- Open access
- Published: 06 April 2020
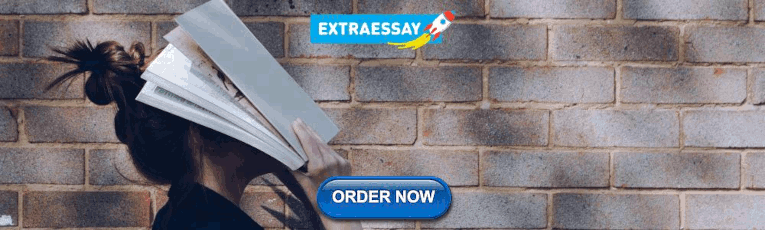
The influence of education on health: an empirical assessment of OECD countries for the period 1995–2015
- Viju Raghupathi 1 &
- Wullianallur Raghupathi 2
Archives of Public Health volume 78 , Article number: 20 ( 2020 ) Cite this article
208k Accesses
249 Citations
170 Altmetric
Metrics details
A clear understanding of the macro-level contexts in which education impacts health is integral to improving national health administration and policy. In this research, we use a visual analytic approach to explore the association between education and health over a 20-year period for countries around the world.
Using empirical data from the OECD and the World Bank for 26 OECD countries for the years 1995–2015, we identify patterns/associations between education and health indicators. By incorporating pre- and post-educational attainment indicators, we highlight the dual role of education as both a driver of opportunity as well as of inequality.
Adults with higher educational attainment have better health and lifespans compared to their less-educated peers. We highlight that tertiary education, particularly, is critical in influencing infant mortality, life expectancy, child vaccination, and enrollment rates. In addition, an economy needs to consider potential years of life lost (premature mortality) as a measure of health quality.
Conclusions
We bring to light the health disparities across countries and suggest implications for governments to target educational interventions that can reduce inequalities and improve health. Our country-level findings on NEET (Not in Employment, Education or Training) rates offer implications for economies to address a broad array of vulnerabilities ranging from unemployment, school life expectancy, and labor market discouragement. The health effects of education are at the grass roots-creating better overall self-awareness on personal health and making healthcare more accessible.
Peer Review reports
Introduction
Is education generally associated with good health? There is a growing body of research that has been exploring the influence of education on health. Even in highly developed countries like the United States, it has been observed that adults with lower educational attainment suffer from poor health when compared to other populations [ 36 ]. This pattern is attributed to the large health inequalities brought about by education. A clear understanding of the health benefits of education can therefore serve as the key to reducing health disparities and improving the well-being of future populations. Despite the growing attention, research in the education–health area does not offer definitive answers to some critical questions. Part of the reason is the fact that the two phenomena are interlinked through life spans within and across generations of populations [ 36 ], thereby involving a larger social context within which the association is embedded. To some extent, research has also not considered the variances in the education–health relationship through the course of life or across birth cohorts [ 20 ], or if there is causality in the same. There is therefore a growing need for new directions in education–health research.
The avenues through which education affects health are complex and interwoven. For one, at the very outset, the distribution and content of education changes over time [ 20 ]. Second, the relationship between the mediators and health may change over time, as healthcare becomes more expensive and/or industries become either more, or less hazardous. Third, some research has documented that even relative changes in socioeconomic status (SES) can affect health, and thus changes in the distribution of education implies potential changes in the relationship between education and health. The relative index of inequality summarizes the magnitude of SES as a source of inequalities in health [ 11 , 21 , 27 , 29 ]. Fourth, changes in the distribution of health and mortality imply that the paths to poor health may have changed, thereby affecting the association with education.
Research has proposed that the relationship between education and health is attributable to three general classes of mediators: economic; social, psychological, and interpersonal; and behavioral health [ 31 ]. Economic variables such as income and occupation mediate the relationship between education and health by controlling and determining access to acute and preventive medical care [ 1 , 2 , 19 ]. Social, psychological, and interpersonal resources allow people with different levels of education to access coping resources and strategies [ 10 , 34 ], social support [ 5 , 22 ], and problem-solving and cognitive abilities to handle ill-health consequences such as stress [ 16 ]. Healthy behaviors enable educated individuals to recognize symptoms of ill health in a timely manner and seek appropriate medical help [ 14 , 35 ].
While the positive association between education and health has been established, the explanations for this association are not [ 31 ]. People who are well educated experience better health as reflected in the high levels of self-reported health and low levels of morbidity, mortality, and disability. By extension, low educational attainment is associated with self-reported poor health, shorter life expectancy, and shorter survival when sick. Prior research has suggested that the association between education and health is a complicated one, with a range of potential indicators that include (but are not limited to) interrelationships between demographic and family background indicators [ 8 ] - effects of poor health in childhood, greater resources associated with higher levels of education, appreciation of good health behaviors, and access to social networks. Some evidence suggests that education is strongly linked to health determinants such as preventative care [ 9 ]. Education helps promote and sustain healthy lifestyles and positive choices, nurture relationships, and enhance personal, family, and community well-being. However, there are some adverse effects of education too [ 9 ]. Education may result in increased attention to preventive care, which, though beneficial in the long term, raises healthcare costs in the short term. Some studies have found a positive association between education and some forms of illicit drug and alcohol use. Finally, although education is said to be effective for depression, it has been found to have much less substantial impact in general happiness or well-being [ 9 ].
On a universal scale, it has been accepted that several social factors outside the realm of healthcare influence the health outcomes [ 37 ]. The differences in morbidity, mortality and risk factors in research, conducted within and between countries, are impacted by the characteristics of the physical and social environment, and the structural policies that shape them [ 37 ]. Among the developed countries, the United States reflects huge disparities in educational status over the last few decades [ 15 , 24 ]. Life expectancy, while increasing for all others, has decreased among white Americans without a high school diploma - particularly women [ 25 , 26 , 32 ]. The sources of inequality in educational opportunities for American youth include the neighborhood they live in, the color of their skin, the schools they attend, and the financial resources of their families. In addition, the adverse trends in mortality and morbidity brought on by opioids resulting in suicides and overdoses (referred to as deaths of despair) exacerbated the disparities [ 21 ]. Collectively, these trends have brought about large economic and social inequalities in society such that the people with more education are likely to have more health literacy, live longer, experience better health outcomes, practice health promoting behaviors, and obtain timely health checkups [ 21 , 17 ].
Education enables people to develop a broad range of skills and traits (including cognitive and problem-solving abilities, learned effectiveness, and personal control) that predispose them towards improved health outcomes [ 23 ], ultimately contributing to human capital. Over the years, education has paved the way for a country’s financial security, stable employment, and social success [ 3 ]. Countries that adopt policies for the improvement of education also reap the benefits of healthy behavior such as reducing the population rates of smoking and obesity. Reducing health disparities and improving citizen health can be accomplished only through a thorough understanding of the health benefits conferred by education.
There is an iterative relationship between education and health. While poor education is associated with poor health due to income, resources, healthy behaviors, healthy neighborhood, and other socioeconomic factors, poor health, in turn, is associated with educational setbacks and interference with schooling through difficulties with learning disabilities, absenteeism, or cognitive disorders [ 30 ]. Education is therefore considered an important social determinant of health. The influence of national education on health works through a variety of mechanisms. Generally, education shows a relationship with self-rated health, and thus those with the highest education may have the best health [ 30 ]. Also, health-risk behaviors seem to be reduced by higher expenditure into the publicly funded education system [ 18 ], and those with good education are likely to have better knowledge of diseases [ 33 ]. In general, the education–health gradients for individuals have been growing over time [ 38 ].
To inform future education and health policies effectively, one needs to observe and analyze the opportunities that education generates during the early life span of individuals. This necessitates the adoption of some fundamental premises in research. Research must go beyond pure educational attainment and consider the associated effects preceding and succeeding such attainment. Research should consider the variations brought about by the education–health association across place and time, including the drivers that influence such variations [ 36 ].
In the current research, we analyze the association between education and health indicators for various countries using empirical data from reliable sources such as the Organization for Economic Cooperation and Development (OECD) and World Bank. While many studies explore the relationship between education and health at a conceptual level, we deploy an empirical approach in investigating the patterns and relationships between the two sets of indicators. In addition, for the educational indicators, we not only incorporate the level of educational attainment, but also look at the potential socioeconomic benefits, such as enrollment rates (in each sector of educational level) and school life expectancy (at each educational level). We investigate the influences of educational indicators on national health indicators of infant mortality, child vaccinations, life expectancy at birth, premature mortality arising from lack of educational attainment, employment and training, and the level of national health expenditure. Our research question is:
What are some key influencers/drivers in the education-health relationship at a country level?
The current study is important because policy makers have an increasing concern on national health issues and on policies that support it. The effect of education is at the root level—creating better overall self-awareness on personal health and making healthcare more accessible. The paper is organized as follows: Section 2 discusses the background for the research. Section 3 discusses the research method; Section 4 offers the analysis and results; Section 5 provides a synthesis of the results and offers an integrated discussion; Section 6 contains the scope and limitations of the research; Section 7 offers conclusions with implications and directions for future research.
Research has traditionally drawn from three broad theoretical perspectives in conceptualizing the relationship between education and health. The majority of research over the past two decades has been grounded in the Fundamental Cause Theory (FCT) [ 28 ], which posits that factors such as education are fundamental social causes of health inequalities because they determine access to resources (such as income, safe neighborhoods, or healthier lifestyles) that can assist in protecting or enhancing health [ 36 ]. Some of the key social resources that contribute to socioeconomic status include education (knowledge), money, power, prestige, and social connections. As some of these undergo change, they will be associated with differentials in the health status of the population [ 12 ].
Education has also been conceptualized using the Human Capital Theory (HCT) that views it as a return on investment in the form of increased productivity [ 4 ]. Education improves knowledge, skills, reasoning, effectiveness, and a broad range of other abilities that can be applied to improving health. The third approach - the signaling or credentialing perspective [ 6 ] - is adopted to address the large discontinuities in health at 12 and 16 years of schooling, which are typically associated with the receipt of a high school diploma and a college degree, respectively. This perspective considers the earned credentials of a person as a potential source that warrants social and economic returns. All these theoretical perspectives postulate a strong association between education and health and identify mechanisms through which education influences health. While the HCT proposes the mechanisms as embodied skills and abilities, FCT emphasizes the dynamism and flexibility of mechanisms, and the credentialing perspective proposes educational attainment through social responses. It needs to be stated, however, that all these approaches focus on education solely in terms of attainment, without emphasizing other institutional factors such as quality or type of education that may independently influence health. Additionally, while these approaches highlight the individual factors (individual attainment, attainment effects, and mechanisms), they do not give much emphasis to the social context in which education and health processes are embedded.
In the current research while we acknowledge the tenets of these theoretical perspectives, we incorporate the social mechanisms in education such as level of education, skills and abilities brought about by enrollment, school life expectancy, and the potential loss brought about by premature mortality. In this manner, we highlight the relevance of the social context in which the education and health domains are situated. We also study the dynamism of the mechanisms over countries and over time and incorporate the influences that precede and succeed educational attainment.
We analyze country level education and health data from the OECD and World Bank for a period of 21 years (1995–2015). Our variables include the education indicators of adult education level; enrollment rates at various educational levels; NEET (Not in Employment, Education or Training) rates; school life expectancy; and the health indicators of infant mortality, child vaccination rates, deaths from cancer, life expectancy at birth, potential years of life lost and smoking rates (Table 1 ). The data was processed using the tools of Tableau for visualization, and SAS for correlation and descriptive statistics. Approaches for analysis include ranking, association, and data visualization of the health and education data.
Analyses and results
In this section we identify and analyze patterns and associations between education and health indicators and discuss the results. Since countries vary in population sizes and other criteria, we use the estimated averages in all our analyses.
Comparison of health outcomes for countries by GDP per capita
We first analyzed to see if our data reflected the expectation that countries with higher GDP per capita have better health status (Fig. 1 ). We compared the average life expectancy at birth, average infant mortality, average deaths from cancer and average potential year of life lost, for different levels of GDP per capita (Fig. 1 ).
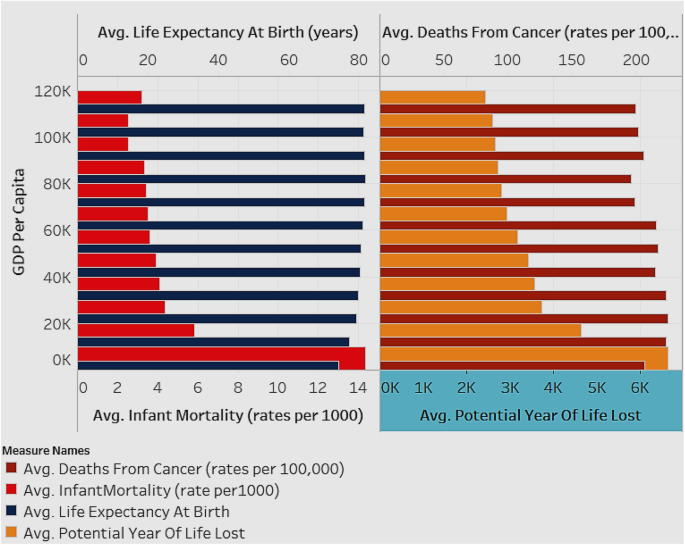
Associations between Average Life Expectancy (years) and Average Infant Mortality rate (per 1000), and between Deaths from Cancer (rates per 100,000) and Average Potential Years of Life Lost (years), by GDP per capita (for all countries for years 1995–2015)
Figure 1 depicts two charts with the estimated averages of variables for all countries in the sample. The X-axis of the first chart depicts average infant mortality rate (per 1000), while that of the second chart depicts average potential years of life lost (years). The Y-axis for both charts depicts the GDP per capita shown in intervals of 10 K ranging from 0 K–110 K (US Dollars). The analysis is shown as an average for all the countries in the sample and for all the years (1995–2015). As seen in Fig. 1 , countries with lower GDP per capita have higher infant mortality rate and increased potential year of life lost (which represents the average years a person would have lived if he or she had not died prematurely - a measure of premature mortality). Life expectancy and deaths from cancer are not affected by GDP level. When studying infant mortality and potential year lost, in order to avoid the influence of a control variable, it was necessary to group the samples by their GDP per capita level.
Association of Infant Mortality Rates with enrollment rates and education levels
We explored the association of infant mortality rates with the enrollment rates and adult educational levels for all countries (Fig. 2 ). The expectation is that with higher education and employment the infant mortality rate decreases.
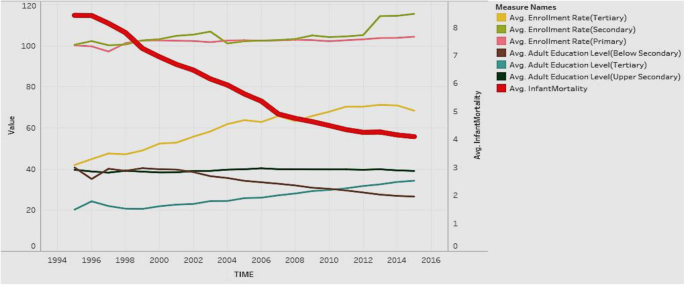
Association of Adult Education Levels (ratio) and Enrollment Rates (ratio) with Infant Mortality Rate (per 1000)
Figure 2 depicts the analysis for all countries in the sample. The figure shows the years from 1995 to 2015 on the X axis. It shows two Y-axes with one axis denoting average infant mortality rate (per 1000 live births), and the other showing the rates from 0 to 120 to depict enrollment rates (primary/secondary/tertiary) and education levels (below secondary/upper secondary/tertiary). Regarding the Y axis showing rates over 100, it is worth noting that the enrollment rates denote a ratio of the total enrollment (regardless of age) at a level of education to the official population of the age group in that education level. Therefore, it is possible for the number of children enrolled at a level to exceed the official population of students in the age group for that level (due to repetition or late entry). This can lead to ratios over 100%. The figure shows that in general, all education indicators tend to rise over time, except for adult education level below secondary, which decreases over time. Infant mortality shows a steep decreasing trend over time, which is favorable. In general, countries have increasing health status and education over time, along with decreasing infant mortality rates. This suggests a negative association of education and enrollment rates with mortality rates.
Association of Education Outcomes with life expectancy at birth
We explored if the education outcomes of adult education level (tertiary), school life expectancy (tertiary), and NEET (not in employment, education, or training) rates, affected life expectancy at birth (Fig. 3 ). Our expectation is that adult education and school life expectancy, particularly tertiary, have a positive influence, while NEET has an adverse influence, on life expectancy at birth.
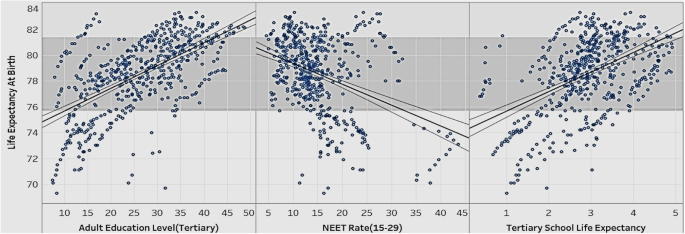
Association of Adult Education Level (Tertiary), NEET rate, School Life Expectancy (Tertiary), with Life Expectancy at Birth
Figure 3 show the relationships between various education indicators (adult education level-tertiary, NEET rate, school life expectancy-tertiary) and life expectancy at birth for all countries in the sample. The figure suggests that life expectancy at birth rises as adult education level (tertiary) and tertiary school life expectancy go up. Life expectancy at birth drops as the NEET rate goes up. In order to extend people’s life expectancy, governments should try to improve tertiary education, and control the number of youths dropping out of school and ending up unemployed (the NEET rate).
Association of Tertiary Enrollment and Education with potential years of life lost
We wanted to explore if the potential years of life lost rates are affected by tertiary enrollment rates and tertiary adult education levels (Fig. 4 ).
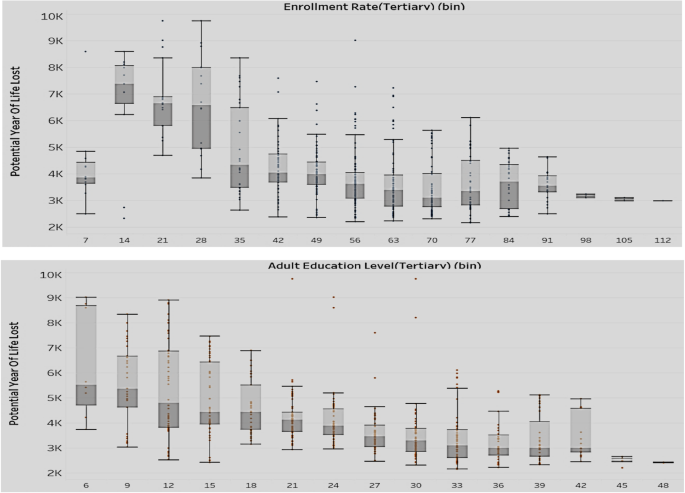
Association of Enrollment rate-tertiary (top) and Adult Education Level-Tertiary (bottom) with Potential Years of Life Lost (Y axis)
The two sets of box plots in Fig. 4 compare the enrollment rates with potential years of life lost (above set) and the education level with potential years of life lost (below set). The analysis is for all countries in the sample. As mentioned earlier, the enrollment rates are expressed as ratios and can exceed 100% if the number of children enrolled at a level (regardless of age) exceed the official population of students in the age group for that level. Potential years of life lost represents the average years a person would have lived, had he/she not died prematurely. The results show that with the rise of tertiary adult education level and tertiary enrollment rate, there is a decrease in both value and variation of the potential years of life lost. We can conclude that lower levels in tertiary education adversely affect a country’s health situation in terms of premature mortality.
Association of Tertiary Enrollment and Education with child vaccination rates
We compared the performance of tertiary education level and enrollment rates with the child vaccination rates (Fig. 5 ) to assess if there was a positive impact of education on preventive healthcare.
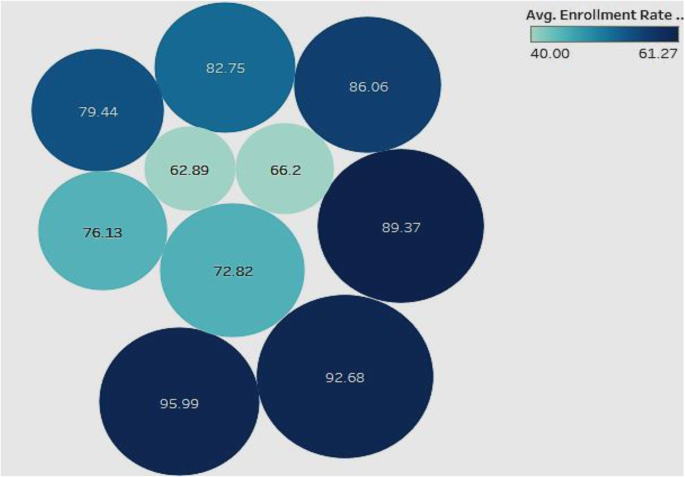
Association of Adult Education Level-Tertiary and Enrollment Rate-Tertiary with Child Vaccination Rates
In this analysis (Fig. 5 ), we looked for associations of child vaccination rates with tertiary enrollment and tertiary education. The analysis is for all countries in the sample. The color of the bubble represents the tertiary enrollment rate such that the darker the color, the higher the enrollment rate, and the size of the bubble represents the level of tertiary education. The labels inside the bubbles denote the child vaccination rates. The figure shows a general positive association of high child vaccination rate with tertiary enrollment and tertiary education levels. This indicates that countries that have high child vaccination rates tend to be better at tertiary enrollment and have more adults educated in tertiary institutions. Therefore, countries that focus more on tertiary education and enrollment may confer more health awareness in the population, which can be reflected in improved child vaccination rates.
Association of NEET rates (15–19; 20–24) with infant mortality rates and deaths from Cancer
In the realm of child health, we also looked at the infant mortality rates. We explored if infant mortality rates are associated with the NEET rates in different age groups (Fig. 6 ).
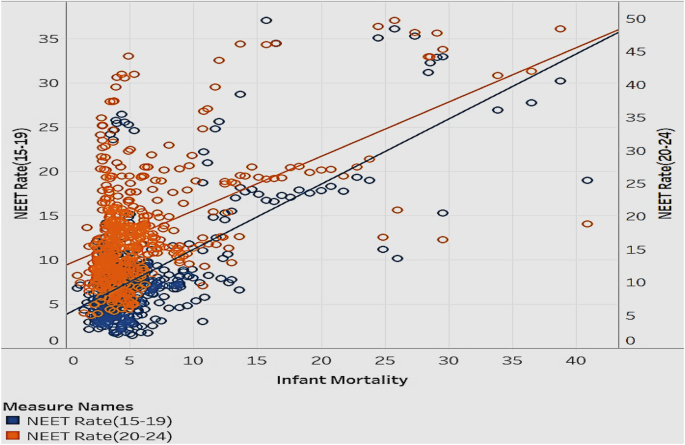
Association of Infant Mortality rates with NEET Rates (15–19) and NEET Rates (20–24)
Figure 6 is a scatterplot that explores the correlation between infant mortality and NEET rates in the age groups 15–19 and 20–24. The data is for all countries in the sample. Most data points are clustered in the lower infant mortality and lower NEET rate range. Infant mortality and NEET rates move in the same direction—as infant mortality increases/decrease, the NEET rate goes up/down. The NEET rate for the age group 20–24 has a slightly higher infant mortality rate than the NEET rate for the age group 15–19. This implies that when people in the age group 20–24 are uneducated or unemployed, the implications on infant mortality are higher than in other age groups. This is a reasonable association, since there is the potential to have more people with children in this age group than in the teenage group. To reduce the risk of infant mortality, governments should decrease NEET rates through promotional programs that disseminate the benefits of being educated, employed, and trained [ 7 ]. Additionally, they can offer financial aid to public schools and companies to offer more resources to raise general health awareness in people.
We looked to see if the distribution of population without employment, education, or training (NEET) in various categories of high, medium, and low impacted the rate of deaths from cancer (Fig. 7 ). Our expectation is that high rates of NEET will positively influence deaths from cancer.
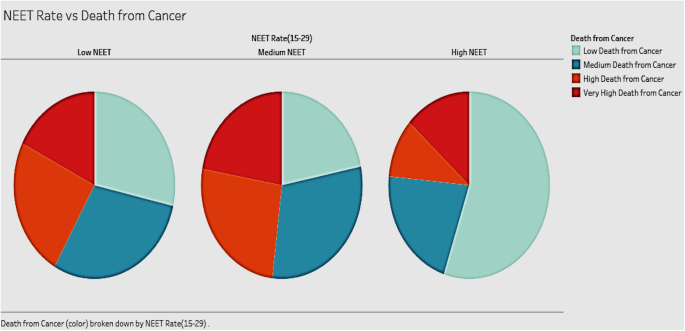
Association of Deaths from Cancer and different NEET Rates
The three pie charts in Fig. 7 show the distribution of deaths from cancer in groups of countries with different NEET rates (high, medium, and low). The analysis includes all countries in the sample. The expectation was that high rates of NEET would be associated with high rates of cancer deaths. Our results, however, show that countries with medium NEET rates tend to have the highest deaths from cancer. Countries with high NEET rates have the lowest deaths from cancer among the three groups. Contrary to expectations, countries with low NEET rates do not show the lowest death rates from cancer. A possible explanation for this can be attributed to the fact that in this group, the people in the labor force may be suffering from work-related hazards including stress, that endanger their health.
Association between adult education levels and health expenditure
It is interesting to note the relationship between health expenditure and adult education levels (Fig. 8 ). We expect them to be positively associated.
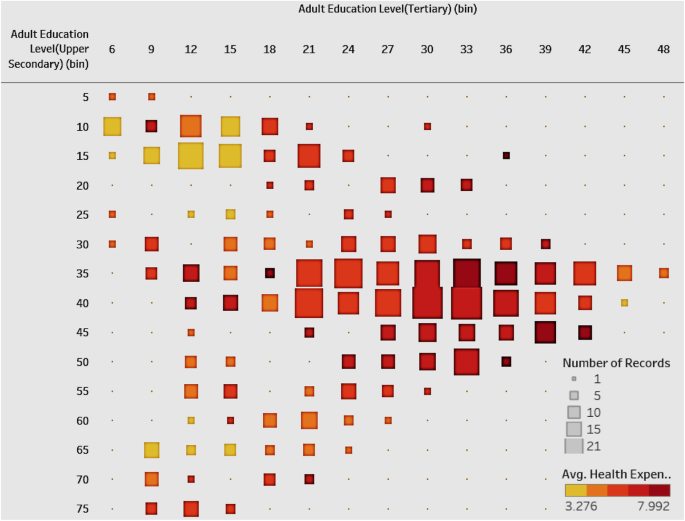
Association of Health Expenditure and Adult Education Level-Tertiary & Upper Secondary
Figure 8 shows a heat map with the number of countries in different combinations of groups between tertiary and upper-secondary adult education level. We emphasize the higher levels of adult education. The color of the square shows the average of health expenditure. The plot shows that most of the countries are divided into two clusters. One cluster has a high tertiary education level as well as a high upper-secondary education level and it has high average health expenditure. The other cluster has relatively low tertiary and upper secondary education level with low average health expenditure. Overall, the figure shows a positive correlation between adult education level and compulsory health expenditure. Governments of countries with low levels of education should allocate more health expenditure, which will have an influence on the educational levels. Alternatively, to improve public health, governments can frame educational policies to improve the overall national education level, which then produces more health awareness, contributing to national healthcare.
Association of Compulsory Health Expenditure with NEET rates by country and region
Having explored the relationship between health expenditure and adult education, we then explored the relationship between health expenditure and NEET rates of different countries (Fig. 9 ). We expect compulsory health expenditure to be negatively associated with NEET rates.
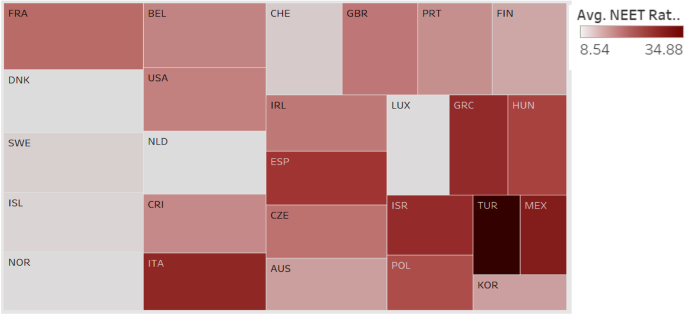
Association between Compulsory Health Expenditure and NEET Rate by Country and Region
In Fig. 9 , each box represents a country or region; the size of the box indicates the extent of compulsory health expenditure such that a larger box implies that the country has greater compulsory health expenditure. The intensity of the color of the box represents the NEET rate such that the darker color implies a higher NEET rate. Turkey has the highest NEET rate with low health expenditure. Most European countries such as France, Belgium, Sweden, and Norway have low NEET rates and high health expenditure. The chart shows a general association between low compulsory health expenditure and high NEET rates. The relationship, however, is not consistent, as there are countries with high NEET and high health expenditures. Our suggestion is for most countries to improve the social education for the youth through free training programs and other means to effectively improve the public health while they attempt to raise the compulsory expenditure.
Distribution of life expectancy at birth and tertiary enrollment rate
The distribution of enrollment rate (tertiary) and life expectancy of all the countries in the sample can give an idea of the current status of both education and health (Fig. 10 ). We expect these to be positively associated.
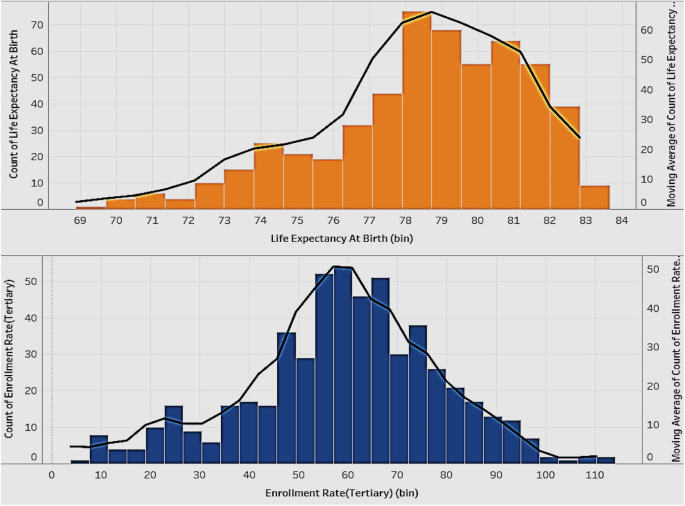
Distribution of Life Expectancy at Birth (years) and Tertiary Enrollment Rate
Figure 10 shows two histograms with the lines representing the distribution of life expectancy at birth and the tertiary enrollment rate of all the countries. The distribution of life expectancy at birth is skewed right, which means most of the countries have quite a high life expectancy and there are few countries with a very low life expectancy. The tertiary enrollment rate has a good distribution, which is closer to a normal distribution. Governments of countries with an extremely low life expectancy should try to identify the cause of this problem and take actions in time to improve the overall national health.
Comparison of adult education levels and deaths from Cancer at various levels of GDP per capita
We wanted to see if various levels of GDP per capita influence the levels of adult education and deaths from cancer in countries (Fig. 11 ).
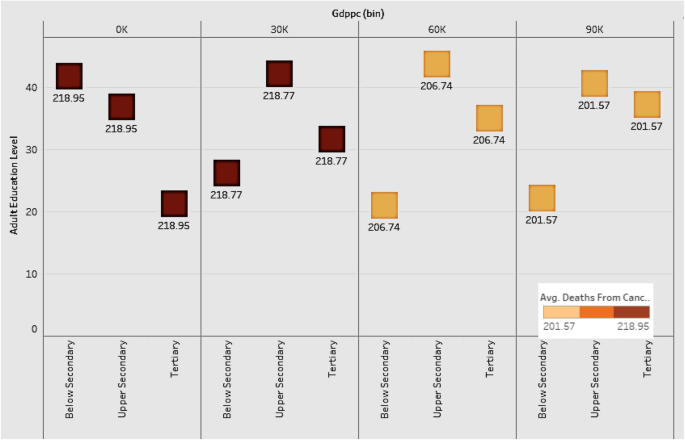
Comparison of Adult Education Levels and Deaths from Cancer at various levels of GDP per capita
Figure 11 shows the distribution of various adult education levels for countries by groups of GDP per capita. The plot shows that as GDP grows, the level of below-secondary adult education becomes lower, and the level of tertiary education gets higher. The upper-secondary education level is constant among all the groups. The implication is that tertiary education is the most important factor among all the education levels for a country to improve its economic power and health level. Countries should therefore focus on tertiary education as a driver of economic development. As for deaths from cancer, countries with lower GDP have higher death rates, indicating the negative association between economic development and deaths from cancer.
Distribution of infant mortality rates by continent
Infant mortality is an important indicator of a country’s health status. Figure 12 shows the distribution of infant mortality for the continents of Asia, Europe, Oceania, North and South America. We grouped the countries in each continent into high, medium, and low, based on infant mortality rates.
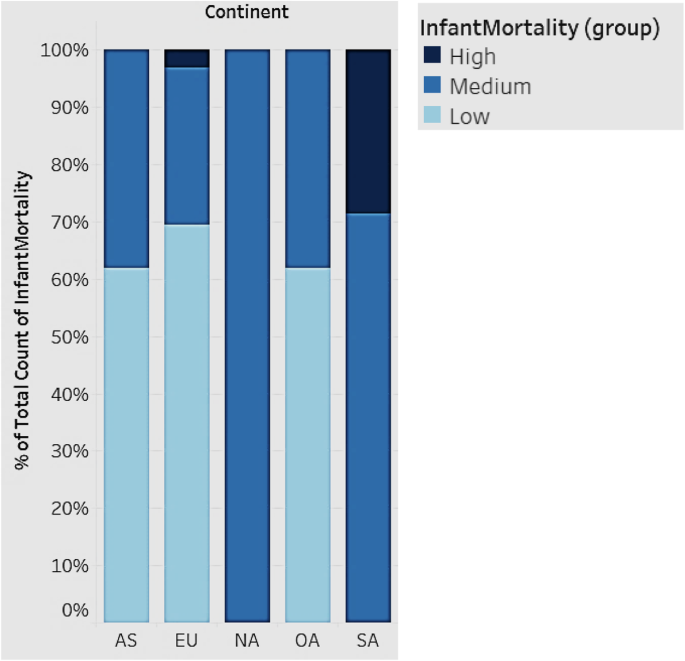
Distribution of Infant Mortality rates by Continent
In Fig. 12 , each bar represents a continent. All countries fall into three groups (high, medium, and low) based on infant mortality rates. South America has the highest infant mortality, followed by Asia, Europe, and Oceania. North America falls in the medium range of infant mortality. South American countries, in general, should strive to improve infant mortality. While Europe, in general, has the lowest infant mortality rates, there are some countries that have high rates as depicted.
Association between child vaccination rates and NEET rates
We looked at the association between child vaccination rates and NEET rates in various countries (Fig. 13 ). We expect countries that have high NEET rates to have low child vaccination rates.
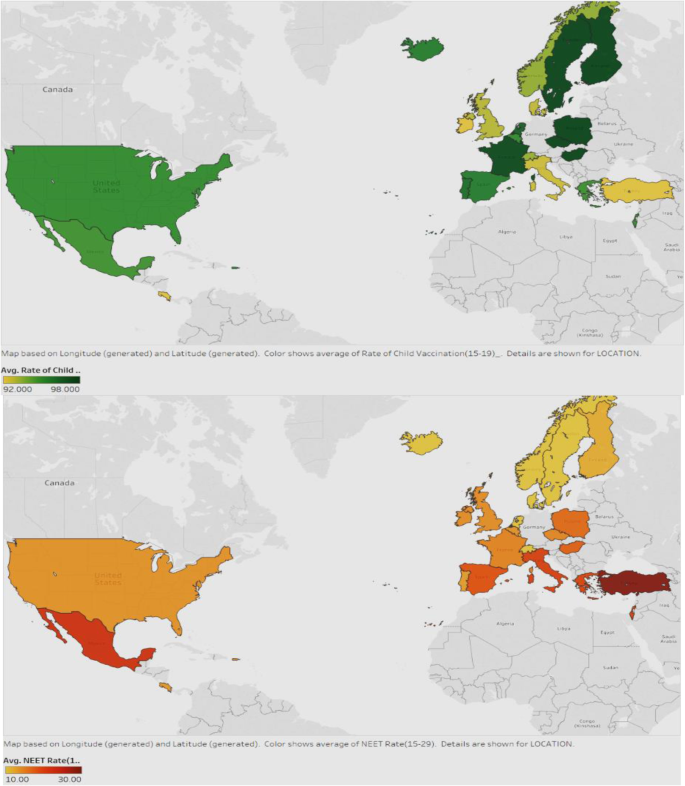
Association between Child Vaccination Rates and NEET rates
Figure 13 displays the child vaccination rates in the first map and the NEET rates in the second map, for all countries. The darker green color shows countries with higher rates of vaccination and the darker red represents those with higher NEET rates. It can be seen that in general, the countries with lower NEET also have better vaccination rates. Examples are USA, UK, Iceland, France, and North European countries. Countries should therefore strive to reduce NEET rates by enrolling a good proportion of the youth into initiatives or programs that will help them be more productive in the future, and be able to afford preventive healthcare for the families, particularly, the children.
Average smoking rate in different continents over time
We compared the trend of average smoking rate for the years 1995–201 for the continents in the sample (Fig. 14 ).
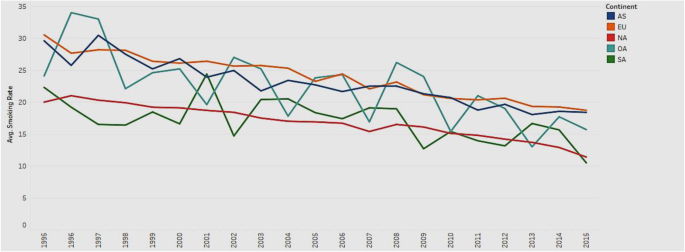
Trend of average smoking rate in different continents from 1995 to 2015
Figure 14 depicts the line charts of average smoking rates for the continents of Asia, Europe, Oceania, North and South America. All the lines show an overall downward trend, which indicates that the average smoking rate decreases with time. The trend illustrates that people have become more health conscious and realize the harmful effects of smoking over time. However, the smoking rate in Europe (EU) is consistently higher than that in other continents, while the smoking rate in North America (NA) is consistently lower over the years. Governments in Europe should pay attention to the usage of tobacco and increase health consciousness among the public.
Association between adult education levels and deaths from Cancer
We explored if adult education levels (below-secondary, upper-secondary, and tertiary) are associated with deaths from cancer (Fig. 15 ) such that higher levels of education will mitigate the rates of deaths from cancer, due to increased awareness and proactive health behavior.
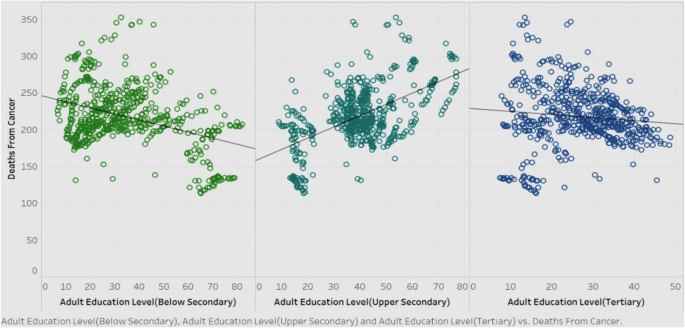
Association of deaths from cancer with adult education levels
Figure 15 shows the correlations of deaths from cancer among the three adult education levels, for all countries in the sample. It is obvious that below-secondary and tertiary adult education levels have a negative correlation with deaths from cancer, while the upper-secondary adult education level shows a positive correlation. Barring upper-secondary results, we can surmise that in general, as education level goes higher, the deaths from cancer will decrease. The rationale for this could be that education fosters more health awareness and encourages people to adopt healthy behavioral practices. Governments should therefore pay attention to frame policies that promote education. However, the counterintuitive result of the positive correlation between upper-secondary levels of adult education with the deaths from cancer warrants more investigation.
We drilled down further into the correlation between the upper-secondary education level and deaths from cancer. Figure 16 shows this correlation, along with a breakdown of the total number of records for each continent, to see if there is an explanation for the unique result.
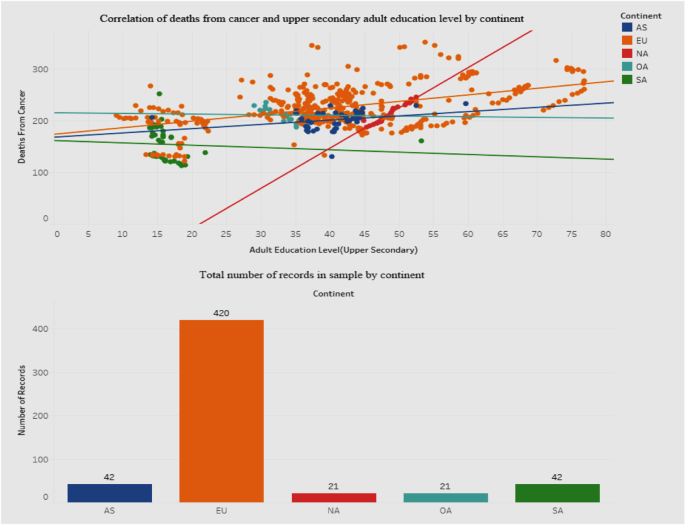
Association between deaths from cancer and adult education level-upper secondary
Figure 16 shows a dashboard containing two graphs - a scatterplot of the correlation between deaths from cancer and education level, and a bar graph showing the breakdown of the total sample by continent. We included a breakdown by continent in order to explore variances that may clarify or explain the positive association for deaths from cancer with the upper-secondary education level. The scatterplot shows that for the European Union (EU) the points are much more scattered than for the other continents. Also, the correlation between deaths and education level for the EU is positive. The bottom bar graph depicts how the sample contains a disproportionately high number of records for the EU than for other continents. It is possible that this may have influenced the results of the correlation. The governments in the EU should investigate the reasons behind this phenomenon. Also, we defer to future research to explore this in greater detail by incorporating other socioeconomic parameters that may have to be factored into the relationship.
Association between average tertiary school life expectancy and health expenditure
We moved our focus to the trends of tertiary school life expectancy and health expenditure from 1995 to 2015 (Fig. 17 ) to check for positive associations.
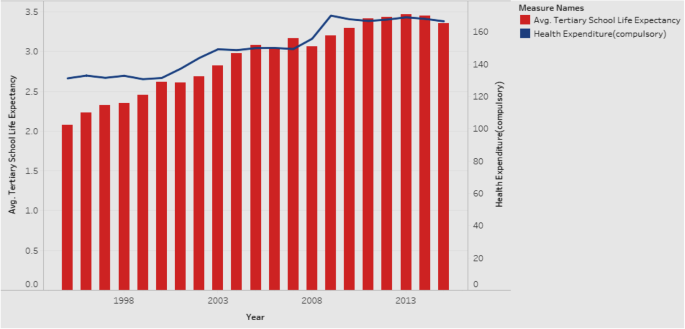
Association between Average Tertiary School Life Expectancy and Health Expenditure
Figure 17 is a combination chart explaining the trends of tertiary school life expectancy and health expenditure, for all countries in the sample. The rationale is that if there is a positive association between the two, it would be worthwhile for the government to allocate more resources towards health expenditure. Both tertiary school life expectancy and health expenditure show an increase over the years from 1995 to 2015. Our additional analysis shows that they continue to increase even after 2015. Hence, governments are encouraged to increase the health expenditure in order to see gains in tertiary school life expectancy, which will have positive implications for national health. Given that the measured effects of education are large, investments in education might prove to be a cost-effective means of achieving better health.
Our results reveal how interlinked education and health can be. We show how a country can improve its health scenario by focusing on appropriate indicators of education. Countries with higher education levels are more likely to have better national health conditions. Among the adult education levels, tertiary education is the most critical indicator influencing healthcare in terms of infant mortality, life expectancy, child vaccination rates, and enrollment rates. Our results emphasize the role that education plays in the potential years of life lost, which is a measure that represents the average years a person would have lived had he/she not died prematurely. In addition to mortality rate, an economy needs to consider this indicator as a measure of health quality.
Other educational indicators that are major drivers of health include school life expectancy, particularly at the tertiary level. In order to improve the school life expectancy of the population, governments should control the number of youths ending up unemployed, dropping out of school, and without skills or training (the NEET rate). Education allows people to gain skills/abilities and knowledge on general health, enhancing their awareness of healthy behaviors and preventive care. By targeting promotions and campaigns that emphasize the importance of skills and employment, governments can reduce the NEET rates. And, by reducing the NEET rates, governments have the potential to address a broad array of vulnerabilities among youth, ranging from unemployment, early school dropouts, and labor market discouragement, which are all social issues that warrant attention in a growing economy.
We also bring to light the health disparities across countries and suggest implications for governments to target educational interventions that can reduce inequalities and improve health, at a macro level. The health effects of education are at the grass roots level - creating better overall self-awareness on personal health and making healthcare more accessible.
Scope and limitations
Our research suffers from a few limitations. For one, the number of countries is limited, and being that the data are primarily drawn from OECD, they pertain to the continent of Europe. We also considered a limited set of variables. A more extensive study can encompass a larger range of variables drawn from heterogeneous sources. With the objective of acquiring a macro perspective on the education–health association, we incorporated some dependent variables that may not traditionally be viewed as pure health parameters. For example, the variable potential years of life lost is affected by premature deaths that may be caused by non-health related factors too. Also there may be some intervening variables in the education–health relationship that need to be considered. Lastly, while our study explores associations and relationships between variables, it does not investigate causality.
Conclusions and future research
Both education and health are at the center of individual and population health and well-being. Conceptualizations of both phenomena should go beyond the individual focus to incorporate and consider the social context and structure within which the education–health relationship is embedded. Such an approach calls for a combination of interdisciplinary research, novel conceptual models, and rich data sources. As health differences are widening across the world, there is need for new directions in research and policy on health returns on education and vice versa. In developing interventions and policies, governments would do well to keep in mind the dual role played by education—as a driver of opportunity as well as a reproducer of inequality [ 36 ]. Reducing these macro-level inequalities requires interventions directed at a macro level. Researchers and policy makers have mutual responsibilities in this endeavor, with researchers investigating and communicating the insights and recommendations to policy makers, and policy makers conveying the challenges and needs of health and educational practices to researchers. Researchers can leverage national differences in the political system to study the impact of various welfare systems on the education–health association. In terms of investment in education, we make a call for governments to focus on education in the early stages of life course so as to prevent the reproduction of social inequalities and change upcoming educational trajectories; we also urge governments to make efforts to mitigate the rising dropout rate in postsecondary enrollment that often leads to detrimental health (e.g., due to stress or rising student debt). There is a need to look into the circumstances that can modify the postsecondary experience of youth so as to improve their health.
Our study offers several prospects for future research. Future research can incorporate geographic and environmental variables—such as the quality of air level or latitude—for additional analysis. Also, we can incorporate data from other sources to include more countries and more variables, especially non-European ones, so as to increase the breadth of analysis. In terms of methodology, future studies can deploy meta-regression analysis to compare the relationships between health and some macro-level socioeconomic indicators [ 13 ]. Future research should also expand beyond the individual to the social context in which education and health are situated. Such an approach will help generate findings that will inform effective educational and health policies and interventions to reduce disparities.
Availability of data and materials
The dataset analyzed during the current study is available from the corresponding author on reasonable request.
Abbreviations
Fundamental Cause Theory
Human Capital Theory
Not in Employment, Education, or Training
Organization for Economic Cooperation and Development
Socio-economic status
Andersen RM, Newman JF. Societal and individual determinants of medical care utilization in the United States. Milbank Mem Fund Q Health Soc. 1973;51(1):95–124.
Article CAS Google Scholar
Andersen RM. Revisiting the behavioral model and access to medical care: does it matter? J Health Social Behav. 1995;36(1):1–10.
Baker DP. The Schooled Society: The Educational Transformation of Global Culture. Stanford, CA: Stanford Univ. Press: 2014; 360.
Becker GS. Human capital: a theoretical and empirical analysis, with special reference to education. Chicago: Univ. Chicago Press; 1964.
Google Scholar
Berkman LF, Syme SL. Social networks, host resistance, and mortality: a nine-year follow-up study of Alameda County residents. Oxford Acad J. 1979;109(2):186–204.
CAS Google Scholar
Crimmins EM, Kim JK, Vasunilashorn S. Biodemography: new approaches to understanding trends and differences in population health and mortality. Demography. 2010;47:S41–64.
Article Google Scholar
Spence M. Job market signalling. The Quarterly J Econ. 1973;87:355–79.
Cutler DM, Lleras-Muney A. Education and Health: Evaluating Theories and Evidence: NBER Working Papers; 2006. p. 12352.
Feinstein L. What are the effects of education on health? OECD Proceedings of the Copenhagen Symposium; 2006 . .
Folkman S, Lazarus RS. An analysis of coping in a middle-aged community sample. J Health Soc Behav. 1980;21(3):219–39.
Freedman VA, Martin LG. The role of education in explaining and forecasting trends in functional limitations among older Americans. Demography. 1999;36(4):461–73.
Freese J, Lutfey K. Fundamental causality: challenges of an animating concept for medical sociology. In: Pescosolido BA, Martin JK, McLeod JD, Rogers A, editors. Handbook of the Sociology of Health, Illness, and Healing: a blueprint for the 21st century. New York: Springer; 2011. p. 67–81.
Chapter Google Scholar
Fouweather T, Gillies C, Wohland P, Van Oyen H, Nusselde W, Robine J, Cambois E, Jagger C. Comparison of socio-economic indicators explaining inequalities in healthy life years at age 50 in Europe: 2005 and 2010. Eur J Pub Health. 2015;25:978–83.
George LK, Gwyther LP. Caregiver Weil-being: a multidimensional examination of family caregivers of demented adults. Gerontologist. 1986;26(3):253–9.
Goldman D, Smith JP. The increasing value of education to health. Soc Sc Med. 2011;72(10):1728–37.
Harper AC, Lambert LJ. The health of populations: an introduction. New York: Springer Publishing Company; 1994.
Health 2020: Education and health through the life-course. WHO Europe Sector Brief on Education Health; 2015. http://www.euro.who.int/__data/assets/pdf_file/0007/324619/Health-2020-Education-and-health-through-the-life-course-en.pdf?ua=1 .
Kino S, Bernabé E, Sabbah W. The role of healthcare and education systems in co-occurrence of health risk behaviours in 27 European countries. Eur J Public Health. 2018;28(1):186–92.
Landerman LR, Burns BJ, Swartz MS, Wagner HR, George LK. The relationship between insurance coverage and psychiatric disorder in predicting use of mental health services. Am J Psychol. 1994;151(12):1785.
Lynch SM. Cohort and life-course patterns in the relationship between education and health: a hierarchical approach. Demography. 2003;40(2):309–31.
Mackenbach J, Kunst A. Measuring the magnitude of socio-economic inequalities in health: an overview of available measures illustrated with two examples from Europe. Soc Sci Med. 1997;44(6):757–71. https://doi.org/10.1016/S0277-9536(96)00073-1 .
Article CAS PubMed Google Scholar
Manton KG, Corder L, Stallard E. Chronic disability trends in elderly United States populations: 1982-1994. Natl Acad Sci. 1997;94(6):2593–8.
Mirowski J, Ross CE. Education, learned effectiveness and health. London Rev Edu. 2005;3(3):205–20.
Montez JK, Berkman LF. Trends in the educational gradient of mortality among US adults aged 45 to 84 years: bringing regional context into the explanation. Am J Pub Health. 2014;104(1):e82–90.
Montez JK, Zajacova A. Trends in mortality risk by education level and cause of death among US white women from 1986 to 2006. Am J Pub Health. 2013;103:473–9.
Olshansky SJ, Antonucci T, Berkman L, Binstock RH, Boersch-Supan A, Cacioppo JT, Carnes BA, Carstensen LL, Fried LP, Goldman DP, Jackson J, Kohli M, Rother J, Zheng Y, Rowe J. Differences in life expectancy due to race and educational differences are widening, and many may not catch up. Health Aff. 2012;31(8):1803–13.
Pamuk ER. Social-class inequality in infant mortality in England and Wales from 1921 to 1980. Eur J Popul 1988; 4 , 1–21, https://doi.org/10.1007/BF01797104.
Phelan JC , Link BG , Tehranifar P . Social conditions as fundamental causes of health inequalities: Theory, evidence, and policy implications . J Health Soc Behav 2010 ; 51 : S28 S40 . doi : 10.1177/0022146510383498.
Renard F, Devleesschauwer B, Speybroeck N, Deboosere P. Monitoring health inequalities when the socio-economic composition changes: are the slope and relative indices of inequality appropriate? Results of a simulation study. BMC Public Health 2019; 19: 662. https://doi.org/10.1186/s12889-019-6980-1.
Ro A, Geronimus A, Bound J, Griffith D, Gee G. Educational gradients in five Asian immigrant populations: do country of origin, duration and generational status moderate the education-health relationship? Prev Med Rep. 2016;4:338–43.
Ross CE, Wu CL. The links between education and health. Am Soc Rev. 1995;60(5):719–45.
Shiels MS, Chernyavskiy P, Anderson WF, Best AF, Haozous EA. Diverging trends in premature mortality in the U.S. by sex, race, and ethnicity in the 21st century. Lancet. 2017;389:1043–54.
Tsou MT. Association of Education, health behaviors, concerns, and knowledge with metabolic syndrome among urban elderly in one medical Center in Taiwan. Int J Gerontology. 2017;11(3:138–43.
Wheaton B. Stress, personal coping resources, and psychiatric symptoms: an investigation of interactive models. J Health Soc Behav. 1983;24(3):208–29.
Williams DR, Collins C. US socioeconomic and racial differences in health: patterns and explanations. Ann Rev Soc. 1995;21:349–86.
Zajacova A, Lawrence EM. The relationship between education and health: reducing disparities through a contextual approach. Ann Rev Pub Health. 2018;39:273–89.
Zimmerman EB, Woolf SH. Understanding the relationship between education and health. Discussion Paper, Inst Med. Washington DC; 2014. https://nam.edu/wp-content/uploads/2015/06/BPH-UnderstandingTheRelationship1.pdf .
Zhong H. An over time analysis on the mechanisms behind the education–health gradients in China. China Econ Rev. 2015;34(C):135–49.
Download references
Acknowledgements
Not applicable.
Author information
Authors and affiliations.
Koppelman School of Business, Brooklyn College of the City University of New York, 2900 Bedford Ave, Brooklyn, NY, 11210, USA
Viju Raghupathi
Gabelli School of Business, Fordham University, 140 W. 62nd Street, New York, NY, 10023, USA
Wullianallur Raghupathi
You can also search for this author in PubMed Google Scholar
Contributions
Both authors contributed equally to all parts of the manuscript.
Corresponding author
Correspondence to Viju Raghupathi .
Ethics declarations
Ethics approval and consent to participate, consent for publication, competing interests.
The authors declare that they have no competing interests.
Additional information
Publisher’s note.
Springer Nature remains neutral with regard to jurisdictional claims in published maps and institutional affiliations.
Rights and permissions
Open Access This article is licensed under a Creative Commons Attribution 4.0 International License, which permits use, sharing, adaptation, distribution and reproduction in any medium or format, as long as you give appropriate credit to the original author(s) and the source, provide a link to the Creative Commons licence, and indicate if changes were made. The images or other third party material in this article are included in the article's Creative Commons licence, unless indicated otherwise in a credit line to the material. If material is not included in the article's Creative Commons licence and your intended use is not permitted by statutory regulation or exceeds the permitted use, you will need to obtain permission directly from the copyright holder. To view a copy of this licence, visit http://creativecommons.org/licenses/by/4.0/ . The Creative Commons Public Domain Dedication waiver ( http://creativecommons.org/publicdomain/zero/1.0/ ) applies to the data made available in this article, unless otherwise stated in a credit line to the data.
Reprints and permissions
About this article
Cite this article.
Raghupathi, V., Raghupathi, W. The influence of education on health: an empirical assessment of OECD countries for the period 1995–2015. Arch Public Health 78 , 20 (2020). https://doi.org/10.1186/s13690-020-00402-5
Download citation
Received : 16 October 2019
Accepted : 26 February 2020
Published : 06 April 2020
DOI : https://doi.org/10.1186/s13690-020-00402-5
Share this article
Anyone you share the following link with will be able to read this content:
Sorry, a shareable link is not currently available for this article.
Provided by the Springer Nature SharedIt content-sharing initiative
- Education level
- Enrollment rate
- Life expectancy
- Potential years of life lost
- Infant mortality
- Deaths from cancer
Archives of Public Health
ISSN: 2049-3258
- General enquiries: [email protected]
Journal of Population Sciences
- Original Article
- Open access
- Published: 07 March 2019
The impact of increasing education levels on rising life expectancy: a decomposition analysis for Italy, Denmark, and the USA
- Marc Luy 1 , 2 ,
- Marina Zannella 1 , 2 , 7 ,
- Christian Wegner-Siegmundt 1 , 2 ,
- Yuka Minagawa 5 ,
- Wolfgang Lutz 1 , 2 , 3 , 4 &
- Graziella Caselli 6
Genus volume 75 , Article number: 11 ( 2019 ) Cite this article
39k Accesses
43 Citations
138 Altmetric
Metrics details
Significant reductions in mortality are reflected in strong increases in life expectancy particularly in industrialized countries. Previous analyses relate these improvements primarily to medical innovations and advances in health-related behaviors. Mostly ignored, however, is the question to what extent the gains in life expectancy are related to structural changes in the populations due to increasing education levels. We decompose changes of the total populations’ life expectancy at age 30 in Italy, Denmark, and the USA, over the 20-year period between 1990 and 2010 into the effects of education-specific mortality changes (“M effect”) and changes in the populations’ educational structure (“P effect”). We use the “replacement decomposition technique” to further subdivide the M effect into the contributions by the individual education groups. While most of the increases in life expectancy are due to the effect of changing mortality, a large proportion of improvements in longevity can indeed be attributed to the changing structure of the population by level of education in all three countries. The estimated contribution of the P effect ranges from around 15% for men in the USA to approximately 40% for women in Denmark. This study demonstrates strong associations between education and overall population health, suggesting that education policies can also be seen as indirect health policies.
Introduction
Reductions in mortality which started in Europe in the middle of the eighteenth century have continued to spread globally and led to huge increases in life expectancy, with industrialized countries being most advanced in this process. Most of these changes were due to a shift in cause-specific mortality patterns from communicable diseases at younger ages, including neo-natal and childhood ages, to non-communicable conditions more prevalent at advanced ages, as described in Omran’s ( 1971 ) “epidemiologic transition theory.” Recently, the potentials of human longevity were further extended by the “cardiovascular revolution” which started in the 1970s and initiated a new period of decreasing mortality (Caselli 2015 ; Olshansky and Ault 1986 ; Omran 1983 ; Rogers and Hackenberg 1987 ). Studies have tended to relate these improvements to public health measures and new medical advancements, such as developments in screening, prevention, and treatment of cardiovascular diseases (Mackenbach et al. 1988 ; Vallin and Meslé 2004 ; Wilmoth 2000 ). Improvements in living conditions and health-related behaviors have also reduced mortality, thereby leading to further increase in survival chances (Denney et al. 2010 ; Janssen and Kunst 2005 ; Rogers et al. 1999 , 2010 ).
In tandem with the decrease of mortality, the populations have experienced significant increases in educational attainment (Lutz et al. 2014a ). Between 1970 and 2009, for instance, the average number of years of education rose by 3.6 years for people aged 25 and older in 175 countries (Gakidou et al. 2010 ). A great deal of research acknowledges the strong impact of socioeconomic resources on various health outcomes, suggesting socioeconomic conditions as a “fundamental cause” of inequalities in health and mortality (Link and Phelan 1995 ). Many studies have shown that highly educated people stand to benefit first and most from the prevailing drivers of decreasing mortality, particularly with regard to the risk factors of cardiovascular diseases (Hummer and Lariscy 2011 ; Mirowsky and Ross 2003 ). These inequalities translate into differences in life expectancy of several years between individuals with low and high educational attainment (Caselli et al. 2014 ). Lleras-Muney ( 2005 ) estimated—with data for the USA in 1960—that one more year of education increased average life expectancy at age 35 by as much as 1.7 years. Further, Woolf et al. ( 2007 ) estimated that eliminating education-specific inequalities in mortality would save even more lives than medical advances.
Naturally, higher education does not automatically lead to better health. It is also unclear to what extent education itself plays a direct role inside the complex network of various health-related socioeconomic factors (Kaplan et al. 2014 ). Although evidence infers that it is justified to assume some direct functional causality (Geyer and Peter 2000 ; Lleras-Muney 2005 ; Lutz and Skirbekk 2014 ; van Kippersluis et al. 2011 ), it appears that the association between education and mortality operates to a larger extent along an indirect pathway in which education acts as access criterion to other drivers of health and longevity (Arendt 2005 ; Clark and Royer 2013 ; Davey Smith et al. 1998 ; Kilpi et al. 2016 ; Kröger et al. 2015 ; Lager and Torssander 2012 ). As Hayward et al. ( 2015 : 16) put it, “…there is no inherent causal association between educational attainment and adult mortality; instead, the causal association is dependent upon time, place, and social environment under study.”
Here, we briefly summarize the major mechanisms linking education to health and mortality (an extensive compilation of recent studies can be found in Montez and Friedman ( 2015 )). Schooling develops basic cognitive functioning, such as reading, writing, and communicating, and teaches individuals how to think logically, critically analyze data, solve problems, and implement plans (Kingston et al. 2003 ). Higher education is the key to stable and well-paid jobs, and increased income helps to pay for nutritious food, better-quality housing, and high-quality medical care (Mirowsky and Ross 2003 ). In addition, education promotes healthy lifestyles through the development of effective human agency. Highly educated people use their knowledge, information, and past experiences to avoid health-related risk factors and engage in health-enhancing behaviors, such as smoking cessation, alcohol abstinence, and frequent physical exercise (Denney et al. 2010 ; Laaksonen et al. 2008 ). Moreover, education provides socio-psychological resources that can contribute to health and longevity through emotional and instrumental support (House et al. 1988 ). That well-educated people are more likely to be and remain married also contributes to the relationship between education and health (Qian and Preston 1993 ).
These examples indicate how higher education contributes to better health and longer lives on the individual level. Furthermore, investments in education also improve the health status on the population level. Baker et al.’s ( 2011 ) systematic review demonstrates the strong relationship between education and adult mortality across countries at different levels of development. These insights raise the question to what extent the increasing education levels constitute an additional factor behind populations’ rising life expectancy. If educational attainment improves in a population, it usually follows that the proportion of individuals with lower education levels decreases, while the proportion of those with higher education increases. Consequently, the health of the overall population should increase accordingly, even if the mortality rates of each education category remain unchanged. The question to what extent the gains in life expectancy were caused by such changes in the populations’ education structure, in addition to the effect of decreasing mortality within the education groups, has been addressed only rarely so far. Shkolnikov et al. ( 2006 ) and Jasilionis et al. ( 2007 ) provided such estimates for some northern, central, and eastern European countries between the late 1980s and 1990s. They found that the changes in the education-specific composition had in fact a remarkable positive effect on the populations’ life expectancy levels. Similar conclusions were derived in studies on specific national subpopulations (De Grande et al. 2014 ; Klein et al. 2006 ; Turra et al. 2016 ).
Guided by the works of Shkolnikov et al. ( 2006 ) and Jasilionis et al. ( 2007 ), we examine, on the basis of the same methodology, how changes in the educational attainment by age and sex contributed to increasing overall life expectancy at age 30 ( e 30 ) in Italy, Denmark, and the USA between around 1990 and 2010. Thus, our study extends the literature on the relationship between populations’ levels of education and life expectancy by adding insights from new populations and by analyzing a longer and more recent observation period. Also, the basic research question differs from the previous studies. Shkolnikov et al. ( 2006 ) and Jasilionis et al. ( 2007 ) examined primarily whether the stalls and decreases of life expectancy in central and eastern Europe during the 1980s were driven by certain education-specific subpopulations. The present study focuses, by contrast, on western populations and the specific question to what extent changes in the educational structure of the populations contributed to their steady increases in life expectancy.
The populations of Italy, Denmark, and the USA form an interesting sample to assess the relationship between populations’ levels of education and life expectancy because they differ in terms of life expectancy, magnitudes of education-specific differentials in mortality, and the education profile of the populations. In the USA, formal schooling lasts 13 years until around age 18 with some variations between the states. Notably, the US population experienced substantial changes over the years in terms of the levels, content, and importance of education (Hummer and Lariscy 2011 ). The proportion of the population at age 25 and older with at least high school education more than doubled since the early 1960s to almost 90% in 2015 (Ryan and Bauman 2016 ). In the two European states, compulsory education is shorter than in the USA (OECD 2016 ). Also, the process of education expansion started later. The proportion of adults with tertiary education is therefore still distinctly lower, above all in Italy (OECD 2016 ). More effective population policies in the past let Denmark move ahead in the process of education expansion (Arendt 2005 ), giving the country the intermediate position among the three study populations. All these characteristics of the national education systems refer exclusively to quantitative aspects. Certainly, education systems differ also in qualitative aspects. These are, however, not the topic of this study. We will come back to this issue briefly in the “ Discussion ” section of the paper.
In the following two sections, we describe the data and methods we used to construct the life tables by education and to decompose the changes in e 30 over the observed 20-year period into the effects of changing education-specific mortality (“M effect”) and changing educational structure of the populations (“P effect”). In the “ Results ” section, we summarize the outcomes of our analyses which confirm that increasing education levels are strongly related to the rising levels of life expectancy. This finding has policy implications, particularly for newly industrializing countries and other populations of the global south where education levels are projected to increase even more rapidly than in the industrialized world (Lutz et al. 2014a ). Because further rises of average education levels are bound to occur by the fact that in most countries the younger cohorts are better educated than the older ones, our results also fuel the expectation of continuing increases in life expectancy—an issue that is highly controversial among demographers and split the community into “optimists” and “pessimists”. All these issues and the limitations of the study are discussed in the final section of the paper.
The analysis requires age- and education-specific data for the populations at risk and the deaths for the start and end years of our observation period. Our study starts at age 30 because this is the age when education is usually completed. We defined educational attainment on the basis of the ISCED-97 scale (UNESCO 1996 ) with the three levels low (ISCED 0–2), medium (ISCED 3–4), and high education (ISCED 5–6). Obtaining this data for our study populations was a challenging task, in particular for the early 1990s. Because the aim of this study is to estimate the impact of changes in populations’ educational structures on overall life expectancy, we gave priority to the most similar derivation of life tables for the studied populations rather than the most accurate estimate of life expectancy by education for each population. A cross-country comparison of differentials in life expectancy by education level would be a different research question which is not only affected by the characteristics of the data sources. The same formal education levels can include significant differences in the quality of education. Finally, the number of considered education levels plays a role with regard to both, the extent of differentials between the levels and the estimation of the effects of corresponding structural changes. The following paragraphs summarize the data sources and how we constructed our database as homogeneous as possible.
Information about the population by age, sex, and education level was taken from Italian census data which is available online at the data warehouse of the National Statistical Institute of Italy (ISTAT). Footnote 1 Age-, sex-, and education-specific numbers of death stem from the Italian national vital statistics registry on causes of death and are based on death certificates. Data for 2011 were downloaded from the ISTAT data warehouse. Data for 1991 are not available online and were manually collected and digitalized by the authors from the printed reports “Nascite e decessi” at the ISTAT archive in Rome, Italy. In all data sources, education refers to the highest educational attainment which allowed us to translate the numbers directly into the ISCED-97 classification. The data for the population at risk and the number of deaths cover the ages 30 to 85+ in 5-year age groups. Cases with unknown education level (4.3% of deaths in 1991) have been distributed according to the education-specific proportions for valid cases for each age and sex group. Because of the introduction of new data-check plans in 2004, there were no cases with missing information in the data for 2011.
Information about the population by age, sex, and education level comes from nationwide population registers, covering the ages 30 to 100. Information about age-, sex-, and education-specific mortality was available in form of probabilities of dying for ages 30 to 64. These were derived by register linkage at the individual level by use of the unique personal identification number assigned to all Danish citizens (Thygesen et al. 2011 ). For ages 65 and older, age- and sex-specific probabilities of dying were only available for the total population without separation by education level. All data were available for single ages and represent averages of two subsequent calendar years (i.e., the data for 1990 refers to the average of 1989 and 1990, and the data for 2010 to the average of 2009 and 2010). The databases did not include any cases with missing information. Education level was defined according to ISCED-97 and categorized into the levels 1–2, 3A, 3C & 4A, and 5–6. We merged the data for ISCED levels 3A, 3C, and 4A (ISCED 3–4) and derived the age-, sex-, and education-specific numbers of death from the data for the population and the corresponding probabilities of dying. Education-specific probabilities of dying above age 64 were derived by extrapolating the available data for ages 30 to 64 with the Brass logit life table model (Brass 1971 ). We used the age-specific mortality schedules of the total Danish population by sex (for 1989 respective to 2009) from the Human Mortality Database ( 2017 ) as standard life tables. Footnote 2 The Brass parameters were estimated from the age- and education-specific probabilities of dying at ages 30 to 64 (see the “ Life tables by education level ” section for more details on our estimations with the Brass model).
Information about the population by age, sex, and education level stems from the data collection IPUMS-USA (Ruggles et al. 2015 ). Footnote 3 Age-, sex-, and education-specific numbers of death stem from the Division of Vital Statistics of the National Center for Health Statistics and are based on death certificates. The data were downloaded from the website of the Centers for Disease Control and Prevention (CDC). Footnote 4 Both datasets are available in micro-data form, and we merged them into 5-year age groups from age 30 to 90+. In the data for the population at risk, education refers to the highest educational attainment, which enabled us to translate the numbers directly into the ISCED-97 classification. In the mortality data for 1990, education is classified by the number of actual years of schooling. In the mortality data for 2010, education refers to the highest educational attainment as defined in the Revision of the Standard Certificate of Death of the year 2003. Footnote 5 Given that not all states have adopted the new classification, the mortality data for 2010 includes both classifications: number of years in school and the highest educational attainment. For the states which used the new regulation, we were able to translate the numbers directly into the ISCED-97 classification. To obtain corresponding education classifications for the deaths of 1990 and for those of 2010 which were still classified by the number of school years, we performed an approximate reclassification into the ISCED-97 system on the basis of the EDATTAIN International Recode of Educational Attainment. Footnote 6 Cases with unknown education levels (23.6% of deaths in 1990 and 2.2% of deaths in 2010) were distributed according to the education-specific proportions for valid cases for each age and sex group.
As described in the previous section, the characteristics of the underlying data differ between our study populations, e.g., with regard to the construction of the three ISCED education groups. Another important difference concerns the data sources for the education-specific populations at risk and the corresponding number of deaths. Whereas for Denmark both stem from linked population registers, we have different and unlinked data sources for Italy and the USA. Consequently, the estimated education-specific differentials in life expectancy cannot be directly compared between the populations. However, as outlined at the beginning of the “ Data ” section and later in more detail in the “ Discussion ” section of this paper, the purpose of this study lies exclusively in the estimation of the relationship between increasing education levels and overall life expectancy. In order to compare results across our study populations, we constructed life tables as basis for the decomposition analysis. Life tables were constructed as consistently as possible in regards to the available data for each study population.
Life tables by education level
We constructed abridged period life tables from age 30 for 5-year age groups for the populations of Italy (1991 and 2011), Denmark (1990 and 2010), and the USA (1990 and 2010) on the basis of age-, sex-, and education-specific death rates derived from the data described in the “ Data ” section. The age-specific fractions of last age interval of life were estimated with the Keyfitz method (Preston et al. 2001 ). In order to get a homogeneous basis for our analysis, we extrapolated all life tables with the Brass logit model (Brass 1971 ) to age 110. The Brass parameters were estimated from the age- and education-specific probabilities of dying at ages 30 to 64 (because of the restriction set by the data for Denmark, see the “ Denmark ” section). Country- and period-specific life tables from the Human Mortality Database ( 2017 ) were used as standard. Footnote 7 The extrapolated life tables for each sex- and education-specific subpopulation were then used to derive the death rates for the country-specific last open age intervals. These start at different ages as determined by the country-specific availability of data for the living population (see the “ Data ” section). The basic data on deaths and populations at risk by education levels and the life tables by educational attainment for each country and observation period are available in the Additional file 1 .
Decomposition of changes in life expectancy
The central aim of this study is to decompose the changes in life expectancy at age 30 ( e 30 ) for the total populations of Italy, Denmark, and the USA between around 1990 (time t 1) and around 2010 (time t 2) into the contributions of changes in education-specific mortality (M effect) and changes in the populations’ educational structure (P effect). The terms are adopted from Shkolnikov et al. ( 2006 ) who introduced this specific decomposition approach. Isolating the M and P effects is difficult because they are complexly interrelated. On the one hand, changes in education-specific mortality cause changes in the education-specific structure of the population through increasing or decreasing survival chances. On the other hand, changes in the educational structure of the population affect the corresponding mortality levels because the risk compositions of the education-specific subpopulations are changing, e.g., when a larger proportion of formerly less-educated individuals with higher mortality risks move to higher education levels or vice versa. Between t 1 and t 2, both changes occur simultaneously with different and varying magnitudes what makes the interrelation even more complex.
Although several approaches have been proposed to decompose the change in the death rates, they all follow the same basic idea of standardization, that is to isolate the effect of changing mortality under constant population composition, or vice versa, the effect of changing composition of the population under constant mortality. The challenging issue is to decide the condition for which mortality and population compositions are standardized for. Three different standards are possible: (1) the conditions prevailing at t 1, (2) the conditions prevailing at t 2, and (3) the average of conditions prevailing at t 1 and t 2. We used the replacement decomposition technique (Andreev et al. 2002 ; Shkolnikov et al. 2003 ) which estimates the M and P effects on the basis of standard (3). The basic approach of this method is to apply a “[…] stepwise replacement of elements from one vector of age-specific mortality rates by respective elements of another vector” (Andreev et al. 2002 : 500).
