
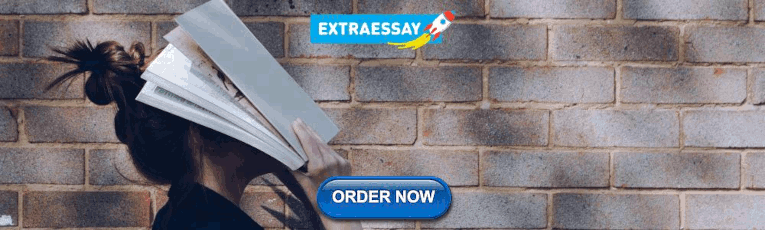
Quantitative and Qualitative Research
- I NEED TO . . .
What is Quantitative Research?
- What is Qualitative Research?
- Quantitative vs Qualitative
- Step 1: Accessing CINAHL
- Step 2: Create a Keyword Search
- Step 3: Create a Subject Heading Search
- Step 4: Repeat Steps 1-3 for Second Concept
- Step 5: Repeat Steps 1-3 for Quantitative Terms
- Step 6: Combining All Searches
- Step 7: Adding Limiters
- Step 8: Save Your Search!
- What Kind of Article is This?
- More Research Help This link opens in a new window
Quantitative methodology is the dominant research framework in the social sciences. It refers to a set of strategies, techniques and assumptions used to study psychological, social and economic processes through the exploration of numeric patterns . Quantitative research gathers a range of numeric data. Some of the numeric data is intrinsically quantitative (e.g. personal income), while in other cases the numeric structure is imposed (e.g. ‘On a scale from 1 to 10, how depressed did you feel last week?’). The collection of quantitative information allows researchers to conduct simple to extremely sophisticated statistical analyses that aggregate the data (e.g. averages, percentages), show relationships among the data (e.g. ‘Students with lower grade point averages tend to score lower on a depression scale’) or compare across aggregated data (e.g. the USA has a higher gross domestic product than Spain). Quantitative research includes methodologies such as questionnaires, structured observations or experiments and stands in contrast to qualitative research. Qualitative research involves the collection and analysis of narratives and/or open-ended observations through methodologies such as interviews, focus groups or ethnographies.
Coghlan, D., Brydon-Miller, M. (2014). The SAGE encyclopedia of action research (Vols. 1-2). London, : SAGE Publications Ltd doi: 10.4135/9781446294406
What is the purpose of quantitative research?
The purpose of quantitative research is to generate knowledge and create understanding about the social world. Quantitative research is used by social scientists, including communication researchers, to observe phenomena or occurrences affecting individuals. Social scientists are concerned with the study of people. Quantitative research is a way to learn about a particular group of people, known as a sample population. Using scientific inquiry, quantitative research relies on data that are observed or measured to examine questions about the sample population.
Allen, M. (2017). The SAGE encyclopedia of communication research methods (Vols. 1-4). Thousand Oaks, CA: SAGE Publications, Inc doi: 10.4135/9781483381411
How do I know if the study is a quantitative design? What type of quantitative study is it?
Quantitative Research Designs: Descriptive non-experimental, Quasi-experimental or Experimental?
Studies do not always explicitly state what kind of research design is being used. You will need to know how to decipher which design type is used. The following video will help you determine the quantitative design type.
- << Previous: I NEED TO . . .
- Next: What is Qualitative Research? >>
- Last Updated: Dec 8, 2023 10:05 PM
- URL: https://libguides.uta.edu/quantitative_and_qualitative_research
University of Texas Arlington Libraries 702 Planetarium Place · Arlington, TX 76019 · 817-272-3000
- Internet Privacy
- Accessibility
- Problems with a guide? Contact Us.
Please enable Javascript in your web browser in order to use the features on this website.
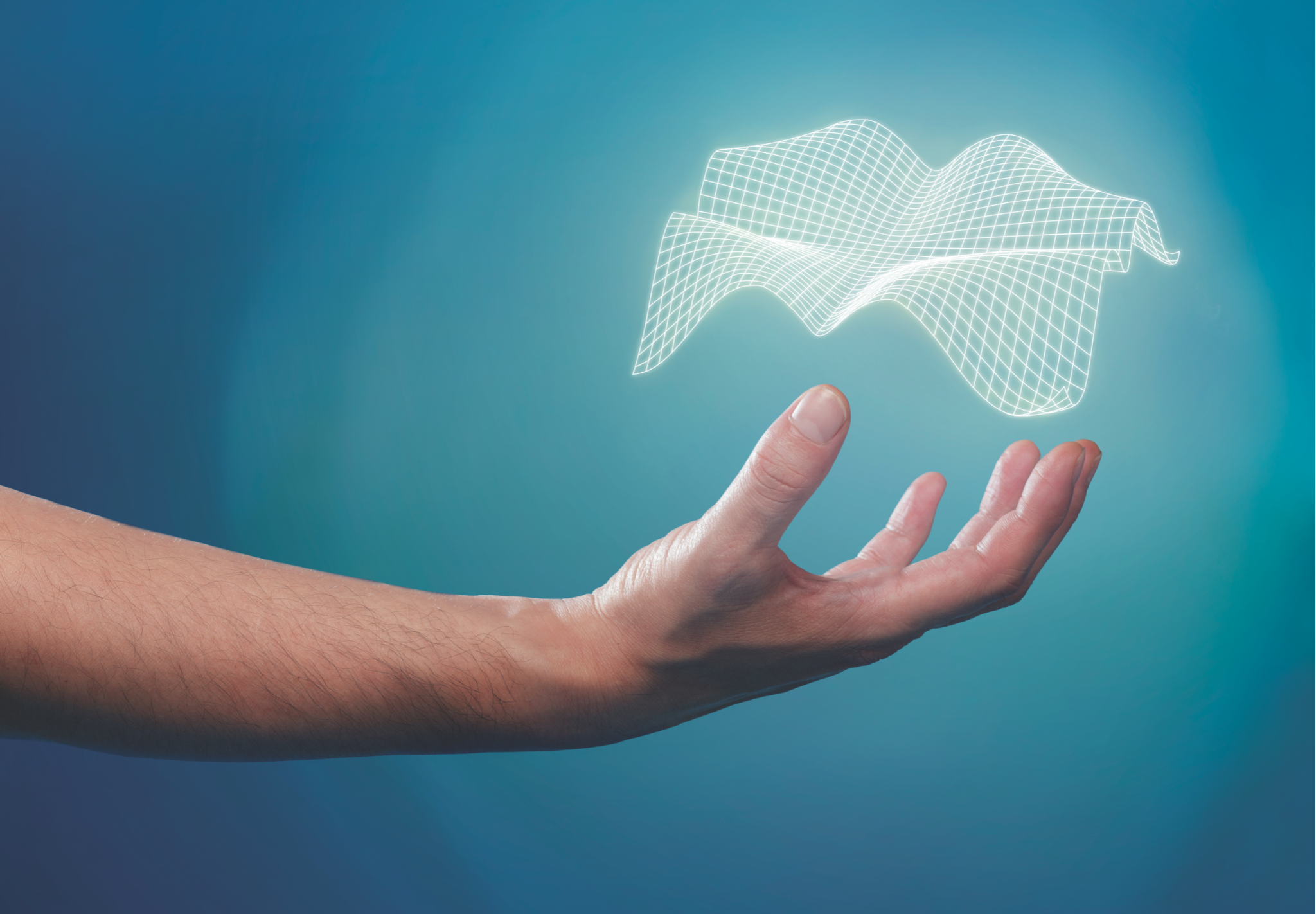
Quantitative Sciences Major
Overview of the qss major.
The Quantitative Sciences (QSS) major is the integration of liberal arts and data science. With the QSS major, students learn data science techniques and quantitative theory while they study the natural sciences, social sciences, or humanities.
You will take quantitative classes (such as statistics, game theory, and experimental methods) and classes in an associated discipline based on your area of interest (such as political science, psychology, neuroscience and behavioral biology, history, and more).
Finally, a major as diverse as your interests!
If you aren't sure what discipline you want to pursue, you can start with the "Undeclared" QSS track and choose your discipline later. The core quantitative coursework is the same no matter what path you eventually pick.
What distinguishes QSS from similar majors?

- A common core of quantitative classes allows you to seamlessly switch between areas of disciplinary interest. Don't want to study QSS with psychology anymore? The core QSS classes (Intro to Scientific Methods, Intro to Statistical Computing, Calculus, Probability and Statistics, and Regression Analysis) will transfer to any other QSS track, simply requiring a new set of electives to get your bearings in your new field.
- Simply put, there is no other major like QSS. There is room for a quantitative approach to data in any field of study: QTM currently offers 19 tracks (and growing). QTM's unique interdisciplinary approach enables you to learn quantitative theory and methods that apply directly to your academic and career interests —whatever they happen to be.
This major will prepare you for:
- Employment in data-oriented or quantitative fields
- Becoming a "Type A" Data Scientist (data analyst)
- Graduate school in business analytics, humanities, social sciences, or physical and natural sciences
Degree Option FAQs
How to complete the qss major.
The checklists linked below are for students who declare(d) their major Fall 2022 or later. Did you declare your QSS major before Fall 2022? Click here to see the correct track checklists for your course of study!
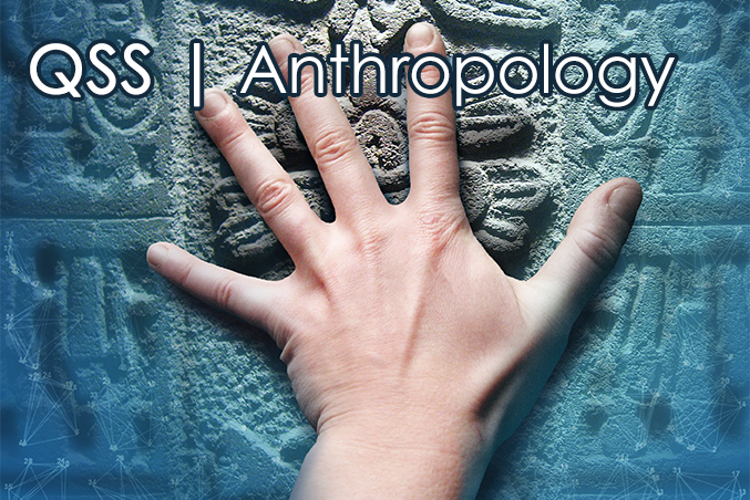
Have a language expert improve your writing
Run a free plagiarism check in 10 minutes, generate accurate citations for free.
- Knowledge Base
Methodology
- What Is Quantitative Research? | Definition, Uses & Methods
What Is Quantitative Research? | Definition, Uses & Methods
Published on June 12, 2020 by Pritha Bhandari . Revised on June 22, 2023.
Quantitative research is the process of collecting and analyzing numerical data. It can be used to find patterns and averages, make predictions, test causal relationships, and generalize results to wider populations.
Quantitative research is the opposite of qualitative research , which involves collecting and analyzing non-numerical data (e.g., text, video, or audio).
Quantitative research is widely used in the natural and social sciences: biology, chemistry, psychology, economics, sociology, marketing, etc.
- What is the demographic makeup of Singapore in 2020?
- How has the average temperature changed globally over the last century?
- Does environmental pollution affect the prevalence of honey bees?
- Does working from home increase productivity for people with long commutes?
Table of contents
Quantitative research methods, quantitative data analysis, advantages of quantitative research, disadvantages of quantitative research, other interesting articles, frequently asked questions about quantitative research.
You can use quantitative research methods for descriptive, correlational or experimental research.
- In descriptive research , you simply seek an overall summary of your study variables.
- In correlational research , you investigate relationships between your study variables.
- In experimental research , you systematically examine whether there is a cause-and-effect relationship between variables.
Correlational and experimental research can both be used to formally test hypotheses , or predictions, using statistics. The results may be generalized to broader populations based on the sampling method used.
To collect quantitative data, you will often need to use operational definitions that translate abstract concepts (e.g., mood) into observable and quantifiable measures (e.g., self-ratings of feelings and energy levels).
Note that quantitative research is at risk for certain research biases , including information bias , omitted variable bias , sampling bias , or selection bias . Be sure that you’re aware of potential biases as you collect and analyze your data to prevent them from impacting your work too much.
Here's why students love Scribbr's proofreading services
Discover proofreading & editing
Once data is collected, you may need to process it before it can be analyzed. For example, survey and test data may need to be transformed from words to numbers. Then, you can use statistical analysis to answer your research questions .
Descriptive statistics will give you a summary of your data and include measures of averages and variability. You can also use graphs, scatter plots and frequency tables to visualize your data and check for any trends or outliers.
Using inferential statistics , you can make predictions or generalizations based on your data. You can test your hypothesis or use your sample data to estimate the population parameter .
First, you use descriptive statistics to get a summary of the data. You find the mean (average) and the mode (most frequent rating) of procrastination of the two groups, and plot the data to see if there are any outliers.
You can also assess the reliability and validity of your data collection methods to indicate how consistently and accurately your methods actually measured what you wanted them to.
Quantitative research is often used to standardize data collection and generalize findings . Strengths of this approach include:
- Replication
Repeating the study is possible because of standardized data collection protocols and tangible definitions of abstract concepts.
- Direct comparisons of results
The study can be reproduced in other cultural settings, times or with different groups of participants. Results can be compared statistically.
- Large samples
Data from large samples can be processed and analyzed using reliable and consistent procedures through quantitative data analysis.
- Hypothesis testing
Using formalized and established hypothesis testing procedures means that you have to carefully consider and report your research variables, predictions, data collection and testing methods before coming to a conclusion.
Despite the benefits of quantitative research, it is sometimes inadequate in explaining complex research topics. Its limitations include:
- Superficiality
Using precise and restrictive operational definitions may inadequately represent complex concepts. For example, the concept of mood may be represented with just a number in quantitative research, but explained with elaboration in qualitative research.
- Narrow focus
Predetermined variables and measurement procedures can mean that you ignore other relevant observations.
- Structural bias
Despite standardized procedures, structural biases can still affect quantitative research. Missing data , imprecise measurements or inappropriate sampling methods are biases that can lead to the wrong conclusions.
- Lack of context
Quantitative research often uses unnatural settings like laboratories or fails to consider historical and cultural contexts that may affect data collection and results.
If you want to know more about statistics , methodology , or research bias , make sure to check out some of our other articles with explanations and examples.
- Chi square goodness of fit test
- Degrees of freedom
- Null hypothesis
- Discourse analysis
- Control groups
- Mixed methods research
- Non-probability sampling
- Inclusion and exclusion criteria
Research bias
- Rosenthal effect
- Implicit bias
- Cognitive bias
- Selection bias
- Negativity bias
- Status quo bias
Quantitative research deals with numbers and statistics, while qualitative research deals with words and meanings.
Quantitative methods allow you to systematically measure variables and test hypotheses . Qualitative methods allow you to explore concepts and experiences in more detail.
In mixed methods research , you use both qualitative and quantitative data collection and analysis methods to answer your research question .
Data collection is the systematic process by which observations or measurements are gathered in research. It is used in many different contexts by academics, governments, businesses, and other organizations.
Operationalization means turning abstract conceptual ideas into measurable observations.
For example, the concept of social anxiety isn’t directly observable, but it can be operationally defined in terms of self-rating scores, behavioral avoidance of crowded places, or physical anxiety symptoms in social situations.
Before collecting data , it’s important to consider how you will operationalize the variables that you want to measure.
Reliability and validity are both about how well a method measures something:
- Reliability refers to the consistency of a measure (whether the results can be reproduced under the same conditions).
- Validity refers to the accuracy of a measure (whether the results really do represent what they are supposed to measure).
If you are doing experimental research, you also have to consider the internal and external validity of your experiment.
Hypothesis testing is a formal procedure for investigating our ideas about the world using statistics. It is used by scientists to test specific predictions, called hypotheses , by calculating how likely it is that a pattern or relationship between variables could have arisen by chance.
Cite this Scribbr article
If you want to cite this source, you can copy and paste the citation or click the “Cite this Scribbr article” button to automatically add the citation to our free Citation Generator.
Bhandari, P. (2023, June 22). What Is Quantitative Research? | Definition, Uses & Methods. Scribbr. Retrieved April 13, 2024, from https://www.scribbr.com/methodology/quantitative-research/
Is this article helpful?
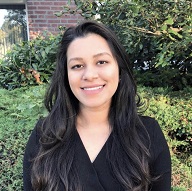
Pritha Bhandari
Other students also liked, descriptive statistics | definitions, types, examples, inferential statistics | an easy introduction & examples, what is your plagiarism score.
Brought to you by:

Quantitative Methods Online Course
By: Janice H. Hammond
Quantitative Methods is an introduction to using statistical methods to solve business problems. The course teaches statistics from the management perspective and places special emphasis on…
- Length: 20 hours
- Publication Date: Oct 24, 2004
- Discipline: General Management
- Product #: 504702-HTM-ENG
What's included:
- Teaching Note
$75.00 per student
degree granting course
$150.00 per student
non-degree granting course
Get access to this material, plus much more with a free Educator Account:
- Access to world-famous HBS cases
- Up to 60% off materials for your students
- Resources for teaching online
- Tips and reviews from other Educators
Already registered? Sign in
- Student Registration
- Non-Academic Registration
- Included Materials
The Regression Section (#6012) is available as an alternative to the complete course. Please note: Faculty requesting the Teaching Note for this course should be aware that they will receive two download links - the first link is restricted/unavailable, but the second link will download the appropriate document. We apologize for the inconvenience.
Quantitative Methods is an introduction to using statistical methods to solve business problems. The course teaches statistics from the management perspective and places special emphasis on developing the skills and instincts needed to make good decisions and become a more effective manager. The course is set in Hawaii at a fictional resort hotel. Students act as consultants hired to develop statistical models to aid the management team in making decisions for running the hotel. Students use sampling and estimation techniques to determine guest satisfaction levels and they analyze the risks and returns in investing in a new business venture for the hotel. Course topics are presented in a highly interactive format with narrated animations and self-correcting exercises. Microsoft Excel worksheets are included to assist students as they work towards mastering the subject matter. The course includes pre- and post-assessments.
Student progress and test scores can be viewed online or downloaded to Excel format. Seat time is 20 to 50 hours, depending on the student's experience with the material. 2017 UPDATES: This online course now features a redesigned administrator section with significantly more information about student performance, randomized ordering of student exam questions, a Certificate of Completion for students, administrator instructions for uploading student scores into an LMS, and additional quality assurance testing across content and browsers.
Learning Objectives
To understand how to analyze quantitative information and make better business decisions.
Oct 24, 2004 (Revised: Jan 24, 2017)
Discipline:
General Management
Harvard Business School
504702-HTM-ENG
We use cookies to understand how you use our site and to improve your experience, including personalizing content. Learn More . By continuing to use our site, you accept our use of cookies and revised Privacy Policy .

Overview of Quantitative Courses for 2023-2024
This is an unofficial list of quantitative courses anticipated to be offered in the coming year. Finalized course schedules are published on the Registrar’s Course Search page. For course categorization, please refer to our Course Overview Table . (Please Note: Given courses are only planned for the upcoming autumn quarter, this list will need to be updated quarterly.)
