
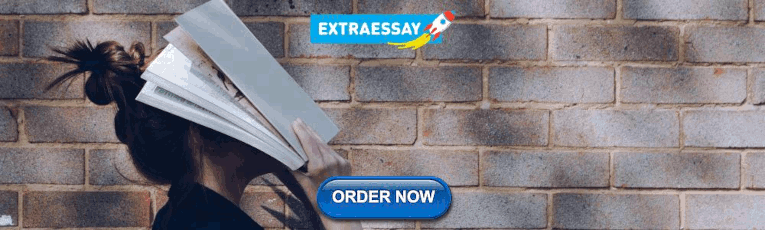
Quantitative and Qualitative Research
- I NEED TO . . .
What is Quantitative Research?
- What is Qualitative Research?
- Quantitative vs Qualitative
- Step 1: Accessing CINAHL
- Step 2: Create a Keyword Search
- Step 3: Create a Subject Heading Search
- Step 4: Repeat Steps 1-3 for Second Concept
- Step 5: Repeat Steps 1-3 for Quantitative Terms
- Step 6: Combining All Searches
- Step 7: Adding Limiters
- Step 8: Save Your Search!
- What Kind of Article is This?
- More Research Help This link opens in a new window
Quantitative methodology is the dominant research framework in the social sciences. It refers to a set of strategies, techniques and assumptions used to study psychological, social and economic processes through the exploration of numeric patterns . Quantitative research gathers a range of numeric data. Some of the numeric data is intrinsically quantitative (e.g. personal income), while in other cases the numeric structure is imposed (e.g. ‘On a scale from 1 to 10, how depressed did you feel last week?’). The collection of quantitative information allows researchers to conduct simple to extremely sophisticated statistical analyses that aggregate the data (e.g. averages, percentages), show relationships among the data (e.g. ‘Students with lower grade point averages tend to score lower on a depression scale’) or compare across aggregated data (e.g. the USA has a higher gross domestic product than Spain). Quantitative research includes methodologies such as questionnaires, structured observations or experiments and stands in contrast to qualitative research. Qualitative research involves the collection and analysis of narratives and/or open-ended observations through methodologies such as interviews, focus groups or ethnographies.
Coghlan, D., Brydon-Miller, M. (2014). The SAGE encyclopedia of action research (Vols. 1-2). London, : SAGE Publications Ltd doi: 10.4135/9781446294406
What is the purpose of quantitative research?
The purpose of quantitative research is to generate knowledge and create understanding about the social world. Quantitative research is used by social scientists, including communication researchers, to observe phenomena or occurrences affecting individuals. Social scientists are concerned with the study of people. Quantitative research is a way to learn about a particular group of people, known as a sample population. Using scientific inquiry, quantitative research relies on data that are observed or measured to examine questions about the sample population.
Allen, M. (2017). The SAGE encyclopedia of communication research methods (Vols. 1-4). Thousand Oaks, CA: SAGE Publications, Inc doi: 10.4135/9781483381411
How do I know if the study is a quantitative design? What type of quantitative study is it?
Quantitative Research Designs: Descriptive non-experimental, Quasi-experimental or Experimental?
Studies do not always explicitly state what kind of research design is being used. You will need to know how to decipher which design type is used. The following video will help you determine the quantitative design type.
- << Previous: I NEED TO . . .
- Next: What is Qualitative Research? >>
- Last Updated: Oct 23, 2024 11:33 AM
- URL: https://libguides.uta.edu/quantitative_and_qualitative_research
University of Texas Arlington Libraries 702 Planetarium Place · Arlington, TX 76019 · 817-272-3000
- Internet Privacy
- Accessibility
- Problems with a guide? Contact Us.