In a nutshell, the replacement decomposition technique works as follows. Starting with the original vector of education-specific death rates at t 1, we estimate e 30 . In the first replacement step, the education-specific death rates of the age group 30–34 of t 1 are substituted by the corresponding death rates of t 2, while the educational structure of the population is kept constant at the level of t 1. The difference between the resulting modeled values for e 30 at t 2 and those of the start year t 1 provides an estimate for the M effect of the age group 30–34 on the basis of standard (1). In the second step, the P effect originating from education-specific structural changes in this age group is estimated accordingly by substituting the population’s educational structure in age group 30–34 of t 1 by those of t 2, while the education-specific death rates are kept constant at the level of t 1. These steps are repeated for all possible combinations of age- and education-specific death rates and the population’s educational structure until the conditions prevailing at t 1 are completely replaced by the corresponding conditions at t 2. Then, the procedure is performed equivalently on the basis of standardization procedure (2) for the transition from t 2 to t 1 through substituting the education- and age-specific death rates and the educational structure at t 2 stepwise by those prevalent at t 1.
The different directions across the replacement paths, the subpopulations, and the age schedule cause different combinations of vectors which should be fully considered in an ideal decomposition procedure. To avoid the corresponding bulk of calculations, the usually applied strategy is to perform the replacement procedure only from the youngest to the oldest age group. The resulting set of age-specific M and P effects is then summed up to the overall effects and averaged for the results obtained by standards (1) and (2). Consequently, the yielded estimates can be regarded as approximate overall M and P effects, i.e., estimates for the contributions of changes in education-specific mortality (respective contributions of changes in the educational structure of the population) to changing death rates between t 1 and t 2, when the population’s educational structure (respective mortality conditions) remain constant. The particular advantage of the replacement decomposition technique is that the replacement can be performed separately for each education subgroup. This allows a subdivision of the M effect into the individual contributions of education group-specific changes in mortality (more and specific details about the method can be found in Shkolnikov et al. 2006 ). For our analyses, we used the MS Excel macro provided by Andreev and Shkolnikov ( 2012 ). Footnote 8
Table 1 presents the distribution of and changes in educational attainment at ages 30 and above for Italy, Denmark, and the USA during the study period. Substantial changes have been made in educational attainment levels in these countries between 1990 and 2010. The proportion of the population with low education strongly declined, and consequently, there were large increases of the proportions in the medium and high education categories. Italy had massive shifts particularly between the low and medium levels, while the proportion of highly educated people increased somewhat less. Denmark experienced similar decreases at the low education level but with more distinct differences between women and men. In contrast to Italy, the high education category increased more than the medium category in both sexes. Partly due to starting from a higher level, the USA saw considerably smaller increases in the education levels over the same period. Nonetheless, the share of those with low educational attainment also declined during the study period, while the proportion of medium education level changed only slightly. Hence, the shift in education level occurred primarily at the extreme ends of low and high education levels. Despite these increases in education levels in all three countries, large proportions of the populations still remained in the low education groups, mostly concentrated in the older ages. In the most recent observation years, 55.4% of the Italian population had less than upper secondary education, and the respective figures are 32.3% in Denmark and 12.8% in the USA.
Table 2 summarizes how life expectancy at age 30 ( e 30 ) increased during the 20 years of observation and how it varied by education group in the three countries. For the total populations, e 30 increased by 4.4 years in Italy, 3.6 years in Denmark, and 2.8 years in the USA. In all populations, there was a clear gradient in e 30 by level of education. Those with high education had the highest life expectancy, followed by medium and low education groups (except for Italian women in 1991 and the total Italian population in 2011). Two points are worth emphasizing. First, the pattern of impact of education on life expectancy was different among countries. In Italy, the largest gap in e 30 existed between the low education level and the two others, suggesting that completion of upper secondary education was a mortality threshold in the Italian population during the 1990s and 2000s. By contrast, tertiary education seems to have been the key to life expectancy improvements in the USA: differences between medium and high education were larger than those between medium and low education. Denmark shows the smallest inequalities across education groups, albeit with more notable sex differences in the highest education category. Second, educational differences in e 30 have widened over time in most cases. The differentials between high and low education groups were consistently larger in more recent years for both sexes in all three countries. Increases can be observed between medium and low education as well, though the extent of changes compared to those between low and high education differed between populations and sexes. The only exceptions are the decreasing differences between high and medium education among men and the total population in Italy, and among women in Denmark.
Results from the decomposition analyses are summarized in Table 3 and in Figs. 1 and 2 . It becomes apparent that improvements in e 30 were driven primarily by the M effect caused by decreasing education-specific death rates. The M effect was responsible for 80.9% of the total gain in e 30 in the USA, for 80.1% in Italy, and for 75.6% in Denmark. As expected, the pattern of the M effect broken down into specific education categories was not uniform across countries. In Italy, most of the M effect was caused by the low education group, resulting in a 61.5% increase in e 30 of the total population between 1991 and 2011 (57.9% for men; 67.7% for women). In the USA, the mortality reductions in the medium education category made the largest contribution to the increase in e 30 (51.5% for the total population; 48.4% for men; 62.5% for women). The low education group contributed least to increasing life expectancy in the USA. Among women, the contribution was even negative due to the decrease of e 30 between 1990 and 2010 (see Table 2 ). In Denmark, no specific education group dominated the mortality decline. The highest contribution originated from the low education level (33.4% for the total population; 34.6% for men; 26.4% for women), but the contribution of the medium education level was not much smaller (27.1% for the total population; 28.3% for men; 23.1% for women). The contribution of mortality declines in the high education level ranged from 3.8% among Italian women to 28.1% in the total population of the USA.
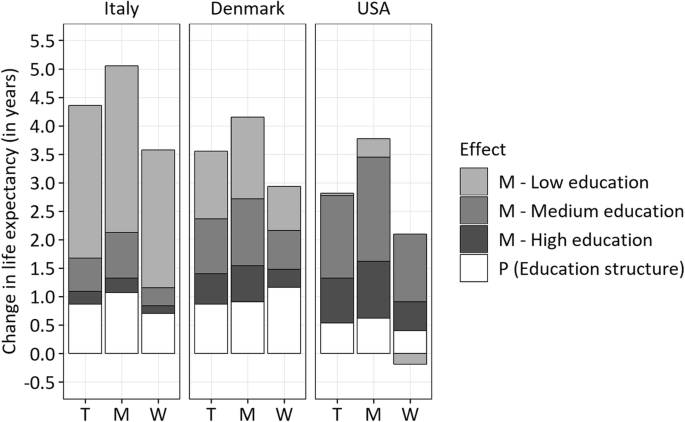
Absolute contributions of changes in mortality (M effect) and populations’ educational structure (P effect) to differences in life expectancy at age 30 (in years) for Italy (1991–2011), Denmark (1990–2010), and the USA (1990–2010), stratified by gender. Source: authors’ own calculations; the data sources for the three populations can be found in the “ Data ” section. Note: T refers to total population, M is men, and W is women
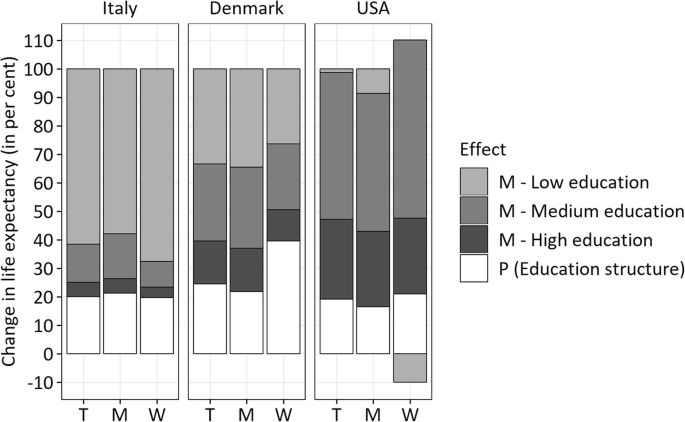
Relative contributions of changes in mortality (M effect) and populations’ educational structure (P effect) to differences in life expectancy at age 30 (in percent) for Italy (1991–2011), Denmark (1990–2010), and the USA (1990–2010), stratified by gender. Source: authors’ own calculations; the data sources for the three populations can be found in the “ Data ” section. Note: T refers to total population, M is men, and W is women
While the most powerful contributor to increasing life expectancy was the effect of decreasing mortality within education groups, the changing educational structure of the populations also contributed substantially to the increase in e 30 in all three countries (see Fig. 1 ). In Italy and Denmark, the structural change in the populations accounted for approximately 1 year of the increases in e 30 between around 1990 and 2010, and in the USA for about half a year. The smaller absolute increase attributable to the P effect in the USA can be primarily explained by the smaller overall increase in life expectancy (see Table 2 ). When looking at the relative contributions, the effect of changes in the educational structure on changes in e 30 was of similar magnitude in all three populations: 19.1% in the USA, 19.9% in Italy, and 24.4% in Denmark.
As shown in Table 3 and Fig. 2 , the relative effects are also similar across the male and female populations in all three countries. The only outlier is Danish women where the effect of changing educational composition on increasing life expectancy was particularly high, accounting for almost 40% of the change in e 30 between 1990 and 2010. This reflects the fact that of all analyzed populations, Danish women experienced the greatest shifts in educational structure during our study period, with the largest decrease in the proportion of less educated and the largest increase in the proportion of highly educated individuals (see Table 1 ). Overall, these results illustrate the substantial contributions of progress in educational attainment to the improvements in life expectancy between 1990 and 2010 in all three countries, in addition to all the other forces leading to decreasing overall mortality.
Methodological and conceptual issues
Several technical notes are in order. We performed a series of sensitivity analyses to test whether, and to what extent, our results are affected by the definition used for education levels, the restriction of the decomposition to changes between a start and an end year of a defined observation period, the chosen observation period itself, and the decomposition technique applied. With regard to the latter issue, we tested an alternative approach developed by Das Gupta ( 1993 ) which combines the classic age decomposition of a change in life expectancy (Andreev 1982 ; Pressat 1985 ) with the general method for decomposing differences between rates (Das Gupta 1978 ). We compared the results of this method with those derived with the replacement decomposition technique on the basis of the correlation coefficients for the estimated age-specific M and P effects. All coefficients were positive and close to 1.0, the minimum value being 0.9883 for the M effect of medium-educated women in Denmark. Thus, both methods do not differ with regard to the direction of the estimated effects and they vary only marginally with regard to their magnitudes.
Further, we tested to what extent different definitions of education levels affect the results of our study. We did this with the data for Italy by performing the analysis on the basis of different classifications of education, including a narrower definition of low education (ISCED 0–1) and a broader definition of medium education (ISCED 2–4) as well as the use of four education classes (ISCED 0–1, 2–3, 4, 5–6). In all cases, the estimated P effects went in the same direction but were larger than in the presented data based on the conventional three education classes (ISCED 0–2, 3–4, 5–6). Note that if a continuous underlying relationship between length of education and level of life expectancy is assumed, then any categorization into discrete education categories will necessarily result in an underestimation of the P effect. This underestimation gets the stronger the smaller the number of categories is and the broader the education ranges they cover. If over time the average length of education within, e.g., the middle category of education increases, this would also lead to an increase in the life expectancy of the people belonging to this category. Under the three-category-framework, this increase in life expectancy would, however, not be classified as an education effect but rather as an exogenous mortality decline effect. If, on the other hand, the middle education category would be split into two, part of this improvement in both education and life expectancy would result in more people moving from the lower middle to the upper middle education category and the resulting life expectancy increase would result in a stronger P effect. The above mentioned results of the sensitivity analysis with four education categories clearly confirm this pattern. More generally, it can be said that the larger the number of education categories—i.e., the more closely it reflects the likely continuous underlying relationship—the larger will be the effect attributed to P.
The data for Italy also allowed us to carry out the decomposition for the 30-year period from 1981 to 2011. This analysis yielded basically identical outcomes in terms of the relative contribution of the increasing education level to rising life expectancy. Finally, a year-by-year decomposition with annual data for Denmark revealed that the P effect builds up gradually and shows less fluctuations than the M effect, indicating that our conclusions with regard to the effect of populations’ changing educational structure on changing life expectancy are not affected by the direct decomposition of changes between the start and the end year of the study period.
Life tables derived from different data sources for deaths and population at risk as done in the present study for Italy and the USA always entail the risk of a numerator-denominator bias (Sorlie and Johnson 1996 ). Note that our study focuses on the estimation of the association of increasing education levels with overall life expectancy. Our data are period life tables which model what would happen to a population if a given set of mortality conditions were experienced: the empirical data are an approximate reflection of actual conditions. Therefore, it is most important to estimate for each population education-specific death rates for the start and end years with the same methodological approach, what was only possible in the performed manner. Yet, it seems unlikely that our conclusions are influenced by this issue as well as by possible inaccuracies in the reporting of education levels and our imputation strategy for missing data. Regarding the latter, it is likely that our proportional redistribution of deaths with unknown education level on the basis of the deaths with information about education leads to a slight underestimation of the P effect. Footnote 9 There might be further sources for bias connected to some other technical and conceptual issues (see Jasilionis and Shkolnikov 2016 ), but we expect these to be of only minor relevance for the studied populations and the quality of the underlying data.
In sum, the sensitivity analyses suggest that none of these methodological and technical issues affected the conclusions of this study, and that the presented magnitude of the P effect is rather conservative. It is important to note, however, that the estimated life expectancy by education level cannot be compared across the studied populations because of differences in the underlying data characteristics as described in the “ Data ” section. For the same reason, our levels of education-specific life expectancy can differ from other studies which use different data for the same populations. In fact, our estimates for life expectancy at age 30 by education level provide larger inequalities between the highest and lowest education levels than comparable data from EUROSTAT or OECD (Corsini 2010 ; Murtin et al. 2017 ). These deviations are strongest for the populations from Italy and the USA, what indicates that our use of unlinked data leads to an overestimation of differences in life expectancy compared to linked data. However, linked data were only available for population subsamples for our earlier observation period. Given that we gave priority to use data that covers the total populations, we could not avoid the use of unlinked data for Italy and the USA. For estimating maximum precise levels of education-specific differences in mortality, such a linkage is preferable to our analysis based on independent data sources for the education-specific risk populations and numbers of death by age (see also Valkonen 2006 ).
Summary of findings
This study examined the extent to which changes in the educational structure are related to changes in life expectancy at age 30 in Italy (1991–2011), Denmark (1990–2010), and the USA (1990–2010). We used the same analytical strategy as Shkolnikov et al. ( 2006 ) and Jasilionis et al. ( 2007 ) to decompose changes in life expectancy at age 30 into the effects of education-specific mortality changes (M effect) and the effects of changes in the populations’ educational structure (P effect). The present study improves upon prior research by (1) testing the association between changes in the educational structure of the populations and changes in life expectancy at adult ages, (2) utilizing data from three different countries which markedly differ in terms of populations’ health status and education profile, and (3) including more recent years in the analyses. Our findings contribute to the debate about the role of educational attainment in changing population health status.
Three main findings emerge from our analyses. First, there were considerable changes in the educational composition in all three countries during the study period. The proportion of those with low educational attainment decreased, while the proportion of medium and highly educated individuals increased. There were particularly large increases in Italy and Denmark. Second, life expectancy was distributed in a graded fashion across education groups. Life expectancy was highest for those who had more than high school education, followed by those with medium and low educational attainment levels. Importantly, the gap in e 30 between the highest and lowest educational levels increased over the two decades of our study period. Third, the results from decomposition analysis show that the structural change in education accounted for a substantial proportion of improvements in overall life expectancy in all three countries. Specifically, we found that populations’ changes in educational attainment explained between around 15% (men in the USA) and approximately 40% (women in Denmark) of the increases in life expectancy at age 30 between 1990 and 2010.
Interpretation of findings
The present study provides some extension of our understanding of the mechanisms behind recent improvements in life expectancy. Prior research has tended to focus on medical advancements, in particular developments in the screening, treatment, and prevention of cardiovascular diseases, as major contributors to improved population health (Mackenbach et al. 1988 ; Vallin and Meslé 2004 ; Wilmoth 2000 ). Our results demonstrate the additional importance of education in this process. As described in the fundamental social causes of health inequalities theory, education helps individuals to develop health-related resources, allowing highly educated people to enjoy longer and healthier lives (Link and Phelan 1995 ). When new health technologies are developed, it is likely that people with higher education use resources they own, namely money, knowledge, prestige, power and beneficial social connections, to gain access to life-saving innovations.
The presented findings contain also some policy implications. For instance, they provide support for previous studies which suggested to consider education policy within the framework of health policy (e.g., Lleras-Muney 2005 ; Schoeni and Ofstedal 2010 ; Stringhini et al. 2017 ). The Millennium Development Goals (MDGs) sought to achieve universal primary education, with a special focus on less-developed countries. The post-MDGs international development agenda, Sustainable Development Goals (SDGs), also includes goals related to education, such as ensuring inclusive and equitable quality of education and lifelong learning for all. The present findings suggest that these efforts not only contribute to the expansion of mass education but are likely to lead also to reductions in mortality and an increase in populations’ life expectancy.
Building on this conclusion, the findings of our study add also insights into the discussion about the future of human longevity. Two major lines of thought have developed in recent years, which are referred to as “optimistic” and “pessimistic” (see Caselli et al. 2014 for details). In principle, both perspectives agree that mortality will continue to decrease. They differ, however, in their opinions on the time course and magnitude of these improvements. According to the pessimists, future progress will be smaller than in the past because the eradication of infectious diseases in industrialized societies will slow down the potential and the pace of mortality declines (Olshansky et al. 1990 ). In addition, new risk factors, such as obesity and global pandemic outbreaks, are thought to moderate improvements in human longevity (Olshansky 2005 ). In contrast, the optimists claim that mortality rates will further decline particularly at older ages, leading to un-damped gains in life expectancy (Christensen et al. 2009 ; Vaupel 2010 ). Although debate continues regarding future scenarios of human longevity, the current study provides a so far neglected factor in support of the optimistic perspective. The average years of education in the world are projected to increase from 7.9 years in 2010 to 11.0 years by 2060 (Lutz et al. 2014a ). It seems likely that these further increases of the average education level will continue to contribute to the process of mortality reduction (see also Deeg et al. 2013 ).
Nonetheless, measures and programs to improve the health of less-educated individuals are needed. Despite impressive changes in the distribution of educational attainment during the past 20 years, a large proportion of the studied populations still had less than upper secondary education. Moreover, educational gradients in life expectancy widened during the last decades in many industrialized populations as reflected in the presented estimations (see also Caselli et al. 2014 ; Shkolnikov et al. 2012 ). Hendi ( 2015 ) and Goldring et al. ( 2016 ) have shown that much of these changes in education-specific life expectancy are due to the shifts in the populations’ educational structure. Note that, the mortality benefits of education appear to be particularly large for better-educated people, while those with low education continue to be left behind. In this context, it is important to note that the improvements in educational attainment might cause the decreasing proportion of people with low education to become increasingly selected towards adverse health conditions (Deboosere et al. 2009 ; Hendi 2017 ).
Some issues should be noted which could provide the ground for fruitful follow-up research. First, our study includes only three countries, though their populations cover a broad spectrum of education levels and transitions across modern western nations. Shkolnikov et al. ( 2006 ) and Jasilionis et al. ( 2007 ) performed a comparable analysis for some northern, central, and eastern European countries between the late 1980s and 1990s. For Finland, they found a similar range of (annual) contributions of the P effect to changes in life expectancy as we did for Italy, Denmark, and the USA. The Czech Republic shows smaller effects of structural changes. For Russia, Estonia, and Lithuania, the P effects were even larger than in our study but overlaid by the drastic negative M effects prevalent at that time. Sweden shows the largest effects of education-specific changes in the population structure. Larger effects of the structural changes compared to our estimates were also presented by Klein et al. ( 2006 ) for the increase in life expectancy between the female cohorts 1925 and 1955 of Germany, by Turra et al. ( 2016 ) for changes in life expectancy among Brazilian women between 1960 and 2010, and by De Grande et al. ( 2014 ) for changes between the periods 1991/1996 and 2001/2006 among specific urban and non-urban subpopulations from Belgium. The latter study restricted the analysis, however, to young adults aged 19–34 and decomposed changes in the standardized mortality rates instead of life expectancy. In any case, all estimates available to date refer to industrialized societies. As improvements in educational attainment have also been (and will further be) made in the less-developed countries of the world (Gakidou et al. 2010 ; Lutz et al. 2014a ), including more countries into the analyses—both more-developed and less-developed nations—should be a topic of future investigations.
Second, we partitioned changes in life expectancy only into changes in mortality and educational structure. Although evidence shows that education itself is in part directly related to mortality (Geyer and Peter 1999 ; Lleras-Muney 2005 ; van Kippersluis et al. 2011 ), there is also a strong correlation with other dimensions of socioeconomic status, such as income, occupation, and wealth (Ross and Wu 1995 ). It would be challenging if it were possible to include these factors into the decomposition. Moreover, the macro-level effect of education on mortality might be moderated through other characteristics of the populations, such as ethnicity or country of birth. For instance, a large literature suggests substantial black-white mortality differences in the USA (Williams 2005 ), which are associated with mortality differences by education level. Montez et al. ( 2012 ) have shown that high school education is a threshold for mortality reduction for blacks in the USA, while whites enjoy mortality benefits from continued education after high school. Such heterogeneity effects are manifold and can arise from different causal pathways.
Finally, we assumed the comparability of education levels across countries during the observation time. Although we used a standardized measurement of education (ISCED-97), educational systems as well as the quality of education may differ significantly. Moreover, the studied populations experienced differences in the timing and dynamics of the process of the educational transition. This point has a notable relevance for the described between-country differences in the education-specific origins of the M effects. Lower secondary education became mandatory in Italy only after the 1950s and until age 16 only after 2007. Therefore, most of the people in the low education group around 1990 had no educational attainment at all, especially among the old and oldest old. By the early 2010s, a large proportion of these cohorts had died and the majority of the less-educated subpopulation had a lower secondary degree. In Denmark and the USA, these shifts occurred already earlier. This explains why enrollment into secondary education yielded larger M effects in Italy than in the other two countries. Thus, our understanding of the education-mortality association on the population level would also be deepened by research that accounts for the context and quality of populations’ education and related cohort effects.
To conclude, this study demonstrates that progress in education has made important contributions to increasing life expectancy in Italy, Denmark, and the USA over the past two decades. These findings are in line with the theoretical heterogeneity approach, which states that mortality levels and differences in mortality are strongly influenced by the specific risk group composition of populations (Vaupel et al. 1979 ; Vaupel and Yashin 1985 ). In addition to all the other important benefits of education (Lutz 2009 ; Lutz et al. 2014b ), it can also be viewed as a powerful health policy which allows more people to enjoy both better and longer lives. As aptly summarized by Kaplan et al. ( 2014 : 193), “Enhancing health outcomes through improved educational attainment is an attractive alternative, although we still need better evidence that interventions to improve educational attainment will increase life expectancy.” Our contribution tried to provide a piece of this evidence.
Web address: http://dati.istat.it/
Web address: http://www.mortality.org
Web address: https://usa.ipums.org/
Web address: http://www.cdc.gov/nchs/nvss.htm
See: http://www.cdc.gov/nchs/nvss/vital_certificate_revisions.htm
See: https://international.ipums.org/international-action/variables/EDATTAIN#description_section
We used the Brass model instead of the more commonly used models of Gompertz or Kannisto because of the comparatively young age 65 as starting point for the extrapolation. The Brass model adjusts the survival function of a complete standard life table (in our case life tables for the total populations with single age data from 30 to 110) to fit the available but truncated information about the mortality of the analyzed population (in our case the subpopulations by education level from ages 30 to 64). The Brass model uses two parameters for this adjustment: the alpha parameter reflecting the level of the subpopulations’ overall mortality and the beta parameter for the subpopulations’ specific age pattern of mortality, both in relation to the standard life table. Luy et al. ( 2015 ) have demonstrated the functionality of this approach in their study of life expectancy by education, income, and occupation for the German population. Details about the extrapolation of age-specific probabilities of dying with the Brass model can be found there. Descriptions of the derivation of the Brass parameters alpha and beta from empirical data can be found in Ngom and Bawah ( 2004 ) and Stewart ( 2004 ).
MS Excel File: Decomposition_replacement_from_young_to_old_ages(4).xls
In an alternative analysis, we assigned all deaths with unknown education to the lowest education level (based on the assumption that the majority of deaths with unknown education level—albeit not all—are likely to belong to this group). This scenario led to huge differences compared to our old model for the population of the USA because the life expectancy of the lowest education decreased strongly for the low education group in period 1 (from 41.7 to 34.4 years among men and from 49.5 to 42.9 years among women). Consequently, the low education group experienced by far the strongest increase in life expectancy between period 1 and period 2. This led (1) to an extreme shift of the education-specific M effects from the high to the low education level and (2) regarding the total effects to a shift from the total M effect to the P effect. Among men of the USA, the P effect increased from 0.6 years in our old model to 2.1 years, and among women of the USA from 0.4 to 1.7 years. For the Italian population, these shifts were considerably smaller (because of the much lower number of deaths with unknown education level in period 1), with the P effects increasing from 1.1 to 1.5 years among men and from 0.7 to 1.2 years among women.
Andreev, E. M. (1982). Metod komponent v analize prodoljitelnosty zjizni [the method of components in the analysis of length of life]. Vestnik Statistiki, 9 (3), 42–47.
Google Scholar
Andreev, E. M., & Shkolnikov, V. M. (2012). An Excel spreadsheet for the decomposition of a difference between two values of an aggregate demographic measure by stepwise replacement running from young to old ages. MPIDR technical report TR-2012-002 . Rostock: Max Planck Institute for Demographic Research.
Andreev, E. M., Shkolnikov, V. M., & Begun, A. Z. (2002). Algorithm for decomposition of differences between aggregate demographic measures and its application to life expectancies, healthy life expectancies, parity-progression ratios and total fertility rates. Demographic Research, 7 (14), 499–522.
Arendt, J. N. (2005). Does education cause better health? A panel data analysis using school reforms for identification. Economics of Education Review, 24 (2), 149–160.
Baker, D. P., Leon, J., Smith Greenaway, E. G., Collins, J., & Movit, M. (2011). The education effect on population health: a reassessment. Population and Development Review, 37 (2), 307–332.
Brass, W. (1971). On the scale of mortality. In W. Brass (Ed.), Biological aspects of demography (pp. 69–110). London: Taylor and Francis.
Caselli, G. (2015). Mortality, epidemiological, and health transitions. In J. D. Wright (Ed.), International encyclopedia of the social & behavioral Sciences (2nd ed., pp. 857–862). Oxford: Elsevier.
Caselli, G., Drefahl, S., Wegner-Siegmundt, C., & Luy, M. (2014). Future mortality in low mortality countries. In W. Lutz, W. P. Butz, & S. KC (Eds.), World population and human capital in the twenty-first century (pp. 226–272). Oxford: Oxford University Press.
Christensen, K., Doblhammer, G., Rau, R., & Vaupel, J. W. (2009). Ageing populations: the challenges ahead. Lancet, 374 (9696), 1196–1208.
Clark, D., & Royer, H. (2013). The effect of education on adult mortality and health: evidence from Britain. American Economic Review, 103 (6), 2087–2120.
Corsini, V. (2010). Highly educated men and women likely to live longer. Life expectancy by educational attainment. Statistics in focus 24/2010 . Brussels: Eurostat.
Das Gupta, P. (1978). A general method of decomposing a difference between two rates into several components. Demography, 15 (1), 99–112.
Das Gupta, P. (1993). Standardisation and decomposition of rates: a user’s manual. Current population reports P23–186 . Wasghington: U.S. Government Printing Office.
Davey Smith, G., Hart, C., Hole, D., MacKinnon, P., Gillis, C., Watt, G., Blane, D., & Hawthorne, V. (1998). Education and occupational social class: which is the more important indicator of mortality risk? Journal of Epidemiology and Community Health, 52 (3), 153–160.
De Grande, H., Vandenheede, H., & Deboosere, P. (2014). Trends in young-adult mortality between the 1990s and the 2000s in urban and non-urban areas in Belgium: the role of a changing educational composition in overall mortality decline. Health & Place, 30 , 61–69.
Deboosere, P., Gadeyne, S., & Van Oyen, H. (2009). The 1991-2004 evolution in life expectancy by educational level in Belgium based on linked census and population register data. European Journal of Population, 25 (2), 175–196.
Deeg, D. J. H., van Vliet, M. J. G., Kardaun, J. W. P. F., & Huisman, M. (2013). Understanding the mortality decline at older ages. Improved life course or improved present period? Annual Review of Gerontology and Geriatrics, 33 (1), 261–291.
Denney, J. T., Rogers, R. G., Hummer, R. A., & Pampel, F. C. (2010). Education inequality in mortality: the age and gender specific mediating effects of cigarette smoking. Social Science Research, 39 (4), 662–673.
Gakidou, E., Cowling, K., Lozano, R., & Murray, C. J. L. (2010). Increased educational attainment and its effect on child mortality in 175 countries between 1970 and 2009: a systematic analysis. The Lancet, 376 (9745), 959–974.
Geyer, S., & Peter, R. (1999). Occupational status and all-cause mortality. A study with health insurance data from Nordrhein-Westfalen, Germany. European Journal of Public Health, 9 (2), 114–118.
Geyer, S., & Peter, R. (2000). Income, occupational position, qualification and health inequalities—competing risks? (comparing indicators of social status). Journal of Epidemiology and Community Health, 54 (4), 299–305.
Goldring, T., Lange, F., & Richards-Shubik, S. (2016). Testing for changes in the SES-mortality gradient when the distribution of education changes too. Journal of Health Economics, 46 , 120–130.
Hayward, M. D., Hummer, R. A., & Sasson, I. (2015). Trends and group differences in the association between educational attainment and U.S. adult mortality: implications for understanding education's causal influence. Social Science & Medicine, 127 , 8–18.
Hendi, A. S. (2015). Trends in U.S. life expectancy gradients: the role of changing educational composition. International Journal of Epidemiology, 44 (3), 946–955.
Hendi, A. S. (2017). Trends in education-specific life expectancy, data quality, and shifting education distributions: a note on recent research. Demography, 54 (3), 1203–1213.
House, J. S., Landis, K. R., & Umberson, D. (1988). Social relationships and health. Science, 241 (4865), 540–545.
Human Mortality Database. 2017. University of California, Berkeley (USA), and Max Planck Institute for Demographic Research (Germany). Available at www.mortality.org or www.humanmortality.de (data downloaded on 25 Sept 2017).
Hummer, R. A., & Lariscy, J. T. (2011). Educational attainment and adult mortality. In R. G. Rogers & E. M. Crimmins (Eds.), International handbook of adult mortality (pp. 241–261). Dordrecht, Heidelberg, London, New York: Springer.
Janssen, F., & Kunst, A. E. (2005). Cohort patterns in mortality trends among the elderly in seven European countries, 1950-99. International Journal of Epidemiology, 34 (5), 1149–1159.
Jasilionis, D., & Shkolnikov, V. M. (2016). Longevity and education: a demographic perspective. Gerontology, 62 (3), 253–262.
Jasilionis, D., Jdanov, D., & Leinsalu, M. (2007). Der Zusammenhang von Bildung und Lebenserwartung in Mittel-und Osteuropa. Jahrbuch der Max-Planck-Gesellschaft, 2006-2007 , 103–108.
Kaplan, R. M., Spittel, M. L., & Zeno, T. L. (2014). Educational attainment and life expectancy. Policy Insights From the Behavioral and Brain Sciences, 1 (1), 189–194.
Kilpi, F., Silventoinen, K., Konttinen, H., & Martikainen, P. (2016). Disentangling the relative importance of different socioeconomic resources for myocardial infarction incidence and survival: a longitudinal study of over 300 000 Finnish adults. The European Journal of Public Health, 26 (2), 260–266.
Kingston, P. W., Hubbard, R., Lapp, B., Schroeder, P., & Wilson, J. (2003). Why education matters. Sociology of Education, 76 (1), 53–70.
van Kippersluis, H., O’Donnell, O., & van Doorslaer, E. (2011). Long-run returns to education: does schooling lead to an extended old age? Journal of Human Resources, 46 (4), 695–721.
Klein, T., Unger, R., & Schulze, A. (2006). Bildungsexpansion und Lebenserwartung. In A. Hadjar & R. Becker (Eds.), Die Bildungsexpansion. Erwartete und unerwartete Folgen (pp. 311–331). Wiesbaden: VS Verlag für Sozialwissenschaften.
Kröger, H., Pakpahan, E., & Hoffmann, R. (2015). What causes health inequality? A systematic review on the relative importance of social causation and health selection. The European Journal of Public Health, 25 (6), 951–960.
Laaksonen, M., Talala, K., Martelin, T., Rahkonen, O., Roos, E., Helakorpi, S., Laatikainen, T., & Prättälä, R. (2008). Health behaviours as explanations for educational level differences in cardiovascular and all-cause mortality: a follow-up of 60 000 men and women over 23 years. The European Journal of Public Health, 18 (1), 38–43.
Lager, A. C. J., & Torssander, J. (2012). Causal effect of education on mortality in a quasi-experiment on 1.2 million Swedes. Proceedings of the National Academy of Sciences, 109 (22), 8461–8466.
Link, B. G., & Phelan, J. (1995). Social conditions as fundamental causes of disease. Journal of Health and Social Behavior, 35 (extra issue), 80–94.
Lleras-Muney, A. (2005). The relationship between education and adult mortality in the United States. Review of Economic Studies, 72 (1), 189–221.
Lutz, W. (2009). Sola schola et sanitate: human capital as the root cause and priority for international development? Philosophical Transactions of the Royal Society of London B: Biological Sciences, 364 (1532), 3031–3047.
Lutz, W., & Skirbekk, V. (2014). How education drives demography and knowledge informs projection. In W. Lutz, W. P. Butz, & S. KC (Eds.), World population and human capital in the twenty-first century (pp. 14–38). Oxford: Oxford University Press.
Lutz, W., Butz, W. P., & S. KC. (2014a). World population and human capital in the twenty-first century . Oxford: Oxford University Press.
Lutz, W., Muttarak, R., & Striessnig, E. (2014b). Universal education is key to enhanced climate adaptation. Science, 346 (6213), 1061–1062.
Luy, M., Wegner-Siegmundt, C., Wiedemann, A., & Spijker, J. (2015). Life expectancy by education, income and occupation in Germany: estimations using the longitudinal survival method. Comparative Population Studies, 40 (4), 399–436.
Mackenbach, J. P., Looman, C. W. N., Kunst, A. E., Habbema, J. D. F., & van der Maas, P. J. (1988). Post-1950 mortality trends and medical care: gains in life expectancy due to declines in mortality from conditions amenable to medical intervention in the Netherlands. Social Science & Medicine, 27 (9), 889–894.
Mirowsky, J., & Ross, C. E. (2003). Education, social status, and health . New York: Aldine de Gruyter.
Montez, J. K., & Friedman, E. M. (2015). Educational attainment and adult health: contextualizing causality . Social Science & Medicine Special Issue. Elsevier.
Montez, J. K., Hummer, R. A., & Hayward, M. D. (2012). Educational attainment and adult mortality in the United States: a systematic analysis of functional form. Demography, 49 (1), 315–336.
Murtin, F., Mackenbach, J., Jasilionis, D., & Mira d'Ercole, M. (2017). Inequalities in longevity by education in OECD countries. Insights from new OECD estimates. OECD statistics working papers 2017/02 . Paris: OECD Publishing.
Ngom, P., & Bawah, A. A. (2004). INDEPTH model life tables for sub-Saharan Africa . Aldershot, Burlington: Ashgate.
OECD. (2016). Education at a glance 2016: OECD indicators . Paris: OECD Publishing.
Olshansky, S. J. (2005). Projecting the future of U.S. health and longevity. Health Affairs, 24 (supplement 2), W5R86–W85R89.
Olshansky, S. J., & Ault, A. B. (1986). The fourth stage of the epidemiologic transition: the age at delayed degenerative diseases. The Milbank Quarterly, 64 (3), 355–391.
Olshansky, S. J., Carnes, B. A., & Cassel, C. (1990). In search of Methuselah: estimating the upper limits to human longevity. Science, 250 (4981), 634–640.
Omran, A. R. (1971). The epidemiologic transition. A theory of the epidemiology of population change. The Milbank Memorial Fund Quarterly, 49 (4), 509–537.
Omran, A. R. (1983). The epidemiologic transition theory. A preliminary update. Journal of Tropical Pediatrics, 29 (6), 305–316.
Pressat, R. (1985). Contribution des écarts de mortalité par âge á la différence des vies moyennes. Population, 4 (5), 766–770.
Preston, S. H., Heuveline, P., & Guillot, M. (2001). Demography: Measuring and modeling population processes . Oxford: Blackwell.
Qian, Z., & Preston, S. H. (1993). Changes in American marriage, 1972 to 1987: availability and forces of attraction by age and education. American Sociological Review, 58 (4), 482–495.
Rogers, R. G., & Hackenberg, R. (1987). Extending epidemiologic transition theory: a new stage. Social Biology, 34 (3–4), 234–243.
Rogers, R. G., Hummer, R. A., & Nam, C. B. (1999). Living and dying in the USA: behavioral, health, and social differentials of adult mortality . San Diego: Academic Press.
Rogers, R. G., Everett, B. G., Zajacova, A., & Hummer, R. A. (2010). Educational degrees and adult mortality risk in the United States. Biodemography and Social Biology, 56 (1), 80–99.
Ross, C. E., & Wu, C.-l. (1995). The links between education and health. American Sociological Review, 60 (5), 719–745.
Ruggles, S., Genadek, K., Goeken, R., Grover, J., & Sobek, M. (2015). Integrated public use microdata series: Version 6.0 [machine-readable database] . Minneapolis: University of Minnesota.
Ryan, C. L., & Bauman, K. (2016). Educational attainment in the United States: 2015 . Current Population Reports Washington: United States Census Bureau.
Schoeni, R. F., & Ofstedal, M. B. (2010). Key themes in research on the demography of aging. Demography, 47 (supplement), S5–S15.
Shkolnikov, V. M., Andreev, E. M., & Begun, A. Z. (2003). Gini coefficient as a life table function: computation from discrete data, decomposition of differences and empirical examples. Demographic Research, 8 (11), 305–358.
Shkolnikov, V. M., Andreev, E. M., Jasilionis, D., Leinsalu, M., Antonova, O. I., & McKee, M. (2006). The changing relation between education and life expectancy in central and eastern Europe in the 1990s. Journal of Epidemiology and Community Health, 60 (10), 875–881.
Shkolnikov, V. M., Andreev, E. M., Jdanov, D. A., Jasilionis, D., Kravdal, Ø., Vågerö, D., & Valkonen, T. (2012). Increasing absolute mortality disparities by education in Finland, Norway and Sweden, 1971–2000. Journal of Epidemiology and Community Health, 66 (4), 372–378.
Sorlie, P. D., & Johnson, N. J. (1996). Validity of education information on the death certificate. Epidemiology, 7 (4), 437–439.
Stewart, Q. T. (2004). Brass' relational model: a statistical analysis. Mathematical Population Studies, 11 (1), 51–72.
Stringhini, S., Carmeli, C., Jokela, M., Avendaño, M., Muennig, P., Guida, F., Ricceri, F., d'Errico, A., Barros, H., Bochud, M., Chadeau-Hyam, M., Clavel-Chapelon, F., Costa, G., Delpierre, C., Fraga, S., Goldberg, M., Giles, G. G., Krogh, V., Kelly-Irving, M., Layte, R., Lasserre, A. M., Marmot, M. G., Preisig, M., Shipley, M. J., Vollenweider, P., Zins, M., Kawachi, I., Steptoe, A., Mackenbach, J. P., Vineis, P., & Kivimäki, M. (2017). Socioeconomic status and the 25x25 risk factors as determinants of premature mortality: a multicohort study and meta-analysis of 1.7 million men and women. The Lancet . https://doi.org/10.1016/S0140-6736(1016)32380-32387 .
Thygesen, L. C., Daasnes, C., Thaulow, I., & Brønnum-Hansen, H. (2011). Introduction to Danish (nationwide) registers on health and social issues: structure, access, legislation, and archiving. Scandinavian Journal of Public Health, 39 (7 suppl), 12–16.
Turra, C. M., Renteria, E., & Guimarães, R. (2016). The effect of changes in educational composition on adult female mortality in Brazil. Research on Aging, 38 (3), 283–298.
UNESCO. (1996). International standard classification of education ISCED 1997 . Montreal: UNESCO-UIS.
Valkonen, T. (2006). Social inequalities in mortality. In G. Caselli, J. Vallin, & G. Wunsch (Eds.), Demography: analysis and synthesis (Vol. 2, pp. 195–206). London: Academic Press.
Vallin, J., & Meslé, F. (2004). Convergences and divergences in mortality. A new approach to health transition. Demographic Research, 2 (2), 11–44.
Vaupel, J. W. (2010). Biodemography of human ageing. Nature, 465 (25 march), 536–542.
Vaupel, J. W., & Yashin, A. I. (1985). Heterogeneity's ruses: some surprising effects of selection on population dynamics. The American Statistician, 39 (3), 176–185.
Vaupel, J. W., Manton, K. G., & Stallard, E. (1979). The impact of heterogeneity in individual frailty on the dynamics of mortality. Demography, 16 (3), 439–454.
Williams, D. R. (2005). The health of U.S. racial and ethnic populations. The Journals of Gerontology Series B: Psychological Sciences and Social Sciences, 60 (special issue 2), S53–S62.
Wilmoth, J. R. (2000). Demography of longevity: past, present, and future trends. Experimental Gerontology, 35 , 1111–1129.
Woolf, S. H., Johnson, R. E., Phillips, R. L., & Philipsen, M. (2007). Giving everyone the health of the educated: an examination of whether social change would save more lives than medical advances. American Journal of Public Health, 97 (4), 679–683.
Download references
Acknowledgements
We thank Henrik Brønnum-Hansen (University of Copenhagen) for preparing and providing us the data for Denmark, two anonymous reviewers of this manuscript, and Werner Richter for language editing.
This research was supported by the European Research Council, within the European Community’s Seventh Framework Programme (FP7/2007–2013), ERC Grant Agreement No. 262663 (HEMOX) and within the EU Framework Programme for Research and Innovation Horizon 2020, ERC Grant Agreement No. 725187 (LETHE).
Availability of data and materials
All data are available online or in printed form as described in the " Data " section. The life table calculations have been made with MS Excel and the decomposition analyses with the MS Excel macro “Decomposition_replacement_from_young_to_old_ages(4).xls” provided by Andreev and Shkolnikov ( 2012 ) (see " Methods " section). All data and results are online available in the Additional file.
Author information
Authors and affiliations.
Wittgenstein Centre for Demography and Global Human Capital (IIASA, VID/ÖAW, WU), Welthandelsplatz 2 / Level 2, 1020, Vienna, Austria
Marc Luy, Marina Zannella, Christian Wegner-Siegmundt & Wolfgang Lutz
Vienna Institute of Demography of the Austrian Academy of Sciences, Welthandelsplatz 2 / Level 2, 1020, Vienna, Austria
International Institute for Applied Systems Analysis, Laxenburg, Austria
Wolfgang Lutz
Vienna University of Economics and Business, Welthandelsplatz 2 / Level 3, 1020, Vienna, Austria
Faculty of Liberal Art, Sophia University, 7-1 Kioicho Chiyodaku, Tokyo, 102-8554, Japan
Yuka Minagawa
Department of Statistical Sciences, Sapienza University of Rome, Viale Regina Elena 295, 00161, Rome, Italy
Graziella Caselli
Department of Methods and Models for Economics, Territory and Finance, Sapienza University of Rome, Via Del Castro Laurenziano 9, 00161, Rome, Italy
Marina Zannella
You can also search for this author in PubMed Google Scholar
Contributions
ML and GC developed the research idea and designed the study. ML directed and supervised the analyses and wrote large parts of the paper. CW-S performed the analyses, directed a systematic literature review with PubMed, and gave inputs to the paper, in particular the “ Methods ” section. MZ collected and prepared the data for Italy and the USA, supported the analyses, and gave inputs to the paper. YM reviewed the literature and prepared large parts of the paper. WL and GC contributed to the interpretation of the data and commented on all parts of the paper with corresponding inputs to the text. All authors read and approved the final manuscript.
Corresponding author
Correspondence to Marc Luy .
Ethics declarations
Competing interests.
The authors declare that they have no competing interests.
Publisher’s Note
Springer Nature remains neutral with regard to jurisdictional claims in published maps and institutional affiliations.
Additional file:
Additional file 1:.
Basic data and life tables by educational attainment. (CSV 54 kb)
Rights and permissions
Open Access This article is distributed under the terms of the Creative Commons Attribution 4.0 International License ( http://creativecommons.org/licenses/by/4.0/ ), which permits unrestricted use, distribution, and reproduction in any medium, provided you give appropriate credit to the original author(s) and the source, provide a link to the Creative Commons license, and indicate if changes were made.
Reprints and permissions
About this article
Cite this article.
Luy, M., Zannella, M., Wegner-Siegmundt, C. et al. The impact of increasing education levels on rising life expectancy: a decomposition analysis for Italy, Denmark, and the USA. Genus 75 , 11 (2019). https://doi.org/10.1186/s41118-019-0055-0
Download citation
Received : 10 October 2018
Accepted : 15 January 2019
Published : 07 March 2019
DOI : https://doi.org/10.1186/s41118-019-0055-0
Share this article
Anyone you share the following link with will be able to read this content:
Sorry, a shareable link is not currently available for this article.
Provided by the Springer Nature SharedIt content-sharing initiative
- Life expectancy
- Human capital
- Population structure
- Decomposition; Health policy
- Search Menu
- Browse content in A - General Economics and Teaching
- Browse content in A1 - General Economics
- A12 - Relation of Economics to Other Disciplines
- A13 - Relation of Economics to Social Values
- A14 - Sociology of Economics
- Browse content in B - History of Economic Thought, Methodology, and Heterodox Approaches
- Browse content in B2 - History of Economic Thought since 1925
- B25 - Historical; Institutional; Evolutionary; Austrian
- B26 - Financial Economics
- Browse content in B4 - Economic Methodology
- B40 - General
- B41 - Economic Methodology
- Browse content in B5 - Current Heterodox Approaches
- B52 - Institutional; Evolutionary
- Browse content in C - Mathematical and Quantitative Methods
- Browse content in C0 - General
- C01 - Econometrics
- Browse content in C1 - Econometric and Statistical Methods and Methodology: General
- C11 - Bayesian Analysis: General
- C12 - Hypothesis Testing: General
- C14 - Semiparametric and Nonparametric Methods: General
- C15 - Statistical Simulation Methods: General
- Browse content in C2 - Single Equation Models; Single Variables
- C21 - Cross-Sectional Models; Spatial Models; Treatment Effect Models; Quantile Regressions
- C23 - Panel Data Models; Spatio-temporal Models
- C25 - Discrete Regression and Qualitative Choice Models; Discrete Regressors; Proportions; Probabilities
- C26 - Instrumental Variables (IV) Estimation
- Browse content in C3 - Multiple or Simultaneous Equation Models; Multiple Variables
- C31 - Cross-Sectional Models; Spatial Models; Treatment Effect Models; Quantile Regressions; Social Interaction Models
- C33 - Panel Data Models; Spatio-temporal Models
- C38 - Classification Methods; Cluster Analysis; Principal Components; Factor Models
- Browse content in C4 - Econometric and Statistical Methods: Special Topics
- C40 - General
- C41 - Duration Analysis; Optimal Timing Strategies
- C49 - Other
- Browse content in C6 - Mathematical Methods; Programming Models; Mathematical and Simulation Modeling
- C60 - General
- C61 - Optimization Techniques; Programming Models; Dynamic Analysis
- C62 - Existence and Stability Conditions of Equilibrium
- Browse content in C9 - Design of Experiments
- C90 - General
- C99 - Other
- Browse content in D - Microeconomics
- Browse content in D0 - General
- D00 - General
- D01 - Microeconomic Behavior: Underlying Principles
- D02 - Institutions: Design, Formation, Operations, and Impact
- D03 - Behavioral Microeconomics: Underlying Principles
- Browse content in D1 - Household Behavior and Family Economics
- D13 - Household Production and Intrahousehold Allocation
- D14 - Household Saving; Personal Finance
- D19 - Other
- Browse content in D2 - Production and Organizations
- D21 - Firm Behavior: Theory
- D22 - Firm Behavior: Empirical Analysis
- D23 - Organizational Behavior; Transaction Costs; Property Rights
- D24 - Production; Cost; Capital; Capital, Total Factor, and Multifactor Productivity; Capacity
- Browse content in D3 - Distribution
- D31 - Personal Income, Wealth, and Their Distributions
- Browse content in D4 - Market Structure, Pricing, and Design
- D41 - Perfect Competition
- D43 - Oligopoly and Other Forms of Market Imperfection
- Browse content in D5 - General Equilibrium and Disequilibrium
- D53 - Financial Markets
- Browse content in D6 - Welfare Economics
- D61 - Allocative Efficiency; Cost-Benefit Analysis
- D62 - Externalities
- D63 - Equity, Justice, Inequality, and Other Normative Criteria and Measurement
- D64 - Altruism; Philanthropy
- Browse content in D7 - Analysis of Collective Decision-Making
- D72 - Political Processes: Rent-seeking, Lobbying, Elections, Legislatures, and Voting Behavior
- D74 - Conflict; Conflict Resolution; Alliances; Revolutions
- D78 - Positive Analysis of Policy Formulation and Implementation
- Browse content in D8 - Information, Knowledge, and Uncertainty
- D80 - General
- D81 - Criteria for Decision-Making under Risk and Uncertainty
- D82 - Asymmetric and Private Information; Mechanism Design
- D83 - Search; Learning; Information and Knowledge; Communication; Belief; Unawareness
- D85 - Network Formation and Analysis: Theory
- Browse content in D9 - Micro-Based Behavioral Economics
- D92 - Intertemporal Firm Choice, Investment, Capacity, and Financing
- Browse content in E - Macroeconomics and Monetary Economics
- Browse content in E2 - Consumption, Saving, Production, Investment, Labor Markets, and Informal Economy
- E21 - Consumption; Saving; Wealth
- E22 - Investment; Capital; Intangible Capital; Capacity
- E24 - Employment; Unemployment; Wages; Intergenerational Income Distribution; Aggregate Human Capital; Aggregate Labor Productivity
- Browse content in E3 - Prices, Business Fluctuations, and Cycles
- E32 - Business Fluctuations; Cycles
- Browse content in E4 - Money and Interest Rates
- E40 - General
- Browse content in E5 - Monetary Policy, Central Banking, and the Supply of Money and Credit
- E58 - Central Banks and Their Policies
- Browse content in F - International Economics
- Browse content in F0 - General
- F00 - General
- F02 - International Economic Order and Integration
- Browse content in F1 - Trade
- F10 - General
- F11 - Neoclassical Models of Trade
- F12 - Models of Trade with Imperfect Competition and Scale Economies; Fragmentation
- F13 - Trade Policy; International Trade Organizations
- F14 - Empirical Studies of Trade
- F15 - Economic Integration
- F16 - Trade and Labor Market Interactions
- F18 - Trade and Environment
- Browse content in F2 - International Factor Movements and International Business
- F20 - General
- F21 - International Investment; Long-Term Capital Movements
- F22 - International Migration
- F23 - Multinational Firms; International Business
- Browse content in F3 - International Finance
- F30 - General
- F31 - Foreign Exchange
- F32 - Current Account Adjustment; Short-Term Capital Movements
- F33 - International Monetary Arrangements and Institutions
- F34 - International Lending and Debt Problems
- F36 - Financial Aspects of Economic Integration
- Browse content in F4 - Macroeconomic Aspects of International Trade and Finance
- F40 - General
- F41 - Open Economy Macroeconomics
- F43 - Economic Growth of Open Economies
- F44 - International Business Cycles
- Browse content in F5 - International Relations, National Security, and International Political Economy
- F50 - General
- F52 - National Security; Economic Nationalism
- F55 - International Institutional Arrangements
- Browse content in F6 - Economic Impacts of Globalization
- F60 - General
- F61 - Microeconomic Impacts
- F63 - Economic Development
- F65 - Finance
- F66 - Labor
- F68 - Policy
- Browse content in G - Financial Economics
- Browse content in G0 - General
- G00 - General
- G01 - Financial Crises
- G02 - Behavioral Finance: Underlying Principles
- Browse content in G1 - General Financial Markets
- G10 - General
- G11 - Portfolio Choice; Investment Decisions
- G12 - Asset Pricing; Trading volume; Bond Interest Rates
- G14 - Information and Market Efficiency; Event Studies; Insider Trading
- G15 - International Financial Markets
- G18 - Government Policy and Regulation
- Browse content in G2 - Financial Institutions and Services
- G20 - General
- G21 - Banks; Depository Institutions; Micro Finance Institutions; Mortgages
- G22 - Insurance; Insurance Companies; Actuarial Studies
- G23 - Non-bank Financial Institutions; Financial Instruments; Institutional Investors
- G24 - Investment Banking; Venture Capital; Brokerage; Ratings and Ratings Agencies
- G28 - Government Policy and Regulation
- Browse content in G3 - Corporate Finance and Governance
- G30 - General
- G31 - Capital Budgeting; Fixed Investment and Inventory Studies; Capacity
- G32 - Financing Policy; Financial Risk and Risk Management; Capital and Ownership Structure; Value of Firms; Goodwill
- G34 - Mergers; Acquisitions; Restructuring; Corporate Governance
- G38 - Government Policy and Regulation
- Browse content in H - Public Economics
- H0 - General
- Browse content in H1 - Structure and Scope of Government
- H11 - Structure, Scope, and Performance of Government
- H13 - Economics of Eminent Domain; Expropriation; Nationalization
- Browse content in H2 - Taxation, Subsidies, and Revenue
- H20 - General
- H21 - Efficiency; Optimal Taxation
- H23 - Externalities; Redistributive Effects; Environmental Taxes and Subsidies
- H25 - Business Taxes and Subsidies
- H26 - Tax Evasion and Avoidance
- H27 - Other Sources of Revenue
- Browse content in H3 - Fiscal Policies and Behavior of Economic Agents
- H31 - Household
- Browse content in H4 - Publicly Provided Goods
- H41 - Public Goods
- H43 - Project Evaluation; Social Discount Rate
- H44 - Publicly Provided Goods: Mixed Markets
- Browse content in H5 - National Government Expenditures and Related Policies
- H52 - Government Expenditures and Education
- H53 - Government Expenditures and Welfare Programs
- H54 - Infrastructures; Other Public Investment and Capital Stock
- H55 - Social Security and Public Pensions
- H56 - National Security and War
- H57 - Procurement
- Browse content in H7 - State and Local Government; Intergovernmental Relations
- H70 - General
- H71 - State and Local Taxation, Subsidies, and Revenue
- H72 - State and Local Budget and Expenditures
- H73 - Interjurisdictional Differentials and Their Effects
- H74 - State and Local Borrowing
- H75 - State and Local Government: Health; Education; Welfare; Public Pensions
- H76 - State and Local Government: Other Expenditure Categories
- H77 - Intergovernmental Relations; Federalism; Secession
- Browse content in H8 - Miscellaneous Issues
- H81 - Governmental Loans; Loan Guarantees; Credits; Grants; Bailouts
- H89 - Other
- Browse content in I - Health, Education, and Welfare
- I0 - General
- Browse content in I1 - Health
- I14 - Health and Inequality
- I18 - Government Policy; Regulation; Public Health
- Browse content in I2 - Education and Research Institutions
- I20 - General
- I21 - Analysis of Education
- I22 - Educational Finance; Financial Aid
- I23 - Higher Education; Research Institutions
- I24 - Education and Inequality
- I25 - Education and Economic Development
- I28 - Government Policy
- I29 - Other
- Browse content in I3 - Welfare, Well-Being, and Poverty
- I30 - General
- I31 - General Welfare
- I32 - Measurement and Analysis of Poverty
- I38 - Government Policy; Provision and Effects of Welfare Programs
- Browse content in J - Labor and Demographic Economics
- Browse content in J0 - General
- J01 - Labor Economics: General
- Browse content in J1 - Demographic Economics
- J10 - General
- J11 - Demographic Trends, Macroeconomic Effects, and Forecasts
- J12 - Marriage; Marital Dissolution; Family Structure; Domestic Abuse
- J13 - Fertility; Family Planning; Child Care; Children; Youth
- J15 - Economics of Minorities, Races, Indigenous Peoples, and Immigrants; Non-labor Discrimination
- J16 - Economics of Gender; Non-labor Discrimination
- J18 - Public Policy
- J19 - Other
- Browse content in J2 - Demand and Supply of Labor
- J21 - Labor Force and Employment, Size, and Structure
- J22 - Time Allocation and Labor Supply
- J23 - Labor Demand
- J24 - Human Capital; Skills; Occupational Choice; Labor Productivity
- Browse content in J3 - Wages, Compensation, and Labor Costs
- J30 - General
- J31 - Wage Level and Structure; Wage Differentials
- J32 - Nonwage Labor Costs and Benefits; Retirement Plans; Private Pensions
- J38 - Public Policy
- Browse content in J4 - Particular Labor Markets
- J40 - General
- J41 - Labor Contracts
- J42 - Monopsony; Segmented Labor Markets
- J44 - Professional Labor Markets; Occupational Licensing
- J45 - Public Sector Labor Markets
- Browse content in J5 - Labor-Management Relations, Trade Unions, and Collective Bargaining
- J50 - General
- J51 - Trade Unions: Objectives, Structure, and Effects
- J53 - Labor-Management Relations; Industrial Jurisprudence
- Browse content in J6 - Mobility, Unemployment, Vacancies, and Immigrant Workers
- J60 - General
- J61 - Geographic Labor Mobility; Immigrant Workers
- J62 - Job, Occupational, and Intergenerational Mobility
- J63 - Turnover; Vacancies; Layoffs
- J64 - Unemployment: Models, Duration, Incidence, and Job Search
- J65 - Unemployment Insurance; Severance Pay; Plant Closings
- Browse content in J7 - Labor Discrimination
- J71 - Discrimination
- Browse content in J8 - Labor Standards: National and International
- J80 - General
- J81 - Working Conditions
- J83 - Workers' Rights
- Browse content in K - Law and Economics
- Browse content in K0 - General
- K00 - General
- Browse content in K2 - Regulation and Business Law
- K23 - Regulated Industries and Administrative Law
- Browse content in K3 - Other Substantive Areas of Law
- K35 - Personal Bankruptcy Law
- K37 - Immigration Law
- Browse content in K4 - Legal Procedure, the Legal System, and Illegal Behavior
- K41 - Litigation Process
- K42 - Illegal Behavior and the Enforcement of Law
- K49 - Other
- Browse content in L - Industrial Organization
- Browse content in L0 - General
- L00 - General
- Browse content in L1 - Market Structure, Firm Strategy, and Market Performance
- L10 - General
- L11 - Production, Pricing, and Market Structure; Size Distribution of Firms
- L13 - Oligopoly and Other Imperfect Markets
- L14 - Transactional Relationships; Contracts and Reputation; Networks
- L16 - Industrial Organization and Macroeconomics: Industrial Structure and Structural Change; Industrial Price Indices
- Browse content in L2 - Firm Objectives, Organization, and Behavior
- L20 - General
- L21 - Business Objectives of the Firm
- L22 - Firm Organization and Market Structure
- L23 - Organization of Production
- L25 - Firm Performance: Size, Diversification, and Scope
- L26 - Entrepreneurship
- Browse content in L3 - Nonprofit Organizations and Public Enterprise
- L30 - General
- L31 - Nonprofit Institutions; NGOs; Social Entrepreneurship
- L38 - Public Policy
- Browse content in L5 - Regulation and Industrial Policy
- L51 - Economics of Regulation
- L52 - Industrial Policy; Sectoral Planning Methods
- L53 - Enterprise Policy
- Browse content in L6 - Industry Studies: Manufacturing
- L60 - General
- L62 - Automobiles; Other Transportation Equipment; Related Parts and Equipment
- L63 - Microelectronics; Computers; Communications Equipment
- L64 - Other Machinery; Business Equipment; Armaments
- L65 - Chemicals; Rubber; Drugs; Biotechnology
- L66 - Food; Beverages; Cosmetics; Tobacco; Wine and Spirits
- L67 - Other Consumer Nondurables: Clothing, Textiles, Shoes, and Leather Goods; Household Goods; Sports Equipment
- Browse content in L7 - Industry Studies: Primary Products and Construction
- L71 - Mining, Extraction, and Refining: Hydrocarbon Fuels
- L74 - Construction
- Browse content in L8 - Industry Studies: Services
- L80 - General
- L81 - Retail and Wholesale Trade; e-Commerce
- L82 - Entertainment; Media
- L83 - Sports; Gambling; Recreation; Tourism
- L84 - Personal, Professional, and Business Services
- L85 - Real Estate Services
- L86 - Information and Internet Services; Computer Software
- Browse content in L9 - Industry Studies: Transportation and Utilities
- L90 - General
- L91 - Transportation: General
- L92 - Railroads and Other Surface Transportation
- L93 - Air Transportation
- Browse content in M - Business Administration and Business Economics; Marketing; Accounting; Personnel Economics
- Browse content in M1 - Business Administration
- M10 - General
- M13 - New Firms; Startups
- M16 - International Business Administration
- Browse content in M2 - Business Economics
- M21 - Business Economics
- Browse content in M3 - Marketing and Advertising
- M30 - General
- M31 - Marketing
- M39 - Other
- Browse content in M5 - Personnel Economics
- M51 - Firm Employment Decisions; Promotions
- M52 - Compensation and Compensation Methods and Their Effects
- Browse content in N - Economic History
- Browse content in N1 - Macroeconomics and Monetary Economics; Industrial Structure; Growth; Fluctuations
- N10 - General, International, or Comparative
- N13 - Europe: Pre-1913
- N16 - Latin America; Caribbean
- Browse content in N2 - Financial Markets and Institutions
- N20 - General, International, or Comparative
- N25 - Asia including Middle East
- Browse content in N3 - Labor and Consumers, Demography, Education, Health, Welfare, Income, Wealth, Religion, and Philanthropy
- N30 - General, International, or Comparative
- N33 - Europe: Pre-1913
- N34 - Europe: 1913-
- N37 - Africa; Oceania
- Browse content in N4 - Government, War, Law, International Relations, and Regulation
- N44 - Europe: 1913-
- Browse content in N5 - Agriculture, Natural Resources, Environment, and Extractive Industries
- N50 - General, International, or Comparative
- N51 - U.S.; Canada: Pre-1913
- N57 - Africa; Oceania
- Browse content in N6 - Manufacturing and Construction
- N61 - U.S.; Canada: Pre-1913
- N64 - Europe: 1913-
- Browse content in N7 - Transport, Trade, Energy, Technology, and Other Services
- N70 - General, International, or Comparative
- N71 - U.S.; Canada: Pre-1913
- N72 - U.S.; Canada: 1913-
- N73 - Europe: Pre-1913
- N74 - Europe: 1913-
- N77 - Africa; Oceania
- Browse content in N9 - Regional and Urban History
- N90 - General, International, or Comparative
- N92 - U.S.; Canada: 1913-
- N93 - Europe: Pre-1913
- N94 - Europe: 1913-
- N95 - Asia including Middle East
- N97 - Africa; Oceania
- Browse content in O - Economic Development, Innovation, Technological Change, and Growth
- Browse content in O1 - Economic Development
- O10 - General
- O11 - Macroeconomic Analyses of Economic Development
- O12 - Microeconomic Analyses of Economic Development
- O13 - Agriculture; Natural Resources; Energy; Environment; Other Primary Products
- O14 - Industrialization; Manufacturing and Service Industries; Choice of Technology
- O15 - Human Resources; Human Development; Income Distribution; Migration
- O16 - Financial Markets; Saving and Capital Investment; Corporate Finance and Governance
- O17 - Formal and Informal Sectors; Shadow Economy; Institutional Arrangements
- O18 - Urban, Rural, Regional, and Transportation Analysis; Housing; Infrastructure
- O19 - International Linkages to Development; Role of International Organizations
- Browse content in O2 - Development Planning and Policy
- O20 - General
- O21 - Planning Models; Planning Policy
- O24 - Trade Policy; Factor Movement Policy; Foreign Exchange Policy
- O25 - Industrial Policy
- Browse content in O3 - Innovation; Research and Development; Technological Change; Intellectual Property Rights
- O30 - General
- O31 - Innovation and Invention: Processes and Incentives
- O32 - Management of Technological Innovation and R&D
- O33 - Technological Change: Choices and Consequences; Diffusion Processes
- O34 - Intellectual Property and Intellectual Capital
- O38 - Government Policy
- Browse content in O4 - Economic Growth and Aggregate Productivity
- O40 - General
- O41 - One, Two, and Multisector Growth Models
- O43 - Institutions and Growth
- O47 - Empirical Studies of Economic Growth; Aggregate Productivity; Cross-Country Output Convergence
- Browse content in O5 - Economywide Country Studies
- O51 - U.S.; Canada
- O52 - Europe
- O53 - Asia including Middle East
- O54 - Latin America; Caribbean
- O55 - Africa
- O57 - Comparative Studies of Countries
- Browse content in P - Economic Systems
- Browse content in P1 - Capitalist Systems
- P12 - Capitalist Enterprises
- P13 - Cooperative Enterprises
- P16 - Political Economy
- P18 - Energy: Environment
- Browse content in P2 - Socialist Systems and Transitional Economies
- P20 - General
- P25 - Urban, Rural, and Regional Economics
- P26 - Political Economy; Property Rights
- Browse content in P3 - Socialist Institutions and Their Transitions
- P30 - General
- P31 - Socialist Enterprises and Their Transitions
- Browse content in P4 - Other Economic Systems
- P48 - Political Economy; Legal Institutions; Property Rights; Natural Resources; Energy; Environment; Regional Studies
- Browse content in P5 - Comparative Economic Systems
- P51 - Comparative Analysis of Economic Systems
- Browse content in Q - Agricultural and Natural Resource Economics; Environmental and Ecological Economics
- Browse content in Q0 - General
- Q00 - General
- Q01 - Sustainable Development
- Q02 - Commodity Markets
- Browse content in Q1 - Agriculture
- Q10 - General
- Q12 - Micro Analysis of Farm Firms, Farm Households, and Farm Input Markets
- Q15 - Land Ownership and Tenure; Land Reform; Land Use; Irrigation; Agriculture and Environment
- Q16 - R&D; Agricultural Technology; Biofuels; Agricultural Extension Services
- Q17 - Agriculture in International Trade
- Browse content in Q2 - Renewable Resources and Conservation
- Q20 - General
- Q28 - Government Policy
- Browse content in Q3 - Nonrenewable Resources and Conservation
- Q32 - Exhaustible Resources and Economic Development
- Q34 - Natural Resources and Domestic and International Conflicts
- Q38 - Government Policy
- Browse content in Q4 - Energy
- Q40 - General
- Q42 - Alternative Energy Sources
- Q48 - Government Policy
- Q49 - Other
- Browse content in Q5 - Environmental Economics
- Q50 - General
- Q51 - Valuation of Environmental Effects
- Q52 - Pollution Control Adoption Costs; Distributional Effects; Employment Effects
- Q53 - Air Pollution; Water Pollution; Noise; Hazardous Waste; Solid Waste; Recycling
- Q54 - Climate; Natural Disasters; Global Warming
- Q55 - Technological Innovation
- Q56 - Environment and Development; Environment and Trade; Sustainability; Environmental Accounts and Accounting; Environmental Equity; Population Growth
- Browse content in R - Urban, Rural, Regional, Real Estate, and Transportation Economics
- Browse content in R0 - General
- R00 - General
- Browse content in R1 - General Regional Economics
- R10 - General
- R11 - Regional Economic Activity: Growth, Development, Environmental Issues, and Changes
- R12 - Size and Spatial Distributions of Regional Economic Activity
- R13 - General Equilibrium and Welfare Economic Analysis of Regional Economies
- R14 - Land Use Patterns
- R15 - Econometric and Input-Output Models; Other Models
- R19 - Other
- Browse content in R2 - Household Analysis
- R20 - General
- R21 - Housing Demand
- R23 - Regional Migration; Regional Labor Markets; Population; Neighborhood Characteristics
- R28 - Government Policy
- R29 - Other
- Browse content in R3 - Real Estate Markets, Spatial Production Analysis, and Firm Location
- R30 - General
- R31 - Housing Supply and Markets
- R32 - Other Spatial Production and Pricing Analysis
- R33 - Nonagricultural and Nonresidential Real Estate Markets
- R34 - Input Demand Analysis
- R38 - Government Policy
- R39 - Other
- Browse content in R4 - Transportation Economics
- R40 - General
- R41 - Transportation: Demand, Supply, and Congestion; Travel Time; Safety and Accidents; Transportation Noise
- R42 - Government and Private Investment Analysis; Road Maintenance; Transportation Planning
- R48 - Government Pricing and Policy
- R49 - Other
- Browse content in R5 - Regional Government Analysis
- R50 - General
- R51 - Finance in Urban and Rural Economies
- R52 - Land Use and Other Regulations
- R53 - Public Facility Location Analysis; Public Investment and Capital Stock
- R58 - Regional Development Planning and Policy
- R59 - Other
- Browse content in Y - Miscellaneous Categories
- Browse content in Y8 - Related Disciplines
- Y80 - Related Disciplines
- Browse content in Z - Other Special Topics
- Browse content in Z1 - Cultural Economics; Economic Sociology; Economic Anthropology
- Z10 - General
- Z11 - Economics of the Arts and Literature
- Z12 - Religion
- Z13 - Economic Sociology; Economic Anthropology; Social and Economic Stratification
- Z19 - Other
- Advance articles
- Author Guidelines
- Submission Site
- Open Access
- Why Publish
- About Journal of Economic Geography
- Editorial Board
- Advertising and Corporate Services
- Journals Career Network
- Self-Archiving Policy
- Dispatch Dates
- Terms and Conditions
- Journals on Oxford Academic
- Books on Oxford Academic