Booth School of Business
BUSN 36906 Stochastic Processes BUSN 37103 Data-Driven Marketing BUSN 37105 Data Science for Marketing Decision Making BUSN 37107 Experimental Market BUSN 37902 Foundations of Advanced Quantitative Marketing BUSN 37904/ECON 40902 Advanced Quantitative Marketing BUSN 37906 Applied Bayesian Econometrics BUSN 37907 Behavioral Science Research Methods in Marketing BUSN 40206 Healthcare Business Analytics BUSN 40721 Healthcare Analytics Lab BUSN 41000 Business Statistics BUSN 41100 Applied Regression Analysis BUSN 41201 Big Data BUSN 41203 Financial Econometrics BUSN 41305 Statistical Insight into Entrepreneurial Quantitative Consulting with Wide Business Applications BUSN 41600/ECPN 51400 Econometrics and Statistics Colloquium BUSN 41901/STAT 32400 Probability and Statistics BUSN 41902/STAT 32900 Statistical Inference BUSN 41903 Applied Econometrics BUSN 41910/STAT 33500 Time Series Analysis for Forecasting and Model Building BUSN 41916 Bayes, AI and Deep Learning BUSN 41917 Causal Machine Learning BUSN 41918 Data, Learning, and Algorithms
Comparative Human Development
CHDV 30102/MACS 50100/PBHS 43201/SOCI 30315/STAT 31900 Introduction to Causal Inference
Committee on Clinical and Transnational Science (CCTS)
CCTS 40500/CCTS 20500/BIOS 29208 Machine Learning & Advanced Analytics in Science
ECMA 31000 Introduction to Empirical Analysis I ECMA 31100 Introduction to Empirical Analysis II ECMA 31210 Time Series Analysis for Macroeconomics and Finance ECMA 31350 Machine Learning for Economists ECMA 31360 Causal Inference ECMA 33220 Introduction to to Advanced Macroeconomic Analysis ECMA 33221 Intro to Advanced Macroeconomic Analysis II ECON 31000 Empirical Analysis I ECON 31100 Empirical Analysis II ECON 31200 Empirical Analysis III ECON 31715 Econometrics with Partial Identification ECON 31720 Applied Microeconometrics ECON 31740/PPHA 48403 Optimization-Conscious Econometrics ECON 33550 Spatial Economics ECON 35550/PPHA 35561/ECMA 35550 The Practicalities of Running Randomized Control Trials
Social Sciences Division
MACS 33002/MAPS 33002 Introduction to Machine Learning MACS 40101/SOCI 40248 Social Network Analysis MACS 30519/SOCI 30519/ GEOG 30519/SOCI 20519/ENST 20519/GEOG 20519 Spatial Cluster Analysis MACS 60000/SOCI 40133/CHDV 30510 Computational Content Analysis MAPS 30289 Intermediate Regression and Data Science MAPS 31755 Longitudinal Research MAPS 31850 Survey and Experimental Methods in Political Science SOSC 36006 Foundations for Statistical Theory SOSC 36007 Overview of Quantitative Methods in Social and Behavioral Sciences SOSC 36008 Principles and Methods of Measurement
Public Health Sciences
PBHS 30910/STAT 22810/PPHA 36410/ENST 27400/BIOS 27810 Epidemiology and Population Health PBHS 31001/STAT 35700 Epidemiologic Methods PBHS 31100 Introduction to Mathematical Modeling in Public Health PBHS 31300/ BIOS 25419 Introduction to Infectious Disease Epidemiology PBHS 32100/ CCTS 45000 Introduction to Biostatistics PBHS 32410/STAT 22401 Regression Analysis for Health and Social Research PBHS 32700/STAT 22700 Biostatistical Methods PBHS 32901/STAT 35201 Introduction to Clinical Trials PBHS 33300/ STAT 36900 Applied Longitudinal Data Analysis PBHS 33400/CHDV 32401 Multilevel Modeling PBHS 33500/STAT 35800/CHDV 32702 Statistical Applications PBHS 34500 Machine Learning for Public Health PBHS 35100/HLTH 29100/PPHA 38010/SSAD 46300 Health Services Research Methods PBHS 40500 Advanced Epidemiologic Methods PBHS 43010/STAT 35920 Applied Bayesian Modeling and Inference
Political Science
PLSC 30500 Introduction to Quantitative Social Sciences PLSC 30600 Causal Inference PLSC 30700 Introduction to Linear Models PLSC 30901 Game Theory 1 PLSC 31000 Game Theory 2 PLSC 40502 Data Analysis with Statistical Models PLSC 40601 Advanced Topics in Causal Inference PLSC 40815/PBPL 40815/PECO 40815/PPHA 40815 New Directions in Formal Theory PLSC 48401/PPHA 39830 Quantitative Security PLSC 57200/SOCI 50096 Network Analysis
Harris School of Public Policy
PBPL 26400 Quantitative Methods in Public Policy PPHA 30545 Machine Learning PPHA 30562 Telling Stories with Data Visualization PPHA 31002 Statistics for Data Analysis I PPHA 31102 Statistics for Data Analysis II: Regressions PPHA 31202 Advanced Statistics for Data Analysis I PPHA 31302 Advanced Statistics for Data Analysis II PPHA 34600 Program Evaluation PPHA 35577 Big Data and Development PPHA 38520 GIS Applications for Public Policy PPHA 41300 Cost Benefit Analysis PPHA 41600 Survey Research Methodology PPHA 41800/PSYC 47500 Survey Questionnaire Design PPHA 42000 Applied Econometrics I PPHA 42100 Applied Econometrics II PPHA 42200 Applied Econometrics III PPHA 44900/ PBPL 28550 Methods Of Data Collection: Social Experiments, Quasi-Experiments and Surveys
PSYC 20250/EDSO 20250/ENST 20250 Introduction to Statistical Concepts and Methods PSYC 26010 Big Data in the Psychological Sciences PSYC 37300 Experimental Design and Statistical Modeling I PSYC 37900 Experimental Design and Statistical Modeling II PSYC 46050 Principles of Data Science and Engineering for Laboratory Research
SOCI 20004/30004 Statistical Methods of Research 1 SOCI 30005 Statistical Methods of Research 2 SOCI 30112 Applications of Hierarchical Linear Models SOCI 30253/MACS 54000 Introduction to Spatial Data Science SOCI 40103 Event History Analysis SOCI 40258 Causal Mediation Analysis SOCI 50132 Seminar: Causal Inference in Studies of Educational Interventions
STAT 22000 Statistical Models and Applications STAT 22200 Linear Models and Experimental Design STAT 22400/PBHS 32400 Applied Regression Analysis STAT 23400 Statistical Models and Methods I STAT 24400 Statistical Theory and Methods I STAT 24410/STAT 30030 Statistical Theory and Methods Ia STAT 24500 Statistical Theory and Methods II STAT 24510/30040 Statistical Theory and Methods IIa STAT 24620/32950 Multivariate Statistical Analysis: Applications and Techniques STAT 24630 Causal Inference Methods and Case Studies STAT 25100 Introduction to Mathematical Probability STAT 25300/31700 Introduction to Probability Models STAT 26100/33600 Time Dependent Data STAT 27410 Introduction to Bayesian Data analysis STAT 27700/CMSC 25300 Mathematical Foundations of Machine Learning STAT 27850/30850 Multiple Testing, Modern Inference, and Replicability STAT 27855 Hypothesis Testing with Empirical Bayes Methodology STAT 30100 Mathematical Statistics-1 STAT 30200 Mathematical Statistics-2 STAT 30400 Distribution Theory STAT 30750/24300 Numerical Linear Algebra STAT 30810 High Dimensional Time Series Analysis STAT 31150/CAAM 31150 Inverse Problems and Data Assimilation STAT 31200 Introduction to Stochastic Processes I STAT 31511 Monte Carlo Simulation STAT 33100 Sample Surveys STAT 33910/FINM 33170 Financial Statistics: Time Series, Forecasting, Mean Reversion, and High Frequency Data STAT 34300 Applied Linear Statistical Methods STAT 34800 Modern Methods in Applied Statistics STAT 35450/HGEN 48600 Fundamentals of Computational Biology: Models and Inference STAT 34700 Generalized Linear Models STAT 37711/CAAM 37711/DATA 37711 Machine Learning 1
Course Descriptions
BUSN 36906-50 Stochastic Processes (Fall) No description available. PhD students only
BUSN 37103 Data Driven Marketing (Spring) Rapid advances in information technology during the last decades have enabled firms to create and analyze large databases of customer interactions and transactions. Data-driven marketing is an approach to implement marketing decisions based on a statistical analysis of big data to improve the profitability of marketing using ROI metrics. The class is designed to provide a broad overview of data-driven marketing techniques. In the first part of the class we study methods to measure store and market level demand using demand models. Applications include base-price optimization, data-driven price discrimination, and promotions management. We also study the measurement of short-run and long-run effects of advertising. In the second part of the class we cover customer relationship management (CRM) and database marketing. We introduce a general framework to implement customer-level targeting using predictive modeling based on customer lifetime value and return on investment (ROI) predictions. We apply this framework to customer development, retention, and acquisition decisions. The final part of the class focuses on digital marketing and how to predict the effectiveness and profitability of display and search advertising. Throughout the class we make use of statistical tools, including regression analysis and logistic regression. In particular, all assignments and the take-home final involve practical applications of the concepts covered in class using data and methods implemented in the R statistical computing language. Prerequisites: Business 37000 or 37100: strict, and 41000 (or 41100). Cannot enroll in 37103 if 37105 taken previously: strict.
BUSN 37105 Data Science for Marketing Decision Making (Fall) Marketing decisions in the era of big data are increasingly based on a statistical analysis of large amounts of transaction and customer data that provides the basis for profitability and ROI predictions. The goal of this class is to introduce modern data-driven marketing techniques and train the students as data scientists who can analyze data and make marketing decisions using some of the state-of-the-art tools that are employed in the industry. We will cover a wide range of topics, including demand modeling, the analysis of household-level data, customer relationship management (CRM) and database marketing, and elements of digital marketing. The focus throughout is on predicting the impact of marketing decisions, including pricing, advertising, and customer targeting, on customer profitability and the return on investment (ROI) from a customer interaction. The students will get immersed in a workflow that begins with the initial processing of the raw data and ends with the implementation of the marketing decision. First, we will learn how to manage and process large databases. The tools that we will use include SQL and some key packages in R that are designed for big data processing. Second, we will discuss and apply some modern statistical tools building on regression analysis, including Bayesian hierarchical models and some key tools from the machine learning literature. Finally, we will learn how to implement key marketing decisions based on the statistical analysis of the data. Note: The broad set of topics in this class overlaps with the topics covered in 37103 (Data-Driven Marketing). However, we will cover these topics at a faster pace and emphasize state-of-the-art techniques that are only briefly surveyed or not covered in 37103. Also, the main goal of the data assignments in 37103 is to make the students familiar with some key concepts in data-driven marketing. This class goes above and beyond this goal and introduces the students to a professional data scientist’s workflow used for marketing decision-making. Prerequisite: Business 37000 and 41000 (or 41100). Cannot enroll in 37105 if 37103 taken previously: strict.
BUSN 37107 Experimental Market (Winter) Traditional marketing tools, such as surveys and transactional data, are widely used to monitor ongoing marketing activities and to course-correct within a given marketing strategy. However, making decisions about changes in marketing strategy requires predicting how consumers will behave in a different market context than the one that currently exists. This course covers the use of experimental methods to quantify the causal effect of marketing decisions. Experimental methods have been used since the early days of marketing in settings such as retail test-markets and direct mail. In recent years, technological change, particularly the proliferation of online A/B testing, has fundamentally altered the methods and benefits of marketing experimentation. This course will cover the fundamentals of conducting marketing experiments and students will learn how to incorporate experimental results into managerial decision making. In particular, we will discuss: 1. The kinds of decisions for which experimental tests are most beneficial compared to alternative approaches. 2. How to design experiments, taking into account factors including cost, sample size and effective treatment rates, analytic complexity, modeling and decision needs, potential information leakage and other sources of bias, and customer reaction. 3. How to statistically analyze experimental results to draw valid conclusions that will generalize reliably to the decisions being made, from basic tools to more advanced methods for complex experimental settings 4. How to incorporate experimental results into decision making, including issues in buy-in for experimentation, identifying and managing threats to internal and external validity, and incorporating experimentation into long-term knowledge-building. The course will use a combination of lectures, case studies, hands-on exercises and an exam. Students will learn the tools needed to implement experimental methods in practice, from lab and survey-based experiments and concept tests to in-store, online and direct-communication field testing. We will discuss cases in which experimentation changed the way organizations made decisions, drawing on examples from advertising, online sales, consumer packaged-goods, consumer finance, fundraising and government. Prerequisites: Business 37000 (Marketing Strategy) and 41000/41100 (Statistics) or equivalent are required (strict), but can be taken concurrently. We will use statistical significance testing and regression analysis throughout the course. While we will review the basics of using these methods in our context, prior experience with statistical data analysis is important. Students who have not taken 41000 or 41100 must obtain the instructor’s approval to enroll. Students who are not enrolled in one of the Booth programs must obtain permission from the instructor to enroll in this class. No auditors.
BUSN 37902 Foundations of Advanced Quantitative Marketing (Winter) This course is meant for Ph.D. students with marketing as dissertation or minor area. The focus of the course is on understanding the methods currently available for analyzing panel data (household purchases, physician prescriptions, etc.). The course begins with an introduction to the various aspects of individual behavior and the econometric models currently available to study them. The remainder of the course focuses on specific advances in such analyses. These include, but are not limited to, the study of purchases across product categories, the analysis of dynamic purchase behavior and accounting for endogeneity in such models. Students will write code in R, Matlab or some similar software; no canned routines are allowed for PhD students. Note: PhD Students. MBA students with permission: strict
BUSN 37904/ECON 40902 Advanced Quantitative Marketing (Winter) This course covers some key topics at the research frontier in quantitative marketing. We formulate and estimate models of consumer decision-making, and then explore the normative and positive consequences of the inferred consumer behavior for optimal marketing decisions and market structure. Topics include: Foundations of demand modeling, measurement of consumer heterogeneity, the origin and evolution of preferences, state dependence in demand, dynamic discrete choice models, learning and memory models, storable goods demand, diffusion models and durable goods demand, stated choice models, advertising dynamics, and search and shopping behavior. This course is geared towards 2nd-year Ph.D. students who have already taken at least one course in Ph.D.-level Price Theory and in Ph.D.-level Empirical Economics.
BUSN 37906-50 Applied Bayesian Econometrics (Winter) This course will discuss applications of Bayesian methods to micro-econometric problems. We will particularly focus on issues pertaining to panel data models with unobserved heterogeneity and the use of hierarchical models to dealing with them. While the course is more generally useful, the applications and illustrations will be focused on Marketing and Industrial Organization. Prereq: PhD students only
BUSN 37907 Behavioral Science Research Methods in Marketing (Spring) This course will focus on both the philosophical and practical questions involved in conducting behavioral research for academic publication. We will discuss specific research methodologies, best practices and current controversies in research methods. The course assumes prior training in statistics, and the goal of the course is to bridge the gap between formal statistics and day-to-day research practice. The course will provide training in commonly used methods as well as develop your intuition for the logic underlying statistical practice, and when that logic is being violated. PhD students only. Non-Booth research master’s students require instructor permission.
BUSN 40206 Healthcare Business Analytics (Winter) In this class, you will learn how data analytics drives the business of healthcare . The course combines lecture and discussion with hands-on work with large, real-world healthcare datasets. You will learn the underlying logic and calculations of value-based hospital reimbursement, outcomes measurement, and benchmarking, working directly with patient-level claims datasets from CMS (Centers for Medicare and Medicaid Services) and elsewhere. Students interested in the Healthcare Analytics Laboratory (Bus 40721) are strongly encouraged to enroll in this class. While this course is designed to complement the Healthcare Analytics Lab, it is a standalone offering and can be taken independently. Students seeking exposure to healthcare data analytics who are unable to make the commitments the Lab requires will find it useful preparation for future endeavors. PREREQUISITES: Basic statistics and introductory exposure to R programming. (The later can be achieved through various online introductory tutorials, if needed.) Students will not be required to write code, but occasionally you will be required to run or slightly edit pieces of code provided by the instructor. Almost all numerical work will be conducted using “point-and-click” recipes in Data Science Studio by Dataiku . Thus, students should have a willingness and interest in learning powerful new software tools and working with real data to transform it into usable management insights. All Non-Booth students require instructor approval: strict. Cannot enroll in 40206 if you have taken 40205 or 40201: strict.
BUSN 40721 Healthcare Analytics Lab (Winter) The healthcare industry is now undergoing a transformation as data analysis is being rapidly deployed to improve clinical, operational, and financial outcomes. The Healthcare Analytics Laboratory will focus on applying data-driven analytics and insights to identify and create healthcare delivery efficiencies. Student teams will work on real-world improvement projects with prominent healthcare institutions. The Laboratory provides students with opportunities to: 1. Apply and reinforce tools and frameworks developed elsewhere in the Booth curriculum 2. Develop leadership skills and build effectiveness in teams; 3. Learn a healthcare context deeply through an intensive project experience 4. Develop proficiency at presenting data analyses to executive audiences 5. Impact real-world healthcare delivery. Thus, the course will help students develop the skills required to successfully deliver evidence-based management analytics in the real world. Projects will be carefully scoped, and most data will be acquired, before the course begins so that students can make steady progress towards clear, attainable goals. Students will present milestones every two weeks to the instructional team (which consists of the faculty instructor and graduate students who serve as project mentors) and, on occasion, to the entire class. Final presentations will be delivered to hospital executives and physician leaders. The course is for students interested in leveraging the academic rigor of data and decision analysis to improve healthcare delivery. It is an excellent course for those interested in careers in or related to the healthcare industry, and business analytics more broadly. PhD students only. Non-Booth research master’s students require instructor permission.
BUSN 41000 Business Statistics (Fall/Winter/Spring) Data science. Machine learning. Statistics. Predictive Analytics. No matter what it’s called, modern business runs on data. This course is an introduction to the fundamentals of probability and statistics with an aim towards building foundational skills in modern data science. Topics to be covered include 1) Exploratory data analysis and descriptive statistics, 2) Basic probability, common pitfalls and fallacies, 3) Statistical modeling, inference, p-values, and A/B testing, 4) Prediction, regression, and classification, 5) Ethics and privacy in data analysis. Emphasis will be placed on developing sound statistical reasoning and real-world applications and case studies.
BUSN 41100 Applied Regression Analysis (Fall) This course is about regression, a powerful and widely used data analysis technique wherein we seek to understand how different random quantities relate to one another. Students will learn how to use regression to analyze a variety of complex real world problems, with the aim of understanding data and prediction of future events. Focus is placed on understanding of fundamental concepts, development of the skills necessary for robust application of regression techniques, and their implementation in a statistical programming language (R, MATLAB, or an alternative). Examples are used throughout to illustrate application of the tools. Topics covered include: (i) short review of simple linear regression; (ii) multiple regression (understanding the model, inference and interpretation for parameters, model building and selection, diagnostics and prediction); (iii) generalized linear models (e.g. logistic regression); (iv) time series models (autocorrelation functions, auto-regression, prediction); (v) time permitting, panel data models and causal inference. Prereq: Business 41000 or familiarity with the topics covered in Business 41000. This course is only for students with a solid background in statistics and preferably some prior exposure to linear regression.
BUSN 41201 Big Data (Spring) BUS 41201 is a course about data mining: the analysis, exploration, and simplification of large high-dimensional datasets. Students will learn how to model and interpret complicated `Big Data’ and become adept at building powerful models for prediction and classification. Techniques covered include an advanced overview of linear and logistic regression, model choice and false discovery rates, multinomial and binary regression, classification, decision trees, factor models, clustering, the bootstrap and cross-validation. We learn both basic underlying concepts and practical computational skills, including techniques for analysis of distributed data. Heavy emphasis is placed on analysis of actual datasets, and on development of application specific methodology. Among other examples, we will consider consumer database mining, internet and social media tracking, network analysis, and text mining. Prereq: Bus 41000 (or 41100). Cannot enroll in BUSN 41201 if BUSN 20800 taken previously.
BUSN 41203 Financial Econometrics (Winter) This course covers a variety of topics in financial econometrics. The topics covered are of real- world, practical interest and are closely linked to material covered in other advance finance courses. Topics covered include ARMA models, volatility models (GARCH), factor models, models for time varying correlations, analysis of panel data, cointegration models for long-run co-movement between prices and models for transactions data and the analysis of transactions cost. Prereq: Business 41000 (or 41100), or instructor consent. Cannot enroll in BUSN 41203 if BUSN 20820 taken previously.
BUSN 41204 Machine Learning (Winter) Students will learn about state-of-the-art machine learning techniques and how to apply them in business related problems. Techniques will be introduced in the context of business applications and the emphasis will be put on how machine learning can be used to create value and provide insights from data. First, and the biggest, part of the class will focus on predictive analytics. Students will learn about decision trees, nearest neighbor classifiers, boosting, random forests, deep neural networks, naive Bayes and support vector machines. Among other examples, we will apply these techniques to detecting spam in email, click-through rate prediction in online advertisement, image classification, face recognition, sentiment analysis and churn prediction. Students will learn what techniques to apply and why. In the second part of the class, students will learn about unsupervised techniques for extracting actionable patterns from data. Examples include clustering, collaborative filtering, probabilistic graphical modelling and dimension reduction with applications to customer segmentation, recommender systems, graph and time series mining, and anomaly detection. Prereq: Bus 41100. Cannot enroll in BUSN 41204 if BUSN 20810 taken previously.
BUSN 41305 Statistical Insight into Entrepreneurial Quantitative Consulting with wide Business Applications (Fall) You decide to establish a start-up in marketing consulting. You search the Internet and find to your dismay well over 650 companies in that area, each one claiming to be best and unique. In order to compete in this arena you need to have the ability to identify upcoming trends and new problems in the marketing area, AND to be able to provide original, sound, fast and applicable solutions to these problems. One such example that is not dealt by many of the marketing consulting companies is the following shelf-planning problem. Imagine a customer in a deli store on a Sunday morning intending to buy bagels. There are only two bagels on the shelf. What would you predict the person would do? Hurry up and buy the only remaining bagels before they are gone? Would he consider the two bagels as being the least fresh, touched and left by all former customers, and therefore decide to wait for a fresher batch? As a consultant to the store manager, how would you determine the optimal number of bagels that should be on the shelf at a given time in order to avoid making customers reluctant to buy? As it turns out, the methodology covered by this course, that solves the above-mentioned problem, can also be used for the analysis of customer attrition, sale promotion and more. Unlike marketing research, marketing consulting is a problem-solving endeavor that requires a great deal of specificity and is fueled by experience. This course is meant to give future consultants and entrepreneurs important tools and ways of thinking that are relevant for dealing with insightful consulting and are useful in the practice of marketing consulting and beyond. The course addresses a variety of practical consulting problems and their solutions. Some examples are: (1) Optimal shelf-planning (see the bagels example above); (2) Analyzing customer attrition as a process (rather than as an event-driven phenomenon); (3) Prediction of a customer’s purchase behavior (buying intentions, buying propensity, etc.) from the customer’s patterns of usage of media, life style, political orientation, etc.; (4) Analysis of satisfaction -how to create a VALID satisfaction scale, how to rank products by satisfaction of customers, how to detect easy-to-please customers, etc.; (5) Analysis of brand loyalty -how to measure loyalty, how to determine whether loyalty to certain brands exists, and how to quantify it; (6) Optimizing predictive modeling when financial rewards and penalties exist in regard to correct and incorrect prediction, respectively. The course is taught in a way that emphasizes the interpretation of results rather than computations. Although this course uses statistical reasoning, it is NOT too mathematical in nature. To aid in the analysis, an interactive and user friendly R-based software containing innovative routines will be used in this course. There is no need of programming, or programming skills in this course – except the ability to use your finger to click a key. Prereq: Bus 41000 (OR 41100) is mandatory: strict . Students that did not take one of these courses but believe they have a strong background in statistics can still bid for the course given the explicit written permission of the instructor. Instructor consent required for non-Booth students.
BUSN 41600 Econometrics and Statistics Colloquium (Fall/Winter/Spring) Workshops in each academic area provide a forum for faculty, PhD students, and invited guests to present, discuss, and debate new research. Prereq: PhD students only. Instructor permission required for MBA students. BUSN 41600=ECON 51400.
BUSN 41901/STAT 32400 Probability and Statistics (Fall) This Ph.D.-level course (in addition to 41902) provides a thorough introduction to Classical and Bayesian statistical theory. The two-quarter sequence provides the necessary probability and statistical background for many of the advanced courses in the Chicago Booth curriculum. The central topic of Business 41901 is probability. Basic concepts in probability are covered. An introduction to martingales is given. Homework assignments are given throughout the quarter. Prereq: One year of calculus; BUSN 41901=STAT 32400
BUSN 41902/STAT 32900 Statistical Inference (Winter) This Ph.D.-level course is the second in a two-quarter sequence with Business 41901. The central topic is statistical inference using asymptotic approximations. We will cover linear regression models, generalized method of moments, time series. Time permitting; we will discuss factor models. Prereq: Business 41901
BUSN 41903 Applied Econometrics (Spring) This Ph.D.-level course covers a variety of techniques that are used in econometric analysis. The class builds heavily on material developed in 41902, and it is strongly recommended that students have taken 41902 or equivalent before enrolling in this course. Some topics that may be covered are (i) heteroscedasticity and correlation robust inference methods including HAC, clustering, bootstrap methods, and randomization inference; (ii) causal inference methods including instrumental variables estimation, difference-in-differences estimation, and estimators of treatment effects under treatment effect heterogeneity; (iii) an introduction to nonparametric and high-dimensional statistical methods. Prereq: Business 41901 and 41902.