Educational resources and simple solutions for your research journey
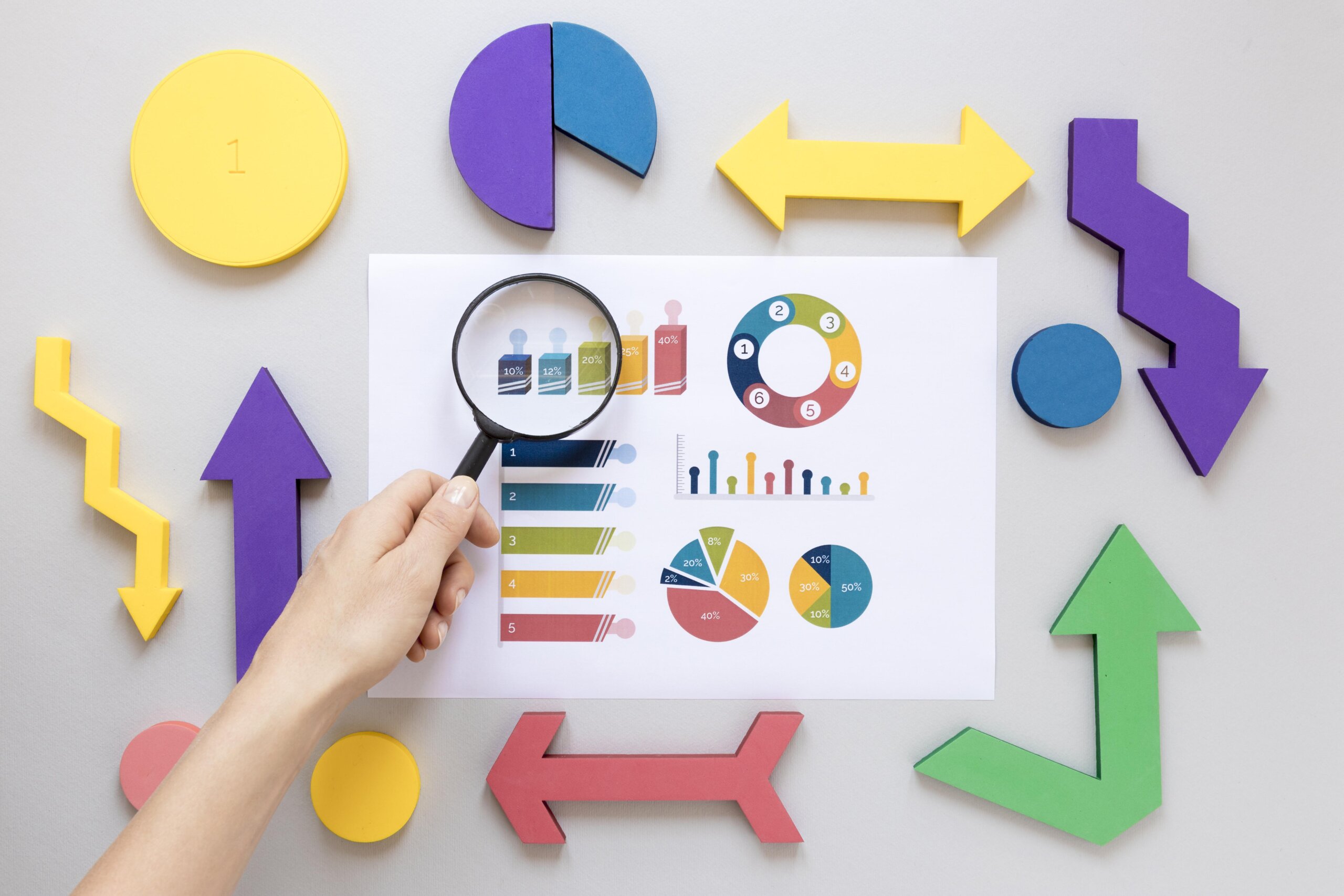
What is Quantitative Research? Definition, Methods, Types, and Examples

If you’re wondering what is quantitative research and whether this methodology works for your research study, you’re not alone. If you want a simple quantitative research definition , then it’s enough to say that this is a method undertaken by researchers based on their study requirements. However, to select the most appropriate research for their study type, researchers should know all the methods available.