Article Contents
1. introduction, 2. related literature, 3. context and data, 4. empirical approach, 6. discussion and conclusion, acknowledgments.
- < Previous
The urban–rural education gap: do cities indeed make us smarter? *
- Article contents
- Figures & tables
- Supplementary Data
Raoul van Maarseveen, The urban–rural education gap: do cities indeed make us smarter?, Journal of Economic Geography , Volume 21, Issue 5, September 2021, Pages 683–714, https://doi.org/10.1093/jeg/lbaa033
- Permissions Icon Permissions
Despite the large urban–rural education gap observed in most countries, little attention has been paid to whether cities actually enjoy a comparative advantage in the production of human capital. Using Dutch administrative data, this paper finds that children growing in urban regions consistently attain higher levels of human capital compared with children in rural regions, conditional on observed cognitive ability and various family characteristics. The elasticity of university attendance with respect to population density is 0.07, which is robust across a variety of specifications. Hence, the paper offers a different explanation to explain the recent success of cities.
The resurgence of the city has received widespread attention from both academics and policymakers in recent decades. Explanations typically highlight the success of cities at attracting well educated and skilled individuals from elsewhere, both by offering higher wages (see Combes et al., 2008 ) and superior consumption amenities (see Glaeser et al., 2001 ). Furthermore, cities are thought to have a comparative advantage in employing human capital in the production of goods and services, due to the sharing, matching and learning mechanisms described in Duranton and Puga (2004) and Rosenthal and Strange (2004) .
A small literature has analyzed the role of cities in the production of human capital itself. Both Glaeser and Mare (2001) and De La Roca and Puga (2017) find evidence that workers in larger cities accumulate human capital at a faster rate. However, little attention has been paid to the role of cities in human capital accumulation in the period prior to labor market entry. This is surprising, since agglomeration economies are likely to influence educational investment decisions through various channels. In this paper, I investigate whether exposure to urban environments during childhood affects the educational attainment of children and young adults.
Cross-sectional evidence reveals a sizeable rural–urban gap in educational outcomes across a wide range of countries. 1 It is unclear whether these differences are fully explained by the spatial sorting of households or whether they partially reflect an advantage of cities in educating their population. To answer this question, I make use of the particular institutional setting in one country, the Netherlands. The Dutch educational system requires students to make conscious decisions about their desired level of human capital investment both at the end of primary school and at the end of secondary school. Just before making the decisions, students participate in national tests of academic ability. These test scores are highly predictive of future academic outcomes and thus provide an excellent measure of the students’ cognitive and academic ability precisely at the moment when they make educational investment decisions. Furthermore, I observe a wide range of household characteristics, including detailed measures of parental education and household income.
The analyses reveal substantial differences in the human capital choices between children growing up in urban areas and rural areas. Conditional on family background and observed cognitive ability, a one log-point increase in population density is associated with a 1.68 percentage point increase in the likelihood that a child enrolls in an academic secondary school, from a base of 23%. Similarly, among high school students who have obtained all prerequisites to enroll for a university degree, a one log-point increase in population density is associated with a 0.8 percentage point increase in the likelihood that a child attends university, from a base of 84%. Children who grow up in more urban environments are thus significantly more likely to select into the schooling tracks that provide higher levels of human capital accumulation. Taken together, a one log-point increase in population density is associated with a 1.4 percentage point increase in the probability that a child attends university, which implies an elasticity of university attendance with respect to population density of 0.07. 2 Expressed differently, growing up in the center of Amsterdam rather than a place at the 25th percentile of the density distribution would increase the probability of attending university by 3 percentage points. Similar to Combes et al. (2008) in the case of the urban wage premium, I find an elasticity about twice as large when individual and family characteristics are not controlled for.
To assess whether differences in unobserved characteristics between urban and rural students might be responsible for differences in human capital investment decisions, I employ the methodology of Altonji et al. (2005) and Oster (2019) , which uses the selection on observed variables as guide to the selection on unobserved variables. Under the assumption of Altonji et al. (2005) and Oster (2019) that selection on unobserved characteristics is weakly less than selection on observed characteristics, omitted variable bias cannot account for the results obtained in this paper. In addition, the coefficients are similar across subgroups, not driven by functional form assumptions and robust to the inclusion of regional fixed effects. Using the historical densities of 1840 as instrument for modern densities to account for the potential endogeneity of urban settlements also leaves the results unaffected.
The findings of this paper contribute to three strands of literature. First, the paper contributes to the urban economics literature by showing that density affects human capital decisions of children. Earlier literature in this field has typically focused on the effect of density at a single decision moment ( Frenette, 2006 ; Newbold and Brown, 2015 ) or on a single outcome variable ( Gibbons and Silva, 2008 ), whereas this paper estimates the effect of population density on educational outcomes throughout the entire schooling career. In addition, the institutional setting and rich administrative data allow me to condition on a wide range of family characteristics and detailed measures of cognitive ability, which has typically remained unobserved in earlier studies. The closest related work is by Gibbons and Silva (2008) , who show that density affects high school test scores in the UK. This paper in comparison takes test scores as given, and focuses on students’ educational choices conditional on the primary and secondary school test scores. The resulting elasticity is around three times larger than the elasticity reported by Gibbons and Silva (2008) , reflecting that a substantial part of the effect of density on educational attainment operates through differences in educational choices. The finding that children in cities accumulate more human capital also well complements the work by Glaeser and Mare (2001) and De La Roca and Puga (2017) , who find that workers in cities accumulate human capital at a faster rate, and the recent work by Eckert et al. (2020) , who use a natural experiment to show that refugees who are placed in cities are more likely to start working in skill-intensive occupations.
Second, the results have implications for the long-term economic growth of regions and the persistence of regional differences. It is well known that the stocks of human capital are typically lower in rural regions. The findings of this study suggest that rural regions are in addition at a disadvantage in the expansion of their human capital stock, since children in rural areas invest less in human capital accumulation throughout their childhood, even when they have the same cognitive ability and family background as children in urban areas. While the lower human capital investment in rural regions might be efficient from the perspective of the individual, it does have significant implications for the long-term inequality between urban and rural regions. This is particularly important due to the fact that human capital is the most important predictor of economic growth in regions ( Gennaioli et al., 2013 ), 3 and hence provides a mechanism that could explain the slow observed convergence in incomes between regions within countries as noted by Gennaioli et al (2014) and others.
Finally, the paper contributes to a rapidly growing literature on the spatial (in)-equality of opportunity, following Chetty et al. (2014a ) and Chetty and Hendren (2018) in the USA, Alesina et al. (2019) in Africa and Deutscher (2020) in Australia. In this paper, I focus on one specific aspect of places, namely population density, and examine the effects on educational attainment. The results indicate that children who grow up in rural communities do not appear to enjoy or take advantage of the same educational opportunities as children in urban communities, even when they appear to have the same cognitive ability and family background as their urban peers. Hence, the findings of this paper highlight that the urban–rural differences in educational outcomes may not just be a result of spatial sorting of households, but may also reflect a lack of opportunities or awareness of opportunities for children residing in rural regions.
Finally, a limitation of this study is that it cannot attribute the overall effect of density on educational attainment to individual mechanisms. Based on the existing literature, there are three main channels through which density is likely to affect educational decisions. First, the returns to education are higher in cities as agglomeration forces mainly complement the productivity of high-skilled workers ( Baum-Snow et al., 2018 ; Autor, 2019 ), which raises the incentive to invest in human capital in urban areas. Second, the costs of obtaining education are lower in cities, both due to the smaller distance to educational institutes ( Frenette, 2006 ), as well as the possibility that the more diverse school choice in cities improves the match between students their needs and interests and the educational institutes. Third, it might be costlier for youth to acquire information about future educational possibilities in rural areas, due to the absence of university outreach programs as well as the absence of strong network linkages to higher educational institutes in rural communities ( Hoxby and Avery, 2012 ). However, the high correlations between the measures of the individual mechanisms make it difficult to attribute the overall effect of density to the individual mechanisms within the scope of this paper, and a detailed investigation along the lines of De La Roca and Puga (2017) and Dauth et al. (2018) in the case of the urban wage premium is thus left for future research.
The remainder of the paper proceeds as follows. Section 2 reviews the existing theoretical and empirical literature linking density to educational outcomes. Section 3 provides an overview of the context and data. Section 4 discusses the methodology and identification strategy. Section 5 presents the results and the various robustness analyses. Section 6 discusses the implications and concludes.
There are good reasons why children with similar capabilities might make different educational choices depending on whether they live in urban or rural communities. This section provides an overview of the theoretical and empirical support for three potential mechanisms, as well as review the empirical literature which links educational outcomes to urbanization.
2.1. Higher returns to education
A key determinant of educational choice in any model of educational investment is the expected returns to schooling. Most models of educational investment, such as the seminal Ben-Porath model, assume that students invest in education until the point where the discounted increase in future wages is equal to the opportunity costs and direct costs of obtaining education, thus providing a tight link between perceived returns to education and human capital investments. Theoretically one would expect highly skilled and educated workers to benefit most from agglomeration economies (see Berry and Glaeser, 2005 ; Behrens et al., 2014 ; Davis and Dingel, 2019 ), which matches the empirical findings that the returns to education are higher in urban environments ( Gould, 2007 ; Combes and Gobillon, 2015 ; Baum-Snow et al., 2018 ). This difference has become more pronounced over the last twenty years, during which the urban wage premium for non-college educated workers has essentially disappeared in the US ( Autor, 2019 ). Hence, in the absence of perfect mobility, one would expect the higher returns to education in cities to lead to higher educational investment by children growing up in urban places. However, a key question is whether children and young adults correctly infer the returns to education from their environment and if they respond accordingly. The way in which people form beliefs with regards to the returns to education is still largely unknown, but the experiment by Jensen (2010) suggests that these beliefs are not necessarily deeply held, and that beliefs are updated when new information is presented. Recent work by Adukia et al. (2020) provides evidence for this in the context of India. Rural villages which were connected to cities by roads experienced significant increases in educational attainment, with the effect increasing with the returns to education in newly connected cities.
2.2. Costs of schooling
A second channel through which population density can affect educational outcomes is through a reduction in the perceived costs of attending education. At the level of primary and secondary school, the higher density of schools in urban areas means that children on average will spend less time and financial resources on commuting to a school of a given level and quality. In the case of tertiary education, commuting to college or university may become simply unfeasible for children in rural areas, hence necessitating a costly move to attend further education. The empirical literature finds some supporting evidence for the importance of commuting costs on educational decisions, particularly in the case of tertiary education. Card (1993) uses distance to college as an instrument for educational attainment and finds a significant effect in the first stage. Frenette (2006) finds in Canada that children whose families live more than 80 km from the nearest university have a 40% lower probability to enroll at university compared with individuals who grow up within 40 km of a university. However, both studies include only a very limited set of control variables, and as such, it is not clear to what degree the results are driven by the spatial selection of households.
Furthermore, the higher density of educational institutions in cities may also allow for better matching between schools and students, as long as students and schools are heterogeneous in some dimensions. Burgess et al. (2019) show that the majority of students do not list the nearest secondary school as their first preference in the UK, indicating that students do not perceive schools as a homogenous good. Such school-child match specific component can be based on academic preferences of the child, such as the level of instruction (see for instance Bau, 2019 ) and the focus on certain specialization tracks, or can be based on more personal preferences of the child, such as the religious orientation of a school ( Cohen-Zada and Sander, 2008 ). Models of school choice typically assume that an outside option is available with zero utility, which depending on the context might consist of staying home, working or choosing a different level of schooling. The smaller the number of schools available, the more likely that unfavorable draws of the school-student match quality will result in the student choosing the outside option, which may reduce human capital investment in rural areas. Gibbons and Silva (2008) argue that greater school choice and competition in cites may explain the better performance of urban schools in the UK.
2.3. Information and network effects
Third, density may affect human capital decisions through the availability of information on future schooling prospects or due to network effects. Hoxby and Avery (2012) find in the USA that high-performing students who apply to non-selective colleges are disproportionally located in rural areas, as the lower density of high-performing students makes it less profitable for colleges to engage in outreach campaigns. In addition, Hoxby and Avery (2012) suggest that the lower Social-Economic Status (SES) composition of households in rural areas limits the exposure of children to alumni of various educational institutes, as well as reduce the expertise of study counselors on how to advise high-performing students. Hence, the limited information about future educational possibilities in rural areas provides a third potential channel through which density may affect educational investment.
2.4. Empirical evidence
Despite theoretical reasons to expect children and young adults in urban areas to invest more in their human capital, the empirical evidence on this subject is limited. Knight and Shi (1996) show large differences in years of educational attainment in China between urban and rural regions, although it remains unclear to what degree this is driven by household heterogeneity. Katz and Goldin (2008 , 222) instead report a negative relationship between town size and high school graduation rates in the early 20th century in the USA, which they suggest might be the result of the higher opportunity costs of education in cities. More recently, Newbold and Brown (2015) show that the likelihood that Canadian youth attend university is increasing in city size.
A key challenge in this literature has been dealing with the spatial sorting by households and unobserved heterogeneity between children. Previous work has established that households sort into cities based on parental education ( De la Roca, 2017 ; Ahlin et al., 2018 ; Bosquet and Overman, 2019 ) and cognitive skills ( Bacolod et al., 2010 ; Bütikofer and Peri, 2020 ). Separating spatial selection from area effects is particularly difficult in the case of education, since panel data on educational outcomes is typically unavailable on the individual level. As such, the methods developed to estimate the urban wage premium, which rely heavily on the inclusion of individual fixed effects, cannot easily be applied in the case of educational outcomes.
As a way to overcome the spatial sorting of households, some studies have relied on quasi-experimental variation to quantify the effect of exposure to neighborhood on educational outcomes. Chetty et al. (2016) use the Moving-to-Opportunity program to study neighborhood effects, which provided a randomly selected group of families the opportunity to move to a better neighborhood with less poverty and higher incomes. They find that children who moved to better neighborhoods before the age of 13 years had significantly higher schooling outcomes compared with those who did not receive the opportunity to move. However, such experiments are typically difficult to scale beyond the individual city. In a more general approach, Chetty and Hendren (2018) use the difference in the age of children when they move between regions to identify neighborhood effects. They find a negative relationship between population density and upward mobility as measured by the income at age 26 years, although it remains unclear to what extent differences in human capital accumulation play a role.
In this paper, I utilize the Dutch context to investigate the relationship between population density and educational outcomes, which is particularly well suited for this question. Tracking in the Dutch educational system starts at the age of 11–12 years, which means that meaningful decisions on human capital investment are taken from an early age onwards. Furthermore, students participate in high-stakes tests of academic ability shortly before making their key educational decisions. Hence, I observe detailed measures of cognitive ability precisely when students make educational decisions, which remains typically unobserved in most other settings. One concern with the Netherlands is that it is relatively urbanized by international standards. However, earlier studies in the Netherlands on the urban wage premium found results in line with other countries ( Groot et al., 2014 ; Verstraten et al., 2019 ). 4
3.1. Context
Figure 1 provides an overview of the Dutch school system, including the flows between school types. 5 Compulsory education starts at the age 6 years, when all students enroll in primary school. The primary school education lasts for 6 years, at the end of which students participate in a national test measuring their ability in reading comprehension, mathematics and vocabulary, as well as their reasoning and studying ability. 6 Tracking begins at the end of primary school when students can enroll in three different levels of secondary school: upper, middle and lower secondary school. The three levels differ both in length of study, the difficulty of the material and the access to tertiary education which a degree grants. Students are free to apply to any of the three levels of secondary school after finishing primary school, but secondary schools can choose whether to accept students. During the admission decision, secondary schools primarily rely on the scores on the standardized test at the end of the primary school, as well as the recommendation of the primary school teacher. In the years 2003–2015, the score on the national standardized test was considered the leading admission criteria for secondary schools. 7