BUSN 41910/ STAT 33500 Time-series Analysis for Forecasting and Model Building (Winter) Forecasting plays an important role in business planning and decision-making. This Ph.D.-level course discusses time series models that have been widely used in business and economic data analysis and forecasting. Both theory and methods of the models are discussed. Real examples are used throughout the course to illustrate applications. The topics covered include: (1) stationary and unit-root non-stationary processes; (2) linear dynamic models, including Autoregressive Moving Average models; (3) model building and data analysis; (4) prediction and forecasting evaluation; (5) asymptotic theory for estimation including unit-root theory; (6) models for time varying volatility; (7) models for time varying correlation including Dynamic Conditional Correlation and time varying factor models.; (9) state-space models and Kalman filter; and (10) models for high frequency data. Prereq: Business 41901 or instructor consent. BUSN 41910=STAT 33500
BUSN 41914/STAT 33700 Multivariate Time Series Analysis (Winter) This course investigates the dynamic relationships between variables, including analysis of large scale dependent data. It starts with linear relationships between two variables, including distributed-lag models and detection of unidirectional dependence (Granger causality). The dynamic models discussed include vector autoregressive models, vector autoregressive moving-average models, multivariate regression models with time series errors, co-integration and error-correction models, dynamic factor models, and multivariate volatility models. The course also addresses classification (or clustering) of large scale time series, principal component analysis, asymptotic principal component analysis, online recursive estimation, deep neural networks, and machine learning for dependent data. Empirical data analysis is an integral part of the course. Students are expected to analyze many real data sets. Finally, the course discusses forecasting under the current data-rich environment. The main software used in the course includes the MTS and SLBDD packages in R, but students may use their own software if preferred. Prerequisites: Business 41910 or equivalent course on univariate time series analysis. MBA/Masters students must have prereq or instructor permission: strict.
BUSN 41916 Bayes, AI, and Deep Learning (Fall) This course focuses on the applications of data analytic, machine learning and deep learning methods. We will start with a quick review of basic Bayesian models followed by tools and concepts from artificial intelligence. Students will learn how to use deep learning to analyze a variety of complex real world problems. Numerous empirical examples from finance, internet analytics, and sports are used to illustrate the material covered. Google’s development of deep neural networks and applications will be discussed in detail. Emphasis will be placed on understanding concepts of Bayes, AI and Deep Learning. The three main topics covered are: (i) Bayesian methods including conditional probability, hierarchical models (ii) Artificial Intelligence including modern regression methods such as lasso and ridge regression. Dimensionality reduction techniques and sparsity are central to data analysis (ii) Deep Learning including Neural Nets, Architecture design, Stochastic Gradient Descent, speeding up convergence. Throughout business and internet applications including machine intelligence, reinforcement learning, image and speech recognition will be used to illustrate the wide range of applications.
BUSN 41917 Causal Machine Learning (Fall) This course will bring students to the cutting edge in causal inference, giving them a solid theoretical understanding and ready-to-deploy tools for research. Using machine learning for estimation and inference of treatment effects has become an important part of modern academic economics. Students in this class will learn the theoretical underpinnings of this material as well as how to carefully and correctly apply the techniques in research. The course will prepare students for both theoretical and applied dissertation research. Each topic will be covered for two weeks, one covering theory and one covering application. Topics will include the basics of causal inference, nonparametric estimation, semiparametric inference, and double machine learning.
BUSN 41918 Data, Learning, and Algorithms (Winter) This Ph.D. level course will provide an overview of machine learning and its algorithmic paradigms, and explore recent topics on learning, inference, and decision-making in the presence of large data sets. Emphasis will be made on theoretical insights and algorithmic principles. Prereq: This is a Ph.D.-level course for students with strong quantitative and mathematical backgrounds. Basic graduate-level probability and statistics classes as prerequisites are recommended. Students should be comfortable with probability theory, statistics, numerical linear algebra, and basic knowledge of continuous optimization. MBA students require instructor permission: strict.
BUSN 40206 Healthcare Business Analytics (Fall) One of the today’s most exciting and important applications of Business Analytics is Healthcare, thanks to the rise of Data Science and the Patient Protection and Affordable Care Act. Every day, more data on provider performance is becoming available to consumers to help them make better informed decisions about their healthcare. Hospital revenues are being driven more and more by clinical results through incentive programs for improving hospital readmissions, patient safety, costs, and patient outcomes. At the same time, population health is improving as Big Data is being used to learn what treatments are most effective at an unprecedented pace and scale. These forces are transforming the healthcare industry and public health.
In this class, you will learn how data analytics drives the business of healthcare . The course combines lecture and discussion with hands-on work with large, real-world healthcare datasets. You will learn the underlying logic and calculations of value-based hospital reimbursement, outcomes measurement, and benchmarking, working directly with patient-level claims datasets from CMS (Centers for Medicare and Medicaid Services) and elsewhere.
Students will use state-of-the-art commercial software tools that permit data preparation and collaboration on datasets too large to work with efficiently using spreadsheets. Data manipulation and analyses will be done using a combination of both point-and-click recipes and pre-prepared analysis scripts in the statistical software package R. By the end of the course, students will be prepared to conduct and/or participate in a real-world data analysis project at a healthcare institution or a consultancy.
Students interested in the Healthcare Analytics Laboratory (Bus 40721) are strongly encouraged to enroll in this class. While this course is designed to complement the Healthcare Analytics Lab, it is a standalone offering and can be taken independently. Students seeking exposure to healthcare data analytics who are unable to make the commitments the Lab requires will find it useful preparation for future endeavors.
CCTS 40500/CCTS 20500/BIOS 29208 Machine Learning & Advanced Analytics in Science (Winter) The age of ubiquitous data is rapidly transforming scientific research, and advanced analytics powered by sophisticated learning algorithms is uncovering new insights in complex open problems in biology and biomedicine. The goal of this course is to provide an introductory overview of the key concepts in machine learning, outlining the potential applications in biomedicine. Beginning from basic statistical concepts, we will discuss concepts and implementations of standard and state of the art classification and prediction algorithms, and go on to discuss more advanced topics in unsupervised learning, deep learning architectures, and stochastic time series analysis. We will also cover emerging ideas in data-driven causal inference, and demonstrate applications in uncovering etiological insights from large scale clinical databases of electronic health records, and publicly available sequence and omics datasets. The acquisition of hands-on skills will be emphasized over machine learning theory. On successfully completing the course, students will have acquired enough knowledge of the underlying machinery to intuit and implement solutions to non-trivial data science problems arising in biology and medicine. Prerequisite(s): Rudimentary knowledge of probability theory, and basic exposure to scripting languages such as python/R is required. This course does not qualify in the Biological Sciences major. Equivalent Course(s): CCTS 20500, BIOS 29208
CHDV 30102/MACS 50100/PBHS 43201/SOCI 30315/STAT 31900 Introduction to Causal Inference. (Winter) This course is designed for graduate students and advanced undergraduate students from the social sciences, education, public health science, public policy, social service administration, and statistics who are involved in quantitative research and are interested in studying causality. The goal of this course is to equip students with basic knowledge of and analytic skills in causal inference. Topics for the course will include the potential outcomes framework for causal inference; experimental and observational studies; identification assumptions for causal parameters; potential pitfalls of using ANCOVA to estimate a causal effect; propensity score based methods including matching, stratification, inverse-probability-of-treatment-weighting (IPTW), marginal mean weighting through stratification (MMWS), and doubly robust estimation; the instrumental variable (IV) method; regression discontinuity design (RDD) including sharp RDD and fuzzy RDD; difference in difference (DID) and generalized DID methods for cross-section and panel data, and fixed effects model.Intermediate Statistics or equivalent such as STAT 224/PBHS 324, PP 31301, BUS 41100, or SOC 30005 is a prerequisite. This course is a prerequisite for “Advanced Topics in Causal Inference” and “Mediation, moderation, and spillover effects.” (=MACS 5100, =PBHS 43201, =PLSC 30102, =SOCI 30315, =STAT 31900). PQ: Intermediate Statistics or equivalent such as STAT 224/PBHS 324, PP1301, BUS 41100, or SOC 30005 .
CHDV 32411/ CCTS 32411/PBPL 29411/PSYC 32411/SOCI 30318/STAT 33211 Mediation, Moderation, and Spillover Effects (Spring) This course is designed for graduate students and advanced undergraduate students from social sciences, statistics, health studies, public policy, and social services administration who will be or are currently involved in quantitative research. Research questions about why an intervention works, for whom, under what conditions, and whether one individual’s treatment could affect other individuals’ outcomes are often key to the advancement of scientific knowledge yet pose major analytic challenges. This course introduces cutting-edge theoretical concepts and methodological approaches with regard to mediation of intervention effects, moderated intervention effects, and spillover effects in a variety of settings. The course content is organized around six case studies. In each case, students will be involved in critical examinations of a working paper currently under review. Background readings will reflect the latest developments and controversies. Weekly labs will provide supplementary tutorials and hands-on experiences with mediation and moderation analyses. All students are expected to contribute to the knowledge building in class through participation in discussions. Students are encouraged to form study groups, while the two written assignments are to be finished and graded on an individual basis.
ECMA 31000 Introduction to Empirical Analysis (Fall) This course introduces students to the key tools of econometric analysis: Probability theory, including probability spaces, random variables, distributions and conditional expectation; Asymptotic theory, including convergence in probability, convergence in distribution, continuous mapping theorems, laws of large numbers, central limit theorems and the delta method; Estimation and inference, including finite sample and asymptotic statistical properties of estimators, confidence intervals and hypothesis testing; Applications to linear models, including properties of ordinary least squares, maximum likelihood and instrumental variables estimators; Non-linear models. Assignments will include both theoretical questions and problems involving data. Necessary tools from linear algebra and statistics will be reviewed as needed. Prereq for Undergraduates : Econ 21030 or Econ 21110 or Econ 21130
ECMA 31100 Introduction to Empirical Analysis II (Spring) This course is an introduction to applied econometrics and builds on tools studied in ECMA 31000. Topics include: Selection on observables, instrumental variables, time series, panel data, discrete choice models, regression discontinuity, nonparametric regression, quantile regression.
ECMA 31130 Topics in Microeconometrics (Fall) This course focuses on micro-econometric methods that have applications to a wide range of economic questions. We study identification, estimation, and inference in both parametric and non-parametric models and consider aspects such as consistency, bias and variance of estimators. We discuss how repeated measurements can help with problems related to unobserved heterogeneity and measurement error, and how they can be applied to panel and network data. Topics include duration models, regressions with a large number of covariates, non-parametric regressions, and dynamic discrete choice models. Applications include labor questions such as labor supply, wage inequality decompositions and matching between workers and firms. Students will be expected to solve programming assignment in R. Prereq for Undergraduates : ECON 21020 OR ECON 21030
ECMA 31210 Time Series Analysis for Macroeconomics and Finance (Spring) This course will cover various methods and their applications in time series analysis and emphasize empirical exercises by students. The structure of the course starts with theoretical foundations drawing from standard textbooks of Hayashi (2000) and Hamilton (1994) and covers applications to answer important questions in macro and finance. The topics include time series OLS with applications in the Fama interest rate regression and Hansen’s study of foreign exchange markets, GMM with the Fama-French model of equity returns, and state-space models with applications to GDP nowcasting. Familiarity with matrix algebra and elementary econometrics is required.
Prerequisite(s): Prerequisites for Undergraduates: ECON 20200/20210 and ECON 21020/21030 Note(s): This course may count as a data science course for the data science specialization in the same set of options as ECON 21300, ECMA 31320, ECMA 31330, ECMA 31340, ECMA 31350 or ECMA 38010.
ECMA 31320 Applications of Econometric and Data Science Methods (Spring) This course builds on the theoretical foundations set in Econ 21030 and explores further topics pertinent to modern economic applications. While the course content may change from year to year according to student and instructor interests, some potential topics are panel data methods, treatment effects/causal inference, discrete choice/limited dependent variable models, demand estimation, and topics in economic applications of supervised and unsupervised learning algorithms. The course will involve analytically and computationally intensive assignments and a significant empirical project component.
ECMA 31340 Big Data Tools in Economics (Fall) The goal of the class is to learn how to apply microeconomic concepts to large and complex datasets. We will first revisit notions such as identification, inference and latent heterogeneity in classical contexts. We will then study potential concerns in the presence of a large number of parameters in order to understand over-fitting. Throughout the class, emphasis will be put on project-driven computational exercises involving large datasets. We will learn how to efficiently process and visualize such data using state of the art tools in python. Topics will include fitting models using Tensor-Flow and neural nets, creating event studies using pandas, solving large-scale SVDs, etc. Prereq for Undergraduates : ECON 20100/20110 and ECON 21020/21030
ECMA 31350 Machine Learning for Economists (Winter) New Course – Description Coming Soon
ECMA 33220 Introduction to Advanced Macroeconomic Analysis (Fall) This course introduces students to advanced methods for macroeconomic analysis. In the first part, we discuss time series methods such as impulse response analysis, vector autoregression, co-integration, shock identification, and business cycle detrending. In the second part, we examine and analyze a simple, yet powerful stochastic dynamic real business cycle model. In that context, the students will learn about dynamic programming, rational expectations, intertemporal optimization, asset pricing, the Frisch elasticity of labor supply, log-linearization, and computational tools to solve for the recursive law of motion of dynamic stochastic general equilibrium models. Finally, we touch upon some further models, such as the overlapping generations model and/or the continuous-time neoclassical growth model. The course is useful for students interested to deepen their knowledge in macroeconomics, in order to read, understand, and replicate some of the recent research in the field; as preparation for careers involving macroeconomic analysis, time series analysis, or asset pricing; or as preparation for graduate school. Decent knowledge of linear algebra and calculus is required. All advanced material will be taught in class.
ECMA 33221 Intro to Advanced Macroeconomic Analysis II (Winter) New Course – Description Coming Soon
ECON 31000 Empirical Analysis I (Fall) This course introduces students to the key tools of econometric analysis. It covers basic OLS regression model, generalized least squares, asymptotic theory and hypothesis testing for maximum likelihood estimation, extremum estimators, instrumental variables, decision theory and Bayesian inference.
ECON 31100 Empirical Analysis II (Winter) This course develops methods of analyzing Markov specifications of dynamic economic models. Models with stochastic growth are accommodated and their properties analyzed. Methods for identifying macroeconomic shocks and their transmission mechanisms are developed. Related filtering methods for models with hidden states are studied. The properties estimation and inference methods based on maximum likelihood and generalized method of moments are derived. These econometric methods are applied to models from macroeconomics and financial economics.
ECON 31200 Empirical Analysis III (Spring) The course will review some of the classical methods you were introduced to in previous quarters and give examples of their use in applied microeconomic research. Our focus will be on exploring and understanding data sets, evaluating predictions of economic models, and identifying and estimating the parameters of economic models. The methods we will build on include regression techniques, maximum likelihood, method of moments estimators, as well as some non-parametric methods. Lectures and homework assignments will seek to build proficiency in the correct application of these methods to economic research questions.
ECMA 31360 Causal Inference (Fall, Winter, Spring) This course reviews modern causal inference techniques and their applications in business and economics. The course covers the treatment-control comparison estimator, regression adjustment, matching (on covariates and propensity score), difference in differences (canonical and with staggered treatment), panel data methods, regression discontinuity design (sharp and fuzzy), instrumental variables and local average treatment effect (LATE) estimator. At different points during the course, we mention how machine learning (ML) techniques have recently been used to enrich the classical methods. The course involves programming (R language) and working with data. Students are expected to have a solid background in statistics (working knowledge of R and familiarity with RStudio) and econometrics. Prerequisite(s): ECON 21020/21030
ECON 31715 Econometrics with Partial Identification (Fall) One of the main new ideas in econometrics over the last three decades was that point identification is unnecessary to draw informative conclusions from the available data. Indeed, in many settings, reasonable modeling assumptions naturally deliver a set of parameter values consistent with the data. Then, one is tasked with (1) obtaining a tractable characterization of the identified set; (2) providing methods to estimate it; and (3) making confidence statements about partially identified parameters. This course covers modern approaches to solving the above problems, focusing on tractability and implementation. It provides some theoretical background, develops the necessary statistical toolkit, and reviews a number of empirical papers that apply the partial identification approach in practice. The course will be of interest to students in econometrics, applied microeconomics, and industrial organization.
ECON 31720 Applied Microeconometrics (Spring) This course is about empirical strategies that are commonly used in applied microeconomics. The topics will include: control variables (matching), instrumental variables, regression discontinuity and kink designs, panel data, difference-in-differences, and quantile regression. The emphasis of the course is on identification and practical implementation. The course also covers the shortcomings of commonly used tools, and discusses recent theoretical research aimed at addressing these deficiencies.
ECON 31703 Topics In Econometrics (Spring) Graduate course covering recent research on the field of econometrics. This course will introduce some current topics in econometrics and statistics with applications to the analysis of randomized experiments. The first half of the course will compare finite-population and super-population approaches to inference in classical randomized experiments. The second half of the course will focus on uniform laws of large numbers and VC theory, with a view towards policy learning in randomized experiments.
ECON 31740/PPHA 48403 Optimization-Conscious Econometrics (Winter) This course studies the core optimization concepts underlying econometric estimation and inference. The objective is to both develop a deep understanding of how estimators are computed, and to get a better theoretical and geometrical understanding of classical econometric estimators through the prism of optimization theory. Each optimization concept or method is studied using a well established econometric estimator as the working example: linear programming is taught through the example of quantile regression, duality is taught via nonparametric inference, numerical linear algebra is taught via partial identification questions in OLS, integer programming is taught as a solution method for instrumental variables quantile regression, and so on.
ECON 31750 Topics on the Analysis of Randomized Experiments (Winter) This course will introduce some current topics in econometrics and statistics with applications to the analysis of randomized experiments. The first half of the course will compare finite-population and super-population approaches to inference in classical randomized experiments. The second half of the course will focus on uniform laws of large numbers and VC theory, with a view towards policy learning in randomized experiments.
ECON 31760 Topics in Modern Econometrics (Spring) New Course – Description Coming Soon
ECON 33550 Spatial Economics (Winter) The course will discuss recent advances in spatial modelling and quantification that allow us to study trade, migration, as well as urban, regional, national, and global growth in a unified spatial general equilibrium framework. These frameworks can be quantified using a variety of data to perform detailed policy counter-factual exercises. These exercises can help us understand the impact of trade and migration policy as well as local and national fiscal policy, transportation policy and the effect of regional shocks including the ones associated with climate change.
ECON 35003 Human Capital, Markets, and the Family (Winter) This course examines the theory and evidence about inequality and social mobility (within and across generations)
ECON 35550/PPHA 35561/ECMA 35550 The Practicalities of Running Randomized Control Trials (Fall) This course is designed for those who plan to run a randomized control trial. It provides practical advice about the trade-offs researchers face when selecting topics to study, the type of randomization technique to use, the content of a survey instruments, analytical techniques and much more. How do you choose the right minimum detectable effect size for estimating the sample size needed to run a high quality RCT? How do you quantify difficult to measure outcomes such as women’s empowerment or ensure people are providing truthful answers when you are asking questions on sensitive topics like sexual health? When should you tie your hands by pre-committing to your analysis plan in advance, and when is a pre-analysis plan not a good idea? This course will draw on lots of examples from RCTs around the world, most (though not all) from a development context. Alongside field tips, it will also cover the concepts and theory behind the tradeoffs researchers face running RCTs. The course is designed for PhD students but given its practical nature is open to and accessible to masters students who plan to work on RCTs.
MACS 33002/MAPS 33002 Introduction to Machine Learning (Spring) This course requires Python programming experience. The course will train students to gain the fundamental skills of machine learning. It will cover knowledge and skills of of running with computational research projects from a machine learning perspective, including the key techniques used in standard machine learning pipelines: data processing (e.g., data cleaning, feature selection, feature engineering), classification models (e.g., logistic regression, decision trees, naive bayes), regression models (e.g., linear regression, polynomial regression), parameter tuning(e.g., grid-search), model evaluation (e.g., cross-validation, confusion matrix, precision, recall, and f1 for classification models; RMSE and Pearson correlation for regression models), and error analysis (e.g., data imbalance, bias-variance tradeoff). Students will learn simple and efficient machine learning algorithms for predictive data analysis as well as gain hands-on experience by applying machine learning algorithms in social science tasks. The ultimate goal of this course is to prepare students with essential machine learning skills that are in demand both in research and industry.
MACS 40101/SOCI 40248 Social Network Analysis (Spring) This course introduces students to concepts and techniques of Social Network Analysis (“SNA”). Social Network Analysis is a theoretical approach and a set of methods to study the structure of relationships among entities (e.g., people, organizations, ideas, words, etc.). Students will learn concepts and tools to identify network nodes, groups, and structures in different types of networks. Specifically, the class will focus on a number of social network concepts, such as social capital, homophily, contagion, etc., and on how to operationalize them using network measures, such as centrality, structural holes, and others.
MACS 30519/SOCI 30519/ GEOG 30519/SOCI 20519/ENST 20519/GEOG 20519 Spatial Cluster Analysis (Winter) This course provides an overview of methods to identify interesting patterns in geographic data, so-called spatial clusters. Cluster concepts come in many different forms and can generally be differentiated between the search for interesting locations and the grouping of similar locations. The first category consists of the identification of extreme concentrations of locations (events), such as hot spots of crime events, and the location of geographical concentrations of observations with similar values for one or more variables, such as areas with elevated disease incidence. The second group consists of the combination of spatial observations into larger (aggregate) areas such that internal similarity is maximized (regionalization). The methods covered come from the fields of spatial statistics as well as machine learning (unsupervised learning) and operations research. Topics include point pattern analysis, spatial scan statistics, local spatial autocorrelation, dimension reduction, as well as spatially explicit hierarchical, agglomerative and density-based clustering. Applications range from criminology and public health to politics and marketing. An important aspect of the course is the analysis of actual data sets by means of open source software, such as GeoDa, R or Python.