Selecting the right research method depends on a few important criteria, such as the research question, study type, time, costs, data availability, and availability of respondents. There are two main types of research methods— quantitative research and qualitative research. The purpose of quantitative research is to validate or test a theory or hypothesis and that of qualitative research is to understand a subject or event or identify reasons for observed patterns.
Quantitative research methods are used to observe events that affect a particular group of individuals, which is the sample population. In this type of research, diverse numerical data are collected through various methods and then statistically analyzed to aggregate the data, compare them, or show relationships among the data. Quantitative research methods broadly include questionnaires, structured observations, and experiments.
Here are two quantitative research examples:
- Satisfaction surveys sent out by a company regarding their revamped customer service initiatives. Customers are asked to rate their experience on a rating scale of 1 (poor) to 5 (excellent).
- A school has introduced a new after-school program for children, and a few months after commencement, the school sends out feedback questionnaires to the parents of the enrolled children. Such questionnaires usually include close-ended questions that require either definite answers or a Yes/No option. This helps in a quick, overall assessment of the program’s outreach and success.

Table of Contents
What is quantitative research ? 1,2

The steps shown in the figure can be grouped into the following broad steps:
- Theory : Define the problem area or area of interest and create a research question.
- Hypothesis : Develop a hypothesis based on the research question. This hypothesis will be tested in the remaining steps.
- Research design : In this step, the most appropriate quantitative research design will be selected, including deciding on the sample size, selecting respondents, identifying research sites, if any, etc.
- Data collection : This process could be extensive based on your research objective and sample size.
- Data analysis : Statistical analysis is used to analyze the data collected. The results from the analysis help in either supporting or rejecting your hypothesis.
- Present results : Based on the data analysis, conclusions are drawn, and results are presented as accurately as possible.
Quantitative research characteristics 4
- Large sample size : This ensures reliability because this sample represents the target population or market. Due to the large sample size, the outcomes can be generalized to the entire population as well, making this one of the important characteristics of quantitative research .
- Structured data and measurable variables: The data are numeric and can be analyzed easily. Quantitative research involves the use of measurable variables such as age, salary range, highest education, etc.
- Easy-to-use data collection methods : The methods include experiments, controlled observations, and questionnaires and surveys with a rating scale or close-ended questions, which require simple and to-the-point answers; are not bound by geographical regions; and are easy to administer.
- Data analysis : Structured and accurate statistical analysis methods using software applications such as Excel, SPSS, R. The analysis is fast, accurate, and less effort intensive.
- Reliable : The respondents answer close-ended questions, their responses are direct without ambiguity and yield numeric outcomes, which are therefore highly reliable.
- Reusable outcomes : This is one of the key characteristics – outcomes of one research can be used and replicated in other research as well and is not exclusive to only one study.
Quantitative research methods 5
Quantitative research methods are classified into two types—primary and secondary.
Primary quantitative research method:
In this type of quantitative research , data are directly collected by the researchers using the following methods.
– Survey research : Surveys are the easiest and most commonly used quantitative research method . They are of two types— cross-sectional and longitudinal.
->Cross-sectional surveys are specifically conducted on a target population for a specified period, that is, these surveys have a specific starting and ending time and researchers study the events during this period to arrive at conclusions. The main purpose of these surveys is to describe and assess the characteristics of a population. There is one independent variable in this study, which is a common factor applicable to all participants in the population, for example, living in a specific city, diagnosed with a specific disease, of a certain age group, etc. An example of a cross-sectional survey is a study to understand why individuals residing in houses built before 1979 in the US are more susceptible to lead contamination.
->Longitudinal surveys are conducted at different time durations. These surveys involve observing the interactions among different variables in the target population, exposing them to various causal factors, and understanding their effects across a longer period. These studies are helpful to analyze a problem in the long term. An example of a longitudinal study is the study of the relationship between smoking and lung cancer over a long period.