Tracking through the Dutch education system for the 1996 cohort. The figure is based on the cohort born in 1996. The secondary school enrollment percentages reflects enrollment 4 years after completing primary school. The tertiary education percentages reflect enrollment within 3 years after finishing the highest secondary school degree. There are some streams within the secondary schooling system (for instance, students dropping from upper secondary school to middle secondary school), which are not displayed here. In addition, some students continue with applied university after obtaining a vocational education degree or with university after obtaining an applied university degree. These streams are not displayed here either, as this usually happens outside of the 3-year window.
Figure 2 shows the distribution of the end of primary school test score for the cohort born in 1996 (panel a) as well as the probability that students with a given test-score enroll in an upper secondary school (panel b). The test score is highly predictive of whether a student enrolls in upper secondary school. Students who score less than 535 (which is about the median test score) are highly unlikely to be enrolled in an upper secondary school 4 years later, whereas among the group of students who obtain the maximum score of 550, 8 95% enrolls at an upper secondary school. Panel c in Figure 2 shows how these probabilities differ between urban and rural regions, providing a first indication of the differences in educational decisions between urban and rural regions.

Frequency and probability of enrolling in upper secondary school by end-of-primary school test score. ( a ) Displays the frequency for each of the 50 potential scores (501–550) that students could receive at the end-of-primary school test for the cohort born in 1996. ( b ) Displays the percentage of students that are enrolled at an upper secondary school 4 years after taking the test by the end-of-primary school test score. ( c ) Splits the sample between those who grew up in a place with less than the median density (rural) and those who grew up in a place with above-median density (urban). The green line shows the difference in upper secondary school enrollment between urban and rural places in percentage points.
Once students enroll in a secondary school, they continue for another 4–6 years depending on the selected type of secondary school. At the end of secondary school, all students take a national examination which determines if they are granted a degree. This end of secondary school test is specific for their level of secondary school. 9 Once students obtain a secondary school degree, they continue to tertiary education. 10 The tracking system is designed such that certain levels of secondary school feed into a specific level of tertiary education. As can be seen from Figure 1 , 84% of the students who obtain a degree from an upper secondary school are registered at a university 3 years after graduation. Similarly, 88% of the students who obtain a degree from a middle secondary school and 98% of the students completing the lower secondary school are, respectively, registered at an applied university 11 and a vocational education 3 years after graduation. 12 Hence, the choice that students make at the end of primary school matters greatly for their future educational prospects.
Tertiary education in the Netherlands differs from many other European countries in the sense that few university degrees have a binding constraint on the number of students they accept. 13 Students who have obtained the relevant qualifications can typically register for their study of choice at the university of their choice, without additional entry conditions. Tuition fees are around 1800 euros per year for universities and applied universities and around 1000 for vocational education, with governmental student loans available if needed. Primary schools and secondary schools are free of tuition. Educational institutes at all levels are financed directly by the national government, with the financing based on the number and type of students enrolled.
The data used in the analysis are primarily for the cohorts born between 1994 and 1998. 14 I restrict the sample to individuals born in the Netherlands for whom both parents can be identified. For these individuals, I observe the place of residence between the ages 1 and 20 years, the enrollment status in all types of secondary and tertiary education and the results on the national tests at the end of primary school 15 and secondary school. In addition, a large number of family characteristics are available, including parental income, education, country of birth and year of birth. The summary statistics for all variables are provided in Table 1 .
Summary statistics for individuals born in the Netherlands between the years 1994 and 1998
Note : The table shows the summary statistics for the individuals born between 1994 and 1998 in the Netherlands. Due to the privacy-sensitive nature of the microdata, it is not possible to report minima or maxima, hence the 1st and 99th percentiles values are displayed instead. As explained in the main text, education is not available for all parents. Secondary school enrollment is measured 4 years after completing primary school. The GPA and specialization track of the upper secondary school degree are only observed for students who graduated from upper secondary school. Tertiary education enrollment is based on cohorts born in 1994–1996, as for the cohorts born in 1997/1998 not enough time has passed for all students to measure. Tertiary education enrollment is defined as the highest enrollment within 3 years of high-school graduation. The graduation rate of middle secondary school is higher than enrollment due to the fact that about 10% of the children enrolled in an upper secondary school drop down to a middle secondary school during their final three years in secondary school.
Parental income is defined similarly as in Chetty and Hendren (2018) and consists of the sum of income for both parents when the child is between ages 14 and 18 years, divided by the number of parent-years with non-missing income. Some parents have a negative income, top-coded income or have missing income for more than 5 parent-years. For the parents with negative income and parents with fewer than 5 parent-year income observations, income is set to zero and a dummy is included for both groups in each regression. A dummy is also included for top-coded incomes. The robustness checks reveal that the results are not sensitive to exclusion of these (small) groups, which together contain about 1.5% of the observations. As the coverage of the income tax data may have improved slightly over the years, I allow the coefficient of parental income as well as the coefficients of the three dummy variables to vary across cohorts.
For each parent, I observe 13 possible levels of education. 16 I do not observe education for all parents, particularly for older parents who completed schooling before some of the national education registers started. Discussions with Statistics Netherlands reveal that parents with missing education are more likely to be low educated, since highly educated parents are more likely to be captured by the various educational registers. In the baseline result, missing education is included as a 14th education type. However, the results are robust to the exclusion of this group. To opt for an as flexible approach as possible when controlling for parental education, I include dummies for each of the 196 possible parental education combinations. Similarly, parental country of birth is grouped into ‘The Netherlands’, ‘Europe’ and ‘Non-European’ for each parent, and nine dummies are included to account for each possible parental combination. Finally, the age of the oldest parent at the time of the birth is added as an additional control variable.
The next step is to construct an index of urbanization. For each individual, the zip code in which he or she resided between the years 1995 and 2018 is observed. The size of the zip codes is relatively small at 8 km 2 . I define urbanization as the log of the number of people living within 10 km of the centroid of the zip code in which an individual resides (see Appendix B for the details of the procedure). 17 The threshold of 10 km is selected based on the fact that the majority of Dutch children travel to school by bicycle, where 10 km seems a reasonable upper bound for their reach. Furthermore, the choice of a 10-km radius is in line with recent studies of agglomeration economies on wages ( De La Roca and Puga, 2017 ; Verstraten et al., 2019 ). Nonetheless, the number of individuals that live within, respectively, 5, 10 and 20 km is highly correlated, and as such, the exact distance cut-off has little influence on the results. 18 Densities are the highest in the urbanized Western part of the Netherlands and are relatively low in the North and East, as shown in Figure 3 .

Urbanization measure per zip code. The map displays the log of number of individuals within 10 km, based on the 1995 population distribution. For graphical purposes, areas with an urbanization grade less than 9 are gray (containing 230 out of the 631.890 observations in the baseline sample). Appendix B provides details on the construction of the urbanization measure.
Under the assumption that the covariance between ε i t and the urbanization measure is zero, Equations (1) and (2) will correctly identify the effect of growing up in an urban environment on educational outcomes. One concern is that parents move to places best fitted to realize the potential outcomes of their children, in which case the estimates of α 4 and β 4 would be biased. However, I find no evidence of this in the Dutch setting. For the cohorts born between 1994 and 1998, only 5.4% of the families with children between the ages 6 and 17 years make a substantial move (more than 20 km), thus limiting the degree to which families respond to the realized potential of their children. In addition, within the group of children who move, there is no correlation between the change in density and the observed academic ability of the children. 21 Nonetheless, I show that the results of Equations (1) and (2) are robust to estimating it on either the full sample or on the group of children who do not move across municipalities between the ages 6 and 17 years. 22
A second concern is that parental characteristics may vary between urban and rural places in ways that are not fully captured by the control variables. For instance, it might be that parents in urban areas are more ambitious than parents in rural areas and that this is not fully captured by the differences in wages. Separating spatial sorting from area effects has been a key challenge in the urban literature and solutions to this have typically relied heavily on individual-fixed effects ( Combes et al., 2008 ; De La Roca and Puga, 2017 ). However, such approaches are typically not possible in the case of educational outcomes due to the lack of consistent national panel data on the educational outcomes of children. 23 Section 5.2 explores this potential threat to identification, using the methodology of Altonji et al. (2005) and Oster (2019) to assess the potential importance of sorting on unobserved characteristics.
Finally, conditioning on the test scores in Equation (1) and (2) might risk overcontrolling for the effect of density on educational attainment, as test scores themselves may also be affected by density. Gibbons and Silva (2008) find that students in urban schools in the UK show more rapid improvements in test scores compared with rural students. Hence, to the degree that urban–rural differences in test scores reflect better learning outcomes in urban areas rather than heterogeneity in cognitive ability between students, the reported coefficients may somewhat underestimate the full effect of population density on educational outcomes.
5.1. Baseline results
Table 2 presents the results of Equation (1) , analyzing how the decision of children to enroll in an upper secondary school depends on population density. Column (1) shows the regression when only the urbanization measure is included, whereas column (2) adds child and family characteristics and column (3) adds dummies for the end of primary school test scores. The coefficient of urbanization is statistically significant in all three specifications at the 1% level. In economic terms, the baseline result of column (3) indicates that an increase in density by one log-point (which coincidentally is close to one standard deviation, see Table 1 ) is associated with a 1.68 percentage point increase in the probability that a child attends upper secondary school. Given that the mean percentage of children attending the upper secondary school is about 23%, this increase is substantial. When comparing the individual columns, the coefficient drops somewhat when family characteristics are added, which is largely driven by the differences in parental education between urban and rural regions. The coefficient declines further when the test scores are added, which might reflect a lower initial academic ability of children in rural regions, or alternatively might be the effect of overcontrolling to the degree that growing up in an urban environment may also affect end-of-primary school test scores. 24
Effect of urbanization on probability of enrolling in upper secondary school
Note : All results apart from column (5) are based on individuals born between 1994 and 1998. Dependent variable is whether a child is enrolled in an upper secondary school 4 years after completing primary school. Individual controls include a gender dummy and cohort dummies. Family controls include dummies for parental education combinations (168 dummies), parental nationality combinations (9 dummies), year-of-birth of oldest parent (40 dummies), family income (interacted with cohort-fixed effects), low-income dummy (interacted with cohort-fixed effects), top-coded income dummy (interacted with cohort-fixed effects) and dummy for insufficient income data (interacted with cohort-fixed effects). For a detailed explanation of the construction of the urbanization measure or the family controls, see Section 3. Column 4 excludes all children who moved municipalities during school-going age. Column 5 instead estimates the model on the cohorts born between the years 1999 and 2002. Column 6 removes all parents for whom uncertainty exists about the education level of one or both parents. Column 7 adds municipality-fixed effects for the municipality in which children live at age 11 years (430 dummies), whereas column (8) adds province-fixed effect for the province in which children live at age 11 years (12 dummies). Column (9) instruments for modern densities (based on 1995 population distribution, see Appendix B) with historical densities. All standard errors are clustered on the municipality level. Columns (1–8) are estimated by OLS, column (9) by 2SLS. First stage results of column (9) are reported in Appendix C3 (first stage F -statistic: 2349.94).
Columns 4–6 test the robustness of the results by excluding various subgroups. Column (4) excludes children who moved between municipalities during their school-going age, as their families may have sorted themselves into places based on the potential outcomes of their children. Column (5) changes the sample and instead estimates the effect on the cohorts born between 1999 and 2002, for whom information is also available. Finally, column (6) reduces the sample to the individuals for whom the exact education level of both parents is known. The coefficient is very stable across the various subgroups, suggesting that these groups are not driving the results.
One concern might be that children in urban environments enroll in classes that are too difficult for their level of ability, resulting in higher drop-out rates in urban areas. 25 Around 21% of the students who are enrolled in an upper secondary school four years after finishing primary school eventually do not obtain an upper secondary school degree, which is far from negligible. 26 To control for the possibility that differences in dropout rates between urban and rural areas are driving the results, Table 3 instead analyzes the probability that a student obtains a degree from an upper secondary school. The results are very similar to Table 2 , indicating that a misallocation of students at the end of primary school in urban communities is unlikely to drive the results. Furthermore, Table C1 shows the results when directly analyzing drop-out rates, by estimating the probability that a student obtains a degree from an upper secondary school, conditional on being enrolled in an upper secondary school 4 years after finishing primary school. The results indicate that children in urban areas are actually slightly less likely to drop out from an upper secondary school, again showing that differential dropout rates between urban and rural areas are not driving the results of Table 2 .
Effect of urbanization on probability of obtaining a degree in upper secondary school
Note : All results are based on individuals born between 1994 and 1998. Dependent variable is whether a child graduates from an upper secondary school. Individual controls include a gender-dummy and cohort-dummies. Family controls include dummies for parental education combinations (168 dummies), parental nationality combinations (nine dummies), year-of-birth of oldest parent (40 dummies), family income (interacted with cohort fixed effects), low-income dummy (interacted with cohort-fixed effects), top-coded income dummy (interacted with cohort-fixed effects) and dummy for insufficient income data (interacted with cohort-fixed effects). For a detailed explanation of the construction of the urbanization measure or the family controls, see Section 3. Column (4) removes all parents for whom uncertainty exists about the education level of one or both parents. Column (5) adds municipality-fixed effects for the municipality in which children live at age 11 years (430 dummies), whereas column (6) adds province-fixed effect for the province in which children live at age 11 years (12 dummies). Column (7) instruments for modern densities (based on 1995 population distribution, see Appendix B) with historical densities. All standard errors are clustered on the municipality level. Columns (1–7) are estimated by OLS, column (8) by 2SLS. First stage results of column (8) are reported in Appendix C3 (first stage F -statistic: 2349.94).
The second key educational decision moment is after children obtain an upper secondary school degree, when they have to decide on the level of tertiary education. Table 4 shows the estimates for Equation (2) , analyzing the effect of urbanization on the probability that a student enrolls at a university within 3 years of obtaining an upper secondary school degree, which provides access to the large majority of university–subject combinations. Column (1) only includes the urbanization measure, whereas column (2) adds student and family characteristics and column (3) adds controls for the specialization track and the Grade Point Average (GPA) on the national examination at the end of upper secondary school. 27 Since I condition on the national end of high school examination scores (instead of end of primary school test scores), it is possible to use a larger sample and to also include the cohorts born between 1989 and 1993. 28
Effect of urbanization on probability of enrolling at university/applied university, conditional on having an upper secondary school degree
Note : All results apart from column (5) are based on individuals born between 1989 and 1998. Dependent variable is whether a child attends university within 3 years of graduating from upper secondary school. Individual controls include a gender dummy and cohort dummies. Family controls include dummies for parental education combinations (168 dummies), parental nationality combinations (nine dummies), year-of-birth of oldest parent (40 dummies), family income (interacted with cohort-fixed effects), low-income dummy (interacted with cohort-fixed effects), top-coded income dummy (interacted with cohort-fixed effects) and dummy for insufficient income data (interacted with cohort-fixed effects). For a detailed explanation of the construction of the urbanization measure or the family controls, see Section 3. Column (4) excludes all children who moved municipalities during school-going age. Column (5) limits the sample to the cohorts born between 1994 and 1998, in line with the baseline sample of Table II . Column (6) removes all parents for whom uncertainty exists about the education level of one or both parents. Column (7) adds municipality-fixed effects for the municipality in which children live at age 11 years (430 dummies), whereas column (8) adds province-fixed effect for the province in which children live at age 11 years (12 dummies). Column (9) instruments for modern densities (based on 1995 population distribution, see Appendix B) with historical densities. All standard errors are clustered on the municipality level. Columns (1–8) are estimated by OLS and column (9) by 2SLS. First stage results of column (9) are reported in Appendix C3 (first stage F -statistic: 1028.11).
The results in Table 4 indicate that children who grow up in urban areas are more likely to enroll at a university, conditional on their academic ability and family characteristics. The preferred specification in column (3) indicates that children who grow up in an area where log density is one-point higher (about one standard deviation) are 0.8 percentage points more likely to enroll in university, from a base of 84%. The coefficient remains very similar when excluding children who moved between municipalities during their childhood (column 4), limiting the sample to the cohorts born between 1994 and 1998 (column 5) or limiting the sample to the children for whom no uncertainty exists over parental education (column 6).
The next question that arises is which alternatives these children select when they decide not to attend university. One alternative is to enroll at the lower rated applied universities. As Figure 1 shows, about 16% of the students who obtained an upper secondary school degree decide to enroll at an applied university, despite having the necessary qualifications to start at a university. Table C2 shows the effect of density on the probability that a student enrolls at an applied university, conditional having obtained a degree from an upper secondary school. The coefficient is significant and negative, indicating that students growing up in rural communities are more likely to instead enroll at an applied university. The coefficients are nearly identical to the coefficients in Table 4 , suggesting that diversion of rural students into the lower rated applied universities fully explains the lower enrollment rates at universities found in Table 4 .
5.2. Robustness
The results in Section 5.1 indicate that population density may play a role in the educational decisions of children. Naturally, some concerns arise over the interpretation and the robustness of this result. This section will discuss four potential threats to identification: the log-linear functional form assumption, the influence of general regional differences, the endogeneity of population density and spatial sorting on unobserved characteristics.
5.2.1. Functional form assumption
All estimations have been based on the assumption of a log-linear relationship between population density and educational outcomes, which may or may not accurately represent the true functional form. If the functional form is misspecified, it might bias the coefficients. To test this possibility, Figure 4 plots the residuals of the baseline estimations (columns 3 in Tables 2 and 4 ) without the urbanization measure against the urbanization measure itself. The residuals are averaged over 0.2 log point intervals. As Figure 4 shows, the log-linear functional form assumption seems to fit the data quite well and hence is unlikely to bias the results. In addition, the residuals reveal that the results do not depend on any specific part of the density distribution: the log-linear relationship seems to describe the actual relationship well throughout the observed density distribution.

Residuals of baseline estimates plotted against urbanization measure. Average residuals of columns (3) of Tables 2 (left) and 4 (right) without the urbanization measure plotted against the urbanization measure. Each dot corresponds to the average residual in a 0.2 log-point bin. Bins containing fewer than 500 observations are not depicted.
5.2.2. Influence of broad regional differences
Second, the map of the urbanization measure displayed in Figure 3 shows that urbanization is highest in the West and relatively low in the North and East of the country. As a result, the urbanization measure may at least partially reflect broader economic or cultural regional differences within the country, rather than urbanization per se . To test this possibility, columns (7) and (8) in Tables 2 and 4 add provincial and municipality fixed effects to the model. The coefficient of the urbanization measure in this case is identified on the variation in population density within provinces and municipalities. However, the coefficients change relatively little and remain statistically significant in all cases, even though the standard errors increase substantially as most of the variation in the urbanization measure is discarded. Hence, the results are not driven by broader differences between the various regions. 29
5.2.3. Endogeneity of Urbanization
Third, one might be concerned that population density itself is endogenous. Factors that attract population to certain areas and hence contribute to city formation may also directly affect educational outcomes. Furthermore, reverse causality can play a role if individuals migrate to areas with good schools or favorable schooling policies. Combes et al. (2008) highlight the potential of such contemporary factors to bias estimations in the case of the urban wage premium and use historical densities as an instrumental variable (IV). To alleviate concerns that the results in this paper are driven by the endogeneity of the urbanization measure, I follow Combes et al. (2008) and instrument current population densities with the population density based on the 1840 Dutch census. 30 , 31 The details of this procedure and a map of the population densities of 1840 are contained in Appendix C3. Even though it is not a perfect historical instrument, as 4 of the 13 Dutch universities predate 1840, it should substantially reduce any bias arising from the endogeneity of the urbanization measure. The first stage is significant as shown in Appendix C3, which indicates that the instrument is relevant. The results of the 2SLS estimations are provided in column (9) in Tables 2 and 4 . In all three cases, the coefficients hardly change when using the 2SLS estimator. Hence, the endogeneity of urbanization is not driving the results.
5.2.4. Sorting on unobserved variables
Finally, a key concern for many urban and regional studies is the spatial sorting of households on unobserved characteristics. Despite observing some of the key factors of importance for educational decisions, such as parental education and cognitive ability of the child, some other factors such as ambition and non-cognitive skills remain unobserved. One method to assess the importance of such sorting on unobserved variables is provided by Altonji et al. (2005) and Oster (2019) . Their procedure relies on the assumption that the sorting on observed variables is informative of sorting on unobserved variables, and in particular that the sorting on unobserved characteristics is weakly less than the sorting on observed characteristics. As such, the degree to which the coefficient of interest and the R 2 change when control variables are added can provide an indication of the potential importance of omitted variable bias.
Altonji et al. (2005) and Oster (2019) argue that sorting on unobserved variables in most cases is less severe than sorting on observable characteristics for two reasons. First of all, they argue that in the most extreme case, researchers observe a random set of variables since they typically have no direct influence over the data collection in surveys and administrative data. In such cases, the observed variables are a random subset, and as such, sorting on observed and unobserved variables should be equally important in generating bias. However, Altonji et al. (2005) argue that researchers typically direct their effort toward obtaining and including the control variables which are seen as being most likely to generate omitted variable bias. Hence, the included control variables in all likelihood contribute more to the omitted variable bias compared with the unobserved variables. For this reason, both Altonji et al. (2005) and Oster (2019) argue that a reasonable upper bound for the degree of selection on unobserved variables is the selection on observed variables. Furthermore, Altonji et al. (2005) argue that when there is a lag between observing the explanatory variables and the outcome measures, any idiosyncratic shocks that occur between observing the explanatory variables and the outcome measure cannot bias the effect of the predetermined explanatory variable. Hence, to the extent that (unobserved) idiosyncratic shocks are present, they provide an additional reason why sorting on unobserved variables may be less important than sorting on observed variables in generating omitted variable bias.
Importance of selection on unobserved variables
Note : Adjustment for unobserved variables based on Oster (2019) . The R 2 based on sibling regression comes from a regression of the model in Table 2 , column (3) on the subset of siblings, with the secondary educational choice of the sibling added as control variable. To provide a conservative inference, the standard errors of Table 2 / 4 have been used to calculate significance.
The results in this paper show evidence that population density affects the educational investment decisions of children in the context of the Netherlands. Conditional on observed ability and parental background, children who grow up in urban areas consistently choose to invest more in their education compared with children who grow up in more rural environments. This result is robust across various specifications, subgroups and spatial scope, and cannot be accounted for by the endogeneity of the urbanization measure or sorting on unobserved variables under the assumptions of Oster (2019) . Taken together, the results imply that conditional on family characteristics and academic ability, a one log-point increase in density is associated with a 1.4 percentage point increase in the probability that a child attends university. Given the mean university attendance rate of 20%, this implies an elasticity of university attendance with respect to density of 0.07. 33 Expressed differently, moving from a place at the 25th percentile of the density distribution to Amsterdam increases the probability that a child attends university by 3 percentage points.
It is surprising that the potential for agglomeration economies to affect human capital decisions of children and young adults has not received more attention in the literature, particularly given the implications for long-term regional and national growth. Children in rural communities do not seem to enjoy or take the same educational opportunities as children who grow up in urban communities, even in a country such as the Netherlands where the rural areas are relatively accessible from an international perspective. Hence, the findings suggest that differences in educational attainment between urban and rural communities observed in a wide range of countries may reflect more than just the spatial sorting of households.
Finally, one question that remains is which mechanisms drive the increased human capital accumulation of children in urban environments. Sections 2.1 and 2.2 indicated that based on existing literature, one would expect the agglomeration mechanisms to play a role in the early-life human capital decisions, either through an increase in the returns to education in cities or by increasing the availability of schooling and by reducing the commuting or moving costs to attend further education. Furthermore, there might also be a role for network effects as highlighted in Section 2.3. However, a detailed exposition of specific mechanisms in the spirit of De La Roca and Puga (2017) or Dauth et al. (2018) in the case of the urban wage premium is beyond the scope of this paper and will be an interesting avenue for future research.
The author would like to express his gratitude to the editor and two anonymous referees, as well as Niklas Bengtsson, Cristina Bratu, Matz Dahlberg, Peter Fredriksson, Edward Glaeser, Matthew Lindquist, Björn Öckert, Albert Saiz, Anna Tompsett, Santiago Truffa, Dinand Webbink and Peter Zwaneveld, and the participants to the 2019 Urban Economics Association conference and VU Amsterdam/Uppsala University seminar series for their excellent comments and suggestions. Furthermore, he would like to thank CPB Netherlands Bureau for Economic Policy Analysis for making the data available for this research, as well as their excellent suggestions. All results in this paper are based on calculations by the author using non-public microdata from Statistics Netherlands. The code used to generate the results is available upon request.
See Appendix A for the urban–rural education gap based on a wide variety of countries.
A total of 1.23 pp of the increase is due to the higher probability that a child enrolls in an upper secondary school, taking into account high school dropout rates and that not all students with an academic secondary school degree pursue university studies. A further 0.16 pp is due to the 0.8% increase in university attendance among the children who complete upper secondary school, which contains about 20% of the population. Given that a one-log point increase in density raises university enrollment by 1.4 pp and the mean university enrollment rate of 0.2, an elasticity of 0.07 is obtained.
Although Gennaioli et al. (2013) may overestimate the returns to entrepreneurial education relative to worker education due to selection into entrepreneurial activities, as pointed out by Behrens et al. (2014) .
Verstraten et al. (2019) find an elasticity of wages with respect to employment density of 0.02, whereas Groot et al. (2014) find an elasticity of 0.048 without including individual fixed effects. Both are very similar to the estimates cited by Combes and Gobillon (2015) for models with and without person fixed effects in other countries.
All data on school enrollment en educational attainment is collected by the Ministry of Education and is made available for research via Statistics Netherlands. The school enrollment data for all levels is taken from the “Onderwijsdeelnemerstab” register, the end of primary school test from the “Citotab” registry and the secondary school final examination grades from the “Examvotab” registry.
This test consists of 200 multiple choice questions (100 on reading comprehension and vocabulary, 60 on Mathematics and 40 on studying and reasoning ability). The tests are centrally graded by the Citigroup agency, which also develops the annual test.
At the end of 2015, the system was reformed to make the primary school teacher’s recommendation binding and secondary schools were no longer allowed to use the end-of-primary school test score. Politicians and teachers felt that the test had become the only selection criteria on which secondary schools evaluated children, which they argued put undue pressure on the children to perform at one specific moment in time.
The maximum score of 550 is obtained by 5.7% of the students in the 1996 cohort.
All students have a basic set of subjects (such as Dutch, English and Math A), as well as a set of subjects related to their chosen subject specialization (health, humanities, natural sciences or social sciences) in which they complete the national examination. The material covered in the examination as well as the difficulty varies depending on the type of secondary school.
Upon obtaining a middle or lower secondary school degree, students with excellent grades have the opportunity to take the final two years of the next secondary school level. When discussing degree completion or conditioning on degree, these students are always classified according to the highest degree they obtained.
The applied university is similar to the Fachhochschule in the German system. Applied university is more focused on practical skills compared with universities and part of the degree requirement typically involves various long-term internships at companies. For illustrational purposes, individuals with a university/applied university/vocational education on average had an annual income of respectively 50.00/36.000/25.000 euro between 2007 and 2009 (Statistics Netherlands, 2011) .
These figures only count students who remained in the Netherlands after completing their secondary school. Around 2% of Dutch students register for a bachelor- or master degree abroad at some point during their education (Department of Education, 2016). As robustness check in the main analysis, I exclude all students from the analysis who are not registered at any educational institution at least 2 out of the 3 years between the ages 19 and 21 years.
The most important exceptions are medicine, veterinary medicine and dentistry, as these studies typically are comparatively expensive to offer.
Some analyses can be carried out using a larger sample. For consistency, results in the main text are based on the cohorts 1994–1998 unless specified otherwise. The main tables also report results for a larger sample as robustness test whenever possible.
A small number of students is exempted from making the test due to disabilities and not all schools have agreed to make the test scores available to Statistics Netherlands. Nonetheless, I observe the test score for the large majority of the population (70%). The coefficients on the urbanization measure of columns (1) and (2) of Tables 2 and 3 are virtually unchanged (within 10% in all cases) when estimating it either on the full sample of individuals born between 1994 and 1998 or the 70% sample for whom I observe the test score. Hence, different selection between rural and urban regions into which schools agreed to make the test scores available to Statistics Netherlands is not driving the results.
These groups are kindergarten, primary school, some secondary education (low, middle or high), secondary education degree (low, middle or high), some university or applied university, applied university bachelor, university bachelor, university master or applied university master, and doctoral degree. Vocational education is not listed separately, but included in the three levels of secondary education.
To avoid potential reverse causality problems, I calculate the density based on the spatial distribution of the population in 1995. As the robustness analysis reveals, the results remain virtually unchanged when I use the spatial distribution of 1840 as instrumental variable.
For instance, the correlation between density within 10 km and the density within 5 km and the density within 20 km is 0.89 in both cases. Alternatively one could also define density based on the number of schools within a given distance as in Gibbons and Silva (2008) or only use the population density of the zip code itself. The results are similar and the conclusions of this paper do not depend on the selected measure of density.
In the empirical framework, I treat the decision for secondary school and tertiary school as two separate decisions. It would also be possible to estimate the two decisions in a joint framework, in which the decision for secondary school and tertiary education are made jointly. While a joint framework might be a better representation for the decision process of certain students, it would significantly complicate the exposition and the interpretation of the coefficients. Furthermore, the most important effect of a joint framework would be a change in the timing when a student does or not does decide to attend university, which should leave the joint effect of density on university attendance across the two decision moments unaffected.
The reason for allowing a 3-year lag is that some students opt for a gap year between completing secondary school and starting tertiary education.
Result not separately included in the paper but available from the author upon request.
A different approach would be to use the Chetty and Hendren (2018) identification strategy and use children who move between regions at different ages to generate urban exposure effects. However, within the group of movers there is a substantial decline in the average end-of-primary school test score by the age of moving, even after the age of 12 years when the test score is essentially predetermined. This differential selection into migration by the age of children hence makes it difficult to apply the Chetty and Hendren (2018) identification strategy within this context.
Such approach is difficult to implement for educational outcomes, as most countries lack repeated outcomes for children on a national level, which would allow fixed-effect estimations similar to Combes et al. (2008) with grades instead of wages. Individual-fixed effect approaches have been successfully applied within schools in the economics of education literature to identify effects of school level variables, such as teacher quality (see Chetty et al., 2014b ).
Children in urban environments indeed have higher average test scores on the end-of-primary school tests, even when conditioning on household and child characteristics. A one-log point increase in population density raises the average end-of-primary school test score by 0.2 points and increases the probability that a child has at least a score of 545 by about 1 percentage point (mean 15 pp).
Alternatively, one may also worry that children in rural areas obtain a degree from a middle secondary school, then continue with some years of applied university before switching to university. In this case, children from rural areas would simply follow a different track to end up at university. I do find some evidence for this, as children who obtain a middle secondary school degree are slightly more likely to enroll in university in the seven years after graduating from middle secondary school if they grow up in a rural area rather than an urban area. However, the increased usage of this alternative route in rural areas is far too small to compensate for the negative effects described in Tables 2 and 4. Overall, a one-log point increase in density reduces the probability that a student enrolls for university by 0.6% among the group of middle secondary school graduates (25% of the sample, see Table 1). Hence, taking this path to university into account reduces the overall effect of density on university enrollment by 0.25 * 0.6 = 0.15%. Hence, this alternative route can compensate for about 10% of the total effect of density on university enrollment of 1.4%.
The majority (62%) of these students instead obtains a degree from a middle secondary school. The drop-out figure of 21% is in line with the statistics reported for this period by Statistics Netherlands (2019) .
The GPA is based on the national examinations at the end of high school. In the final 2 years of secondary school, students can enroll in one of the possible four tracks (humanities, social sciences, biology or natural sciences). The track determines the subjects in which the students take their final examinations.
For the students born in 1994–1998, it is possible to include both the secondary school test score as well as end of primary school test score. However, adding the end of primary test score results offers little explanatory power over the high school GPA and leaves the coefficient virtually unchanged. Hence, the loss of this variable due is more than outweigh by the higher precision obtained due to the larger sample.
Kelly (2019 , 2020 ) shows that spatial autocorrelation in the dependent and independent variables means that the standard errors might be too small in many spatial regressions. Using the standard error correction proposed by Kelly (2020) increases the standard errors of column 3 in Table 2 by a factor of 1.8, which thus remains significant at the 0.1% level. The correction for Table 4 standard errors is somewhat more complicated due to the low number of observations in many zip codes, which results in very noisy estimates of the zip code fixed effect. If we assume the same spatial autocorrelation in Table 2 as in Table 4 then the estimates in Table 4 remain significant at the 5% level with a t -statistic of 2.6.
I would like to express my gratitude to Paul Verstraten (CPB Netherlands Bureau for Economics Policy Analysis) for providing the shape files containing the boundaries of the 1840 municipalities as well as the digitalized 1840 census.
A small number of zip codes cannot be matched to the densities of 1840, as they are located on land that has been reclaimed from the sea after 1840. Nonetheless, the instrument is available for 99.6% of the observations.
The reported coefficients in Table 5 are based on the general estimator as reported in Oster (2019 , Section 3.2), which allows for a more general covariance structure of the unobserved variable with the observed explanatory and outcomes variables. However, the adjusted coefficients are virtually identical when using the simplified and general estimator in this study.
1.23% of this increase is due to the increase 1.46% in probability that a child graduates from upper secondary school as seen in Table 3, taking into account that on average 84% continues with university. A further 0.15% of the increase is due to the 0.8% increase in university attendance among the children who complete upper secondary school, which contains about 20% of the population (see Table 1).
This means that I implicitly assume that the population is spread equally across zip codes. However, as zip codes are fairly small (average of 8 km 2 ) compared to the 10-km radius (314 km 2 ) radius, this assumption has little effect on the relative differences in urbanization between regions.
For some very isolated zip codes (for instance, on the islands in the North), there are no other zip codes within 10 km, and hence the population with 10 km is simply the zip codes own population.
The author wishes to acknowledge the statistical offices that provided the underlying data making this research possible: National Institute of Statistics and Censuses, Argentina; National Statistical Service, Armenia; Bangladesh Bureau of Statistics, Bangladesh; Ministry of Statistics and Analysis, Belarus; National Institute for Statistics and Economic Analysis, Benin; National Institute of Statistics, Bolivia; Central Statistics Office, Botswana; Institute of Geography and Statistics, Brazil; National Institute of Statistics and Demography, Burkina Faso; National Institute of Statistics, Cambodia; Central Bureau of Census and Population Studies, Cameroon; Statistics Canada, Canada; National Bureau of Statistics, China; National Administrative Department of Statistics, Colombia; National Institute of Statistics and Censuses, Costa Rica; National Statistics Office, Dominican Republic; National Institute of Statistics and Censuses, Ecuador; Central Agency for Public Mobilization and Statistics, Egypt; Bureau of Statistics, Fiji; National Institute of Statistics and Economic Studies, France; Federal Statistical Office, Germany; National Institute of Statistics, Guatemala; Institute of Statistics and Informatics, Haiti; Central Statistical Office, Hungary; BPS Statistics Indonesia, Indonesia; Central Statistics Office, Ireland; Central Bureau of Statistics, Israel; National Institute of Statistics, Italy; Statistical Institute, Jamaica; National Bureau of Statistics, Kenya; National Statistical Committee, Kyrgyz Republic; Statistics Bureau, Laos; Bureau of Statistics, Lesotho; Institute of Statistics and Geo-Information Systems, Liberia; National Statistical Office, Malawi; Department of Statistics, Malaysia; National Directorate of Statistics and Informatics, Mali; National Institute of Statistics, Geography, and Informatics, Mexico; National Institute of Statistics, Mozambique; Central Bureau of Statistics, Nepal; National Institute of Information Development, Nicaragua; Statistics Division, Pakistan; Central Bureau of Statistics, Palestine; Census and Statistics Directorate, Panama; National Statistical Office, Papua New Guinea; General Directorate of Statistics, Surveys, and Censuses, Paraguay; National Institute of Statistics and Informatics, Peru; Central Statistics Office, Poland; National Institute of Statistics, Portugal; National Institute of Statistics, Romania; Federal State Statistics Service, Russia; National Institute of Statistics, Rwanda; Government Statistics Department, Saint Lucia; Statistics Sierra Leone, Sierra Leone; Statistical Office of the Republic of Slovenia, Slovenia; Statistics South Africa, South Africa; National Bureau of Statistics, South Sudan; Central Bureau of Statistics, Sudan; Bureau of Statistics, Tanzania; National Statistical Office, Thailand; National Institute of Statistics, Togo; Bureau of Statistics, Uganda; State Committee of Statistics, Ukraine; Bureau of the Census, United States; National Institute of Statistics, Venezuela; General Statistics Office, Vietnam; Central Statistics Office, Zambia and National Statistics Agency, Zimbabwe.
Adukia A. , Asher S. , Novosad P. ( 2020 ) Educational investment responses to economic opportunity: evidence from Indian road construction . American Economic Journal: Applied Economics , 12 : 348 – 376 .
Google Scholar
Ahlin L. , Andersson M. , Thulin P. ( 2018 ) Human capital sorting: the “when” and “who” of the sorting of educated workers to urban regions . Journal of Regional Science , 58 : 581 – 610 .
Alesina A. , Hohmann S. , Michalopoulos S. , Papaioannou E. ( 2019 ) Intergenerational Mobility in Africa (No. w25534). National Bureau of Economic Research.
Altonji J. G. , Elder T. E. , Taber C. R. ( 2005 ) Selection on observed and unobserved variables: assessing the effectiveness of Catholic schools . Journal of Political Economy , 113 : 151 – 184 .
Autor D. ( 2019 ) Work of the past. Work of the future. American Economic Association: Papers and Proceeding.
Bacolod M. , Blum B. S. , Strange W. C. ( 2010 ) Elements of skill: traits, intelligence, education, and agglomeration . Journal of Regional Science , 50 : 245 – 280 .
Bau N. ( 2019 ) Estimating an Equilibrium Model of Horizontal Competition in Education . London: Centre for Economic Policy Research.
Baum-Snow N. , Freedman M. , Pavan R. ( 2018 ) Why has urban inequality increased? American Economic Journal: Applied Economics , 10 : 1 – 42 .
Behrens K. , Duranton G. , Robert-Nicoud F. ( 2014 ) Productive cities: sorting, selection, and agglomeration . Journal of Political Economy 122 : 507 – 53 .
Berry C. R. , Glaeser E. L. ( 2005 ) The divergence of human capital levels across cities . Papers in Regional Science , 84 : 407 – 444 .
Bosquet C. , Overman H. G. ( 2019 ) Why does birthplace matter so much? Journal of Urban Economics , 110 : 26 – 34 .
Burgess S. , Greaves E. , Vignoles A. ( 2019 ) School choice in England: evidence from national administrative data . Oxford Review of Education , 1 – 21 .
Bütikofer A Peri G. How cognitive ability and personality traits affect geographic mobility . Journal of Labor Economics, forthcoming .
Card D. ( 1993 ) Using geographic variation in college proximity to estimate the return to schooling (No. w4483). National Bureau of Economic Research.
Chetty R. , Hendren N. , Kline P. , Saez E. ( 2014 a) Where is the land of opportunity? The geography of intergenerational mobility in the United States . The Quarterly Journal of Economics , 129 : 1553 – 1623 .
Chetty R. , Friedman J. N. , Rockoff J. E. ( 2014 b) Measuring the impacts of teachers I: evaluating bias in teacher value-added estimates . American Economic Review , 104 : 2593 – 2632 .
Chetty R. , Hendren N. ( 2018 ) The impacts of neighborhoods on intergenerational mobility I: childhood exposure effects . The Quarterly Journal of Economics , 133 : 1107 – 1162 .
Chetty R. , Hendren N. , Katz L. F. ( 2016 ) The effects of exposure to better neighborhoods on children: new evidence from the Moving to Opportunity experiment . American Economic Review , 106 : 855 – 902 .
Cohen-Zada D. , Sander W. ( 2008 ) Religion, religiosity and private school choice: implications for estimating the effectiveness of private schools . Journal of Urban Economics , 64 : 85 – 100 .
Combes P. P. , Duranton G. , Gobillon L. ( 2008 ) Spatial wage disparities: sorting matters ! Journal of Urban Economics , 63 : 723 – 742 .
Combes P. P. , Gobillon L. ( 2015 ) The empirics of agglomeration economies. In Duranton G. , Henderson J. V. , Strange W. C. (eds) Handbook of Regional and Urban Economics , Vol. 5 , pp. 247 – 348 . Amsterdam : Elsevier .
Google Preview
Dauth W. , Findeisen S. , Moretti E. , Suedekum J. ( 2018 ). Matching in cities (No. w25227). National Bureau of Economic Research.
Davis D. R. , Dingel J. I. ( 2019 ). A spatial knowledge economy . American Economic Review , 109 : 153 – 70 .
Deutscher N. ( 2020 ) Place, peers, and the teenage years: long-run neighborhood effects in Australia . American Economic Journal: Applied Economics , 12 : 220 – 249 .
Duranton G. , Puga D. ( 2004 ) Micro-foundations of urban agglomeration economies. In Duranton G. , Henderson J. V. , Strange W. C. (eds) Handbook of Regional and Urban Economics , Vol. 4 , pp. 2063 – 2117 . Amsterdam : Elsevier .
Eckert F. , Hejlesen M. , Walsh C. ( 2020 ) The Return to Big City Experience: Evidence from Refugees in Denmark . NBER Working Paper.
Frenette M. ( 2006 ) Too far to go on? Distance to school and university participation . Education Economics , 14 : 31 – 58 .
Gennaioli N. , La Porta R. , Lopez-de-Silanes F. , Shleifer A. ( 2013 ) Human capital and regional development . The Quarterly Journal of Economics , 128 : 105 – 164 .
Gennaioli N. , La Porta R. , De Silanes F. L. , Shleifer A. ( 2014 ) Growth in regions . Journal of Economic Growth , 19 : 259 – 309 .
Gibbons S. , Silva O. ( 2008 ) Urban density and pupil attainment . Journal of Urban Economics , 63 : 631 – 650 .
Glaeser E. L. , Kolko J. , Saiz A. ( 2001 ) Consumer city . Journal of Economic Geography , 1 , 27 – 50 .
Glaeser E. L. , Mare D. C. ( 2001 ). Cities and skills . Journal of Labor Economics , 19 : 316 – 342 .
Gould E. D. ( 2007 ) Cities, workers, and wages: a structural analysis of the urban wage premium . The Review of Economic Studies , 74 : 477 – 506 .
Groot S. P. , de Groot H. L. , Smit M. J. ( 2014 ) Regional wage differences in the Netherlands: micro evidence on agglomeration externalities . Journal of Regional Science , 54 : 503 – 523 .
Hoxby C. M. , Avery C. ( 2012 ) The missing “one-offs”: the hidden supply of high-achieving, low income students (No. w18586). National Bureau of Economic Research.
Jensen R. ( 2010 ). The (perceived) returns to education and the demand for schooling . The Quarterly Journal of Economics , 125 : 515 – 548 .
Katz L. F. , Goldin C. D. ( 2008 ) The Race between Education and Technology . Cambridge : Harvard University Press .
Kelly M. ( 2019 ) The Standard Errors of Persistence . London : CEPR
Kelly M. ( 2020 ) Understanding Persistence . London : CEPR
Knight J. , Shi L. ( 1996 ) Educational attainment and the rural–urban divide in China . Oxford Bulletin of Economics and Statistics , 58 : 83 – 117 .
Newbold K. B. , Brown W. M. ( 2015 ) The urban–rural gap in university attendance: determinants of university participation among Canadian youth . Journal of Regional Science , 55 : 585 – 608 .
Minnesota Population Center. ( 2019 ). Integrated Public Use Microdata Series , International: Version 7.2 [dataset]. Minneapolis (MN): IPUMS ( https://doi.org/10.18128/D020.V7.2).
Oster E. ( 2019 ) Unobservable selection and coefficient stability: theory and evidence . Journal of Business & Economic Statistics , 37 : 187 – 204 .
De La Roca J. , Puga D. ( 2017 ) Learning by working in big cities . The Review of Economic Studies , 84 : 106 – 142 .
De la Roca J. ( 2017 ). Selection in initial and return migration: evidence from moves across Spanish cities . Journal of Urban Economics , 100 , 33 – 53 .
Rosenthal S. S. , Strange W. C. ( 2004 ) Evidence on the nature and sources of agglomeration economies. In Duranton G. , Henderson J. V. , Strange W. C. (eds) Handbook of Regional and Urban Economics , Vol. 4 , pp. 2119 – 2171 . Amsterdam : Elsevier .
Statistics Netherlands. ( 2011 ) Studeren loont. Inkomens van afgestudeerden in het mbo, hbo en wetenschappelijk onderwijs [Online publication].
Statistics Netherlands. ( 2019 ). Statline. https://opendata.cbs.nl/statline/#/CBS/en/
Verstraten P. , Verweij G. , Zwaneveld P. J. ( 2019 ) Complexities in the spatial scope of agglomeration economies . Journal of Regional Science , 59 : 29 – 55 .
A. The Urban–Rural Educational Gap