MACS 31300 AI Applications in the Social Sciences (Winter) Artificial Intelligence (AI) describes algorithms constructed to reason in uncertain environments. This course provides an introduction to AI applications in the social sciences. Driven by the rapid increase in accessible big data documenting social behavior, AI has been applied to: increase effective diagnosis and prediction under different conditions, improve our understanding of human interaction, and increase the effectiveness of data management in different social and human services. Random forests and neural networks are among the most frequent AI methods used for prediction, while natural language processing and computer vision contribute to understanding decision-making and improving service provision. We begin with careful consideration for what AI can achieve and where current limitations exist by looking at a variety of real-world applications. We will focus on three core sections: search, representation, and uncertainty. In each section, we will explore major approaches, representational techniques and core algorithms. We will examine the trade-offs between model structure and the algorithmic constraints that this structure implies. The course is driven by hands-on exercises with AI algorithms written in Python. At the end of the term, you should be able to apply and tweak these algorithms to accommodate your own data and research interests.
MACS 33002 – Introduction to Machine Learning (Winter) This course will train students to gain the fundamental skills of machine learning. It will cover everything needed for getting up and running with computational research projects from a machine learning perspective, including the key techniques used in standard machine learning pipelines: data processing (e.g., data cleaning, feature selection, feature engineering), classification models (e.g., logistic regression, decision trees, naive bayes), regression models (e.g., linear regression, polynomial regression), parameter tuning(e.g., grid-search), model evaluation (e.g., cross-validation, confusion matrix, precision, recall, and f1 for classification models; RMSE and Pearson correlation for regression models), and error analysis (e.g., data imbalance, bias-variance tradeoff). Students will learn simple and efficient machine learning algorithms for predictive data analysis as well as gain hands-on experience by applying machine learning algorithms in social science tasks. The ultimate goal of this course is to prepare students with essential machine learning skills that are in demand both in research and industry.
MACS 35130 Applied Multivariate Analysis For Social Scientists: An Overview With Latin American Data (Spring) This course introduces multivariate analysis methods applied to continuous and categorical data, providing an overview of Latin America’s harmonized data and/or country-specific data. It includes classical methods of regional analysis and various multivariate methods for summarizing data and spatial/non-spatial clustering. Students will be introduced to the application of multivariate techniques to health, crime, education, and employment issues. The course is primarily based on the open-source software R, but no previous knowledge of the software is required.
MACS 37000/SOCI 30332 Thinking with Deep Learning for Complex Social and Cultural Data Analysis (Spring) A deluge of digital content is generated daily by web-based platforms and sensors that capture digital traces of human communication and connection, and complex states of society, culture, economy, and the world. Emerging deep learning methods enable the integration of these complex data into unified social and cultural “spaces” that enable new answers to classic social and cultural questions, and also pose novel questions. From the perspective of deep learning, everything can be viewed as data-novels, field notes, photographs, lists of transactions, networks of interaction, theories, epistemic styles-and our treatment examines how to configure deep learning architectures and multi-modal data pipelines to improve the capacity of representations, the accuracy of complex predictions, and the relevance of insights to substantial social and cultural questions. This class is for anyone wishing to analyse textual, network, image or arbitrary structured and unstructured data, especially in concert with one another to solve complex social and cultural analysis problems (e.g., characterize a culture; predict next year’s ideology). Prereq : Familiarity with Python is required.
MACS 40101/SOCI 40248 Social Network Analysis (Fall) This course introduces students to concepts and techniques of Social Network Analysis (“SNA”). Social Network Analysis is a theoretical approach and a set of methods to study the structure of relationships among entities (e.g., people, organizations, ideas, words, etc.). Students will learn concepts and tools to identify network nodes, groups, and structures in different types of networks. Specifically, the class will focus on a number of social network concepts, such as social capital, homophily, contagion, etc., and on how to operationalize them using network measures, such as centrality, structural holes, and others.
MACS 40800 – Unsupervised Machine Learning (Spring) Though armed with rich datasets, many researchers are confronted with a lack of understanding of the structure of their data. Unsupervised machine learning offers researchers a suite of computational tools for uncovering the underlying, non-random structure that is assumed to exist in feature space. This course will cover prominent unsupervised machine learning techniques such as clustering, item response theory (IRT) models, multidimensional scaling, factor analysis, and other dimension reduction techniques. Further, mechanics involved in unsupervised machine learning will also be covered, such as diagnosing clusterability of a feature space (visually and mathematically), measures of distance and distance matrices, different algorithms based on data size (k-medoids/k-means vs. PAM vs. CLARA), visualizing patterns, and methods of validation (e.g., internal vs. external validation).
MACS 60000/ SOCI 40133/ CHDV 30510 – Computational Content Analysis (Winter) A vast expanse of information about what people do, know, think, and feel lies embedded in text, and more of the contemporary social world lives natively within electronic text than ever before. These textual traces range from collective activity on the web, social media, instant messaging and automatically transcribed YouTube videos to online transactions, medical records, digitized libraries and government intelligence. This supply of text has elicited demand for natural language processing and machine learning tools to filter, search, and translate text into valuable data. The course will survey and practically apply many of the most exciting computational approaches to text analysis, highlighting both supervised methods that extend old theories to new data and unsupervised techniques that discover hidden regularities worth theorizing. These will be examined and evaluated on their own merits, and relative to the validity and reliability concerns of classical content analysis, the interpretive concerns of qualitative content analysis, and the interactional concerns of conversation analysis. We will also consider how these approaches can be adapted to content beyond text, including audio, images, and video. We will simultaneously review recent research that uses these approaches to develop social insight by exploring (a) collective attention and reasoning through the content of communication; (b) social relationships through the process of communication; and (c) social states, roles, and moves identified through heterogeneous signals within communication. The course is structured around gaining understanding and experimenting with text analytical tools, deploying those tools and interpreting their output in the context of individual research projects, and assessment of contemporary research within this domain. Class discussion and assignments will focus on how to use, interpret, and combine computational techniques in the context of compelling social science research investigations.
MACS XXXXX Uncertainty, Causality, and the Politics of Science (Spring) No description provided yet. TBD
MAPS 30289 Intermediate Regression and Data Science (Winter) This course is designed to provide intermediate-level training in research methods that would pick up immediately after traditionally introductory-level classes that end with multiple regression. This course is designed to be a standalone package of training that will provide tools of immediate use in students’ own research or to make them more capable RAs in larger projects. I expect the course will provide the most utility to advanced BA and MA students that will not have time to complete many advanced, specialized courses. However, it would also serve as a useful bridge to more advanced statistical coursework. Students will also learn how to present findings in competent and accessible ways suitable for poster or conference presentations. Conducted using R. Cross-listed: EDSO 20289/30289, SOCI 20289/30289
MAPS 31755 Longitudinal Research (Winter) This course will introduce students to longitudinal research methods used in psychological research. This includes both the design of longitudinal studies and the use of statistical techniques to analyze longitudinal data. Students will gain experience with reading longitudinal research reports using longitudinal data and develop the skills necessary to conduct and report on their own longitudinal research.
MAPS 31701 Data Analytics & Statistics (Fall) This course is designed for graduate students and advanced undergraduate students and aims to provide a strong foundation in the statistical and data analyses commonly used in the behavioral and social sciences. Topics include logistic regression, statistical inference, chi-square, analysis of variance, and repeated measures models. In addition, this course also place greater emphasis on developing practical skills, including the ability to conduct common analyses using statistical software. You will learn how to build models to investigate your data, formulate hypothesis tests as comparisons between statistical models and critically evaluate model assumptions. The goal of the course is for students to be able to define and use descriptive and inferential statistics to analyze and interpret statistical findings. Open only for Graduate students and 3rd and 4th year undergraduates. Undergraduates must have instructor consent.
MAPS 31702 Data Science (Winter) This course is a graduate-level methods class that aims to train you to solve real-world statistical problems. The goal of the course is for students to be able to choose an appropriate statistical method to solve a given problem of data analysis and communicate your results clearly and succinctly. There will be an extensive hands-on experience of analysis of real data through practical classes.
MAPS 31850 Survey and Experimental Methods in Political Science (Winter) This is an introductory research design and methods course for graduate students who are interested in quantitative research methods – particularly survey and experimental approaches. We will focus on the ways in which political scientists collect, analyze, and interpret survey and experimental data. Students will learn about the fundamentals of research design and quantitative analysis, including theory building, measurement, hypothesis testing, as well as data cleaning, management, and analysis. Prior coursework in statistical methods or coding is not required and will be covered as part of the course.
PBHS 30910/STAT 22810/PPHA 36410/ENST 27400/BIOS 27810 Epidemiology and Population Health (Fall) Epidemiology is the basic science of public health. It is the study of how diseases are distributed across populations and how one designs population-based studies to learn about disease causes, with the object of identifying preventive strategies. Epidemiology is a quantitative field and draws on biostatistical methods. Historically, epidemiology’s roots were in the investigation of infectious disease outbreaks and epidemics. Since the mid-twentieth century, the scope of epidemiologic investigations has expanded to a fuller range non-infectious diseases and health problems. This course will introduce classic studies, study designs and analytic methods, with a focus on global health problems. Prerequisite(s): PBHS 32100 or STAT 22000 or other introductory statistics highly desirable.
PBHS 31001/STAT 35700 Epidemiologic Methods (Winter) This course expands on the material presented in “Principles of Epidemiology,” further exploring issues in the conduct of epidemiologic studies. The student will learn the application of both stratified and multivariate methods to the analysis of epidemiologic data. The final project will be to write the “specific aims” and “methods” sections of a research proposal on a topic of the student’s choice. PBHS 30700 or PBHS 30910 and PBHS 32400/STAT 22400 or applied statistics courses through multivariate regression or consent of instructor.
PBHS 31100 Introduction to Mathematical Modeling in Public Health (Spring) Modeling is a simplified representation of reality that aims to capture essential features of a real-life object or process. Mathematical modeling in public health encompasses a wide array of methodologies offering a powerful toolkit to approach questions that would otherwise be extremely difficult or impossible to answer. This course will introduce students to the conceptual framework of mechanistic modeling and cover the basics of the most widely used mathematical modeling approaches in public health. The course will combine lectures and interactive computer sessions to help students develop practical skills of using basic quantitative techniques.
PBHS 31300/ BIOS 25419 Introduction to Infectious Disease Epidemiology (Spring) This intermediate-level course will build off basic epidemiology foundations to understand principles of infectious disease epidemiology as well as focus on specific diseases & their public health significance. We will examine disease transmission and the interactions between pathogens, hosts, and environment. This course introduces key pathogens, diagnostics, and immune responses. In addition, we will explore the roles of climate change, globalization, and social determinants of health on infectious diseases. Students will learn about research and public health responses to infectious diseases, including study design, modeling, molecular epidemiology, surveillance, outbreak investigation, and prevention. Prerequisite(s): PBHS 30910 (STAT 22810/ENST 27400/HLTH 20910/PPHA 36410) or introductory epidemiology
PBHS 32100/ CCTS 45000 Introduction to Biostatistics (Fall) This course will provide an introduction to the basic concepts of statistics as applied to the bio-medical and public health sciences. Emphasis is on the use and interpretation of statistical tools for data analysis. Topics include (i) descriptive statistics; (ii) probability and sampling; (iii) the methods of statistical inference; and (iv) an introduction to linear and logistics regression.
PBHS 32410/STAT 22401 Regression Analysis for Health and Social Research (Winter) This course is an introduction to the methods and applications of fitting and interpreting multiple regression models. The main emphasis is on the method of least squares. Topics include the examination of residuals, the transformation of data, strategies and criteria for the selection of a regression equation, the use of dummy variables, tests of fit. Stata computer package will be used extensively, but previous familiarity with Stata is not assumed. The techniques discussed will be illustrated by real examples involving health and social science data. Prerequisite(s): PBHS 32100 or STAT 22000 or equivalent.
PBHS 32700/STAT 22700 Biostatistical Methods (Spring) This course is designed to provide students with tools for analyzing categorical, count, and time-to-event data frequently encountered in medicine, public health, and related biological and social sciences. This course emphasizes application of the methodology rather than statistical theory (e.g., recognition of the appropriate methods; interpretation and presentation of results). Methods covered include contingency table analysis, Kaplan-Meier survival analysis, Cox proportional-hazards survival analysis, logistic regression, and Poisson regression. Prerequisite(s): PBHS 32400, STAT 22400 or STAT 24500 or equivalent or consent of instructor.
PBHS 32901/STAT 35201 Introduction to Clinical Trials (Fall) This course will review major components of clinical trial conduct, including the formulation of clinical hypotheses and study endpoints, trial design, development of the research protocol, trial progress monitoring, analysis, and the summary and reporting of results. Other aspects of clinical trials to be discussed include ethical and regulatory issues in human subjects research, data quality control, meta-analytic overviews and consensus in treatment strategy resulting from clinical trials, and the broader impact of clinical trials on public health.
PBHS 33300/STAT 36900 Applied Longitudinal Data Analysis. 100 Units. (Winter) Longitudinal data consist of multiple measures over time on a sample of individuals. This type of data occurs extensively in both observational and experimental biomedical and public health studies, as well as in studies in sociology and applied economics. This course will provide an introduction to the principles and methods for the analysis of longitudinal data. Whereas some supporting statistical theory will be given, emphasis will be on data analysis and interpretation of models for longitudinal data. Problems will be motivated by applications in epidemiology, clinical medicine, health services research, and disease natural history studies. Prerequisite(s): PBHS 32400/STAT 22400 or equivalent, and PBHS 32600/STAT 22600 or PBHS 32700/STAT 22700 or equivalent; or consent of instructor. Equivalent Course(s): PBHS 33300
PBHS 33400/CHDV 32401 Multilevel Modeling (Fall) This course will focus on the analysis of multilevel data in which subjects are nested within clusters (e.g., health care providers, hospitals). The focus will be on clustered data, and several extensions to the basic two-level multilevel model will be considered including three-level, cross-classified, multiple membership, and multivariate models. In addition to models for continuous outcomes, methods for non-normal outcomes will be covered, including multilevel models for dichotomous, ordinal, nominal, time-to-event, and count outcomes. Some statistical theory will be given, but the focus will be on application and interpretation of the statistical analyses. Prerequisite(s): PBHS 32400 and PBHS 32700 or consent of instructor.
PBHS 33500/STAT 35800/CHDV 32702 Statistical Applications (Fall) This course provides a transition between statistical theory and practice. The course will cover statistical applications in medicine, mental health, environmental science, analytical chemistry, and public policy. Lectures are oriented around specific examples from a variety of content areas. Opportunities for the class to work on interesting applied problems presented by U of C faculty will be provided. Although an overview of relevant statistical theory will be presented, emphasis is on the development of statistical solutions to interesting applied problems. PQ: PBHS 32400/STAT 22400 or equivalent, and PBHS 32600/STAT 22600, or PBHS 32700/STAT 22700 or equivalent; or consent of instructor. ID: STAT 35800
PBHS 34500 Machine Learning (Spring) This course provides an introduction to machine learning in the context of public health and medical applications. Key concepts in the design and evaluation of machine learning algorithms will be presented. A variety of algorithms will be covered (e.g. random forests, splines, boosting, neural networks, and ensembles) and include hands-on experience with programming in R . Prereq : PBHS 32410 or equivalent and PBHS 34400 or equivalent programming course
PBHS 35100/HLTH 29100/PPHA 38010/SSAD 46300 Health Services Research Methods (Spring) The purpose of this course is to better acquaint students with the methodological issues of research design and data analysis widely used in empirical health services research. To deal with these methods, the course will use a combination of readings, lectures, problem sets (using STATA), and discussion of applications. The course assumes that students have had a prior course in statistics, including the use of linear regression methods. Prereq : At least one course in linear regression and basic familiarity with STATA; or consent of instructor.
PBHS 43010/STAT 35920 Applied Bayesian Modeling and Inference (Spring) Course begins with basic probability and distribution theory, and covers a wide range of topics related to Bayesian modeling, computation, and inference. Significant amount of effort will be directed to teaching students on how to build and apply hierarchical models and perform posterior inference. The first half of the course will be focused on basic theory, modeling, and computation using Markov chain Monte Carlo methods, and the second half of the course will be about advanced models and applications. Computation and application will be emphasized so that students will be able to solve real-world problems with Bayesian techniques. PQ: STAT 24400 and STAT 24500 or master level training in statistics.
PBHS 40500 Advanced Epidemiologic Methods (Spring) This course examines some features of study design, but is primarily focused on analytic issues encountered in epidemiologic research. The objective of this course is to enable students to conduct thoughtful analysis of epidemiologic and other population research data. Concepts and methods that will be covered include: matching, sampling, conditional logistic regression, survival analysis, ordinal and polytomous logistic regressions, multiple imputation, and screening and diagnostic test evaluation. The course follows in sequence the material presented in “Epidemiologic Methods.” Prerequisite(s): PBHS 31001
PBHS 43010/STAT 35920 Applied Bayesian Modeling and Inference (Spring) Course begins with basic probability and distribution theory, and covers a wide range of topics related to Bayesian modeling, computation, and inference. Significant amount of effort will be directed to teaching students on how to build and apply hierarchical models and perform posterior inference. The first half of the course will be focused on basic theory, modeling, and computation using Markov chain Monte Carlo methods, and the second half of the course will be about advanced models and applications. Computation and application will be emphasized so that students will be able to solve real-world problems with Bayesian techniques. Prerequisite(s): STAT 24400 and STAT 24500 or master level training in statistics.
PBPL 26400 Quantitative Methods in Public Policy (Winter) Policy designers and policy analysts should understand the quantitative methods whereby social and economic reality can be described and policy outcomes evaluated; this course will introduce the basic methodologies used in quantitative social description. The underlying discipline is statistics, and this course will focus on statistical thinking and applications with real data sets. Students will be introduced to sampling, hypothesis testing, and regression, as well as other components of the basic toolkit of quantitative policy analysis.
PLSC 30500 Introduction to Quantitative Social Sciences (Fall) This is the first course in the quantitative methods sequence in political science. Students will build skills to execute and evaluate key research designs for causal and descriptive research. The course also lays the necessary foundation for future coursework in quantitative methods.
PLSC 30600 Causal Inference (Winter) This is the third course in quantitative methods in the Political Science PhD program. The course is an introduction to the theory and practice of causal inference from quantitative data. It will cover the potential outcomes framework, the design and analysis of experiments, matching, weighting, regression adjustment, differences-in-differences, instrumental variables, regression discontinuity designs and more. Students will examine and implement these approaches through a variety of examples from across the social sciences. The course will use the R programming language for statistical computing.
PLSC 30700. Introduction to Linear Models. 100 Units. (Spring) This course will provide an introduction to the linear model, the dominant form of statistical inference in the social sciences. The goals of the course are to teach students the statistical methods needed to pursue independent large-n research projects and to develop the skills necessary to pursue further methods training in the social sciences. Part I of the course reviews the simple linear model (as seen in STAT 22000 or its equivalent) with attention to the theory of statistical inference and the derivation of estimators. Basic calculus and linear algebra will be introduced. Part II extends the linear model to the multivariate case. Emphasis will be placed on model selection and specification. Part III examines the consequences of data that is “poorly behaved” and how to cope with the problem. Depending on time, Part IV will introduce special topics like systems of simultaneous equations, logit and probit models, time-series methods, etc. Little prior knowledge of math or statistics is expected, but students are expected to work hard to develop the tools introduced in class.
PLSC 30901 Game Theory 1 (Fall) This course introduces students to games of complete information through solving problem sets. We will cover the concepts of dominant strategies, rationalizable strategies, Nash equilibrium, subgame perfection, backward induction, and imperfect information. The course will be centered around several applications of game theory to politics: electoral competition, agenda control, lobbying, voting in legislatures and coalition games.
PLSC 31000 Game Theory 2 (Winter) This course introduces students to games of incomplete information and several advanced topics through solving problem sets. We will cover the concepts of Bayes Nash equilibrium, perfect Bayesian equilibrium, and the basics of mechanism design and information design. In terms of applications, the course will extend the topics examined in the prerequisite, PLSC 30901. Game Theory I to allow for incomplete information, with a focus on the competing challenges of moral hazard and adverse selection in those settings.
PLSC 40502 Data Analysis with Statistical Models (Winter) This course is part of the second year of the Quantitative Methodology sequence in the Department of Political Science and builds on the first year sequence (PLSC 30500, 30600, 30700). It will introduce students to likelihood and Bayesian inference with a focus on multilevel/hierarchical regression models. The overarching framework of this class is model-based inference for description and prediction — a complement to the design-based framework of PLSC 30600 Causal Inference. Students will learn both the theory behind Bayesian modeling as well as how to implement common estimators (e.g. Expectation-Maximization, Markov Chain Monte Carlo (MCMC)) in the R statistical programming language. Applied examples will be drawn from across the political science literature, with a particular emphasis on the analysis of large survey data (e.g. the American National Election Survey (ANES), the Cooperative Election Survey (CES), the European Social Survey (ESS)).
PLSC 40601 Advanced topics in causal inference (Spring) This is a graduate-level course considering modern advances in causal inference and experimental design. In particular, we will consider how machine learning methods can be leveraged to address causal questions. We will read a selection of papers introducing and implementing techniques and research designs, with applications to the social and health sciences and public policy. We will discuss what these new methods are able to offer, and where they may have limitations. The course will be oriented around class discussion and student presentations on the readings. An introductory course in probability and statistics is required; this prerequisite can be met by courses in statistics, biostatistics, economics, political science, sociology, or related fields. Coursework in causal inference is recommended but not required; additional reading references will be provided for students who have not had prior exposure to causal inference methodology.