– Descriptive research : Explains the current status of an identified and measurable variable. Unlike other types of quantitative research , a hypothesis is not needed at the beginning of the study and can be developed even after data collection. This type of quantitative research describes the characteristics of a problem and answers the what, when, where of a problem. However, it doesn’t answer the why of the problem and doesn’t explore cause-and-effect relationships between variables. Data from this research could be used as preliminary data for another study. Example: A researcher undertakes a study to examine the growth strategy of a company. This sample data can be used by other companies to determine their own growth strategy.

– Correlational research : This quantitative research method is used to establish a relationship between two variables using statistical analysis and analyze how one affects the other. The research is non-experimental because the researcher doesn’t control or manipulate any of the variables. At least two separate sample groups are needed for this research. Example: Researchers studying a correlation between regular exercise and diabetes.
– Causal-comparative research : This type of quantitative research examines the cause-effect relationships in retrospect between a dependent and independent variable and determines the causes of the already existing differences between groups of people. This is not a true experiment because it doesn’t assign participants to groups randomly. Example: To study the wage differences between men and women in the same role. For this, already existing wage information is analyzed to understand the relationship.
– Experimental research : This quantitative research method uses true experiments or scientific methods for determining a cause-effect relation between variables. It involves testing a hypothesis through experiments, in which one or more independent variables are manipulated and then their effect on dependent variables are studied. Example: A researcher studies the importance of a drug in treating a disease by administering the drug in few patients and not administering in a few.
The following data collection methods are commonly used in primary quantitative research :
- Sampling : The most common type is probability sampling, in which a sample is chosen from a larger population using some form of random selection, that is, every member of the population has an equal chance of being selected. The different types of probability sampling are—simple random, systematic, stratified, and cluster sampling.
- Interviews : These are commonly telephonic or face-to-face.
- Observations : Structured observations are most commonly used in quantitative research . In this method, researchers make observations about specific behaviors of individuals in a structured setting.
- Document review : Reviewing existing research or documents to collect evidence for supporting the quantitative research .
- Surveys and questionnaires : Surveys can be administered both online and offline depending on the requirement and sample size.
The data collected can be analyzed in several ways in quantitative research , as listed below:
- Cross-tabulation —Uses a tabular format to draw inferences among collected data
- MaxDiff analysis —Gauges the preferences of the respondents
- TURF analysis —Total Unduplicated Reach and Frequency Analysis; helps in determining the market strategy for a business
- Gap analysis —Identify gaps in attaining the desired results
- SWOT analysis —Helps identify strengths, weaknesses, opportunities, and threats of a product, service, or organization
- Text analysis —Used for interpreting unstructured data
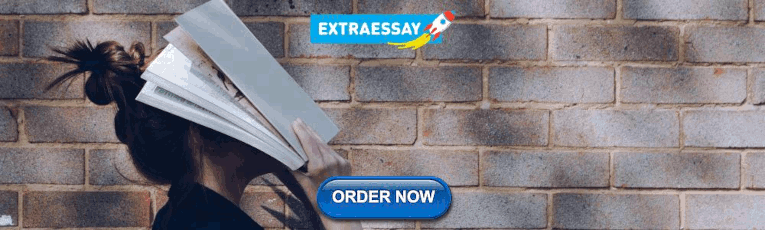
Secondary quantitative research methods :
This method involves conducting research using already existing or secondary data. This method is less effort intensive and requires lesser time. However, researchers should verify the authenticity and recency of the sources being used and ensure their accuracy.
