subjects for MBA students. Such topics in finance are essential because they provide MBA students with a solid grounding in financial theory and practice. Here are a few suggestions for MBA students looking for research topics in finance:
- Risk Management Strategies in Financial Institutions.
- Behavioral Finance in Investment Decision-Making.
- Financial Inclusion and Economic Development.
- Comparative Analysis of IFRS Adoption and Financial Reporting Quality.
- Impact of Financial Technology (Fintech) on Traditional Banking.
Finance Management Research Topics
Finance management topics include a broad spectrum of areas that dive into the complexities of managing financial resources in different contexts. Investment analysis, risk management, financial markets, and corporate finance all fall under finance management. Writing a finance research paper helps you understand financial decision-making, develop effective strategies, and advance the field. Before commencing your research paper, consider the following finance research paper ideas:
- Corporate Risk Management Strategies On Firm Performance.
- Benefit Investment Management Practices In Pension Funds.
- Assessing Financial Risks And Mitigation Techniques In Developing Market Multinationals.
- Electronic Banking And Financial Inclusion In Developed And Developing Nations.
- An Empirical Study Of Investor Behavior And Global Finance Data.
Healthcare Finance Research Topics
Explore the application of financial theory to the healthcare sector while writing about finance research paper topics. This financial research is essential for expanding our knowledge of healthcare economics, investment strategies, cost control, and healthcare policy. Finance researchers may also investigate intricate monetary systems to enhance healthcare services and the health of patients. Some healthcare finance topics might include the following:
- Impact Of Healthcare Policy On Financial Sustainability.
- Cost-Effectiveness Analysis Of Healthcare Interventions.
- Healthcare Reimbursement Models And Their Impact On Healthcare Providers.
- Economic Evaluation Of Preventive Healthcare Programs.
- Healthcare Financing And Access To Care For Underserved Populations.
Interesting Finance Dissertation Topics
For the purposes of writing finance research papers and finishing a dissertation, investigating interesting finance topics is essential. You can gain a more thorough comprehension of economic principles and their real-world applications. In order to have a high-quality research paper done quickly and with no effort, it’s a good idea to look into help with dissertation writing services. For your next research paper, you can consider the following interesting financial topics:
- The banking sector and digital transformation: customer experience and operational effectiveness.
- Corporate risk management strategies in the banking industry: Traditional vs. developing risk management procedures.
- A case study of emerging nations and how well-functioning financial systems foster economic progress.
- Financial aid programs in promoting access to higher education
- A post-pandemic examination of banking institutions’ resilience and regulatory measures’ systemic risk mitigation.
Current Research Topics in Finance
Examining current finance research paper topics is essential due to the dynamic nature of the financial industry. By digging into current financial topics to write about, you learn more about the market, investing methods, risk management, and more. This financial research supports decision-making, policy-making, and the development of new financial solutions. Here are a few lists of subjects to consider if you are looking for current financial topics to write about.
- Financial Statement Analysis And Investment Decisions In Different Industries.
- Exploring The Effectiveness Of Machine Learning Algorithms In Predicting Financial Asset Prices.
- The Role Of Financial Derivatives In Managing Risk And Enhancing Returns In The Business Sector.
- Corporate Governance Practices On Financial Performance And Asset Valuation.
- Sustainable Finance Projects In Promoting Environmental, Social, And Governance (ESG) Goals.
Best Finance Research Topics
A finance research paper topic requires the identification of intriguing subjects for extensive research. The best financial research opens the door to explorations of many facets of finance, including investing tactics and the stock market. As you start to write research papers on finance topics, you’ll open up opportunities for self-discovery, theory-building, and prudent decision-making. You’ll also help them become better researchers and writers, leading to better articles.
- Artificial Intelligence and Financial Decision-Making.
- Financial Risk Management in the Age of Cryptocurrencies.
- Behavioral Finance and Investment Decision-Making.
- The Effectiveness of Financial Regulations in Preventing Market Manipulation.