PLSC 40815/PBPL 40815/PECO 40815/PPHA 40815 New Directions in Formal Theory (Spring) In this graduate seminar we will survey recent journal articles that develop formal (mathematical) theories of politics. The range of topics and tools we touch on will be broad. Topics include models of institutions, groups, and behavior, and will span American politics, comparative politics, and international relations. Tools include game theory, network analysis, simulation, axiomatic choice theory, and optimization theory. Our focus will be on what these models are theoretically doing: What they do and do not capture, what makes one mathematical approach more compelling than another, and what we can ultimately learn from a highly stylized (and necessarily incomplete) mathematical representation of politics. The goal of the course is for each participant, including the professor, to emerge with a new research project. Prerequisite(s): PLSC 30901, PLSC 31000 or consent of instructor.
PLSC 48401/PPHA 39830 Quantitative Security (Fall) Since Quincy Wright’s A Study of War, scholars of war and security have collected and analyzed data. This course guides students through an intellectual history of the quantitative study of war. The course begins with Wright, moves to the founding of the Correlates of War project in the late 1960s, and then explores the proliferation of quantitative conflict studies in the 1990s and 2000s. The course ends by considering the recent focus on experimental and quasi-experimental analysis. Throughout the course, students will be introduced to the empirical methods used to study conflict and the data issues facing quantitative conflict scholars. For students with limited training in quantitative methods, this course will serve as a useful introduction to such methods. For students with extensive experience with quantitative methods, this course will deepen their understanding of when and how to apply these methods.
PLSC 57200/SOCI 50096 Network Analysis (Winter) This seminar explores the sociological utility of the network as a unit of analysis. How do the patterns of social ties in which individuals are embedded differentially affect their ability to cope with crises, their decisions to move or change jobs, their eagerness to adopt new attitudes and behaviors? The seminar group will consider (a) how the network differs from other units of analysis, (b) structural properties of networks, consequences of flows (or content) in network ties, and (c) dynamics of those ties. Equivalent Course(s): SOCI 50096
PPHA 30545 Machine Learning (Winter/Spring) The objective of this course is to train students to be insightful users of modern machine learning methods. The class covers regularization methods for regression and classification, as well as large-scale approaches to inference and testing. In order to have greater flexibility when analyzing datasets, both frequentist and Bayesian methods are investigated. Typical applications of the methods presented in this course include, but are not limited to: predicting restaurants’ sanitation inspection scores, uncovering the determinants of recidivism, testing for judges’ impartiality, and carrying out regression analysis and model selection using surveys with very many variables, such as the Current Population Survey.
PPHA 30562 Telling Stories with Data Visualization (Fall) This course will teach students how to create a well-crafted data visualization that can tell a story or communicate an idea in an instant. In this course, students will learn data mining, chart construction, and most importantly, they will learn strategies for communicating a complex concept with a single image. Prerequisite(s): Students should have some familiarity with programs like excel or R and the ability to do basic functions in these programs. No prior data visualization training is necessary.
PPHA 31002 Statistics for Data Analysis I (Fall) This is the first quarter of the statistics sequence at the Harris School. This course aims to provide students with a basic understanding of statistical analysis for policy research. This course makes no assumptions about prior knowledge, apart from basic mathematics skills. Examples will draw on current events and policy debates when possible.
PPHA 31102 Statistical Data Analysis II: Regressions (Winter) A continuation of PP31002, this course focuses on the statistical concepts and tools used to study the association between variables. This course will introduce students to regression analysis and explore its uses in policy analysis. PP31102 or PP31301 required of all first-year students.
PPHA 31202 Advanced Statistics for Data Analysis I (Fall) This course focuses on the statistical concepts and tools used to study the association between variables and causal inference. This course will introduce students to regression analysis and explore its uses in policy analyses. This course will assume a greater statistical sophistication on the part of students than is assumed in PPHA 31002.
PPHA 31302, Advanced Statistics for Data Analysis I (Winter) This course focuses on the statistical concepts and tools used to study the association between variables and causal inference. This course will introduce students to regression analysis and explore its uses in policy analyses. This course will assume a greater statistical sophistication on the part of students than is assumed in PPHA 31002.
PPHA 34600, Program Evaluation (Fall/Spring) The goal of this course is to introduce students to program evaluation and provide an overview of current issues and methods in impact evaluation. We will focus on estimating the causal impacts of programs and policy using social experiments, panel data methods, instrumental variables, regression discontinuity designs, and matching techniques. We will discuss applications and examples from the fields of education, demography, health, crime, job training, and others. Prerequisites: PPHA 31001 or PPHA 31002 and PPHA 31101 or PPHA 31102 or equivalent statistics coursework.
PPHA 35577 Big Data and Development (Winter) A seminar course focused on the use of innovative data capture and analysis techniques to investigate topics related to economic and political development. Microlevel data is increasingly used to target and evaluate development interventions. In this course, students will engage with cutting-edge theoretical and quantitative research, drawing on readings in economics, political science, and data science. The course is organized around a set of core topics, including political and economic development, community-driven aid interventions, causes and consequences of conflict, and climate change. Course assessments will include three short research briefs and a final paper.
PPHA 38520 GIS Applications for Public Policy (Spring) Geographic Information Systems (GIS) refers to tools and techniques for handling, analyzing, and presenting spatial data. GIS has become a powerful tool for social sciences applications over the past thirty years, permitting lines of scientific inquiry that would not otherwise be possible. This course provides an introduction to GIS with a focus on how it may be applied to common needs in the social sciences, such as economics, sociology, and urban geography, as distinct from physical or environmental sciences. Students will learn basic GIS concepts as applied to specific research questions through lectures, lab exercises, and in-class demonstrations. Examples of the kinds of topics we will pursue include how we can use GIS to understand population trends, crime patterns, asthma incidence, and segregation in Chicago. Priority will be given to students pursuing the Survey Research Certificate.
PPHA 41300 Cost Benefit Analysis (Fall/Spring) The goals of this course include learning (1) how to read, or judge, a cost-benefit analysis; (2) how to incorporate elements of cost-benefit analysis into policy work; and (3) when CBA is a good tool to use and when it isnt. This class also presents an opportunity to reflect on big picture issues of how to treat uncertainty and risk; discount costs and benefits received in the future; value lives saved; and manage other difficult matters. In brief, this class offers a comprehensive treatment of the cost benefit analysis methodology, with attention devoted to the microeconomic underpinnings of the technique as well as applications drawn from many areas, including health, the environment, and public goods.
PPHA 41600, Survey Research Methodology (Winter) The goal of this course is to learn about the methods used to collect publicly available survey data that can be used for policy research so that students can appropriately use these data to answer policy relevant questions. Students will learn about the methods used to collect survey data, how to develop researchable policy questions that can be answered with the survey data, and about the limitations of the survey data for answering policy research questions. In order to analyze policy questions using available survey data, students will also learn about actual survey instruments, survey sample designs, survey data processing, and survey data systems that the major public policy relevant surveys use. The course will also examine specific measurement and analysis issues that are of interest to policy research (e.g., measuring public program enrollment and public program eligibility simulation). By the end of the course each student will understand the methods used to collect survey data, have developed a researchable policy question, carried out the appropriate analysis to answer the question, produced high quality analytical tables, and written up descriptions of the methods used to produce the numbers in the tables in a style that is consistent with professional policy research.
PPHA 41800/PSYC 47500 Survey Questionnaire Design (Spring) The questionnaire has played a critical role in gathering data used to assist in making public policy, evaluating social programs, and testing theories about social behavior (among other uses). This course offers a systematic way to construct and evaluate questionnaires. We will learn to think about survey questions from the perspective of the respondent and in terms of cognitive and social tasks that underlie responding. We will examine the impact of questions on data quality and will review past and recent methodological research on questionnaire development. The course will help students to tell the difference between better and worse types of survey questions, find and evaluate existing questions on different topics, and construct and test questionnaires for their own needs.
PPHA 42000, Applied Econometrics I (PhD Level) (Fall) This course is the first in a two-part sequence designed to cover applied econometrics and regression methods at a fairly advanced level. This course provides a theoretical analysis of linear regression models for applied researchers. It considers analytical issues caused by violations of the Gauss-Markov assumptions, including linearity (functional form), heteroscedasticity, and panel data. Alternative estimators are examined to deal with each. Prerequisites: This course is intended for first or second-year Ph.D. students or advanced masters-level students who have taken the Statistics 24400/24500 sequence. Familiarity with matrix algebra is necessary.
PPHA 42100 Applied Econometrics II (Winter) This course is the second in a three-part sequence designed to cover applied econometrics and regression methods at a fairly advanced level. This course provides a theoretical analysis of linear regression models for applied researchers. It considers analytical issues caused by violations of the Gauss-Markov assumptions, including linearity (functional form), heteroscedasticity, and panel data. Alternative estimators are examined to deal with each. Prerequisites: This course is intended for first or second-year Ph.D. students or advanced masters-level students who have taken Applied Econometrics I. Familiarity with matrix algebra is necessary.
PPHA 42200 Applied Econometrics III (Spring) Public Policy 42200, the third in a Three-part sequence, is a basic course in applied econometrics designed to provide students with the tools necessary to evaluate and conduct empirical research. It will focus on the analysis of theoretical econometric problems and the hands-on use of economic data. Topics will include non-linear estimation, multi-variate and simultaneous systems of equations, and qualitative and limited dependent variables. Some familiarity with linear algebra is strongly recommended. Required of all first-year Ph.D. students.
PPHA 44900/PBPL 28550 Methods Of Data Collection: Social Experiments, Quasi-Experiments and Surveys (Winter) The pressure in many fields (notably medicine, health research, and education) for evidence-based results has increased the importance of the design and analysis of social investigations. This course will address three broad issues: the design and analysis of social experiments and quasi-experiments; the design and analysis of sample surveys; and how the interrelationships between the two approaches can inform generalization from experiments. There are two parallel streams in the course. First, the course will tackle the issues of generalization from three different perspectives: (i) the classic statistical design of experiments; (ii) the design of experiments and quasi-experiments in the social sciences; (iii) the design and analysis of sample surveys. Second, using a set of readings on research design in a variety of settings, we will consider how evidence from research is gathered and used. Randomized clinical trials in medicine, tests of interventions in education and manpower planning, and the use of scientific evidence in policy formulation will be among the examples. The course will explore the relative relevance of evidence from these different sources in formulating policy.
PSYC 20250/EDSO 20250/ENST 20250 Introduction to Statistical Concepts and Methods (Winter) Statistical techniques offer psychologists a way to build scientific theories from observations we make in the laboratory or in the world at large. As such, the ability to apply and interpret statistics in psychological research represents a foundational and necessary skill. This course will survey statistical techniques commonly used in psychological research. Attention will be given to both descriptive and inferential statistical methodology. Prereq : It is recommended that students complete MATH 13100 and MATH 13200 (or higher) before taking this course.
PSYC 26010 Big Data in the Psychological Sciences (Spring) Innovative research in Psychology has been pushing the bounds of traditional experiments through the usage of “Big Data”, where experiments are conducted at humungous scales-at the levels of thousands to millions of participants, images, or neurons. With these developments in the field, fluency in these new technologies, methods, and computational skills are becoming increasingly important. In this course, students will develop an understanding of these new directions, and will learn practical plug-and-play tools that will allow them to easily incorporate Big Data in their lives and research. We will also discuss the looming ethical issues and societal implications that come with Big Data. The class will culminate in a final project in which students will be able to collect and analyze their own Big Data. Prereq : Familiarity with basic statistics and Excel. PSYC 20100 (Statistics) and PSYC 20200 (Research Methods) recommended but not required.
PSYC 34410/CPNS 33200 Computational Approaches for Cognitive Neuroscience (Spring) This course is concerned with the relationship of the nervous system to higher order behaviors such as perception and encoding, action, attention, and learning and memory. Modern methods of imaging neural activity are introduced, and information theoretic methods for studying neural coding in individual neurons and populations of neurons are discussed. Prerequisite(s): BIOS 24222 or CPNS 33100.
PSYC 36210/CPNS 31000 Mathematical Methods for Biological Sciences I (Winter) This course builds on the introduction to modeling course biology students take in the first year (BIOS 20151 or 152). It begins with a review of one-variable ordinary differential equations as models for biological processes changing with time, and proceeds to develop basic dynamical systems theory. Analytic skills include stability analysis, phase portraits, limit cycles, and bifurcations. Linear algebra concepts are introduced and developed, and Fourier methods are applied to data analysis. The methods are applied to diverse areas of biology, such as ecology, neuroscience, regulatory networks, and molecular structure. The students learn computations methods to implement the models in MATLAB. Prerequisite(s): BIOS 20151 or BIOS 20152 or consent of the instructor.
PSYC 36211/CPNS 31100 Mathematical Methods for Biological Sciences II. (Fall) This course is a continuation of BIOS 26210. The topics start with optimization problems, such as nonlinear least squares fitting, principal component analysis and sequence alignment. Stochastic models are introduced, such as Markov chains, birth-death processes, and diffusion processes, with applications including hidden Markov models, tumor population modeling, and networks of chemical reactions. In computer labs, students learn optimization methods and stochastic algorithms, e.g., Markov Chain, Monte Carlo, and Gillespie algorithm. Students complete an independent project on a topic of their interest. Prerequisite(s): BIOS 26210 Equivalent.
PSYC 37300 Experimental Design and Statistical Modeling I (Winter) This course covers topics in research design and analysis. They include multifactor, completely randomized procedures and techniques for analyzing data sets with unequal cell frequencies. Emphasis is on principles, not algorithms, for experimental design and analysis.
PSYC 37900 Experimental Design II (Spring) Experimental Design II covers more complex ANOVA models than in the previous course, including split-plot (repeated-measures) designs and unbalanced designs. It also covers analysis of qualitative data, including logistic regression, multinomial logit models, and log linear models. An introduction to certain advanced techniques useful in the analysis of longitudinal data, such as hierarchical linear models (HLM), also is provided. For course description contact Psychology. PQ: PSYC 37300 (No substitutions) or permission of instructor.
PPHA 44900 Social Experiments Design and Generalization (Winter) The pressure in many fields (notably medicine, health research, and education) for evidence-based results has increased the importance of the design and analysis of social investigations. We will consider the complementary strengths of surveys and experiments in assessing evidence for generalization in policy areas; randomized clinical trials in medicine, field experiments in economics and psychology, and the use of scientific evidence in policy formulation will be among the examples. The course will comprise three broad streams: the design and analysis of social experiments and quasi-experiments; the design and analysis of sample surveys; and how the interrelationships between the two approaches can strengthen causal claims from social data. There are two major challenges in providing evidence [generalizing findings] from social research: (i) determining causation and (ii) generalizing results from a sample of observed cases to the rest of the (unobserved) population. Statistics has provided the two fundamental approaches to addressing these challenges: (randomized) field experiments and (random) sample surveys. The course will tackle the issues of generalization from these two perspectives: (i) the classical statistical design of experiments (developed by statisticians between the 1910s and the 1950s) that can be found in texts by Fisher, Cox, Snedecor and Cochran, and others); this approach relates closely to the design of quasi-experiments and experiments in the social sciences, as described by Campbell and Stanley in the 1950s, and extended by Cook, Shadish, and others; (ii) the design and analysis of sample surveys, originating in the 1890s, in particular multi-stage clustered designs, and experiments embedded in them, as presented by Cochran, Kish, and others.
PSYC 46050 Principles of Data Science and Engineering for Laboratory Research (Winter) The quantity of data gathered from laboratory experiments is constantly increasing. This course will explore the latest concepts, techniques and best-practice to create efficient data analysis pipelines. We will focus on the python ecosystem. By the end of the course, you are expected to be able to apply appropriate tools to streamline your own data analysis. Prerequisite(s): Familiarity with coding in python
SOCI 20559/30559 Spatial Regression Analysis (Spring) This course covers statistical and econometric methods specifically geared to the problems of spatial dependence and spatial heterogeneity in cross-sectional data. The main objective for the course is to gain insight into the scope of spatial regression methods, to be able to apply them in an empirical setting, and to properly interpret the results of spatial regression analysis. While the focus is on spatial aspects, the types of methods covered have general validity in statistical practice. The course covers the specification of spatial regression models in order to incorporate spatial dependence and spatial heterogeneity, as well as different estimation methods and specification tests to detect the presence of spatial autocorrelation and spatial heterogeneity. Special attention is paid to the application to spatial models of generic statistical paradigms, such as Maximum Likelihood and Generalized Methods of Moments. An import aspect of the course is the application of open source software tools such as various R packages, GeoDa and the Python Package PySal to solve empirical problems. Prerequisite(s): An intermediate course in multivariate regression or econometrics. Familiarity with matrix algebra
SOCI 20004/30004 Statistical Methods of Research 1. (Fall) This course provides a comprehensive introduction to widely used quantitative methods in sociology and related social sciences. Topics covered include analysis of variance and multiple regression, considered as they are used by practicing social scientists.
SOCI 30005 Statistical Methods of Research 2 (Winter) Social scientists regularly ask questions that can be answered with quantitative data from a population-based sample. For example, how much more income do college graduates earn compared to those who do not attend college? Do men and women with similar levels of training and who work in similar jobs earn different incomes? Why do children who grow up in different family or neighborhood environments perform differently in school? To what extent do individuals from different socioeconomic backgrounds hold different types of political attitudes and engage in different types of political behavior? This course explores statistical methods that can be used to answer these and many other questions of interest to social scientists. The main objectives are to provide students with a firm understanding of linear regression and generalized linear models and with the technical skills to implement these methods in practice.
SOCI 30112 Applications of Hierarchical Linear Models . (Spring) A number of diverse methodological problems such as correlates of change, analysis of multi-level data, and certain aspects of meta-analysis share a common feature–a hierarchical structure. The hierarchical linear model offers a promising approach to analyzing data in these situations. This course will survey the methodological literature in this area and demonstrate how the hierarchical linear model can be applied to a range of problems.
SOCI 20157/30157 Mathematical Models (Winter) This course examines mathematical models and related analyses of social action, emphasizing a rational-choice perspective. About half the lectures focus on models of collective action, power, and exchange as developed by Coleman, Bonacich, Marsden, and Yamaguchi. Then the course examines models of choice over the life course, including rational and social choice models of marriage, births, friendship networks, occupations, and divorce. Both behavioral and analytical models are surveyed.
SOCI 30253 /MACS 54000 Introduction to Spatial Data Science. (Fall) Spatial data science consists of a collection of concepts and methods drawn from both statistics and computer science that deal with accessing, manipulating, visualizing, exploring and reasoning about geographical data. The course introduces the types of spatial data relevant in social science inquiry and reviews a range of methods to explore these data. Topics covered include formal spatial data structures, geovisualization and visual analytics, rate smoothing, spatial autocorrelation, cluster detection and spatial data mining. An important aspect of the course is to learn and apply open source software tools, including R and GeoDa.
SOCI 40103 Event History Analysis (Fall) An introduction to the methods of event history analysis will be given. The methods allow for the analysis of duration data. Non-parametric methods and parametric regression models are available to investigate the influence of covariates on the duration until a certain even occurs. Applications of these methods will be discussed i.e., duration until marriage, social mobility processes organizational mortality, firm tenure, etc.
SOCI 40242 Parametric and Semi-parametric Methods of Categorical Data Analysis (Winter) This course introduces various regression and related methods and models for the analysis of categorical data with an emphasis on their applications to social‐science research. The course covers various regression models with a categorical dependent variable, including (1) logistic regression, (2) probit regression, (3) multinomial logit regression, (4) ordered logit regression, (5) nested logit regression, (6) bivariate probit regression, and (7) regression models with a latent-class dependent variable. In addition, the course also tries to cover (8) the use of a categorical regression model for the estimation of propensity scores in causal analysis, (9) the use of propensity scores in the statistical decomposition analysis of a categorical outcome variable, and (10) the use of propensity scores in segregation analysis with covariates. The course also provides students with examples of various substantive social‐science applications of the categorical data analysis. The course employs STATA for models without using latent-class variable and employs LEM for models with a latent-class variable. LEM is made available free of charge to students. The course requires as a prerequisite only an introductory-level
SOCI 40258 Causal Mediation Analysis (Spring) Causal mediation analysis lies at the very heart of social science. It seeks to uncover not just whether but al so why an exposure affects an outcome by quantifying the processes and mechanisms through which a causal effect operates. That is, it aims to identify causal chains that connect an exposure to an outcome via intermediate variables known as mediators. This class will cover methods for analyzing causal mediation with an emphasis on social science applications. It will use precise notation (potential outcomes) and accessible conceptual diagrams (directed acyclic graphs) to lead students from basic definitions of effects, via minimally necessary identification assumptions, to cutting-edge estimation procedures. It will provide a guide for analyzing causal mediation using modern techniques, including effect decomposition, adjustment for both pre- and post-exposure confounding, analysis of multiple mediators, and estimation via regression modeling, inverse probability weighting, and machine learning methods. The class will address both theory and conceptual material alongside practical implementation using R or Stata.
SOCI 50132 Seminar: Causal Inference in Studies of Educational Interventions (Spring) This course will engage students in evaluating the validity of causal claims made in important educational studies conducted within multiple disciplines. A focus will be on what can be learned about the school as an organization and the work of teaching by evaluating attempts to improve education. Fellows will re-analyze data from such studies, write reports that critically evaluate published study findings, and consider implications for research on educational improvement. This course is required of second year Fellows in the Education Sciences. Otherwise, admission to the seminar requires permission of the instructor. Introductory coursework in applied statistics is a prerequisite; prior study of causal inference is recommended.
SOSC 36006: Foundations for Statistical Theory (Fall) This course is designed for graduate and advanced undergraduate students who aim to develop conceptual understanding of the fundamentals of statistical theory underlying a wide array of quantitative research methods. The course introduces students to probability and statistical theory and emphasizes the connection between statistical theory and the routine practice of statistical applications in quantitative research. Students will gain basic understanding of the concepts of joint, marginal, and conditional probability, Bayes rule, probability distributions of random variables, principles of statistical inference, sampling distributions, and estimation strategies. The course can serve as a preparation for mathematical statistics courses such as STAT 244 (Statistical Theory and Methods 1) and as a theoretical foundation for various advanced quantitative methods courses in the social, behavioral, and health sciences. Prereq: Basic knowledge of linear algebra and calculus, and specifically differentiation and integration, is necessary to understand the material on continuous distributions, multivariate distributions and functions of random variables.
