Advertisement
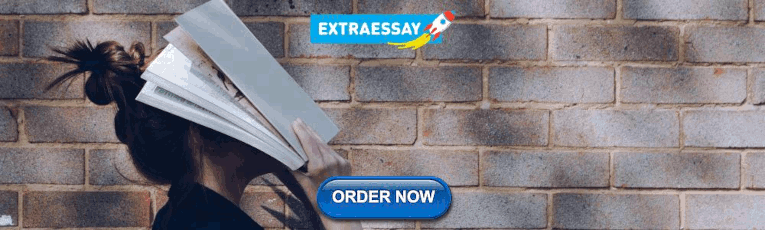
Data-Driven Requirements Elicitation: A Systematic Literature Review
- Review Article
- Open access
- Published: 04 January 2021
- Volume 2 , article number 16 , ( 2021 )
Cite this article
You have full access to this open access article
- Sachiko Lim 1 ,
- Aron Henriksson 1 &
- Jelena Zdravkovic 1
16k Accesses
44 Citations
Explore all metrics
Requirements engineering has traditionally been stakeholder-driven. In addition to domain knowledge, widespread digitalization has led to the generation of vast amounts of data (Big Data) from heterogeneous digital sources such as the Internet of Things (IoT), mobile devices, and social networks. The digital transformation has spawned new opportunities to consider such data as potentially valuable sources of requirements, although they are not intentionally created for requirements elicitation. A challenge to data-driven requirements engineering concerns the lack of methods to facilitate seamless and autonomous requirements elicitation from such dynamic and unintended digital sources. There are numerous challenges in processing the data effectively to be fully exploited in organizations. This article, thus, reviews the current state-of-the-art approaches to data-driven requirements elicitation from dynamic data sources and identifies research gaps. We obtained 1848 hits when searching six electronic databases. Through a two-level screening and a complementary forward and backward reference search, 68 papers were selected for final analysis. The results reveal that the existing automated requirements elicitation primarily focuses on utilizing human-sourced data, especially online reviews, as requirements sources, and supervised machine learning for data processing. The outcomes of automated requirements elicitation often result in mere identification and classification of requirements-related information or identification of features, without eliciting requirements in a ready-to-use form. This article highlights the need for developing methods to leverage process-mediated and machine-generated data for requirements elicitation and addressing the issues related to variety, velocity, and volume of Big Data for the efficient and effective software development and evolution.
Similar content being viewed by others
Data-Driven Requirements Engineering: A Guided Tour
Towards Integrating Data-Driven Requirements Engineering into the Software Development Process: A Vision Paper
Data-Driven Requirements Engineering. The SUPERSEDE Way
Avoid common mistakes on your manuscript.
Introduction
Requirements elicitation is one of the most critical activities in requirements engineering, which, in turn, is a major determinant of successful development of information systems [ 1 ]. In conventional requirements engineering, requirements are elicited from domain knowledge obtained from stakeholders, relying primarily on qualitative data collection methods (e.g., interviews, workshops, and focus group discussions) [ 2 ]. The ongoing digitalization of organizations and society at large—as seen, for instance, by the proliferation of e-commerce and the advent of IoT—has led to an unprecedented and increasing amount of high-velocity and heterogeneous data, which is often referred to as Big Data [ 3 ].
The digital transformation has spawned new opportunities to consider this type of dynamic data from digital sources as potentially valuable sources of requirements, in addition to domain knowledge. Harnessing both traditional and new data sources in a complementary fashion may help improve the quality of existing or facilitate the development of new software systems. Nevertheless, conventional elicitation techniques are often time-consuming and not sufficiently scalable for processing such fast-growing data or capable of considering stakeholder groups that are becoming increasingly large and global. This highlights the need for a data-driven approach to support continuous and automated requirements engineering from ever-growing amounts of data.
There have been numerous efforts to automate requirements elicitation from static data, i.e., data that are generated with a relatively low velocity and rarely updated. These efforts can be grouped according to the following three aims: (1) eliciting requirements from static domain knowledge (e.g., documents written in natural languages [ 4 , 5 ], ontologies [ 6 , 7 ], and various types of models, e.g., business process models [ 8 ], UML use cases and sequence diagrams [ 9 ]), (2) performing specific requirements engineering activities based on requirements that have been already elicited (e.g., requirements prioritization [ 10 ], classification of natural language requirements [ 11 ], management of requirements traceability [ 12 ], requirements validation [ 13 ], generation of a conceptual model from natural language requirements [ 14 ]), or (3) developing tools to enhance stakeholders’ ability to perform requirements engineering activities based on static domain knowledge or existing requirements (e.g., tool-support for collaborative requirements prioritization [ 15 ] and requirements negotiation with rule-based reasoning [ 16 ]).
Several systematic reviews have been conducted on automated requirements elicitation from static domain knowledge. Meth et al. conducted a systematic review on tool support for automated requirements elicitation from domain documents written in natural language, where they analyzed and categorized the identified studies according to an analytical framework which consists of tool categories, technological concepts, and evaluation approaches [ 17 ]. Nicolás and Toval conducted a systematic review of the methods and techniques for transforming domain models (e.g., business models, UML models, and user interface models), use cases, scenarios, and user stories into textual requirements [ 18 ]. In both of these reviews, the requirements sources contained static domain knowledge.
Much less focus has been placed on eliciting requirements from dynamic data, and data that were not intentionally collected for the purpose of requirements elicitation. There are four main advantages to focus on dynamic data from such “unintended” digital sources. First, dynamic data-driven requirements engineering facilitates secondary use of data, which eliminates the need for collecting data specifically for requirements engineering, in turn enhancing scalability. Second, unintended digital sources can include data relevant for new system requirements that otherwise would not be discovered since utilizing such data sources allows for the collection of data from larger and global stakeholders who are beyond the reach of an organization relying on traditional elicitation methods [ 19 ]. Including such requirements, which a current software system is not supporting, can bring business values in the form of improved customer satisfaction, cost and time reduction, and optimized operations [ 20 ]. Third, focusing on dynamic data allows for capturing up-to-date user requirements, which in turn enables timely and effective operational decision making. Finally, dynamic data from unintended digital sources are machine-readable, which facilitates automated and continuous requirements engineering. A fitting requirements elicitation approach provides new opportunities and competitive advantages in a fast-growing market by extracting real-time business insights and knowledge from a variety of digital sources.
Crowd-based requirements engineering (CrowdRE) is a good example that has taken advantage of dynamic data from unintended digital sources. A primary focus of CrowdRE has been on eliciting requirements from explicit user feedback from crowd users (e.g., app reviews and data from social media) by applying various techniques based on machine learning and natural language processing [ 21 ]. Genc-Nayebi and Abran conducted a systematic review on opinion mining from mobile app store user reviews to identify existing solutions and challenges for mining app reviews, as well as to propose future research directions [ 22 ]. They focused on specific data-mining techniques used for review analysis, domain adaptation methods, evaluation criteria to assess the usefulness and helpfulness of the reviews, techniques for filtering out spam reviews, and application features. Martin et al. [ 26 ] surveyed on studies that performed app store analysis to extract both technical and non-technical attributes for software engineering. Tavakoli et al. [ 27 ] conducted a systematic review on techniques and tools for extracting useful software development information through mobile app review mining. The aforementioned literature reviews only focus on utilizing app reviews, while leaving out other types of human-sourced data that are potentially useful as requirement sources. There is also a growing interest in embracing contextual and usage data of crowd users (i.e., implicit user feedback) for requirements elicitation. This systematic review, thus, broadens the scope of previous literature reviews by considering more diverse data sources than merely app reviews for requirements elicitation.
Another relevant approach to data-driven requirements engineering is the application of process mining capabilities for requirements engineering. Process mining is an evidence-based approach to infer valuable process-related insights primarily from event logs, discovered models, and pre-defined process models. Process mining can be divided into three types: process discovery, conformance checking, and process enhancement [ 23 ]. Ghasemi and Amyot performed a systematic review on goal-oriented process modeling in which the selected studies were categorized into three areas: (1) goal modeling and requirements elicitation, (2) intention mining (i.e., the discovery of intentional process models going beyond mere activity process models), and (3) key performance indicators (i.e., means for monitoring goals) [ 23 ]. Their findings indicate that the amount of research on goal-oriented process mining is still limited. In addition to explicit and implicit user feedback, as well as event logs and process models, there may be more opportunities to leverage a broader range of dynamic data sources for requirements engineering, such as sensor readings.
Zowghi and Coulin [ 24 ] performed a comprehensive survey on techniques, approaches, and tools used for requirements elicitation. However, their work exclusively focused on conventional, stakeholder-driven requirements elicitation methods. Our study instead investigated the data-driven requirements elicitation. More recently, Arruda and Madhavji [ 25 ] systematically reviewed the literature on requirements engineering to develop Big Data applications. They identified the process and type of requirements needed for developing Big Data applications, identified challenges associated with requirements engineering in the context of Big Data applications, discussed the available requirements engineering solutions for the development of Big Data applications, and proposed future research directions. This study is different from their work because we studied methods to elicit requirements from Big Data rather than eliciting requirements for Big Data applications.
To our knowledge, no systematic review has been performed with an explicit focus on automated requirements elicitation for information systems from three types of dynamic data sources: human-sourced data sources, process-mediated data sources, and machine-generated data sources. The aim of this study is, therefore, to perform a comprehensive and systematic review of the research literature on existing state-of-the-art methods for facilitating automatic requirements elicitation for information systems driven by dynamic data from unintended digital sources.
This review may help requirements engineers and researchers understand the existing data-driven requirements elicitation techniques and gaps need to be addressed to facilitate data-driven requirements elicitation. Those insights may provide a basis for further development of algorithms and methods to leverage the increasing availability of Big Data as requirements sources.
Definitions and Scope
In this study, dynamic data are defined as raw data available in a digital form that changes frequently and have not already been analyzed or aggregated . Dynamic data certainly include but are not limited to Big Data, which in itself is challenging to define [ 28 ]. In addition to Big Data, dynamic data also include data that does not strictly meet the 4 Vs of Big Data (i.e., Volume, Variety, Veracity, and Velocity) but are still likely to contain relevant requirements-related information. Domain knowledge includes, for example, intellectual property, business documents, existing system specifications, goals, standards, conferences, and knowledge from customers or external providers.
This study excludes static domain knowledge that is less frequently created or modified and has been the primary focus of existing automated requirements engineering. Unintended digital sources are defined as sources of data generated via digital technologies that are unintended with respect to requirements elicitation. Thus, dynamic data from unintended digital sources are the digital data pulled from data sources that are created/modified frequently without the intention of eliciting requirements.
Of note is that the two terms “dynamic data” and “unintended digital source” together define the scope of this systematic review. For example, although domain documents are often created without the intention of performing requirements engineering, they are not considered to be dynamic data and, therefore, outside of the scope of this study.
Dynamic data from unintended digital sources expand explicit and implicit user feedback, defined by Morales-Ramirez et al. [ 29 ]. In their study, user feedback is considered as “a reaction of users, which roots in their perceived quality of experience”, which indicates the existence of a specific user is assumed. However, there are many devices which collect Big Data such as environmental IoT sensors to measure temperature, humidity, and pollution level, without interacting users. Since we foresee the possibility of eliciting requirements from such data sources, we decided to use a different term from the term “implicit user feedback”. To categorize the sources of data, we used human-sourced, process-mediated, and machine-generated data, following Firmani et al. [ 30 ].
Research Questions
To achieve the aim of the study, we formulated the main research question as follows: how can requirements elicitation from dynamic data be supported through automation? The main research question has been further divided into the following sub-research questions:
RQ1: What types of dynamic data are used for automated requirements elicitation?
We focus on describing the sources of the data, but also study whether there have been attempts to integrate multiple types of data sources and whether domain knowledge has been used in addition to dynamic data.
RQ2: What types of techniques and technologies are used for automating requirements elicitation?
We are interested in learning which underlying techniques and technologies are used in the proposed methods, as well as how they are put together and evaluated.
RQ3: What are the outcomes of automated requirements elicitation?
We assess how far the proposed methods go in automating requirements elicitation, the form of the outputs generated by the data-driven elicitation method, and what types of requirements are elicited.
This systematic review will advance scientific knowledge on data-driven requirements engineering for continuous system development and evolution by (1) providing a holistic analysis of the state-of-the-art methods that support automatic requirements elicitation from dynamic data, (2) identifying associated research gaps, and (3) providing directions for future research. The paper is structured as follows: the second section presents the research methods used in our study; the third section presents an overview of the selected studies and the results based on our analytical framework; the fourths section provides a detailed analysis and discussion of each component of the analytical framework; the fifth section describes potential threats to validity; finally, the last section concludes the paper and suggests directions for future work.
A systematic literature review aims to answer a specific research question using systematic methods to consolidate all relevant evidence that meets pre-defined eligibility criteria [ 3 ]. It consists of three main phases: planning, conducting, and reporting the review. The main activities of the planning phase are problem formulation and protocol development. Before the actual review process started, we formulated research questions. The study protocol was then developed, conforming to the guideline of the systematic literature review proposed by Kitchenham and Charters [ 31 ]. The protocol included the following contents: background, the aim of the study, research questions, selection criteria, data sources (i.e., electronic databases), search strategy, data collection, data synthesis, and the timeline of the study. The protocol was approved by the research group, which consists of the first author and two research experts: one expert in requirements engineering and one expert in data science. The actual review process starts during the conducting phase. The phase includes the following activities: identifying potentially eligible studies based on title, abstract and keywords, selecting eligible studies through full-text screening, extracting and synthesizing data that are relevant to answer the defined research question(s), performing a holistic analysis, and interpreting the findings. During the reporting phase, the synthesized findings are documented and disseminated to an appropriate channel.
Selection Criteria
Inclusion and exclusion criteria were developed to capture the most relevant articles for answering our research questions.
Inclusion Criteria
We included articles that met all the following inclusion criteria:
Requirements elicitation is supported through automation.
Requirements are elicited from digital and dynamic data sources.
Digital and dynamic data sources are created without intention with respect to requirements engineering.
Changes in requirements should involve the elicitation of new requirements.
The article has been peer-reviewed.
The full text of the article is written in English.
Exclusion Criteria
We excluded articles that met at least one of the following exclusion criteria:
Requirements are elicited solely from non-dynamic data.
The proposed method is performed based on existing requirements.
Studies that merely presented the proposed artifact without any or sufficient descriptions of evaluation methods.
Review papers, keynote talks, or abstracts of conference proceedings.
Data Sources
We performed a comprehensive search in six electronic databases (Table 1 ). In the first iteration, we searched Scopus, Web of Science, ACM Digital Library, and IEEE Xplore. Those databases were selected because they together cover the top ten information systems journals and conferences [ 17 ]. In addition, EBSCOhost and ProQuest, which are two major databases in the field of information systems, were searched to maximize the coverage of relevant publications, in line with a previous systematic review in the area [ 17 ]. ProQuest and EBSCOhost include both peer-reviewed and non-peer-reviewed articles. We, however, considered only peer-reviewed articles to be consistent with our inclusion criteria. The differences in the search field across databases are due to the different search functionalities of each electronic database.
Search Strategy
A comprehensive search strategy was developed in consultation with a librarian and the two co-authors who are experts in the fields of requirements engineering and data science, respectively. First, we extracted three key components from the first research question: requirements elicitation, automation, and Big Data sources and related analytics (Table 2 ). These components formed the basis for creating a logical search string. Big Data can refer either to data sources or to analytics/data-driven techniques to process Big Data. The term is also closely related to data-mining/machine-learning/data science/artificial intelligence techniques. We thus included keywords and synonyms that cover both Big Data sources and related analytics.
To construct a search string, keywords and synonyms that were grouped in the same component were connected by OR-operators, while each key component was connected by AND-operators, which means at least one keyword from each component must be present. The search string was adapted using the specific syntax of each database’s search function. The search string was iteratively tested and refined to optimize search results through trial search.
Study Selection
The entire search was performed by the first author (SL). Before starting the review process, we tested a small number of articles to establish agreement and consistency among reviewers. We then conducted a pilot study in which three reviewers independently assessed 50 randomly selected papers to estimate the sample size that is needed to ensure a substantial level of agreement (i.e., 0.61–0.80) based on the Landis and Koch-Kappa’s benchmark scale [ 32 ]. Each paper was screened by assessing its title, abstract, and keywords against our selection criteria (level 1 screening). During level 1 screening, articles were classified into one of the three categories: (1) included, (2) excluded, or (3) uncertain. Studies that fell into category 1 and 3 proceeded to full-text screening (level 2 screening) since the aim of the level 1 screening was to identify potentially relevant articles or those that lack sufficient information to be excluded.
After each reviewer had assessed 50 publications, we computed the Fleiss’s Kappa to calculate the inter-rater reliability. We, however, did not discuss the results of each reviewer’s assessment. The Fleiss’s Kappa was used because there were more than two reviewers. The Fleiss’ Kappa was computed to be 0.786. Sample size estimation was performed, following a confidence interval approach suggested by Rotondi and Donner [ 33 ]. Using 0.786 as the point estimate of Kappa and 0.61 as the expected lower bound, the required minimum sample size was estimated to be 139. The value of 0.61 was used as the lower bound of Kappa because it is the lower limit of “substantial” inter-rater reliability based on the Landis and Koch-Kappa’s benchmark scale [ 32 ], which is what we had aimed for. Since we achieved a substantial level of agreement and did not discuss results not to influence each other’s decisions, each of three reviewers independently continued to screen the remainder of the 89 randomly chosen publications based on titles, abstracts, and keywords (level 1 screening). The overall Fleiss’ Kappa for reviewing 139 articles was 0.850, which indicates an “almost perfect” agreement, according to the benchmark scale proposed by Landis and Koch [ 32 ]. Since we were able to achieve a very high inter-rater reliability, the rest of the level 1 screening was conducted by a single reviewer (SL). However, all of the three reviewers discussed and reached a consensus on the articles which SL classified as uncertain or could not decide on with sufficient confidence.
Before conducting the level 2 screening, we discussed which information should be extracted from the eligible articles. Based on the discussion, we developed a preliminary analytical framework to standardize the information to be extracted. We tested this on a small number of full-text papers and refined the data extraction form accordingly. In the level 2 screening, at least two authors reviewed the full-text of each paper that has been identified in level 1 screening to assess its eligibility in the final analysis. In addition to keyword-based search on the databases, we also performed forward/backward reference searching of all the included studies. SL extracted data from all the eligible studies, while each of AH and JZ divided the data extraction task by half. This was done to ensure that data extracted by SL could be cross-checked by at least one of the two reviewers who have richer experiences and knowledge. Any disagreements between the two reviewers were referred to the third reviewer and resolved by consensus.
To update the search results, an additional search was performed on July 3, 2020, using the same search query and introducing the two-level screening process (i.e., keyword-based search followed by full-text screening). While filtering can be performed by specifying the publication date and year in some databases, in other databases, the search can only be filtered by the publican year. Thus, we manually excluded the studies that have been published before the date of the initial search. However, we did not perform a backward and forward reference search during the updating phase. We then applied the same selection criteria used for the initial search to identify the relevant studies.
Analytical Framework and Data Collection
After considering the selected articles, we iteratively developed and refined an analytical framework, which covers both design and evaluation perspectives, to answer our research questions. The framework consists of three components: types of dynamic data sources used for automated requirements elicitation, techniquesand technologies used for automated requirements elicitation, and the outcomes of automated requirements elicitation. Table 3 summarizes the extracted data that are associated with each component of the analytical framework. Each component of the analytical framework is described in detail below.
Types of Dynamic Data Sources Used for Automated Requirements Elicitation
To answer RQ1, we extracted the following information: (1) types of dynamic data sources, (2) types of dynamic data, (3) integration of data sources, (4) relation of dynamic data to a given organization, and (5) additional domain knowledge that is used to elicit system requirements.
Types of Dynamic Data Sources
Dynamic data sources are categorized into one or a combination of human-sourced data sources, process-mediated data sources, and machine-generated data sources [ 30 ]. This provides insights into which types of data sources have drawn the most or the least attention as potential requirements sources in the existing literature. The categorization also helps to analyze whether there exists any process pattern in the automated requirements elicitation within each data source type.
Human-sourced data sources refer to the digitized records of human experiences. To name a few, examples of human-sourced data sources include social media, blogs, and contents from mobile phones. Process-mediated data sources are records of business processes and business events that are monitored, which includes electronic health records, commercial transactions, banking records, credit card payments. Machine-generated data sources are the records of fixed and mobile sensors and machines that are used to measure the events and situations in the physical world. They include, for example, readings from environmental and barometric pressure sensors, outputs of medical devices, satellite image data, and location data such as RFID chip readings and GPS outputs.
Types of Dynamic Data
To understand what types of dynamic data have been used for eliciting system requirements in the existing literature, we extracted the specific types of dynamic data that were used in each of the selected studies and grouped them into seven categories. Those categories are online reviews (e.g., app reviews, expert reviews, and user reviews), micro-blogs (e.g., Twitter), online discussions/forums, software repositories (e.g., issue tracking systems and GitHub), usage data, sensor readings, and mailing lists.
Integration of Data Sources
We explored whether the study integrates multiple types of dynamic data sources (i.e., any combination of human-sourced, process-mediated, and machine-generated data sources). We classified the selected studies into “yes” if the study has used multiple dynamic data sources, otherwise into “no.”
Relation of Dynamic Data to a Given Organization
Understanding whether requirements are elicited from external or internal data sources in relation to a given organization is important for requirements engineers to identify potential sources that can bring innovations into the requirements engineering process and facilitate software evolution and development of new promising software systems. We thus classified the selected studies into “yes” if the platform is owned by the organization and “no” if it is owned by a third party.
Additional Domain Knowledge that was Used to Elicit System Requirements
We assessed whether the study uses any domain knowledge in combination with dynamic data to explore the possible ways of integrating both dynamic data and domain knowledge. The selected studies were classified into “yes,” if the study uses any domain knowledge in addition to dynamic data, otherwise classified into “no.”
Techniques Used for Automated Requirements Elicitation
To answer RQ2, the following four types of information were extracted: (1) technique(s) used for automated requirements elicitation, including process pattern of automating requirements elicitation, (2) use of aggregation/summarization, (3) use of visualization, and (4) evaluation methods.
Technique(s) Used for Automation
Implementing promising algorithms is a prerequisite for effective and efficient automation of the requirements elicitation process. To identify the state-of-the-art algorithms, specific methods that were used for automating requirements elicitation were extracted and categorized into machine learning, rule-based classification, model-oriented approach, topic modeling, and traditional clustering.
Aggregation/Summarization
Summarization helps navigate requirements engineers to pinpoint the relevant information efficiently out of the ever-growing amount of data. We thus assessed whether the study summarizes/aggregates requirements-related information to obtain high-level requirements. If summarization/aggregation is performed, we also extracted specific techniques used for summarization/aggregation.
Visualization
Visualization facilitates requirements engineers to interpret the results of data analysis efficiently and effectively as well as to gain (new) insights in data. We assessed whether the study visualizes the output of the study to enhance their interpretability. If visualization is provided, the specific method used for visualization was also extracted.
Evaluation Methods
To understand how rigorously the performance of the proposed artifact was evaluated, we extracted methods that were used to assess the artifact. Evaluation methods were further divided into two dimensions: evaluation approach and evaluation concepts and metrics [ 17 ]. The evaluation concept of each selected study was categorized into one of the following groups: controlled experiment, case study, proof of concept, and other concepts. In a controlled experiment, the proposed artifact is evaluated in a controlled environment [ 34 ]. A case study aims to assess the artifact in-depth in a real-world context [ 34 ]. A proof of concept is defined as a demonstration of the proposed artifact to verify its feasibility for a real-world application. Other concepts refer to studies using other approaches to evaluate their artifact that does not fall into any category of the aforementioned evaluation approach. We also extracted evaluation concepts and metrics used for the artifact evaluation. Evaluation concepts were classified into one or more of the following categories: completeness, correctness, efficiency, and other evaluation concepts.
The Outcomes of Automated Requirements Elicitation
To answer RQ3, we assessed the outcomes of automated requirements elicitation by extracting the following information: (1) types of requirements, (2) expression of the elicited requirements (i.e., in what form outputs that were generated by automated requirements elicitation were expressed), and (3) additional requirements engineering activity supported through automation.
Expression of the Elicited Requirements
To understand how the obtained requirements are expressed and how far the elicitation activity reached, outputs of automated requirements elicitation were extracted, which were grouped into the following categories: identification and classification of requirements-related information, identification of candidate features related to requirements, and elicitation of requirements.
Intended Degree of Automation
Based on the degree of the proposed automated method, the selected studies were classified into either full automation or semi-automation. We classified the study as full automation if the study fulfilled either of the following conditions: (1) the proposed artifact automated the entire requirements elicitation process without human interaction, or (2) the proposed artifact only supports the partial process of requirements elicitation; however, the part it addressed was fully automated. Semi-automation refers to having a human-in-the-loop for automating requirements elicitation, thus requirements are directed by human interactions.
Additional Requirements Engineering Activity Supported Through Automation
Understanding to what extent the entire requirements engineering process has already been automated is essential to clarify the direction of future research that aims at increasing the level of automation in performing the requirements engineering process. We thus extracted the requirements engineering activity that was supported through automation other than requirements elicitation, if any.
Quality Assessment
We simply assessed the quality of the selected studies based on CORE Conference Rankings for conferences, workshops, and symposia, and SCImago Journal Rank (SJR) indicators for journal papers. We assumed that a study with a higher score of CORE or SJR has higher quality than one with a lower score. The papers that have been ranked A*, A, B, or C for the CORE index get the point of 1.5, 1.5, 1, and 0.5, respectively. If a paper is ranked Q1 or Q2 for the SJR indicator, the paper receives 2 and 1.5, respectively, while a paper that is ranked Q3 or Q4 gets 1. If a conference/journal paper is not included in the CORE/SJR ranking, the paper scores 0 points.
Data Synthesis
We narratively synthesized the findings of this systematic review, which includes basic descriptive statistics and qualitative analyses of (semi-)automated elicitation methods that are sub-grouped by dynamic data source as well as identified research gap(s), and implications and recommendations for future research.
Figure 1 shows a flow diagram of the article selection. We obtained 1,848 hits when searching the 6 electronic databases. We removed 458 duplicates, leaving 1,390 articles for level 1 screening (Table 4 ). After level 1 screening, we identified 40 articles to proceed to level 2 screening. The level 2 screening resulted in the inclusion of 29 articles for data extraction. We excluded the remaining eleven papers due to: the study not using dynamic data for requirements elicitation; the study being based on existing requirements that had already been elicited; the study not automating requirements elicitation to any degree; and the study proposing a method for automated requirements elicitation without sufficient evaluation.