The main sources of secondary data are:
- The Internet
- Government and non-government sources
- Public libraries
- Educational institutions
- Commercial information sources such as newspapers, journals, radio, TV
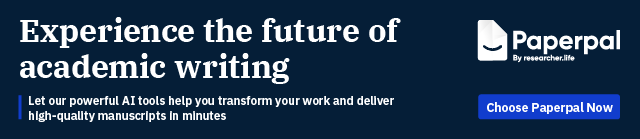
When to use quantitative research 6
Here are some simple ways to decide when to use quantitative research . Use quantitative research to:
- recommend a final course of action
- find whether a consensus exists regarding a particular subject
- generalize results to a larger population
- determine a cause-and-effect relationship between variables
- describe characteristics of specific groups of people
- test hypotheses and examine specific relationships
- identify and establish size of market segments
A research case study to understand when to use quantitative research 7
Context: A study was undertaken to evaluate a major innovation in a hospital’s design, in terms of workforce implications and impact on patient and staff experiences of all single-room hospital accommodations. The researchers undertook a mixed methods approach to answer their research questions. Here, we focus on the quantitative research aspect.
Research questions : What are the advantages and disadvantages for the staff as a result of the hospital’s move to the new design with all single-room accommodations? Did the move affect staff experience and well-being and improve their ability to deliver high-quality care?
Method: The researchers obtained quantitative data from three sources:
- Staff activity (task time distribution): Each staff member was shadowed by a researcher who observed each task undertaken by the staff, and logged the time spent on each activity.
- Staff travel distances : The staff were requested to wear pedometers, which recorded the distances covered.
- Staff experience surveys : Staff were surveyed before and after the move to the new hospital design.
Results of quantitative research : The following observations were made based on quantitative data analysis:
- The move to the new design did not result in a significant change in the proportion of time spent on different activities.
- Staff activity events observed per session were higher after the move, and direct care and professional communication events per hour decreased significantly, suggesting fewer interruptions and less fragmented care.
- A significant increase in medication tasks among the recorded events suggests that medication administration was integrated into patient care activities.
- Travel distances increased for all staff, with highest increases for staff in the older people’s ward and surgical wards.
- Ratings for staff toilet facilities, locker facilities, and space at staff bases were higher but those for social interaction and natural light were lower.
Advantages of quantitative research 1,2
When choosing the right research methodology, also consider the advantages of quantitative research and how it can impact your study.
- Quantitative research methods are more scientific and rational. They use quantifiable data leading to objectivity in the results and avoid any chances of ambiguity.
- This type of research uses numeric data so analysis is relatively easier .
- In most cases, a hypothesis is already developed and quantitative research helps in testing and validatin g these constructed theories based on which researchers can make an informed decision about accepting or rejecting their theory.
- The use of statistical analysis software ensures quick analysis of large volumes of data and is less effort intensive.
- Higher levels of control can be applied to the research so the chances of bias can be reduced.
- Quantitative research is based on measured value s, facts, and verifiable information so it can be easily checked or replicated by other researchers leading to continuity in scientific research.
Disadvantages of quantitative research 1,2
Quantitative research may also be limiting; take a look at the disadvantages of quantitative research.
- Experiments are conducted in controlled settings instead of natural settings and it is possible for researchers to either intentionally or unintentionally manipulate the experiment settings to suit the results they desire.
- Participants must necessarily give objective answers (either one- or two-word, or yes or no answers) and the reasons for their selection or the context are not considered.
- Inadequate knowledge of statistical analysis methods may affect the results and their interpretation.
- Although statistical analysis indicates the trends or patterns among variables, the reasons for these observed patterns cannot be interpreted and the research may not give a complete picture.
- Large sample sizes are needed for more accurate and generalizable analysis .
- Quantitative research cannot be used to address complex issues.
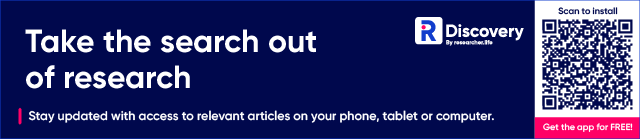
Frequently asked questions on quantitative research
Q: What is the difference between quantitative research and qualitative research? 1
A: The following table lists the key differences between quantitative research and qualitative research, some of which may have been mentioned earlier in the article.