- The Role of Fintech in Financial Inclusion: Case Studies from the United States.
Interesting Finance Topics for College Students
Among the many subsets that make up the umbrella term finance topics for college students are financial research and finance topics for paper. Financial research topics are important because they help students learn the fundamentals of finance, get them ready for the issues they’ll face in the real world, and develop the analytical thinking they’ll need to make sound judgments in the future. Here are a few examples of finance topics to talk about among college students:
- A Comparative Study of E-commerce on Traditional Retail Banking.
- Comparing Interest Rate Changes with Stock Market Volatility in Developed and Emerging Markets.
- The Effectiveness of Microfinance Institutions in Alleviating Poverty.
- Financial Education Programs and College Students’ Financial Decision-Making.
- Initial Public Offering (IPO) Underpricing: Comparative Study of Developed and Developing Markets.
Finance Research Paper Topics for University Students
Investing, banking, corporate finance, and other areas fall under the umbrella of finance-related topics for the purposes of a university research paper. Because it deepens their knowledge, sparks new ideas, and helps the financial sector expand, topics in finance are more important for college students to study. Students who buy custom assignments benefit from individualized attention, time savings, and the insight of subject matter experts. Check out our extensive finance research topic list to uncover interesting topics for your next paper.
- Interest Rate Changes On Corporate Borrowing And Investment Decisions.
- Financial Literacy And Investment Behavior Among University Students.
- Impact Of International Trade And Globalization On Financial Markets.
- Factors Influencing Mergers And Acquisitions In The Financial Industry.
- Financial Derivatives In Managing Risk In The Stock Market.
Public Finance Research Topics
Research Topics in Public Finance include a broad spectrum of questions concerning fiscal and monetary policy at the national, state, and local levels of government. Understanding the effects of government spending and fiscal policies on GDP growth, income distribution, and social welfare is essential, which is why studies in this field are so important. Policymakers can do better for the world when they have access to information on financial research paper topics to read about.
- The potential of digital currencies as financial assets in public finance management.
- Impact of Tax Policy on Economic Growth: A Comparative Study.
- Government Debt and its Implications on Fiscal Sustainability.
- Public-Private Partnerships in Infrastructure Development.
- Effectiveness of Fiscal Stimulus Packages in Times of Economic Crisis.
Corporate Finance Research Topics
Corporate Finance Research explores various financial management topics within businesses. Conducting research in this area is crucial for understanding financial decision-making, risk management, capital structure, and valuation. It helps companies optimize their financial strategies, make informed investment decisions, and enhance overall financial performance.
- Corporate Governance and Financial Performance: An Industry Comparison.
- Debt Financing in Manufacturing Sector Corporate Investment Decisions.
- Corporate Taxation and Capital Structure Decisions: A Comparative Study of Countries.
- Corporate Venture Capital and Startup Financing: A Comparative Analysis.
- Corporate Governance Mechanisms and Capital Allocation Efficiency: Emerging Markets.
Business Finance Research Topics
Subjects that fall under the umbrella of business finance topics include any and all discussions of how businesses handle their money, from budgeting to investing to making important business decisions. Researching business finance is essential since it reveals new tendencies, aids in the creation of cutting-edge tactics, and boosts monetary output. It helps companies maintain competitiveness in a fast-paced industry and make well-informed choices. These samples can assist you whether you are looking for financial research paper topics or investment research paper ideas.
- Corporate Social Responsibility and Financial Performance.
- Exchange Rate Fluctuations on International Business Transactions.
- Financial Innovation and SME Financing.
- Financial Markets in Economic Development.
- Financial Leverage and Firm Value in Different Industries.