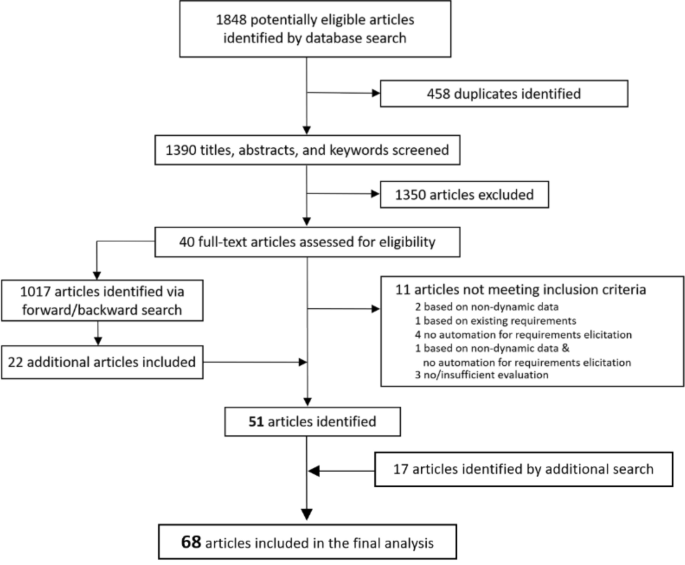
Flow diagram of article selection
In addition, a forward and backward reference search identified 1017 additional articles. Out of these, 22 articles met our inclusion criteria. Thus, a total of 51 papers were considered in the final analysis. Reasons for similar numbers of articles being identified in the query-based search and the backward/forward search include: the studies using terms such as “elicit requirements”, “requirements”, “requirements evolution” instead of “requirements elicitation”; using keywords which cover only one or two of the three keyword blocks despite being relevant; using only the name of a specific analytics technique (e.g., Long Short-term Memory) and not more general terms included in the identified keywords, e.g., machine learning.
To update the search results, we performed additional search and two-level screening, using the same search query process. The updated search identified 401 after removing duplicates (Table 4 ). Two-level screening resulted in including 17 additional studies. However, we did not perform a backward and forward reference search during this phase. We also included one study that was not captured by the search query but was recommended by an expert due to its relevance to our research question. We, therefore, selected a total 68 studies to be included in this review.
General Characteristics of the Selected Studies
Of the 68 selected articles, conference proceedings are the most frequent publication type ( n = 41), followed by journal articles ( n = 16), workshop papers ( n = 7), and symposium papers ( n = 4). All selected studies except one (2009) were published between 2012 and 2020. Figure 2 depicts the total number of the included papers per publication year. Although the number of publications dropped in 2018, in general, there is an increasing trend of publications between 2012 and 2019. For the year 2020, the result is shown as of July 3. A further observation is thus needed to confirm the increasing trend at the end of the year. The median score for study quality was 1 with the interquartile range of 0–1.5 (Appendix 2).
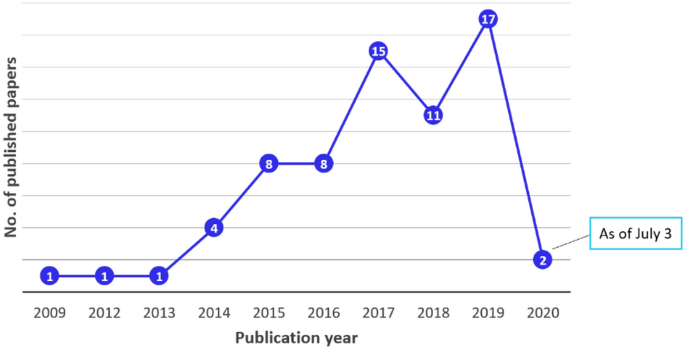
Publication trend
Types of Dynamic Data Sources Used for Requirements Elicitation
Dynamic data sources used for automated requirements elicitation.
Among dynamic data sources, human-sourced data sources have been primarily used as requirements sources. Among the three types of dynamic data sources, the vast majority (93%, n = 63) of the studies used human-sourced data sources for eliciting requirements. Only four studies (6%) explored using either machine-generated ( n = 2) or process-mediated ( n = 2) data sources. Almost all the studies focused on a single type of dynamic data source. We identified only one study attempting to integrate multiple types of dynamic data sources (1%).
The Specific Types of Dynamic Data Used for Automated Requirements Elicitation
The following seven data sources have been used for automated requirements elicitation: online reviews, micro-blogs, online discussions/forums, software repositories, software/app production descriptions, sensor readings, usage data from system–user interactions, and mailing lists (Table 5 ). Online reviews are reviews of a product or service that is posted and shown publicly online by people who have purchased a given service or product. Microblogs, which are typically published on social media sites, are a type of blog in which users can post a message in a form of different content formats such as short texts, audio, video, and images. They are designed for quick conversational interactions among users. Online discussions/forums are online discussion sites where people can post messages to exchange knowledge. Software repositories are platforms for sharing software packages or source codes, which primarily contain three elements: a trunk, branches, and tags. This study also considered issue-tracking systems as software repositories, which are detailed reports of bugs or complaints written in the form of free texts. Sensor readings are electrical outputs of devices that detect and respond to inputs from a physical phenomenon, which results in a large amount of streaming data. Usage data are run-time data collected when users are interacting with a given system. Mailing lists are a type of electronic discussion forums. E-mail messages sent by specific subscribers are shared by everyone on a mailing list.
Figure 3 depicts the types of dynamic data that have been used for automated requirements elicitation. Online reviews are the most frequently used type of dynamic data for eliciting requirements (53%), followed by micro-blogs (18%) and online discussions/forums (12%), software repositories (10%), and software/app product descriptions (7%). Other types of dynamic data include sensor readings (3%), usage data from system–user interactions (4%), and mailing lists (3%).
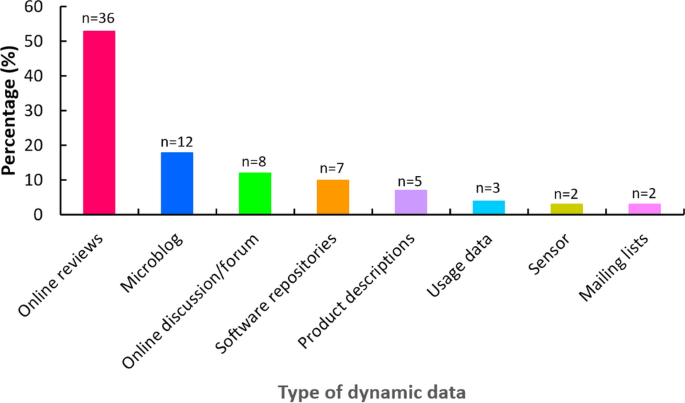
Types of dynamic data used for automated requirements elicitation
Several studies used multiple types of human-sourced data to gain complementary information and improve the quality of the analysis. Wang et al. [ 92 ] assessed whether the use of app changelogs improves the accuracy of identifying and classifying functional and non-functional requirements from app reviews, compared to the results obtained from the mere use of app reviews. Although there were no additional positive effects of app changelogs on improving the accuracy of automatic requirements classification, their subsequent study [ 93 ] shows that the accuracy of classifying requirements in app reviews by augmenting the reviews with the text feature words extracted from app changelogs.
Takahashi et al. in [ 100 ] used Apache Commons User List and App Store reviews. However, those two types of datasets were used independently without being integrated to evaluate their proposed elicitation process. Moreover, Stanik et al. [ 65 ] used three datasets: app reviews, tweets written in English, and tweets written in Italian. On the other hand, Johann et al. [ 94 ] integrated both app reviews and descriptions to provide information on which app features are or are not actually reviewed. In addition, Ali et al. [ 66 ] combined tweets for a smartwatch and Facebook comments of wearable and smartwatch.
Some studies used multiple types of software repositories. Morales-Ramirez et al. [ 84 ] used two types of datasets obtained from the issue tracking system of the Apache OpenOffice community and the feedback gathering system of SEnerCON, which is an industrial project in the home energy management domain. In their different study [ 79 ], open-source software mailing lists, and OpenOffice online discussions were used to identify relevant requirements information. Nyamawe et al. [ 87 ] used commits from GitHub repository and feature requests from JIRA issue tracker, while Oriol et al. [ 89 ] and Franch et al. [ 88 ] considered heterogenous software repositories.
Only one study used multiple types of data sources (e.g., human-sourced data and machine-generated data). Wüest et al. in [ 99 ] used both app user feedback (i.e., human-sourced data) and app usage data (i.e., process-mediated data).
Relation of Dynamic Data to an Organization of Interest
The majority of the studies used dynamic data that was external to the organization of interest. Of the 68 studies included in the analysis, 57 studies (85%) used dynamic data which was externally related to a given organization (i.e., data were collected outside of an organization’s platforms) [ 36 , 37 , 38 , 39 , 40 , 41 , 42 , 43 , 44 , 45 , 46 , 47 , 48 , 49 , 50 , 51 , 52 , 53 , 54 , 55 , 56 , 57 , 58 , 59 , 60 , 61 , 62 , 63 , 64 , 65 , 66 , 67 , 68 , 69 , 70 , 71 , 72 , 73 , 74 , 75 , 76 , 77 , 80 , 81 , 82 , 86 , 87 , 88 , 89 , 90 , 91 , 92 , 93 , 94 , 96 , 101 ]. Nine studies (13%) used dynamic data that were collected from platforms belonging to the organization: issue tracking systems [ 84 , 85 , 102 ]; user feedback from the online discussion and open-source software mailing lists [ 79 ]; sensors equipped with an intelligent product which is also known as a product embedded information devices (PEID) [ 95 ]; software production forum [ 103 ]; user feedback tool [ 99 ]. On the other hand, only two studies (3%) used both internal and external dynamic data [ 78 , 100 ].
Additional Use of Domain Knowledge Used for Requirements Elicitation
Only one study considered additional inclusion of domain knowledge in eliciting requirements. Yang et al. [ 44 ] combined the app review analysis and the Wizard-of-Oz technique for the requirements elicitation process. The results indicate that integrating the two sources can complement each other to elicit more comprehensive requirements that cannot be obtained from either one of the sources.
Approaches for Automated Requirements Elicitation
Approaches used for human-sourced data.
Since human-sourced data are typically expressed in natural language, natural language processing (NLP) is commonly used for analyzing this type of data. All of the 63 studies which used human-sourced information started the requirements elicitation process by preprocessing the raw data using NLP techniques. Data preprocessing typically involves removing noise (e.g., HTML tags) to retain only text data. Another critical data preparation activity is tokenization, which means splitting the text into sentences and tokens (words, punctuation marks, and digits), respectively.
Further analysis of the text using NLP typically involves syntactic analysis, such as part-of-speech tagging. Two studies have used speech-acts, which are acts performed by a speaker when making an utterance, as parameters to train supervised learning algorithms [ 79 , 84 ]. For eliciting requirements, nouns, verbs, and adjectives are often identified since they are more likely used for describing requirements-related information than other parts of speech, including adverbs, numbers, and quantifiers [ 40 ].
A common preprocessing activity is stopword filtering, which involves removing tokens that are common but carry little meaning, including function words (e.g., “the”, “and”, and “this”), punctuations (e.g., “.”, “?”, and “!”), and special characters (e.g., “#” and “@”), and numbers. Normalization is moreover often carried out by lowercasing (i.e., convert all text data to lowercase), stemming (i.e., reduce inflectional word forms to their root form such as reducing “play”, “playing” and “played” to their common root form of “play”) and lemmatization (i.e., grouping the different inflected forms of words which are syntactically different but semantically equal to be analyzed as a base form, called lemma, such as grouping “sees” and “saw” into a single base form of “see”).
Once the text data have been preprocessed, features are typically extracted for the subsequent modeling phase. Feature extraction can be done using a bag of words (i.e., simply count occurrences of tokens without considering word order nor normalizing counters), n -grams (i.e., extract the contiguous sequence of n tokens such as bi-gram which indicates the extraction of token pairs), and collocations (i.e., extract a sequence of words that co-occur more often than by chance, for example, “strong tea”). To evaluate how important a word is for a given document, a bag of words are often weighted, using a weighting scheme such as term frequency-inverse document frequency (tf-idf), which gives high weights to words that have a high frequency in a particular document, while having a low frequency in an entire set of documents. Other common features are based on syntactic or semantic analysis of the text (e.g., part-of-speech tags). Sentiment analysis, which is the automated process of identifying and quantifying the opinion or emotional tone of a piece of text through NLP, was used in 18 studies (38%), either to feed into algorithms as features to increase the accuracy of the algorithms or to understand user satisfaction.
After preprocessing the human-sourced data and extracting features for data modeling, the next step of requirements elicitation was to perform either classification or clustering. Classification refers to classifying (text) data into pre-defined categories related to requirements, for example, classifying app reviews into bug reports, feature requests, user experiences, and text ratings [ 38 ]. Classification has been performed using three approaches: machine learning (ML), rule-based classification, or model-oriented approaches. In the ML approach, classification is performed by a model built by a learning algorithm based on pre-labeled data.
In the ML approach, various learning algorithms automatically learn statistical patterns within a set of training data, such that a predictive model is able to predict a class for unseen data. In most studies, ML relied on supervised ML. In supervised ML, a predictive model is built based on instances that were pre-assigned with known class labels (i.e., training set). The model is then used to predict a label associated with unseen instances (i.e., test set). A downside with supervised ML is that it typically requires a large amount of labeled data (i.e., ground-truth set) to learn accurate predictive models.
To reduce the cost of labeling a large amount of data, a few studies used the active learning paradigm and semi-supervised machine learning for classification. Active learning enables machines to wisely select unlabeled data points to be labeled next in a way that optimizes a decision boundary created by a given learning algorithm and interactively queries the user to label the selected data points to improve classification accuracy. Semi-supervised learning is an intermediate technique between supervised and unsupervised ML, which utilizes both labeled and unlabeled data in the training process.
Rule-based classification is a classification scheme that uses certain rules, such as language patterns. Rule-based classification excels in performing simpler tasks where domain experts can define rules, while classification using ML works well for the tasks which are easily performed by humans but where (classification) rules are hard to formulate. However, listing all the rules can be tedious and needs to be hand-crafted by skilled experts with abundant domain knowledge. Moreover, rules might need to be refined as new datasets become available, which requires additional resources and limits scalability [ 77 ]. A model-oriented approach, which includes utilizing conceptual or meta-models, are applied to define and relate the mined terms and drive classification.
On the other hand, clustering has been performed using either topic modeling or more traditional clustering techniques. Topic modeling is an unsupervised (i.e., learn from unlabeled instances) dimension reduction and clustering technique, which aims to discover hidden semantic patterns in the collection of a document. Topic modeling is used to represent an extensive collection of documents as abstract topics consisting of a set of keywords. In automated requirements elicitation, topic modeling is mainly used for either discovering system features or grouping similar fine-grained features that are extracted using different approaches into high-level features. Traditional clustering is an unsupervised ML technique that aims to discover the intrinsic structure of the data by partitioning a set of data into groups based on their similarity and dissimilarity. Among the selected studies, traditional clustering has been mainly used to discover inherent groupings of features in requirements-related information.
Some studies have performed clustering after classification. Classification was first performed to identify and classify requirements-related information, using machine learning or rule-based classification. Clustering is then applied to the identified requirements-related information (e.g., improvement requests), while ignoring data irrelevant to requirements, to discover inherent groupings of features, using topic modeling or traditional clustering. Table 6 provides a more detailed description of the automated approaches proposed in each study.
Figure 4 depicts the descriptive statistics of the approaches for automated requirements elicitation used in the selected studies. For classification, the most commonly used approach was based on the ML approach (60%), followed by rule-based classification (17%) and model-oriented approach (6%). For clustering, topic modeling (16%) was the most commonly used approach, followed by more traditional clustering techniques (13%) and unsupervised rule-based clustering (2%).
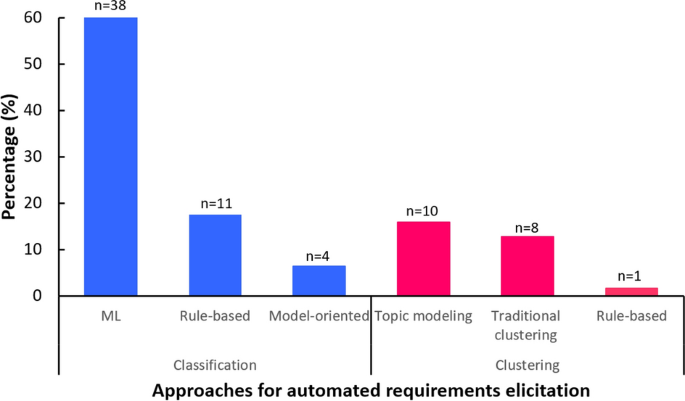
Techniques used for requirements elicitation from human-sourced data that are grouped according to classification (i.e., machine learning (ML), rule-based classification, and model-oriented approach) and clustering (i.e., topic modeling, traditional clustering, and rule-based unsupervised NLP)
In nine studies, two different approaches have been combined. Two studies performed classification with supervised ML for filtering and subsequently conducted clustering with topic modeling [ 47 , 68 ]. Guzman et al. [ 68 ] first ran Multinomial Naïve Bayes and Random Forest, which are both supervised learning algorithms, to extract tweets that request software improvement. Biterm Topic Model, which is a topic modeling used for short texts, was then used to group semantically similar tweets for software evolution. Zhao and Zhao [ 47 ] ran a supervised deep-learning neural network was first used to extract software features, and their corresponding sentiments and hierarchical LDA was subsequently to extract hierarchical software features with positive and negative sentiments.
Two studies performed classification using ML, which was followed by unsupervised clustering analysis [ 53 , 58 ]. Jiang et al. [ 58 ] used Support Vector Machine, or a supervised machine-learning algorithm, for pruning incorrect software features that were extracted from online reviews. K-means clustering, an unsupervised clustering analysis, was then performed to categorize the extracted features into semantically similar system aspects. Sun and Peng [ 53 ] first used Naïve Bayes, a supervised machine-learning algorithm, for filtering informative comments, which were subsequently clustered using K-means, an unsupervised clustering analysis.
Jiang et al. [ 41 ] first performed rule-based classification based on syntactic parsing and sentiment analysis to extract opinions about software features and their corresponding sentiment words. Subsequently, S-GN, whose base algorithms are a type of K-means clustering, was performed to cluster similar opinion expressions about software features into a category which represents an overall, functional, or quality requirements. On the other hand, Bakar et al. [ 63 ] combined unsupervised clustering analysis and topic modeling in which K-means was first run to identify the similar documents. They then performed latent semantic analysis, which is a type of topic modeling, to group similar software features within the documents.
Guzman and Maalej [ 40 ] and Dalpiaz and Parente [ 46 ] first extracted software features based on rule-based classification, which uses collocation finding algorithm and the LDA was subsequently applied to group similar software features. Zhang et al. [ 60 ] first used linear regressions based on supervised ML to select helpful online reviews. Then conjoint analysis (i.e., a statistical technique used in market research to assess and quantify the consumers’ values on product features or service) was performed to assess the impact of the features from helpful online reviews on the consumers’ overall rating.
In several studies, visualization has been provided to help requirements engineers efficiently sift through and effectively interpret the most important requirements-related information. Bakiu and Guzman [ 55 ] first performed the aggregation of features. The results were then visualized at two levels of granularity (i.e., high-level and detailed). Sun and Peng [ 53 ] first extracted scenario information of similar user comments and then aggregated and visualized as aggregated scenario models. Software features [ 52 ] and technically informative information from the potential requirements sources [ 64 , 86 ] were summarized, ranked, and visualized using word clouds. Luiz et al. [ 49 ] summarized overall user evaluation of the mobile applications, their features, and the corresponding user sentiment polarity and scores in a single graphical interface. Oriol et al. [ 89 ] implemented a quality-aware strategic dashboard, which has various functionalities (e.g., quality assessment, forecasting techniques, and what-if analysis) and allows for maintaining traceability of quality requirements generation and documentation process. Wüest et al. [ 99 ] fused user feedback and correlated GPS data and visualize the fused data on a map, equipping the parking app with context-awareness.
Techniques Used for Process-Mediated Data
The two studies that used process-mediated data focused on eliciting emerging requirements through observations and analysis of time-series user behavior (i.e., run-time observation of system–user interactions) and the corresponding environmental context values [ 97 , 98 ]. In both studies, Conditional Random Fields (CRF), which is a statistical modeling method, was used to infer goals (i.e., high-level requirements).
Xie et al. [ 97 ] proposed a method to elicit requirements consisting of the three steps. First, a computational model is trained and built based on pre-defined user’s goals in the domain knowledge, using supervised CRF to infer user’s implicit goals (i.e., outputs) from the observation and analysis of run-time user behavior and the corresponding environmental values (i.e., inputs). After the goal inference, the user’s intention (i.e., the execution path) for achieving a given goal is obtained by connecting the situation (i.e., a time-stamped sequence of user behavior that is labeled with a goal and environmental context values) labeled with the same goal into a sequence. Finally, an emerging intention, which is a new sequence pattern of user behavior that has not been pre-defined or captured in the domain knowledge base, is detected.
An emerging intention can occur in three cases; when a user has a new goal; when a user has a new strategy for achieving an existing goal; when a user cannot perform operations in an intended way due to system flaws. Requirements, thus, can be elicited by validating emerging intentions by domain experts based on the analyses of goal transition, divergent behaviors from the optimal usage, and erroneous behavior.
In the analysis of goal transition, domain experts look at two goals that frequently appear consecutively based on the results of goal inference with a high confidence level assigned by the CRF and elicit requirements that make the goal transition smoother.
In the analysis of divergent behavior, domain experts focus on user behaviors that deviate from an expected way to operate the system because the user’s irregular behavior may indicate user’s misunderstanding of required operational procedures, dissatisfaction with the system, and emerging desires. Those divergent behaviors are given a low confidence level by the CRF model.
In the analysis of erroneous behavior, requirements can be elicited by investigating the error reports with high occurrences that may reflect users’ emerging desires that are not supported by the current system. In addition, requirements can be elicited from user behaviors, which are actually normal behavior but are mistakenly considered as erroneous due to the system flaws. The proposed method is assumed to be used in a sensor-laden computer application domain. Thus, it may also be applicable to machine-generated data. The main challenge, however, is to increase the level of automation for analyzing potential emerging intentions and users’ emerging requirements.
Yang et al. in [ 98 ] used CRF to infer goals based on a time-stamped sequence of user behavior that is labeled with a goal and environmental context values, which is called a situation. Based on the results of goal inference, intention inference was performed by relating a sequence of situations that are labeled as the same goal. When an intention has not been pre-defined in the domain knowledge base, the intention is detected as an emerging intention and exported as possible new requirements for future system development or evolution.
However, the method proposed in both studies still requires a substantial degree of human oracles, which needs to be reduced in future research to increase the scalability and promote the implementation of their approach in real-life settings. In addition, the proposed method does not yet support diverse requirements. The method proposed by Xie et al. [ 97 ], capture only emerging functional but not non-functional requirements. The approach proposed in [ 98 ] can only support the identification of the low-level design alternatives (i.e., new ways of fulfilling a given intention).
Notably, Wüest et al. in [ 99 ] proposed to use both human-sourced and process-generated data. Their approach is based on the control loop for self-adaptive systems for collecting and analyzing user feedback (i.e., human source data) as well as system usage and the location data (i.e., GPS data). The analysis is driven by rules or models of expected system usage. The system decides how to interpret the results of the analysis and modify its behavior at run-time, which allows for understanding changing user requirements for software evolution.
Techniques Used for Machine-Generated Data
Voet et al. [ 95 ] first extracted goal-relevant usage elements as features, from the data recorded via a handheld grinder, a type of product embedded information devices (PEID) equipped with sensors and onboard capabilities. Feature selection was then performed to reduce system workload and improve the prediction accuracy of the machine-learning algorithm, compared to using raw sensor data. Specifically, the support vector machine classifier, which is a supervised machine-learning algorithm, was used to build and train the model to predict the four different usage element states. The model was then tested on the sensor data from the two different usage scenarios that have not been used for training. The collection of the predicted usage element states, or user profiles, can be analyzed manually or by clustering to identify the deviation from the intended optimal usage profile. Requirements can be inferred by analyzing users’ deviant behaviors.
Liang et al. [ 96 ] mined user behavior patterns from instances of user behavior, which consist of user context (i.e., time, the location and the motion state of the crowd mobile users) and the currently running apps, using Apriori-M algorithm, which is an efficient algorithm based on Apriori algorithm that is used for frequent item set mining. User behavior patterns, which infer emergent requirements or requirements changes, are ranked and used for service recommendation. Service recommendation is performed periodically, using the service recommendation algorithm. The algorithm takes mined user behavior as inputs and outputs the apps to remind the user. In service recommendation, matching is performed between the current user context and the context of user behavior patterns mined from mobile crowd users, according to the ranking order. If the two matches, the mobile app(s) in the user behavior patterns are automatically recommended to the user as solutions to meet the requirements inferred from user behavior patterns.
In summary, most of the existing solutions support the elicitation of requirements from a single data source, primarily from human source data. There is a lack of methods to support requirements elicitation from heterogeneous data sources. In addition, only a few studies have supported context-awareness and real-time data processing and analysis. Those features are crucial to enable continuous and dynamic elicitation of requirements, which are especially important for context-aware applications and time-critical systems such as health systems. Moreover, many studies lack the argument on how each proposed solution help processing a large volume of data.
Evaluation methods include three components: evaluation approach, concept, and metrics. Of the 68 selected studies, controlled experiments were the most frequently applied approach for evaluating the proposed artifact (75%), followed by a case study (19%) and a proof of concept (6%) (Fig. 5 a).
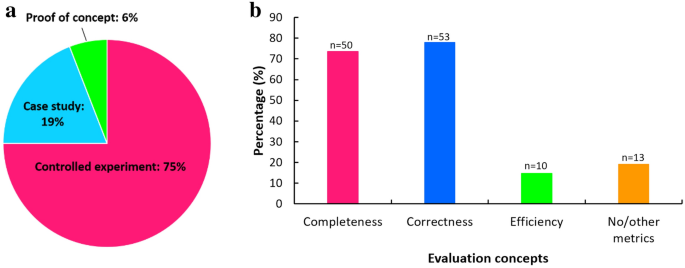
a Evaluation approach, b Evaluation concepts
Among the 51 studies that used controlled experiments, 46 studies compared the results produced by the proposed artifacts against a manually annotated ground-truth set. For example, Bakiu and Guzman [ 55 ] compared the performance of multi-label classification against a manually created golden standard in classifying features extracted from unseen user reviews into different dimensions of usability and user experience.