SOSC 36007: Overview of Quantitative Methods in the Social and Behavioral Sciences (Winter) The course is designed to offer an overview of and present the common logic underlying a wide range of methods developed for rigorous quantitative inquiry in the social and behavioral sciences. Students will become familiar with various research designs, measurement, and advanced analytic strategies broadly applicable to theory-driven and data-informed quantitative research in many disciplines. Moreover, they will understand the inherent connections between different statistical methods, and will become aware of the strengths and limitations of each. In addition, this course will provide a gateway to the numerous offerings of advanced quantitative methods courses. It is suitable for undergraduate and graduate students at any stage of their respective programs. Prereq: Introductory level statistics
SOSC 36008/CHDV 36008/EDSO 36008/PSYC 28926 Principles and Methods of Measurement (Spring) Accurate measurement of key theoretical constructs with known and consistent psychometric properties is one of the essential steps in quantitative social and behavioral research. However, measurement of phenomena that are not directly observable (such as psychological attributes, perceptions of organizational climate, or quality of services) is difficult. Much of the research in psychometrics has been developed in an attempt to properly define and quantify such phenomena. This course is designed to introduce students to the relevant concepts, principles, and methods underlying the construction and interpretation of tests or measures. It provides in-depth coverage of test reliability and validity, topics in test theory, and statistical procedures applicable to psychometric methods. Such understanding is essential for rigorous practice in measurement as well as for proper interpretation of research. The course is highly recommended for students who plan to pursue careers in academic research or applied practice involving the use or development of tests or measures in the social and behavioral sciences. Prereq: Couse work or background experience in statistics through inferential statistics and linear regression.
STAT 22000. Statistical Methods and Applications. 100 Units. (Fall, Winter, Spring) This course introduces statistical techniques and methods of data analysis, including the use of statistical software. Examples are drawn from the biological, physical, and social sciences. Students are required to apply the techniques discussed to data drawn from actual research. Topics include data description, graphical techniques, exploratory data analyses, random variation and sampling, basic probability, random variables and expected values, confidence intervals and significance tests for one- and two-sample problems for means and proportions, chi-square tests, linear regression, and, if time permits, analysis of variance. Prerequisite(s): MATH 13100 or 15100 or 15200 or 15300 or 16100 or 16110 or 15910 or 19520 or 19620 or 20250 or 20300 or 20310. Note(s): Students may count either STAT 22000 or STAT 23400, but not both, toward the forty-two credits required for graduation. Students with credit for STAT 23400 not admitted. This course meets on of the general education requirements in the mathematical sciences. Only one of STAT 20000, STAT 20010, or STAT 22000, can count toward the general education requirement in the mathematical sciences.
STAT 22200. Linear Models and Experimental Design. 100 Units. (Spring) This course covers principles and techniques for the analysis of experimental data and the planning of the statistical aspects of experiments. Topics include linear models; analysis of variance; randomization, blocking, and factorial designs; confounding; and incorporation of covariate information. Prerequisite(s): STAT 22000 or 23400 with a grade of at least C+, or STAT 22400 or 22600 or 24500 or 24510 or PBHS 32100, or AP Statistics credit for STAT 22000. Also two quarters of calculus (MATH 13200 or 15200 or 15300 or 16200 or 16210 or 15910 or 19520 or 19620 or 20250 or 20300 or 20310).
STAT 22400/PBHS 32400 Applied Regression Analysis. 100 Units. (Fall) This course introduces the methods and applications of fitting and interpreting multiple regression models. The primary emphasis is on the method of least squares and its many varieties. Topics include the examination of residuals, the transformation of data, strategies and criteria for the selection of a regression equation, the use of dummy variables, tests of fit, nonlinear models, biases due to excluded variables and measurement error, and the use and interpretation of computer package regression programs. The techniques discussed are illustrated by many real examples involving data from both the natural and social sciences. Matrix notation is introduced as needed. Prerequisite: PBHS 32100. Equivalent Course(s): PBHS 32400 a grade of at least C, or STAT 22200 or 22600 or 24500 or 24510 or PBHS 32100, or AP Statistics credit for STAT 22000. Also two quarters of calculus (MATH 13200 or 15200 or 15300 or 16200 or 16210 or 15910 or 19520 or 19620 or 20250 or 20300 or 20310). Equivalent Course(s): PBHS 32400
STAT 22600/PBHS 32600 Analysis of Categorical Data (Winter) This course covers statistical methods for the analysis of qualitative and counted data. Topics include description and inference for binomial and multinomial data using proportions and odds ratios; multi-way contingency tables; generalized linear models for discrete data; logistic regression for binary responses; multi-category logit models for nominal and ordinal responses; loglinear models for counted data; and inference for matched-pairs and correlated data. Applications and interpretations of statistical models are emphasized.
STAT 23400. Statistical Models and Methods 1 (Fall) This course is recommended for students throughout the natural and social sciences who want a broad background in statistical methodology and exposure to probability models and the statistical concepts underlying the methodology. Probability is developed for the purpose of modeling outcomes of random phenomena. Random variables and their expectations are studied; including means and variances of linear combinations and an introduction to conditional expectation. Binomial, Poisson, normal and other standard probability distributions are considered. Some probability models are studied mathematically, and others are studied via computer simulation. Sampling distributions and related statistical methods are explored mathematically, studied via simulation, and illustrated on data. Methods include, but are not limited to, inference for means and proportions for one- and two-sample problems, two-way tables, correlation, and simple linear regression. Graphical and numerical data description are used for exploration, communication of results, and comparing mathematical consequences of probability models and data. Mathematics employed is to the level of single-variable differential and integral calculus and sequences and series.
STAT 24400 Statistical Theory and Methods I (Fall) This course is the first quarter of a two-quarter systematic introduction to the principles and techniques of statistics, as well as to practical considerations in the analysis of data, with emphasis on the analysis of experimental data. This course covers tools from probability and the elements of statistical theory. Topics include the definitions of probability and random variables, binomial and other discrete probability distributions, normal and other continuous probability distributions, joint probability distributions and the transformation of random variables, principles of inference (including Bayesian inference), maximum likelihood estimation, hypothesis testing and confidence intervals, likelihood ratio tests, multinomial distributions, and chi-square tests. Examples are drawn from the social, physical, and biological sciences. The coverage of topics in probability is limited and brief, so students who have taken a course in probability find reinforcement rather than redundancy. Students who have already taken STAT 25100 have the option to take STAT 24410 (if offered) instead of STAT 24400.
STAT 24410/30030 Statistical Theory and Methods Ia (Fall) This course is the first quarter of a two-quarter sequence providing a principled development of statistical methods, including practical considerations in applying these methods to the analysis of data. The course begins with a brief review of probability and some elementary stochastic processes, such as Poisson processes, that are relevant to statistical applications. The bulk of the quarter covers principles of statistical inference from both frequentist and Bayesian points of view. Specific topics include maximum likelihood estimation, posterior distributions, confidence and credible intervals, principles of hypothesis testing, likelihood ratio tests, multinomial distributions, and chi-square tests. Additional topics may include diagnostic plots, bootstrapping, a critical comparison of Bayesian and frequentist inference, and the role of conditioning in statistical inference. Examples are drawn from the social, physical, and biological sciences. The statistical software package R will be used to analyze datasets from these fields and instruction in the use of R is part of the course.
STAT 24500 Statistical Theory and Methods II (Winter, Spring) This course is the second quarter of a two-quarter systematic introduction to the principles and techniques of statistics, as well as to practical considerations in the analysis of data, with emphasis on the analysis of experimental data. This course continues from either STAT 24400 or STAT 24410 and covers statistical methodology, including the analysis of variance, regression, correlation, and some multivariate analysis. Some principles of data analysis are introduced, and an attempt is made to present the analysis of variance and regression in a unified framework. Statistical software is used. Prerequisite(s): Linear algebra (MATH 19620 or 20250 or STAT 24300 or equivalent) and STAT 24400 or STAT 24410. Note(s): Students may count either STAT 24500 or STAT 24510, but not both, toward the forty-two credits required for graduation.
STAT 24510/30040 Statistical Theory and Methods IIa (Winter) This course is the second quarter of a two-quarter systematic introduction to the principles and techniques of statistics, as well as to practical considerations in the analysis of data, with emphasis on the analysis of experimental data. This course continues from either STAT 24400 or STAT 24410 and covers statistical methodology, including the analysis of variance, regression, correlation, and some multivariate analysis. Some principles of data analysis are introduced, and an attempt is made to present the analysis of variance and regression in a unified framework. Statistical software is used. Prerequisite(s): Linear algebra (MATH 19620 or 20250 or STAT 24300 or PHYS 22100 or equivalent) and (STAT 24400 or STAT 24410). Note(s): Students may count either STAT 24500 or STAT 24510, but not both, toward the forty-two credits required for graduation.
STAT 24620/32950 Multivariate Statistical Analysis: Applications and Techniques (Spring) This course focuses on applications and techniques for analysis of multivariate and high dimensional data. Beginning subjects cover common multivariate techniques and dimension reduction, including principal component analysis, factor model, canonical correlation, multi-dimensional scaling, discriminant analysis, clustering, and correspondence analysis (if time permits). Further topics on statistical learning for high dimensional data and complex structures include penalized regression models (LASSO, ridge, elastic net), sparse PCA, independent component analysis, Gaussian mixture model, Expectation-Maximization methods, and random forest. Theoretical derivations will be presented with emphasis on motivations, applications, and hands-on data analysis.Prerequisite(s): (STAT 24300 or MATH 20250) and (STAT 24500 or STAT 24510). Graduate students in Statistics or Financial Mathematics can enroll without prerequisites. Note(s): Linear algebra at the level of STAT 24300. Knowledge of probability and statistical estimation techniques (e.g. maximum likelihood and linear regression) at the level of STAT 24400-24500. Equivalent Course(s): STAT 32950
STAT 24630 Causal Inference Methods and Case Studies (Winter) In many applications of statistics, a large proportion of the questions of interest are about causality rather than questions of description or association. Would booster shots reduce the chance of getting infected by the new variant of COVID-19? How does a new tax policy affect the economic activity? Can a universal health insurance program improve people’s health? In this class, we will introduce some basic concepts and methods in causal inference and discuss examples from various disciplines. The course plans to cover the potential outcome framework, randomize experiments, randomization and model-based inference, matching, sensitivity analysis, and instrumental variables. Examples include the evaluation of job training programs, educational voucher schemes, clinical trials and observational data of medical treatments, smoking, the influenza vaccination study, and more.
STAT 25100. Introduction to Mathematical Probability. 100 Units. (Fall) This course covers fundamentals and axioms; combinatorial probability; conditional probability and independence; binomial, Poisson, and normal distributions; the law of large numbers and the central limit theorem; and random variables and generating functions. Prerequisite(s): ((MATH 16300 or MATH 16310 or MATH 20500 or MATH 20510 or MATH 20900), with no grade requirement), or ((MATH 19520 or MATH 20000) with (either a minimum grade of B-, or STAT major, or currently enrolled in prerequisite course)). Or instructor consent. Note(s): Students may count either STAT 25100 or STAT 25150, but not both, toward the forty-two credits required for graduation.
STAT 25150 Introduction to Mathematical Probability-A (TBD) This course covers fundamentals and axioms; combinatorial probability; conditional probability and independence; binomial, Poisson, and normal distributions; the law of large numbers and the central limit theorem; and random variables and generating functions. Prerequisite(s): MATH 20000 or 20500, or consent of instructor
STAT 25300/31700 Introduction to Probability Models (Winter) This course introduces stochastic processes as models for a variety of phenomena in the physical and biological sciences. Following a brief review of basic concepts in probability, we introduce stochastic processes that are popular in applications in sciences (e.g., discrete time Markov chain, the Poisson process, continuous time Markov process, renewal process and Brownian motion). Prerequisite(s): STAT 24400 or STAT 25100 or STAT 25150
STAT 26100/33600 Time Dependent Data. 100 Units. (Fall) This course considers the modeling and analysis of data that are ordered in time. The main focus is on quantitative observations taken at evenly spaced intervals and includes both time-domain and spectral approaches. Prerequisite(s): STAT 24500 w/B- or better or STAT 24510 w/C+ or better is required; alternatively STAT 22400 w/B- or better and exposure to multivariate calculus (MATH 16300 or MATH 16310 or MATH 19520 or MATH 20000 or MATH 20500 or MATH 20510 or MATH 20800). Graduate students in Statistics or Financial Mathematics can enroll without prerequisites. Some previous exposure to Fourier series is helpful but not required. Equivalent Course(s): STAT 33600
STAT 26300/35490 Introduction to Statistical Genetics (Spring) As a result of technological advances over the past few decades, there is a tremendous wealth of genetic data currently being collected. These data have the potential to shed light on the genetic factors influencing traits and diseases, as well as on questions of ancestry and population history. The aim of this course is to develop a thorough understanding of probabilistic models and statistical theory and methods underlying analysis of genetic data, focusing on problems in complex trait mapping, with some coverage of population genetics. Although the case studies are all in the area of statistical genetics, the statistical inference topics, which will include likelihood-based inference, linear mixed models, and restricted maximum likelihood, among others, are widely applicable to other areas. No biological background is needed, but a strong foundation in statistical theory and methods is assumed. Note(s): STAT 26300 can count as either a List A or List B elective in the Statistics major. Prerequisite(s): STAT 24500 or STAT 24510
STAT 27400/37400 Nonparametric Inference (Winter) Nonparametric inference is about developing statistical methods and models that make weak assumptions. A typical nonparametric approach estimates a nonlinear function from an infinite dimensional space rather than a linear model from a finite dimensional space. This course gives an introduction to nonparametric inference, with a focus on density estimation, regression, confidence sets, orthogonal functions, random processes, and kernels. The course treats nonparametric methodology and its use, together with theory that explains the statistical properties of the methods. Prerequisite(s): STAT 24400 is required; alternatively STAT 22400 and exposure to multivariate calculus and linear algebra.
STAT 27410 Introduction to Bayesian Data analysis (Winter) In recent years, Bayes and empirical Bayes (EB) methods have continued to increase in popularity and impact. These methods combine information from similar and independent experiments and yield improved estimation of both individual and shared model characteristics. In this course, we introduce Bayes and EB methods, as well as the necessary tools needed to evaluate their performances relative to traditional, frequentist methods. We shall focus on more practical, data analytic and computing issues. Various computing methods will be discussed, in order to find the posterior distributions, including Markov chain Monte Carlo methods such as the Gibbs sampler. We will use R to implement these methods to solve real world problems. The methods will be illustrated from applications in various areas, such as biological science, biomedical science, public health, epidemiology, education, social science, economics, psychology, agriculture and engineering. Recent developments of Bayesian methods on nonlinear models, longitudinal data analysis, hierarchical models, time series, survival analysis, spatial statistics will also be explored. Prerequisite(s): [STAT 22000 or STAT 23400 or STAT 22400 or STAT 22600 or STAT 24500 or STAT 24510[ AND [(MATH 13200 or MATH 15200 or MATH 15300 or MATH 16200 or MATH 16210 or MATH 15910 or MATH 19520 or MATH 19620 or MATH 20250 or MATH 20300 or MATH 20310) with a grade of C+ or higher] Note(s): Students should be comfortable with coding in R software.
STAT 27420 Introduction to Causality with Machine Learning (Fall) This course is an introduction to causal inference. We’ll cover the core ideas of causal inference and what distinguishes it from traditional observational modeling. This includes an introduction to some foundational ideas—structural equation models, causal directed acyclic graphs, and then do calculus. The course has a particular emphasis on the estimation of causal effects using machine learning methods. Prerequisites: [STAT 24500 or STAT 24510 or STAT 27725] with a grade of B or higher or consent of instructor.
STAT 27700/CMSC 25300 Mathematical Foundations of Machine Learning. 100 Units. (Fall) This course is an introduction to the mathematical foundations of machine learning that focuses on matrix methods and features real-world applications ranging from classification and clustering to denoising and data analysis. Mathematical topics covered include linear equations, regression, regularization, the singular value decomposition, and iterative algorithms. Machine learning topics include the lasso, support vector machines, kernel methods, clustering, dictionary learning, neural networks, and deep learning. Students are expected to have taken calculus and have exposure to numerical computing (e.g. Matlab, Python, Julia, R). Prerequisite(s): CMSC 12200 or CMSC 15200 or CMSC 16200, and the equivalent of two quarters of calculus (MATH 13200 or higher). Equivalent Course(s): CMSC 25300
STAT 27725 Machine Learning (Winter) This course offers a practical, problem-centered introduction to machine learning. Topics covered include the Perceptron and other online algorithms; boosting; graphical models and message passing; dimensionality reduction and manifold learning; SVMs and other kernel methods; artificial neural networks; and a short introduction to statistical learning theory. Weekly programming assignments give students the opportunity to try out each learning algorithm on real world datasets. CMSC 15400 or CMCS 12300. STAT 22000 or STAT 23400 strongly recommended
STAT 27751/37787 Trustworthy Machine Learning (Spring) Machine learning systems are routinely used in safety critical situations in the real world. However, they often dramatically fail! This course covers foundational and practical concerns in building machine learning systems that can be trusted. Topics include foundational issues—when do systems generalize, and why, essential results in fairness and domain shifts, and evaluations beyond standard test/train splits. This is an intermediate level course in machine learning; students should have at least one previous course in machine learning.
STAT 27850/30850 Multiple Testing, Modern Inference, and Replicability (Fall) This course examines the problems of multiple testing and statistical inference from a modern point of view. High-dimensional data is now common in many applications across the biological, physical, and social sciences. With this increased capacity to generate and analyze data, classical statistical methods may no longer ensure the reliability or replicability of scientific discoveries. We will examine a range of modern methods that provide statistical inference tools in the context of modern large-scale data analysis. The course will have weekly assignments as well as a final project, both of which will include both theoretical and computational components.
STAT 27855 Hypothesis Testing with Empirical Bayes Methodology (Winter) Large scale data sets regularly produced in fields such as biology, social sciences, and neuroscience bring new challenges, like controlling the amount of false positives when testing many hypotheses, as well as the opportunity to leverage information across the entire dataset toward making individual inferences. In this course, we will study theoretical foundations and practical aspects of hypothesis testing in a Bayesian framework. We will focus attention on the local false discovery rate (lfdr), which represents the probability that the null hypothesis is true given the data, and learn several methods for estimating this quantity. Decision theory provides a formal connection between quantities of interest in a Bayesian framework to population parameters in a strictly frequentist model, where the truth status of each null hypothesis is fixed and unknown. We may also discuss methodology for estimating the null distribution, and methods for finite-sample lfdr control if time permits. Homework assignments will have theoretical and computational components.
STAT 30100 Mathematical Statistics-1 (Winter) This course is part of a two-quarter sequence on the theory of statistics. Topics will include exponential, curved exponential, and location-scale families; mixtures, hierarchical, and conditional modeling including compatibility of conditional distributions; principles of estimation; identifiability, sufficiency, minimal sufficiency, ancillarity, completeness; properties of the likelihood function and likelihood-based inference, both univariate and multivariate, including examples in which the usual regularity conditions do not hold; elements of Bayesian inference and comparison with frequentist methods; and multivariate information inequality. Part of the course will be devoted to elementary asymptotic methods that are useful in the practice of statistics, including methods to derive asymptotic distributions of various estimators and test statistics, such as Pearson’s chi-square, standard and nonstandard asymptotics of maximum likelihood estimators and Bayesian estimators, asymptotics of order statistics and extreme order statistics, Cramer’s theorem including situations in which the second-order term is needed, and asymptotic efficiency. Other topics (e.g., methods for dependent observations) may be covered if time permits. Prerequisite(s): STAT 30400 or consent of instructor.
STAT 30200 Mathematical Statistics (Spring) This course continues the development of Mathematical Statistics, with an emphasis on hypothesis testing. Topics include comparison of Bayesian and frequentist hypothesis testing; admissibility of Bayes’ rules; confidence and credible sets; likelihood ratio tests and their asymptotics; Bayes factors; methods for assessing predictions for normal means; shrinkage and thresholding methods; sparsity; shrinkage as an example of empirical Bayes; multiple testing and false discovery rates; Bayesian approach to multiple testing; sparse linear regressions (subset selection and LASSO, proof of estimation errors for LASSO, Bayesian perspective of sparse regressions); and Bayesian model averaging.
STAT 30400. Distribution Theory. 100 Units. (Fall) This course is a systematic introduction to random variables and probability distributions. Topics include standard distributions (i.e. uniform, normal, beta, gamma, F, t, Cauchy, Poisson, binomial, and hypergeometric); properties of the multivariate normal distribution and joint distributions of quadratic forms of multivariate normal; moments and cumulants; characteristic functions; exponential families; modes of convergence; central limit theorem; and other asymptotic approximations. Prerequisite(s): STAT 24500 or STAT 24510 and MATH 20500 or MATH 20510, or consent of instructor.
STAT 30750/24300 Numerical Linear Algebra. 100 Units. (Fall) This course is devoted to the basic theory of linear algebra and its significant applications in scientific computing. The objective is to provide a working knowledge and hands-on experience of the subject suitable for graduate level work in statistics, econometrics, quantum mechanics, and numerical methods in scientific computing. Topics include Gaussian elimination, vector spaces, linear transformations and associated fundamental subspaces, orthogonality and projections, eigenvectors and eigenvalues, diagonalization of real symmetric and complex Hermitian matrices, the spectral theorem, and matrix decompositions (QR, Cholesky and Singular Value Decompositions). Systematic methods applicable in high dimensions and techniques commonly used in scientific computing are emphasized. Students enrolled in the graduate level STAT 30750 will have additional work in assignments, exams, and projects including applications of matrix algebra in statistics and numerical computations implemented in Matlab or R. Some programming exercises will appear as optional work for students enrolled in the undergraduate level STAT 24300.Prerequisite(s): Multivariate calculus (MATH 15910 or MATH 16300 or MATH 16310 or MATH 19520 or MATH 20000 or MATH 20500 or MATH 20510 or MATH 20900 or PHYS 22100 or equivalent). Previous exposure to linear algebra is helpful. Equivalent Course(s): STAT 24300
STAT 30810 High Dimensional Time Series Analysis (Spring) This course will include lectures on the following topics: review of asymptotics for low dimensional time series analysis (linear and nonlinear processes; nonparametric methods; spectral and time domain approaches); covariance, precision, and spectral density matrix estimation for high dimensional time series; factor models; estimation of high dimensional vector autoregressive processes; prediction; and high dimensional central limit theorems under dependence.