Urban–rural education gap based on 25-year-old males in DHS data. The figure is based on 57 countries included in the Demographic and Health Survey (DHS). Reported are the average years of schooling in urban and rural areas for 25-year-old males. The urban–rural definition follows the definition of DHS, which is based on the urban–rural definition of the country in question. Countries with fewer than 100 18-year old males for either the rural or urban region of the country have been excluded from the original sample of 75 countries.

Urban–rural school attendance gap based on 18-year-old males in the IPUMS International database (Minnesota Population Center, 2019) . The figure is based on the latest census for 55 countries included in the IPUMS International data which include an urban/rural definition in their census (which excludes most developed countries). The y -axis shows the percentage of children that indicate that they were attending school at the time of the census in urban and rural areas for 18-year-old males. The urban–rural definition is based on the definition of the statistical office of the country in question.
B. Construction of Urbanization Measure
The administrative data include information on place of residence from January 1995 onward. Hence, I start by calculating the number of individuals registered per zip code on the 5th January 1995. As the next step, I determine the centroid of each zip code using GIS software. The map of the Netherlands with all the centroids is displayed in Figure A1 . For each centroid, I determine which other zip codes lie within a 10-km radius of the centroids and add up the population of these zip codes. For zip codes that lie partially in the 10-km radius, I multiply the share of the zip code area covered by the 10-km radius with the population of that particular zip code. 34 , 35 The average zip code has 43 other zip-codes within a 10-km radius and even the first percentile of zip codes has 8 other zip-codes within a 10-km radius. The correlation between the number of individuals living within 5, 10 and 20 km is fairly high at 0.89. Finally, I take the log of the number of individuals within the 10-km radius as urbanization measure. Figure 3 in the main text shows the resulting map of the urbanization measure. In addition, Figure B2 shows a histogram of the urbanization measure.

Map of Dutch zip codes and centroids.

Histogram of urbanization measure at the age 11 years. The histogram of urbanization measure for the cohorts born between 1994 and 1998. Areas with an urbanization score below 9 (containing 230 out of the 631.815 observations in the baseline sample) not displayed here.
C. Additional Robustness Analyses
C.1. probability of upper secondary school graduation, conditional on being registered at an upper secondary school.
Effect of urbanization on probability of obtaining a degree in upper secondary school, conditional on enrollment in upper secondary school
All results are based on individuals born between 1994 and 1998. Dependent variable is whether a child graduates from an upper secondary school, conditional on being enrolled at the third grade of upper secondary school. Individual controls include a gender dummy and cohort dummies. Family controls include dummies for parental education combinations (168 dummies), parental nationality combinations (nine dummies), year-of-birth of oldest parent (40 dummies), family income (interacted with cohort-fixed effects), low-income dummy (interacted with cohort-fixed effects), top-coded income dummy (interacted with cohort-fixed effects) and dummy for insufficient income data (interacted with cohort-fixed effects). For a detailed explanation of the construction of the urbanization measure or the family controls, see Section 3. Column (4) excludes all children who moved municipalities during school-going age. Column (5) removes all parents for whom uncertainty exists about the education level of one or both parents. Column (6) adds municipality-fixed effects for the municipality in which children live at age 11 years (430 dummies), whereas column (7) adds province-fixed effect for the province in which children live at age 11 years (12 dummies). Column (8) instruments for modern densities (based on 1995 population distribution, see Appendix B) with historical densities. All standard errors are clustered on the municipality level. Columns (1–7) are estimated by OLS and column 8 by 2SLS. First stage results of column (8) are reported in Appendix C3 (first stage F -statistic: 596.93).
C.2. Applied university enrollment
Effect of urbanization on applied university attendance
Note : All results apart from column (5) are based on individuals born between 1989 and 1998. Dependent variable is whether a child attends applied university as highest education within 3 years of graduating from upper secondary school. Individual controls include a gender dummy and cohort dummies. Family controls include dummies for parental education combinations (168 dummies), parental nationality combinations (nine dummies), year-of-birth of oldest parent (40 dummies), family income (interacted with cohort-fixed effects), low-income dummy (interacted with cohort-fixed effects), top-coded income dummy (interacted with cohort-fixed effects) and dummy for insufficient income data (interacted with cohort-fixed effects). For a detailed explanation of the construction of the urbanization measure or the family controls, see Section 3. Column (4) excludes all children who moved municipalities during school-going age. Column (5) limits the sample to the cohorts born between 1994 and 1998, in line with the baseline sample of Table 2 . Column (6) removes all parents for whom uncertainty exists about the education level of one or both parents. Column (7) adds municipality-fixed effects for the municipality in which children live at age 11 years (430 dummies), whereas column (8) adds province-fixed effect for the province in which children live at age 11 years (12 dummies). Column (9) instruments for modern densities (based on 1995 population distribution, see Appendix B) with historical densities. All standard errors are clustered on the municipality level. Columns (1–8) are estimated by OLS and column (9) by 2SLS. First stage results of column (9) are reported in Appendix C3 (first stage F -statistic: 1028.11).
C.3. IV first stage
Figure C3.1 displays the population densities of Dutch municipalities in 1840. The number of municipalities (1340) is relatively large compared the modern day number of municipalities (380). The map below is used as input to construct the IV measure, namely the number of individuals in 1840 living within 10 km of all current zip codes, using the procedure outlined in Appendix B. Notice that I make the implicit assumption that the population in 1840 was spread homogenously within municipalities, as the 1840 population statistics are only available at the municipality level. Figure C3.2 shows the resulting IV-density measure for the zip codes.

Population density per municipality in 1840. When calculating the densities based on the 1840 population map, I implicitly assumed that population is spread homogenously within municipalities. Notice that the Netherlands contained a substantially larger amount of inland water compared with the contemporary Netherlands, due to the land reclamation programs in the 19th and 20th centuries.

Urbanization measure based on 1840 population distribution. The map displays the urbanization measure for each zip code (log of number of people living within 10 km), based the 1840 population distribution. See Appendix B for the construction of the measure and Figure C3.1 for the 1840 population distribution. The gray areas have an urbanization measure below 8. The gray mass in the center of the Netherlands consists of land that has been reclaimed since 1840, and hence had very few to no individuals living with 10 km in 1840, as can be also seen in Figure C3.1 .
First stage of regressing urbanization based on the 1840 densities on contemporary urbanization measure (based on 1995 densities)
Note : First stage of 2SLS regression of baseline urbanization measure (based on the 1995 population distribution) on the historical urbanization measure (based on the 1840 population distribution). The first stage of Tables 4 and C2 are virtually identical and not separately reported here, with a slightly smaller F -statistics (1028.11) due to the smaller number of observations.
Email alerts
Companion article.
- Erratum to: The urban–rural education gap: do cities indeed make us smarter?
Citing articles via
- Recommend to your Library
Affiliations
- Online ISSN 1468-2710
- Print ISSN 1468-2702
- Copyright © 2024 Oxford University Press
- About Oxford Academic
- Publish journals with us
- University press partners
- What we publish
- New features
- Open access
- Institutional account management
- Rights and permissions
- Get help with access
- Accessibility
- Advertising
- Media enquiries
- Oxford University Press
- Oxford Languages
- University of Oxford
Oxford University Press is a department of the University of Oxford. It furthers the University's objective of excellence in research, scholarship, and education by publishing worldwide
- Copyright © 2024 Oxford University Press
- Cookie settings
- Cookie policy
- Privacy policy
- Legal notice
This Feature Is Available To Subscribers Only
Sign In or Create an Account
This PDF is available to Subscribers Only
For full access to this pdf, sign in to an existing account, or purchase an annual subscription.
Thank you for visiting nature.com. You are using a browser version with limited support for CSS. To obtain the best experience, we recommend you use a more up to date browser (or turn off compatibility mode in Internet Explorer). In the meantime, to ensure continued support, we are displaying the site without styles and JavaScript.
- View all journals
- My Account Login
- Explore content
- About the journal
- Publish with us
- Sign up for alerts
- Open access
- Published: 17 April 2024
How popularising higher education affects economic growth and poverty alleviation: empirical evidence from 38 countries
- Jian Li ORCID: orcid.org/0000-0002-3228-8163 1 na1 ,
- Eryong Xue ORCID: orcid.org/0000-0002-7079-5027 2 na1 ,
- Yukai Wei ORCID: orcid.org/0000-0002-5202-7307 2 &
- Yunshu He ORCID: orcid.org/0000-0003-4814-9835 2
Humanities and Social Sciences Communications volume 11 , Article number: 520 ( 2024 ) Cite this article
506 Accesses
3 Altmetric
Metrics details
The popularisation of higher education supports UNESCO’s aim of developing inclusive and equitable quality education to achieve the fourth Sustainable Development Goal. However, the effect of popularising higher education on economic growth and poverty alleviation remains unexplored. Therefore, this study investigated the effects of higher education and adult education within populations (popularisation of higher education) on economic growth (gross domestic product; GDP) and the poverty line using panel data from 38 countries. OLS and quantile regression were performed using data for the period 1995–2021 extracted from the OECD and World Bank databases. The results showed that the population segments with higher education had a significantly positive impact on GDP growth. Moreover, an increased proportion of the population with higher education, of working age, was found to be a contributing factor to GDP growth. Popularising higher education also played a positive role during the initial stage of social and economic development. This study also highlighted that popularising higher education play a key role to influence a country’s educational development and scientific and technological innovation drives the deepening of a country’s economy. It suggested that both national and local governments worldwide should pay much attention to the popularisation degree of higher education to greatly improve the innovative ability of talents and scientific and technological innovation in higher education for both the economic growth and poverty alleviation.
Similar content being viewed by others
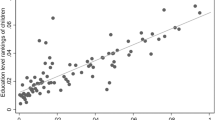
The effect of intergenerational mobility on family education investment: evidence from China
Nan Zhao, Wanqing Liao, … Zizhe Zhang
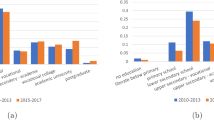
Dynamics of returns to vocational education in China: 2010–2017
Jie Chen & Francesco Pastore
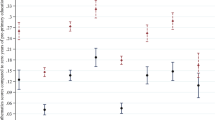
Measuring and forecasting progress in education: what about early childhood?
Linda M. Richter, Jere R. Behrman, … Hirokazu Yoshikawa
Introduction
The popularisation of higher education critically contributes to UNESCO’s efforts to realise the fourth Sustainable Development Goal of inclusive and equitable quality education (Ainscow, 2020 ; Bamberger and Kim, 2022 ).Popularisation of higher education expands the scale of higher education and its high growth rate introduces considerable challenges to the management structure of higher education, triggering a series of theoretical and practical concerns relating to the nature and function of higher education (Balestra and Ruiz, 2015 ; Brand, 2021 ). Given that education and social and economic development are mutually reinforcing, the expansion of higher education leads to an ascending spiral of development for individuals and/or economies. By contrast, a lack of education or early withdrawal from education leads to a downward spiral for them (Camilla, 2023 ). This relationship between education and development undergirds the model of poverty alleviation based on the return on education (Decancq, 2017 ). The previous studies emphasise the importance of the return on education as a multidimensional anti-poverty mechanism and thus a key factor in poverty alleviation (Fang et al., 2022 ; Chelli et al., 2022 ; Garritzmann, 2016 ). For example, return on education is the key factor enabling a transition from poverty alleviation through education to poverty alleviation through education (Gillian et al., 2021 ; Gong and Hong, 2021 ). Poverty alleviation is realised through an interlinking of these two processes and the promotion of the latter (Granata, 2022 ; Habibi and Zabardast, 2020 ). The educational resources can meet the needs of the poor mainly through the return on education at the levels of survival and life quality. In addition, the previous studies highlighted that, with a continuous expansion in the scale of higher education, its economic effect gradually appears to become marginal (Hoeller et al., 2014 ). The density of colleges and universities worldwide has increased considerably in recent years, but it is still inadequate to meet increasing demands resulting from the ongoing popularisation of higher education (Jericho, 2016 ). The increase in the number of colleges and universities has a positive effect in promoting economic development but with marginal benefits. (Julian, 2018 ).
Through reviewed the current relevant studies, it is found that there have limited studies that have simultaneously explored the effects of popularising higher education on economic growth and poverty alleviation. The previous research revealed that most studies have focused on the relations between popularisation of higher education and economic growth. However, a few empirical investigations have examined the effect of population segments with higher education and adult education (popularisation of higher education) on economic growth (GDP) and poverty reduction. Considering the scope and limitations of previous studies, it aimed to address the above research gap by investigating the effect of a population segment with high levels of higher education and adult education (popularisation of higher education) on economic growth (GDP) and the poverty line at a wide scale using panel data from 38 countries. The main research questions addressed in this study are as follows.
Q1: What is the effect of a population segment with higher education on GDP growth?
Q2: What is the effect of adult education on GDP growth?
Q3: What impact does a population segment with higher education have on reducing the proportion of those experiencing poverty?
Q4: What is the relation between an increased level of adult education and the proportion of the population experiencing poverty?
All these questions are relevant to an exploration of the effect of the population segment with higher education and adult education (popularisation of higher education) on economic growth (GDP) and the poverty line. This study is divided into several sections: the first section concentrates on examining the effect of popularising higher education on economic growth and the poverty line, the relationship between popularisation of higher education and poverty alleviation, and the relationship between popularisation of higher education and poverty alleviation. In the second section of method, to address this research gap, this study performed OLS and quantile regressions using data extracted from the OECD and World Bank databases for the period 1995–2021. An OLS regression model and a panel quantile model were used to analyse the effect of a population segment with higher education and adult education (popularisation of higher education) on economic growth (GDP) and the poverty line within 38 OECD countries. The impact of the proportion of people aged 24–64 years and 25–34 years who had completed higher education in relation to their peers on GDP and the proportion of people living in poverty in 38 OECD countries have been measured and analysed. The results and discussion have been provided at the last.
Literature review
The effect of popularising higher education on economic growth.
The population segment with higher education is regarded as an important contributor to economic growth, generating scientific knowledge and providing labour, which in turn increases human capital and productivity (Jungblut, 2017 ; Kalamova, 2020 ; Liu, 2017 ). As the scale of higher education expands, the emergence of knowledge power as a large-scale global phenomenon reflects the important role of an expanded educated labour force in the advancement of science and technology and the economy. For example, the relationship between higher education and economic development in European Union countries between 1997 and 2016 was analysed. Their findings revealed a statistically significant correlation between expanding higher education and economic growth in the selected countries. The one-way cause-and-effect relationship between education and economic development in these countries suggests that an increase in the proportion of the population enroled in higher education boosts economic performance. In addition, using a survey sample of 35 households, a retrospective study in Brazil, examined the role of educational expansion in reducing income inequality and poverty. Its findings suggest that it would take decades to reduce inequality and poverty in this country and that this outcome could only be achieved through a major expansion of the higher education sector. The growth needed to achieve this outcome would be considerable (Lamichhane et al., 2021 ). This reduction in inequality and poverty could only be achieved if optimistic assumptions about growth, matching job skills and the return on education do not fall short. In brief, education is not a panacea for reducing poverty and inequality. How three major stages of education contributed to the growth in labour productivity in 125 countries during the period 1999–2014 was also explored. They found that human capital is consistent with the educational returns of an average number of years of formal education at the levels of primary, secondary, and higher education. Their analysis showed that higher education had the greatest impact on labour productivity in the economies under study (Ledger et al., 2019 ). In addition, popularising higher education plays an important role in promoting economic growth, as the scale of higher education can guarantee the scale of human resources development by improving the quality of human resources and cultivating and distributing innovative scientific and technological talents. The scale of higher education guarantees the spread of science and technology and the popularisation of scientific and technological achievements (Mathias, 2023 ; Megyesiova and Lieskovska, 2018 ). The expanded scale of higher education worldwide has a spatial spillover effect on economic growth, which is strengthened through international cooperation in the fields of science and technology.
Popularising higher education also plays a direct role in cultivating and transporting scientific and technological talents to promote international scientific and technological cooperation (Mitic, 2018 ; Özdoğan Özbal, 2021 ; OECD, 2022 ; Pinheiro and Pillay, 2016 ). The scale of postgraduate education inhibited the total number of scientific and technological innovation achievements, indicating that there may be a trade-off between ‘quantity expansion’ and ‘quality upgrading’ of scientific and technological innovation achievements. Nevertheless, the positive effect on the number of high-tech innovation outcomes is significant, indicating that the supporting effect of graduate education on scientific and technological innovation is mainly concentrated in the high-tech fields (Pinheiro and Pillay, 2016 ; Rowe, 2019 ; Sahnoun and Abdennadher, 2022 ). The ‘talent increment’ of regional expansion and the ‘resource stock’ of graduate education have a combined promoting effect on high-tech innovation. There are differences in the effect of graduate education supporting high-tech innovation among provinces with different characteristics relating to the development of graduate education. The incremental expansion of high-quality talent is essential for enhancing the efficiency of material capital and stabilising the advantage of resource stocks. Using education statistics from OECD countries, Russia, and several other countries that participate in OECD education research, comparative and correlational analysis methods were applied to analyse how the scale of growth in young people’s participation in higher education is reflected in changes in their employment and economic activity. The results of their analysis showed that the growth in economic activity involving young graduates with a master’s degree exceeded that of college graduates after the 2009 financial crisis, and graduates fared better in the 2020 crisis, which was triggered by the COVID-19 pandemic.
The effect of popularisation of higher education on poverty alleviation
Popularisation of higher education is regarded as an essential factor contributing to poverty alleviation (Samo, 2022 ; Adams, 2013 ; Zapp, 2022 ). The higher education’s role in promoting economic growth can only be fully realised through the cultivation of talents suitable for the actual development situation of the country. Countries with food shortages, for example in Africa, also need to procure and train the right agricultural talent. Key drivers of sustainable agricultural production include access to improved technologies, sustainable growth of human, biological and natural resource capital, improvements in institutional performance and a favourable economic policy environment. Higher education graduates with the necessary ‘soft skills and business skills constitute an important pillar. Chakeredza ( 2008 ), who explored the effect of popularising higher education on poverty alleviation, suggested that the number of hungry people in Africa will continue to increase. Higher education in agriculture must be transformed, and efforts must focus on retaining faculty and on reviewing and redesigning institutional management systems, curriculum content and education delivery.
There are many reasons for poverty, with a lack of education being an important one. Insufficient quality education leads to educational poverty. Using PISA data, Agasisti et al. ( 2021 ) investigated the extent of educational poverty in European countries, considering its incidence, breadth, depth and severity. For this study, they adopted an additive multidimensional poverty measure proposed by Alkirew and Foster. Their findings indicated that between 2006 and 2015, the depth and severity of poverty decreased in most of the countries under study. Moreover, the incidence of educational poverty in many European countries was related mainly to student characteristics and school factors. The expansion of higher education has a positive effect on economic development and poverty reduction by improving work skills within the labour force. Increased enrolment in higher education encourages individuals born in families with low education levels to avail of higher education opportunities. Evidently, the expanded scale of higher education in the process of promoting economic growth has enhanced the equity effect of intergenerational social mobility. The expansion of higher education improves total factor productivity, thus promoting economic transformation and advancement globally (Samo, 2022 ; Adams, 2013 ; Zapp, 2022 ). Furthermore, the previous studies have shown that the structure of higher education talent training has a significant impact on economic development. Therefore, government departments need to make constant efforts to improve relevant systems and promote the optimisation and upgrading of the structure of higher education talent training to meet the needs of future economic development.
Theoretical underpinnings
The relationship between education and economic growth is a classic issue in the study of educational economics. For example, in Solow’s view, the growth of per capita output comes from per capita capital stock and technological progress, but capital investment has the problem of diminishing marginal returns, and the long-term sustainable development of the economy depends on technological progress (Solow, 1957 ). The emphasis on technological progress is a very important point in Solow’s growth theory. It was Schultz who systematically analyzed the contribution of education to economic growth. Influenced by the progress of economic growth theory and national accounting methods, Schulz proposed human capital theory in the process of explaining Solow residuals (Schultz, 1961 ). believes that once human capital is included in economic growth, it will solve the paradoxes and puzzles faced in economic growth research. Starting with the difference in income of different types of workers in the labour market, he found that education and health factors are the main reasons for the income difference, and further clarified that the reason for the income difference is the difference in labor productivity (Schultz, 1961 ). Schultz ( 1961 ) believes that human resources include the quantity and quality of labor, and he mainly focuses on the skills and knowledge of people who can improve labor productivity. As for how to measure human capital investment, Schulz believes that the cost of human capital can be measured in the same way as physical capital. Lucas ( 1988 ) focuses on the mechanism of human capital accumulation and why human capital does not show diminishing marginal returns like physical capital. Lucas divides the effect of human capital into internal effect and external effect. Romer ( 1990 ) internalised technological progress, revealed the relationship between human capital and technological progress, and proposed that the stock of human capital determines the economic growth rate, and it is human capital rather than population that determines economic growth. Romer starts with three hypotheses: first, technological progress is central to long-term economic growth; Second, technological progress is formed by people’s response to market incentives, and market incentives determine technological progress. Third, technology is a special kind of product, and once the cost of the initial input is produced, the technology can be reproduced indefinitely at no cost or very low cost.
In other words, higher education is more about improving students’ ability and productivity, thereby increasing students’ income, and promoting economic growth. Higher education mainly affects economic growth through two aspects: one is the same as Schulz’s improvement of individual ability, and the internal effect of human capital, which directly affects the production process (Schultz, 1961 ). Second, Lucas emphasised the external effect of human capital, and the comprehensive effect of human capital on the whole society, which has the characteristics of increasing marginal benefit (Lucas, 1988 ). It emphasises that the human capital invested in technological innovation and the existing knowledge and technology stock of the whole society jointly determine technological innovation.
Research hypotheses and analytical model
In this study, an OLS regression model and a panel quantile model were used to analyse the effect of a population segment with higher education and adult education (popularisation of higher education) on economic growth (GDP) and the poverty line within 38 OECD countries. The study’s hypotheses were as follows:
Hypothesis 1: The effect of a population segment with higher education has a positive impact on GDP growth.
Hypothesis 2: Some level of adult education has a positive impact on GDP growth.
Hypothesis 3: A population segment with higher education has a positive impact by reducing the proportion of the population experiencing poverty.
Hypothesis 4: An increase in the level of adult education has a positive impact by reducing the proportion of the population experiencing poverty.
The widely used Mankiw-Romer-Weil model was applied in this study. The overall level of development of higher education and the popularisation of higher education were considered core elements that independently promote economic development and alleviate poverty. The following model was constructed by incorporating the variable of quality higher education into the Solow model:
where Y it refers to the output of i country in t year. The independent variables Qit and P it respectively represent the scale of development and the degree of popularisation of higher education in i country in t year. The following specific model was constructed:
The independent variables were the proportion of people aged 25–64 years with higher education (A) and the proportion of people aged 25–34 years with higher education within the same age group (B). The first variable reflects the population segment that has completed higher education and can work in the corresponding age group. The second reflects the degree of popularisation of higher education. The proportion of those who have completed higher education in relation to their peers is in the normal state, which can reflect the enrolment rate for the previous process of higher education, thus indicating the degree of popularisation of higher education.
The dependent variables were GDP and the poverty line (D). GDP is a measure the overall level of a country’s economic and social development. The poverty line refers to the proportion of people living on less than US$1.25 a day as a percentage of the country’s total population or the proportion of people living in poverty. Thus, it reflects the level of equity in social development. The figure of US$2.15 is used in the World Bank’s index and is based on the purchasing power parity in 2017 (see Table 1 ).
Data sources and selection of variables
This study measured the impact of the proportion of people aged 24–64 years and 25–34 years who had completed higher education in relation to their peers on GDP and the proportion of people living in poverty in 28 OECD countries. Specifically, this study assessed the impact of the overall level of development of higher education and the degree of its popularisation (the breadth of development of higher education) on GDP (the height of development of economic and social development) and the poverty line (the breadth of development of economic and social development). Data were sourced from the OECD database and the World Bank website covering the period 1995–2021. This study selected 38 OECD countries for this study: the United States, UK, France, Germany, Italy, Canada, Ireland, the Netherlands, Belgium, Luxembourg, Austria, Switzerland, Norway, Iceland, Denmark, Sweden, Spain, Portugal, Greece, Turkey, Japan, Finland, Australia, New Zealand, Mexico, the Czech Republic, Hungary, Poland, South Korea, Slovakia, Chile, Slovenia, Estonia, Israel, Latvia, Lithuania Colombia and Costa Rica. Figure 1 shows the distribution of the 38 OECD countries. Of these countries, 20 were founding members of the OECD when it was established in 1961, while the remaining 18 subsequently became members. After 1994, OECD membership expanded rapidly. Five new members were added within three years. OECD then entered a period of accelerated development, and its operations and advancement reached an optimal stage. Therefore, this study selected data from the OECD database and the World Bank website covering the period 1995–2021 to explore the relationship between higher education and economic and social development in OECD member countries.
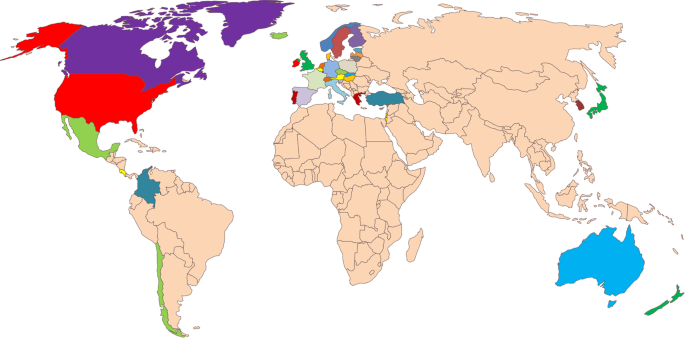
It expresses the geographical relations of the Atlantic region and simplifies the latitude and longitude lines and country symbols, highlighting the geographical distribution by highlighting OECD countries in color and other countries in apricot color.
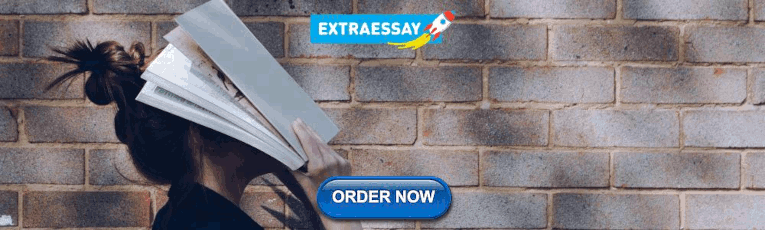
The impact of the population segment with higher education on GDP growth
This study explored the impact of the population segment with higher education on GDP, taking the proportion of people aged 25–34 years who had completed higher education (B) and the proportion of people aged 25–64 years who had completed higher education (A) as the independent variables for the OLS regression. The square value of model R was 0.097, indicating that the two independent variables could explain 9.73% of the change in GDP. The model passed an F test ( F = 46.137, p = 0.000 < 0.05), indicating that at least one of the two independent variables impacted the GDP regression coefficient (C). The following formula was used:
The final analysis revealed that the regression coefficient value of A was 1.553 and the significance level was 0.01 ( t = 7.141, p = 0.000 < 0.01). Therefore, A had a significantly positive influence on C. Accordingly, the proportion of the population aged 25–64 years who had completed higher education, that is, the overall level of development of higher education was found to have a positive impact on GDP. The influence coefficient value was 1.533, indicating that an increase in the proportion of the population with completed higher education led to an increase in GDP.
The regression coefficient value of B was −0.813 at a 0.01 level of significance ( t = −4.300, p = 0.000 < 0.01), indicating that B had a significantly negative influence on C. The proportion of the population aged 25–34 years who had completed higher education, that is, the degree of popularisation of higher education had a negative effect on GDP, and the influence coefficient value was −0.813.
The negative impact on economic and social development caused by an increase in the popularity of higher education and the proportion of young people’s higher education experience may be attributed to the excess capacity of higher education. The development of higher education should be adapted to the national context. An excess of higher education and a lack of investment lead to a rise in the social cost of education and a decline in social outputs, which hinder social and economic development. At the same time, young people aged between 25 and 34 years occupy the position of’ export’ in the education process. With the increasing popularity of higher education, the supply of talents in the labour market generated through the recruitment of former higher education exceeds the demand for graduates with higher education within recruiting organisations. Consequently, issues such as wasted educational resources and knowledge, unemployment, excessive education, excess talents, an imbalance in the structure of higher education, excessive expansion and decreasing compatibility undermine economic operations and hinder GDP growth.
In this study, the variance decomposition and Pearson coefficient based on covariance calculation were analyzed. The variable of the number of 25–34-year-old who have completed higher education as a percentage of their peers explains 50.74% of the change in GDP. The variable of the proportion of 25–64-year-old who have completed higher education explains 49.26% of the change in GDP. The variable of 25- to 34-year-olds who completed higher education as a percentage of their peers explained 45.88% of the change in poverty line. The variable of the proportion of people aged 25–64 who have completed higher education explains 54.12% of the change in GDP (See Table 2 ).
The proportion of people aged 25–34 who have completed higher education in their peers and the proportion of people aged 25–64 who have completed higher education in their peers, GDP and poverty line showed significant correlation coefficients. The correlation between the proportion of people who have completed higher education at the age of 25–34 and the proportion of people who have completed higher education at the age of 25–64 is 0.931, and shows a significance of 0.01, which indicates that there is a significant positive correlation between the proportion of people who have completed higher education at the age of 25–34 and the proportion of people who have completed higher education at the age of 25–64. The correlation between the proportion of the number of people who have completed higher education at the age of 25–34 and the GDP is 0.209, and the significance is 0.01, which indicates that there is a significant positive correlation between the number of people who have completed higher education at the age of 25–34 and the GDP. The correlation between the number of people who have completed higher education and the poverty line at the age of 25–34 is −0.365, with a significance of 0.01, indicating a significant negative correlation between the number of people who have completed higher education and the poverty line at the age of 25–34 (See Table 2 ).
White test and BP test were used in this study. The test null hypothesis is that the model has no heteroscedasticity. The table above shows that both tests reject the null hypothesis ( p < 0.05), indicating that the model does have heteroscedasticity. When there is a heteroscedasticity problem, Robust and robust standard false regression is used (See Table 3 ).
The impact of a population segment with higher education on the poverty line
This study also explored the impact of a population segment with higher education on the poverty line. Specifically, this study performed an OLS regression in which the proportion of people aged 25–34 years who had completed higher education (B) and the proportion of those aged 25–64 years who had completed higher education (A) were the independent variables. As Table 2 shows, the R squared value was 0.134. This means that variables A and B could explain 13.37% of the change in the poverty line (D). The model passed the F test ( F = 48.771, p = 0.000 < 0.05), which means that at least one variable (A or B) had an impact on the poverty line. The formula for the change in the poverty line was expressed as follows:
The final analysis revealed that the regression coefficient value of the proportion of people aged 25–64 years who had completed higher education (A) was 0.005 but with no significance ( t = 0.428, p = 0.669 > 0.05), indicating that the population segment with higher education did not have an impact on the poverty line.
The regression coefficient value of the proportion of people aged 25–34 years who had completed higher education (B) was −0.048 at a significance level of 0.01 ( t = −4.305, p = 0.000 < 0.01), which means that in relation to their peers, the proportion of people aged 25–34 years who had completed higher education had a significantly negative impact on the proportion of poor people. A higher proportion of people aged 25–34-years who had completed higher education corresponded to a higher penetration rate of higher education and a lower proportion of those living in poverty. This phenomenon can be attributed to OECD’s support for the development of higher education in various countries. When the development of higher education reaches a certain level, the reduction of the proportion of the population segment experiencing poverty will no longer be affected by a simple expansion of the scale of extended higher education and the superposition of the total number of highly educated human resources. It will be influenced more by the reasonable distribution of educational resources and educational equity within higher education and its popularisation, that is, the increase in the proportion of the school-aged population aged 25–34 years based on the increase of the previous enrolment rate (see Table 4 ).
The effect of adult education on GDP growth
For quantile regression analysis, a total of nine models (with decimal points ranging from 0.10 to 0.90 and at intervals of 0.10) were estimated in this analysis, which aimed to explore the impact of the independent variables A and B on the dependent variable, GDP (C). When the quantile value was between 0.1 and 0.3, the proportion of the population aged 25–64 years who had completed higher education (A) had no significant positive impact on GDP growth, indicating that the development of higher education did not significantly affect economic and social development in poorer OECD countries. When the quantile value was between 0.4 and 0.6, the level of development of higher education had a significantly negative impact on economic and social development. Thus, for a country that had developed over a period, the advancement of higher education required multiple inputs, such as capital, material, and human resources.
During the early stage of the development of higher education, such inputs may, however, have a negative and weakening impact on social and economic development. The added cost of education and the lag between the output of educational achievements and the input of talents puts increased pressure on economic and social development during a certain period. When the quantile value was 0.7 or higher, the improvement of the overall level of higher education had a significantly positive impact on GDP growth, indicating the realisation of the talent training outcomes of higher education. Teaching and research outcomes were thus transformed into socially productive resources and power, with talents with higher education contributing to economic and social development.
When the quantile value was 0.1, the proportion of people aged 25–34 years who had completed higher education in relation to their peers (variable B), indicating the popularisation of higher education, had no significant impact on GDP growth. Thus, in extremely backward countries, the popularisation of higher education had little effect on economic and social development. When the quantile value ranged between 0.2 and 0.6, the popularisation of higher education had a significantly positive effect on GDP growth, indicating its contribution to economic growth.
When the quantile value was 0.7, the influence of variable B on variable C was no longer significant, indicating that social development would soon face the problem of overcapacity in higher education. When it exceeded 0.7, the ratio of eligible people aged 25–34 years who had completed higher education in relation to their peers had a significantly negative impact on GDP growth, revealing that with the development of the economy, society and education, higher education had become overexpanded. Thus, the cost of investing in education exceeded the social benefits, leading to overcapacity whereby the supply of higher education talents exceeded the demand. This situation led to wasted educational resources and excessive competition of talents, hindering economic growth (See Table 5 ).
The increased level of adult education and the proportion of the population experiencing poverty
Using the same model, this study explored the influence of the independent variables, A and B, on the poverty line (dependent variable D). The proportion of the population aged 25–64 years who had completed higher education (independent variable A) had no significant influence on the proportion of the population living in poverty, indicating that popularisation of education and economic and social development have been achieved to a certain extent in OECD countries, and improvements targeting the population experiencing poverty could no longer be achieved simply by increasing the volume and quantity of higher education. When the quantile value was 0.1, the proportion of people aged 25–34 years who had completed higher education in relation to their peers (independent variable B) had no significant effect on the proportion of the population experiencing poverty (dependent variable D). Therefore, the strategy of increasing higher education enrolment and the ratio of the eligible population through the fair allocation of educational resources, and thus the popularisation of education, would not be effective for a small population segment experiencing poverty. In other words, the population segment experiencing poverty in highly developed countries is less receptive to the popularisation of higher education. When the quantile value was 0.2, the independent variable, B, had a significantly positive impact on the dependent variable D, that is, an increase in the popularity of higher education led to an increase in the population segment experiencing poverty. This phenomenon can be interpreted as reflecting the inherent disadvantages of the welfare state in the field of education. A rise in the number of eligible young people aged 25–34 years who have completed higher education reflects the development trend of higher education towards fairness and popularisation following the redistribution of higher education resources.
The fair distribution of higher education resources leads to a lack of competition in the areas of teaching and career development. To a certain extent, reducing students’ willingness and enthusiasm to work may lead to poverty caused by the failure to achieve teaching results. When the quantile value was between 0.3 and 0.4, the independent variable, B, had no significant influence on the dependent variable D. In relatively poor countries, the popularisation of higher education contributes little to reducing the degree of poverty, so it may be necessary to explore ways of alleviating poverty from the perspective of improving the overall level and expanding the scale of basic higher education. When the quantile value was 0.5 or above, the independent variable B had a significantly negative impact on the dependent variable D, indicating that for countries with a relatively high proportion of their population experiencing poverty, the following strategy would be more effective.
Considering the quantile data, this study deemed that the degree of sensitivity of countries at different stages of economic development to the level of development and popularisation of higher education could be more intuitively evaluated using a radar map (see Fig. 2 ). Countries with sub-points 0.1–0.9 were defined along a spectrum as extremely backward, backward, moderately backward, slightly backward, moderate, preliminarily developed, moderately developed, developed, and highly developed. From the perspective of economic development, increasing the proportion of young people who complete higher education and popularising higher education had an obvious positive effect in backward and medium-developed countries, whereas the effect in highly developed countries was not obvious. Overall, the sensitivity of OECD countries to the high level of education penetration was found to be higher than the level of development of higher education. From the perspective of equitable economic development, the overall level of development of higher education had no significant impact on the poverty link in OECD countries, whereas OECD countries with differing economic development backgrounds and at varying stages of development evidenced relatively significant and stable sensitivity to the proportion of young and middle-aged people who completed higher education and the popularisation of higher education.
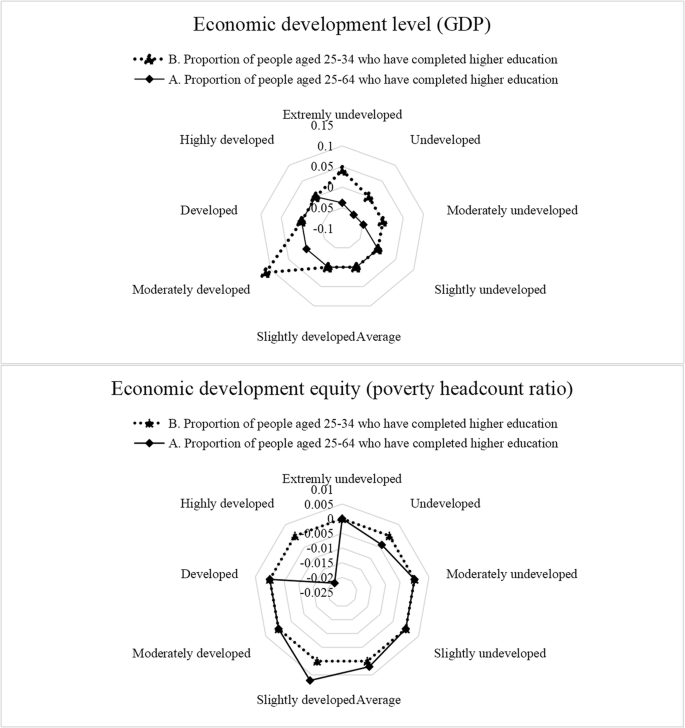
The dashed line represents the proportion of people aged 25–34 years who have completed higher education. The solid line represents the proportion of people aged 25–64 years who have completed higher education, the impact of the overall level of higher education.
Our findings indicated that population segments with higher education had a significantly positive impact on GDP growth in 38 OECD countries. An increase in the proportion of the population segment of working age who completed higher education was found to contribute to GDP growth. Moreover, an improvement in the popularity of higher education played a positive role during the initial stage of economic and social development.
At the same time, oversupply and overcapacity may result from a continuous improvement of higher education. A very large number of young people who have completed higher education can lead to excessive competition and wasted academic qualifications (Mathias, 2023 ; Megyesiova and Lieskovska, 2018 ). In turn, higher education knowledge unemployment, overinvestment, a structural imbalance, disorderly expansion and wasted resources can occur, which have detrimental impacts on economic operations.
Some studies have shown that strengthening the quality of higher education helps to improve cognitive abilities within the labour force, thereby enhancing the growth of the knowledge economy (Ainscow, 2020 ; Bamberger and Kim, 2022 ). Other studies have reported regional heterogeneity relating to the marginal effect of improving the quality of higher education on economic growth. Some scholars have analysed the influence of the quality of higher education on economic development from the perspective of human capital investment theory. Their findings indicate that the quality of higher education determines the composition and growth trend of social human capital. Because of differences in the degrees of development of different economies, the quality of higher education has a phased influence on economic growth (Balestra and Ruiz, 2015 ; Brand, 2021 ). Case studies of African developing countries by international scholars have revealed that quality factors are key to realising the economic development function of higher education. From the perspectives of both efficient financial investments by states in education poverty alleviation and the effects of economic, time and emotional investments of poor families and individuals in education poverty alleviation, it is necessary to take the return on education into consideration. Moreover, it is important to respond to reasonable concerns regarding the return on education for poor people and to strengthen their cognitive capacities to rationalise as well as their expectations regarding returns on education (Li et al., 2023 ). In this way, the intention to participate and behaviour of anti-poverty education will be generated, and the strategic upgrading of poverty alleviation combined with the promotion of aspirations and cognitive capacities will be emphasised.
Implications
Our use of panel data from 38 countries to deepen understanding of the effect of popularising higher education on economic growth and poverty reduction also has practical implications. The economic, social, and higher education undertakings in OECD countries evidence a certain level of development. The population segment with higher education has no significant impact on reducing the proportion of the population segment experiencing poverty. Simply increasing the proportion of people who complete higher education and expanding the scale of higher education will not effectively reduce poverty (Li and Xue, 2021 ). Providing more educational opportunities to poor people through the slanting of educational resources can help to reduce the proportion of poor people (Ainscow, 2020 ; Bamberger and Kim, 2022 ). For example, popularising higher education plays a key role to influence a country’s development level and scientific and technological innovation drives the deepening of a country’s economy (Bamberger and Kim, 2022 ). Technological progress is the core of economic growth, scientific and technological innovation brings technological change and development in all aspects, human capital promotes economic growth, and higher education trains talents and improves the capital attribute of human (Camilla, 2023 ). For endogenous economic growth theory, the economy does not rely on external forces to achieve sustained growth, and endogenous technological progress determines sustained economic growth. Popularising higher education worldwide brings the accumulation of human capital, improves the quality of workers, and scientific and technological innovation makes technological progress and high-quality economic development, practically. Human capital accumulation is also the process of continuous input of labour force, which covers the accumulation of human capital by labour force factors in formal education, training, and other learning processes. From the perspective of human capital, popularising higher education is the most direct and efficient way to promote the accumulation of human capital and improve the quality of labour force (Balestra and Ruiz, 2015 ; Brand, 2021 ). The popularisation degree of higher education is one of the important indicators to measure the development level of a country’s economic, and it is also the common trend of the development of higher education in all countries after World War II. In this transitional era, how to continue the achievements of higher education in the popular era and solve the existing problems as soon as possible is the heavy responsibility of our times. Therefore, at the initial stage of popularisation of higher education, it is necessary to re-examine the process of higher education popularisation globally and explore the internal logics between the popularisation of higher education and Sustainable Development Goal of inclusive and equitable quality education (Ainscow, 2020 ; Bamberger and Kim, 2022 ).
For policy suggestions, this study suggests that both national and local governments worldwide should pay much attention to the popularisation degree of higher education to greatly improve the innovative ability of talents and scientific and technological innovation in higher education. For example, they could promote scientific and technological innovation in an organised manner to serve national and regional economic and social development. Faced with the current situation in which global higher education has entered a stage of popularisation and new challenges and problems in serving regional economic and social development, national governments should continue to optimise the distribution and structure of higher education resources to support different regions, focusing on the major strategy of enhancing national competitiveness, serving economic and social development, and promoting common prosperity.
Contributions
This study novelty contributes on examining how popularising higher education affects economic growth and poverty alleviation, conceptually, methodologically, and practically. For instance, this study focuses on epitomising the conceptual and analytical model to explore the effects of higher education and adult education within populations (popularisation of higher education) on economic growth (gross domestic product; GDP) and the poverty line. In addition, this study novelty combines both Mankiw-Romer-Weil model Solow model to investigate the effects of higher education and adult education within populations on economic growth and the poverty through OLS regression model and quantile model. For the practical aspect, this study practically uncovers the implicit significance of the popularisation of higher education for advocating UNESCO’s aim of developing inclusive and equitable quality education to achieve the fourth Sustainable Development Goal.
Limitations
This study had some limitations. Data could have been collected from a larger sample of OECD countries to explore the effect of population segments with higher education and adult education (popularisation of higher education) on economic growth (GDP) and the poverty line. In addition, a qualitative component could be included in future studies to uncover the cultural and historical contexts of the effect of popularising higher education on economic growth and poverty reduction at the local level. Future studies should also investigate the causal relationship between the popularisation of higher education and economic growth. Additional empirical data and advanced research methods can be used to enable a shift from correlation to causality.
In conclusion, this study examined the effect of the population segment with higher education and adult education (popularisation of higher education) on economic growth (GDP) and the poverty line using panel data from 38 countries. The population segment with higher education was found to have a significant positive impact on promoting GDP growth. An increase in the proportion of the working-age population segment that had completed higher education was evidently conducive to GDP growth. Popularisation of higher education was also found to play a positive role in the initial stage of economic and social development.
Data availability
The data of OECD country GDP is retrieved from https://data.worldbank.org/indicator/NY.GDP.MKTP.CD?locations=1W , The data of OECD country poverty line is retrieved from https://data.worldbank.org/indicator/SI.POV.DDAY?locations=1W&start=1984&view=chart , The data of OECD country Population with tertiary education 25–34-year-old is retrieved from https://data.oecd.org/eduatt/population-with-tertiary-education.htm#indicator-chart , The data of OECD country Percentage of 25–64-year old’s who have completed higher education (%) is retrieved from https://data.oecd.org/eduatt/adult-education-level.htm#indicator-chart , The datasets generated during and/or analysed during the current study are available in Harvard Dataverse https://doi.org/10.7910/DVN/TP43QS .
Adams, R (2013). Education: poverty and inequality blamed as UK sinks in adult literacy league: OECD survey flags up big problems for England: Post-16 education blamed for failure to address issue. The Guardian . (2013, October 9). London, England
Agasisti T, Longobardi S, Prete V, Russo F (2021) The relevance of educational poverty in europe: determinants and remedies. J Policy Model 43(3):692–709. https://doi.org/10.1016/j.jpolmod.2020.03.015
Article Google Scholar
Ainscow M (2020) Promoting inclusion and equity in education: lessons from international experiences. Nord J Stud Educ Policy 6(1):7–16
Balestra C, Ruiz N (2015) Scale-invariant measurement of inequality and welfare in ordinal achievements: an application to subjective well-being and education in OECD Countries. Soc Indic Res 123(2):479–500. https://doi.org/10.1007/s11205-014-0751-2
Bamberger, A, & Kim, MJ (2022) The OECD’s influence on national higher education policies: internationalisation in Israel and South Korea. Comp Educ 18. https://doi.org/10.1080/03050068.2022.2147635
Brand M (2021) The OECD poverty rate: lessons from the russian case. Glob Soc Policy 21(1):144–147
Camilla S. (2023) The design of upper secondary education across OECD countries: Managing choice, coherence and specialisation. OECD Education Working Papers. https://doi.org/10.1787/158101f0-en
Chakeredza S, Temu A, Saka J, Munthali D, Muir-Leresche K, Akinnifesi F, Ajayi O, Sileshi G (2008) Tailoring Tertiary Agricultural Education for Sustainable Development in Sub-Saharan Africa: Opportunities and Challenges. J Sci Res Essay 3(8):326–332
Google Scholar
Chelli F, Ciommi M, Mariani F, Polinesi G, Recchioni MC, Lamonica GR, Salvati L (2022) A story of strengths and weaknesses in tertiary education: evaluating “Mobility” and “Opportunities” in OECD countries with composite indicators. Sustainability 14(24):16463. https://doi.org/10.3390/su142416463
Decancq, K (2017) Measuring multidimensional inequality in the OECD member countries with a distribution-sensitive better life index. Soc Indic Res 131(3):1057–1086. https://doi.org/10.1007/s11205-016-1289-2
Fang W, Farooq U, Liu Z, Lan J, Iram R (2022) Measuring energy efficiency financing: a way forward for reducing energy poverty through financial inclusion in OECD. Environ Sci Pollut Res 29(47):71923. https://doi.org/10.1007/s11356-022-20139-8
Garritzmann, JL (2016) The political economy of higher education finance: the politics of tuition fees and subsidies in OECD Countries, 1945–2015. Garritzmann. Germany, Berlin: Springer International Publishing
Gillian G, Lisa T, & Thomas W (2021) How are higher education systems in OECD countries resourced?: Evidence from an OECD Policy Survey.OCDE. https://doi.org/10.1787/0ac1fbad-en
Gong HJ, Hong JE (2021) Does postsecondary education attainment matter in community service engagement? Evidence from Across 18 OECD Countries. Educ Sci 11(3):1–17. https://doi.org/10.3390/educsci11030096
Article MathSciNet Google Scholar
Granata, M (2022) The OECD and technical education in post-war Mediterranean Europe. Labor History. https://doi.org/10.1080/0023656X.2022.2057459
Habibi, F & Zabardast, MA (2020) Digitalization, education and economic growth: a comparative analysis of Middle East and OECD countries. Technol Soc 63. https://doi.org/10.1016/j.techsoc.2020.101370
Hoeller P, Joumard I, Koske I (2014) Reducing income inequality while boosting economic growth: can it be done? Evidence from OECD Countries. Singap Econ Rev 59(1):1–22
Jericho, G (2016) Australia didn’t have a ‘great recession’? Tell that to young people; an OECD report has found a rise in youth not in employment, education or training (Neets) since 2008. While historically the rate isn’t high, benefits are so low that youth are now more likely to be living in poverty. Guardian. London, England. https://link.gale.com/apps/doc/A463552813/AONE?u=unimelb&sid=bookmark-AONE&xid=701996a9
Julian LG (2018) The political economy of higher education finance. the politics of tuition fees and subsidies in OECD Countries, 1945–2015. Czech Sociol Rev 54(3):479–482
Jungblut J (2017) The political economy of higher education finance. The politics of tuition fees and subsidies in OECD countries, 1945–2015. Eur J High Educ 7(1):96–99. https://doi.org/10.1080/21568235.2017.1265789
Kalamova, M (2020) Resourcing higher education: challenges, choices and consequences. Higher Education Policy Team. Paris: OECD Publishing
Lamichhane, S, Egilmez, G, Gedik, R, Bhutta, MKS, & Erenay, B (2021) Benchmarking OECD countries’ sustainable development performance: a goal-specific principal component analysis approach. J Clea Prod 287. https://doi.org/10.1016/j.jclepro.2020.125040
Ledger, S, Thier, M, Bailey, L, & Pitts, C (2019) OECD’s approach to measuring global competency: powerful voices shaping education. Teachers College Record, 121(8):1–40
Li, J & Xue. E (2021) Returnee faculty responses to internationalizing “Academic Ecology” for Creating World-class Universities in China’ Elite Universities. Higher Education. 81(5), 1063–1078
Li, J, & Xue. E, Liu, C, Li, X (2023) Integrated macro and micro analyses of student burden reduction policies in China: call for a collaborative “family–school–society” model. Humanities & Social Sciences Communications. 10, 184 (2023). https://doi.org/10.1057/s41599-023-01695-x
Liu, L (2017) Exploring the relationship between education economy and individual civic attitudes: a cross-national analysis in England, Spain, Sweden, Poland and Chinese Taipei
Lucas R (1988) On the mechanics of economic development. J. Monetary Econ 22(1):342
Mathias B (2023). The assessment of students’ creative and critical thinking skills in higher education across OECD countries: a review of policies and related practices. OECD Education Working Papers. https://doi.org/10.1787/35dbd439-en
Megyesiova, S, & Lieskovska, V (2018) Analysis of the sustainable development indicators in the OECD Countries. Sustainability 10(12). https://doi.org/10.3390/su10124554
Mitic RR (2018) The political economy of higher education finance: the politics of tuition fees and subsidies in OECD Countries, 1945-2015. Rev High Educ 41(3):484–487
OECD (2022) Education at a Glance 2022: OECD Indicators, OECD Publishing, Paris, https://doi.org/10.1787/3197152b-en
Özdoğan Özbal E (2021) Dynamic effects of higher education expenditures on human capital and economic growth: an evaluation of OECD countries. Policy Rev High Educ 5(2):174–196. https://doi.org/10.1080/23322969.2021.1893125
Pinheiro R, Pillay P (2016) Higher education and economic development in the OECD: policy lessons for other countries and regions. J High Educ Policy Manag 38(2):150
Romer M (1990) Endogenous technological change. J Political Econ 98(5):S71–S102
Rowe E (2019) Capitalism without capital: the intangible economy of education reform. Discourse 40(2):271
Sahnoun M, Abdennadher C (2022) Returns to investment in education in the OECD Countries: does governance quality matter? J Knowldege Econ 13(3):1819–1842. https://doi.org/10.1007/s13132-021-00783-0
Samo V. (2022). A snapshot of equity and inclusion in OECD education systems: findings from the Strength through Diversity Policy Survey. OECD Eduction Working Papers. https://doi.org/10.1787/801dd29b-en
Schultz (1961) Investment in human capital. Am Econ Rev 51(1):1–17
Solow RM (1957) Technical change and the aggregate production function. Rev Econ Stat 39(3):312–320
Zapp M (2022) Revisiting the global knowledge economy: the worldwide expansion of research and development personnel, 1980–2015. Minerva 60(2):181–208. https://doi.org/10.1007/s11024-021-09455-4
Article PubMed PubMed Central Google Scholar
Download references
Acknowledgements
This study is funded by 2021 National Social Science Foundation of Higher Education Ideological and Political Course research (Key project) Ideological and Political Education System Construction System Mechanism Research in New Era (No.: 21VSZ004).
Author information
These authors contributed equally: Jian Li, Eryong Xue.
Authors and Affiliations
Institute of International and Comparative Education, Beijing Normal University, Beijing, 100875, China
China Institute of Education Policy, Faculty of Education, Beijing Normal University, Beijing, 100875, China
Eryong Xue, Yukai Wei & Yunshu He
You can also search for this author in PubMed Google Scholar
Substantial contributions to the conception or design of the work: Jian Li and Eryong Xue; the acquisition, analysis, or interpretation of data for the work: Jian Li and Eryong Xue; drafting the work or revising it critically for important intellectual content: Eryong Xue, Jian Li, Yukai Wei, and Yunshu He; final approval of the version to be published: Eryong Xue and Jian Li.
Corresponding authors
Correspondence to Jian Li or Eryong Xue .
Ethics declarations
Competing interests.
The authors declare no competing interests.
Ethical approval
Ethical approval was not required as the study did not involve human participants. It was not required as this article used published data.
Informed consent
This article does not contain any studies with human participants performed by any of the authors.
Additional information
Publisher’s note Springer Nature remains neutral with regard to jurisdictional claims in published maps and institutional affiliations.
Rights and permissions
Open Access This article is licensed under a Creative Commons Attribution 4.0 International License, which permits use, sharing, adaptation, distribution and reproduction in any medium or format, as long as you give appropriate credit to the original author(s) and the source, provide a link to the Creative Commons licence, and indicate if changes were made. The images or other third party material in this article are included in the article’s Creative Commons licence, unless indicated otherwise in a credit line to the material. If material is not included in the article’s Creative Commons licence and your intended use is not permitted by statutory regulation or exceeds the permitted use, you will need to obtain permission directly from the copyright holder. To view a copy of this licence, visit http://creativecommons.org/licenses/by/4.0/ .
Reprints and permissions
About this article
Cite this article.
Li, J., Xue, E., Wei, Y. et al. How popularising higher education affects economic growth and poverty alleviation: empirical evidence from 38 countries. Humanit Soc Sci Commun 11 , 520 (2024). https://doi.org/10.1057/s41599-024-03013-5
Download citation
Received : 29 June 2023
Accepted : 02 April 2024
Published : 17 April 2024
DOI : https://doi.org/10.1057/s41599-024-03013-5
Share this article
Anyone you share the following link with will be able to read this content:
Sorry, a shareable link is not currently available for this article.
Provided by the Springer Nature SharedIt content-sharing initiative
Quick links
- Explore articles by subject
- Guide to authors
- Editorial policies