Only three studies compared the performance of the proposed artifact with the results of manual analysis without the aid of automation [ 57 , 62 , 78 ]. For example, Bakar et al. [ 62 ] compared the software features that were extracted using their proposed semi-automated method with those that were obtained manually.
Two studies conducted an experiment in different ways. Liang et al. [ 96 ] used a longitudinal approach for conducting an experiment. They compared obtained user behavior patterns with those that were collected after some time interval to confirm the correctness of the Apriori-M algorithm. Abad et al. [ 44 ] compared Wizard-of-Oz (WOz) and user review analysis qualitatively. In a few studies [ 46 , 88 , 90 ], the proposed techniques have been evaluated with intended users. The rest of the studies used a case study or a proof of concept as an evaluation approach.
Most frequently used evaluation concept was correctness (78%), followed by completeness (74%), no/other metrics (13%), and efficiency (10%) (Fig. 5 b). Other metrics, for example, include usability, creativity, the intended user’s perceived usefulness, and satisfaction. Most of the studies combined several evaluation concepts. Three different combinations of the concepts were identified: (1) completeness and correctness ( n = 42), (2) completeness and correctness and efficiency ( n = 7), and (3) correctness and efficiency ( n = 2). In most cases, the correctness and completeness were assessed using precision (i.e., the fraction of correctly predicted instances among the total predicted instances) and recall (i.e., the fraction of correctly predicted positive instances among all the instances in actual class), respectively. In addition, F-measure was also used to address a trade-off between precision and recall.
Efficiency has been assessed in terms of the size of training data [ 38 , 39 ], the time to recognize and classify software features [ 76 ], the time required to identify relevant requirements information for both manual and automated analysis [ 57 ], the time taken to complete the extraction of software features [ 63 ], the time and space needed to build the classification model [ 48 , 50 , 51 ], and the total execution time of the machine-learning algorithm [ 78 ]. The user’s perceived efficiency was measured using a 5-point Likert scale [ 89 ]. (Fig. 6 )
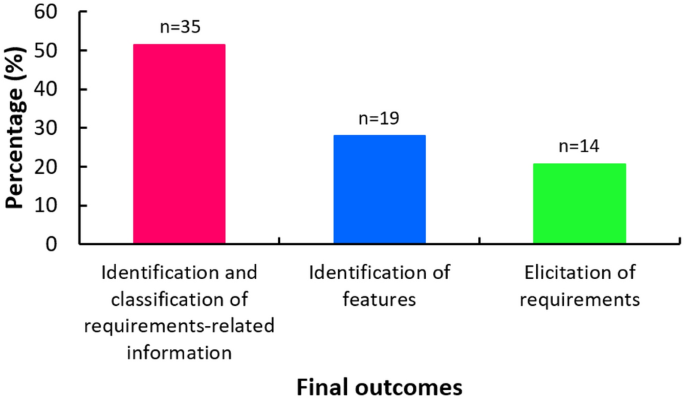
Final outcomes of automated requirements elicitation
The Outcomes of the Automated Requirements Elicitation
Expression of final outcomes produced by the automated part of requirements elicitation.
Outcomes of the automated requirements elicitation have been classified into the following three categories: (1) identification and classification of requirements-related information, (2) identification of candidate features related to requirements, and (3) elicitation of requirements (Table 7 ). Only 21% of the studies have enabled the automated elicitation of requirements. A majority of the studies have resulted in automated identification and classification of requirements-related information (51%), or identification of candidate features related to requirements (28%) (Fig. 5 ).
Identification and classification of requirements-related information have been made by classifying dynamic data into different classes of issues based on; relevance to different stakeholders for identifying responsibilities, the technical relevance for filtering only relevant data (e.g., classifying into either feature request or other), and types of technical issues to be inspected (e.g., classifying into feature requests, bug reports, user experiences, and user ratings, and classifying into functional or non-functional requirements). Some studies performed classification at a deeper level (e.g., classifying into four types of non-functional requirements (i.e., usability, reliability portability, or performance, or functional requirements).
Identification of candidate features related to requirements refers to discovering functional components of a system. Features, however, typically have less granularity than requirements and do not tell what behavior, conditions, and details would be needed to obtain the full functionality. They, thus, need to be further processed to become full requirements.
Elicitation of requirements has been done mostly at high level. Most of them elicited requirements at high level in the form of goals, aggregated scenarios, or high-level textual requirements. Franch et al. [ 88 ] and Oriol et al. [ 89 ] semi-automated the elicitation of complete requirements in the form of user stories and requirements specified in semi-formal language.
Degree of Intended Automation
A proposed artifact was classified into the two levels of the intended automation: intended full automation or semi-automation. Of note is that we consider artifacts that support the automation of requirements elicitation either entirely or partially. Artifacts are classified into intended full automation in the following two circumstances: (1) when the proposed part is automated without human intervention for completion or (2) when only minimum interactions are needed for completion. Minimum human interaction is defined as human oracles being in the loop once at the initial stage of the elicitation process, which includes the creation of the ground-truth set and conceptual models as well as the specification of a set of keywords and language patterns. Based on the definitions, the majority of the proposed methods (84%) were intended to be fully automated, while the rest are semi-automated methods that require human oracles to be in the loop for each iteration of the process.
The majority of the selected studies exclusively focused on enabling requirements elicitation from dynamic data, without considering other requirements engineering activities. Of the 68 studies included in the analysis, 50 studies (74%) exclusively proposed methods to enable automated requirements elicitation, while 18 studies (26%) supported other requirements engineering activities in addition to requirements elicitation. Prioritization was the most frequently supported additional requirements engineering activity ( n = 11), followed by elicitation for change management ( n = 7), and documentation ( n = 2). More detailed information is provided in Table 8 .
We conducted a systematic literature review on the existing data-driven methods for automated requirements elicitation. The main motivations for this review were two-fold: (1) using dynamic data has the potential to enrich stakeholder-driven requirements elicitation by eliciting new requirements which cannot be obtained from other sources, and (2) no systematic review has been conducted on the state-of-the-art methods to elicit requirements from dynamic data from unintended digital sources. Of 1848 records retrieved from 6 electronic database search and 1017 articles identified through backward and forward reference search, we selected 51 studies that met our inclusion criteria and included in the final analysis to answer the following three research questions. RQ1: What types of dynamic data are used for automated requirements elicitation? RQ2: What types of techniques and technologies are used for automating requirements elicitation? RQ3: What are the outcomes of automated requirements elicitation? In the following sections, we provide a discussion of the main findings, the identified research gaps, and issues to be addressed in future research.
RQ1: What Types of Dynamic Data Are Used for Automated Requirements Elicitation?
Existing research on data-driven requirements elicitation from dynamic data sources has primarily focused on utilizing human-sourced data in the form of online reviews, micro-blogs, online discussions/forums, software repositories, and mailing lists. The use of online reviews was substantially more prevalent, compared to other types of human-sourced data. The result indicates the current data-driven requirements elicitation is largely crowd-based. On the contrary, process-mediated and machine-generated data sources have only, in some instances, been explored as potential sources of requirements. The predominance of human-sourced information is rather expected and can be explained by the following two reasons: (1) users’ preferences and needs regarding system are typically explicitly expressed in natural language, from which it is—relatively speaking—straightforward to obtain requirements compared to process-mediated and machine-generated data, and (2) there are abundant sources of human-sourced data that are publicly available and readily accessible.
Much more research is, thus, needed to develop methods capable of eliciting requirements from process-mediated and machine-generated data that are not expressed in natural language and from which requirements need to be inferred. There is still a lack of methods to infer requirements as well as evidence regarding the applicability of the proposed approach to more diverse types of process-mediated and machine-generated data. Process-mediated and machine-generated data enable run-time requirements elicitation [ 19 ]. They also help system developers to understand usage data and the corresponding context, which allows elicitation of performance-related as well as context-dependent requirements [ 19 ]. In addition, almost all of the studies have focused on using only a single type of dynamic data and typically also a single data source.
A few studies have utilized multiple human-sourced data sources; however, there has been only one attempt to combine different types of dynamic data sources. As such, there is currently insufficient evidence that using multiple types of data leads to more effective requirements elicitation, but it remains an open issue that merits investigation. We believe that research in this direction would be highly interesting in an attempt to improve data-driven requirements elicitation, both in terms of the coverage and quality of the elicited requirements. Utilizing semantic technologies can be useful for enabling the integration of heterogeneous data sources [ 107 ].
In addition, only one study integrated dynamic data and domain knowledge to elicit requirements [ 44 ]. The results from that study indicate the potential benefits of using dynamic data together with domain knowledge to elicit requirements that cannot be captured using either one of the data sources. It is likely that domain knowledge, which is typically relatively static but of high quality, can help to enrich data-driven requirements elicitation efforts from dynamic data sources. A larger number of studies are needed to confirm the impacts of integrating domain knowledge with dynamic data on the quality and diversity of outcomes obtained from the automated requirements process.
RQ2: What Types of Techniques and Technologies Are Used for Automating Requirements Elicitation?
Techniques used for the automated requirement elicitation.
Human-sourced data are typically expressed in natural language, which is inherently difficult to analyze computationally due to its ambiguous nature and lack of rigid structure. In all the selected studies, human-sourced data have been (pre-)processed using natural language processing techniques to facilitate subsequent analysis. Although the techniques used for preprocessing varies across studies, data cleaning, text normalization, and feature extraction for data modeling are frequently performed preprocessing steps in automated requirements engineering. Commonly used features include surface-level tokens, words, and phrases, but also syntactic (e.g., part of speech tags) and semantic features (e.g., the positive/negative/neutral sentiment of a sentence). After data preparation and feature extraction, data modeling or analysis for the purpose of requirements elicitation is typically performed using classification or clustering, or classification followed by clustering.
Classification in the context of automated requirements elicitation involves either of the following three tasks: (1) filtering out data irrelevant to requirements, (2) classifying text based on the relevance to different stakeholder groups, or (3) classifying text into different categories of technical issues, such as bug reports and feature requests. The classification tasks have been tackled using either rule-based approaches or machine learning, which is mostly done within the supervised learning paradigm. Although supervised machine learning can achieve high predictive performance in a well-defined classification task, it requires access to a sufficient amount of human-annotated data. As a result, many studies involved human to annotate data into pre-defined classes. The labeling task, however, is labor-intensive, time-consuming, and error-prone due to a considerable amount of noise and the ambiguous nature inherent in natural language [ 35 ].
Two solutions have been proposed to reduce the cost of labeling a large amount of data: active learning [ 35 ] and semi-supervised machine learning [ 43 ]. Dhinakaran et al. in [ 35 ] showed that classifiers trained with active learning strategies outperformed in classifying app reviews into feature requests, bug reports, user rating, or user experience than the baseline classifies that were passively trained on the randomly selected dataset. Deocadez et al. in [ 43 ] demonstrated that three semi-supervised algorithms (i.e., Self-training, RASCO, and Rel-RASCO) with four base classifiers achieved comparable predictive performance as that of classical supervised machine learning in classifying app reviews into functional or non-functional requirements. Although there is not a sufficient number of studies to draw a generalizable conclusion, classification using active learning and semi-supervised machine-learning strategies may have similar potential as conventional supervised machine learning in identifying and classifying requirements-related information, but requires a much smaller amount of labeled data compared to conventional supervised machine learning.
Another issue that needs to be addressed when using supervised learning is that human-sourced data sources include a significant proportion of non-informative and irrelevant data. Eliciting requirements from this source is thus often compared to “looking for a needle in a haystack” [ 70 ]. This leads to a highly unbalanced class distribution in terms of the non-informative and irrelevant data compared to the informative and relevant classes. The underlying class distribution largely affects the performances of machine learning-based classifiers [ 42 , 71 ]. In one study [ 42 ], the precision, recall, and F 1 measures for the under-represented classes were worse than those for the better-represented classes. Given that the classes relevant to requirements are not represented equally in most real-life occasions, the issue needs to be addressed in future research. One possible solution to resolve this issue may be applying different sampling techniques such as Synthetic Minority Oversampling Technique (SMOTE) to the training set to increase the number of instances in the class with fewer observations [ 71 , 84 ].
Contextualization may be another possible solution, which is done by filtering out non-informative and irrelevant data. Several studies [ 47 , 53 , 58 , 68 ] have used supervised classification before performing finer-grained classification or clustering. Filtering out noisy data can improve the classification or clustering accuracy. It also helps requirements engineers pinpoint the data relevant to requirements by automatically discarding non-informative data for requirements elicitation [ 69 ] as well as supports efficiently distributing data to the appropriate stakeholders within an organization [ 69 ]. Since contextualization can reduce the volume of data to be processed further, it mitigates the volume issue of Big Data.
Various supervised learning algorithms have been used to automate the requirements elicitation process. However, there is no “one-size-fits-all” algorithm that performs best for every single case, which is often referred to as the “No free lunch” theorem [ 108 ]. Experimenting and comparing many different algorithms for a specific problem demands time and domain knowledge related to machine learning from requirements engineers in addition to routine work. It would thus be helpful for them if the support tool were to accommodate functions that automatically identify and recommend the best algorithm among possible options.
Moreover, it would be even more valuable if the tool supports automatic optimization of the parameter configuration, which includes preprocessing, selection of machine-learning features, hyper-parameter settings, and evaluation metrics. Supervised machine learning has mainly been used for identifying and classifying data into pre-defined categories related to requirements. This is because supervised machine learning works well for tasks for which classification rules are difficult to formulate. Nevertheless, it requires a sufficient amount of human-annotated data to build a reliable predictive model, which is a time-consuming and error-prone task. On the other hand, rule-based classification, which was the second most frequently used classification approach, excels in performing simpler tasks for which rules can be formulated. In the literature, rule-based classification has been used for identifying candidate features more frequently than identifying and classifying requirements-related information. For rule-based classification to function well, however, sound domain knowledge is required to appropriately define rules that drive the classification process and determine the effectiveness of the classification.
Clustering has been used primarily for identifying candidate features or grouping semantically similar features. In the selected studies, clustering has been performed, using topic modeling or traditional clustering, which can be valuable alternatives to supervised learning in the absence of labeled historical data. More than half the studies that used clustering first classified data into pre-assigned categories relevant to requirements, which was primarily done using supervised machine learning or rule-based classification. Clustering is subsequently performed on the requirements-related information identified by classification, using topic modeling or traditional clustering. Those unsupervised machine-learning techniques, however, often lead to less accurate results than supervised leaning since there is no knowledge about output data.
The effectiveness of clustering can be affected by many factors (e.g., the number of clusters and selection of initial seeds), and evaluating unsupervised learning is problematic due to a lack of well-defined metrics. This may be a reason that classification is performed before clustering. Nevertheless, there are some efforts to ensure high quality of clustering. Cleland-Huang et al. [ 78 ] proposed the automated forum management (AFM) system that employs Stable Spherical K-Means (SPK) to mine feature requests from discussion threads in open source forums. In their study, Normalized Mutual Information (NMI) was computed to evaluate and ensure the quality of the cluster. In addition, since the selection of initial seeds highly influence on clustering results, the problem is mitigated by applying consensus clustering for the initial clustering. On the other hand, Sun and Peng [ 53 ] used the recommended cluster number (RCN) to determine the optimal number of clusters. There are also other metrics available to evaluate the quality of clustering, such as the Silhouette index. However, the consensus has not been reached regarding which measure to use for the evaluation because it depends on the nature of data and the desired clustering task.
Moreover, only a small proportion of the studies supported the visualization of the obtained results. Data visualization increases the interpretability of the results by leveraging visual capacity, which helps identify new and hidden patterns, outliers, and trends [ 16 ]. It also facilitates communication among different stakeholders within an organization. Providing visualizations, thus, is recommended to help requirements engineers understand the results and make a subsequent decision more efficiently and effectively.
Process-Mediated and Machine-Generated Data Sources
As described in the previous section (i.e., “RQ1: What types of dynamic data are used for automated requirements elicitation?”), our results indicate that there is a huge research gap in eliciting requirements from process-mediated and machine-generated data. Much more research should focus on exploring the methods to elicit requirements from data that are not written in natural language. Only two studies leveraged process-mediated data, both utilizing CRF, to infer goalswhich are high-level requirements. More research is need to develop methods and algorithms to elicit requirements from various types of process-mediated data.
Likewise, machine-generated data were used as requirements sources in two studies. Liang et al. [ 96 ] proposed to use the Apriori-M algorithm to infer context-aware requirements from behavior patterns that are mined from the run-time behavior of the mobile user. The results of the analysis lead to provide the user solutions that satisfy the inferred requirements. On the other hand, Voet et al. [ 95 ] proposed a method to classify goal-relevant usage element states using supervised machine learning and infer requirements based on the deviation from the optimal usage profile, which can be detected by manual analysis or unsupervised clustering.
Given that IoT data are one of the main driving forces of Big Data generation, there is a pressing need to develop a framework to elicit requirements from IoT data. Applying semantic technologies may be a promising solution to help machines interpret the meaning of data by semantically representing raw data in a human/machine interpretable form [ 107 ], which can facilitate the automatic requirements elicitation from large volumes of heterogeneous IoT data.
Rigorous evaluation is essential for ensuring that a proposed artifact meets its intended objectives, justifying its effectiveness and/or efficiency, and identifying its weaknesses, which need to be rectified in future work. The artifacts proposed in most of the identified studies were primarily evaluated through controlled experiments. Controlled experiments eliminate the influence of extraneous and unwanted variables that could account for a change of the dependent variable(s) other than the independent variable(s) of interest. Thus, their two main advantages are: (1) they are the most powerful method for inferring causal relationships between variables, and (2) they can achieve high internal validity [ 109 ]. Nevertheless, their main disadvantage is that since they are typically conducted in an artificial environment, conclusions may not be valid in real-life settings, which threatens the external validity [ 109 ].
Most studies that used controlled experiments as an evaluation approach evaluated results derived from a proposed artifact against a manually created ground-truth set. The quality of the ground-truth set, however, determines the performance of machine-learning algorithms. The majority of the studies, thus, recruited multiple annotators for the labeling task to obtain a “reliable” ground-truth set, which only contains peer-agreed labels. Some studies used an annotation guideline, performed a pilot run of classification tasks with small samples to avoid subjective assessment, reduce disagreements, and increase the quality of manual labeling [ 38 , 39 , 68 ].
Besides, a few studies compared the performance of automated analysis with a proposed artifact with the performance achieved by solely relying on manual analysis without the aid of the proposed artifact. Groen et al. [ 57 ] justified the efficiency and scalability of automated user review analysis and emphasized the need of automation for analyzing a large volume of dynamic data to support continuous requirements engineering. A case study was the second most frequently used evaluation approach in which the proposed methods are assessed through in-depth investigations of a specific instance in a real-life context. Proof of concept was used in a small proportion of the selected studies. It is used to demonstrate the feasibility of a proposed artifact theoretically to achieve an intended task. Although it may be suitable as a preliminary or formative evaluation, it has lower explanatory power compared to comparative evaluations (e.g., controlled experiments and case studies).
Most studies used standard metrics that are often used in the field of information retrieval. Completeness and correctness were the evaluation concepts that were the most frequently used in the studies, while some studies also assessed the efficiency of an artifact. Recall and precision were often used as metrics to measure completeness and correctness, respectively. Since there is a trade-off between precision and recall, many studies additionally used F-measure, which is the weighted harmonic mean of precision and recall. Most of the studies used F 1 -measure, which assigns equal weights on precision and recall (i.e., the harmonic mean of precision and recall). However, Guzman et al. [ 69 ] recall was assigned more importance (i.e., weights) than precision based on the study which claims that recall should be favored over precision since missing relevant requirements is more detrimental [ 110 ]. On the other hand, precision is also important when dealing with a dataset that contains large amounts of irrelevant information. Future research may explore techniques to optimize F-measures, including a weighted maximum likelihood solution [ 111 ]. Moreover, few studies have compared the effectiveness of automated requirements elicitation with that of traditional requirements elicitation driven by stakeholders. This can largely be explained by the fact that research on automated requirements elicitation is not mature enough since most methods have focused on identifying and classifying requirements-related information rather than eliciting requirements. However, this needs to be addressed in future research to demonstrate the value of automated requirements elicitation.
RQ3: What Are the Outcomes of Automated Requirements Elicitation?
Expression of requirements elicitation.
In traditional requirements engineering, requirements elicitation begins with the identification of relevant requirements sources such as stakeholders and domain documents, which is followed by two other sub-activities: the elicitation of existing requirements from the identified sources and elicitation of new and innovative requirements [ 1 ].
On the other hand, dynamic data-driven requirements elicitation has been done in the form of the following three activities: (1) identification and classification of requirements-related information, (2) identification of candidate features related to requirements, and (3) elicitation of requirements. However, those three activities have not necessarily been performed entirely nor sequentially. For example, many studies that aim to identify candidate features first performed classification, using supervised learning or rule-based classification, before clustering features, using topic modeling or traditional clustering, while the rest of them directly identified candidate software features, mainly using topic modeling or rule-based classification. One possible reason for performing classification before clustering is that classification can only classify data into coarse categories, which may include the repetitive information and the same sentiment, while clustering can further group individual data in a meaningful way. Thus, the specific combination of the two approaches can facilitate the work of requirements engineers (e.g., requirements reuse).
Most of the proposed methods supported the identification and classification of requirements-related information or the identification of candidate features. Identification and classification of requirements-related information help requirements engineers save time for the data analysis by filtering out a significant amount of irrelevant data and selectively identify a specific type of information which they are interested in such as feature request. It also helps to allocate the extracted data based on the relevance to stakeholder groups to support parallel data analysis within the same organization. Identification of candidate features helps requirements engineers understand user-preferred features and select features to be considered in software development and evolution. Features, however, are not yet formulated as requirements because those features require the engagement of requirements engineers to transform into requirements.
On the other hand, only about 20% of the studies automated the entire requirements elicitation. In most cases, the elicited requirements are high-level such as goals, aggregated scenarios, or high-level textual requirements. Those high-level requirements, however, do not include details of the objects (i.e., features) which are being concerned, nor conditions. This highlights the need for developing additional automated approaches or using traditional elicitation techniques with the involvement of human stakeholders to complete the requirements elicitation process.
A majority of the studies proposed methods that are intended to be fully automated after the minimum human interventions at the initial stage of the continuous elicitation process. However, most studies do not yet support the entire requirements elicitation. Given the high volume and velocity of dynamic data, requirements elicitation certainly needs to be automated to enhance efficiency and scalability.
However, fully automated methods are not necessarily better than semi-automated methods concerning the quality of requirements and the ease of implementing into an existing requirements engineering process as well as the organizational workflow. There is a lack of evidence on what level of automation leads to the most effective requirements elicitation within an organization. More research, thus, needs to be done on whether it is possible and better to automate the entire elicitation process, or whether some extent of human-in-the-loop is necessary.
If a semi-automated approach is considered preferable, another issue that needs to be addressed is where and when in the elicitation process human should come into play to facilitate effective automated requirements elicitation. In addition, the characteristics of dynamic data can be changed over time. The proposed automated approach should be flexible enough to incorporate and reflect these dynamic changes over time.
Our results show that three-quarters of the selected studies exclusively focused on requirements elicitation, while only one-quarter supported additional requirements engineering activities, which were requirements prioritization and management of requirements change. Therefore, no studies supported the automation of the entire requirements engineering process. A holistic framework, therefore, needs to be developed to increase the automation level of dynamic data-driven requirements engineering.
Threats to Validity
The results of the review need to be interpreted with caution due to the following limitations.
External validity
All the studies included in the review, except one utilizing user feedback in both English and Italian [ 65 ], focus on eliciting English requirements. Thus, our results cannot be generalizable to requirements elicitation in other languages. Further studies are needed to assess the applicability of the techniques used for eliciting English requirements to other languages.
Internal validity
Our search query might have missed potentially important keywords such as “requirements mining”, “feedback”, and “tool”. Not including those keywords affects the number of studies included in the analysis. Our search query also failed to capture the work following DevOps and human–computer interaction approaches, which may have resulted in omitting some important work. We did not perform a backward and forward reference search for updating the review. The absence also may have reduced the number of studies included in this review.
In addition, a single reviewer performed a large part of study selection and data extraction, which may cause errors that impact the results. We partially mitigate the risk by ensuring high inter-rater reliability tested on a small proportion of randomly selected samples and discussing with at least one of the other reviewers to decide the inclusion of undecided papers, as explained in the “Study Selection” section. Ideally, the entire study selection and data extraction process should have been performed by at least two reviewers.
Another limitation is that we defined an analytical framework to synthesize retrieved data in advance. However, the analytical framework was based on the previous systematic review of the automated requirements elicitation from domain documents. Moreover, we assessed the quality of individual study solely based on the SJR or CORE scores. Those scores may not always reflect the “true” strength of evidence provided by each study. A more detailed and formal quality assessment could have added value to the review by increasing the reliability of the results.
Publication bias
This review included only published peer-reviewed studies and excluded gray literature and commercial products, which may fill many of the gaps identified in this review. Thus, the frequencies of the techniques and concepts do not imply real-life usage frequencies or degree of usefulness. Including gray literature and commercial products would increase the review’s completeness and timeliness.
Conclusions and Future Work
We have conducted a systematic literature review concerning requirements elicitation from data generated via digital technologies that are unintended with respect to requirements. These sources can include data that is highly relevant for new system requirements, which otherwise could not be obtained from other sources. The motivation behind the proposed approaches lies in the fact that by including such requirements, which existing or new software systems are not supporting, important improvements concerning system functionality and quality can be made, as well as ensuring that requirements are up-to-date and enabling further automation of a continuous elicitation process.
This literature review provides an overview of the state-of-the-art with respect to data-driven requirements elicitation from dynamic data sources. This is the first systematic review focusing on efforts to automate or support requirements elicitation from these types of data sources—often referred to as Big Data—that include not only human-sourced data but also process-mediated and machine-generated data.
We obtained 1848 relevant studies by searching six electronic databases. After two levels of screening, and a complementary forward and backward reference search, 51 papers were selected for data analysis. We further performed additional 2-level screening to update our search, which resulted in including 17 more studies. Thus, in total, 68 studies are included in the final analysis. Those selected studies were analyzed to answer the defined research questions concerning (a) identification of specific data sources and data types used for the elicitation, (b) methods and techniques used for processing the data, and (c) classification of the content of obtained outputs in relation to what is expected from the traditional elicitation process.