Related posts:
- Proposal Essay Topics Ideas
200 Best Ideas for Research Paper Topics in 2023
- Good Essay Topics & Ideas for College by Edusson
- Medical Research Paper Topics: Ideas on Healthcare and Medical Science
Improve your writing with our guides
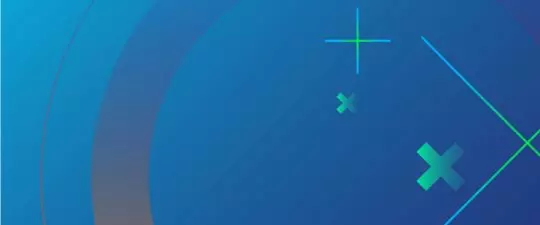
Psychology Essay Topic: Theories Explaining Human growth and Development
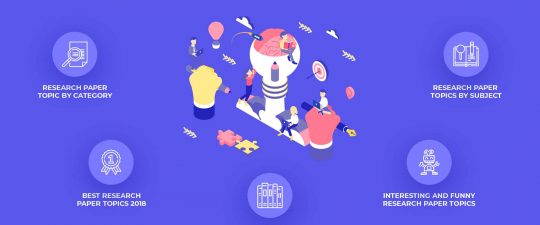
Reflection Paper Topics: Art
Get 15% off your first order with edusson.
Connect with a professional writer within minutes by placing your first order. No matter the subject, difficulty, academic level or document type, our writers have the skills to complete it.
100% privacy. No spam ever.
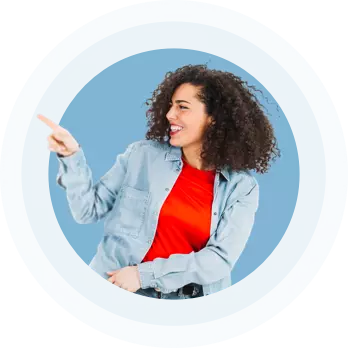
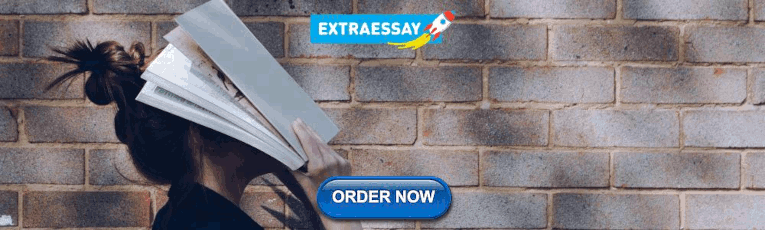
IMAGES
VIDEO
COMMENTS
Table 1a provides a review summary of various research papers, including their problem type, the model used, and the Dataset. In the past, various quantitative and qualitative methods have been utilized for financial and accounting research. ... While we provided a systematic analysis of financial data from various sources, including the ...
The key takeaways from the PwC study, regarding the respondent's choice of analytics, are that the "highly data-driven" decision-makers: 1) "look backwards, when needed"; 2) "use predictive and prescriptive analytics to model the future"; and 3) "take a more holistic approach" to decision-making (PwC, 2016 ). Table 1.
In this paper, I provide an overview of the research on the real effects of financial reporting on investing and financing decisions made by firms. Accounting can improve investment efficiency and affect nearly every aspect of the financing decision by reducing information asymmetry and improving monitoring.
The Journal of Finance and Data Science (JFDS) is the leading interdisciplinary journal on finance and data science, providing detailed analyses of theoretical and empirical foundations and their applications in financial economics. JFDS publishes evaluations of both well-established and new theories using financial data, data-scientific measurements of variables relevant to financial decision ...
Abstract. Data analysis has become a cornerstone in the realm of finance management, transforming the way financial decisions are made and strategies are formulated. In an era where information is ...
Big data is one of the most recent business and technical issues in the age of technology. Hundreds of millions of events occur every day. The financial field is deeply involved in the calculation of big data events. As a result, hundreds of millions of financial transactions occur in the financial world each day. Therefore, financial practitioners and analysts consider it an emerging issue of ...
In this introduction, we try to define what "big data" encompasses in the context of finance research. We then review the six papers included in the special issue, discussing how they are related to each other and to the general theme. Finally, we provide some thoughts for future research directions. 2.