Among nurses, how does education level impact professional values? A systematic review
Affiliation.
- 1 School of Nursing, College of Human and Health Services, George Mason University, Fairfax, VA, USA.
- PMID: 28657173
- DOI: 10.1111/inr.12390
Background: Professional nursing values have been acknowledged globally as the foundation of daily nursing care practice. Understanding how nurses identify, comprehend and apply their professional nursing values is an important step towards improving nursing practice and patient care quality. Research has demonstrated that nurses' professional values are cultivated during prelicensure academic education.
Aim: The aim of this systematic review was to determine how level of education affects professional nursing values of clinical practising nurses.
Methods: A systematic search of quantitative research published through December 2015 was performed in the following five electronic databases: CINAHL, Cochrane Library, MEDLINE, Web of Science and Religion and Philosophy Collection. The search was not limited to country of origin. The studies were assessed for methodological quality using established criteria.
Results: Of 1501 articles identified through the literature search, only seven studies met the inclusion criteria with the majority being of good to high quality. Most of the studies found registered nurses pursuing a bachelor of science in nursing or higher had a greater awareness and application of professional values than nurses with lower levels of academic or non-academic education. Nurses with higher education also embraced professional values as fundamental for quality nursing care practice.
Implications for nursing and health policy: Health and academic institutions should support nurses through quality continuing and higher education that reinforces professional values, thus improving the quality of patient care.
Conclusions: The level of nurses' education appears to play an important role in developing both an awareness and an integration of professional values into practice. More research is needed to discover methods that may be used to promote nurses' professional values among nurses already practising clinically.
Keywords: Educational Level; Educational Status; Ethics; Morals; Nurse; Nursing Education; Nursing Staff; Professional Values; Values.
© 2017 International Council of Nurses.
Publication types
- Systematic Review
- Attitude of Health Personnel*
- Attitude to Health
- Educational Status*
- Middle Aged
- Nursing Care / psychology*
- Nursing Staff / psychology*
- Nursing Staff / standards*
- Professional Competence*
ORIGINAL RESEARCH article
The impact of education level and economic freedom on gender inequality: panel evidence from emerging markets.
- 1 Institute of Social Sciences, Netkent Mediterranean Research and Science University, Nicosia, Cyprus
- 2 Hasan Ali Yucel Faculty of Education, Department of Educational Sciences, İstanbul University-Cerrahpaşa, İstanbul, Turkey
- 3 Department of Economics, Plekhanov Russian University of Economics, Moscow, Russia
- 4 Department of Economics, Financial University under the Government of the Russian Federation, Moscow, Russia
- 5 Management Sciences Department, Business School, German Jordanian University, Amman, Jordan
Gender equality is a critical factor for all ingredients of a healthy society and sustainable development. Therefore, measures to decrease gender inequalities in economic, social, and political life are important for the economic and social development of a society. This study analyzes the influence of education level and economic freedom on gender inequality in emerging markets over the 2000–2020 term through causality and cointegration tests. The results of the causality test uncover a bidirectional causality between education level, economic freedom, and gender inequality. In other words, there exists a mutual interaction among education level, economic freedom, and gender inequality in the short term. Furthermore, the findings of cointegration analysis indicate that education level and economic freedom have a negative impact on gender inequality in the long term, but education level is much more effective on gender inequality than economic freedom in nearly all emerging markets.
1. Introduction
The women population constitutes nearly half of the world population ( World Bank, 2023 ). Therefore, the women population also represents half of human capital, a significant determinant of economic sustainability and social development. However, men and women do not generally have equal rights and opportunities in education, health, economic and social life in many countries, but gender inequality remarkably differs among countries depending on their cultural norms, and social and economic development levels. In this context, the gender inequality index (GII) (lower GII values show lower gender inequality) of 2021 calculated by UNDP (2023) indicated that Denmark (GII: 0.013), Norway (GII: 0.016), Switzerland (GII: 0.018), Sweden (GII: 0.023), and the Netherlands (GII: 0.025) were the first five countries with the lowest gender inequality, but Yemen (GII: 0.820), Papua New Guinea (GII: 0.725), Nigeria (GII: 0.680), Afghanistan (GII: 0.678), and Central African Republic (GII: 0.672) were the last five countries with the highest gender inequality. Countries with lower gender inequality generally have lower human development when GII figures and human development levels of the countries are together considered.
Women generally have less access to economic and social resources, the labor market, and the political world and use their resources in non-economic activities such as housework and care, education, and the health of their children ( Schultz, 2002 ; Ferrant, 2015 ; Bertay et al., 2020 ). Thus, gender inequalities in education, health, social, economic, and political activities have the potential to negatively influence capital accumulation, technological development, productivity, and institutional development which are the main factors underlying economic growth and development ( Ferrant, 2015 ). Thereby, gender inequality becomes more of an issue for economic growth and development.
In this context, Wu et al. (2022) discovered a negative interaction between gender inequality and economic growth in China in the short and long term. Koengkan et al. (2022) also uncovered a negative influence of gender inequality on economic growth in Latin America and the Caribbean states. Many other researchers such as Kleven and Landais (2017) , Karoui and Feki (2018) , Bertay et al. (2020) , and Farooq et al. (2020) have discovered a negative influence of gender inequality on economic growth in different countries and country groups. Ferrant (2015) also suggested gender inequality is a significant determinant of economic and human development and found that gender inequality decreased human development by 4.6% in 109 countries mainly driven by gender inequalities in family, education and access to economic activities. Therefore, gender inequality can negatively influence social development through education and health channels. Furthermore, gender gaps in wages, labor participation, and the informal sector can positively influence income inequality ( Gonzales et al., 2015 ).
Moreover, gender equality is one of the 17 sustainable development goals (SDGs) accepted by all United Nations Member States in 2015 ( United Nations, 2023a ) and the United Nations suggest that gender equality is a crucial factor for all aspects of a healthy society, including decreasing poverty, increasing education and health protection, and the well-being of all persons ( United Nations, 2023b ). Hence, the revelation of the factors behind gender inequality would be useful to arrange the right policies to decrease gender inequality. However, researchers have generally analyzed the relationship between gender inequality and economic growth in the empirical literature, and the determinants of gender inequality have been investigated by few researchers. The studies on the determinants of gender inequality have set forth that GDP per capita , education, fertility rate, cultural norms, economic freedom, public governance, trade, globalization, and foreign direct investments are significant factors underlying gender inequality ( Baliamoune-Lutz, 2007 ; Juhn et al., 2014 ; Jayachandran, 2015 ; Sangaji et al., 2018 ; Adeosun and Owolabi, 2021 ; Kim, 2021 ; Apergis and Lynch, 2022 ).
In this research, we focus on the short-and long-term influence of economic freedom and education level on gender inequality, because both variables have the potential to affect gender inequality through multiple diverse channels. First, education is one of the dominant factors behind human capital which is a key determinant of economic growth and development. Therefore, education raises the awareness of society about the role of women in economic and social development ( Kane, 1995 ). Furthermore, decreases in gender inequality also can foster education by increasing the roles of women in social and economic life. As a result, a bilateral interaction between education and gender inequality seems possible in theoretical terms. Furthermore, economic freedom can also decrease gender inequality, because market-oriented economies generally introduce equal opportunities for everybody regardless of an individual’s gender ( Stroup, 2008 ). The improvements in gender equality can also foster economic freedom by raising the roles of women in economic, social, and political life. Similarly, a mutual interplay between economic freedom and gender inequality is also expected.
This article explores the influence of education level and economic freedom on gender inequality in a sample of 21 emerging markets, presented in Table 1 . The economic size and technological progress in the emerging markets have grown considerably and they have become the drivers of the global economy ( MSCI, 2023 ). Furthermore, the emerging markets have different country-specific characteristics and achieved different progress in gender equality as seen in Table 1 .
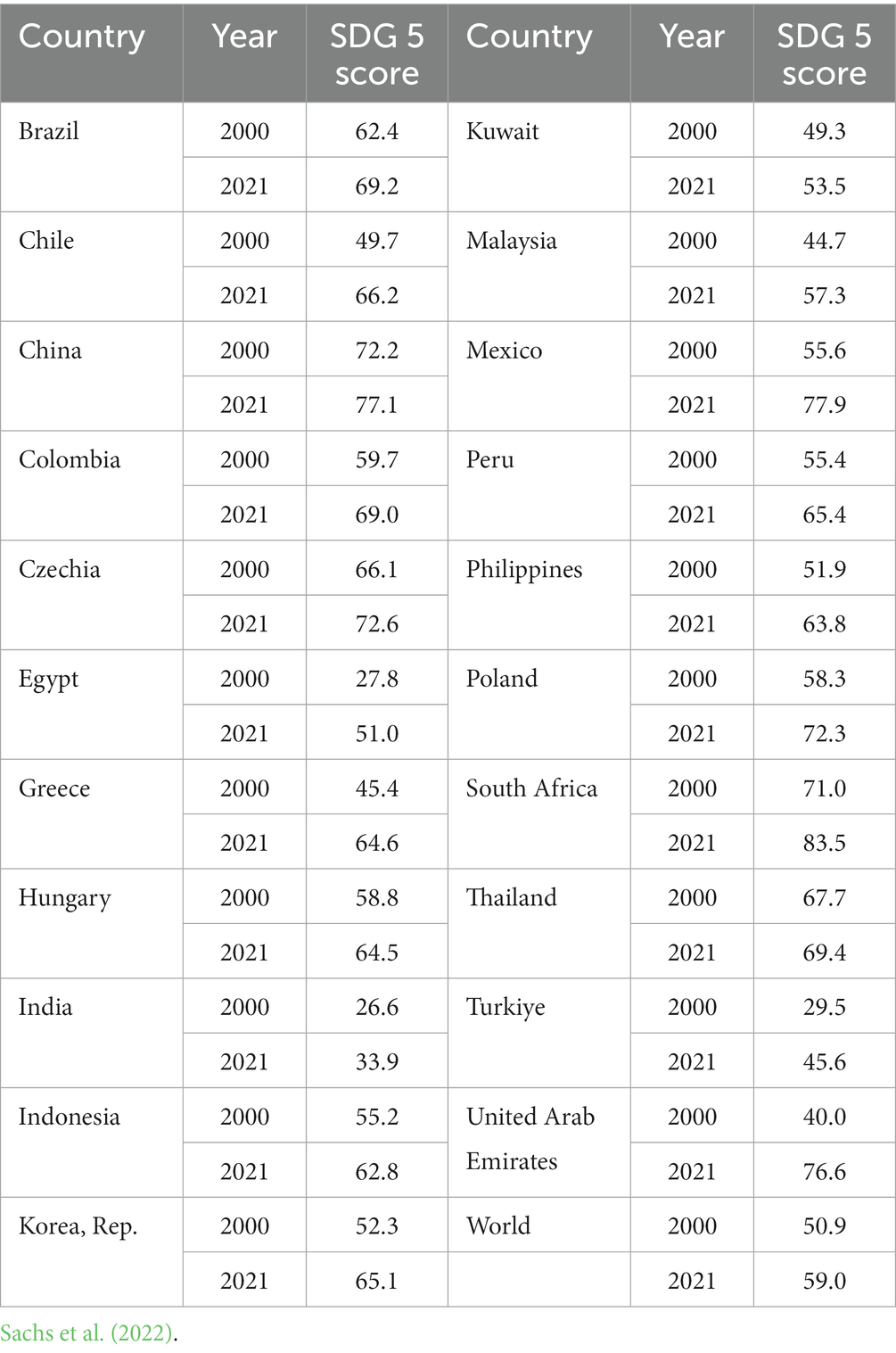
Table 1 . SDG-5 (Gender equality) score of emerging markets.
The empirical literature on gender inequality has generally investigated the influence of gender inequality on economic growth and development [e.g., see Kleven and Landais, 2017 ; Karoui and Feki, 2018 ; Bertay et al., 2020 ; Farooq et al., 2020 ; Koengkan et al., 2022 and Wu et al., 2022 ] but the empirical studies on determinants of gender inequality have stayed very limited and uncovered diverse social and economic factors behind gender inequality. In this context, Nyiransabimana (2015) and Kim (2021) focused on the influence of education on gender inequality. However, only Apergis and Lynch (2022) investigated the influence of economic freedom and education on the gender pay gap in the empirical literature. Therefore, we evaluate that this article will be one of the first studies to investigate the interplay among economic freedom, education level, and gender inequality in both the short and long term and thus targets making a contribution to the related empirical literature. The second contribution of the article is to employ cointegration and causality tests, taking notice of heterogeneity and cross-sectional properties of the panel dataset, as the empirical studies on determinants of gender inequality have usually performed a regression analysis. The subsequent section of the article scrutinizes the studies about the determinants of gender inequality, and then data and methods are disclosed. The econometric tests are performed and their results are disputed regarding the related literature in Section 4. The article arrives at a conclusion in Section 5.
2. Literature review
The achievement of worldwide gender equality is one of the 17 SDGs and gender equality is vital not only for the establishment of human rights but also for the achievement of other SDGs such as good health and well-being, zero hunger, no poverty, decent work, and economic growth. In this context, Nguyen (2021) analyzed the impact of gender equality on economic complexity in 119 countries for the 1991–2017 period and revealed the benefits of gender equality on economic complexity. Vyas-Doorgapersad (2019) reached the conclusion that gender equality was a significant instrument to combat poverty in South Africa. Pinho-Gomes et al. (2023) analyzed the relationship between gender equality and gender differences in life expectancy in 156 countries over the 2010–2021 period through regression analysis and revealed that higher gender equality was related to longer life expectance for women and men, but that the gender gap in life expectancy had widened. Kolip and Lange (2018) found a similar interaction between gender inequality and the gender gap in life expectancy for the European Union member states. Milner et al. (2021) also discovered that higher gender equality was associated with better health outcomes. However, the factors behind gender inequality have not been sufficiently addressed in the empirical literature yet. In this context, researchers have specified different socioeconomic factors underlying gender inequality for different countries and country groups.
Baliamoune-Lutz (2007) investigated the impact of globalization on gender inequality in Africa through regression and reached the conclusion that globalization did not have a significant impact on gender inequality in non-Sub-Saharan African developing countries, but higher global integration had a positive impact on gender inequality. Moreover, Juhn et al. (2014) developed a theoretical model of the impact of trade on gender inequality and suggested that a decrease in tariffs increases the employment of women in blue-collar jobs, but not in white-collar jobs and tested their model with data from the trade of Mexico in the context of the North American Free Trade Agreement and revealed that the findings supported their model.
Nyiransabimana (2015) researched the socioeconomic factors behind gender inequality in higher education in rural parts of Rwanda through qualitative research and uncovered that the education level of the parents, early marriage, poverty, pregnancies, and child care were the main determinants of gender inequality. Jayachandran (2015) also suggested that cultural norms were significant determinants of gender inequality in developing countries. Sangaji et al. (2018) explored the effect of GDP per capita , trade, and foreign direct investments on gender inequality in the Association of Southeast Asian Nations (ASEAN) members from 2010 to 2015 via dynamic regression and reached the conclusion that GDP per capita , foreign direct investments, and trade negatively influenced the gender inequality.
Kim (2021) examined the factors behind gender inequality in 34 countries (18 Organisation for Economic Co-operation and Development (OECD) members and 16 non-OECD states) via a regression method and reached the conclusion that compulsory education length and government educational expenditures promoted gender equality. Furthermore, fertility rate and unemployment had a positive impact on gender inequality, but all worldwide governance indicators (regulatory quality, voice and accountability, government effectiveness, rule of law, political stability, and absence of violence and corruption) and rate of employers and wage and salaried workers had a negative influence on gender inequality.
Some researchers have analyzed the interaction between education and the gender pay gap and reached different conclusions. Olarewaju et al. (2019) , Adeosun and Owolabi (2021) , and Akdoğan Gedik and Günel (2021) uncovered a negative influence of education on the gender pay gap, but Apergis and Lynch (2022) discovered a positive impact of education together with economic freedom on the gender pay gap.
Olarewaju et al. (2019) analyzed the influence of gender and minimum wage on returns to occupation in Nigeria with the 2004 data from the Nigerian Living Standards Survey and discovered a negative influence of education on the gender pay gap. Adeosun and Owolabi (2021) explored the determinants underlying the gender pay gap in Nigeria for the 2015–2016 period through regression and found that education decreased the gender pay gap because women had a higher return rate on education than men. Akdoğan Gedik and Günel (2021) also analyzed the determinants behind the gender wage gap in selected OECD states over the 1997–2016 period through regression analysis and they discovered that education negatively influenced the gender wage gap. However, Apergis and Lynch (2022) investigated the influence of economic freedom and education on the gender pay gap in the United Kingdom using data over the 2009–2016 period through a regression method and revealed that economic freedom and education affected the gender pay gap positively because the positive influence of economic freedom and education on wages of men were found to be higher than those of women.
Based on the related literature review, the research question of the study is:
What is the role of education level and economic freedom in gender inequality?
The hypotheses of the research are as follows:
H1 : There is a significant relationship between education level and gender inequality.
H2 : There is a significant relationship between economic freedom and gender inequality.
3. Data and method
The research investigated the influence of education level and economic freedom on gender inequality in a sample of emerging markets. Gender inequality (GII) was represented by the Gender Inequality Index of UNDP (2023) . The Gender Inequality Index is calculated depending on disadvantages labor market, empowerment, and reproductive health through the association-sensitive inequality measure by Seth (2009) and gives a value between 0 (women and men fare equally) and 1 (one gender fares as poorly as possible in these three dimensions) ( UNDP, 2023 ). On the other hand, education (EDUCATION) was proxied by the Education Index of UNDP (2023) and gives a value between 0 and 1 and higher index values show higher education levels. Last, economic freedom (EFI) was substituted by the Economic Freedom Index of the Fraser Institute (2023) , and the index gives a value between 0 and 10 and higher index values indicate higher economic freedom levels. The study duration covers 2000–2020 because economic freedom data is available for this period. Furthermore, emerging markets were selected based on MSCI (2023) , but Qatar, Saudi Arabia, and Taiwan were excluded from the analyses owing to data non-availability.
The major statistics of the Gender Inequality Index, Education Index, and Economic Freedom Index presented in Table 2 show that the means of the Gender Inequality Index, Education Index, and Economic Freedom Index are, respectively, 0.35, 0.6788, and 6.9152. The variation of the Economic Freedom Index among the emerging markets is relatively higher when compared those of the Gender Inequality Index and Education Index.
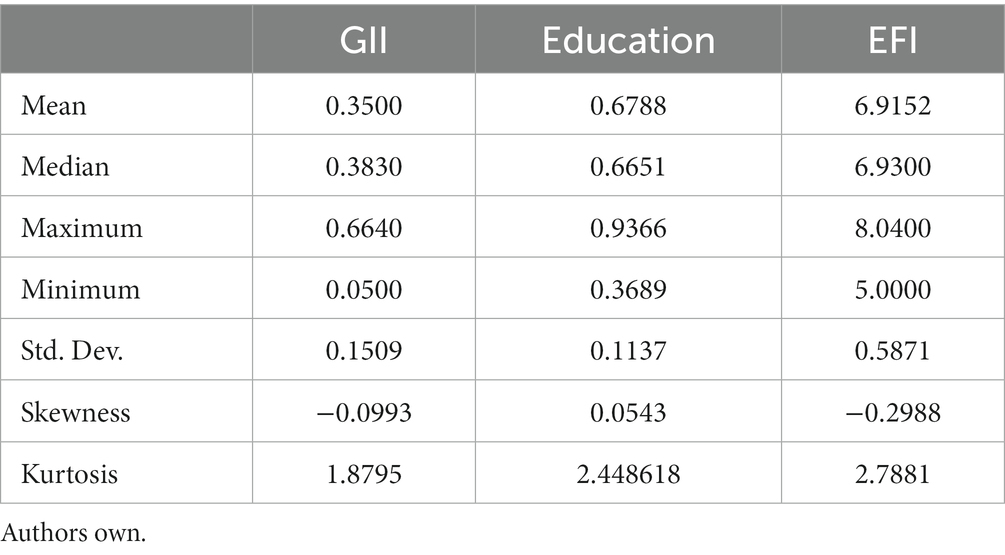
Table 2 . Main characteristics of the variables.
The interplay among economic freedom, education level, and gender inequality was analyzed by Westerlund and Edgerton (2007) using an LM (Lagrange Multiplier) bootstrap cointegration test and Dumitrescu and Hurlin (2012) causality test because a significant heterogeneity and cross-sectional dependence among three variables are unveiled. The causality analysis is useful to see the mutual interplay among economic freedom, education level, and gender inequality. Furthermore, the cointegration test examines whether the long-term linear relationship between two or more variables is stationary even if there does not exist a linear interplay in the short term ( Tu et al., 2019 ). Therefore, the cointegration test enables us to see the long-term interplay among economic freedom, education level, and gender inequality.
The second-generation LM bootstrap cointegration test allows autocorrelation and heteroscedasticity in the cointegration equation and gives relatively more consistent results, especially for small datasets. The cointegration test based upon McCoskey and Kao’s (1998) LM test and the critical values generated from bootstrapping were considered to examine whether there was significant cross-sectional dependence among economic freedom, education level, and gender inequality ( Westerlund and Edgerton, 2007 ). The cointegration test is generated from Equation (1) :
t = 2000,2001,….,2020 and i = 1,2….,21, respectively, show the time and cross-sections of the panel dataset and z i t ( z i t = μ i t + v i t ∑ j = 1 t η i j ) is the error term. η i j is an error term with a zero mean and σ i 2 variance.
The null hypothesis of the LM bootstrap cointegration test takes a significant cointegration among economic freedom, education level, and gender inequality in the emerging markets under consideration and the existence of significant cointegration among the three variables was tested by the LM test statistic presented in Equation (2) .
s i t 2 is the partial sum of z i t , and ω ̂ i − 2 is the long-term variance of μ i t .The causality test analyzed the bidirectional interplay between economic freedom, education level, and gender inequality. In other words, it tests whether economic freedom has a significant influence on gender inequality and whether gender inequality has a significant influence on economic freedom. The Dumitrescu and Hurlin (2012) causality test can be performed if the panel is unbalanced or there exists heterogeneity and cross-sectional dependence, N > T, and T > N. The causality test uses the (3) numbered equation for causality analysis:
In (3) numbered equation, k is lag length, γ and β are, respectively, the dependent and independent variables’ coefficients. In the causality analysis, the series under consideration should be stationary. The null hypothesis of the causality test claims a non-causality between two variables and it is tested by Wald ( W N , T H n c ( H o m o g e n e o u s n o n c a u s a l i t y ) ) and Z N , T H n c test statistics in equations (4) and (5):
W N T H n c test statistic with asymptotic distribution is considered if N < T , but the Ztild ( Z N H n c ) test statistic with a semi-asymptotic distribution is taken into account if T < N .
4. Results and discussion
The relationship between economic freedom, education level, and gender inequality was analyzed through a second-generation cointegration test and causality test. Therefore, first, cross-section dependence and heterogeneity were, respectively, examined by the tests shown in Table 3 . The presence of cross-sectional dependence among economic freedom, education level, and gender inequality was tested through L M a d j . , LM CD, and LM tests, and the tests’ findings are reported in Table 3 . The alternative hypothesis of three tests (“there is significant cross-sectional dependence among three series”) was accepted because the probability values were found to be lower than 0.05. Then, the presence of homogeneity was questioned through delta tilde tests, and the tests’ results are shown in Table 3 . The alternative hypothesis of the delta tests (“there is heterogeneity”) was accepted because their probability values were found to be lower than 0.05. As a result, the preference for econometric tests regarding cross-sectional dependency and heterogeneity induces us to obtain relatively more consistent results. As a result, use of unit root, cointegration, and causality tests taking notice of cross-sectional dependency and heterogeneity leads us to attain relatively more robust results.
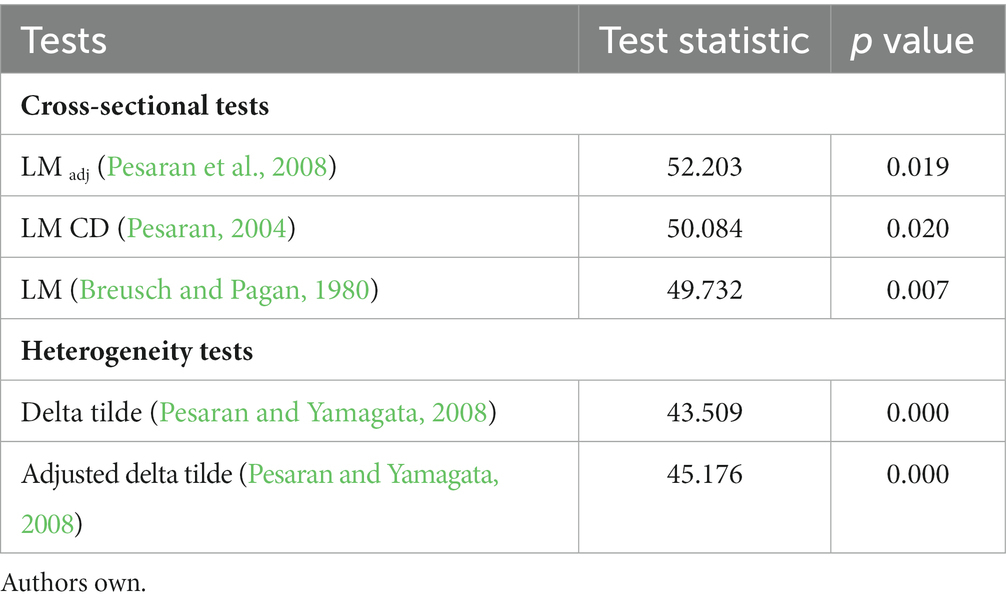
Table 3 . Findings of cross-sectional dependence and heterogeneity analyses.
The stationarity analysis of GII, EDUCATION, and EFI was performed via Pesaran (2007) cross-sectional augmented Dickey–Fuller (CADF) unit root test, and the findings of the unit root test are depicted in Table 4 . All three series were non-stationary at level values but they became stationary at first-differenced values.

Table 4 . Findings of CADF unit root analysis.
The cointegration interplay among economic freedom, education level, and gender inequality in emerging markets was analyzed by an LM bootstrap cointegration test due to cross-section dependence and a relatively small dataset. The findings of the cointegration test are reported in Table 5 . As a consequence, the null hypothesis supporting the entity of significant cointegration was accepted, and in turn, it was concluded there exists a long-term relationship between economic freedom, education level, and gender inequality.