The results revealed remarkable insights, which, when summarized, have shown the current clear dominance of the human-sourced data, compared to the process-mediated and machine-generated data sources. As a result of that the techniques used for data processing are based on natural language processing, while the use of machine learning for classification and clustering is prevalent. The dominant intention of the proposed methods was to automate the elicitation process fully, rather than to combine it with traditional stakeholder-involved approaches.
Furthermore, the results showed that the majority of the studies were considering both functional and non-functional (i.e., quality) requirements. The final results regarding the completeness and the readiness of the elicited data for use in system development or evolution are currently limited—most of the studies obtain some of the information relevant for requirement’s content, some studies target the identification of the core functionality or quality in terms of features, and only a few of the studies achieve a high-level requirement content. Finally, the majority of the studies evaluated the results in experimental environments, thus indicating rather a low extent of implementation of the method in a real-life requirements engineering setting.
The obtained results provide several directions for future work. One possible direction concerns the investigation of more extensive use and analysis of non-human-sourced data types. In addition, automatic data fusion and contextualization methods need to be investigated for integrating, processing, and analyzing a large volume of heterogeneous data sources to elicit requirements. Semantic technologies can be a promising solution to address the variety and volume issues of Big Data. Other direction leads to enabling real-time data processing and analyzing to facilitate continuous requirements elicitation from Big Data with high velocity.
Moreover, each proposed solution needs to be evaluated against traditional requirements to convince practitioners for its implementation in real-life. Further improvements also need to be made in the content and quality of the elicited data in relation to fully detailed requirements. Finally, a very important direction relates to the proposals for enabling context-awareness to capture requirements that changes dynamically over time.
Pohl K. Requirements engineering: fundamentals, principles, and techniques. Heidelberg: Springer; 2010.
Book Google Scholar
Pacheco C, García I, Reyes M. Requirements elicitation techniques: a systematic literature review based on the maturity of the techniques. IET Softw. 2018;12(4):365–78. https://doi.org/10.1049/iet-sen.2017.0144 .
Article Google Scholar
Chen H, Chiang RHL, Storey VC. Business intelligence and analytics: from big data to big impact. MIS Quart. 2012;36(4):1165–88.
Manrique-Losada B, Zapata-Jaramillo CM, Burgos DA. Re-expressing business processes information from corporate documents into controlled language. In: International Conference on Applications of Natural Language Processing to Information Systems . Cham: Springer, 2016, pp. 376–383.
Hauksdóttir D, Ritsing B, Andersen JC, Mortensen NH. Establishing reusable requirements derived from laws and regulations for medical device development. In 2016 IEEE 24th International Requirements Engineering Conference Workshops (REW) , 2016, pp. 220–228, https://doi.org/10.1109/REW.2016.045 .
Kaiya H, Saeki M. Using domain ontology as domain knowledge for requirements elicitation. In 14th IEEE International Requirements Engineering Conference (RE'06) , pp. 189–198. IEEE, 2006, pp. 186–195, https://doi.org/10.1109/RE.2006.72 .
Zong-yong L, Zhi-xue W, Ying-ying Y, Yue W, Ying L. Towards a multiple ontology framework for requirements elicitation and reuse. In 31st Annual International Computer Software and Applications Conference (COMPSAC 2007) , vol. 1, pp. 189–195. IEEE, 2007, https://doi.org/10.1109/COMPSAC.2007.216 .
Nogueira FA, De Oliveira HC. Application of heuristics in business process models to support software requirements specification. ICEIS. 2017;2:40–51.
Google Scholar
Bendjenna H, Zarour NE, Charrel P. MAMIE: A methodology to elicit requirements in inter-company co-operative information systems. In 2008 International Conference on Computational Intelligence for Modelling Control & Automation , 2008, pp. 290–295, https://doi.org/10.1109/CIMCA.2008.101 .
Shao F, Peng R, Lai H, Wang B. DRank: a semi-automated requirements prioritization method based on preferences and dependencies. J Syst Softw. 2017;126:141–56. https://doi.org/10.1016/j.jss.2016.09.043 .
Abad ZSH, Karras O, Ghazi P, Glinz M, Ruhe G, Schneider K. “What works better? A study of classifying requirements. In 2017 IEEE 25th International Requirements Engineering Conference (RE) , pp. 496–501. IEEE, 2017, https://doi.org/10.1109/RE.2017.36 .
Hayes JH, Antoniol G, Adams B, Guehénéuc YG. Inherent characteristics of traceability artifacts: Less is more. In: 2015 IEEE 23rd International Requirements Engineering Conference (RE) , pp. 196–201. IEEE, 2015.
Kamalrudin M, Hosking J, Grundy J. MaramaAIC: tool support for consistency management and validation of requirements. Automat Softw Eng. 2017;24(1):1–45. https://doi.org/10.1007/s10515-016-0192-z .
Ahmed MA, Butt WH, Ahsan I, Anwar MW, Latif M, Azam F. A novel natural language processing (NLP) approach to automatically generate conceptual class model from initial software requirements. In International Conference on Information Science and Applications , pp. 476–484. Springer, Singapore, 2017.
Kifetew F, Munante D, Perini A, Susi A, Siena A, Busetta P. DMGame: A gamified collaborative requirements prioritisation tool. In: 2017 IEEE 25th International Requirements Engineering Conference (RE) , 2017, pp. 468–469, https://doi.org/10.1109/RE.2017.46 .
Ahmad S, Jalil IEA, Ahmad SSS. An enhancement of software requirements negotiation with rule-based reasoning: a conceptual model. J Telecommun Electron Comput Eng (JTEC). 2016;8(10):193–8.
Meth H, Brhel M, Maedche A. The state of the art in automated requirements elicitation. Inf Softw Technol. 2013;55(10):1695–709. https://doi.org/10.1016/j.infsof.2013.03.008 .
Nicolás J, Toval A. On the generation of requirements specifications from software engineering models: a systematic literature review. Inf Softw Technol. 2009;51(9):1291–307. https://doi.org/10.1016/j.infsof.2009.04.001 .
Groen EC, et al. The crowd in requirements engineering: the landscape and challenges. IEEE Softw. 2017;34(2):44–52. https://doi.org/10.1109/MS.2017.33 .
Ferguson M. Big data-why transaction data is mission critical to success. Intelligence Business Strategies Limited. https://public.dhe.ibm.com/common/ssi/ecm/im/en/iml14442usen/IML14442USEN.PDF , 2014.
Maalej W, Nayebi M, Johann T, Ruhe G. Toward data-driven requirements engineering. IEEE Softw. 2016;33(1):48–54. https://doi.org/10.1109/MS.2015.153 .
Genc-Nayebi N, Abran A. A systematic literature review: opinion mining studies from mobile app store user reviews. J Syst Softw. 2017;125:207–19. https://doi.org/10.1016/j.jss.2016.11.027 .
Ghasemi M, Amyot D. From event logs to goals: a systematic literature review of goal-oriented process mining. Requir Eng. 2019;25(1):67–93. https://doi.org/10.1007/s00766-018-00308-3 .
Zowghi D, Coulin C. Requirements elicitation: A survey of technique, approaches and tools. In: Engineering and managing software requirements. Berlin: Springer; 2005. p. 19–46.
Chapter Google Scholar
Arruda D, Madhavji NH. State of requirements engineering research in the context of Big Data applications. In International Working Conference on Requirements Engineering: Foundation for Software Quality . Cham: Springer, 2018, pp. 307–323.
Martin W, Sarro F, Jia Y, Zhang Y, Harman M. A survey of app store analysis for software engineering. IEEE Trans Software Eng. 2017;43(9):817–47. https://doi.org/10.1109/TSE.2016.2630689 .
Tavakoli M, Zhao L, Heydari A, Nenadić G. Extracting useful software development information from mobile application reviews: a survey of intelligent mining techniques and tools. Exp Syst Appl. 2018;113:186–99.
De Mauro A, Greco M, Grimaldi M. What is big data? A consensual definition and a review of key research topics. In: AIP conference proceedings , vol. 1644, no. 1, pp. 97–104. American Institute of Physics, 2015, https://doi.org/10.1063/1.4907823 .
Morales-Ramirez I, Perini A, Guizzardi RSS. An ontology of online user feedback in software engineering. Appl Ontol. 2015;10(3–4):297–330. https://doi.org/10.3233/AO-150150 .
Firmani D, Mecella M, Scannapieco M, Batini C. On the meaningfulness of ‘big data quality’ (invited paper). Data Sci Eng. 2016;1(1):6–20. https://doi.org/10.1007/s41019-015-0004-7 .
Kitchenham B, Charters S. Guidelines for performing systematic literature reviews in software engineering. 2007.
Landis JR, Koch GG. The measurement of observer agreement for categorical data. Biometrics. 1977;33(1):159–74. https://doi.org/10.2307/2529310 .
Article MATH Google Scholar
Rotondi MA, Donner A. A confidence interval approach to sample size estimation for interobserver agreement studies with multiple raters and outcomes. J Clin Epidemiol. 2012;65(7):778–84. https://doi.org/10.1016/j.jclinepi.2011.10.019 .
Hevner AR, March ST, Park J, Ram S. Design science in information systems research. MIS Quart. 2004;28(1):75–105. https://doi.org/10.2307/25148625 .
Dhinakaran VT, Pulle R, Ajmeri N, Murukannaiah PK. App review analysis via active learning: Reducing supervision effort without compromising classification accuracy. In 2018 IEEE 26th International Requirements Engineering Conference (RE) , pp. 170–181. IEEE, 2018, https://doi.org/10.1109/RE.2018.00026 .
Do QA, Bhowmik T. Automated generation of creative software requirements: a data-driven approach. In Proceedings of the 1st ACM SIGSOFT International Workshop on Automated Specification Inference , pp. 9–12. 2018, https://doi.org/10.1145/3278177.3278180 .
Groen EC, Kopczynska S, Hauer MP, Krafft TD, Doerr J. Users-the hidden software product quality experts?: A study on how app users report quality aspects in online reviews. In 2017 IEEE 25th International Requirements Engineering Conference (RE) , pp. 80–89. IEEE, 2017, https://doi.org/10.1109/RE.2017.73 .
Maalej W, Kurtanović Z, Nabil H, Stanik C. On the automatic classification of app reviews. Requir Eng. 2016;21(3):311–31. https://doi.org/10.1007/s00766-016-0251-9 .
Maalej W, Nabil H. Bug report, feature request, or simply praise? On automatically classifying app reviews. In 2015 IEEE 23rd international requirements engineering conference (RE) , pp. 116–125. IEEE, 2015, https://doi.org/10.1109/RE.2015.7320414 .
Guzman E, Maalej W. How do users like this feature? A fine grained sentiment analysis of app reviews. In 2014 IEEE 22nd international requirements engineering conference (RE) , pp. 153–162. IEEE, 2014, https://doi.org/10.1109/RE.2014.6912257 .
Jiang W, Ruan H, Zhang L, Lew P, Jiang J. For user-driven software evolution: Requirements elicitation derived from mining online reviews. In Pacific-Asia Conference on Knowledge Discovery and Data Mining , pp. 584–595. Springer, Cham, 2014.
Lu M, Liang P. Automatic classification of non-functional requirements from augmented app user reviews. In Proceedings of the 21st International Conference on Evaluation and Assessment in Software Engineering , 2017, pp. 344–353.
Deocadez R, Harrison R, Rodriguez D. Automatically classifying requirements from app stores: A preliminary study. In 2017 IEEE 25th International Requirements Engineering Conference Workshops (REW) , Sep. 2017, pp. 367–371, https://doi.org/10.1109/REW.2017.58 .
Abad ZSH, Sims SDV, Cheema A, Nasir MB, Harisinghani P. Learn more, pay less! Lessons learned from applying the wizard-of-oz technique for exploring mobile app requirements. In 2017 IEEE 25th International Requirements Engineering Conference Workshops (REW) , Sep. 2017, pp. 132–138, https://doi.org/10.1109/REW.2017.71 .
Panichella S, Di Sorbo A, Guzman E, Visaggio CA, Canfora G, Gall HC. How can I improve my app? Classifying user reviews for software maintenance and evolution. In 2015 IEEE International Conference on Software Maintenance and Evolution (ICSME) , pp. 281–290. IEEE, 2015, https://doi.org/10.1109/ICSM.2015.7332474 .
Dalpiaz F, Parente M. RE-SWOT: From user feedback to requirements via competitor analysis. In International Working Conference on Requirements Engineering: Foundation for Software Quality , pp. 55–70. Springer, Cham, 2019.
Zhao L, Zhao A. Sentiment analysis based requirement evolution prediction. Fut Internet. 2019;11(2):52.
Jha N, Mahmoud A. Using frame semantics for classifying and summarizing application store reviews. Empir Softw Eng. 2018;23(6):3734–67.
Luiz W, et al. A feature-oriented sentiment rating for mobile app reviews. In Proceedings of the 2018 World Wide Web Conference , pp. 1909–1918. 2018.
Jha N, Mahmoud A. MARC: a mobile application review classifier. In REFSQ Workshops . 2017.
Jha N, Mahmoud A. Mining user requirements from application store reviews using frame semantics. In International working conference on requirements engineering: Foundation for software quality , pp. 273–287. Springer, Cham, 2017.
Carreno LVG, Winbladh K. Analysis of user comments: an approach for software requirements evolution. In 2013 35th international conference on software engineering (ICSE) , pp. 582–591. IEEE, 2013, https://doi.org/10.1109/ICSE.2013.6606604 .
Sun D, Peng R. A scenario model aggregation approach for mobile app requirements evolution based on user comments. In: Requirements engineering in the big data era. Berlin: Springer; 2015. p. 75–91.
Higashi K, Nakagawa H, Tsuchiya T. Improvement of user review classification using keyword expansion. In: Proceedings of the International Conference on Software Engineering and Knowledge Engineering (SEKE) , pp. 125–124. 2018.
Bakiu E, Guzman E. Which feature is unusable? Detecting usability and user experience issues from user reviews. In 2017 IEEE 25th International Requirements Engineering Conference Workshops (REW) , pp. 182–187. IEEE, 2017, https://doi.org/10.1109/REW.2017.76 .
Srisopha K, Behnamghader P, Boehm B. Do users talk about the software in my product? Analyzing user reviews on IoT products. In the Proceedings of CIbSE XXI Ibero-American Conference on Software Engineering (CIbSE) , pp. 551–564, 2018.
Groen EC, Iese F, Schowalter J, Kopczynska S. Is there really a need for using NLP to elicit requirements? A benchmarking study to assess scalability of manual analysis. In REFSQ Workshops. 2018.
Jiang W, Ruan H, Zhang L. Analysis of economic impact of online reviews: an approach for market-driven requirements evolution. In: Zowghi D, Jin Z, editors. Requirements engineering communications in computer and information science, vol. 432. Berlin: Springer; 2014. p. 45–59. https://doi.org/10.1007/978-3-662-43610-3_4 .
Buchan J, Bano M, Zowghi D, Volabouth P. Semi-automated extraction of new requirements from online reviews for software product evolution. In 2018 25th Australasian Software Engineering Conference (ASWEC) , pp. 31–40. IEEE, 2018.
Zhang Z, Qi J, Zhu G. Mining customer requirement from helpful online reviews. 2014 Enterprise Systems Conference , pp. 249–254. IEEE, 2014, https://doi.org/10.1109/ES.2014.38 .
Bakar NH, Kasirun ZM, Salleh N, Jalab HA. Extracting features from online software reviews to aid requirements reuse. Appl Soft Comput J. 2016;49:1297–315. https://doi.org/10.1016/j.asoc.2016.07.048 .
Bakar NH, Kasirun ZM, Salleh N. Terms extractions: an approach for requirements reuse. In 2015 2nd International Conference on Information Science and Security (ICISS) , pp. 1–4. IEEE, 2015.–254. IEEE, 2015, https://doi.org/10.1109/ICISSEC.2015.7371034 .
Bakar NH, Kasirun ZM, Salleh N, Halim A. Extracting software features from online reviews to demonstrate requirements reuse in software engineering. In Proceedings of the International Conference on Computing & Informatics , pp. 184–190. 2017.
Williams G, Mahmoud A. Mining twitter feeds for software user requirements. In 2017 IEEE 25th International Requirements Engineering Conference (RE) , pp. 1–10. IEEE, 2017, https://doi.org/10.1109/RE.2017.14 .
Stanik C, Haering M, Maalej W. Classifying multilingual user feedback using traditional machine learning and deep learning. In 2019 IEEE 27th International Requirements Engineering Conference Workshops (REW) , pp. 220–226. IEEE, 2019.
Ali N, Hwang S, Hong J-E. Your opinions let us know: mining social network sites to evolve software product lines. KSII Trans Internet Inf Syst. 2019;13(8):4191–211. https://doi.org/10.3837/tiis.2019.08.021 .
Alwadain A, Alshargi M. Crowd-generated data mining for continuous requirements elicitation. Int J Adv Comput Sci Appl. 2019;10(9):45–50.
Guzman E, Ibrahim M, Glinz M. A little bird told me: Mining tweets for requirements and software evolution. In 2017 IEEE 25th International Requirements Engineering Conference (RE) , pp. 11–20. IEEE, 2017, https://doi.org/10.1109/RE.2017.88 .
Guzman E, Alkadhi R, Seyff N. An exploratory study of twitter messages about software applications. Requir Eng. 2017;22(3):387–412.
Guzman E, Alkadhi R, Seyff N. A needle in a haystack: What do twitter users say about software? In 2016 IEEE 24th International Requirements Engineering Conference (RE) , pp. 96–105. IEEE, 2016, https://doi.org/10.1109/RE.2016.67 .
Kuehl N. Needmining: towards analytical support for service design. In International Conference on Exploring Services Science , pp. 187–200. Springer, Cham, 2016.
Nguyen V, Svee EO, Zdravkovic J. A semi-automated method for capturing consumer preferences for system requirements. In IFIP Working Conference on The Practice of Enterprise Modeling , pp. 117–132. Springer, Cham, 2016.
Svee EO, Zdravkovic J. A model-based approach for capturing consumer preferences from crowdsources: the case of twitter. In 2016 IEEE Tenth International Conference on Research Challenges in Information Science (RCIS) , pp. 1–12. IEEE, 2016, https://doi.org/10.1109/RCIS.2016.7549323 .
Martens D, Maalej W. Extracting and analyzing context information in user-support conversations on twitter. In 2019 IEEE 27th International Requirements Engineering Conference (RE) , pp. 131–141. IEEE, 2019.
Han X, Li R, Li W, Ding G, Qin S. User requirements dynamic elicitation of complex products from social network service. In 2019 25th International Conference on Automation and Computing (ICAC) , pp. 1–6. IEEE, 2019. https://doi.org/10.23919/IConAC.2019.8895140 .
Vlas RE, Robinson WN. Two rule-based natural language strategies for requirements discovery and classification in open source software development projects. J Manag Inf Syst. 2012;28(4):11–38. https://doi.org/10.2753/MIS0742-1222280402 .
Xiao M, Yin G, Wang T, Yang C, Chen M. Requirement acquisition from social Q&A sites. In: Liu L, Aoyama M, editors. Requirements engineering in the big data era Communications in Computer and Information Science, vol. 558. Berlin: Springer; 2015.
Cleland-Huang J, Dumitru H, Duan C, Castro-Herrera C. Automated support for managing feature requests in open forums. Commun ACM. 2009;52(10):68–74. https://doi.org/10.1145/1562764.1562784 .
Morales-Ramirez I, Kifetew FM, Perini A. Analysis of online discussions in support of requirements discovery. In International Conference on Advanced Information Systems Engineering (CAiSE) , pp. 159–174. Springer, Cham, 2017, https://doi.org/10.1007/978-3-319-59536-8_11 .
Khan JA, Liu L, Wen L. Requirements knowledge acquisition from online user forums. IET Softw. 2020;14(3):242–53. https://doi.org/10.1049/iet-sen.2019.0262 .
Khan JA, Xie Y, Liu L, Wen L. Analysis of requirements-related arguments in user forums. In 2019 IEEE 27th International Requirements Engineering Conference (RE) , pp. 63–74. IEEE, 2019.
Khan JA. Mining requirements arguments from user forums. In 2019 IEEE 27th International Requirements Engineering Conference (RE) , pp. 440–445. IEEE, 2019.
Tizard J, Wang H, Yohannes L, Blincoe K. Can a conversation paint a picture? Mining Requirements in software forums. In 2019 IEEE 27th International Requirements Engineering Conference (RE) , pp. 17–27. IEEE, 2019.
Morales-Ramirez I, Kifetew FM, Perini A. Speech-acts based analysis for requirements discovery from online discussions. Inf Syst. 2018;86:94–112. https://doi.org/10.1016/j.is.2018.08.003 .
Merten T, Falis M, Hübner P, Quirchmayr T, Bürsner S, Paech B. Software feature request detection in issue tracking systems. In 2016 IEEE 24th International Requirements Engineering Conference (RE) , pp. 166–175. IEEE, 2016, https://doi.org/10.1109/RE.2016.8 .
Portugal RLQ, Do Prado Leite JCS, Almentero E. Time-constrained requirements elicitation: Reusing GitHub content. In 2015 IEEE Workshop on Just-In-Time Requirements Engineering (JITRE) , pp. 5–8. IEEE, 2015, https://doi.org/10.1109/JITRE.2015.7330171 .
Nyamawe AS, Liu H, Niu N, Umer Q, Niu Z. Automated recommendation of software refactorings based on feature requests. In 2019 IEEE 27th International Requirements Engineering Conference (RE) , pp. 187–198. IEEE, 2019.
Franch X, et al. Data-driven elicitation, assessment and documentation of quality requirements in agile software development. In International Conference on Advanced Information Systems Engineering , pp. 587–602. Springer, Cham, 2018.
Oriol M, et al. Data-driven and tool-supported elicitation of quality requirements in agile companies. Softw Qual J. 2020. https://doi.org/10.1007/s11219-020-09509-y .
Do QA, Chekuri SR, Bhowmik T. Automated support to capture creative requirements via requirements reuse. In International Conference on Software and Systems Reuse , pp. 47–63. Springer, Cham, 2019, https://doi.org/10.1007/978-3-030-22888-0_4 .
Kang Y, Li H, Lu C, Pu B. A transfer learning algorithm for automatic requirement model generation. J Intell Fuzzy Syst. 2019;36(2):1183–91. https://doi.org/10.3233/JIFS-169892 .
Wang C, Zhang F, Liang P, Daneva M, van Sinderen M. Can app changelogs improve requirements classification from app reviews?: An exploratory study. In Proceedings of the 12th ACM/IEEE International Symposium on Empirical Software Engineering and Measurement , pp. 1–4. 2018, https://doi.org/10.1145/3239235.3267428 .
Wang C, Wang T, Liang P, Daneva M, Van Sinderen M. Augmenting app reviews with app changelogs: An approach for app reviews classification. In Proceedings of the International Conference on Software Engineering and Knowledge Engineering (SEKE) , pp. 398–512. 2019, https://doi.org/10.18293/SEKE2019-176 .
Johann T, Stanik C, Maalej W. SAFE: A simple approach for feature extraction from app descriptions and app reviews. In 2017 IEEE 25th International Requirements Engineering Conference (RE) , pp. 21–30. IEEE, 2017, https://doi.org/10.1109/RE.2017.71 .
Voet H, Altenhof M, Ellerich M, Schmitt RH, Linke B. A framework for the capture and analysis of product usage data for continuous product improvement. J Manuf Sci Eng. 2019;141(2):021010.
Liang W, Qian W, Wu Y, Peng X, Zhao W. Mining context-aware user requirements from crowd contributed mobile data. In Proceedings of the 7th Asia-Pacific Symposium on Internetware , pp. 132–140. 2015, https://doi.org/10.1145/2875913.2875933 .
Xie H, Yang J, Chang CK, Liu L. A statistical analysis approach to predict user’s changing requirements for software service evolution. J Syst Softw. 2017;132:147–64. https://doi.org/10.1016/j.jss.2017.06.071 .
Yang J, Chang CK, Ming H. A situation-centric approach to identifying new user intentions using the mtl method. In 2017 IEEE 41st Annual Computer Software and Applications Conference (COMPSAC) , vol. 1, pp. 347–356. IEEE, 2017.
Wüest D, Fotrousi F, Fricker S. Combining monitoring and autonomous feedback requests to elicit actionable knowledge of system use. In International Working Conference on Requirements Engineering: Foundation for Software Quality , pp. 209–225. Springer, Cham, 2019.
Takahashi H, Nakagawa H, Tsuchiya T. Towards automatic requirements elicitation from feedback comments: Extracting requirements topics using LDA. In Proceedings of the International Conference on Software Engineering and Knowledge Engineering (SEKE) , pp. 489–494. 2015, https://doi.org/10.18293/SEKE2015-103 .
Dhinakaran VT, Pulle R, Ajmeri N, Murukannaiah PK. App review analysis via active learning. In 2018 IEEE 26th International Requirements Engineering Conference (RE) , pp. 170–181. IEEE, 2018.
Licorish SA. Exploring the prevalence and evolution of android concerns: a community viewpoint. JSW. 2016;11(9):848–69.
Tizard J, Wang H, Yohannes L, Blincoe K. Can a conversation paint a picture? Mining requirements in software forums. In 2019 IEEE 27th International Requirements Engineering Conference (RE) , pp. 17–27. IEEE, 2019, https://doi.org/10.1109/RE.2019.00014 .
Al Kilani N, Tailakh R, Hanani A. Automatic classification of apps reviews for requirement engineering: Exploring the customers need from healthcare applications. In 2019 6th International Conference on Social Networks Analysis, Management and Security (SNAMS) , pp. 541–548. IEEE, 2019, https://doi.org/10.1109/SNAMS.2019.8931820 .
Yan X, Guo J, Lan Y, Cheng X. A biterm topic model for short texts. In Proceedings of the 22nd international conference on World Wide Web , pp. 1445–1456. 2013.
Merten T, Falis M, Hübner P, Quirchmayr T, Bürsner S, Paech B. Software feature request detection in issue tracking systems. In 2016 IEEE 24th International Requirements Engineering Conference (RE) , pp. 166–175. IEEE, 2016.
Barnaghi P, Wang W, Henson C, Taylor K. Semantics for the internet of things: early progress and back to the future. Int J Semant Web Inf Syst (IJSWIS). 2012;8(1):1–21. https://doi.org/10.4018/jswis.2012010101 .
Wolpert DH. The lack of a priori distinctions between learning algorithms. Neural Comput. 1996;8(7):1341–90. https://doi.org/10.1162/neco.1996.8.7.1341 .