Abstract. We live in an era of big data. Large volumes of complex and difficult-to-analyze data exist in a variety of industries, including the financial sector. In this paper, we investigate the ...
The literature data used in this paper are obtained from WoS (Falagas et al. 2008), one of the most widely used databases in academics, owned by Thomson Reuters Corporation.In this paper, we derived data through the search function in WoS by selecting as Database = Web of Science ™ Core Collection database; Topic search = FinTech or "Financial technology" or "Financial technologies ...
Deep Learning provided powerful tools for forecasting financial time series data. However, despite the success of these approaches on many challenging financial forecasting tasks, it is not always straightforward to employ DL-based approaches for highly volatile and non-stationary time financial series. To this end, in this paper, an adaptive input normalization layer that can learn to ...
The balance of this paper is organised as follows: ... As the digital currency industry has become increasingly important in the financial world, future research should study the impact of regulations and blockchain progress on the ... (2016) Features selection, data mining and financial risk classification: a comparative study. Intell Syst ...
Financial analysis is a study of the company's finan cial statements by analyzing the reports. Report. analysis is a tool that easily calculates and interprets reports that are used by investors ...
The potential value of open financial data ranges from 1 percent to as much as 5 percent of GDP, depending on economic structure and levels of financial access. Aggregating the potential GDP impact across the 24 use cases to the economy level, we find significant value at stake overall and for all market participants.
on historical costs. The financial statements are prepared periodically for the accounting period. 1. Financial statements as composed of data, which are the results. 2. Recorded facts concerning business transaction. 3. Convention adopted to facilitate the accounting technique. 4. Postulates or assumptions made to personal judgment. 5.
Financial Stress Index. This index is a daily market-based snapshot of stress in global markets. The OFR Financial Stress Index is positive when stress levels are above average, and negative when stress levels are below average. View OFR's Financial Stress Index. Current Index: -1.869. Apr. 11, 2024 (not seasonally adjusted)
The amount of research related to financial technologies (fintech) has grown rapidly since these modalities have been implemented. A review of this literature base will help identify the topics that have been explored and identify topics for further research. This research project collects, synthesizes, and analyzes both the research strategies (i.e., methodologies) and content (e.g., topics ...
Here are a few suggestions for MBA students looking for research topics in finance: Risk Management Strategies in Financial Institutions. Behavioral Finance in Investment Decision-Making. Financial Inclusion and Economic Development. Comparative Analysis of IFRS Adoption and Financial Reporting Quality.
Financial fraud, considered as deceptive tactics for gaining financial benefits, has recently become a widespread menace in companies and organizations. Conventional techniques such as manual verifications and inspections are imprecise, costly, and time consuming for identifying such fraudulent activities. With the advent of artificial intelligence, machine-learning-based approaches can be ...
This paper analyzes the effects of Environmental, Social, and Governance (ESG) performance on corporate financial performance (CFP), enriching the research on the intrinsic mechanism between ESG and financial performance in developing countries. This study uses a data sample of A-share listed companies in Shanghai and Shenzhen, China from 2009 to 2021, and adopts a two-way fixed effects model ...
The financial community has been boosted by the recent introduction of DL models for financial prediction and their accompanying publications. The success of DL over ML models is the major attractive point for finance researchers. With more financial time series data and different deep architectures, new DL methods will be proposed.
Based on a Fairlie decomposition method, this paper analyzes the drivers of gender gaps in financial inclusion in Cameroon. We use Finscope 2017 data for Cameroon and assess six distinct financial inclusion variables grouped into two dimensions mainly, access to and use of financial products and services.
This paper provides a comprehensive overview of intelligent financial fraud detection practices. We analyze the new features of fraud risk caused by the pandemic and review the development of data types used in fraud detection practices from quantitative tabular data to various unstructured data. The evolution of methods in financial fraud ...