Table 5 . Findings of LM Bootstrap cointegration analysis.
The panel and country-level cointegration coefficients were forecasted through an AMG estimator ( Eberhardt and Bond, 2009 ; Teal and Eberhardt, 2010 ), and the coefficients are reported in Table 6 . The panel-level coefficients uncovered that education level and economic freedom have a negative influence on gender inequality. The country-level cointegration coefficients also unveiled that education has a negative influence on gender inequality in all countries, similarly, economic freedom has a negative on gender inequality in all emerging markets except Egypt, India, the Philippines, and Kuwait. However, the negative impact of education level on gender inequality was found to be stronger than that of economic freedom in all emerging markets.
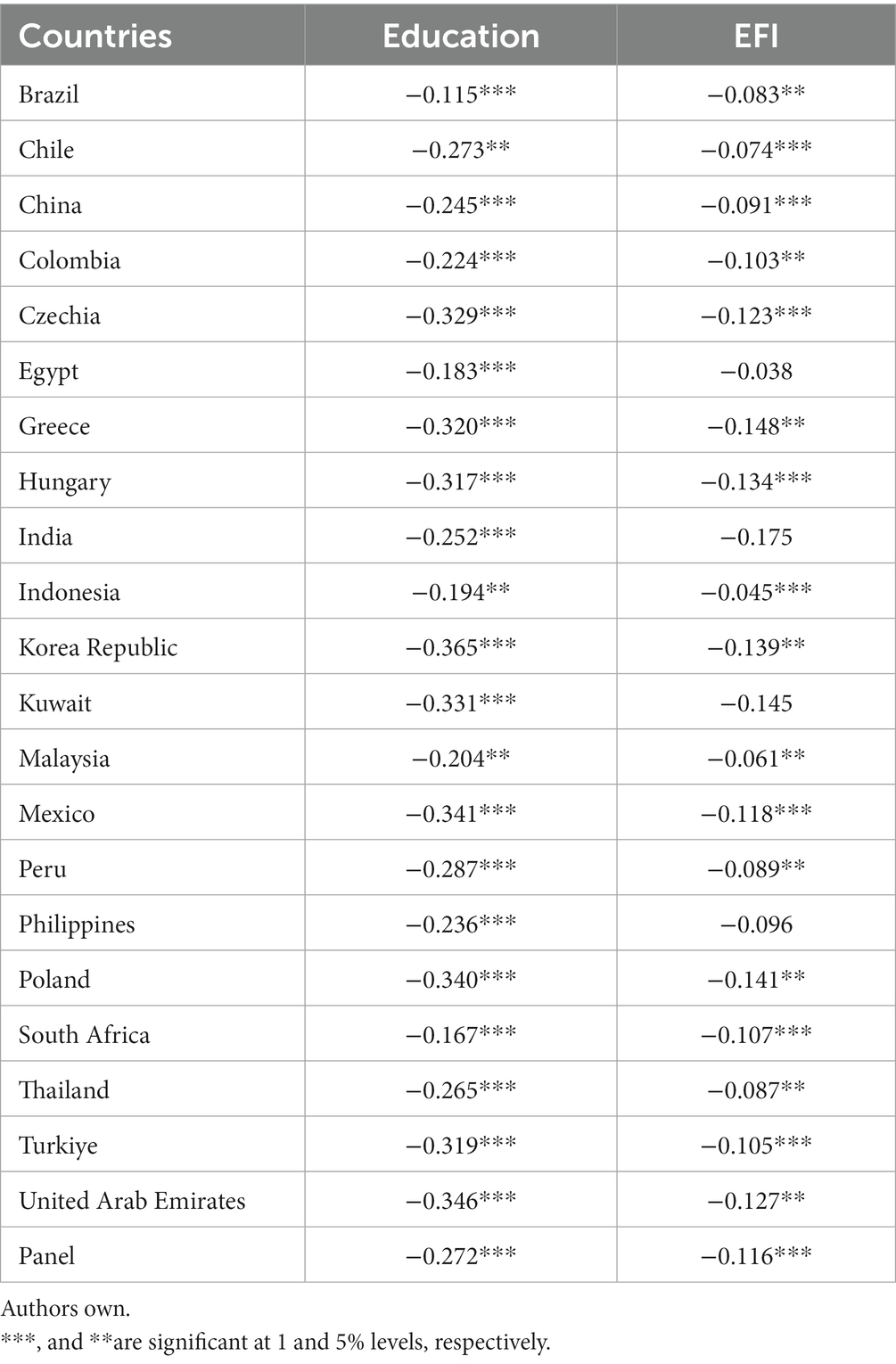
Table 6 . Cointegration coefficient estimation.
Both education level and economic freedom are expected to decrease gender inequality depending on country-specific characteristics through raising the awareness of society about the role of women in economic and social development and introducing equal opportunities for everybody regardless of gender. However, human development, cultural norms, and institutional and legal factors in the countries are significant for the interplay among education level, economic freedom, and gender inequality ( Jayachandran, 2015 ; Kim, 2021 ). In the empirical literature, Nyiransabimana (2015) and Kim (2021) focused on the factors underlying gender inequality in Rwanda and OECD countries, respectively. Nyiransabimana (2015) suggested the education level of parents together with other socioeconomic factors were significant determinants of gender inequality. Kim (2021) uncovered that compulsory education length and government educational expenditures were determinants of gender equality with public governance indicators and other socioeconomic factors. Furthermore, Jayachandran (2015) suggested that cultural norms were significant determinants of gender inequality in developing countries and Sangaji et al. (2018) also found that GDP per capita , foreign direct investments, and trade were significant determinants of gender inequality. Therefore, the limited empirical literature supports the significant role of education in gender inequality uncovered by the cointegration analysis in this study and also suggests that country-specific characteristics such as economic development, cultural norms, and public governance influence the interaction between education level and gender inequality.
On the other hand, some researchers have analyzed the impact of education and economic freedom on the gender pay gap and reached different results. Olarewaju et al. (2019) , Adeosun and Owolabi (2021) , and Akdoğan Gedik and Günel (2021) uncovered a negative influence of education on the gender pay gap, but Apergis and Lynch (2022) discovered a positive impact of education and economic freedom on the wages of women and men, but the positive impact of education and economic freedom on the wages of men was found to be relatively higher than those of women. So, these studies also verify that education and economic freedom have a positive influence on gender equality by decreasing the gender pay gap.
Furthermore, a negative influence of education level on gender inequality is discovered for all emerging markets under consideration, but the size of the cointegration coefficients remarkably varies among emerging markets. The findings uncover that countries with higher human and economic development such as Czechia, Greece, Hungary, the Republic of Korea, Kuwait, Malaysia, Poland, Thailand, Turkiye, and the United Arab Emirates generally experience a higher negative impact of education level and economic freedom on gender inequality.
The causal relationship between education level, economic freedom, and gender inequality was analyzed by the Dumitrescu and Hurlin (2012) causality test, and the findings of the causality analysis are reported in Table 7 . The findings of causality analysis support the theoretical expectations of a two-way causal relationship between education level, economic freedom, and gender inequality because both education level and economic freedom have a significant influence on gender inequality and gender inequality also has a significant impact on education level and economic freedom by raising the role of women in social and economic life. In the empirical literature, the two-way interplay between economic freedom, education level, and gender inequality has not been analyzed yet. Therefore, this study will be useful to see the mutual interaction among these variables.
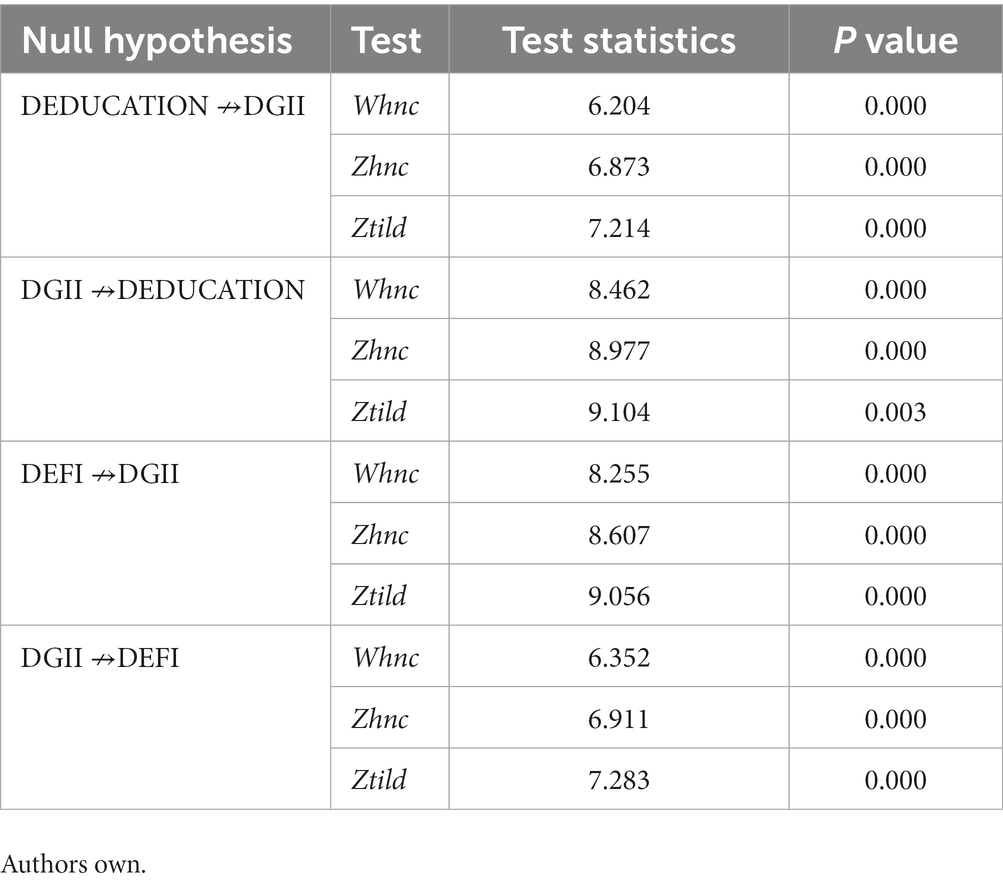
Table 7 . Findings of causality test.
5. Conclusion
The world has already had significant gender gaps in all aspects of life such as economic and political participation, education, and health. Therefore, gender equality is accepted as one of the 17 SDGs. Furthermore, gender equality is also a critical factor for the achievement of other SDGs considering that women constitute half of the human capital in the world. However, nearly 16% improvement has been achieved in gender equality (SDG-5) between 2000 and 2021. Therefore, more struggles and measures to reach gender equality globally are required. Similarly, we reveal that studies on the factors underlying gender inequality have been limited. The limited literature motivated us to analyze the interplay among economic freedom, education level, and gender inequality in a sample of emerging markets. Data availability limited our study period and sample. The study period was specified as 2000–2020, because annual economic freedom data was available over the 2000–2020 duration, and the emerging markets of Qatar, Saudi Arabia, and Taiwan were not included in the econometric analyses owing to data absence.
The findings of causality analysis uncovered a two-way interplay between economic freedom, education level, and gender inequality in keeping with theoretical considerations. In other words, gender inequality, economic freedom, and education level impact each other in the short term. On the other hand, the findings of the cointegration analysis indicated that economic freedom and education level have a negative impact on gender inequality in the long run, but the negative impact of education level on gender inequality was unveiled to be relatively stronger than that of economic freedom in all emerging markets. Furthermore, the findings of the long-term analysis showed that the negative impact of education level and economic freedom on gender inequality is higher in countries with higher human and economic development and institutional quality. As a consequence, both education and market-oriented economic structures are significant instruments to decrease gender inequality in both the short and long term. However, country-specific characteristics such as economic development, public governance, cultural norms, customs, and traditions have an influence on the impact of education and economic freedom on gender inequality. Therefore, countries should consider their country-specific characteristics when designing educational and market-oriented policies to improve gender equality. Future studies can focus on the role that cultural and institutional norms have in the interplay among education level, economic freedom, and gender inequality.
Data availability statement
The raw data supporting the conclusions of this article will be made available by the authors, without undue reservation.
Ethics statement
Ethical review and approval was not required for the study on human participants in accordance with the local legislation and institutional requirements. Written informed consent from the participants was not required to participate in this study in accordance with the national legislation and the institutional requirements.
Author contributions
Conceptualization: PT, GS, MD and MAT. Formal analysis: PT, GS, MD and MAT. Investigation: PT, GS, MD and MAT. Methodology: PT, GS, MD and MAT. Supervision: PT and GS. Writing – original draft and editing: PT, GS, MD and MAT. All authors have read and agreed to the published version of the manuscript.
Conflict of interest
The authors declare that the research was conducted in the absence of any commercial or financial relationships that could be construed as a potential conflict of interest.
Publisher’s note
All claims expressed in this article are solely those of the authors and do not necessarily represent those of their affiliated organizations, or those of the publisher, the editors and the reviewers. Any product that may be evaluated in this article, or claim that may be made by its manufacturer, is not guaranteed or endorsed by the publisher.
Adeosun, O. T., and Owolabi, K. E. (2021). Gender inequality: determinants and outcomes in Nigeria. J. Bus. and Socioecon. Dev. 1, 165–181. doi: 10.1108/JBSED-01-2021-0007
CrossRef Full Text | Google Scholar
Akdoğan Gedik, M., and Günel, T. (2021). Factors affecting gender wage gap: emprical evidences from selected OECD countries. Ömer Halisdemir Üniversitesi İktisadi ve İdari Bilimler Fakültesi Dergisi 14, 1051–1061. doi: 10.25287/ohuiibf.823479
Apergis, N., and Lynch, N. (2022). The impact of economic freedom on the gender pay gap: evidence from a survey of UK households. J. Econ. Stud. 49, 61–76. doi: 10.1108/JES-09-2020-0444
Baliamoune-Lutz, M. (2007). Globalisation and gender inequality: is Africa different? J. Afr. Econ. 16, 301–348. doi: 10.1093/jae/ejl037
Bertay, A.C., Dordevic, L., and Sever, C. (2020). Gender inequality and economic growth: evidence from industry-level data. IMF Working Paper, WP/20/119. https://ssrn.com/abstract=3658594
Google Scholar
Breusch, T. S., and Pagan, A. R. (1980). The Lagrange multiplier test and its applications to model specification tests in econometrics. Rev. Econ. Stud. 47, 239–253. doi: 10.2307/2297111
Dumitrescu, E.-I., and Hurlin, C. (2012). Testing for granger non-causality in heterogeneous panels. Econ. Model. 29, 1450–1460. doi: 10.1016/j.econmod.2012.02.014
PubMed Abstract | CrossRef Full Text | Google Scholar
Eberhardt, M., and Bond, S. (2009). Cross-section dependence in nonstationary panel models: a novel estimator. MPRA paper, no. 17692 , University Library of Munich: Munich, Germany.
Farooq, F., Yusop, Z., Chaudhry, I. S., and Iram, R. (2020). Assessing the impacts of globalisation and gender parity on economic growth: empirical evidence from OIC countries. Environ. Sci. Pollut. Res. 27, 6904–6917. doi: 10.1007/s11356-019-07289-y
Ferrant, G. (2015). How do gender inequalities hinder development? Cross-country evidence. Ann. Econ. Stat. 117–118, 313–352. doi: 10.15609/annaeconstat2009.117-118.313
Fraser Institute. Economic Freedom (2023). Available at: https://www.fraserinstitute.org/studies/economic-freedom (Accessed January 12, 2023).
Gonzales, C., Jain-Chandra, S., Kochhar, K., Newiak, M., and Zeinullayev, T. (2015). Catalyst for change: empowering women and tackling income inequality. IMF Staff Discuss. Note . doi: 10.5089/9781513533384.006
Jayachandran, S. (2015). The roots of gender inequality in developing countries. Ann. Rev. Econ. 7, 63–88. doi: 10.1146/annurev-economics-080614-115404
Juhn, C., Ujhelyi, G., and Villegas-Sanchez, C. (2014). Men, women, and machines: how trade impacts gender inequality. J. Dev. Econ. 106, 179–193. doi: 10.1016/j.jdeveco.2013.09.009
Kane, E. W. (1995). Education and beliefs about gender inequality. Soc. Probl. 42, 74–90. doi: 10.2307/3097006
Karoui, K., and Feki, R. (2018). The effect of gender inequality on economic development: case of African countries. J. Knowl. Econ. 9, 294–300. doi: 10.1007/s13132-015-0341-9
Kim, S. Y. (2021). Determining critical factors of gender inequality: evidence from 34 OECD and non-OECD countries. World Dev. Perspect. 21:100284. doi: 10.1016/j.wdp.2020.100284
Kleven, H., and Landais, C. (2017). Gender inequality and economic development: fertility, education and norms. Economica 84, 180–209. doi: 10.1111/ecca.12230
Koengkan, M., Fuinhas, J. A., Belucio, M., Kazemzadeh, E., Poveda, Y. E. M., Alavijeh, N. K., et al. (2022). The consequences of gender inequality on Latin America’s economic growth: macroeconomic evidence. Theol. Sex. 3, 396–412. doi: 10.3390/sexes3030030
Kolip, P., and Lange, C. (2018). Gender inequality and the gender gap in life expectancy in the European Union. Eur. J. Pub. Health 28, 869–872. doi: 10.1093/eurpub/cky076
McCoskey, S., and Kao, C. (1998). A residual-based test of the null of cointegration in panel data. Econ. Rev. 17, 57–84. doi: 10.1080/07474939808800403
Milner, A., Kavanagh, A., Scovelle, A. J., O'Neil, A., Kalb, G., Hewitt, B., et al. (2021). Gender equality and health in high-income countries: a systematic review of within-country indicators of gender equality in relation to health outcomes. Women's Health Reports 2, 113–123. doi: 10.1089/whr.2020.0114
MSCI (2023). Emerging markets. Available at: https://www.msci.com/our-solutions/indexes/emerging-markets (Accessed January 12, 2023).
Nguyen, C. P. (2021). Gender equality and economic complexity. Econ. Syst. 45:100921. doi: 10.1016/j.ecosys.2021.100921
Nyiransabimana, V. (2015). Socio-economic determinants of gender inequality in higher education in rural areas in Rwanda. Direct Res. J. Soc. Sci. Educ. Stud. 2, 69–76.
Olarewaju, T. I. A., Mickiewicz, T., and Tamvada, J. P. (2019). The returns to occupations: the role of minimum wage and gender in Nigeria. World Dev. Perspect. 13, 75–86. doi: 10.1016/j.wdp.2019.02.012
Pesaran, M. H. (2004). “General diagnostic tests for cross-section dependence in panels” in University of Cambridge Working Paper CWPE 0435 (Cambridge: Cambridge University).
Pesaran, M. H. (2007). A simple panel unit root test in the presence of cross-section dependence. J. Appl. Econ. 22, 265–312. doi: 10.1002/jae.951
Pesaran, M. H., Ullah, A., and Yamagata, T. (2008). A bias-adjusted LM test of error cross-section independence. Econom. J. 11, 105–127. doi: 10.1111/j.1368-423X.2007.00227.x
Pesaran, H., and Yamagata, T. (2008). Testing slope homogeneity in large panels. J. Econom. 142, 50–93. doi: 10.1016/j.jeconom.2007.05.010
Pinho-Gomes, A. C., Peters, S. A. E., and Woodward, M. (2023). Gender equality related to gender differences in life expectancy across the globe gender equality and life expectancy. PLOS Glob. Public Health 3:e0001214. doi: 10.1371/journal.pgph.0001214
Sachs, J., Lafortune, G., Kroll, C., Fuller, G., and Woelm, F. (2022). From crisis to sustainable development: the SDGs as roadmap to 2030 and beyond . Cambridge: Cambridge University Press.
Sangaji, J., Kurnia, M., and Kurnia, A. S. (2018). Macroeconomic determinants of gender inequality index in eight ASEAN countries. E3S Web Conf. 73:11002. doi: 10.1051/e3sconf/20187311002
Schultz, T. P. (2002). Why governments should invest more to educate girls. World Dev. 30, 207–225. doi: 10.1016/S0305-750X(01)00107-3
Seth, S. (2009). Inequality, interactions, and human development. J. Human Dev. Capab. 10, 375–396. doi: 10.1080/19452820903048878
Stroup, M. (2008). Separating the influence of capitalism and democracy on women’s wellbeing. J. Econ. Behav. Organ. 67, 560–572. doi: 10.1016/j.jebo.2007.08.005
Teal, F., and Eberhardt, M. (2010). Productivity analysis in global manufacturing production. Economics series working papers, no. 515 , University of Oxford: Oxford, UK.
Tu, C., Fan, Y., and Fan, J. (2019). Universal cointegration and its applications. IScience 9, 986–995. doi: 10.1016/j.isci.2019.08.048
UNDP (2023). Data downloads. Available at: https://hdr.undp.org/data-center/documentation-and-downloads ().
United Nations (2023a). The 17 goals. Available at: https://sdgs.un.org/goals (Accessed January 14, 2023).
United Nations (2023b). Gender equality: why it matters. Available at: https://www.un.org/sustainabledevelopment/wp-content/uploads/2016/08/5_Why-It-Matters-2020.pdf (Accessed January 12, 2023).
Vyas-Doorgapersad, S. (2019). Gender equality for achieving sustainable development goal one (no poverty) in south African municipalities. Int. J. Soc. Sci. Humanit. Stud. 11, 84–98.
Westerlund, J., and Edgerton, D. L. (2007). A panel bootstrap cointegration test. Econ. Lett. 97, 185–190. doi: 10.1016/j.econlet.2007.03.003
World Bank (2023). Population, female (% of Total population). Available at: https://data.worldbank.org/indicator/SP.POP.TOTL.FE.ZS (Accessed January 04, 2023).
Wu, X., Ali, A., Zhang, T., Chen, J., and Hu, W. (2022). An empirical analysis of the impact of gender inequality and sex ratios at birth on China’s economic growth. Front. Psychol. 13:1003467. doi: 10.3389/fpsyg.2022.1003467
Keywords: education level, economic freedom, gender inequality, panel causality analysis, panel cointegration analysis, emerging market economies
Citation: Tokal P, Sart G, Danilina M and Ta’Amnha MA (2023) The impact of education level and economic freedom on gender inequality: panel evidence from emerging markets. Front. Psychol . 14:1202014. doi: 10.3389/fpsyg.2023.1202014
Received: 07 April 2023; Accepted: 17 July 2023; Published: 02 August 2023.
Reviewed by:
Copyright © 2023 Tokal, Sart, Danilina and Ta’Amnha. This is an open-access article distributed under the terms of the Creative Commons Attribution License (CC BY) . The use, distribution or reproduction in other forums is permitted, provided the original author(s) and the copyright owner(s) are credited and that the original publication in this journal is cited, in accordance with accepted academic practice. No use, distribution or reproduction is permitted which does not comply with these terms.
*Correspondence: Pinar Tokal, [email protected]
Disclaimer: All claims expressed in this article are solely those of the authors and do not necessarily represent those of their affiliated organizations, or those of the publisher, the editors and the reviewers. Any product that may be evaluated in this article or claim that may be made by its manufacturer is not guaranteed or endorsed by the publisher.
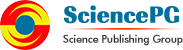
Journals By Subject
- Proceedings
Information

Impacts of Parental Involvement and Parents’ Level of Education on Student’s Academic Accomplishment
Won-Tack Lim
Margaret Warner Graduate School of Education and Human Development, University of Rochester, Rochester, USA
Add to Mendeley

Parental involvement in school has been demonstrated to play a crucial role in children’s academic achievement. This article examines the relation of adolescent's academic achievement to parental involvement and parent’s education level, separately, grounded on a quantitative approach. All participants were middle-class dual-earner parents from eight cities across the United States. Spearman's correlation coefficient was used to find out if adolescent academic performance is correlated with parental involvement, and Mann–Whitney U test was utilized when children’s academic performance was compared between groups of parents with different educational levels. Results show that children’s academic achievement is only correlated with certain dimensions of parental involvement. Results further show that, across the board, children’s school achievements do not significantly differ across the groups with different levels of parents’ education if parents in all groups are equally committed to children’s education. As a result, this study concludes that the effect of parental involvement on children’s academic success differs by the type of parental engagement and that parents’ level of education does not carry much weight over children’s academic performance when all parents are actively involved in their schooling. Both limitations and suggestions for future studies are also discussed.
Parental Involvement, Academic Achievement, Education Level, Quantitative Approach, Middle-Class Dual-Earner Parents
Won-Tack Lim. (2021). Impacts of Parental Involvement and Parents’ Level of Education on Student’s Academic Accomplishment. Education Journal , 10 (1), 35-39. https://doi.org/10.11648/j.edu.20211001.15

Won-Tack Lim. Impacts of Parental Involvement and Parents’ Level of Education on Student’s Academic Accomplishment. Educ. J. 2021 , 10 (1), 35-39. doi: 10.11648/j.edu.20211001.15
Won-Tack Lim. Impacts of Parental Involvement and Parents’ Level of Education on Student’s Academic Accomplishment. Educ J . 2021;10(1):35-39. doi: 10.11648/j.edu.20211001.15
Cite This Article
- Author Information
Verification Code/
The verification code is required.
Verification code is not valid.