Johannesson P, Perjons E. An introduction to design science. Berlin: Springer; 2014.
Berry DM. Evaluation of tools for hairy requirements engineering and software engineering tasks. In 2017 IEEE 25th International Requirements Engineering Conference Workshops (REW) , pp. 284–291. IEEE, 2017.
Dimitroff G, Georgiev G, Toloşi L, Popov B. Efficient F measure maximization via weighted maximum likelihood. Mach Learn. 2015;98(3):435–54. https://doi.org/10.1007/s10994-014-5439-y .
Article MathSciNet MATH Google Scholar
Download references
Open Access funding provided by Stockholm University. This study was funded by the Department of Computer and System Sciences, Stockholm University.
Author information
Authors and affiliations.
Department of Computer and Systems Sciences, Stockholm University, DSV, PO Box 7003, 164 07, Kista, Stockholm, Sweden
Sachiko Lim, Aron Henriksson & Jelena Zdravkovic
You can also search for this author in PubMed Google Scholar
Corresponding author
Correspondence to Sachiko Lim .
Ethics declarations
Conflict of interest.
The authors declare that they have no conflict of interest.
Additional information
Publisher's note.
Springer Nature remains neutral with regard to jurisdictional claims in published maps and institutional affiliations.
Electronic supplementary material
Below is the link to the electronic supplementary material.
Supplementary file1 (PDF 127 KB)
See Table 9
J1: J. A. Khan, L. Liu, and L. Wen. “Requirements knowledge acquisition from online user forums.” IET Software , vol. 14, no. 3, pp. 242–253, https://doi.org/10.1049/iet-sen.2019.0262 .
J2: M. Oriol et al., “Data-driven and tool-supported elicitation of quality requirements in agile companies,” Software Quality Journal , pp. 1–33, 2020, https://doi.org/10.1007/s11219-020-09509-y .
J3: N. Ali, S. Hwang, and J.-E. Hong. “Your opinions let us know: Mining social network sites to evolve software product lines.” KSII Transactions on Internet and Information Systems , vol. 13, no. 8, pp. 4191–4211, 2019, https://doi.org/10.3837/tiis.2019.08.021 .
J4: A. Alwadain and M. Alshargi. “Crowd-generated data mining for continuous requirements elicitation.” International Journal of Advanced Computer Science and Applications , vol. 10, no. 9, pp. 45–50, 2019.
J5: Y. Kang, H. Li, C. Lu, and B. Pu. “A transfer learning algorithm for automatic requirement model generation.” Journal of Intelligent and Fuzzy Systems , vol. 36, no. 2, pp. 1183–1191, 2019, https://doi.org/10.3233/JIFS-169892 .
J6: H. Voet, M. Altenhof, M. Ellerich, R. H. Schmitt, and B. Linke. “A Framework for the capture and analysis of product usage data for continuous product improvement.” Journal of Manufacturing Science and Engineering, vol.141, no. 2, 2019.
J7: L. Zhao and A. Zhao. “Sentiment analysis based requirement evolution prediction.” Future Internet , vol. 11, no. 2, p. 52, 2019.
J8: N. Jha and A. Mahmoud. "Using frame semantics for classifying and summarizing application store reviews." Empirical Software Engineering 23, no. 6 (2018): 3734–3767.
J9: I. Morales-Ramirez, F. M. Kifetew, and A. Perini, “Speech-acts based analysis for requirements discovery from online discussions,” Information Systems , vol. 86, pp.94–112, 2018, https://doi.org/10.1016/j.is.2018.08.003 .
J10: E. Guzman, R. Alkadhi, and N. Seyff. “An exploratory study of Twitter messages about software applications.” Requirements Engineering , vol. 22, no. 3, pp. 387–412, 2017.
J11: H. Xie, J. Yang, C. K. Chang, and L. Liu. “A statistical analysis approach to predict user’s changing requirements for software service evolution.” Journal of Systems and Software , vol. 132, pp. 147–164, 2017, https://doi.org/10.1016/j.jss.2017.06.071 .
J12: N. H. Bakar, Z. M. Kasirun, N. Salleh, and H. A. Jalab. “Extracting features from online software reviews to aid requirements reuse.” Applied Soft Computing Journal , vol. 49, pp. 1297–1315, 2016, https://doi.org/10.1016/j.asoc.2016.07.048 .
J13: S. A. Licorish. “Exploring the prevalence and evolution of Android concerns: A community viewpoint.” JSW , vol. 11, no. 9, pp. 848–869, 2016.
J14: W. Maalej, Z. Kurtanović, H. Nabil, and C. Stanik. “On the automatic classification of app reviews.” Requirements Engineering , vol. 21, no. 3, pp. 311–331, 2016, https://doi.org/10.1007/s00766-016-0251-9 .
J15: R. E. Vlas and W. N. Robinson. “Two rule-based natural language strategies for requirements discovery and classification in open source software Development Projects.” Journal of Management Information Systems , vol. 28, no. 4, pp. 11–38,, https://doi.org/10.2753/MIS0742-1222280402 .
J16: J. Cleland-Huang, H. Dumitru, C. Duan, and C. Castro-Herrera. “Automated support for managing feature requests in open forums.” Communications of the ACM , vol. 52, no. 10, pp. 68–74, 2009, https://doi.org/10.1145/1562764.1562784 .
C1: F. Dalpiaz and M. Parente. “RE-SWOT: From user feedback to requirements via competitor analysis.” In International Working Conference on Requirements Engineering: Foundation for Software Quality , pp. 55–70. Springer, Cham, 2019.
C2: Q. A. Do, S. R. Chekuri, and T. Bhowmik. “Automated support to capture creative requirements via requirements reuse.” In International Conference on Software and Systems Reuse , pp. 47–63. Springer, Cham, 2019, https://doi.org/10.1007/978-3-030-22888-0_4 .
C3: X. Han, R. Li, W. Li, G. Ding, and S. Qin. “User requirements dynamic elicitation of complex products from social network service.” In 2019 25th International Conference on Automation and Computing (ICAC) , pp. 1–6. IEEE, 2019. https://doi.org/10.23919/IConAC.2019.8895140 .
C4: J. A. Khan. “Mining requirements arguments from user forums.” In 2019 IEEE 27th International Requirements Engineering Conference (RE) , pp. 440–445. IEEE, 2019.
C5: J. A. Khan, Y. Xie, L. Liu, and L. Wen. “Analysis of requirements-related arguments in user forums.” In 2019 IEEE 27th International Requirements Engineering Conference (RE) , pp. 63–74. IEEE, 2019.
C6: N. Al Kilani, R. Tailakh, and A. Hanani. “Automatic classification of apps reviews for requirement engineering: exploring the customers need from healthcare applications.” In 2019 Sixth International Conference on Social Networks Analysis, Management and Security (SNAMS) , pp. 541–548. IEEE, 2019, https://doi.org/10.1109/SNAMS.2019.8931820 .
C7: D. Martens and W. Maalej. “Extracting and analyzing context information in user-support conversations on twitter,” In 2019 IEEE 27th International Requirements Engineering Conference (RE) , pp. 131–141. IEEE, 2019.
C8: A. S. Nyamawe, H. Liu, N. Niu, Q. Umer, and Z. Niu. “Automated recommendation of software refactorings based on feature requests.” In 2019 IEEE 27th International Requirements Engineering Conference (RE) , pp. 187–198. IEEE, 2019.
C9: J. Tizard, H. Wang, L. Yohannes, and K. Blincoe. “Can a conversation paint a picture? Mining requirements in software forums.” In 2019 IEEE 27th International Requirements Engineering Conference (RE) , pp. 17–27. IEEE, 2019.
C10: C. Wang, T. Wang, P. Liang, M. Daneva, and M. Van Sinderen. "Augmenting app review with app changelogs: An approach for app review classification." In Proceedings of the International Conference on Software Engineering and Knowledge Engineering (SEKE) , pp. 398–512. 2019, https://doi.org/10.18293/SEKE2019-176 .
C11: D. Wüest, F. Fotrousi, and S. Fricker. “Combining monitoring and autonomous feedback requests to elicit actionable knowledge of system use.” In International Working Conference on Requirements Engineering: Foundation for Software Quality , pp. 209–225. Springer, Cham, 2019.
C12: J. Buchan, M. Bano, D. Zowghi, and P. Volabouth. “Semi-automated extraction of new requirements from online reviews for software product evolution.” In 2018 25th Australasian Software Engineering Conference (ASWEC) , pp. 31–40. IEEE, 2018.
C13: V. T. Dhinakaran, R. Pulle, N. Ajmeri, and P. K. Murukannaiah. "App review analysis via active learning: reducing supervision effort without compromising classification accuracy." In 2018 IEEE 26th International Requirements Engineering Conference (RE) , pp. 170–181. IEEE, 2018, https://doi.org/10.1109/RE.2018.00026 .
C14: X. Franch et al . . “Data-driven elicitation, assessment and documentation of quality requirements in agile software development.” In International Conference on Advanced Information Systems Engineering , pp. 587–602. Springer, Cham, 2018.
C15: E. C. Groen, F. Iese, J. Schowalter, and S. Kopczynska. “Is there really a need for using NLP to elicit requirements? A benchmarking study to assess scalability of manual Analysis.” In REFSQ Workshops . 2018.
C16: K. Higashi, H. Nakagawa, and T. Tsuchiya. “Improvement of user review classification using keyword expansion.” In Proceedings of the International Conference on Software Engineering and Knowledge Engineering (SEKE) , pp. 125–124. 2018.
C17: W. Luiz et al . . "A feature-oriented sentiment rating for mobile app reviews." In Proceedings of the 2018 World Wide Web Conference , pp. 1909–1918. 2018.
C18: K. Srisopha, P. Behnamghader, and B. Boehm. “Do users talk about the software in my product? Analyzing user reviews on IoT products.” In the Proceedings of CIbSE XXI Ibero-American Conference on Software Engineering (CIbSE) , pp.551–564, 2018
C19: N. H. Bakar, Z. M. Kasirun, N. Salleh, and A. Halim. “Extracting software features from online reviews to demonstrate requirements reuse in software engineering.” In Proceedings of the International Conference on Computing & Informatics , pp. 184–190. 2017.
C20: M. Lu and P. Liang. “Automatic classification of non-functional requirements from augmented app user reviews.” In Proceedings of the 21st International Conference on Evaluation and Assessment in Software Engineering , 2017, pp. 344–353.
C21: E. C. Groen, S. Kopczynska, M. P. Hauer, T. D. Krafft, and J. Doerr. "Users—the hidden software product quality experts?: A study on how app users report quality aspects in online reviews." In 2017 IEEE 25th International Requirements Engineering Conference (RE) , pp. 80–89. IEEE, 2017, https://doi.org/10.1109/RE.2017.73 .
C22: E. Guzman, M. Ibrahim, and M. Glinz. “A little bird told me: Mining tweets for requirements and software evolution.” In 2017 IEEE 25th International Requirements Engineering Conference (RE) , pp. 11–20. IEEE, 2017, https://doi.org/10.1109/RE.2017.88 .
C23: N. Jha and A. Mahmoud. "Mining user requirements from application store reviews using frame semantics." In International working conference on requirements engineering: Foundation for software quality , pp. 273–287. Springer, Cham, 2017.
C24: I. Morales-Ramirez, F. M. Kifetew, and A. Perini. “Analysis of online discussions in support of requirements discovery.” In International Conference on Advanced Information Systems Engineering (CAiSE) , pp. 159–174. Springer, Cham, 2017, https://doi.org/10.1007/978-3-319-59536-8_11 .
C25: G. Williams and A. Mahmoud. “Mining twitter feeds for software user requirements.” In 2017 IEEE 25th International Requirements Engineering Conference (RE) , pp. 1–10. IEEE, 2017, https://doi.org/10.1109/RE.2017.14 .
C26: T. Johann, C. Stanik, A. M. A. B, and W. Maalej. “SAFE: A simple approach for feature extraction from app descriptions and app reviews.” In 2017 IEEE 25th International Requirements Engineering Conference (RE) , pp. 21–30. IEEE, 2017, https://doi.org/10.1109/RE.2017.71 .
C27: J. Yang, C. K. Chang, and H. Ming. “A situation-centric approach to identifying new user intentions using the mtl method.” In 2017 IEEE 41st Annual Computer Software and Applications Conference (COMPSAC) , vol. 1, pp. 347–356. IEEE, 2017.
C28: E. Guzman, R. Alkadhi, and N. Seyff. “A needle in a haystack: What do twitter users say about software?,” In 2016 IEEE 24th International Requirements Engineering Conference (RE) , pp. 96–105. IEEE, 2016, https://doi.org/10.1109/RE.2016.67 .
C29: N. Kuehl. "Needmining: Towards analytical support for service design." In International Conference on Exploring Services Science , pp. 187–200. Springer, Cham, 2016.
C30: T. Merten, M. Falis, P. Hübner, T. Quirchmayr, S. Bürsner, and B. Paech. “Software feature request detection in issue tracking systems.” In 2016 IEEE 24th International Requirements Engineering Conference (RE) , pp. 166–175. IEEE, 2016,, https://doi.org/10.1109/RE.2016.8 .
C31: V. Nguyen, E. Svee, and J. Zdravkovic. "A semi-automated method for capturing consumer preferences for system requirements." In IFIP Working Conference on The Practice of Enterprise Modeling , pp. 117–132. Springer, Cham, 2016.
C32: E. Svee and J. Zdravkovic. "A model-based approach for capturing consumer preferences from crowdsources: the case of Twitter." In 2016 IEEE Tenth International Conference on Research Challenges in Information Science (RCIS) , pp. 1–12. IEEE, 2016, https://doi.org/10.1109/RCIS.2016.7549323 .
C33: N. H. Bakar, Z. M. Kasirun, and N. Salleh. “Terms extractions: An approach for requirements reuse.” In 2015 2nd International Conference on Information Science and Security (ICISS) , pp. 1–4. IEEE, 2015.–254. IEEE, 2015, https://doi.org/10.1109/ICISSEC.2015.7371034 .
C34: W. Maalej and H. Nabil. "Bug report, feature request, or simply praise? on automatically classifying app reviews." In 2015 IEEE 23rd international requirements engineering conference (RE) , pp. 116–125. IEEE, 2015, https://doi.org/10.1109/RE.2015.7320414 .
C35: S. Panichella, A. Di Sorbo, E. Guzman, C. A. Visaggio, G. Canfora, and H. C. Gall. "How can I improve my app? Classifying user reviews for software maintenance and evolution." In 2015 IEEE International Conference on Software Maintenance and Evolution (ICSME) , pp. 281–290. IEEE, 2015, https://doi.org/10.1109/ICSM.2015.7332474 .
C36: H. Takahashi, H. Nakagawa, and T. Tsuchiya, “Towards automatic requirements elicitation from feedback comments: Extracting requirements topics using LDA,” In Proceedings of the International Conference on Software Engineering and Knowledge Engineering (SEKE) , pp. 489–494. 2015, https://doi.org/10.18293/SEKE2015-103 .
C37: D. Sun and R. Peng. “A scenario model aggregation approach for mobile app requirements evolution based on user comments.” In Requirements Engineering in the Big Data Era , pp. 75–91. Springer, Berlin, Heidelberg, 2015.
C38: E. Guzman and W. Maalej. “How do users like this feature? A fine grained sentiment analysis of app reviews.” In 2014 IEEE 22nd international requirements engineering conference (RE) , pp. 153–162. IEEE, 2014, https://doi.org/10.1109/RE.2014.6912257 .
C39: W. Jiang, H. Ruan, L. Zhang, P. Lew, and J. Jiang. “For user-driven software evolution: requirements elicitation derived from mining online reviews.” In Pacific-Asia Conference on Knowledge Discovery and Data Mining , pp. 584–595. Springer, Cham, 2014.
C40: Z. Zhang, J. Qi, and G. Zhu. “Mining customer requirement from helpful online reviews,” In 2014 Enterprise Systems Conference , pp. 249–254. IEEE, 2014, https://doi.org/10.1109/ES.2014.38 .
C41: L. V. G. Carreno and K. Winbladh. “Analysis of user comments: An approach for software requirements evolution” In 2013 35th international conference on software engineering (ICSE) , pp. 582–591. IEEE, 2013, https://doi.org/10.1109/ICSE.2013.6606604 .
W1: C. Stanik, M. Haering, and W. Maalej. “Classifying multilingual user feedback using traditional machine learning and deep learning.” In 2019 IEEE 27th International Requirements Engineering Conference Workshops (REW) , pp. 220–226. IEEE, 2019.
W2: Q. A. Do and T. Bhowmik. "Automated generation of creative software requirements: a data-driven approach." In Proceedings of the 1st ACM SIGSOFT International Workshop on Automated Specification Inference , pp. 9–12. 2018, https://doi.org/10.1145/3278177.3278180 .
W3: Z. S. H. Abad, S. D. V. Sims, A. Cheema, M. B. Nasir, and P. Harisinghani. “Learn more, pay less! Lessons learned from applying the wizard-of-oz technique for exploring mobile app requirements.” In 2017 IEEE 25th International Requirements Engineering Conference Workshops (REW) , Sep. 2017, pp. 132–138, https://doi.org/10.1109/REW.2017.71 .
W4: E. Bakiu and E. Guzman. “Which feature is unusable? Detecting usability and user experience issues from user reviews.” In 2017 IEEE 25th International Requirements Engineering Conference Workshops (REW) , pp. 182–187. IEEE, 2017, https://doi.org/10.1109/REW.2017.76 .
W5: R. Deocadez, R. Harrison, and D. Rodriguez. “Automatically classifying requirements from app stores: A preliminary study.” In 2017 IEEE 25th International Requirements Engineering Conference Workshops (REW) , Sep. 2017, pp. 367–371, https://doi.org/10.1109/REW.2017.58 .
W6: N. Jha and A. Mahmoud. “MARC: A mobile application review classifier.” In REFSQ Workshops . 2017.
W7: R. L. Q. Portugal, J. C. S. Do Prado Leite, and E. Almentero. “Time-constrained requirements elicitation: Reusing GitHub content.” In 2015 IEEE Workshop on Just-In-Time Requirements Engineering (JITRE) , pp. 5–8. IEEE, 2015, https://doi.org/10.1109/JITRE.2015.7330171 .
S1: C. Wang, F. Zhang, P. Liang, M. Daneva, and M. van Sinderen. “Can app changelogs improve requirements classification from app reviews?: An exploratory study.” In Proceedings of the 12th ACM/IEEE International Symposium on Empirical Software Engineering and Measurement , pp. 1–4. 2018, https://doi.org/10.1145/3239235.3267428 .
S2: W. Liang, W. Qian, Y. Wu, X. Peng, and W. Zhao. “Mining context-aware user requirements from crowd contributed mobile data.” In Proceedings of the 7th Asia–Pacific Symposium on Internetware , pp. 132–140. 2015, https://doi.org/10.1145/2875913.2875933 .
S3: M. Xiao, G. Yin, T. Wang, C. Yang, and M. Chen. “Requirement acquisition from social Q&A sites.” In Liu L., Aoyama M. (eds) Requirements Engineering in the Big Data Era. Communications in Computer and Information Science , vol 558. Springer, Berlin, Heidelberg, 2015.
S4: W. Jiang, H. Ruan, and L. Zhang. “Analysis of economic impact of online reviews: An approach for market-driven requirements evolution.” In Zowghi D., Jin Z. (eds) Requirements Engineering. Communications in Computer and Information Science, vol. 432, pp. 45–59, 2014, Springer, Berlin, Heidelberg, https://doi.org/10.1007/978-3-662-43610-3_4 .
See Table 10
Rights and permissions
Open Access This article is licensed under a Creative Commons Attribution 4.0 International License, which permits use, sharing, adaptation, distribution and reproduction in any medium or format, as long as you give appropriate credit to the original author(s) and the source, provide a link to the Creative Commons licence, and indicate if changes were made. The images or other third party material in this article are included in the article's Creative Commons licence, unless indicated otherwise in a credit line to the material. If material is not included in the article's Creative Commons licence and your intended use is not permitted by statutory regulation or exceeds the permitted use, you will need to obtain permission directly from the copyright holder. To view a copy of this licence, visit http://creativecommons.org/licenses/by/4.0/ .
Reprints and permissions
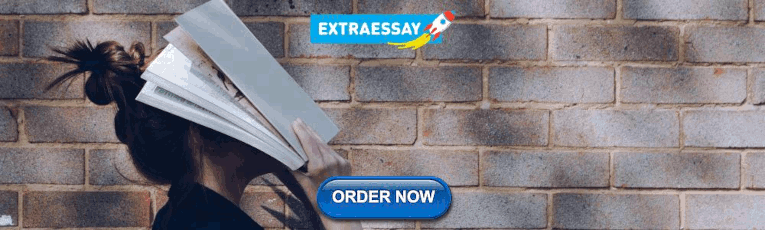
About this article
Lim, S., Henriksson, A. & Zdravkovic, J. Data-Driven Requirements Elicitation: A Systematic Literature Review. SN COMPUT. SCI. 2 , 16 (2021). https://doi.org/10.1007/s42979-020-00416-4
Download citation
Received : 25 March 2020
Accepted : 02 December 2020
Published : 04 January 2021
DOI : https://doi.org/10.1007/s42979-020-00416-4
Share this article
Anyone you share the following link with will be able to read this content:
Sorry, a shareable link is not currently available for this article.
Provided by the Springer Nature SharedIt content-sharing initiative
- Requirements engineering
- Requirements elicitation
- Find a journal
- Publish with us
- Track your research
Requirements Elicitation for Collaborative Systems: A Systematic Review
Ieee account.
- Change Username/Password
- Update Address
Purchase Details
- Payment Options
- Order History
- View Purchased Documents
Profile Information
- Communications Preferences
- Profession and Education
- Technical Interests
- US & Canada: +1 800 678 4333
- Worldwide: +1 732 981 0060
- Contact & Support
- About IEEE Xplore
- Accessibility
- Terms of Use
- Nondiscrimination Policy
- Privacy & Opting Out of Cookies
A not-for-profit organization, IEEE is the world's largest technical professional organization dedicated to advancing technology for the benefit of humanity. © Copyright 2024 IEEE - All rights reserved. Use of this web site signifies your agreement to the terms and conditions.
An official website of the United States government
Official websites use .gov A .gov website belongs to an official government organization in the United States.
Secure .gov websites use HTTPS A lock ( Lock Locked padlock icon ) or https:// means you've safely connected to the .gov website. Share sensitive information only on official, secure websites.
- Publications
- Account settings
- Advanced Search
- Journal List

Eliciting and modeling emotional requirements: a systematic mapping review
Mashail n alkhomsan, malak baslyman, mohammad alshayeb.
- Author information
- Article notes
- Copyright and License information
Corresponding author.
Received 2023 Apr 11; Accepted 2023 Dec 6; Collection date 2024.
This is an open access article distributed under the terms of the Creative Commons Attribution License , which permits unrestricted use, distribution, reproduction and adaptation in any medium and for any purpose provided that it is properly attributed. For attribution, the original author(s), title, publication source (PeerJ Computer Science) and either DOI or URL of the article must be cited.
Considering users’ emotions plays an extremely crucial role in the adoption and acceptance of recent technology by the end user. User emotions can also help to identify unknown requirements, saving resources that would otherwise be wasted if discovered later. However, eliciting and modeling users’ emotional requirements in software engineering is still an open research area.
This systematic mapping review analyzes emotional requirements (ER) practices in software engineering from two perspectives: elicitation and modeling. For elicitation techniques, we investigate the techniques, evaluation methods, limitations, and application domains. For modeling techniques, we examine the modeling languages, analyses, limitations, and domains.
We systematically reviewed studies on emotional requirements engineering published between 1993–2023 and identified 46 relevant primary studies.
A total of 34 studies investigated ER elicitation techniques, five examined modeling techniques, and seven covered both. Illustrative case studies were the main evaluation method for proposed elicitation techniques. Identified limitations include time consumption and extensive human involvement. The dominant application domains were healthcare and well-being, and game development.
This review summarizes the current landscape of emotional requirements research, highlighting key elicitation and modeling techniques, evaluations, limitations, and domains. Further research can build on these findings to advance emotional requirements practices in software engineering. Future research may address (1) managing conflicting emotional requirements across users, (2) evaluating the value and impact of considering emotional requirements during the development and (3) Modeling and analyzing emotional requirements in relation to other requirements.
Keywords: Emotional requirements, Elicitation, Modeling, Users’ emotions
Introduction
The increasingly competitive software industry requires rapid adaptation to evolving market needs. To develop products that customers love and adopt, providers must deliver exceptional user experiences (UX). This requires engineers to prioritize UX early in development through effective requirements engineering (RE). RE encompasses determining user needs and defining product functionality and quality. For superior UX, RE should go beyond basic requirements to capture human factors like emotions and values that attract and satisfy users. Emotions are the main dimension that defines UX, and it plays a significant role in the acceptance and adoption of innovative technology by end users ( Law et al., 2008 ; Levy, 2020 ). Considering them reveals latent needs ( Lopez-Lorca et al., 2014 ) and prevents wasted resources after release. By incorporating emotions and values, RE can shape products into rewarding long-term user experiences. Though complex, the sensitive and systematic understanding of human dynamics builds connections between users and technology. This deeper knowledge encourages innovation and competitive advantage.
Recent research papers have examined the motivations for integrating emotions into requirements engineering. By determining the emotional experiences users wish to elicit or avoid, designers can anticipate human emotional reactions and mitigate unintended negative effects through specific design choices. This approach benefits persuasive technologies aimed at influencing behavior change, such as e-health interventions seeking to motivate healthier lifestyle habits ( Fogg, 2002 ). The widespread adoption of social software provides further rationale for incorporating human emotion into requirements engineering (RE) processes. Designing social applications that foster active engagement and feelings of belonging necessitates understanding social emotions, such as empathy, to build meaningful connections between users ( Sutcliffe, 2011 ; Mooses et al. , 2022 ). However, the elicitation of users’ emotional requirements remains an underexplored area demanding greater focus within software engineering research and practice.
This research seeks to identify, assess, and synthesize existing literature on emotional RE elicitation and modeling following guidelines established by Kitchenham & Brereton (2013) . This research study aims to address the following research questions:
What elicitation techniques have been proposed for eliciting emotional requirements?
What are the methods and approaches used to validate the techniques’ effectiveness in collecting emotional requirements?
What are the benefits and limitations of emotional requirements eliciting/modeling techniques identified in the literature?
What are the emotional requirements modeling languages and representation formats that have been investigated in the studies?
What are the application domains for which emotional requirements have been elicited and represented?