STAT 31050/ CAAM 31050 Applied Approximation Theory (Spring) This course covers a range of introductory topics in applied approximation theory, the study of how and when functions can be approximated by linear combinations of other functions. The course will start with classical topics including polynomial and Fourier approximation and convergence, as well as more general theory on bases and approximability. We will also look at algorithms and applications in function compression, interpolation, quadrature, denoising, compressive sensing, finite-element methods, spectral methods, and iterative algorithms.
STAT 31150/CAAM 31150 Inverse Problems and Data Assimilation. 100 Units. (Fall) This class provides an introduction to Bayesian Inverse Problems and Data Assimilation, emphasizing the theoretical and algorithmic inter-relations between both subjects. We will study Gaussian approximations and optimization and sampling algorithms, including a variety of Kalman-based and particle filters as well as Markov chain Monte Carlo schemes designed for high-dimensional inverse problems. Prerequisite(s): Familiarity with calculus, linear algebra, and probability/statistics at the level of STAT 24400 or STAT 24410. Some knowledge of ODEs may also be helpful. Equivalent Course(s): CAAM 31150
STAT 31200 Introduction to Stochastic Processes I (Fall) This course introduces stochastic processes not requiring measure theory. Topics include branching processes, recurrent events, renewal theory, random walks, Markov chains, Poisson, and birth-and-death processes.
STAT 31240 Variational Methods in Image Processing (Spring) This course discusses mathematical models arising in image processing. Topics covered will include an overview of tools from the calculus of variations and partial differential equations, applications to the design of numerical methods for image denoising, deblurring, and segmentation, and the study of convergence properties of the associated models. Students will gain an exposure to the theoretical basis for these methods as well as their practical application in numerical computations.
STAT 31511 Monte Carlo Simulation (Spring) This class primarily concerns the design and analysis of Monte Carlo sampling techniques for the estimation of averages with respect to high dimensional probability distributions. Standard simulation tools such as importance sampling, Metropolis-Hastings, Langevin dynamics, and hybrid Monte Carlo will be introduced along with basic theoretical concepts regarding their convergence to equilibrium. The class will explore applications of these methods in Bayesian statistics and machine learning as well as to other simulation problems arising in the physical and biological sciences. Particular attention will be paid to the major complicating issues like conditioning (with analogies to optimization) and rare events and methods to address them.
STAT 32940/FINM 33180/CAAM 32940 Multivariate Data Analysis via Matrix Decompositions. 100 Units. (Fall) This course is about using matrix computations to infer useful information from observed data. One may view it as an “applied” version of Stat 30900 although it is not necessary to have taken Stat 30900; the only prerequisite for this course is basic linear algebra. The data analytic tools that we will study will go beyond linear and multiple regression and often fall under the heading of “Multivariate Analysis” in Statistics. These include factor analysis, correspondence analysis, principal components analysis, multidimensional scaling, linear discriminant analysis, canonical correlation analysis, cluster analysis, etc. Understanding these techniques require some facility with matrices in addition to some basic statistics, both of which the student will acquire during the course. Program elective. Equivalent Course(s): FINM 33180, CAAM 32940
STAT 33100 Sample Surveys (Fall) This course covers random sampling methods; stratification, cluster sampling, and ratio estimation; and methods for dealing with nonresponse and partial response.
STAT 33400/ FINM 33210 Bayesian Statistical Inference and Machine Learning (Spring) The course will develop a general approach to building models of economic and financial processes, with a focus on statistical learning techniques that scale to large data sets. We begin by introducing the key elements of a parametric statistical model: the likelihood, prior, and posterior, and show how to use them to make predictions. We shall also discuss conjugate priors and exponential families, and their applications to big data. We treat linear and generalized-linear models in some detail, including variable selection techniques, penalized regression methods such as the lasso and elastic net, and a fully Bayesian treatment of the linear model. As applications of these techniques, we shall discuss Ross’ Arbitrage Pricing Theory (APT), and its applications to risk management and portfolio optimization. As extensions, we will discuss multilevel and hierarchical models, and conditional inference trees and forests. We also treat model-selection methodologies including cross-validation, AIC, and BIC and show how to apply them to all of the financial data sets presented as examples in class. Then we move on to dynamic models for time series including Markov state-space models, as special cases. As we introduce models, we will also introduce solution techniques including the Kalman filter and particle filter, the Viterbi algorithm, Metropolis-Hastings and Gibbs Sampling, and the EM algorithm.
STAT 33910/FINM 33170 Financial Statistics: Time Series, Forecasting, Mean Reversion, and High Frequency Data (Winter) This course is an introduction to the econometric analysis of high-frequency financial data. This is where the stochastic models of quantitative finance meet the reality of how the process really evolves. The course is focused on the statistical theory of how to connect the two, but there will also be some data analysis. With some additional statistical background (which can be acquired after the course), the participants will be able to read articles in the area. The statistical theory is longitudinal, and it thus complements cross-sectional calibration methods (implied volatility, etc.). The course also discusses volatility clustering and market microstructure. Prerequisite(s): STAT 39000/FINM 34500 (may be taken concurrently), also some statistics/econometrics background as in STAT 24400–24500, or FINM 33150 and FINM 33400, or equivalent, or consent of instructor.
STAT 34300 Applied Linear Statistical Methods (Fall) This course introduces the theory, methods, and applications of fitting and interpreting multiple regression models. Topics include the examination of residuals, the transformation of data, strategies and criteria for the selection of a regression equation, nonlinear models, biases due to excluded variables and measurement error, and the use and interpretation of computer package regression programs. The theoretical basis of the methods, the relation to linear algebra, and the effects of violations of assumptions are studied. Techniques discussed are illustrated by examples involving both physical and social sciences data.
STAT 34700 Generalized Linear Models (Winter) This applied statistics course is a successor of STAT 34300 and covers the foundations of generalized linear models (GLM). We will discuss the general linear modeling idea for exponential family data and introduce specifically models for binary, multinomial, count and categorical data, and the challenges in model fitting, and inference. We will also discuss approaches that supplement the classical GLM, including quasi-likelihood for over-dispersed data, robust estimation, and penalized GLM. The course also covers related topics including mixed effect models for clustered data, the Bayesian approach of GLM, and survival analysis. This course will make a balance between practical real data analysis with examples and a deeper understanding of the models with mathematical derivations. Prerequisite(s): STAT 34300 or consent of instructor
STAT 34800 Modern Methods in Applied Statistics (Spring) This course covers latent variable models and graphical models; definitions and conditional independence properties; Markov chains, HMMs, mixture models, PCA, factor analysis, and hierarchical Bayes models; methods for estimation and probability computations (EM, variational EM, MCMC, particle filtering, and Kalman Filter); undirected graphs, Markov Random Fields, and decomposable graphs; message passing algorithms; sparse regression, Lasso, and Bayesian regression; and classification generative vs. discriminative. Applications will typically involve high-dimensional data sets, and algorithmic coding will be emphasized.
STAT 35450/HGEN 48600 Fundamentals of Computational Biology: Models and Inference (Winter) Covers key principles in probability and statistics that are used to model and understand biological data. There will be a strong emphasis on stochastic processes and inference in complex hierarchical statistical models. Topics will vary but the typical content would include: Likelihood-based and Bayesian inference, Poisson processes, Markov models, Hidden Markov models, Gaussian Processes, Brownian motion, Birth-death processes, the Coalescent, Graphical models, Markov processes on trees and graphs, and Markov Chain Monte Carlo. Prerequisite(s): STAT 24400
STAT 35460/HGEN 48800 Fundamentals of Computational Biology: Algorithms and Applications (Spring) This course will cover principles of data structure and algorithms, with emphasis on algorithms that have broad applications in computational biology. The specific topics may include dynamic programming, algorithms for graphs, numerical optimization, finite-difference, schemes, matrix operations/factor analysis, and data management (e.g. SQL, HDF5). We will also discuss some applications of these algorithms (as well as commonly used statistical techniques) in genomics and systems biology, including genome assembly, variant calling, transcriptome inference, and so on.
STAT 36510 Random Growth Model and the Kardar-Parisi-Zhang Equation (Winter) In this course, we will show how a variety of physical systems and mathematical models, including randomly growing interfaces, queueing systems, stochastic PDEs, and traffic models, all demonstrate the same universal statistical behaviors in their long-time/large-scale limit. These systems are said to lie in the Kardar-Parisi-Zhang universality class. We will also study a central object in this universality class: the Kardar-Parisi-Zhang equation.
STAT 37711/CAAM 37711/DATA 37711 Machine Learning 1 (Fall) This course is a graduate level introduction to machine learning. We will cover both practical and (probabilistic) foundational aspects of the subject. Topics include empirical risk minimization and overfitting, regression, ensembling, as well as selected topics on representation learning and structure learning. Open to graduate students in data science, statistics, and computer science, or by instructor consent. Students should have a strong background in programming, basic probability, and a graduate-student level of mathematical maturity.
STAT 37792 Topics in Deep Learning: Generative Models (Fall) This course will be a hands on exploration of various approaches to generative modeling with deep networks. Topics include variational auto encoders, flow models, GAN models, and energy models. Participation in this course requires familiarity with pytorch and a strong background in statistical modeling. The course will primarily consist of paper presentations. Each presenter would be required to report on experiments performed with the algorithm proposed in the paper, exploring strengths and weaknesses of the methods.
STAT 37799 Topics in Machine Learning: Machine Learning and Inverse Problems (Winter)
STAT 38100 Measure: Theoretical Probability 1 (Winter) This course provides a detailed, rigorous treatment of probability from the point of view of measure theory, as well as existence theorems, integration and expected values, characteristic functions, moment problems, limit laws, Radon-Nikodym derivatives, and conditional probabilities. Prerequisite(s): STAT 30400 or consent of instructor
** Courses in MACSS, MAPSS and STAT are only available for the Autumn and Winter quarters, and will be updated for Spring quarters once they become available.
College of the Environment
Quantitative courses for undergrads.
Quantitative courses in the College of the Environment focus on examples from the physical world and from the living natural world, including conservation at landscape scale and impacts of global change. We teach the basics of math, stats, coding and mapping, through to advanced coursework in modeling, computation and remote sensing. Our courses prepare students to engage in actionable science in support of a sustainable, biodiverse world.
The Basics – Math and Stat Skills
For students just starting out in calculus and statistics, these courses provide natural world examples to learn from.
Math and Statistics Courses
Q sci 291: calculus for natural systems i: derivatives.
Introduction to differential calculus, emphasizing development of basic skills. Examples promote understanding of mathematics and applications to modeling and solving biological problems. Topics include optimization and curve analysis. Cannot be taken for credit if student received a minimum grade of 2.0 or above in MATH 124 (or equivalent).
- Autumn, Winter
- 5 credits (NSc, RSN)
- Prerequisites: Either MATH 120, Q SCI 190, a minimum score of 2 on the AP MATH test (AB or BC).
- Faculty: Steve Scherba
Open in MyPlan
Q SCI 292: Calculus For Natural Systems II: Integrals
Introduction to integral calculus, emphasizing development of basic skills. Examples promote understanding of mathematics and applications to modeling and solving biological problems. Topics include areas under curves, volumes, and differential equations. Cannot be taken for credit if student received a minimum grade of 2.0 or above in MATH 125 (or equivalent).
- Winter, Spring
- Prerequisites: Either MATH 124, Q SCI 391, or a minimum score of 3 on the AP MATH test (AB or BC).
- Faculty: Andrew Berdahl, Steve Scherba
Q SCI 381 Introduction to Probability and Statistics
Applications to biological and natural resource problems stressing the formulation and interpretation of statistical tests. Random variables, expectations, variances, binomial, hypergeometric, Poisson, normal, chi-square, “t” and “F” distributions.
- Autumn, Winter, Spring
- Prerequisites: Either MATH 120, MATH 124, MATH 125, MATH 126, Q SCI 190, or Q SCI 291, or a minimum score of 2 on the AP MATH test (AB or BC).
- Faculty: John Horne, Tim Jones, Patrick Tobin, Manjaree Binjolkar
ENVIR 310 Data Analysis in Environmental Studies
Practical data-analysis workshop that introduces analysis of quantitative and qualitative data from social and environmental topics. Students use public data or research data to develop skills in data organization, visualization, and statistics. Students evaluate the use of socio-environmental data in graphics and learn to use data to make informed decisions. Quantitative methods, qualitative analysis, and survey analysis are introduced.
- Spring, Summer
- Prerequisites: None
- Faculty: Yen-Chu Weng
Programming, Coding and Data Analysis
These courses focus on data science: manipulating data in programming languages, including Python and R.
Programming and Data Courses
Fish 274 introduction to data analysis for aquatic sciences.
Basic knowledge of data acquisition, manipulation, and visualization are necessary for conducting biological research. Students will be introduced to bash, R, and Jupyter Notebooks, but the concepts learned will easily apply to other computational work. By the end of this course students will be able to import data into R, perform analysis on that data, and export the results to graphs, and presentations.
- Prerequisites: BIOL 180
- Faculty: Steven Roberts
OCEAN 215 Methods of Oceanographic Data Analysis
Statistics, graphical representations, and analysis methods for oceanographic datasets using the PYTHON programming language.
- Prerequisites: MATH 125
- Faculty: Stephen Riser
ATM S 310 Programming for Atmospheric Data Analysis
Introduces students to data analysis in the atmospheric sciences using modern programming languages and techniques. Provides hands-on experience through the exploration of atmospheric datasets.
- 4 credits (NSc)
- Faculty: Alexandra Anderson-Frey
Advanced Statistics and Ecological Modeling
For advanced students wishing to continue exploring multivariate statistics and computational models in ecology and conservation.
Advanced Statistics and Ecological Modeling Courses
Q sci 482 statistical inference in applied research i: hypothesis testing and estimation for ecologists and resource managers.
Theory and application of foundational statistical tests such as chi-square tests, t-tests, analysis of variance; parametric and non-parametric tests; estimating statistical power and sample size needed for experiments. Use of computer programming in R to apply standard statistical tests to solve biological problems.
- 5 credits (NSc)
- Prerequisites: Either STAT 311 or Q SCI 381.
- Faculty: Trevor Branch, Indroneil Ganguly
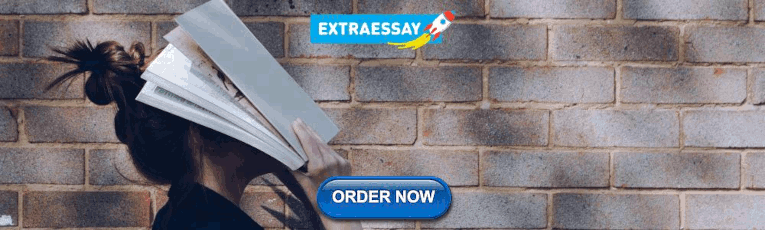
Q SCI 483 Statistical Inference in Applied Research II: Regression Analysis for Ecologists and Resource Managers
Analysis of linear regression models and introduction to nonlinear models. Model selection using generalized F-tests; residual analysis. Application to categorical, count, binomial, transformed variables. Introduction to matrix formation of regression models and applications.
- Prerequisites: Q SCI 482
- Faculty: Tim Jones
Q SCI / FISH 454 Introduction to Quantitative Ecology
Examines concepts in quantitative ecology ranging from model development, interpretation, and fitting model to data. Develops skills to implement quantitative models in spreadsheets and R software.
- Faculty: Tim Essington
Q SCI / FISH 458 Advanced Ecological Modeling: Applying Ecological Models to Manage and Conserve Natural Resources
Models of fish and wildlife population abundance, including age-structured models, the interaction between human exploitation and protected areas, calculating extinction risk, and examining the effect of alternative management strategies on natural populations. A core part of the course is fitting models to data using both maximum likelihood and Bayesian approaches, and increasing the programming abilities of students by implementing models in R.
- Faculty: Trevor Branch
Q SCI / ESRM 451 Analytical Methods in Wildlife Science
This course provides a foundation of techniques commonly used by wildlife biologists in data collection and analysis. Predominantly focused on parameter estimation of demographic rates of animal populations. This course will explore, and discuss in detail, quantitative methods needed to address conservation and management problems in the real world.
- Prerequisites: ESRM 351 and Q SCI 482.
- Faculty: Taylor Ganz
Instrumentation and Technology
These courses are perfect for students wanting hands-on experience building and deploying data collection sensors and understanding the basics of data analysis.
Instrumentation and Technology Courses
Ocean 161 introduction to environmental monitoring and technology.
Introduces a range of environmental monitoring technologies in the context of their scientific application and case studies spanning ocean, atmosphere, and land examples. Includes hands-on experience with sensors and working with data from various technologies and monitoring techniques. Integrates knowledge across environmental science, technology, and engineering.
- Faculty: Sasha Seroy
OCEAN 261 Introduction to Ocean Technology
Introduces ocean science and technology in observational ocean science. Covers the basics of underwater sensor design, power, and data communication, and introduces the design and build process and the operational scope of the ERIS cabled observatory.
- 2 credits (NSc)
- Faculty: Rick Rupan, Sasha Seroy, Fritz Stahr
OCEAN 351 Foundations of Ocean Sensors
Experiential learning giving students foundational knowledge of methods used to observe key quantities that characterize marine environments. Includes theory and application of sensor design and construction principles, in the context of inferences and hypothesis-testing about key physical, biological, chemical, and geological dynamics in the oceans.
- 3 credits (NSc, RSN)
- Prerequisites: OCEAN 215, OCEAN 285
OCEAN 361 Ocean Technology I: Introduction to Underwater Observational Sensor Design and Build
Combines ocean science and technology in a design and build course utilizing the ERIS cabled observatory at the UW Oceanography dock. Students apply the basics of sensor design, power, and data communication to individual underwater observatory projects. Prerequisite: OCEAN 261, which may be taken concurrently.
- Prerequisites: OCEAN 261, may be taken concurrently
OCEAN 461 Advanced Ocean Technology – Project Design and Management
Project management practicum in the design, build, operations, and management of observational ocean science technology. Students oversee and mentor ongoing design and build projects, with attention to design criteria and deployment timelines.
- Prerequisites: OCEAN 361
ATM S 451 Instruments and Observations
Principles of operating instruments for measuring important atmospheric parameters (e.g., temperature, humidity, aerosol concentration). Concepts of sensitivity, accuracy, representativeness, time response. Manipulation of output data including signal processing and statistical analysis. Experimental design and implementation of the design in actual field experiments is included.
- Prerequisites: ATM S 370; either STAT/MATH 390 or Q SCI 381
- Faculty: Alexandra Anderson-Frey, Becky Alexander
GIS and Remote Sensing
From introductory to advanced, these courses focus on satellite-based data collection (remote sensing) and data mapping and geostatistics (GIS).
GIS and Remote Sensing Courses
Esrm 250 introduction to geographic information systems in forest resources.
Applications of GIS technology to forest science and management. Fundamentals of GIS systems: data sources, preprocessing, map analysis, output; remote sensing as a source of GIS data, image analysis, and classification. Emphasis on GIS as a source of management and technical information requests.
- Autumn, Winter, Spring, Summer
- Faculty: Varies
ESS 401 Field Geology with GIS
Geologic mapping in a variety of rock types in the Western United States coupled with and augmented through geospatial analyses. Enhances students’ knowledge of geologic phenomena and processes. Development of skills in mapping, field interpretation, GIS/remote sensing analysis, and report writing. Students responsible for own living expenses while in the field.
- 12 credits (NSc)
- Prerequisites: ESS 211; ESS 212; ESS 213; ESS 420; and either ESS 311, ESS 312, ESS 313, ESS 314, or ESS 316
- Faculty: Steven Walters (co-instructors vary)
ESS 420 Introduction to Geographic Information Systems for the Earth Sciences
Examines principles of GISA applied to the geological sciences. Covers basics of GIScience, data types, and GIS analyses. Includes hands-on analysis applied to geologic patterns and phenomena: sources geological data; geological mapping; measures of topography; hydrologic flow patterns; and topics of the students’ own interests.
- Autumn, Spring
- Faculty: Steven Walters
ESRM 190 Digital Earth
Focuses on developing remote sensing literacy through addressing large scale problems by using big data from global to local levels across a full spectrum of environmental applications. Knowledge and skills developed in this course can be taken and applied to many fields.
- Faculty: Monika Moskal
This is a special topics course. Ask your advisor for more information.
ESRM 430 Remote Sensing of the Environment
Focuses on hyperspatial remote sensing fundamentals, interpretation and manipulation of aerial photography, satellite imagery, and Light Detection and Ranging (LiDAR). Uses traditional and ‘state of the art’ image processing techniques. Students learn to evaluate available hyperspatial remote sensing data sources and design simple projects related to environmental applications.
ESRM / CEE 432 Advanced Remote Sensing and Earth Observation
Covers the theory and application of satellite remote sensing as a tool for environmental science. Topics include the fundamentals of electromagnetic radiation, reflection and absorption, black body radiation, use of the Plank Function, satellite and sensor technology, map projections, integration of GIS data, and digital image analysis. Practical training with advanced image processing software (ENVI and open source). Recommended: GIS; statistics; and basic physics.
- 4 credits (NSc, RSN)
- Faculty: David Butman
ESS 421 Introduction to Geological Remote Sensing
Principles of image interpretation for geologists. Study of land forms, structure, lithology, surface processes using aircraft and satellite data. Use of digital multispectral images and radar images for geological mapping.
ESRM 433 Airborne Lidar for Remote Sensing of Vegetation and Geomorphology
Focuses on the applications of lidar data, its interpretation, and processing. Students apply information learned from lectures and laboratory projects to a final project that analyzes vegetation structure, geomorphology, or hydrology.
- Prerequisites: either ESRM 250, SEFS 520, ESRM 430, FISH 452, FISH 502, FISH 552, or GEOG 360
- Faculty: Monika Moskal (Doctoral co-instructors vary)
Dean's Office Contact:
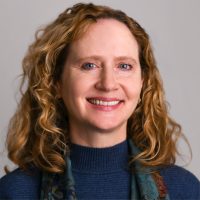
Michelle Hall
Director of student & academic services.