Q: What is the difference between reliability and validity? 8,9
A: The term reliability refers to the consistency of a research study. For instance, if a food-measuring weighing scale gives different readings every time the same quantity of food is measured then that weighing scale is not reliable. If the findings in a research study are consistent every time a measurement is made, then the study is considered reliable. However, it is usually unlikely to obtain the exact same results every time because some contributing variables may change. In such cases, a correlation coefficient is used to assess the degree of reliability. A strong positive correlation between the results indicates reliability.
Validity can be defined as the degree to which a tool actually measures what it claims to measure. It helps confirm the credibility of your research and suggests that the results may be generalizable. In other words, it measures the accuracy of the research.
The following table gives the key differences between reliability and validity.
Q: What is mixed methods research? 10
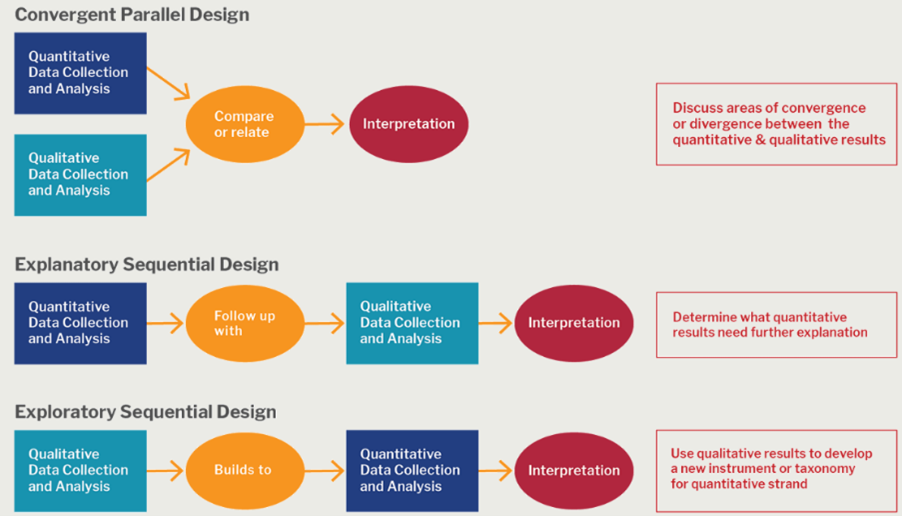
A: A mixed methods approach combines the characteristics of both quantitative research and qualitative research in the same study. This method allows researchers to validate their findings, verify if the results observed using both methods are complementary, and explain any unexpected results obtained from one method by using the other method. A mixed methods research design is useful in case of research questions that cannot be answered by either quantitative research or qualitative research alone. However, this method could be more effort- and cost-intensive because of the requirement of more resources. The figure 3 shows some basic mixed methods research designs that could be used.
Thus, quantitative research is the appropriate method for testing your hypotheses and can be used either alone or in combination with qualitative research per your study requirements. We hope this article has provided an insight into the various facets of quantitative research , including its different characteristics, advantages, and disadvantages, and a few tips to quickly understand when to use this research method.