Emotional requirements engineering is an emerging area within human-centered design and software development. Although emotions powerfully influence how people perceive, choose, and interact with technology, requirements engineering methodologies typically focus on more rational and functional needs while overlooking emotional ones. While research has examined emotions in requirements engineering broadly, there remains a need to specifically synthesize findings on emotional requirements elicitation and modeling techniques. This represents an important gap, as a focused understanding of existing emotional requirements elicitation and modeling approaches is crucial to mapping the landscape and enabling continued progress. Furthermore, this review aspires to understand how emotions are defined and operationalized, which is particularly crucial for examining an interdisciplinary topic like emotions in requirements engineering, where conceptual clarity is needed to integrate perspectives from affective science, psychology, and software engineering. This review provides a targeted investigation of the current state of research and open questions about emotional requirements elicitation and modeling. The results will benefit researchers and practitioners in human–computer interaction, user experience design, software engineering, and related fields. Practitioners may improve their understanding of emotional requirements and their ability to elicit and model them. Researchers can gain a comprehensive view of techniques and outstanding needs to guide future emotional requirements research directions.
Related Work
Emotional requirements engineering is an emerging area gaining increasing focus. The role of users’ emotions is being explored for several reasons within software engineering research and practice. While numerous systematic reviews have examined requirements elicitation and modeling methods broadly, few have centered specifically on the emotions dimension. This systematic mapping review examines applications, challenges, techniques, and outcomes surrounding emotional requirements elicitation and modeling. The following subsections highlight several systematic reviews related to the process of requirements elicitation and requirements modeling.
Requirements elicitation techniques
The requirements elicitation process is the first and most significant step in the requirements engineering process. Having the elicitation conducted incorrectly will lead to low-quality products, delayed deliveries, or high expenses. Wong, Mauricio & Rodriguez (2017) applied the systematic literature review (SLR) to gather and evaluate the available aspects that have been covered by the different requirements elicitation approaches. These aspects are classified to cover type of contribution, level of automation, knowledge reuse, importance of human factors, collaborative approach, and types of projects. The authors also identified the activities of the requirements elicitation process as covered by the different approaches, such as identify requirements, document, and refine, and identifying factors influencing the requirements elicitation process positively or negatively, such as different stakeholders’ perspectives and the project complexity.
Pacheco, García & Reyes (2018) conducted a systematic review of the set of validated elicitation techniques used between 1993 and 2015 to investigate effective elicitation techniques used at that time. It describes which elicitation techniques are effective and in what circumstances they are most effective. Among other factors, the results were determined by the type of product to be developed, stakeholder characteristics, and the type of collected information.
Iqbal et al. (2023a) conducted a systematic mapping focusing specifically on categorizing and analyzing the state-of-the-art research on emotions in RE, providing an overview of the field. Also, it elucidates how emotions are elicited and represented across different RE phases. However, the scope was broad, and additional reviews building a focused understanding of emotional requirements elicitation or modeling techniques alone could strengthen practice.
Requirements modeling techniques
Yang et al. (2014) systematically investigated the research literature on requirements modeling and analysis for self-adaptive systems. The authors summarized the state-of-the-art research trends and categorized the modeling methods used and relevant RE activities. A total of 16 modeling methods were identified to be used in 11 RE activities. They also categorized the quality attributes related to self-adaptive systems and application domains that can assist researchers and practitioners in choosing appropriate demonstrations and designing reasonable experiments.
These studies examined elicitation techniques and modeling languages with functional and non-functional requirements, as seen in Table 1 . However, unlike prior reviews that either focus on general requirements elicitation techniques or provide a broad overview of emotions in requirements engineering, our systematic mapping study offers an in-depth, focused synthesis specifically targeting practices and research on eliciting and modeling emotional requirements. Therefore, we were motivated to conduct this study as emotions have a different and complex nature, and elicitation techniques and modeling would be different from those used for other types of requirements.
Table 1. Comparative analysis of related work.
Methodology.
This section describes the methodology for conducting the systematic mapping review. It reports the research questions, search strategy, quality assessment approach, data extraction process, and synthesis techniques to be utilized.
Research questions
Based on study objectives, five research questions (RQs) grouped under two categories were developed: (1) Requirements elicitation techniques and (2) emotional requirements modeling. Table 2 presents the research questions and their motivation.
Table 2. Systematic mapping questions.
Search strategy.
The search strategy phase involves identifying search terms, selecting resources, and explaining the search process for retrieving primary studies.
Search string
Search terms were formed by decomposing the research questions into main keywords: emotion, requirements, and engineering. Alternative spellings and synonyms were examined for each keyword. The resulting search string is presented in Table 3 .
Table 3. Search string.
Selected databases.
Four electronic search engines from the most relevant software engineering sources were selected to search for the papers: ACM Digital Library, IEEE-Xplore, Scopus, and ISI Web of Knowledge. We selected those four databases because they are recognized as the most relevant resources in software engineering, including requirements engineering, user experience, and human factors. Table 4 depicts the databases and retrieval studies after using the search string in the four electronic databases.
Table 4. Database retrieval results.
Search process.
The search process was executed in three phases, as depicted in Fig. 1 :
Figure 1. Search process.
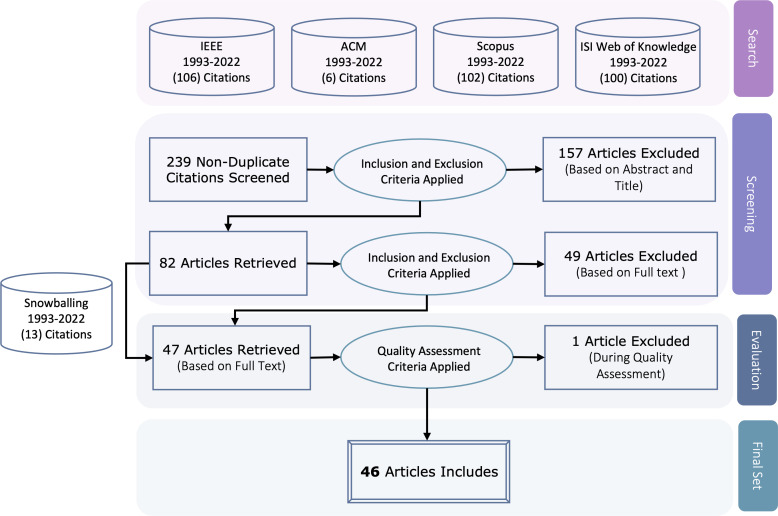
Search phase. Search the selected electronic databases to find relevant studies using the search string based on the abstract and title.
Screening phase. During the screening phase, 239 non-duplicated studies were assessed for eligibility. To identify duplicate studies, automated detection in Excel was used with manual review. The retrieved studies underwent two rounds of screening. In the first round, titles and abstracts were examined to determine whether studies fulfilled the inclusion and exclusion criteria, yielding a relevant subset for the review. The second round entailed a full-text assessment of each study to ascertain eligibility as per the inclusion and exclusion criteria, thus generating the final subset of relevant studies for the review.
Evaluation phase. The included studies will be evaluated using quality assessment criteria.
Final phase. The studies that pass the quality assessment will be the primary studies. In addition, references to the primary studies will be tracked to find more relevant ones. The tracked studies will be examined against inclusion and exclusion criteria and then assessed using quality criteria.
This multi-stage approach aimed for a broad initial search followed by progressive filtering to obtain primary studies most directly and thoroughly addressing the topics of interest. The search and screening phases cast a wide net to capture all potentially useful literature. The evaluation and final phases then refined results by systematically assessing relevance and quality, retaining only the most significant contributions meeting standards for empirical research and depth of insight. This process seeks to balance an exhaustive search with a filtered result set focused on the available evidence at the highest level of rigor and relevance.
Selection criteria
By applying well-defined selection criteria, the most relevant and rigorous literature can be identified from an initial broad search. The inclusion and exclusion criteria serve as a mechanism for systematically filtering a large result set down to a focused subset of primary sources. Papers satisfying all inclusion criteria were included, and papers meeting any exclusion criteria, presented in Table 5 , were excluded. Database searches were restricted to papers published in the date range of 1993–2023. This excluded any papers published outside this date range. The primary focus is on software engineering with an emphasis on emotional requirements. Also, during the full-text review, papers were assessed to confirm they addressed eliciting or modeling emotional requirements. For example, a paper may have proposed a new requirements elicitation technique and briefly noted it could be useful for emotional requirements but did not provide in-depth discussion.
Table 5. Selection criteria.
Quality assessment.
The quality of the included studies was evaluated based on five criteria, shown in Table 6 . Each criterion was scored on a scale of 0 to 1, with studies fully meeting the criterion receiving a score of 1, studies partially meeting the criterion receiving a 0.5, and studies not meeting the criterion receiving a 0. The criteria focus on the quality and rigor of the study design, methodology, validity of results, and adequacy of reporting. A threshold score of ≥ 2.5 was used to identify high-quality primary studies for inclusion. This threshold ensures only studies that sufficiently meet the majority of quality criteria are used for data analysis and synthesis.
Table 6. Quality assessment criteria.
Data extraction.
A data extraction form was tailored to fulfill the identified RQs and will be filled out for each included study. This form ensures that all relevant information will be obtained from each study, which makes it possible to analyze and compare the results. Data will be extracted into three categories: research areas and directions, requirements elicitation techniques, and emotional requirements modeling. As a first step, we will collect metadata for each paper, including the title, authors, publication year, type of publication, and venue for statistical investigation. Additionally, we will extract the main gap of each paper and the future directions (if any), so we can analyze the gaps and future directions. Afterward, we will extract the data to answer questions related to the emotion requirements elicitation techniques, including the approach to proposing the technique, the validation method, the limitations and benefits, and the application domains. Finally, we will identify the emotional requirements modeling languages, contributions, limitations, and application domains if they are applied in a specific context. The extracted data will be organized using an Excel sheet, as seen in Table 7 , for further data synthesis.
Table 7. Data extraction sheet contents.
Data synthesis strategy.
Extracted data from the retrieved studies will be synthesized to extract outcomes using the narrative synthesis method ( Popay et al., 2006 ). The data will be organized into three main themes. The first theme is to address emotional requirements elicitation techniques, validation methods, benefits and limitations, and application domains. The second theme is to investigate the emotional requirements modeling languages, their overlaps and contributions, and limitations and application domains. The third theme includes research areas, research gaps, and future directions from the literature. Finally, we will determine how best to organize and visualize the data ( i.e., figures/tables) based on the findings at the review stage.
Study validation
A pilot run of the data extraction process and templates was conducted on five papers by one author to validate the study methods. This resulted in modifications to the templates and the development of guidelines for defining keywords likely to arise in selected papers. These steps improved consistency in coding and data capture. Additionally, two authors reviewed the retrieved studies, primary source selection, and data extraction. This validation helped resolve any disagreements, ensuring consensus and reliability in the systematic search and review process.
The study includes 46 primary studies, as indicated in Fig. 1 , which represents the number of studies examined during various phases of the mapping study. The list of the 46 primary studies is represented in the Appendix. The distribution of these papers across the venue types indicates that the emotional requirements area has grown considerably. As seen in Fig. 2 , most primary studies (21 out of 46) are published in conference proceedings. As for the publications over the years, Fig. 3 gives an overview of the number of papers published in Emotional Requirement Engineering over the years. The number of publications in Emotional requirements is at its peak in 2019–2023. Analyzing recent published studies’ motives can help in explaining the reasons why emotional requirements have gained significant attention lately. Most of the studies published between 2019–2023 aimed to increase user acceptance and trust towards new IT solutions, such as smart home systems for the elderly ( Curumsing et al., 2019 ).
Figure 2. Distribution of papers by venue types.
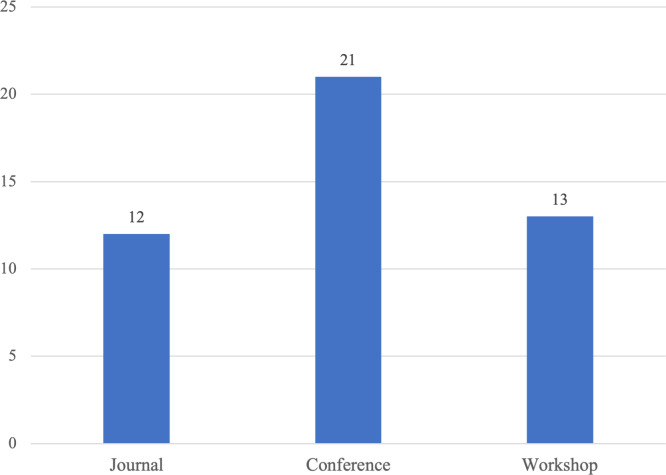
Figure 3. Publication trends over the year.
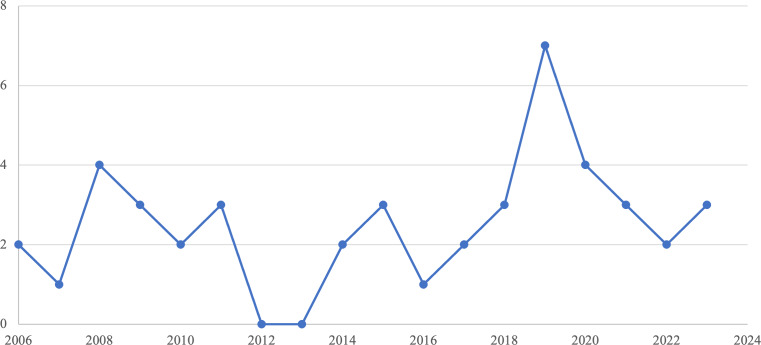
Emotions definitions in requirements engineering
Understanding how emotions are defined and operationalized is particularly crucial for examining an interdisciplinary topic like emotions in requirements engineering, where conceptual clarity is needed to integrate perspectives from affective science, psychology, and software engineering. To analyze how emotions have been characterized and incorporated across the selected studies, we have summarized the definitions of emotion mentioned in the 46 studies. Table 8 categorizes definitions and lists supporting studies. Although some definitions were adopted more frequently than others, these frequencies should not be considered indicative, as many of the studies were conducted by overlapping research groups.
Table 8. Emotions definitions groups.
• Group 1: Emotional valence dimension
The valence dimension categorizes emotions as positive or negative ( Izard, 1991 ). Studies ( Sutcliffe, 2011 ; Jiang & Li, 2020 ; Thew & Sutcliffe, 2018 ; Abdullah et al. , 2020 ; Yeewai et al., 0000 ; Stade et al., 2019 ; Levy, 2020 ; Zhou, Jianxin Jiao & Linsey, 2015 ; Mulvenna et al., 2017 ; Levy, 2020 ; Maier & Berry, 2017 ; Sutcliffe, 2012 ; Cheng et al., 2023 ; Bolchini, Garzotto & Paolini, 2007 ; Thew & Sutcliffe, 2008 ; Curumsing et al., 2015 ; Yu et al., 2021 ) underscore considering emotion valence in requirements engineering, product design, and user experience. Analyzing valence provides insight into the potential impact of emotions on users.
• Group 2: Valence-arousal model
The valence-arousal model classifies emotions based on their positive/negative valence and high/low arousal ( Sutcliffe, 2011 ; Russell, 1989 ). Study ( Alkhomsan, Baslyman & Alshayeb, 2022 ) adopts this approach, discussing how software interactions can evoke emotions with different valence-arousal combinations. While universally defining emotional requirements is challenging, ( Alkhomsan, Baslyman & Alshayeb, 2022 ) attempts to formally define “emotional requirements” using class diagrams. The proposed metamodel captures the key components of emotional requirements to represent user needs meaningfully. It consists of three main classes: User, Emotion, and Condition. The metamodel associates emotional requirements with other requirement types like functional and non-functional. This allows extending any requirement to include emotional aspects.
• Group 3: Appraisal theory
Appraisal theory is a psychological theory ( Roseman & Smith, 2001 ) proposing that emotional responses are shaped not just by situations themselves but by how individuals interpret and understand those situations. The study that explicitly adopted this theory is ( Sherkat et al., 2018 ), defining emotions as intuitive reactions towards a product or service based on appraising its ability to meet personal concerns or goals. This cognitive interpretation can lead to positive or negative emotional responses. While not mentioned explicitly, studies ( Callele, Neufeld & Schneider, 2009a ; Callele, Neufeld & Schneider, 2008c ; Callele, Neufeld & Schneider, 2006 ; Callele, Neufeld & Schneider, 2008a ; Callele, Neufeld & Schneider, 2009b ; Callele, Neufeld & Schneider, 2008b ) align with this perspective in their conceptualization of emotions as context-dependent outcomes of subjective evaluative processes.
• Group 4: Theory of constructed emotion
The theory of constructed emotion states emotions arises from processing goals, experiences, and situational factors. Studies ( Taveter et al., 2019 ; Taveter & Iqbal, 2021 ; Iqbal et al., 2023b ) refer to this theory.
• Group 5: Norman’s three levels of design
Study ( Miller et al., 2015 ) relates emotions to Norman’s reflective design level. While Visceral and Behavioral Levels: Focus on software functionality, usability, and performance. Reflective Level: Introduces emotions as “properties desired at a reflective level” regarding cultural meaning and personal resonance.
Emotional requirements elicitation techniques
This section addresses the elicitation techniques for emotional requirements. The extracted techniques were categorized and analyzed in terms of the elicitation approach, validation methodology, and domain of application. Table 9 depicts the elicitation techniques extracted from the literature reviewed. The following subsections discuss the categorization of the elicitation approaches, methods for validation, benefits, and limitations, and domain of application of these techniques.
Table 9. Categorization of emotional requirements eliciting techniques.
Elicitation techniques categorization.
• Interviews and workshops
Interviews and workshops involve direct engagement with stakeholders through open discussions, focus groups, or other interactive sessions to elicit requirements. These techniques enable analysts to explore stakeholders’ perspectives in depth through open-ended questions, brainstorming exercises, and collaborative activities. Key benefits of interviews and workshops include gaining contextual insights, uncovering hidden needs, and building bonds with stakeholders. However, results can be influenced by facilitator bias and limited participant availability.
Interviews and workshops were used in two studies (5% of the total), as seen in Fig. 4 . Miller et al. (2015) conducted semi-structured interviews with emergency dispatch officers, asking them to describe challenging calls and the emotions experienced. Through qualitative analysis of these emergency call narratives, key emotions like stress, urgency, and empathy were identified. The interviews also used emotion-oriented questions to directly elicit affective needs and priorities from the participants. Thew and Sutcliffe carried out interviews and workshops and inquired about the values driving different user groups ( Thew & Sutcliffe, 2018 ). By constructing value hierarchies and using laddering questioning techniques, they derived underlying emotional goals from the expressed values. These emotional goals were then mapped to quality attributes and more detailed requirements.
Figure 4. RE elicitation techniques categories.
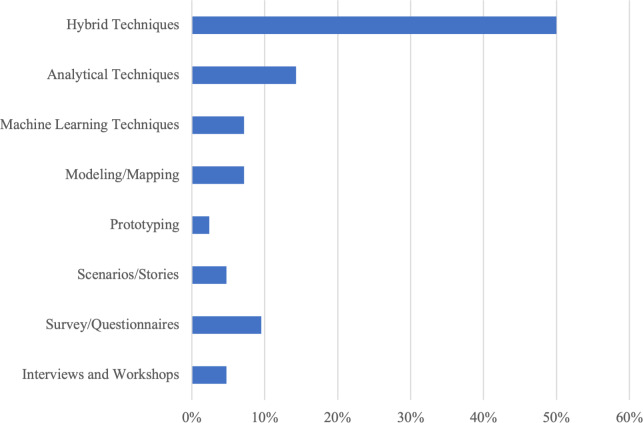
• Survey and questionnaires
Surveys and questionnaires present stakeholders with standardized sets of questions to gather requirements data. Closed-ended questions and rating scales allow for quantitative analysis of stakeholder priorities, preferences, and concerns. Surveys provide an efficient way to collect input from large, geographically dispersed groups. However, they limit the deeper discovery of stakeholder viewpoints found in interviews. Question biases also pose a threat to validity. Surveys and questionnaires accounted for four studies (10%). They were mainly validated via case studies, with applications in information systems, healthcare, and game development.
Xiang, Yang & Zhang (2016) surveyed team members for emotional requirements in an information system project. Proynova et al. (2010) and Proynova et al. (2011) proposed value and attitude-driven elicitation techniques based on mapping questionnaires to quality attributes. The conceptual approach is outlined ( Proynova et al., 2010 ), while Proynova et al. (2011) represents an initial implementation and piloting. Cockton et al. (2009) applied sentence completion surveys to uncover player emotions, motivations, and values about gaming. The textual responses were analyzed to create worth maps of important qualities for players.
• Prototyping
Prototypes provide stakeholders with simulations of the final system to interact with and provide feedback. Low-fidelity prototypes focus on conceptual design and workflows, while high-fidelity prototypes offer near-complete functionality. Observing user interactions and emotions elicited by prototypes reveals experiential requirements. However, users may focus excessively on surface details. Prototypes also entail development costs and can raise unrealistic expectations of the final system. Prototyping was used in only one study (2%). Callele, Neufeld & Schneider (2009b) followed an iterative prototyping process to elicit emotional needs for games without validation, signaling a need for more research on this elicitation approach.
• Scenarios/stories
Scenarios and stories paint a narrative picture of how users will interact with the system. By contextualizing requirements in real-world situations, analysts can identify usability issues, edge cases, and emotional elements. However, scenarios rely on analyst perceptions and may miss unexpected use cases. Representing all stakeholder needs through scenarios can also prove challenging. This approach was utilized by two studies (5%). Maier & Berry (2017) adopted a user story elicitation approach, having participants provide open user stories for a messaging application. User stories were annotated manually to identify ones expressing hedonic/emotional quality versus pragmatic quality. Ramos, Berry & Carvalho (2005) used storyboarding to elicit stakeholder emotions, values, and beliefs during organizational transformation requirements engineering.
• Modeling and mapping
Models and maps provide abstract visual representations of stakeholders, systems, processes, or other elements. Flow charts, customer journey maps, goal models, and other diagrams help analysts elicit and organize requirements. Visual models also aid communication with stakeholders. However, complex real-world dynamics may not fully translate to models. Analysts must take care to keep models simple enough to understand and use.
Modeling and mapping techniques accounted for three studies (7%). Sherkat et al. (2018) proposed the Emotional Attachment Framework that adapted existing models of emotional attachment to categorize drivers and map them to emotional goals in software design. This modeling enabled capturing and understanding emotional requirements. Sutcliffe used goal analysis to map obstacles to motivations and plan system responses to transform negative emotions into positive ones ( Sutcliffe, 2012 ). This mapping of goals and emotions facilitated tracing the sources of affective requirements. Bolchini, Garzotto & Paolini (2007) proposed mapping brand values to communication goals and then deriving aligned requirements. This modeling linked brand concepts to requirements through intermediate communication goals.
• Machine learning techniques
Machine learning techniques apply algorithms to large datasets to uncover patterns and insights. For requirements elicitation, machine learning can analyze user behaviors, feedback, and communication to identify preferences. Benefits include finding trends in unstructured data that humans could miss. Challenges include needing sufficient training data, interpreting complex algorithms, and addressing biases in data.
Machine learning was rarely used for elicitation, with only two studies (7%) employing it in different domains. Jean-Charles, Haas & Drennan (2019) proposed using supervised machine learning techniques to convey the emotional state of stakeholders during requirements elicitation interviews in real-time. This would allow analysts to adjust questioning approaches for sensitive topics based on the predicted emotional range. However, specific machine learning methods and validation were not detailed. Jiang and Li developed a multi-aspect sentiment analysis method using machine learning for mining customer requirements from online reviews ( Jiang & Li, 2020 ). This involved feature-sentiment word pair extraction, modifying an algorithm for enhanced accuracy, and converting sentiments into Kano categories. The technique was verified through real automobile review data, but limitations exist in handling reviewer value differences. Another recent study ( Cheng et al., 2023 ) proposes a multi-modal emotion recognition platform that integrates facial expressions, vocal intonation, and textual sentiment analysis. It captures stakeholders’ emotional cues in real-time.
• Analytical techniques
Analytical techniques apply mathematical and statistical methods to derive requirements insights from data. Key advantages are quantifying subjective qualities and uncertainties in requirements. However, solely relying on analytical techniques can overlook emotional and experiential aspects of requirements. Analytical techniques have been applied in (14%) of studies to uncover emotional requirements.
Affective grid analysis has been used to measure stakeholder emotions during requirements elicitation sessions, providing a quantitative analytical lens ( Colomo-Palacios et al., 2010 ; Colomo-Palacios et al., 2011 ). Fuzzy cognitive models have analyzed relationships between customer emotions, perceptual patterns, and product design elements, enabling emotion-focused product optimization ( Dong, Guo & Liu, 2014 ). Facial expression mapping to emotions via camera data has been proposed to enrich software requirements validation and verification, but further evaluation is needed ( Scherr et al., 2019 ). Analyzing users’ affective reactions to requirements and prototypes has been suggested to trace the source of emotions, improve specifications to remove negative emotion triggers, reassure users, and transform negative emotions through system responses in applications like healthcare ( Sutcliffe, 2011 ). Agent-based storyboards and scenarios illustrated the potential. Overall, analytical techniques demonstrate promise in eliciting emotional requirements but need expanded validation through additional case studies across problem domains.
• Hybrid techniques
Hybrid techniques combine two or more of the above elicitation methods to leverage their complementary strengths. For example, using surveys and interviews/workshops provides both quantitative insights and qualitative depth. However, hybrid techniques require expertise in multiple methods and can be resource-intensive to implement fully. Analysts must also carefully integrate divergent datasets from different techniques.
Hybrid techniques were most common in 21 studies (50%). They were validated mainly by case studies across diverse domains. This highlights the potential value of using mixed methods, but the need for expanded validation remains.
Structured interviews and workshops have been utilized to gather functional, quality, and emotional needs from users ( Taveter et al., 2019 ). Interviews have also been supplemented with biometric sensing of emotions during system usage to connect user preferences and concerns to requirements ( Stade et al., 2019 ). Customer journey mapping within a design thinking framework has helped uncover emotional needs through interviews and stakeholder feedback ( Levy, 2020 ). Customized interviews, prototyping, and think-aloud techniques have identified emotional characteristics and goals for healthcare applications ( Alkhomsan, Baslyman & Alshayeb, 2022 ). Content analysis of interviews, surveys, and trials combined with affinity diagrams and goal modeling has elicited smart home users’ emotional goals ( Curumsing et al., 2019 ). Conversational agents with multimodal sentiment analysis capabilities have been proposed to recognize user intentions and gather service requirements ( Yu et al., 2021 ). Focus groups and storytelling have been used in a participatory design approach to elicit requirements from users with specialized needs, like people with dementia ( Mulvenna et al., 2017 ). Surveys, focus groups, and statistical analysis have also revealed airport kiosk users’ emotional needs ( Hsu & Chen, 2021 ).