- hallm@uw.edu
- 206-543-6233

© 2024 University of Washington
- Link Policy
You are using an outdated browser. Please upgrade your browser to improve your experience.

- Mission and Strategic Plan
- Accreditation
- Facts and Figures
- Campus Safety
- Buildings and Maps
- Majors & Programs
- Class Search
- Academic Calendar
- Academic Affairs
- Online Learning
- International
- Undergraduate Admissions
- Graduate Admissions
- Tuition and Fees
- Financial Aid
- Request Info
- Student Life
- Division of Student Life and Wellbeing
- Maverick Advising Center
- Career Services
- Accessibility
- Housing & Residence Life
- Student Leadership, Involvement, and Inclusion
- Student Conduct & Community Standards
- Spirit, Tradition, and Events
- Student Safety
- Office of Engagement
- Campus Resources for Community
- Service Learning Academy
- Barbara Weitz Community Engagement Center
- Volunteer and Civic Engagement Programs
- Samuel Bak Museum: The Learning Center
- Faculty Resources
- Student Resources
- Voter Engagement
- Community Engagement Partnership Initiative
- Research News
- Centers and Institutes
- Baxter Arena
- Omaha Mavericks Website
- Transcripts
- Thompson Center
Quantitative Literacy
Related content.
- General Education Home
- General Education Curriculum
- General Education Courses Distribution Area
- Student Learning Outcomes
- General Education Approval Process
- General Education Assessment
- General Education Committee
- Transfer Guide and Articulation Agreements
- Value of General Education
- Frequently Asked Questions
- Faculty and Staff Resources
Quantitative Literacy involves using mathematical, computational, or statistical methods, with significant applications across a wide variety of disciplines.
It emphasizes the process of formulating, solving, interpreting, and applying equations of different types to solve many different real-world problems.
UNO Graduates will demonstrate competency in using mathematical, computational, or statistical methods to:
- Solve real-world problems;
- Draw inferences based on a set of data or quantitative information; and
- Justify conclusions derived from quantitative information
Fundamental Academic Skills
Quantitative Literacy, English & Writing, and Public Speaking coursework comprise 15 hours of the general education requirements. Students must complete three credit hours of Quantitative Literacy coursework.
Learn more about fundamental academic skills
Below is a list of Mathematics courses that have been approved for inclusion in the general education curriculum.
Quantitative Literacy Approved Courses
** Effective fall semester 2018 MATH 1220 will replace MATH 1310 to fulfill General Education Quantitative Literacy requirement. MATH 1310 will be accepted if it is taken prior to Fall 2018.
All students will still be required to meet any additional math requirements and/or math prerequisites in their program of study, and are highly encouraged to consult with their academic advisor before enrolling in a particular course.
Students are considered proficient in meeting their general education Quantitative Literacy requirement if they have an ACT Math score of 23 or higher or an SAT Math score of 540 or higher or SAT2016 of 570 or higher.
There are several other ways students can fulfill their general education quantitative literacy requirement if they do not have a high enough ACT/SAT score:
- Take one of the courses identified above
- Transfer credit for one of these courses from another institution
- Credit for another college-level math course determined to exceed UNO general education quantitative literacy requirements.
The Math Placement Exam or Accuplacer Exam into a General Education math course does not equal proficiency in meeting General Education Quantitative Literacy requirements.
Additional information about MATH Placement .
- Academic advising at the UW
- Working with us
- Schedule an advising appointment
- Springboard
- Your UW degree
- Requirements by college and school
- List of Undergraduate Majors
- Majors by Topic
- Majors and Careers
- Double degree and double major
- Satisfactory Progress Policy and Pre-major Extension
- Plan your degree
- For new students
- Transfer Newsletter
- Pre-health/pre-law
- Registration restrictions
- Cross-campus registration
- Placement testing
- Chemistry, math, & physics
- Academic terms and policies
- Co-curricular engagement
- Academic support at the UW
- Finding help
- Departmental & Major Advising Offices
- Dropping classes
- Satisfactory progress policy and pre-major extension
- Academic Warning and Academic Probation
- Reinstatement for College of Arts & Sciences
- Frequently asked questions
- Second-year
- First-generation
- International student guide
- Faculty & Staff
Although many students meet the requirement with a mathematics course, either because their intended majors require math or because they enjoy it, other students prefer to take a course that emphasizes reasoning or mathematical applications rather than traditional math.
Many students, for example, take economics to gain some insight into the world of business and finance. Many economic principles are expressed in mathematical terms, and in an introductory economics course you will apply simple mathematical principles to real-life situations.
Students interested in the study of language — students who may major or minor in a foreign language, English, communications, a behavioral science, or speech, for example — may wish to take linguistics, which sharpens awareness of verbal reasoning through the study of the history, nature, and structure of language.
We also offer courses entirely devoted to the study of reasoning and logical argument: PHIL 115: Practical Reasoning, and PHIL 120: Introduction to Logic.
Grade required
Any passing grade (0.7 or higher) is acceptable. The course may not be taken on the satisfactory/not satisfactory (S/NS) grading option.
The Math Department recommends taking the Guided Self-PacementAssessment before registration in MATH 111, 120, and 124. For the prerequisites and registration restrictions of other Reasoning courses, consult the Course Descriptions .
Overlaps with other requirements
You may also count your Reasoning course toward the Areas of Inquiry requirement, in whichever Area it is listed. (A few Reasoning courses do not count toward Areas of Inquiry.) If your Reasoning course happens to be a W-course and/or is part of your major or minor, it can count toward those requirements as well.
There are several AP scores that can be used to satisfy the Reasoning requirement and can also be counted toward Areas of Inquiry. See the AP tables for more information.
There are several International Baccalaureate Higher Level exams that can be used to satisfy the Reasoning requirement and can also be counted toward Areas of Inquiry. See the IB tables for more information.
For transfer students
Any course that transfers as a "Q" course (for example, PSYCH Q-1XX), or any MATH X or STAT X course, will satisfy the Reasoning requirement if the course transfers as at least 4 credits. For other courses, consult an adviser. Bring a copy of the course syllabus or the description from the catalog of the college offering the course.
You can check the UW Equivalency Guide for Washington Community and Technical Colleges to determine which courses from Washington community colleges count toward the UW's Reasoning requirement; they are marked in the lists with an RSN notation.
Postbaccalaureate students
Postbaccalaureate students are not required to complete the Reasoning requirement.
Registering for Reasoning courses
You can generate a complete list of Reasoning courses with space still available using the MyPlan course search .
Degree overview
© 2024 University of Washington | Seattle, WA
The Quantitative Experience Statement Explained
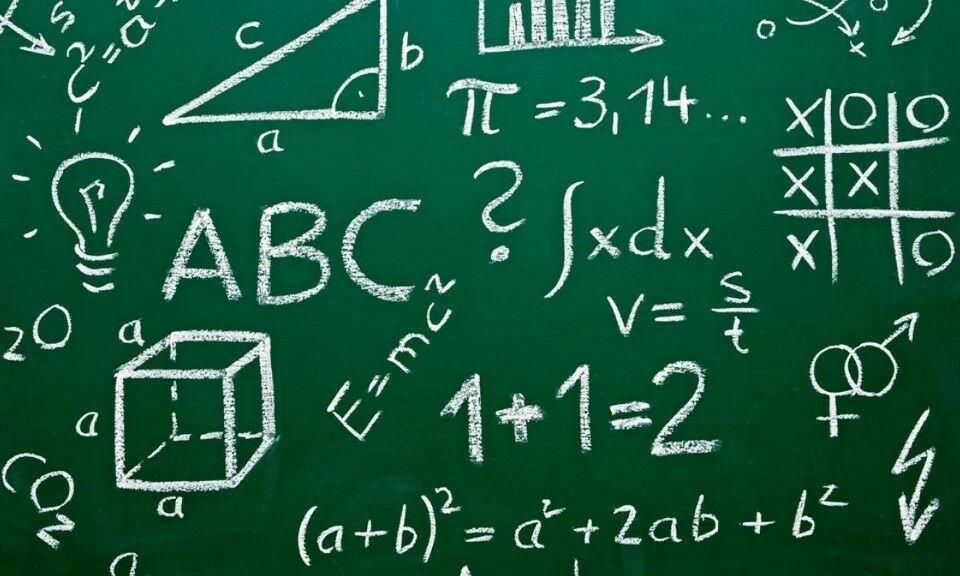
A common question we get asked is – what is the quantitative experience statement? All MSc applicants are required to complete this section of the application form and it helps us to assess your academic background.
We understand that this may seem daunting if your first degree was in a non-quantitative subject and if you have studied a quantitative subject at degree level, then you might also be confused about which topics to include. The quantitative experience statement is essentially a list of the five most quantitative modules you have studied. If you have not studied five quantitative modules at degree level you can include older achievements e.g. A-level Maths, IB Maths.
Familiarity with basic mathematics will help you whilst on the programme and with your future career
All of our MSc programmes contain quantitative elements so all candidates are expected to be familiar with a basic level of mathematical knowledge. For the less quantitative MSc Programmes such as MSc Strategic Marketing , MSc International Health Management , and MSc Climate Change, Management & Finance , it is totally fine if you haven’t studied maths beyond compulsory classes in high school.
Sometimes really strong candidates can be intimidated by the quantitative experience statement, don’t be put off by this – we ask about your quantitative abilities to encourage you to consider the programme content and your future career path. Regardless of the role, there is no harm in being confident with numbers so that you can better understand data in your job.
Most of our Management MSc programmes are open to graduates from all academic backgrounds and a considerable proportion of our current classes have studied an arts or humanities degree.
If you haven’t studied maths for a long time, doing a GMAT or GRE test will give you a chance to brush up on your quantitative skills and can add weight to your application. The confidence from a good score in the quantitative section of the test will place you in a good position for the online self-studying modules over the summer and may even encourage you to consider programme pathways and careers that you had not previously.
Check the quantitative experience requirements before applying
For our more quantitative programmes ( MSc Finance , MSc Finance & Accounting , MSc Financial Technology , MSc Investment & Wealth Management , MSc Business Analytics , MSc Risk Management & Financial Engineering and MSc Economics & Strategy for Business ) this a key section of the application form that does influence the selection process so please check the entry requirements for your programme before you apply.
Jerry Voong, Admissions Officer for MSc Economics and Strategy for Business has some advice for prospective applicants.
“If it isn’t clear on your transcript that you have studied macro AND microeconomics then the quantitative experience statement is a great place to show us. Expand on the “introduction to economics” module on your transcript and outline what you studied.”
The finance suite of programmes are much more rigorous and students are expected to join the programme with an advanced level of mathematical skills. Ensuring that you have chosen the programme that best suits your previous studies can be key to a successful application. Sarah Eggleton, the Admissions Officer for the Finance Masters' programmes comments on this saying “it’s always really disappointing when you see a really great candidate without the quantitative grounding to allow them to succeed on a Finance Masters''”. This means that we won’t be able to make an offer even if the student would otherwise be a really good fit for the Business School.
Applicants for MSc Business Analytics must have a quantitative background to ensure that they have the best chance of success to be proficient in R, Python and SQL by the end of the programme. Most of the modules in the programme use at least one of these languages. Whilst prior experience in coding is not required, all students must be willing to learn programming from the start of the programme. Many admitted students will have already taken free online classes out of general interest if they did not study coding as part of their degree or through their job.
Applying for the finance suite of programmes? Take our online maths test!
We advise all prospective students for MSc Finance, MSc Finance & Accounting, MSc Financial Technology, MSc Investment & Wealth Management and MSc Risk Management & Financial Engineering programmes to take our online maths test . This gives candidates a chance to test their quantitative ability and determine if they are suitable to apply for one of these programmes. This maths test is the minimum level that is required to succeed. Almost 50% of the current Risk Management & Financial Engineering students have a background in mathematics, with the remainder having studied subjects such as engineering, economics and finance.
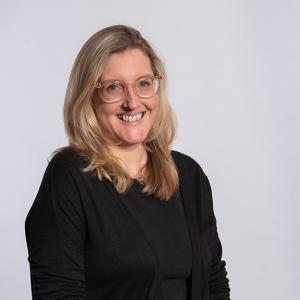
About Sarah Eggleton
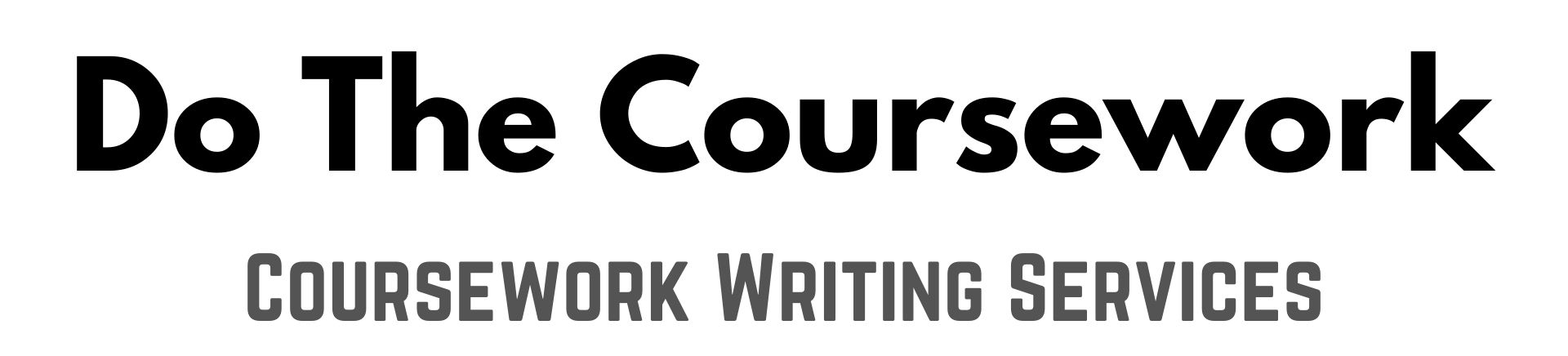
What Is Quantitative Coursework?
Some students are taught to optimize the use of textbook information and refer to notes in class. While this is not a bad thing, it can oftentimes lead to a poor grade average and lower test scores. It can also lead to unnecessary frustration because so many students are given the same tests. Professors often assign tests based on the class workload. This means that some students have to spend extra time studying for their exams, while others barely have time for class.
Most professors want their students to learn as much as they can, but they do not want to spend all of the hours necessary to get there. In an effort to make sure that their courses are as easy as possible for their students, instructors will often make sure that homework is not done on the class day. Homework should only be done after class or two hours before class. Doing your homework ahead of time will enable you to study and learn more, thus increasing your grade.
Students need to learn what is considered quantitative coursework, too. They need to understand what is included in the syllabus, what types of information they will be presenting, and how they will present it. This will not only enhance their skills as students, but it will also help them when it comes to qualifying for an exam. Knowing the requirements before getting started will save the student from spending extra time researching the course. Doing the course may be considered as part of the coursework, depending on the instructor.
Another thing that needs to be understood about what is considered quantitative coursework is that there are usually set class times for classes that involve this type of material. There may also be tests given at the end of the class in order for the instructor to gauge how well the students have done with the material. The tests will determine whether or not the student has learned the material thoroughly and have been able to apply the material accordingly. If the test scores are poor, instructors may assign more homework or assign a different class next semester.
What is considered quantitative coursework? It is a type of course that combines math with business. It is usually used by business majors in order to prepare themselves for taking the MBA (Master of Business Administration) examination. Usually, MBA applicants must first take this examination, and this class helps them learn what they will be facing on the MBAT. By taking the course, students will know exactly what to expect during the exam.
An important part of what is considered quantitative coursework is the homework assignments. In most cases, these are based on previous learning in the class, either through notes or directly from the professor. The assignments should help the student to understand the subject well enough in order to answer the questions that will appear on the test. In some cases, the instructor will give homework to be done immediately, and the student will need to do them one at a time and understand each thoroughly before moving on to the next section. Homework should also help the student to see the types of statistical methods that will be tested on the test.
It is important to understand what is quantitative coursework before taking the class. Studying what is involved and what it will lead to, will help make sure that the class is the right one for one’s needs. Students should also have a full understanding about what will be expected in the final project, which is what will ultimately be passed out and graded. By using the guidance in class, understanding what is considered quantitative coursework will ensure success on the test day.
We Are Here To Assist You
Here are a few letters your customers love. s a l e. do you know how we know because the days when retailers offer their biggest discounts..
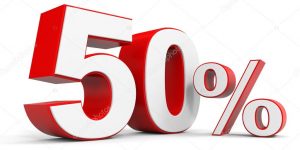
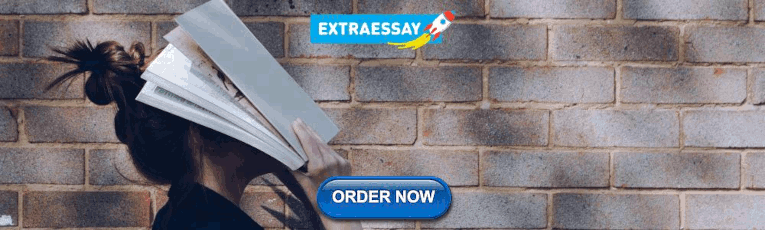
IMAGES
VIDEO
COMMENTS
What is Quantitative Research? Quantitative methodology is the dominant research framework in the social sciences. It refers to a set of strategies, techniques and assumptions used to study psychological, social and economic processes through the exploration of numeric patterns. Quantitative research gathers a range of numeric data.
The Quantitative Sciences (QSS) major is the integration of liberal arts and data science. With the QSS major, students learn data science techniques and quantitative theory while they study the natural sciences, social sciences, or humanities. ... The core quantitative coursework is the same no matter what path you eventually pick.
This course will cover the fundamental principles of science, some history and philosophy of science, research designs, measurement, sampling and ethics. The course is comparable to a university level introductory course on quantitative research methods in the social sciences, but has a strong focus on research integrity.
Learn Quantitative or improve your skills online today. Choose from a wide range of Quantitative courses offered from top universities and industry leaders. Our Quantitative courses are perfect for individuals or for corporate Quantitative training to upskill your workforce.
Our quantitative research courses are tailored to provide you with the knowledge and skills needed to excel in the field of data analysis, statistical modeling, and research methodology. Whether you are pursuing a career in academia, market research, or data-driven decision making, our courses will equip you with the tools to succeed in this ...
Revised on June 22, 2023. Quantitative research is the process of collecting and analyzing numerical data. It can be used to find patterns and averages, make predictions, test causal relationships, and generalize results to wider populations. Quantitative research is the opposite of qualitative research, which involves collecting and analyzing ...
course are in the areas of probability theory, estimation, hypothesis testing, regression analysis, causal inference and prediction. While many students taking this class will have already taken courses in statistical inference and regression analysis, this course will place a much stronger emphasis than typical
Quantitative Methods is an introduction to using statistical methods to solve business problems. The course teaches statistics from the management perspective and places special emphasis on developing the skills and instincts needed to make good decisions and become a more effective manager. The course is set in Hawaii at a fictional resort hotel. Students act as consultants hired to develop ...
For many, understanding what quantitative coursework means is a puzzle that can be solved with a bit of information. The rest of this article provides some of the answers you. are probably looking for regarding what is quantitative coursework. Quotient coursework refers to a specific segment of study. Typically, it includes statistics ...
The course also lays the necessary foundation for future coursework in quantitative methods. PLSC 30600 Causal Inference (Winter) This is the third course in quantitative methods in the Political Science PhD program. The course is an introduction to the theory and practice of causal inference from quantitative data. It will cover the potential ...
Quantitative reasoning is the act of understanding mathematical facts and concepts and being able to apply them to real-world scenarios. A nice four-step process to tackle these types of problems ...
This video lecture discusses the meaning and characteristics of Quantitative Research. The content of this video lecture is different from the content of the...
Quantitative courses in the College of the Environment focus on examples from the physical world and from the living natural world, including conservation at landscape scale and impacts of global change. We teach the basics of math, stats, coding and mapping, through to advanced coursework in modeling, computation and remote sensing. Our ...
Finance & Quantitative Modeling for Analysts. Skills you'll gain: Financial Analysis, Financial Modeling, Cash Flow Forecasting, Financial Statements, Mathematics and Mathematical Modeling, Microsoft Excel, Probability and Statistics, Regression Analysis, Finance, Simulation and Simulation Software, Spreadsheets. 4.5. (16K reviews)
Quantitative Reasoning Part A (QR‐A) Description: A Quantitative Reasoning Part A course is an introductory course in college‐level mathematics, computer science, statistics or formal logic that prepares students for more advanced work in a disciplinary context. Quantitative Reasoning Part A Learning Outcomes: Using quantitative information ...
Quantitative research: Uses statistical analysis tools. Is usually expressed in numbers, charts and graphs. Requires a large group of study participants. Gathers data using closed questions. In contrast, qualitative research: Uses categorizing, summarizing and interpreting to develop understanding. Is usually expressed in codes, themes and ...
Quantitative Literacy. Quantitative Literacy involves using mathematical, computational, or statistical methods, with significant applications across a wide variety of disciplines. It emphasizes the process of formulating, solving, interpreting, and applying equations of different types to solve many different real-world problems.
Reasoning. Although many students meet the requirement with a mathematics course, either because their intended majors require math or because they enjoy it, other students prefer to take a course that emphasizes reasoning or mathematical applications rather than traditional math. Many students, for example, take economics to gain some insight ...
The quantitative experience statement is essentially a list of the five most quantitative modules you have studied. If you have not studied five quantitative modules at degree level you can include older achievements e.g. A-level Maths, IB Maths. Familiarity with basic mathematics will help you whilst on the programme and with your future career
Students can access learning tools through websites that can help them complete what is considered a quantitative course. These include using spreadsheets to track data, creating charts and graphs, and inputting data into computer programs that can further analyze the data. The tools and processes used in what is considered a quantitative ...
The Quantitative and Language Resume is basically a tally of, in your own words, the quantitative experience (both academic and professional) and linguistic exposure you posses. Your content should show you can keep up with the required courses for the degree program you are applying to. For example, if you are applying to the Economic and ...
What is considered quantitative coursework? It is a type of course that combines math with business. It is usually used by business majors in order to prepare themselves for taking the MBA (Master of Business Administration) examination. Usually, MBA applicants must first take this examination, and this class helps them learn what they will be ...