References
- Qualitative vs quantitative research: Differences, examples, & methods. Simply Psychology. Accessed Feb 28, 2023. https://simplypsychology.org/qualitative-quantitative.html#Quantitative-Research
- Your ultimate guide to quantitative research. Qualtrics. Accessed February 28, 2023. https://www.qualtrics.com/uk/experience-management/research/quantitative-research/
- The steps of quantitative research. Revise Sociology. Accessed March 1, 2023. https://revisesociology.com/2017/11/26/the-steps-of-quantitative-research/
- What are the characteristics of quantitative research? Marketing91. Accessed March 1, 2023. https://www.marketing91.com/characteristics-of-quantitative-research/
- Quantitative research: Types, characteristics, methods, & examples. ProProfs Survey Maker. Accessed February 28, 2023. https://www.proprofssurvey.com/blog/quantitative-research/#Characteristics_of_Quantitative_Research
- Qualitative research isn’t as scientific as quantitative methods. Kmusial blog. Accessed March 5, 2023. https://kmusial.wordpress.com/2011/11/25/qualitative-research-isnt-as-scientific-as-quantitative-methods/
- Maben J, Griffiths P, Penfold C, et al. Evaluating a major innovation in hospital design: workforce implications and impact on patient and staff experiences of all single room hospital accommodation. Southampton (UK): NIHR Journals Library; 2015 Feb. (Health Services and Delivery Research, No. 3.3.) Chapter 5, Case study quantitative data findings. Accessed March 6, 2023. https://www.ncbi.nlm.nih.gov/books/NBK274429/
- McLeod, S. A. (2007). What is reliability? Simply Psychology. www.simplypsychology.org/reliability.html
- Reliability vs validity: Differences & examples. Accessed March 5, 2023. https://statisticsbyjim.com/basics/reliability-vs-validity/
- Mixed methods research. Community Engagement Program. Harvard Catalyst. Accessed February 28, 2023. https://catalyst.harvard.edu/community-engagement/mmr
Editage All Access is a subscription-based platform that unifies the best AI tools and services designed to speed up, simplify, and streamline every step of a researcher’s journey. The Editage All Access Pack is a one-of-a-kind subscription that unlocks full access to an AI writing assistant, literature recommender, journal finder, scientific illustration tool, and exclusive discounts on professional publication services from Editage.
Based on 22+ years of experience in academia, Editage All Access empowers researchers to put their best research forward and move closer to success. Explore our top AI Tools pack, AI Tools + Publication Services pack, or Build Your Own Plan. Find everything a researcher needs to succeed, all in one place – Get All Access now starting at just $14 a month !
Related Posts
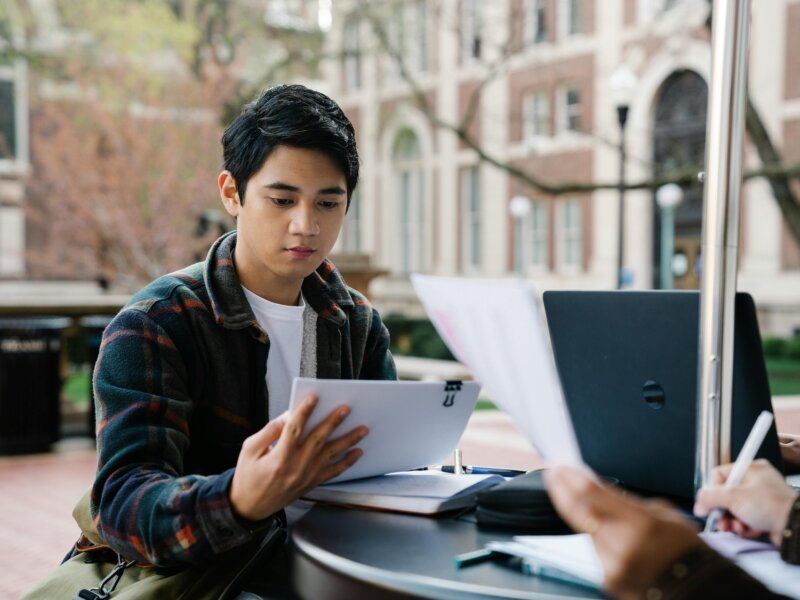
What is a good h-index?

What is Correlational Research: Definition, Types, and Examples
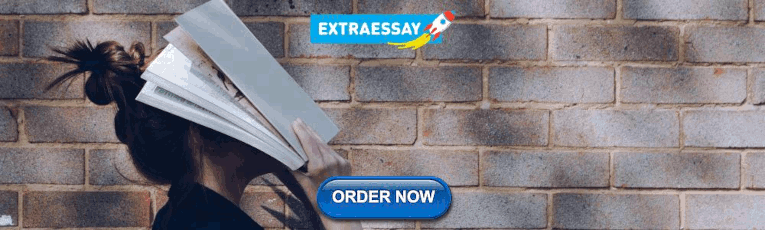
IMAGES
VIDEO