Across these studies, Callele, Neufeld & Schneider (2009a) ; Callele, Neufeld & Schneider (2008c) ; Callele, Neufeld & Schneider (2006) ; Callele, Neufeld & Schneider (2008a) , and Callele, Neufeld & Schneider (2008b) developed a coherent line of research using interviews, surveys, and tools to elicit emotional requirements in video gaming contexts. First, Callele, Neufeld & Schneider (2006) developed emotional intensity maps to capture designer intent for player experiences. It proposed using focus groups with designers to elicit statements such as “I want the player to feel anxious as they approach the entry to this room.” Another study introduced emotion markers to visualize requirements based on categorizing primary vs secondary emotions identified through interviews ( Callele, Neufeld & Schneider, 2009a ). Then, Callele, Neufeld & Schneider (2008c) is built on this by incorporating threat analysis and demonstrations to balance the potentially conflicting emotional and security needs of different stakeholders. Moreover, they used surveys and interviews to systematically analyze emotional and security requirements relationships in gaming ( Callele, Neufeld & Schneider, 2008a ). Finally, they enriched emotional requirements representation by capturing contextual positioning, temporal and relational information in Callele, Neufeld & Schneider (2008b) . While proposing techniques for emotional requirements in games, these studies lacked empirical evaluation. Further research should evaluate the utility of these proposed approaches.
Emotional goal modeling has been fused with other techniques like remote elicitation and prototyping to capture emotionally driven requirements ( Jackson & Norta, 2020 ). Combining motivational goal modeling with the theory of constructed emotion has been suggested to holistically elicit contextually grounded emotional requirements ( Taveter & Iqbal, 2021 ; Iqbal et al., 2023b ). In Iqbal et al. (2023b) the approach aims to represent stakeholders’ emotional perspectives through iterative development and evaluation of prototypes, aided by appraisal meetings to build consensus on functional, quality and emotional goals. Techniques like work system analysis, user stories, and personas have also been combined with emotional goal modeling to elicit digital health system requirements ( Abdullah et al., 2020 ).
Other proposed elicitation methods include sentiment analysis, association rule mining, and analogical reasoning to uncover latent customer needs from online reviews ( Zhou, Jianxin Jiao & Linsey, 2015 ). Conceptual frameworks have also been developed to improve the elicitation and analysis of soft contextual factors like values, motivations, and emotions through interviews, surveys, and structured analysis ( Thew & Sutcliffe, 2008 ).
Methods for validating emotional requirements elicitation techniques
This section investigates the methods and approaches used to validate the proposed techniques of emotional requirements elicitation, as seen in Table 9 . The results showed that 15% of studies proposed elicitation techniques but did not include validation. Most studies (76%) relied on illustrative case studies to demonstrate their approaches, as illustrated in Table 9 . For example, in Alkhomsan, Baslyman & Alshayeb (2022) , the case study illustrated the proposed approach with 5 participants to elicit emotional requirements related to using virtual clinics within the healthcare app. The multi-method approach demonstrates the potential of emotion-oriented requirements engineering to uncover latent user requirements and enhance adoption by integrating emotional perspectives in goal models.
Only 9% of studies utilized experiments to empirically verify the proposed methods. For example, ( Yu et al., 2021 ) conducted a controlled experiment using a conversational AI bot integrated with multimodal sentiment analysis to validate the effectiveness of incorporating sentiments for user intention mining.
Benefits and limitations of emotional requirements eliciting techniques
Currently, the elicitation techniques have several limitations, which can be categorized into four general groups: time-consuming and human involvement, subjectivity, resource, and technique complexity, as seen in Table 10 . Studies utilizing machine learning and sentiment analysis, such as in Zhou, Jianxin Jiao & Linsey (2015) , faced challenges with data sets, labeling, and the accuracy of human decisions in labeling specific reviews or sentences. Other studies proposed elicitation techniques requiring different resources, such as experts in psychology. A psychological expert was needed for the technique proposed in Sutcliffe (2012) . In Abdullah et al. (2020) , clinic staff struggled to articulate emotional goals clearly. Knowledge of machine learning classification techniques was required for the technique proposed in Jiang & Li (2020) . Additionally, each review should be processed equally, and the technique’s success depends on the quality of the reviewers.
Table 10. Limitations of emotional requirements eliciting techniques.
The complex nature of emotions makes identifying requirements challenging like typical requirements. This explains the sophisticated methods used to elicit emotions. Several difficulties and confusion were associated with the techniques proposed in Cockton et al. (2009) and Maier & Berry (2017) . Some studies offered techniques more prone to subjectivity than others due to the lack of tools or systematic methods for handling emotions. For instance, the approach proposed in Ramos, Berry & Carvalho (2005) places a heavy burden on the requirements engineer to find relevant emotional requirements and satisfactory solutions, as some stakeholders have strongly differing emotions.
The benefits of the techniques discussed in the literature review include their ability to communicate emotions to stakeholders and consider diverse roles. A visual representation of emotional requirements was proposed as a straightforward way to convey emotional requirements to designers in Dong, Guo & Liu (2014) . The approach proposed in Abdullah et al. (2020) can capture emotional goals relating to different roles interacting with the system. Another approach considered improved user experience by recognizing user emotions and developing user-friendly conversational AI bots ( Yu et al., 2021 ). To help users express emotions and address challenges in describing emotions, an approach was proposed using a circumplex model built on valence and arousal dimensions ( Alkhomsan, Baslyman & Alshayeb, 2022 ).
Emotional requirements applications domains
The purpose of this section is to identify the domains in which emotional requirements are most commonly investigated. Several key issues will be discussed, including understanding the domains that tend to consider emotional requirements and determining which elicitation techniques may be reused or improved for similar domains. Figure 5 outlines the distribution of primary studies across various domains pertinent to emotional requirements elicitation. The healthcare and well-being domain encompasses 11 studies (26% of the total), highlighting the prominence of emotional requirements in systems intended for healthcare and well-being purposes. Gaming represents the second most prevalent domain, with seven studies (17%). 14 studies (38%) fall under the broad domain of general emotional requirements not confined to a particular application area. The remaining domains of self-service technology, e-commerce, education, automobiles, and mobile applications contain 1–3 primary studies each, representing 3–7% of the total studies.
Figure 5. Elicitation techniques domains of applications.
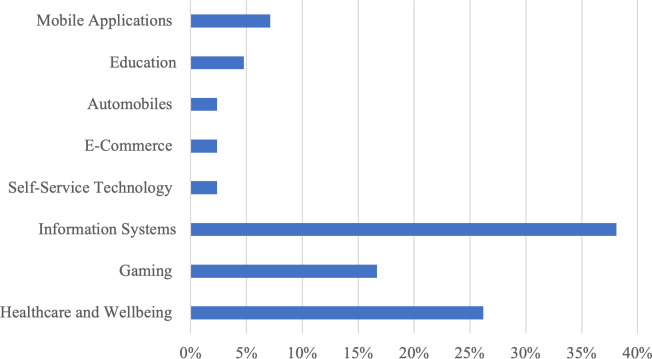
Emotional requirements modeling languages
Modeling is a crucial activity in requirements engineering to visually represent system goals, behaviors, and stakeholder needs. However, only a few studies have investigated modeling approaches tailored to emotional requirements, given their subjective and complex nature. This section discusses the requirements of modeling languages used to capture and convey emotional goals. Table 11 . summarizes several studies that have proposed extensions or novel applications of modeling languages for capturing and analyzing requirements, with a focus on emotional and soft goals. The studies cover a diverse set of domains, including information systems, healthcare, learning, and video game development.
Table 11. Requirements modeling languages used to model emotional goals.
Motivational goal modeling has been used in several studies to represent stakeholders and their goals. The motivational power of emotions is leveraged in these models to drive requirement elicitation and design processes. In Miller et al. (2015) , the authors extended Sterling and Taveter’s notion—motivational goal modeling notation—to capture emotions ( Sterling & Taveter, 2009 ). The key extension proposed by the authors was the addition of “emotional goals” to capture the reflective-level emotions and desires of stakeholders. They defined two types - personal emotional goals for inherent user emotions and context-specific goals for system-evoked emotions. The notation for emotional goals involves connecting a role to an emotion and then to a functional or quality goal. This captures that the role has the associated emotion with respect to that goal. The People-Oriented Software Engineering (POSE) framework exemplifies this approach, adding simple heart symbols to agent-modeling diagrams to signify emotional elements indicating how stakeholders should feel when engaging with the system. The study distinguished between two types of emotional goals: personal emotional goals, which model the emotional desires of users, and context-specific emotional goals, which model the desired effects that a system has on its users. The strength of POSE models lies in their straightforward notation and emphasis on high-level concepts, which make them particularly useful in the early phases of requirements engineering. Moreover, POSE models have a greater value as shared artifacts in meetings to communicate with different stakeholders. Built on this work, the authors’ group combined emotional goal modeling with viewpoint modeling, where they can capture the viewpoints of multiple stakeholders, including their emotional needs ( Curumsing et al., 2015 ), which is extended by more recent work in Goschnick (2018) ; Sterling, Lopez-Lorca & Kissoon Curumsing (2021) . They found that early-phase POSE models are most effective for understanding needs and applied this in a smart home system design in Curumsing et al. (2019) . In Taveter et al. (2019) and Jackson & Norta (2020) the POSE framework was also adopted for eliciting holistic emotional requirements. In Taveter et al. (2019) , the study uses POSE to elicit emotional requirements for two e-healthcare systems through interviews to capture emotions related to using the system. In Jackson & Norta (2020) , the study applies POSE remotely via an online questionnaire. The participants were asked about emotions in different usage scenarios. POSE Framework provides a means to conceptualize emotional requirements; however, it does not provide an emotional requirements-specific analysis method.
Another study in the domain of interactive quiz applications presents an agent-oriented modeling approach ( Yeewai et al., 0000 ). The approach comprises two phases—requirements elicitation and system design. The requirements phase focuses on understanding user needs through agent-oriented competency questions. The system design phase covers modeling interactive elements. Based on elicited responses, multiple-agent models are constructed to represent emotional factors like motivations.
Goal-oriented Requirements Languages (GRL) GRL was used to model emotional goals in relation to other goals that exist in the goal models ( Alkhomsan, Baslyman & Alshayeb, 2022 ). The study first defined emotional requirements elements and then mapped them to the elements of the GRL model. Also, it proposed modeling emotional goals in a specific entity called an actor’s emotions, which includes only an actor’s emotions. As a result, requirements will be better managed, and concerns will be separated. Emotional goals are defined as soft goals linked to other goals or tasks through contribution links. Overall, these studies demonstrate the prevalence of extending proven goal modeling notations with emotion-oriented constructs, given their ability to formalize subjective concepts.
The study of Schindel (2006) suggests extending SysML to include a “Human View” for soft requirements elicitation. The approach proposes modeling logical subsystems representing human behaviors, including emotional aspects, using features, attributes, and couplings. These logical human models can capture emotional behaviors, outcomes, and requirements while avoiding the need to model their physical basis. The human model is then integrated with the technical product model within a unified SysML model. This allows emotional attributes to be tied to product features and behaviors affecting human experience.
Domain-specific modeling languages allow tuning modeling semantics and notation precisely for expressing emotions within a particular application context. A study proposed the Videogame Emotion Language (VEL) specifically for representing emotional requirements in games, implementing a prototype editor for constructing VEL models ( Miguéis, Araujo & Moreira, 2019 ). This provides customized language constructs like “Emotions”, “Objects”, and “Triggers” tuned for modeling gaming experiences from an affective perspective. VEL was developed through domain analysis to identify core gaming emotion concepts, metamodeling to define abstract syntax, capturing key semantics and rules, and visually representing concrete syntax. It aims to help game developers understand and analyze player emotions and conflicts during initial design phases.
Overall, the prevalence of agent-oriented and goal-oriented modeling aligns with the focus on understanding stakeholder intentions, motivations, and emotions. However, model analysis, such as trade-off or conflict analysis, is not addressed.
Emotional requirements modeling languages benefits and limitations
A variety of modeling languages have been proposed to represent emotional requirements, offering potential benefits as well as facing limitations. Extensions to SysML integrate requirements focused on human-experienced qualities, emotions, and subjective perspectives (referred to here as “soft requirements”) into systems models, enabling the capture of diverse expert perspectives in Schindel (2006) , but require further research for real-world applications. Approaches extending motivational goal modeling ( Miller et al., 2015 ; Curumsing et al., 2015 ) allow simple graphical communication of emotional goals with stakeholders early in requirements engineering but risk introducing complexity through implicit relations. Alternative motivational goal modeling techniques link requirements and system design through competency questions; however, the multi-model process can be challenging to implement fully to derive designs ( Yeewai et al., 0000 ).
Motivational goal modeling represents functional, quality, and emotional goals, roles, and relations to support communication throughout the design process, yet needs integration into development activities ( Taveter et al., 2019 ). Domain-specific languages like VEL provide customized elicitation concepts and visual notation tailored to video games, though they involve extensive modeling overhead ( Miguéis, Araujo & Moreira, 2019 ). Enhanced agent-oriented models can identify user concerns early and improve requirements completeness but require extension across problem domains ( Curumsing et al., 2019 ). In summary, the key benefits of modeling languages are facilitating communication, while limitations include complexity, implementation difficulties, and insufficient validation across application contexts.
Emotional requirements modeling languages applications domains
The healthcare and wellbeing domain leads with five studies employing agent-oriented and goal-oriented modeling to capture emotional requirements in applications like mental health, caregiving, and emergency response systems. Capturing emotional requirements appears highly valuable in these contexts where user experience and emotional outcomes are central. Beyond healthcare, RML-based emotional modeling also holds promise in information systems design, as in Schindel (2006) proposed extensions for incorporating “soft” user requirements into model-based engineering. Emotional factors may enhance user acceptance and adoption of enterprise systems.
Additionally, the gaming domain offers an expected application, given the significant role of experience, narrative, and emotion in games. This is evidenced by domain-specific Videogame Emotion Language proposed in Miguéis, Araujo & Moreira (2019) . Tailored vocabularies and semantics can capture the nuances of emotion in gaming scenarios.
In this section, we discuss the results of this study under the elicitation technique and modeling language sections. In addition, we present some of the challenges associated with Emotional requirements elicitation and modeling. Finally, the section addresses some of the threats to validity and identifies interesting future research directions considering gaps and opportunities highlighted through this comprehensive literature review.
Emotions in RE
The topic of ER is relatively new, with 46 studies published from 2006 to 2023. The majority of studies published between 2019–2023 aimed to increase users’ acceptance and trust in smart home systems for the elderly ( Curumsing et al., 2019 ). Such solutions became a necessity to overcome issues caused by the aging population and the huge burden of hospitalization on the healthcare infrastructure. In other studies, machine learning (ML) techniques, specifically natural language processing (NLP), were widely employed to extract requirements from online reviews and facilitate the collection of requirements. Recently, ML techniques have been explored and utilized more for requirements elicitation since COVID-19 imposes a social distance, which hinders the work of requirements engineers ( Stade et al., 2019 ). The findings indicate that healthcare and well-being contexts are the most prevalent because their design focuses on how they can meet the personal and social needs of users. Incorporating emotional requirements can ensure these systems effectively support human-technology interaction. Overall, emotional requirements have growing relevance across domains, especially healthcare, to address emerging needs. Continued adoption of ML techniques may further enable remote elicitation. Healthcare and well-being systems represent a prime area for ER research, given their focus on users’ social and personal needs.
Emotions definitions
The examination of these studies highlights some key issues and limitations in how emotions have been conceptualized and defined within requirements engineering research. It was noticed that many of the papers lack clear, rigorous definitions regarding the central construct of emotion. Most studies did not provide explicit definitions, and those that did offer some characterization that describe emotions in broad, imprecise terms such as subjective experiences or internal states.
The reviewed studies reveal several key conceptual perspectives on emotions in requirements engineering and human–computer interaction research. The valence dimension provides a useful starting point for differentiating between positive and negative emotions to understand their potential impact on users’ experiences and reactions. However, this binary categorization has limitations in capturing the complexity of emotional states.
Incorporating arousal as an additional dimension aids in characterizing emotions based on intensity as well as valence ( Alkhomsan, Baslyman & Alshayeb, 2022 ). However, some critics argue that discrete categories cannot fully represent the fluid, dynamic nature of emotions. Appraisal theories address this by defining emotions as context-dependent intuitive reactions shaped by subjective evaluations of events, agents, or objects ( Roseman & Smith, 2001 ; Sherkat et al., 2018 ; Callele, Neufeld & Schneider, 2009a ; Callele, Neufeld & Schneider, 2008c ; Callele, Neufeld & Schneider, 2006 ; Callele, Neufeld & Schneider, 2008a ; Callele, Neufeld & Schneider, 2009b ; Callele, Neufeld & Schneider, 2008b ). This aligns with psychological constructionist views.
The theory of constructed emotion further proposes that emotions emerge from the neurocognitive processing of goals, prior experiences, and situational factors ( Taveter et al., 2019 ; Taveter & Iqbal, 2021 ; Taveter & Iqbal, 2021 ). Rather than fixed internal states, emotions are constructed transiently based on the interaction between the individual and their environment. This dynamical systems perspective resonates with trends in psychology emphasizing contextualized, embodied cognition. Linking emotions to Norman’s reflective level of design also highlights their close ties to sociocultural meanings, values, and purposes ( Miller et al., 2015 ). The paper argues that explicitly incorporating this level in requirements engineering can better capture emotional goals. Differentiating personal versus context-specific emotions further underscores the need for a multi-perspective approach.
The reviewed studies show an evolution in how emotions are conceptualized, moving away from static, decontextualized models towards more dynamic, constructivist perspectives aligned with modern affective science. This reflects a shift from viewing emotions as fixed internal states to seeing them as complex experiences constructed through processing goals, contexts, and prior interactions. Synthesizing diverse conceptual lenses provides a more detailed and multi-layered foundation for engaging with emotions in technology design and requirements engineering. Further interdisciplinary integration can enhance approaches to capturing emotional factors that shape human-technology relationships. Overall, the field displays progress in developing more holistic, human-centered understandings of the role of emotions.
There was a wide variety of elicitation techniques for emotional requirements, with hybrid approaches being the most common (21 studies, 50% of the total). This suggests combining methods may help capture emotional requirements from multiple angles, rather than assuming any single technique is fully comprehensive. For example, the mix of direct techniques like interviews with indirect ones like biometric sensing provides flexibility to elicit emotions that may be difficult for users to express directly ( Stade et al., 2019 ). In addition, Interviews, along with prototyping, help reveal emotions experienced during realistic user interactions rather than just self-reported emotions ( Alkhomsan, Baslyman & Alshayeb, 2022 ). Interviews and surveys were most common but require demonstrating reliability, rather than assuming inherent efficacy. Given the complexity of emotions, comprehensive validation of any elicitation technique is infeasible. However, researchers should seek evidence for reliability and appropriateness through replicating studies across contexts. Machine learning techniques were rarely used with a count of three studies. ML has strong potential for emotion analysis but has yet to be widely explored for requirements elicitation. ML techniques can detect emotional cues and sentiments from textual data like requirements documents. Also, it can analyze large volumes of requirements data efficiently for emotions. More research is needed to demonstrate its capabilities in this area. For example, apply natural language processing to better detect emotions in text and study which ML methods are most effective for emotion extraction from requirements.
Current research reveals promising opportunities in the well-being and game development domains. In particular, the gaming domain is primed for advancing emotional requirements practices, as games intrinsically motivate strong user emotions. Testing and validating contextual elicitation techniques like prototypes and playtesting in this domain could strengthen best practices more widely. Additionally, the emerging domain of wellness technologies requires eliciting sensitive, emotional factors to drive adoption and effectiveness. The application area substantively impacts progress, with gaming’s affective emphasis necessitating urgent elicitation practice improvements versus industrial domains with strict usability requirements that may see slower adoption. Thus, rigorous validation efforts on gaming and wellness can demonstrate tangible benefits and develop evidence-based guidelines.
While case studies can provide initial practical demonstrations, more controlled experiments are needed to thoroughly examine and compare the effectiveness of techniques, as case studies alone cannot isolate impact. While case studies demonstrate real-world feasibility, experiments better assess causality between methods and requirements quality. Prioritizing empirical studies with both experiments and case studies can strengthen the utility evidence base for emotional requirements elicitation.
The modeling of emotional requirements provides several key benefits. First, it allows for analyzing how various emotional needs relate to and impact other functional and non-functional requirements. Mapping out emotions as explicit requirements enables designers to reason about how inducing certain emotions could enable or inhibit other goals and behaviors. For example, designing to promote feelings of trust may facilitate greater disclosure of personal information, while anxiety may inhibit usage altogether. Also, emotional requirements modeling enables traceability from these affective considerations to downstream design decisions. This supports the assessment of whether certain features are properly aligned with intended emotional outcomes. Design choices can be evaluated based on their consistency with specified emotional needs.
The modeling of emotional requirements presents inherent challenges due to the subjective, intangible nature of human emotions. Initial efforts have been made to adapt standard modeling techniques or develop customized languages specifically for representing emotional user needs and goals. A predominant approach is extending established goal-oriented and agent-modeling methods to incorporate graphical notations denoting emotional goals, as exemplified in the People-Oriented Software Engineering (POSE) framework proposed across multiple studies. This reflects a conceptual orientation toward depicting emotions from a stakeholder-centric perspective. The POSE framework’s differentiation between personal and contextual emotional goals also provides a useful abstraction for modeling the various sources and targets of affective requirements. While POSE provides rich details on emotions, it lacks analytical capabilities. Goal-Oriented Requirements Language (GRL) has been extended to capture emotional goals and their relationships with other goals ( Alkhomsan, Baslyman & Alshayeb, 2022 ). GRL can be further extended to conduct a quantitative analysis of how emotional goals contribute to other goals. GRL also can simulate how different design choices could impact satisfaction levels of emotional goals. A promising direction is to extend modeling languages by leveraging the complementary strengths of both qualitative emotion representations from frameworks like POSE and the quantitative goal analysis techniques provided by languages such as GRL. This would allow comprehensive modeling of emotions along with systematic analysis of their interdependencies and influences.
For highly interactive systems like games, domain-specific languages like the Videogame Emotion Language (VEL) allow tuning modeling vocabulary and semantics precisely to the application context to capture experiential requirements. However, rigorous empirical evaluation of the effectiveness of domain-specific emotion modeling languages remains limited. In contrast, viewpoint-oriented modeling shows promise as a general technique for eliciting comprehensive emotional requirements by explicitly capturing the perspectives of diverse stakeholders. Mapping these emotional goals onto standard goal modeling languages promotes interoperability with existing tools and processes, such as in Alkhomsan, Baslyman & Alshayeb (2022) .
Challenges associated with developing er elicitation and modeling techniques
The complex nature of emotions makes it difficult for software engineers to build systems that reflect users’ emotional goals. The key challenge is identifying and understanding people’s subjective emotional needs, which are distinct from functional requirements and relate to desired feelings from using the software. Often, people struggle to articulate emotions directly. Although people can recognize their own emotions when experienced, they may find it hard to express them in advance since emotions are a subjective component of consciousness. Further, even if people communicate emotions, there remains uncertainty in how those emotions will be interpreted and transformed into specific requirements due to the ambiguous, unstructured, and difficult-to-quantify nature of emotional requirements.
Many techniques for eliciting emotions have limitations stemming from their inherent subjectivity or their complexity, time intensity, and need for human involvement. Requirements engineers can find themselves overwhelmed by the complexity of techniques, while project teams become overburdened by time-consuming tasks and limited resources. Per the study findings, elicitation techniques adapted from existing methods can be more effective regarding time, cost, and resources. However, without supporting tools, these techniques risk misinterpretation of the elicited emotions by the requirements engineer. Additionally, validating the effectiveness of elicitation techniques presents challenges. Most have only been demonstrated through illustrative case studies without validating their effectiveness and efficiency. This stems from the lack of metrics and the subjective nature of emotional requirements. Clear definitions of emotional requirements elements are needed first to validate a technique’s ability to elicit those elements. As a result, most proposed techniques have only been assessed for potential and feasibility, which is insufficient to determine their efficacy and efficiency in eliciting emotional requirements.
Modeling emotions in requirements engineering remains a significant research challenge due to the inherently complex, subjective nature of emotional states and experiences. Two primary issues are representing the dynamic changes in emotions over time and contexts and quantifying emotional data for enhanced analysis and decision-making. A core modeling challenge is the lack of representations of how emotions evolve over time and in response to contextual triggers. More research is needed on capturing emotion dynamics. Factors like demographics, environment, and social influences can alter emotions, so models should incorporate these factors. Longitudinal studies are required to understand emotion patterns, stability, and transitions. Also, capturing emotions through quantitative metrics poses significant research challenges for requirements modeling languages. It has important analytical benefits for requirements prioritization, impact estimation, and system optimization.
Comparing results to related literature
To situate the findings of this review in the broader literature, it is informative to systematically compare the conclusions reached in our work to those of highly relevant prior reviews on emotional requirements engineering. In particular, the recent systematic mapping study by Pacheco, García & Reyes (2018) provides an opportunity for an in-depth contrast of the conclusions and future directions identified regarding elicitation techniques, modeling languages, challenges, and research gaps. Iqbal et al. (2023a) conducted a systematic mapping focusing specifically on categorizing and analyzing the state-of-the-art research on emotions in requirements engineering, providing an overview of the field. A subset of the reviewed studies targeted elicitation and modeling, which are the main focus areas in our review.
Both reviews identified a similar number of primary studies on emotional requirements engineering, with our review including 46 papers and Iqbal et al.’s review containing 34 papers. There was an overlap of 23 studies included as primary sources in both reviews, indicating substantial agreement on the core relevant literature. This demonstrates clear consensus on foundational literature, while diversity remains in peripheral sources depending on the review’s precise scope, selected databases, and inclusion criteria.
For elicitation techniques, the categorization revealed strong parallels between the two reviews, with interviews, surveys/questionnaires, and hybrid approaches being dominant. Our review offers an enhanced analysis of limitations by categorizing them into four groups: time/human involvement, subjectivity, resources, and complexity. This structured understanding exceeded Iqbal et al.’s high-level discussion. Also, our review provides a clearer view of validation methods by quantifying the use of case studies (76%) and experiments (9%) to validate techniques. Iqbal et al. did not examine validation approaches in this level of detail.
For emotional requirements modeling, the two reviews exhibit close alignment. Extensions of goal-oriented and agent-oriented notations were central in both, enabling graphical representations of emotional goals and stakeholder perspectives. Domain-specific languages like the Videogame Emotion Language also emerged in each. The modeling technique similarities signify convergence on core approaches applied for emotional requirements to date. However, our review provides a more comprehensive coverage of modeling techniques with a dedicated focus on five studies, specifically on modeling languages. Iqbal et al. (2023a) had a broader scope without an in-depth focus on modeling.
Regarding application domains, healthcare, and well-being were dominant contexts in both reviews, underscoring the value of emotional requirements in these areas. Gaming was also a prominent domain in each. Some minor differences arose—our review included more enterprise/information system papers, while Iqbal et al. (2023a) covered more mobile applications. But both agreed on health and gaming as the central domains that currently consider emotional requirements.
Finally, the overarching conclusions and future directions show strong parallels between the two reviews. Our identification of opportunities like elicitation tools and modeling dynamics offers more precise future directions compared to the high-level discussion by Iqbal et al. (2023a) . Further synthesis across reviews will continue to strengthen collective knowledge.
Future directions
Users’ emotions are a promising aspect that can augment the user experience if identified effectively. However, requirement elicitation and modeling that incorporate users’ emotional needs remain limited. More effort is warranted to facilitate the integration of emotional requirements into practice. Considering our findings, we propose future research directions for eliciting and modeling emotional requirements.
Firstly, while some initial attempts have been made, eliciting emotional requirements remains challenging due to complexity, subjectivity, and resource constraints. Opportunities exist to investigate novel elicitation techniques and holistic ways of capturing and conveying ER. Validating collected ER also poses difficulties, as individuals perceive experiences uniquely. Resolving conflicting user emotions, especially across cultures, merits exploration. Developing tools to assist in eliciting emotions could prove beneficial, given the intricacy of feelings and the challenges of finding descriptive words universally. Such tools may facilitate cross-cultural, language-independent emotional expression. Secondly, modeling and systematically analyzing ER in relation to other requirements warrants examination. Bridging the gap between eliciting emotional goals and implementing design solutions requires a methodical approach to identify options that account for user emotions. Further research might evaluate ER-driven systems to quantify the value of considering ER during development.
Finally, additional domains that could benefit from emotional considerations include government, education, and e-commerce. Determining which emotions users wish to feel or avoid could enhance the system delivery of core value propositions across industries. Exploring privacy concerns and acceptance of emotion collection mechanisms also offer critical future directions. In summary, our findings highlight promising opportunities to advance the integration of users’ emotional needs through collecting and modeling software requirements. Furthermore, to explore the full potential of emotions to augment systems and enhance user experiences across domains.
Threats To Validity
In this section, we discuss how threats undermine the validity of research results but were addressed through rigorous procedures. Several types of validity were considered, including internal, external, and construct validity. Threats to each type were identified, and steps taken to limit impact are discussed in the following.
Internal validity
Internal validity refers to the integrity of the research methodology ( Kitchenham & Brereton, 2013 ) and analysis in representing the topic evaluated. This includes the search strategy, which significantly impacts the results. A rigorous approach developed an accurate string using keywords published in papers. However, the search string may have limitations in capturing all relevant papers, indicating compromised internal validity. This was mitigated by snowballing references to find additional papers and discussing the impact on results. Researcher bias threatened study selection and data extraction. Two inclusion/exclusion phases (title/abstract, full-text) and a data extraction sheet mitigated bias.
External validity
External validity considers how well findings can be generalized. The search was limited to four databases, potentially missing relevant studies published elsewhere. The threat was addressed by searching references for additional resources (snowballing). Furthermore, the exclusion of book chapters and technical reports from the search may have limited discovery of relevant practices and reduced generalizability.
Construct validity
Construct validity examines if the review methodology measures what it intends to. In some studies, information to assess quality, methodology, or tool/method details was implicit, requiring subjective interpretation and reducing construct validity. Two researchers conducted quality assessments and resolved differences through discussion to limit subjectivity.
Emotional requirements have gained more attention, and many studies have investigated how to incorporate emotional requirements into software engineering. As part of this systematic mapping, we have attempted to cover all the trends and areas in which emotional requirements have been utilized. During the study, elicitation techniques, modeling techniques, and application domains of emotional requirements were examined. In addition, the elicitation techniques were evaluated in terms of their benefits and limitations. According to our results, emotional requirements are a newly developed area that requires a lot of in-depth research into how to identify users’ emotions. A total of 41 out of 46 selected studies either proposed or customized elicitation techniques to collect emotional requirements. A total of 12 studies have examined the modeling of emotional requirements, but many considerations remain to be addressed. In addition, the results emphasized the limitations of the elicitation techniques, which were categorized into four broad categories: time-consuming and human involvement, subjectivity, resources, and technique complexity. Finally, a set of open issues and research directions were presented as a result of this review.
Supplemental Information
Acknowledgments.
We acknowledge the comments and suggestions made by the anonymous reviewers to improve the manuscript.
Funding Statement
This article is published under funded project # INFE2204 from the Interdisciplinary Research Center for Finance and Digital Economy, King Fahd University of Petroleum and Minerals, Dhahran, Saudi Arabia. The funders had no role in study design, data collection and analysis, decision to publish, or preparation of the manuscript.
Additional Information and Declarations
Competing interests.
The authors declare there are no competing interests.
Author Contributions
Mashail N. Alkhomsan conceived and designed the experiments, performed the experiments, analyzed the data, prepared figures and/or tables, authored or reviewed drafts of the article, and approved the final draft.
Malak Baslyman conceived and designed the experiments, performed the experiments, analyzed the data, prepared figures and/or tables, authored or reviewed drafts of the article, and approved the final draft.
Mohammad Alshayeb conceived and designed the experiments, performed the experiments, analyzed the data, prepared figures and/or tables, authored or reviewed drafts of the article, and approved the final draft.
Data Availability
The following information was supplied regarding data availability:
This is a literature review.
- Abdullah et al. (2020). Abdullah NNB, Grundy J, McIntosh J, How YC, Saharuddin S, Tat KK, ShinYe E, Rastom AJA, Othman NL. Using work system design, user stories and emotional goal modeling for an mHealth system. 2020 IEEE first international workshop on Requirements Engineering for Well-Being, Aging, and Health (REWBAH); Piscataway: IEEE; 2020. pp. 1–10. [ Google Scholar ]
- Alkhomsan, Baslyman & Alshayeb (2022). Alkhomsan MN, Baslyman M, Alshayeb M. Toward emotion-oriented requirements engineering: a case study of a virtual clinics application. IEEE 30th international requirements engineering conference workshops (REW); 2022. pp. 48–56. [ DOI ] [ Google Scholar ]
- Bolchini, Garzotto & Paolini (2007). Bolchini D, Garzotto F, Paolini P. Branding and communication goals for content-intensive interactive applications. 15th IEEE international requirements engineering conference (RE) 2007; Piscataway: IEEE; 2007. pp. 173–182. [ Google Scholar ]
- Callele, Neufeld & Schneider (2006). Callele D, Neufeld E, Schneider K. Emotional requirements in video games. 14th IEEE international requirements engineering conference (RE’06); Piscataway. 2006. pp. 299–302. [ Google Scholar ]
- Callele, Neufeld & Schneider (2008a). Callele D, Neufeld E, Schneider K. Balancing security requirements and emotional requirements in video games. 2008 16th IEEE international requirements engineering conference; 2008a. pp. 319–320. [ DOI ] [ Google Scholar ]
- Callele, Neufeld & Schneider (2008b). Callele D, Neufeld E, Schneider K. Emotional requirements. IEEE Software. 2008b;25(1):43–45. doi: 10.1109/MS.2008.5. [ DOI ] [ Google Scholar ]
- Callele, Neufeld & Schneider (2008c). Callele D, Neufeld E, Schneider K. Requirements in conflict: Player vs. designer vs. cheater. Third international workshop on multimedia and enjoyable requirements engineering-beyond mere descriptions and with more fun and games; Piscataway: IEEE; 2008c. pp. 12–21. [ Google Scholar ]
- Callele, Neufeld & Schneider (2009a). Callele D, Neufeld E, Schneider K. Visualizing emotional requirements. Fourth international workshop on requirements engineering visualization.Piscataway: IEEE; 2009a. pp. 1–10. [ Google Scholar ]
- Callele, Neufeld & Schneider (2009b). Callele D, Neufeld E, Schneider K. Augmenting emotional requirements with emotion markers and emotion prototypes. 2009 17th IEEE international requirements engineering conference; Piscataway: IEEE; 2009b. pp. 373–374. [ Google Scholar ]
- Cheng et al. (2023). Cheng B, Arora C, Liu X, Hoang T, Wang Y, Grundy J. Multi-modal emotion recognition for enhanced requirements engineering: a novel approach. Proceedings of the IEEE international conference on requirements engineering; 2023. pp. 9–304. [ DOI ] [ Google Scholar ]
- Cockton et al. (2009). Cockton G, Kujala S, Nurkka P, Hölttä T. Supporting worth mapping with sentence completion. IFIP conference on human-computer interaction; Cham: Springer; 2009. pp. 566–581. [ Google Scholar ]
- Colomo-Palacios et al. (2011). Colomo-Palacios R, Casado-Lumbreras C, Soto-Acosta P, García-Crespo Á. Using the affect grid to measure emotions in software requirements engineering 2011.
- Colomo-Palacios et al. (2010). Colomo-Palacios R, Hernández-López A, García-Crespo Á, Soto-Acosta P. A study of emotions in requirements engineering. World summit on knowledge society; Cham: Springer; 2010. pp. 1–7. [ Google Scholar ]
- Curumsing et al. (2019). Curumsing MK, Fernando N, Abdelrazek M, Vasa R, Mouzakis K, Grundy J. Emotion-oriented requirements engineering: a case study in developing a smart home system for the elderly. Journal of Systems and Software. 2019;147:215–229. doi: 10.1016/j.jss.2018.06.077. [ DOI ] [ Google Scholar ]
- Curumsing et al. (2015). Curumsing MK, Lopez-Lorca A, Miller T, Sterling L, Vasa R. Viewpoint modelling with emotions: a case study. International Journal of People-Oriented Programming. 2015;4(2):25–53. [ Google Scholar ]
- Dong, Guo & Liu (2014). Dong Y, Guo G, Liu W. Requirement elicitation in product emotional design using fuzzy clustering and fuzzy cognitive model. Proceedings of the Institution of Mechanical Engineers, Part B: Journal of Engineering Manufacture. 2014;228(1):156–160. doi: 10.1177/0954405413498732. [ DOI ] [ Google Scholar ]
- Fogg (2002). Fogg BJ. Persuasive technology: using computers to change what we think and do. Ubiquity. 2002;(December):2. [ Google Scholar ]
- Goschnick (2018). Goschnick S. Innovative methods, user-friendly tools, coding, and design approaches in people-oriented programming. IGI Global, 2018; [4 November 2023]. [ Google Scholar ]
- Han et al. (2022). Han Y, Moghaddam M, Suthar MT, Nanda G. Aspect-sentiment-guided opinion summarization for user need elicitation from online reviews. International design engineering technical conferences and computers and information in engineering conference; American Society of Mechanical Engineers; 2022. p. V002T02A007. [ Google Scholar ]
- Hsu & Chen (2021). Hsu H-M, Chen H-J. Integrating SSTQUAL, Kano model and attractiveness engineering to analyze user’s emotional needs in self check-in service. International conference on human-computer interaction; Springer; 2021. pp. 439–450. [ Google Scholar ]
- Roseman & Smith (2001). Roseman IJ, Smith CA. Appraisal theory, apprais. Process. Emotion Theory and Research. 2001:3–19. [ Google Scholar ]
- Iqbal et al. (2023a). Iqbal T, Anwar H, Filzah S, Gharib M, Mooses K, Taveter K. Emotions in requirements engineering: a systematic mapping study. IEEE/ACM 16th international conference on cooperative and human aspects of software engineering (CHASE); IEEE; 2023a. [21 October 2023]. pp. 111–120. [ Google Scholar ]
- Iqbal et al. (2023b). Iqbal T, Marshall JG, Taveter K, Schmidt A. Theory of constructed emotion meets RE: an industrial case study. Journal of Systems and Software. 2023b;197:111544. doi: 10.1016/j.jss.2022.111544. [ DOI ] [ Google Scholar ]
- Izard (1991). Izard CE. The psychology of emotions. New York: Plenum Press, Springer Science & Business Media; 1991. [27 October 2023]. . https://books.google.com/books?hl=ar&lr=&id=RPv-shA_sxMC&oi=fnd&pg=PA1&dq=Izard,C.E.(1991),ThePsychologyofEmotions(NewYork:Plenum)&ots=cUGcaj07W9&sig=fOFR3iFVCXc2qxjWXQqBNd8zvhU . [ Google Scholar ]
- Jackson & Norta (2020). Jackson E, Norta A. Design of a remote emotional requirement elicitation feedback method. IEEE third international workshop on affective computing in requirements engineering (AffectRE); 2020. pp. 3–8. [ DOI ] [ Google Scholar ]
- Jean-Charles, Haas & Drennan (2019). Jean-Charles N, Haas G, Drennan A. Using machine learning to convey emotions during requirements elicitation interviews. Proceedings of the 2019 ACM Southeast conference; 2019. pp. 266–267. [ Google Scholar ]
- Jiang & Li (2020). Jiang K, Li Y. Mining customer requirement from online reviews based on multi-aspected sentiment analysis and KANO model. 2020 16th Dahe Fortune China Forum and Chinese High-educational Management Annual Academic Conference (DFHMC); IEEE; 2020. pp. 150–156. [ Google Scholar ]
- Kitchenham & Brereton (2013). Kitchenham B, Brereton P. A systematic review of systematic review process research in software engineering. Information and Software Technology. 2013;55(12):2049–2075. doi: 10.1016/j.infsof.2013.07.010. [ DOI ] [ Google Scholar ]
- Law et al. (2008). Law E, Roto V, Vermeeren AP, Kort J, Hassenzahl M. Towards a shared definition of user experience. CHI’08 extended abstracts on human factors in computing systems; 2008. pp. 2395–2398. [ Google Scholar ]
- Levy (2020). Levy M. Emotional requirements for well-being applications : the customer journey. 2020 IEEE first international workshop on requirements engineering for well-being, aging, and health (REWBAH); 2020. pp. 35–40. [ DOI ] [ Google Scholar ]
- Lopez Lorca, Burrows & Sterling (2018). Lopez Lorca A, Burrows R, Sterling L. Teaching motivational models in agile requirements engineering. 2018 IEEE 8th international workshop on requirements engineering education and training (REET); 2018. pp. 30–39. [ DOI ] [ Google Scholar ]
- Lopez-Lorca et al. (2014). Lopez-Lorca AA, Miller T, Pedell S, Sterling L, Curumsing MK. Modelling emotional requirements. 2014. https://people.eng.unimelb.edu.au/tmiller/pubs/modelling-emotional-requirements.pdf https://people.eng.unimelb.edu.au/tmiller/pubs/modelling-emotional-requirements.pdf
- Maier & Berry (2017). Maier A, Berry DM. Improving the identification of hedonic quality in user requirements—a controlled experiment. 2017 IEEE 25th international requirements engineering conference (RE).Piscataway: IEEE; 2017. pp. 213–222. [ Google Scholar ]
- Miguéis, Araujo & Moreira (2019). Miguéis G, Araujo J, Moreira A. Towards a requirements language for modeling emotion in videogames. Proceedings of the 34th ACM/SIGAPP symposium on applied computing; 2019. pp. 1878–1880. [ Google Scholar ]
- Miller et al. (2015). Miller T, Pedell S, Lopez-Lorca AA, Mendoza A, Sterling L, Keirnan A. Emotion-led modelling for people-oriented requirements engineering: the case study of emergency systems. Journal of Systems and Software. 2015;105:54–71. doi: 10.1016/j.jss.2015.03.044. [ DOI ] [ Google Scholar ]
- Mooses et al. (2022). Mooses K, Camacho M, Cavallo F, Burnard MD, Dantas C, D’Onofrio G, Fernandes A, Fiorini L, Gama A, Perandrés Gómez A. Involving older adults during COVID-19 restrictions in developing an ecosystem supporting active aging: overview of alternative elicitation methods and common requirements from five European countries. Frontiers in Psychology. 2022;13:818706. doi: 10.3389/fpsyg.2022.818706. [ DOI ] [ PMC free article ] [ PubMed ] [ Google Scholar ]
- Mulvenna et al. (2017). Mulvenna M, Zheng H, Bond R, McAllister P, Wang H, Riestra R. Participatory design-based requirements elicitation involving people living with dementia towards a home- based platform to monitor emotional wellbeing. 2017 IEEE international conference on bioinformatics and biomedicine (BIBM).Piscataway: IEEE; 2017. pp. 2026–2030. [ Google Scholar ]
- Pacheco, García & Reyes (2018). Pacheco C, García I, Reyes M. Requirements elicitation techniques: a systematic literature review based on the maturity of the techniques. IET Software. 2018;12(4):365–378. doi: 10.1049/iet-sen.2017.0144. [ DOI ] [ Google Scholar ]
- Popay et al. (2006). Popay J, Roberts H, Sowden A, Petticrew M, Arai L, Rodgers M, Britten N, Roen K, Duffy S. Guidance on the conduct of narrative synthesis in systematic reviews. A Product from the ESRC Methods Programme Version 1. 2006:b92. [ Google Scholar ]
- Proynova et al. (2011). Proynova R, Paech B, Koch SH, Wicht A, Wetter T. Investigating the influence of personal values on requirements for health care information systems. Proceedings of the 3rd workshop on software engineering in health care; 2011. pp. 48–55. [ Google Scholar ]
- Proynova et al. (2010). Proynova R, Paech B, Wicht A, Wetter T. Use of personal values in requirements engineering—a research preview. International working conference on requirements engineering: foundation for software quality; Cham: Springer; 2010. pp. 17–22. [ Google Scholar ]
- Ramos, Berry & Carvalho (2005). Ramos I, Berry DM, Carvalho JÁ. Requirements engineering for organizational transformation. Information and Software Technology. 2005;47(7):479–495. doi: 10.1016/j.infsof.2004.09.014. [ DOI ] [ Google Scholar ]
- Russell (1989). Russell JA. The measurement of emotions. Elsevier; 1989. [27 October 2023]. Measures of emotion; pp. 83–111. [ Google Scholar ]
- Scherr et al. (2019). Scherr SA, Mennig P, Kammler C, Elberzhager F. On the road to enriching the app improvement process with emotions. 2019 IEEE 27th international requirements engineering conference workshops (REW); Piscataway: IEEE; 2019. pp. 84–91. [ Google Scholar ]
- Schindel (2006). Schindel WD. Feelings and physics: emotional, psychological, and other soft human requirements, by model-based systems engineering. INCOSE international symposium; Wiley Online Library; 2006. pp. 222–236. [ Google Scholar ]
- Sherkat et al. (2018). Sherkat M, Mendoza A, Miller T, Burrows R. Emotional attachment framework for people-oriented software. 20181803.08171
- Stade et al. (2019). Stade M, Scherr SA, Mennig P, Elberzhager F, Seyff N. Don’t worry, be happy–exploring users’ emotions during app usage for requirements engineering. 2019 IEEE 27th international requirements engineering conference (RE); Piscataway: IEEE; 2019. pp. 375–380. [ Google Scholar ]
- Sterling, Lopez-Lorca & Kissoon Curumsing (2021). Sterling L, Lopez-Lorca A, Kissoon Curumsing M. Adding emotions to models in a viewpoint modelling framework from agent-oriented software engineering: a case study with emergency alarms. 2021. pp. 439–478. [ DOI ]
- Sterling & Taveter (2009). Sterling L, Taveter K. The art of agent-oriented modeling. Cambridge: MIT Press; 2009. [ Google Scholar ]
- Sutcliffe (2011). Sutcliffe A. Emotional requirements engineering. 2011 IEEE 19th international requirements engineering conference, Trento, Italy; 2011. pp. 321–322. [ DOI ] [ Google Scholar ]
- Sutcliffe (2012). Sutcliffe A. Usability-and accessibility-focused requirements engineering. Springer; Cham: 2012. User-oriented requirements engineering; pp. 11–33. [ Google Scholar ]
- Taveter & Iqbal (2021). Taveter K, Iqbal T. A method for eliciting and representing emotional requirements: two case studies in e-healthcare. 2021 IEEE 29th international requirements engineering conference workshops (REW); 2021. pp. 100–105. [ DOI ] [ Google Scholar ]
- Taveter et al. (2019). Taveter K, Sterling L, Pedell S, Burrows R, Taveter EM. A method for eliciting and representing emotional requirements: two case studies in e-healthcare. 2019 IEEE 27th international requirements engineering conference workshops (REW); 2019. pp. 100–105. [ DOI ] [ Google Scholar ]
- Thew & Sutcliffe (2008). Thew S, Sutcliffe A. Investigating the Role of’Soft issues’ in the RE Process. 2008 16th IEEE international requirements engineering conference.Piscataway: IEEE; 2008. pp. 63–66. [ Google Scholar ]
- Thew & Sutcliffe (2018). Thew S, Sutcliffe A. Value-based requirements engineering: method and experience. Requirements Engineering. 2018;23(4):443–464. doi: 10.1007/s00766-017-0273-y. [ DOI ] [ PMC free article ] [ PubMed ] [ Google Scholar ]
- Wong, Mauricio & Rodriguez (2017). Wong LR, Mauricio DS, Rodriguez GD. A systematic literature review about software requirements elicitation. Journal of Engineering Science and Technology. 2017;12(2):296–317. [ Google Scholar ]
- Xiang, Yang & Zhang (2016). Xiang C, Yang Z, Zhang L. Improving IS development teams’ performance during requirement analysis in project—the perspectives from shared mental model and emotional intelligence. International Journal of Project Management. 2016;34(7):1266–1279. doi: 10.1016/j.ijproman.2016.06.009. [ DOI ] [ Google Scholar ]
- Yang et al. (2014). Yang Z, Li Z, Jin Z, Chen Y. A systematic literature review of requirements modeling and analysis for self-adaptive systems. International working conference on requirements engineering: foundation for software quality; Cham: Springer; 2014. pp. 55–71. [ Google Scholar ]
- Yeewai et al. (0000). Yeewai S, Waishiang C, Zulkifli CF, Jali NB, Khairuddin MAB. Requirement engineering meets emotion: a case study of quiz MASTer. International Journal of Advanced Science and Technology. 28(2):215–222. [ Google Scholar ]
- Yu et al. (2021). Yu D, Tian J, Su T, Tu Z, Xu X, Wang Z. Incorporating multimodal sentiments into conversational bots for service requirement elicitation. IEEE international conference on service-oriented system engineering (SOSE); 2021. pp. 81–90. [ DOI ] [ Google Scholar ]
- Zhou, Jianxin Jiao & Linsey (2015). Zhou F, Jianxin Jiao R, Linsey JS. Latent customer needs elicitation by use case analogical reasoning from sentiment analysis of online product reviews. Journal of Mechanical Design. 2015;137(7):071401. doi: 10.1115/1.4030159. [ DOI ] [ Google Scholar ]
- Zulkifli & Shiang (2023). Zulkifli SF, Shiang CW. Engineering emotional requirements for interactive digital narratives. Proceedings - 31st IEEE international requirements engineering conference workshops, REW 2023; 2023. pp. 5–11. [ DOI ] [ Google Scholar ]
Associated Data
This section collects any data citations, data availability statements, or supplementary materials included in this article.
Supplementary Materials
Data availability statement.
- View on publisher site
- PDF (1.3 MB)
- Collections
Similar articles
Cited by other articles, links to ncbi databases.
- Download .nbib .nbib
- Format: AMA APA MLA NLM
Add to Collections
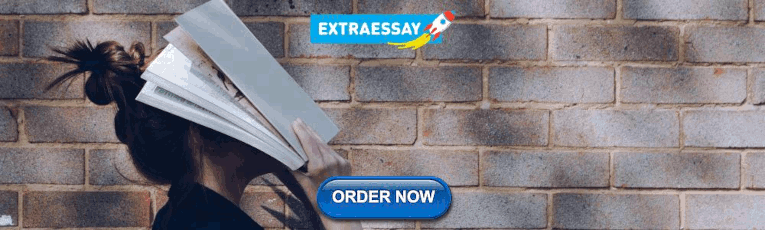
IMAGES
VIDEO
COMMENTS
This study presents a systematic review of relevant literature on requirements elicitation techniques, from 1993 to 2015, by addressing two research questions: Which mature techniques are currently used for eliciting software requirements? and Which mature techniques improve the elicitation effectiveness?
A systematic literature review aims to answer a specific research question using systematic methods to consolidate all relevant evidence that meets pre-defined eligibility criteria . It consists of three main phases: planning, conducting, and reporting the review.
This study presents a systematic review of relevant literature on requirements elicitation techniques, from 1993 to 2015, by addressing two research questions: Which mature techniques are currently used for eliciting software requirements? and Which mature techniques improve the elicitation effectiveness?
Data-Driven Requirements Elicitation: A Systematic Literature Review. February 2021. SN Computer Science 2 (1) DOI: 10.1007/s42979-020-00416-4. License. CC BY 4.0....
The aim of the present study is therefore to employ a systematic review to investigate the state-of-the-art of those approaches that leverage creativity in requirements elicitation within Agile Software Development, as well as the benefits, limitations and strength of evidence of these approaches.
111. Abstract. Requirements engineering has traditionally been stakeholder-driven. In addition to domain knowledge, widespread digitalization has led to the generation of vast amounts of data (Big Data) from heterogeneous digital sources such as the Internet of Things (IoT), mobile devices, and social networks.
TLDR. The purpose of this interview study was to elicit practitioners' overall expectations and needs for a method for the elicitation of system requirements from digital data sources, and lead to the identification of a set of high-level requirements related to the method for the elicitation from digital data sources. Expand.
Requirements elicitation is one of the most essential activities in requirements engineering. A growing trend in collaborative applications has imposed a challe.
This paper aims to identify the current status of the latest researches related to software requirements elicitation through general framework for literature review, in order to answer the...
This systematic mapping review analyzes emotional requirements (ER) practices in software engineering from two perspectives: elicitation and modeling. For elicitation techniques, we investigate the techniques, evaluation methods, limitations, and application domains.