Science Publishing Group (SciencePG) is an Open Access publisher, with more than 300 online, peer-reviewed journals covering a wide range of academic disciplines.
Learn More About SciencePG
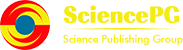
- Special Issues
- AcademicEvents
- ScholarProfiles
- For Authors
- For Reviewers
- For Editors
- For Conference Organizers
- For Librarians
- Article Processing Charges
- Special Issues Guidelines
- Editorial Process
- Peer Review at SciencePG
- Open Access
- Ethical Guidelines
Important Link
- Manuscript Submission
- Propose a Special Issue
- Join the Editorial Board
- Become a Reviewer
Advertisement
How education level influences internet security knowledge, behaviour, and attitude: a comparison among undergraduates, postgraduates and working graduates
- Regular Contribution
- Published: 24 November 2022
- Volume 22 , pages 305–317, ( 2023 )
Cite this article
- Qin An ORCID: orcid.org/0000-0002-1937-1587 1 ,
- Wilson Cheong Hin Hong ORCID: orcid.org/0000-0002-9858-2015 2 ,
- XiaoShu Xu ORCID: orcid.org/0000-0002-0667-4511 3 , 4 ,
- Yunfeng Zhang ORCID: orcid.org/0000-0002-9995-8432 5 &
- Kimberly Kolletar-Zhu ORCID: orcid.org/0000-0002-5347-9575 3
4091 Accesses
7 Citations
1 Altmetric
Explore all metrics
During the pandemic, the prevailing online learning has brought tremendous benefits to the education field. However, it has also become a target for cybercriminals. Cybersecurity awareness (CSA) or Internet security awareness in the education sector turns out to be critical to mitigating cybersecurity risks. However, previous research indicated that using education level alone to judge CSA level received inconsistent results. This study postulated Social Educational Level (SEL) as a moderator with an extended Knowledge-Attitude-Behaviour model, used students’ year level as a proxy for the impact of education level, and used work exposure for the influence of social education level, to compare CSA among undergraduates, postgraduates and working graduates. The participants in the study were divided into six groups, namely year 1 university students, year 2-3university students, final-year students, postgraduate students, young working graduates, and experienced working graduates. The Human Aspects of Information Security Questionnaire was used to conduct a large-scale survey. The multivariate regression model analysis showed significant differences among the knowledge, attitude and behaviour dimensions across groups with different conditions of year-level and work exposure. However, it was found that SEL played a more significant role than an individual’s education level. The study suggested that a greater endeavour be committed to educating the public at large together with individuals, institutes, corporate and governments to improve the national CSA level.
Similar content being viewed by others
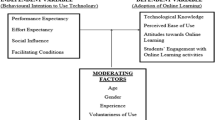
Adoption of online mathematics learning in Ugandan government universities during the COVID-19 pandemic: pre-service teachers’ behavioural intention and challenges
Geofrey Kansiime & Marjorie Sarah Kabuye Batiibwe
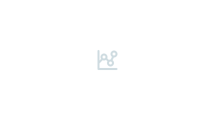
Ethical principles for artificial intelligence in education
Andy Nguyen, Ha Ngan Ngo, … Bich-Phuong Thi Nguyen
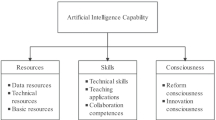
Effects of higher education institutes’ artificial intelligence capability on students' self-efficacy, creativity and learning performance
Shaofeng Wang, Zhuo Sun & Ying Chen
Avoid common mistakes on your manuscript.
1 Introduction
The coronavirus' global spread was causing ripples in all domains. The Internet has become a target for cybersecurity threats because it has become a place and platform for students to learn and employees to get to work. However, students and employees who did not major in information technology (IT) were forced to switch from offline to online in a short period of time with relatively less ISA, posing significant security risks. For example, since the spread of COVID-19, cyber-attacks have grown in sophistication and quantity. Since such rapid digital transformations in education, for example, online learning has become a new target for cybercriminals. The investigation of cybersecurity awareness and the impact of social educational level on Chinese netizens was beneficial.
Previous research mostly used behavioural models to assess ISA, such as the Theory of Planned Behaviour (TPB) [ 1 , 2 ], Protection Motivation Theory (PMT) [ 3 ], and the Knowledge-Attitude-Behaviour (KAB) model [ 4 ]. Based on KAB, the HAIS-Q was a comprehensive scale with high internal consistency and external reliability that was used to investigate internet security knowledge, attitude, and behaviour [ 5 ]. Parsons et al. acknowledged that social influence should be considered when using the HAIS-Q [ 6 ]. Hence, an extended KAB model, proposed by Hong et al., was applied [ 7 ], which suggested that more social contact at work implied a decrease in SEL.
There were numerous studies on ISA; however, few of them were in the Chinese context, and even fewer focused on empirical research on cybersecurity risks in higher education [ 8 ], not to mention that the influence of education level on ISA might be contradictory in previous research. Some studies found that education level has a positive effect [ 9 ], while others found that it has no effect [ 10 , 11 ]. This was due to the fact that in addition to the individual’s internal factors (e.g. education level), external factors of social influence were rarely the focus of research. Social influence is known as the effect of social interactions and communications with others on one’s beliefs and actions [ 12 ]. Family, friends and colleagues are the common sources of social influence. To this end, Hong et al. investigated the impact of the average education level of a society on one’s ISA [ 7 ], which was referred to as social education level (SEL). SEL was found to be a better predictor of social influence on ISA than nationalities and gross domestic product (GDP), which refers to the total market values of goods and services produced by workers and capital within a country during a given period (usually 1 year).
To assess the positive effects of education level and the negative influence of SEL, we recruited respondents with various education levels, including undergraduates and postgraduate students, and took into account three levels of work exposure, ranging from no exposure to the work environment (non-final-year undergraduates) to new exposure (young working graduates) and long-term exposure (experienced working graduates). This study assumed that a higher year level of university students means a higher (personal) education level and that a lower SEL could be observed in increased social contact at work. Students’/graduates’ educational level and SEL exposure were expected to mediate cybersecurity knowledge, attitude, and behaviour.
Given that relatively few large-scale ISA studies have been conducted in higher education and few attempts have been made to compare undergraduate students, postgraduate students, and working graduates, this study aimed to examine the influence of education level and SEL on students’ and graduates’ ISA, which may contribute in a theoretical and practical way by quantifying education influence and social impact on ISA.
2 Literature review
2.1 hais-q and extended kab model.
A review of previous ISA studies revealed that some pre-existing, well-established behavioural models were used to investigate the issue of ISA. The Theory of Planned Behaviour (TPB) [ 2 ], which evolved from the Theory of Reasoned Action [ 13 ], the Protection Motivation Theory (PMT) [ 14 ], the Health Belief Model [ 15 ], the General Deterrence Theory [ 16 ], the Technology Acceptance Model (TAM) [ 17 ], and the Knowledge-Attitude-Behaviour (KAB) model [ 4 ], have all attracted the interest of many ISA scholars [ 18 ].
Take the Theory of Planned Behaviour, whose key points include intention (attitude, subjective norms) and perceived behavioural control [ 13 ], which have been applied to adolescents' acceptance of friendship requests sent by online strangers on social networking sites [ 19 ]. This theory has also been used to assess students' levels of Cybersecurity awareness (CSA) at a private tertiary education institution [ 20 ], as well as to investigate Information Systems Security Policy (ISSP) compliance using two relevant theories, TPB and PMT [ 21 ].
The Theory of Planned Behaviour was composed of two items: perceived vulnerability and perceived severity [ 3 ], which have been found to be very useful in predicting behaviours related to an individual's computer security behaviours both at home and in organisations [ 22 ]; an individual's internet security perceptions and behaviours in a poly context [ 23 ] or an individual’s continued engagement in protective security [ 24 ] and Information Systems Security Policy compliance [ 25 , 26 ].
These models, however, have been criticised for failing to capture the complexities and specific phenomena of cybersecurity [ 27 ]. The KAB, a dynamic and sometimes reciprocal model, on the other hand, was originally used in the health and environmental psychology area [ 28 ] as well as in the criminology and education fields [ 29 ] and has also been applied to the ISA context [ 30 , 31 ]. KAB has received much attention among scholars and has been applied to various fields of research because previous research found that knowledge alone was not sufficient to cause behavioural changes [ 32 ].
Simultaneously, based on the KAB model, the conceptualised Human Aspects of Information Security Questionnaire (HAIS-Q) has been developed [ 33 ]. HAIS-Q research has shown that age, gender, resilience, job stress, education level, and some other personal characteristics can predict ISA to some extent [ 34 ]. In particular, education in information and communication technology (ICT) could positively benefit one’s IS behaviour [ 35 , 36 ]. In addition, it was also found that ISA would be higher as age increased, or among the female group, which was not consistent with findings from previous research results that no ISA difference could be seen between men and women [ 37 ], if individuals possess higher education [ 9 ], if one is more conscientious and agreeable [ 38 ]; and if one owned a propensity to take fewer risks [ 5 ]. Furthermore, if one was more resilient but underwent less job stress, they would have a higher ISA [ 34 ]. Moreover, an inverse relationship between Internet addiction, cyber-loafing tendencies, and ISA has been discovered [ 39 ]. A significant positive relationship between organizational culture, security culture, and ISA [ 9 ] has also been tested from the social influence or work environment perspective. Furthermore, the bank's employees have higher ISA than the general workforce participants in all focus areas and overall [ 37 ].
Although Parson et al. [ 6 ] acknowledged in their initial HAIS-Q proposal that other social factors should be considered when using the KAB model or HAIS-Q in future research, there was no easy way to quantify social influence. To adapt the framework, Hong et al. [ 7 ] proposed an extended model of KAB (see Fig. 1 ), which suggested that more social contact at work implied a decrease in SEL. SEL was about how the general education level of an internet user’s immediate circle, such as family, friends, and colleagues, influenced the user's cybersecurity behaviours [ 7 ].
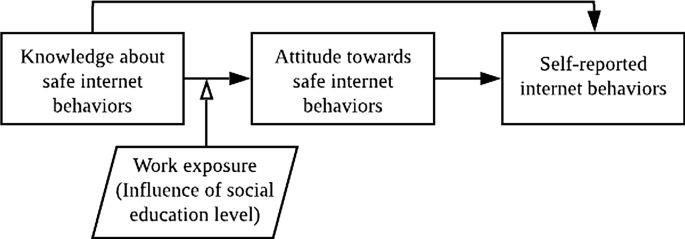
An extended KAB model, Source : Hong, W. C. H., et al. [ 7 ]. The influence of social education level on cybersecurity awareness and behaviour: a comparative study of university students and working graduates. Education and Information Technologies
@@@An extended KAB model, Source : Hong, W. C. H., et al. [ 7 ].
2.2 ISA influencing factors
When attempting to understand what shapes human behaviour, looking at an individual in isolation could be problematic. It has been found that individuals of different nationality backgrounds but similar levels of ISA exhibited highly varied cybersecurity behaviours and safety responses [ 31 ]. Past studies have found personal education level [ 35 ], gender [ 40 ], age [ 41 ], stress level [ 34 ] self-efficacy [ 42 ], and cultural beliefs [ 9 ] to be salient predictors of cybersecurity awareness and behaviours. An individual’s knowledge of information and communication technology (ICT), especially, has been widely suggested to positively correlate with ISA and safe behaviours [ 35 , 43 , 44 ]. However, no internet users live or work in isolation from other internet users, but they are under the constant influence of one another to adjust and readjust thoughts and actions. Hence, personal factors must be viewed in conjunction with social factors. Existing studies have found that social influence is a key motivator to safe or unsafe cybersecurity behaviours [ 12 , 19 , 45 , 46 , 47 ]. Some found that the online experience of friends and family impacted our own beliefs and behaviours [ 46 , 48 ], and that imitation of others is a primary reason for safe practices [ 45 ]. Similarly, the working environment where one is immersed has a tremendous impact on individuals’ ISA and practice [ 7 , 47 ]. Hong et al. [ 7 ] found that workplace influence in China, especially from colleagues of lower education levels, severely deteriorated university graduates’ attitudes towards safe internet practices. The deterioration emerged as early as in the (full-time) internship period, resulting in poor cybersecurity behaviours. Worse still, respondents’ cybersecurity knowledge appeared to be reshaped by the working environment. This echoes the findings of Elkhannoubi & Belaissaoui [ 12 ], who contended that social influence plays a more significant role in developing countries, as the individuals high in ISA are only the minority of the society. SEL as a relatively objective factor that is quantifiable by published indices such as the Education Index [ 49 ] and literacy rate [ 50 ] can serve to measure how much an individual is under social influence.
2.3 The influences of education level and SEL on ISA in China
Previous research found that ISA scores increased with age [ 34 , 37 , 41 ] and that individuals with a higher education had higher ISA scores [ 9 , 51 ]. However, some ISA education research discovered that final-year students, who were more educated and older, consistently scored the lowest in ISA when compared to other year-levels of students. To name a few, Li et al. [ 10 ] discovered that the senior group had significantly less awareness than the junior groups. Huang et al. [ 11 ] discovered that senior students were the most vulnerable to safety incidents. These findings in the Chinese context differed significantly from previous international research, in which an individual's education level was known to positively correlate with ISA [ 51 ].
At the same time, the impact of a country's GDP and development level on its population's ISA was volatile. As a result, SEL could be used as a factor to quantify external influence, including social influence and national context. SEL is concerned with how the general education level of an internet user's immediate circle, such as family, friends, and colleagues, influences the user's cybersecurity behaviours [ 7 ].
Previous research revealed some additional limitations. For example, to determine whether increased exposure to society affects ISA or whether academic growth influences it, it was necessary to compare the ISA of non-final-year students, final-year students, postgraduate students, as well as interns (final-year students), young working graduates, and experienced working graduates. A thorough literature review, however, revealed that there had been little comparative ISA research among these three groups, namely, different year levels of university students, postgraduate students, and working graduates. Furthermore, the majority of previous research on ISA in higher education had relatively small sample sizes. A sample size of 333 to 400 for each group of respondents has been recommended for better generalizability [ 52 ]. Despite the abundance of ISA research, a recent systematic review of ISA in higher education revealed that the majority of existing ISA surveys did not meet such criteria [ 8 ].
According to the findings of the preceding study, learners' attitudes towards cybersecurity would be influenced by their internal education level and external environment, which would eventually change their internet behaviours. Furthermore, education level had a positive effect and work exposure time had a negative effect on a person's ISA [ 7 , 9 ].
Hence, the following hypotheses were proposed:
University students of different years of study, postgraduate students, and working graduates will display differing cybersecurity knowledge.
Postgraduate students will score significantly higher in knowledge than final-year students.
Postgraduate students will score significantly higher in knowledge than working graduates.
Postgraduate students will score significantly higher in knowledge than non-final-year students.
University students of different years of study, postgraduate students and working graduates will display differing cybersecurity attitudes.
Postgraduate students will score significantly higher in attitudes than final-year students.
Postgraduate students will score significantly higher in attitudes than working graduates.
Postgraduate students will score significantly higher in attitudes than non-final-year students.
University students of different years of study, postgraduate students, and working graduates will display differing cybersecurity behaviours.
Postgraduate students will score significantly higher in behaviours than final-year students.
Postgraduate students will score significantly higher in behaviours than working graduates.
Postgraduate students will score significantly higher in behaviours than non-final-year students.
3 Methodology
3.1 sample and sampling method.
Snowball and criterion sampling methods were used to recruit participants. Students were invited to complete the questionnaire via email or personal contact, and then they contacted their peers to participate in the survey. Furthermore, as a criterion for participation, all students were first screened as having received cybersecurity training during their higher education and as having completed a mandatory full-time internship for half a year or a full year in their final year of study, allowing comparisons to be made between different levels of work exposure (i.e. prior, short-term and long-term work exposure).
In total, 1882 valid responses were obtained from more than ten higher education institutions and 110 Chinese businesses. There were 480 year 1 students, 372 year 2–3 students, 325 final-year students, 230 postgraduate students (age range = 18–25), and 343 young working graduates, 132 experienced working graduates (age range = 18–46); 938 majored in liberal arts and social sciences, 499 natural sciences, and 445 technology and engineering; 691 males and 1191 females. Table 1 contains descriptive information about the participants.
The participants were divided into two groups based on our hypothesis, which stated that work exposure time had a negative effect on a person's ISA and education level had a positive effect. Internships, young graduates, and experienced graduates were thus regarded as the first short-term and long-term work exposure to a work environment. Furthermore, non-final-year students, final-year students, and postgraduates were viewed as three distinct educational levels.
3.2 Instrument design
Hong et al.'s [ 7 ] survey questions were adopted. They translated, verified, and adjusted the questionnaire based on the Questionnaire on Human Aspects of Information Security (HAIS-Q). It was then distributed online via one of the largest local survey platforms, Wenjuanxing, also known as Sojump [ 53 ]. Meanwhile, each question was scored using a five-point Likert scale (5 = strongly agree, 1 = strongly disagree).
The study proposal submission form was submitted to and approved by the academic committee of the first author's university in December 2021. All survey responses were kept anonymous, and their participation in this study was entirely voluntary. When participants clicked on the survey, they would be prompted to a page containing brief information about the survey and their rights to privacy and anonymity. They then provided their consent by clicking “agree to continue”.
3.3 Data analyses
Data results ( N = 1882) were analysed by using IBM Statistic Package for Social Science (SPSS). Cronbach’s alpha was used to check internal reliability. Normality testing, correlations and multivariate regressions were adopted to analyse the variables.
4.1 Internal reliability
Each of the three dimensions was checked for internal reliability. The knowledge dimension reported a Cronbach’s alpha of 0.84, while attitude and behaviour had an alpha value of 0.91 and 0.77, respectively, which indicated good to excellent levels of internal consistency in the subscales.
4.2 Data normality
The moderating effects of education level and work exposure on Internet security knowledge, attitude, and behaviour variables were investigated in this study.
The education level was divided into four levels: freshmen (low education level), sophomores and juniors (medium education level), seniors (higher-medium education level), and postgraduates (advanced education level). Meanwhile, work exposure was classified as follows: non-graduation years (low exposure), graduation years (medium exposure), and working graduates (high exposure).
The ISA variables were then examined for normality. Direct hypothesis testing was not recommended because both Kolmogorov–Smirnov and Shapiro–Wilk were designed for smaller sample sizes ( n ≤ 50) [ 54 ]. Skewness and kurtosis values, on the other hand, were determined manually. A larger sample with skewness between −2 and + 2 and kurtosis between −7 and 7 were considered normally distributed. Mean knowledge (skewness = −0.270, kurtosis = −0.393), mean attitude (skewness = −0.263, kurtosis = −0.783), and mean behaviour (skewness = −0.342, kurtosis = 0.351) were all within the normal range.
4.3 Demographic information
According to the range provided by the questionnaire, grades were segmented into six sequential levels (year 1, year 2–3, postgraduate students, young working graduates, experienced working graduates, and final year). Ages (18–25, 26–35, 36–45, 46), disciplines (liberal arts and social sciences, natural sciences, technology and engineering), and genders (male, female) were also used as classification variables.
4.4 Multicollinearity
Next, a multicollinearity test was performed on the data. Correlation tests were conducted for variables such as knowledge, attitude, behaviour, grade, age, discipline, and gender (see Table 2 for correlation results). The mean knowledge, attitude and behaviour were discovered to be significantly positive and highly correlated ( p < 0.001). There was a significant positive correlation between grade and age ( p < 0.001), and they showed significant negative correlations with mean knowledge, attitude, and behaviour ( p < 0.001). Therefore, grade and age as independent variables were considered covariables. To test the effect of grade, age has to be controlled.
As a result, the regression analysis hypothesis was completely satisfied. Therefore, the data and residuals were normally distributed, with no heteroscedasticity or correlation between regression residuals. The potential collinearity between grade and age was identified, but it could be accommodated in the proposed regression model by controlling for the latter.
4.5 Data analysis
A multivariate regression model was used to examine the effects of different grade levels and levels of work exposure on mean score of knowledge, attitude, and behaviour, with gender, age, and discipline controlled, as detailed below according to the corresponding assumptions. For simplicity, the changes are illustrated in Figs. 2 , 3 , and 4 ).
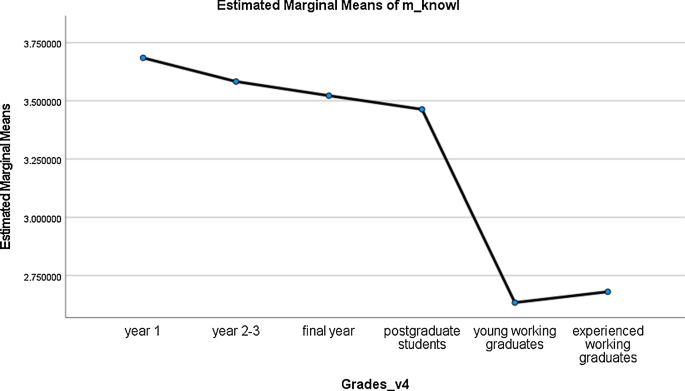
Means of cybersecurity knowledge across groups
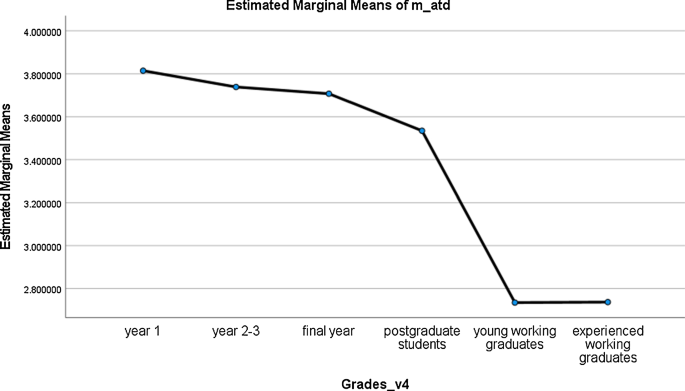
Means of cybersecurity attitude across groups
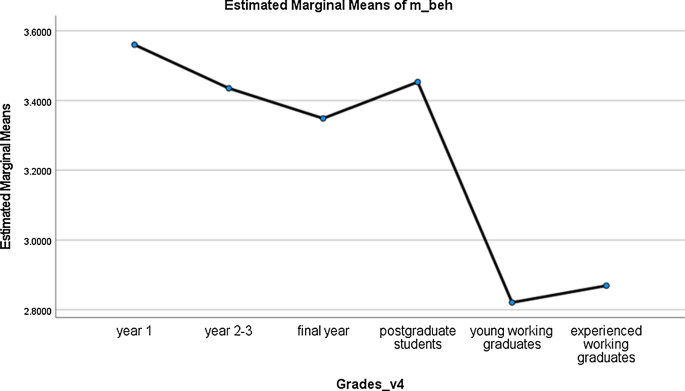
Means of cybersecurity behaviour across groups
University students of different years of study, postgraduate students and working graduates will display differing cybersecurity knowledge.
H1 was verified. There were significant differences between the knowledge of postgraduate students ( M = 3.53, SD = 0.47) and non-final-year university students including year 1 students ( M = 3.72, SD = 0.78) and year 2-3students ( M = 3.64, SD = 0.77). Moreover, the knowledge of postgraduate students was found to be different from final-year ones ( M = 3.57, SD = 0.77). At the same time, the knowledge of postgraduate students was also found to be visibly different from working graduates that contained young working graduates ( M = 2.63, SD = 0.47) and experienced working graduates ( M = 2.65, SD = 0.47). For simplicity, the differences in mean values of the three dimensions are illustrated in Table 2 .
H2a was rejected. The attitude of postgraduate students ( M = 3.73, SD = 0.69) was found to be significantly lower than final-year students ( M = 3.80, SD = 1.00).
H2b was verified. The attitude of postgraduate students was found to be significantly higher than young working graduates ( M = 2.73, SD = 0.32) and experienced working graduates ( M = 2.73, SD = 0.36).
H2c was rejected. The attitude of postgraduate students was found to be significantly lower than year 1 students ( M = 3.88, SD = 1.00), and year 2–3students ( M = 3.77, SD = 1.02).
H3a was verified. The behaviour of postgraduate students ( M = 3.54, SD = 0.47) was found to be significantly higher than final-year ones ( M = 3.39, SD = 0.82).
H3b was verified. The behaviour of postgraduate students was found to be significantly higher than working graduates containing young ( M = 2.83, SD = 0.28) and experienced ( M = 2.88, SD = 0.28).
H3c was partially verified. The behaviour of postgraduate students was found to be significantly lower than year 1 students ( M = 3.62, SD = 0.82), but significantly higher than that of year 2–3students ( M = 3.50, SD = 0.85).
In terms of knowledge, as students advanced to higher levels, their cybersecurity knowledge deteriorated. Their cybersecurity knowledge also dropped significantly at work. As graduates gained more work experience, their knowledge seemed to rebound a little. In terms of behaviour, postgraduate students performed similarly to year 2–3 students but not as well as year-1 students. Working graduates performed similarly poorly, but slight improvements could be seen after gaining more work experience. In terms of attitude, as students advanced to higher levels as postgraduate students, or went to work, their cybersecurity attitudes deteriorated. Even inexperienced employees showed no improvement.
4.6 Gender difference
Many studies have hypothesised that personal factors such as gender influence behavioural decisions [ 37 , 41 ]. The analysis revealed that the hypothesis was statistically supported. Females' knowledge ( M = 3.44, SD = 0.78) was found to be significantly higher than males' ( M = 3.27, SD = 0.78), as was their attitude ( M = 3.66, SD = 0.94 vs M = 3.32, SD = 0.99). The same was true for female and male behaviour ( M = 3.44, SD = 0.70 vs M = 3.19, SD = 0.81). As a result, females had higher ISA scores than males in all three dimensions.
4.7 Significant predictors
A multivariate regression model was also run to see if age, discipline, grade, or gender were significant predictors of ISA. Previous research suggested that age was a significant predictor of ISA (e.g. [ 55 ]), but a recent study found that age was not a significant predictor [ 7 ]. Pillai's Trace was used to evaluate the sum of the matrix's eigenvalues, and Roy's Largest Root statistic, to test the maximum value in a matrix's eigenvalues, have been used. And the greater the magnitude of these values, the greater the contribution of this effect to the model. Wilk's Lambda was also used, with a value between 0 and 1. In Wilk's Lambda, the smaller the value, the greater the contribution. As a result of the above analysis, the results showed that overall, grade and gender were significant predictors of ISA, whereas age and discipline were not.
4.8 Significant correlations
In addition, tests of between-subjects effects were examined further, with mean knowledge, mean attitude, and mean behaviour as the dependent variables.
Significant correlations were discovered between grades, discipline, and gender.
According to the Sum of Squares, the grade had the greatest impact on knowledge (112.96), attitude (123.35), and behaviour (70.33), in that order. (See Table 3 .)
4.9 Discipline differences
To the best of the authors' knowledge, ISA research in higher education rarely focuses on discipline. Thus, Fisher's LSD was used in this study for inter-group multiple comparisons to compare disciplines. The analysis found no difference between the disciplines of natural sciences and technology and engineering, but there was a difference between the above two disciplines and the discipline of liberal arts and social sciences. That could be because, in Chinese universities, the disciplines of natural sciences, technology, and engineering had at least two advanced information and communication technology (ICT) courses and one professional computer application course, whereas students majoring in liberal arts and social sciences only took the common course on basic ICT skills in the first year, according to the Computer Basic Curriculum System and the actual investigation [ 55 , 56 ].
5 Summary of results
The current survey was one of the few initial studies that used students' year-level as a proxy for the impact of education level as an internal aspect, and work exposure for the influence of social education level as an external factor, both of which we consider being quantifiable sources of impact. We compared the impact of (personal) education and social education levels on year-1 university students, year-2 and year-3 university students, final-year students, postgraduate students, young working graduates and experienced working graduates. The context of this study was in China, where university graduates were more likely to work with colleagues who received less education than themselves. Differences have been found among university students, postgraduate students, and working graduates, which could suggest changes in online security knowledge, attitudes, and behaviour among individuals.
Then, there was little difference in overall ISA between graduate students and college students, implying that EL had little impact on these students. In the current context of China, the most important reason was probably that there were few computer-related courses at the higher levels of undergraduate and postgraduate study in most Chinese universities. The findings showed that above undergraduate education, the education level had minimal positive effect on postgraduates' cybersecurity behaviours, but with a negative impact on postgraduates' Internet security knowledge and attitude. To be more specific, only a few universities provided optional computer-related courses or computer-common courses to postgraduate students [ 56 , 57 ], implying that the majority of them did not receive better ICT education at the postgraduate level, despite taking the basic ICT course at their first year as undergraduate students [ 55 , 56 ]. As a result, higher levels of undergraduate and postgraduate students' information and communication technology (ICT) knowledge could not be enriched, and thus could not positively influence their attitudes. After all, ICT education could be beneficial to one's ISA [ 35 , 36 ]. This also explains the differences between students majoring in disciplines of natural sciences/technology/engineering and liberal arts/social sciences, as liberal arts/social sciences students may have only taken one basic ICT course. Furthermore, females had higher ISA scores than males in all three dimensions, while age was not a significant predictor of ISA.
6 Discussion and implications
6.1 discussion.
To our surprise, there was little difference in overall ISA between graduate students and college students, implying that EL had little impact on these students. What we did not expect was that education level had no positive effect on postgraduates' cybersecurity behaviours, while it had a negative impact on postgraduates' Internet security knowledge and attitude. This result differed from previous international findings that individuals with higher education had higher ISA scores [ 9 , 51 ] but was consistent with previous findings in the Chinese context [ 7 , 10 , 11 ]. Among past studies that investigated both undergraduate and postgraduate students [ 58 , 59 , 60 ], very few compared them as separate groups. However, our results echo findings that undergraduate and postgraduate students had similarly inadequate ISA [ 60 ] or that the postgraduates had slightly weaker awareness than the undergraduate group [ 58 ]. ICT education is known to benefit to one's ISA [ 35 , 36 ], but computer-related courses are typically not provided at higher undergraduate levels and postgraduate study in most Chinese universities. Many tertiary-level students in China were only required to take a basic ICT course in year-1 [ 55 , 56 ], the lack of ICT education in subsequent years and postgraduate programmes might have led to ISA deterioration. In fact, similarly inadequate training/education was found in non-Chinese institutes [ 60 ]. Although this is one of the few studies that investigated comprehensive ISA changes among university students and graduates, other studies have indicated that senior-year students were more prone to risky online behaviours [ 61 ] and weak preventative awareness of cyber-threats [ 62 ]. Increasing involvement in social activities such as interest groups and part-time jobs is potentially a major contributor to such peer-level changes, as argued by researchers [ 7 , 62 ]. This social influence reaches a high point upon complete detachment from tertiary education, while others who choose to continue/return to their studies at postgraduate level may receive a less negative impact.
We also argue one major reason for existing ISA studies to yield contradicting results was that they only examined individual factors [ 34 , 35 , 40 , 41 ], which should be considered alongside the influence of social forces [ 7 , 12 , 47 ] to paint a fuller picture. This study found that when all the factors (i.e. grades, age, discipline, and gender) were analysed together, age did not show significant correlations with any ISA dimensions. Our results indicated that year-levels/work exposure and age were highly correlated, which makes sense as the higher one’s education level and work experience are, the older they typically are. In past studies, age alone seemed to predict ISA [ 34 , 37 , 41 ]. However, it is evident in this study that age is not the cause for changes in ISA, but rather, a co-founder or covariate of other directly related factors such as education levels. In particular, social influence is found to be more important than an individual's level of education. Although this study has provided further evidence of social influence [ 19 , 21 ], much has yet to be done to examine how the external environment impacts one’s ISA as studies beyond individual factors are relatively few [ 12 , 45 , 47 ]. This also explains why the results of previous studies that used GDP and country development to predict citizens' ISA were inconsistent [ 37 , 57 , 63 ], suggesting that the educational level of the whole country (SEL) plays a crucial role. Previous studies have argued that social influence may be more pronounced in developing countries than developed ones [ 12 , 64 , 65 ]. Apart from weaker cybersecurity infrastructure [ 65 ], the general lack of basic knowledge in ICT [ 64 ], added to the rampant access to pirate software [ 66 ], shaped people’s risk-prone attitudes in developing countries. Despite the high GDP, China is a developing country, where people’s education level and ICT knowledge vary largely [ 7 , 49 ]. University students, graduates and postgraduates are undoubtedly under constant but varying levels of negative social influence, which, if strong enough, can result in a critical downturn of ISA.
Further, as expected, females had better attitudes and behaviour than males, which was consistent with previous findings [ 37 , 40 ]. Another expected outcome was that there was a significant difference between liberal arts, social sciences and natural sciences, technology, and engineering which was rarely focused on in the previous studies. The reason was that different levels and amounts of ICT curriculum were designed among these disciplines, which also proved the previous finding that ICT education could be beneficial to one's ISA [ 35 , 36 ].
6.2 Implications
An important methodological implication for the future was that, with readily available figures of national education level, the year-level (education level) and length of time at work (SEL) were quantifiable measures, similar to existing cross-national findings (e.g. [ 23 , 58 , 59 ]). As a result, it addresses the issue that GDP and nationality are inconsistent predictors of national ISA.
Because of the influence of SEL, the fact that the average difference in ISA between working graduates and the other two student groups was significant provided insight into the importance of conducting a survey on working postgraduates and working PhDs, given that the SEL of the working environment for working postgraduates may be better. Nonetheless, the behaviour of postgraduate students was significantly higher than that of final-year students, indicating that the negative effects of society (SEL) were offset by the positive effects of the university environment (education level). With this in mind, an ISA survey of PhD students was undoubtedly a worthwhile topic worthy of further investigation.
This study recommends that a greater effort is needed to be made to educate the general public, particularly while they were still in school. With 95.41% of the population in China having received an education, cybersecurity awareness training at the school level was critical to improving the national ISA and SEL. What was more important was the higher education group's knowledge of ICT. The ICT knowledge that remained only in the freshmen stage could no longer meet the current era of extensive and in-depth popularization of the Internet, and thus the ICT knowledge needed to be provided according to the needs of students at various stages, including the postgraduate stage. Simultaneously, because teachers' ICT knowledge can influence the younger generation, the quickest and most effective strategy was to train the teacher population on ICT knowledge [ 67 ].
Meanwhile, companies should consider providing more training for employees with lower education, who make up 48.8% of the workforce in China, as well as maintaining young graduates' better ISAs, to ensure that all employees have a broad range of ICT knowledge. Simultaneously, it was critical for the government to take actions to promote safe Internet behaviours among citizens, such as broadcasting knowledge and the importance of ISA via social media, online advertising, television, government-led talks, establishing network security policies, and collaborating with businesses to initiate social transformation.
7 Limitations and conclusions
7.1 limitations.
The study collected a larger sample in the hope that a larger sample would increase generalizability and thus mitigate the problem of non-random sampling. However, due to the varying difficulty of data collection in each group, the number of respondents in each of the six groups varied.
We attempted to understand changes in ISA and behaviour from year-1 to postgraduate studies to graduated employees. However, the change is not observed longitudinally, but it was obtained through a cross-sectional survey. Therefore, factors such as generation difference cannot be duly represented in the data. Readers are forewarned of this potential confounder.
Future research could also conduct longitudinal and cross-country studies to investigate the influence of education level and SEL on ISA using this extended model to further our understanding of education level and SEL.
7.2 Conclusions
This study used an extended KAB model, which proposed that year-level (grade) could act as a moderator in the relationship between knowledge and attitude. As a result, three main hypotheses and three sub-hypotheses were proposed based on the respondents' various conditions. The postgraduate student group has the highest education level, while the final-year students and non-final-year students decrease in order. These conditions represent various levels of educational influence. At the same time, postgraduate students return to the university environment after having had some contact with work, representing the group that has been influenced by both education level and SEL.
On this basis, the Human Aspects of Information Security Questionnaire (HAIS-Q) was used to assess the extended KAB model. The effect of grade and work exposure on mean knowledge, attitude, and behaviour scores was investigated. The findings confirmed that education level and SEL had a statistically significant effect on variables.
Three major hypotheses were found to be correct. H1-University students of various years of study, postgraduate students, and working graduates will demonstrate varying levels of cybersecurity knowledge was confirmed. There were significant differences in knowledge among university students of various years of study, postgraduate students, and working graduates.
H2a–H2c examined whether there would be statistically significant differences in attitudes among four groups of respondents. Non-final-year students performed better than the other three groups. Furthermore, final-year students had higher scores than postgraduate students, and postgraduate students had higher scores than working graduates.
H3a–H3c examined whether the behaviours of four groups of respondents will differ. Postgraduate students were found to score higher than final-year students and working graduates, but their scores were comparable to non-final-year students.
The current study was one of the first to compare postgraduate students with university students and working graduates in order to examine the cognitive and behavioural changes of well-educated individuals. It contributed to methodology and practice guidance. The findings of this study need to be confirmed by additional research, but it could serve as a starting point for future investigations.
Data Availability
Datasets are available in a funder-mandated or public (institutional, general, or subject-specific) repository that assigns persistent identifiers to datasets. A submission to the journal implies that materials described in the manuscript, including all relevant raw data, will be freely available to any researcher wishing to use them for non-commercial purposes, without breaching participant confidentiality. The datasets generated during and/or analysed during the current study are available in the Figshare repository, https://figshare.com/s/b840bda7b96e12ce93a7 .
Rahim, N.H.A., Hamid, S., Mat Kiah, M.L., Shamshirband, S., Furnell, S.: A systematic review of approaches to assessing cybersecurity awareness. Kybernetes 44 , 606–622 (2015). https://doi.org/10.1108/k-12-2014-0283
Article Google Scholar
Ajzen, I.: The theory of planned behavior. Organ. Behav. Hum. Decis. Process. 50 , 179–211 (1991). https://doi.org/10.1016/0749-5978(91)90020-t
Rogers, E.M.: Diffusion of Innovations. Free Press, New York (2003)
Google Scholar
Kruger, H.A., Kearney, W.D.: A prototype for assessing information security awareness. Comput. Secur. 25 , 289–296 (2006). https://doi.org/10.1016/j.cose.2006.02.008
McCormac, A., Calic, D., Butavicius, M., Parsons, K., Zwaans, T., Pattinson, M.: A reliable measure of information security awareness and the identification of bias in responses. Australas. J. Inf. Syst. (2017). https://doi.org/10.3127/ajis.v21i0.1697
Parsons, K., McCormac, A., Butavicius, M., Pattinson, M., Jerram, C.: Determining employee awareness using the human aspects of information security questionnaire (HAIS-Q). Comput. Secur. 42 , 165–176 (2014). https://doi.org/10.1016/j.cose.2013.12.003
Hong, W.C.H., Chi, C., Liu, J., Zhang, Y., Lei, V.N.-L., Xu, X.: The influence of social education level on cybersecurity awareness and behaviour: a comparative study of university students and working graduates. Educ. Inf. Technol. (2022). https://doi.org/10.1007/s10639-022-11121-5
Ulven, J.B., Wangen, G.: A systematic review of cybersecurity risks in higher education. Future Internet 13 , 39 (2021). https://doi.org/10.3390/fi13020039
Wiley, A., McCormac, A., Calic, D.: More than the individual: examining the relationship between culture and information security awareness. Comput. Secur. 88 , 101640 (2020). https://doi.org/10.1016/j.cose.2019.101640
Li, Y.-L., Li, Y., Li, A.: A study on college students’ internet information ethics cognition and influencing factors [大学生网络信息伦理认知与影响因素研究]. Inf. Doc. Work 35 , 10–16 (2014)
Huang, X., He, W., Hua, C., Shang, Y.: The Statistical Analysis about Status and Influencing Factors of University Students’ Safety Accidents. Statistical and Application [高校学生安全事故发生状况及其影响因素的统计分析]. 3, 57–67 (2014). https://doi.org/10.12677/sa.2014.32009
Elkhannoubi, H., & Belaissaoui, M.: Assess developing countries’ cybersecurity capabilities through a social influence strategy. In: 2016 7th International Conference on Sciences of Electronics, Technologies of Information and Telecommunications (SETIT) pp.19–23 IEEE. (2016). https://doi.org/10.1109/SETIT.2016.7939834
Fishbein, M., Ajzen, I.: Belief, attitude, intention, and behavior: an Introduction to Theory and Research. Addison-Wesley Pub. Co, Reading, Mass. (1975)
Vance, A.: Why Do Employees Violate Is Security policies?: Insights from Multiple Theoretical Perspectives, http://urn.fi/urn:isbn:9789514262876
Ng, B.-Y., Kankanhalli, A., Xu, Y.: (Calvin): studying users’ computer security behavior: a health belief perspective. Decis. Support Syst. 46 , 815–825 (2009). https://doi.org/10.1016/j.dss.2008.11.010
Fan, J., Zhang, P.: Study on e-government Information Misuse Based on General Deterrence Theory. In: ICSSSM11. pp. 1–6. IEEE Institute of Electrical & Electronic Engineers (2011)
Mathieson, K.: Predicting user intentions: comparing the technology acceptance model with the theory of planned behaviour. Inf. Syst. Res. 2 , 173–191 (1991). https://doi.org/10.1287/isre.2.3.173
Siponen, M.T.: A conceptual foundation for organizational information security awareness. Inf. Manag. Comput. Secur. 8 , 31–41 (2000). https://doi.org/10.1108/09685220010371394
Heirman, W., Walrave, M., Vermeulen, A., Ponnet, K., Vandebosch, H., Hardies, K.: Applying the theory of planned behavior to adolescents’ acceptance of online friendship requests sent by strangers. Telemat. Inform. 33 , 1119–1129 (2016). https://doi.org/10.1016/j.tele.2016.01.002
Chandarman, R., Van Niekerk, B.: Students’ cybersecurity awareness at a private tertiary educational institution. Afr. J. Inf. Commun. (2017). https://doi.org/10.23962/10539/23572
Ifinedo, P.: Understanding information systems security policy compliance: an integration of the theory of planned behavior and the protection motivation theory. Comput. Secur. 31 , 83–95 (2012). https://doi.org/10.1016/j.cose.2011.10.007
Anderson, C., Agarwal, R.: Practicing safe computing: a multimethod empirical examination of home computer user security behavioral intentions. MIS Q. 34 , 613 (2010). https://doi.org/10.2307/25750694
Chen, Y., Zahedi, F.M.: Individuals’ internet security perceptions and behaviors: polycontextual contrasts between the United States and China. MIS Q. 40 , 205–222 (2016). https://doi.org/10.25300/misq/2016/40.1.09
Warkentin, M., Johnston, A.C., Shropshire, J., Barnett, W.D.: Continuance of protective security behavior: a longitudinal study. Decis. Support Syst. 92 , 25–35 (2016). https://doi.org/10.1016/j.dss.2016.09.013
Herath, T., Rao, H.R.: Protection motivation and deterrence: a framework for security policy compliance in organisations. Eur. J. Inf. Syst. 18 , 106–125 (2009). https://doi.org/10.1057/ejis.2009.6
Herath, T., Rao, H.R.: Encouraging information security behaviors in organizations: role of penalties, pressures and perceived effectiveness. Decis. Support Syst. 47 , 154–165 (2009). https://doi.org/10.1016/j.dss.2009.02.005
Roberts, S.A.: Exploring the Relationships between User Cybersecurity Knowledge, Cybersecurity and Cybercrime Attitudes, and Online Risky Behaviors, https://www.proquest.com/openview/c1c31d84698165e5843133986323a773/1.pdf?pq-origsite=gscholar&cbl=18750&diss=y , (2021)
De-Graft Aikins, A., Boynton, P., Atanga, L.L.: Developing effective chronic disease interventions in Africa: insights from Ghana and Cameroon. Glob. Health (2010). https://doi.org/10.1186/1744-8603-6-6
Schrader, P.G., Lawless, K.A.: The knowledge, attitudes, & behaviors approach how to evaluate performance and learning in complex environments. Perform. Improv. 43 , 8–15 (2004). https://doi.org/10.1002/pfi.4140430905
Parsons, K., Calic, D., Pattinson, M., Butavicius, M., McCormac, A., Zwaans, T.: The human aspects of information security questionnaire (HAIS-Q): two further validation studies. Comput. Secur. 66 , 40–51 (2017). https://doi.org/10.1016/j.cose.2017.01.004
Zwilling, M., Klien, G., Lesjak, D., Wiechetek, Ł, Cetin, F., Basim, H.N.: Cyber security awareness, knowledge and behavior: a comparative study. J. Comput. Inf. Syst. 62 , 1–16 (2020). https://doi.org/10.1080/08874417.2020.1712269
Worsley, A.: Nutrition knowledge and food consumption: can nutrition knowledge change food behaviour? Asia Pac. J. Clin. Nutr. 11 , S579–S585 (2002). https://doi.org/10.1046/j.1440-6047.11.supp3.7.x
Parsons, K., McCormac, A., Pattinson, M.R., Butavicius, M.A., Jerram, C.: An Analysis of Information Security Vulnerabilities at Three Australian Government Organisations, In Furnell, S. M., Clarke, N. L. & Katos, V (Eds). Proceedings of the European Information Security Multi-Conference (EISMC 2013). 34–44 (2013)
McCormac, A., Calic, D., Parsons, K., Butavicius, M., Pattinson, M., Lillie, M.: The effect of resilience and job stress on information security awareness. Inf. Comput. Secur. 26 , 277–289 (2018). https://doi.org/10.1108/ics-03-2018-0032
Bostan, A., Akman, I.: Impact of Education on Security Practices in ICT. Tehnicki Vjesnik—Technical Gazette. 22, 161–168 (2015). https://doi.org/10.17559/tv-20140403122930
Brilingaitė, A., Bukauskas, L., Juozapavičius, A.: A framework for competence development and assessment in hybrid cybersecurity exercises. Comput. Secur. 88 , 101607 (2020). https://doi.org/10.1016/j.cose.2019.101607
Pattinson, M., Butavicius, M., Parsons, K., McCormac, A., Calic, D.: Managing information security awareness at an Australian bank: a comparative study. Inf. Comput. Secur. 25 , 181–189 (2017). https://doi.org/10.1108/ics-03-2017-0017
Shropshire, J., Warkentin, M., Sharma, S.: Personality, attitudes, and intentions: predicting initial adoption of information security behavior. Comput. Secur. 49 , 177–191 (2015). https://doi.org/10.1016/j.cose.2015.01.002
Hadlington, L., Parsons, K.: Can cyberloafing and internet addiction affect organizational information security? Cyberpsychol. Behav. Soc. Netw. 20 , 567–571 (2017). https://doi.org/10.1089/cyber.2017.0239
Chaudhary, S., Zhao, Y., Berki, E., Valtanen, J., Li, L., Helenius, M., Mystakidis, S.: A cross-cultural and gender-based perspective for online security: exploring knowledge, skills and attitudes of higher education students. IADIS Int. J. WWW/Internet 13 , 57–71 (2015)
Cain, A.A., Edwards, M.E., Still, J.D.: An exploratory study of cyber hygiene behaviors and knowledge. J. Inf. Secur. Appl. 42 , 36–45 (2018). https://doi.org/10.1016/j.jisa.2018.08.002
Choi, M., Levy, Y., & Anat, H.: The Role of User Computer Self-Efficacy, Cybersecurity Countermeasures Awareness, and Cybersecurity Skills Influence on Computer Misuse. In Proceedings of the Eighth Pre-ICIS Workshop on Information Security and Privacy. 1. December (2013)
Brilingaitė, A., Bukauskas, L., Juozapavičius, A.: A framework for competence developmentand assessment in hybrid cybersecurity exercises. Comput. Secur. 88 , 1–13 (2020). https://doi.org/10.1016/j.cose.2019.101607
Sheng, S., Holbrook, M., Kumaraguru, P., Cranor, L. F., & Downs, J.: Who falls for phish? A demographic analysis of phishing susceptibility and effectiveness of interventions. In: Proceedings of the SIGCHI Conference on Human Factors in Computing Systems pp. 373–382 (2010)
Das, S.: Social cybersecurity: understanding and leveraging social influence to increase security sensitivity. It-inf. Technol 58 (5), 237–245 (2016). https://doi.org/10.1515/itit-2016-0008
Rader, E., Wash, R., & Brooks, B.: Stories as informal lessons about security. In: Proceedings of the Eighth Symposium on Usable Privacy and Security, pp. 1–17 (2012). https://doi.org/10.1145/2335356.2335364
Kam, H.-J., Mattson, T., Goel, S.: A cross industry study of institutional pressures on organizational effort to raise information security awareness. Inf. Syst. Front. 22 , 1241–1264 (2020). https://doi.org/10.1007/s10796-019-09927-9
Watson, H., Moju-Igbene, E., Kumari, A., Das, S.: “We Hold Each Other Accountable”: unpacking How Social Groups Approach Cybersecurity and Privacy Together. Proceedings of the 2020 CHI Conference on Human Factors in Computing Systems. (2020). https://doi.org/10.1145/3313831.3376605
United Nations Development Programme. Human Development Report 2020—The next frontier:Human Development and the Anthropocene. (2020). http://hdr.undp.org/sites/default/files/hdr2020.pdf . Accessed 12 July 2021
National Bureau of Statistics of China. 2020 China statistical yearbook. China Statistics Press. (2021). http://www.stats.gov.cn/tjsj/ndsj/2020/indexeh.htm . Accessed 2 Aug 2021
Aivazpour, Z., Rao, V.S.: (Chino): information disclosure and privacy paradox. ACM SIGMIS Database DATABASE Adv. Inf. Syst. 51 , 14–36 (2020). https://doi.org/10.1145/3380799.3380803
Lipsitz, S.R., Parzen, M.: Sample size calculations for non-randomized studies. Statistician 44 , 81 (1995). https://doi.org/10.2307/2348619
Mei, B., Brown, G.T.L.: Conducting online surveys in China. Soc. Sci. Comput. Rev. 36 , 721–734 (2017). https://doi.org/10.1177/0894439317729340
Razali, N.M., Wah, Y.B.: Power comparisons of Shapiro-Wilk, Kolmogorov-Smirnov, Lilliefors and Anderson-Darling tests. J. Stat. Model. Anal. 2 , 21–33 (2011)
Jin, R.: Discussion on the Teaching Reform of Computer Fundamental Course for non-computer Majors in Applied Universities [应用型本科院校非计算机专业《计算机基础》教学改革探讨]. Fujian Comput. 10, 174–175 (2018). https://doi.org/10.16707/j.cnki.fjpc.2018.10.088
Chen, S.: Research on VC + + Curriculum Construction for Non-computer Major Postgraduate Students [非计算机专业研究生 VC + +课程建设研究]. J. Lanzhou Inst. Educ. 35, 80–81, 145 (2019)
A. Farooq, J. Isoaho, S. Virtanen and J. Isoaho, "Information security awareness in educational institution: an analysis of students' individual factors," IEEE Trustcom/BigDataSE/ISPA, 2015, pp. 352–359 (2015) https://doi.org/10.1109/Trustcom.2015.394
Alqahtani, M.A.: Cybersecurity awareness based on software and e-mail security with statistical analysis. Comput. Intell. Neurosci. (2022). https://doi.org/10.1155/2022/6775980
Mutunhu, B., Dube, S., Ncube, N., & Sibanda, S.: Cyber Security Awareness and Education Framework for Zimbabwe Universities: A Case of National University of Science and Technology. Proceedings of the International Conference on Industrial Engineering and Operations Management Nsukka, Nigeria, 5–7 April, 2022
Moallem, A.: Cyber security awareness among college students. In International conference on applied human factors and ergonomics, pp. 79–87. Springer, New York (2018) https://doi.org/10.1007/978-3-319-94782-2_8
Li, Y.-L., Li, Y., & Li, A. A study on college Students’ internet information ethics cognition and influencing factors [大学生网络信息伦理认知与影响因素研究]. Information and Documentation Work, 35(2), 10–16 (2014). http://qbzl.ruc.edu.cn/EN/abstract/abstract669.shtml Accessed 10 June 2022
Sun, W.: Investigation of Safety Consciousness of University Students in Dalian City [大连市大学生网络安全意识调查研究]., (2018)
Berki, E., Kandel, C., Zhao, Y., Chaudhary, S.: Comparative study of cyber-security knowledge in higher education institutes of five countries. Educ. Comput. Sci. (2017). https://doi.org/10.21125/edulearn.2017.1591
Senali, M. G., Cripps, H., Meek, S., & Ryan, M. M.: A comparison of Australians, Chinese and Sri Lankans' payment preference at point-of-sale. Market. Intell. Plan. 40(1), 18–32 (2021). https://doi.org/10.1108/MIP-07-2021-0235
Mezzour, G., Carley, K.M., Carley, L.R.: An empirical study of global malware encounters. In: Proceedings of the 2015 Symposium and Bootcamp on the Science of Security. ACM, p. 8, (2015)
Gantz, J.F., Vavra, T., Lim, V.: Unlicensed Software and Cybersecurity Threats, BSA- The Software Alliance, January (2015)
Zhao, J., Xu, F.: The state of ICT education in China: a literature review. Front. Educ. China 5 , 50–73 (2010). https://doi.org/10.1007/s11516-010-0006-1
Download references
Acknowledgements
We are thankful to Dr. ChanFai Man (ORCID: 0000-0002-2458-1992) who provided expertise that greatly assisted the research.
National Social Science Foundation of China (NSSF) Post-grant Project (21FJKB013).
Author information
Authors and affiliations.
International Business School, Chengdu Institute Sichuan International Studies University, Chengdu, China
Centre for Teaching and Learning Enhancement, Macao Institute for Tourism Studies, Macau, Macao
Wilson Cheong Hin Hong
School of Foreign Studies, Wenzhou University, Wenzhou, China
XiaoShu Xu & Kimberly Kolletar-Zhu
Stamford International University, Bangkok, Thailand
Centre for Portuguese Studies, Macau Polytechnic University, Macau, Macao
Yunfeng Zhang
You can also search for this author in PubMed Google Scholar
Contributions
All authors contributed to the study conception and design. Material preparation, data collection and analysis were performed by Qin Ana and Cheong Hin Hong. The first draft of the manuscript was written by Qin Ana and all authors commented on previous versions of the manuscript. Conceptualization: Qin Ana; Methodology: Xiaoshu Xu; Formal analysis and investigation: Cheong Hin Hong and Xiaoshu Xu; Writing - original draft preparation: Qin Ana; Writing - review and editing: Xiaoshu Xu, Kimberly Kolletar-Zhu; Resources: Yunfeng Zhang, Kimberly Kolletar-Zhu; Supervision: Cheong Hin Hong. All authors read and approved the final manuscript.
Corresponding author
Correspondence to XiaoShu Xu .
Ethics declarations
Conflict of interest.
The authors have no relevant financial or non-financial interests to disclose. The authors have no competing interests to declare that are relevant to the content of this article. All authors certify that they have no affiliations with or involvement in any organization or entity with any financial interest or non-financial interest in the subject matter or materials discussed in this manuscript. The authors have no financial or proprietary interests in any material discussed in this article. The authors declare no competing interests.
Additional information
Publisher's note.
Springer Nature remains neutral with regard to jurisdictional claims in published maps and institutional affiliations.
Rights and permissions
Springer Nature or its licensor (e.g. a society or other partner) holds exclusive rights to this article under a publishing agreement with the author(s) or other rightsholder(s); author self-archiving of the accepted manuscript version of this article is solely governed by the terms of such publishing agreement and applicable law.
Reprints and permissions
About this article
An, Q., Hong, W.C.H., Xu, X. et al. How education level influences internet security knowledge, behaviour, and attitude: a comparison among undergraduates, postgraduates and working graduates. Int. J. Inf. Secur. 22 , 305–317 (2023). https://doi.org/10.1007/s10207-022-00637-z
Download citation
Accepted : 11 November 2022
Published : 24 November 2022
Issue Date : April 2023
DOI : https://doi.org/10.1007/s10207-022-00637-z
Share this article
Anyone you share the following link with will be able to read this content:
Sorry, a shareable link is not currently available for this article.
Provided by the Springer Nature SharedIt content-sharing initiative
- Cybersecurity awareness
- Internet security awareness
- Social educational level
- Online learning
- Find a journal
- Publish with us
- Track your research

An official website of the United States government
The .gov means it’s official. Federal government websites often end in .gov or .mil. Before sharing sensitive information, make sure you’re on a federal government site.
The site is secure. The https:// ensures that you are connecting to the official website and that any information you provide is encrypted and transmitted securely.
- Publications
- Account settings
Preview improvements coming to the PMC website in October 2024. Learn More or Try it out now .
- Advanced Search
- Journal List
- J Adv Med Educ Prof
- v.4(4); 2016 Oct
Effective Teaching Methods in Higher Education: Requirements and Barriers
Nahid shirani bidabadi.
1 Psychology and Educational Sciences School, University of Isfahan, Isfahan, Iran;
AHMMADREZA NASR ISFAHANI
Amir rouhollahi.
2 Department of English, Management and Information School, Isfahan University of Medical Science, Isfahan, Iran;
ROYA KHALILI
3 Quality Improvement in Clinical Education Research Center, Education Development Center, Shiraz University of Medical Sciences, Shiraz, Iran
Introduction:
Teaching is one of the main components in educational planning which is a key factor in conducting educational plans. Despite the importance of good teaching, the outcomes are far from ideal. The present qualitative study aimed to investigate effective teaching in higher education in Iran based on the experiences of best professors in the country and the best local professors of Isfahan University of Technology.
This qualitative content analysis study was conducted through purposeful sampling. Semi-structured interviews were conducted with ten faculty members (3 of them from the best professors in the country and 7 from the best local professors). Content analysis was performed by MAXQDA software. The codes, categories and themes were explored through an inductive process that began from semantic units or direct quotations to general themes.
According to the results of this study, the best teaching approach is the mixed method (student-centered together with teacher-centered) plus educational planning and previous readiness. But whenever the teachers can teach using this method confront with some barriers and requirements; some of these requirements are prerequisite in professors' behavior and some of these are prerequisite in professors’ outlook. Also, there are some major barriers, some of which are associated with the professors’ operation and others are related to laws and regulations. Implications of these findings for teachers’ preparation in education are discussed.
Conclusion:
In the present study, it was illustrated that a good teaching method helps the students to question their preconceptions, and motivates them to learn, by putting them in a situation in which they come to see themselves as the authors of answers, as the agents of responsibility for change. But training through this method has some barriers and requirements. To have an effective teaching; the faculty members of the universities should be awarded of these barriers and requirements as a way to improve teaching quality. The nationally and locally recognized professors are good leaders in providing ideas, insight, and the best strategies to educators who are passionate for effective teaching in the higher education. Finally, it is supposed that there is an important role for nationally and locally recognized professors in higher education to become more involved in the regulation of teaching rules.
Introduction
Rapid changes of modern world have caused the Higher Education System to face a great variety of challenges. Therefore, training more eager, thoughtful individuals in interdisciplinary fields is required ( 1 ). Thus, research and exploration to figure out useful and effective teaching and learning methods are one of the most important necessities of educational systems ( 2 ); Professors have a determining role in training such people in the mentioned field ( 3 ). A university is a place where new ideas germinate; roots strike and grow tall and sturdy. It is a unique space, which covers the entire universe of knowledge. It is a place where creative minds converge, interact with each other and construct visions of new realities. Established notions of truth are challenged in the pursuit of knowledge. To be able to do all this, getting help from experienced teachers can be very useful and effective.
Given the education quality, attention to students’ education as a main product that is expected from education quality system is of much greater demand in comparison to the past. There has always been emphasis on equal attention to research and teaching quality and establishing a bond between these two before making any decision; however, studies show that the already given attention to research in universities does not meet the educational quality requirements.
Attention to this task in higher education is considered as a major one, so in their instruction, educators must pay attention to learners and learning approach; along with these two factors, the educators should move forward to attain new teaching approaches. In the traditional system, instruction was teacher-centered and the students’ needs and interests were not considered. This is when students’ instruction must change into a method in which their needs are considered and as a result of the mentioned method active behavior change occurs in them ( 4 ). Moreover, a large number of graduated students especially bachelor holders do not feel ready enough to work in their related fields ( 5 ). Being dissatisfied with the status quo at any academic institution and then making decision to improve it require much research and assistance from the experts and pioneers of that institute. Giving the aforementioned are necessary, especially in present community of Iran; it seems that no qualitative study has ever been carried out in this area drawing on in-depth reports of recognized university faculties; therefore, in the present study the new global student-centered methods are firstly studied and to explore the ideas of experienced university faculties, some class observations and interviews were done. Then, efficient teaching method and its barriers and requirements were investigated because the faculty ideas about teaching method could be itemized just through a qualitative study.
The study was conducted with a qualitative method using content analysis approach. The design is appropriate for this study because it allows the participants to describe their experiences focusing on factors that may improve the quality of teaching in their own words. Key participants in purposeful sampling consist of three nationally recognized professors introduced based on the criteria of Ministry of Science, Research and Technology (based on education, research, executive and cultural qualifications) and seven other locally recognized professors according to Isfahan University of Technology standards and students votes. The purposive sampling continued until the saturation was reached, i.e. no further information was obtained for the given concept. All the participants had a teaching experience of above 10 years ( Table 1 ). They were first identified and after making appointments, they were briefed about the purpose of the study and they expressed their consent for the interview to be performed. The lack of female nationally recognized professors among respondents (due to lack of them) are restrictions of this research.
The participants’ characteristics
The data were collected using semi-structured in-depth interviews. Interviews began with general topics, such as “Talk about your experiences in effective teaching” and then the participants were asked to describe their perceptions of their expertise. Probing questions were also used to deeply explore conditions, processes, and other factors that the participants recognized as significant. The interview process was largely dependent on the questions that arose in the interaction between the interviewer and interviewees.
In the process of the study, informed consent was obtained from all the participants and they were ensured of the anonymity of their responses and that the audio files will be removed after use; then, after obtaining permission from the participants, the interview was recorded and transcribed verbatim immediately. The interviews were conducted in a private and quiet place and in convenient time. Then, verification of documents and coordination for subsequent interviews were done. The interviews lasted for one hour on average and each interview was conducted in one session with the interviewer’s notes or memos and field notes. Another method of data collection in this study was an unstructured observation in the educational setting. The investigator observed the method of interactions among faculty members and students. The interviews were conducted from November 2014 to April 2015. Each participant was interviewed for one or two sessions. The mean duration of the interviews was 60 minutes. To analyze the data, we used MAXQDA software (version 10, package series) for indexing and charting. Also, we used qualitative content analysis with a conventional approach to analyze the data. The data of the study were directly collected from the experiences of the study participants. The codes, categories and themes were explored through an inductive process, in which the researchers moved from specific to general. The consequently formulated concepts or categories were representative of the participants’ experiences. In content analysis at first, semantic units should be specified, and then the related codes should be extracted and categorized based on their similarities. Finally, in the case of having a high degree of abstraction, the themes can be determined. In the conventional approach, the use of predetermined classes is avoided and classes and their names are allowed to directly come out of the data. To do so, we read the manuscripts and listened to the recorded data for several times until an overall sense was attained. Then, the manuscript was read word by word and the codes were extracted. At the same time, the interviews were continued with other participants and coding of the texts was continued and sub-codes were categorized within the general topics. Then, the codes were classified in categories based on their similarities ( 6 ). Finally, by providing a comprehensive description about the topics, participants, data collection and analysis procedures and limitations of the study, we intend to create transferability so that other researchers clearly follow the research process taken by the researchers.
To improve the accuracy and the rigor of the findings, Lincoln and Cuba’s criteria, including credibility, dependability, conformability, and transferability, were used ( 7 ). To ensure the accuracy of the data, peer review, the researchers’ acceptability, and the long and continuing evaluation through in-depth, prolonged, and repeated interviews and the colleague’s comments must be used ( 8 ). In addition, the findings were repeatedly assessed and checked by supervisors (expert checking) ( 9 ). In this research, the researcher tried to increase the credibility of the data by keeping prolonged engagement in the process of data collection. Then, the accuracy of data analysis was confirmed by one specialist in the field of qualitative research and original codes were checked by some participants to compare the findings with the participants’ experiences. To increase the dependability and conformability of data, maximum variation was observed in the sampling. In addition, to increase the power of data transferability, adequate description of the data was provided in the study for critical review of the findings by other researchers.
Ethical considerations
The aim of the research and interview method was explained to the participants and in the process of the study, informed consent was obtained from all the participants and they were ensured of the anonymity of their responses and that audio files were removed after use. Informed consent for interview and its recording was obtained.
The mean age of faculty members in this study was 54.8 years and all of them were married. According to the results of the study, the best teaching approach was the mixed method one (student-centered with teacher-centered) plus educational planning and previous readiness. Meaning units expressed by professors were divided into 19 codes, 4 categories and 2 themes. In the present study, regarding the Effective Teaching Method in Higher Education, Requirements and Barriers, the experiences and perceptions of general practitioners were explored. As presented in Table 2 , according to data analysis, two themes containing several major categories and codes were extracted. Each code and category is described in more details below.
Examples of extracting codes, categories and themes from raw data
New teaching methods and barriers to the use of these methods
Teachers participating in this study believed that teaching and learning in higher education is a shared process, with responsibilities on both student and teacher to contribute to their success. Within this shared process, higher education must engage the students in questioning their preconceived ideas and their models of how the world works, so that they can reach a higher level of understanding. But students are not always equipped with this challenge, nor are all of them driven by a desire to understand and apply knowledge, but all too often aspire merely to survive the course, or to learn only procedurally in order to get the highest possible marks before rapidly moving on to the next subject. The best teaching helps the students to question their preconceptions, and motivates them to learn, by putting them in a situation in which their existing model does not work and in which they come to see themselves as authors of answers, as agents of responsibility for change. That means, the students need to be faced with problems which they think are important. Also, they believed that most of the developed countries are attempting to use new teaching methods, such as student-centered active methods, problem-based and project-based approaches in education. For example, the faculty number 3 said:
“In a project called EPS (European Project Semester), students come together and work on interdisciplinary issues in international teams. It is a very interesting technique to arouse interest, motivate students, and enhance their skills (Faculty member No. 3).”
The faculty number 8 noted another project-based teaching method that is used nowadays especially to promote education in software engineering and informatics is FLOSS (Free/Liber Open Source Software). In recent years, this project was used to empower the students. They will be allowed to accept the roles in a project and, therefore, deeply engage in the process of software development.
In Iran, many studies have been conducted about new teaching methods. For example, studies by Momeni Danaie ( 10 ), Noroozi ( 11 ), and Zarshenas ( 12 ), have shown various required methods of teaching. They have also concluded that pure lecture, regardless of any feedback ensuring the students learning, have lost their effectiveness. The problem-oriented approach in addition to improving communication skills among students not only increased development of critical thinking but also promoted study skills and an interest in their learning ( 12 ).
In this study, the professors noted that there are some barriers to effective teaching that are mentioned below:
As to the use of new methods of training such as problem-based methods or project-based approach, faculty members No. 4 and 9 remarked that "The need for student-centered teaching is obvious but for some reasons, such as the requirement in the teaching curriculum and the large volume of materials and resources, using these methods is not feasible completely" (Faculty member No. 9).
"If at least in the form of teacher evaluation, some questions were allocated to the use of project-based and problem-based approaches, teachers would try to use them further" (Faculty member No. 2).
The faculty members No. 6 and 7 believed that the lack of motivation in students and the lack of access to educational assistants are considered the reasons for neglecting these methods.
"I think one of the ways that can make student-centered education possible is employing educational assistants (Faculty member No. 6).”
"If each professor could attend crowded classes with two or three assistants, they could divide the class into some groups and assign more practical teamwork while they were carefully supervised (Faculty member No. 7).”
Requirements related to faculty outlook in an effective teaching
Having a successful and effective teaching that creates long-term learning on the part of the students will require certain feelings and attitudes of the teachers. These attitudes and emotions strongly influence their behavior and teaching. In this section, the attitudes of successful teachers are discussed.
Coordination with the overall organizational strategies will allow the educational system to move toward special opportunities for innovation based on the guidelines ( 13 ). The participants, 4, 3, 5 and 8 know that teaching effectively makes sense if the efforts of the professors are aligned with the goals of university.
"If faculty members know themselves as an inseparable part of the university, and proud of their employment in the university and try to promote the aim of training educated people with a high level of scientific expertise of university, it will become their goal, too. Thus, they will try as much as possible to attain this goal" (Faculty member No.9).
When a person begins to learn, according to the value of hope theory, he must feel this is an important learning and believe that he will succeed. Since the feeling of being successful will encourage individuals to learn, you should know that teachers have an important role in this sense ( 14 ). The interviewees’ number 1, 2, 3 and 10 considered factors like interest in youth, trust in ability and respect, as motivating factors for students.
Masters 7 and 8 signified that a master had a holistic and systematic view, determined the position of the teaching subject in a field or in the entire course, know general application of issues and determines them for students, and try to teach interdisciplinary topics. Interviewee No. 5 believed that: "Masters should be aware of the fact that these students are the future of the country and in addition to knowledge, they should provide them with the right attitude and vision" (Faculty member No.5).
Participants No. 2, 4 and 8 considered the faculty members’ passion to teach a lesson as responsible and believed that: "If the a teacher is interested in his field, he/she devotes more time to study the scriptures of his field and regularly updates his information; this awareness in his teaching and its influence on students is also very effective" (Faculty member No. 8).
Requirements related to the behavior and performance of faculty members in effective teaching
Teachers have to focus on mental differences, interest, and sense of belonging, emotional stability, practical experience and scientific level of students in training. Class curriculum planning includes preparation, effective transition of content, and the use of learning and evaluating teaching ( 15 ).
Given the current study subjects’ ideas, the following functional requirements for successful teaching in higher education can be proposed.
According to Choi and Pucker, the most important role of teachers is planning and controlling the educational process for students to be able to achieve a comprehensive learning ( 16 ).
"The fact that many teachers don’t have a predetermined plan on how to teach, and just collect what they should teach in a meeting is one reason for the lack of creativity in teaching" Faculty member No.4).
Klug and colleagues in an article entitled “teaching and learning in education” raise some questions and want the faculty members to ask themselves these questions regularly.
1- How to increase the students' motivation.
2- How to help students feel confident in solving problems.
3- How to teach students to plan their learning activities.
4- How to help them to carry out self-assessment at the end of each lesson.
5- How to encourage the students to motivate them for future work.
6- How I can give feedback to the students and inform them about their individual learning ( 14 ).
Every five faculty members who were interviewed cited the need to explain the lessons in plain language, give feedback to students, and explain the causes and reasons of issues.
"I always pay attention to my role as a model with regular self-assessment; I'm trying to teach this main issue to my students" (Faculty member No. 9).
Improving the quality of learning through the promotion of education, using pre-organizers and conceptual map, emphasizing the student-centered learning and developing the skills needed for employment are the strategies outlined in lifelong learning, particularly in higher education ( 17 ).
"I always give a five to ten-minute summary of the last topic to students at first; if possible, I build up the new lesson upon the previous one" (Faculty member No. 4).
The belief that creative talent is universal and it will be strengthened with appropriate programs is a piece of evidence to prove that innovative features of the programs should be attended to continually ( 18 ). Certainly, in addition to the enumerated powers, appropriate fields should be provided to design new ideas with confidence and purposeful orientation. Otherwise, in the absence of favorable conditions and lack of proper motivations, it will be difficult to apply new ideas ( 19 ). Teacher’s No. 3, 5 and 7 emphasized encouraging the students for creativity: "I always encourage the students to be creative when I teach a topic; for example, after teaching, I express some vague hints and undiscovered issues and ask them what the second move is to improve that process" (Faculty member No.3).
Senior instructors try to engage in self-management and consultation, tracking their usage of classroom management skills and developing action plans to modify their practices based on data. Through consultation, instructors work with their colleagues to collect and implement data to gauge the students’ strengths and weaknesses, and then use protocols to turn the weaknesses into strengths. The most effective teachers monitor progress and assess how their changed practices have impacted the students’ outcomes ( 20 ).
"It is important that what is taught be relevant to the students' career; however, in the future with the same information they have learned in university, they want to work in the industry of their country" (Faculty member No.1).
Skills in documenting the results of the process of teaching-learning cannot only facilitate management in terms of studying the records, but also provides easier access to up to date information ( 21 ). Faculty members No. 7 and 3 stressed the need for documenting learning experiences by faculty.
"I have a notebook in my office that I usually refer to after each class. Then, I write down every successful strategy that was highly regarded by students that day" (Faculty member No.3).
Developing a satisfactory interaction with students
To connect with students and impact their lives personally and professionally, teachers must be student-centered and demonstrate respect for their background, ideologies, beliefs, and learning styles. The best instructors use differentiated instruction, display cultural sensitivity, accentuate open communication, offer positive feedback on the students’ academic performance ( 20 ), and foster student growth by allowing them to resubmit assignments prior to assigning a grade ( 22 ).
"I pay attention to every single student in my class and every time when I see a student in class is not focused on a few consecutive sessions, I ask about his lack of focus and I help him solve his problem" (Faculty member No. 5).
The limitation in this research was little access to other nationally recognized university faculty members; also their tight schedule was among other limitations in this study that kept us several times from interviewing such faculties. To overcome such a problem, they were briefed about the importance of this study and then some appointments were set with them.
This study revealed the effective teaching methods, requirements and barriers in Iranian Higher Education. Teachers participating in this study believed that teaching and learning in higher education is a shared process, with responsibilities on both student and teacher to contribute to their success. Within this shared process, higher education must engage the students in questioning their preconceived ideas and their models of how the world works, so that they can reach a higher level of understanding. They believed that to grow successful people to deal with the challenges in evolving the society, most developed countries are attempting to use new teaching methods in higher education. All these methods are student-centered and are the result of pivotal projects. Research conducted by Momeni Danaei and colleagues also showed that using a combination of various teaching methods together will lead to more effective learning while implementing just one teaching model cannot effectively promote learning ( 10 ). However, based on the faculty member’s experiences, effective teaching methods in higher education have some requirements and barriers.
In this study, barriers according to codes were divided two major categories: professor-related barriers and regulation-related ones; for these reasons, the complete use of these methods is not possible. However, teachers who are aware of the necessity of engaging the student for a better understanding of their content try to use this method as a combination that is class speech presentation and involving students in teaching and learning. This result is consistent with the research findings of Momeni Danaei and colleagues ( 10 ), Zarshenas et al. ( 12 ) and Noroozi ( 11 ).
Using student-centered methods in higher education needs some requirements that according to faculty members who were interviewed, and according to the codes, such requirements for effective teaching can be divided into two categories: First, things to exist in the outlook of faculties about the students and faculties' responsibility towards them, to guide them towards effective teaching methods, the most important of which are adaptation to the organizational strategies, interest in the students and trust in their abilities, systemic approach in higher education, and interest in their discipline.
Second, the necessary requirements should exist in the faculties’ behavior to make their teaching methods more effective. This category emerged from some codes, including having lesson plan; using appropriate educational strategies and metacognition training and self-assessment of students during teaching; using concept and pre-organizer maps in training, knowledge; and explaining how to resolve problems in professional career through teaching discussion, documenting of experience and having satisfactory interaction with the students. This result is consistent with the findings of Klug et al., Byun et al., and Khanyfr et al. ( 14 , 17 , 18 ).
In addition and according to the results, we can conclude that a major challenge for universities, especially at a time of resource constraints, is to organize teaching so as to maximize learning effectiveness. As mentioned earlier, a major barrier to change is the fact that most faculty members are not trained for their teaching role and are largely ignorant of the research literature on effective pedagogy. These findings are in agreement with the research of Knapper, indicating that the best ideas for effective teaching include: Teaching methods that focus on the students’ activity and task performance rather than just acquisition of facts; Opportunities for meaningful personal interaction between the students and teachers; Opportunities for collaborative team learning; More authentic methods of assessment that stress task performance in naturalistic situations, preferably including elements of peer and self-assessment; Making learning processes more explicit, and encouraging the students to reflect on the way they learn; Learning tasks that encourage integration of information and skills from different fields ( 23 ).
In the present study, it was illustrated that a good teaching method helps the students to question their preconceptions, and motivates them to learn, by putting them in a situation in which they come to see themselves as the authors of answers and the agents of responsibility for change. But whenever the teachers can teach by this method, they are faced with some barriers and requirements. Some of these requirements are prerequisite of the professors' behavior and some of these are prerequisite of the professors’ outlook. Also, there are some major barriers some of which are associated with the professors’ behavior and others are related to laws and regulations. Therefore, to have an effective teaching, the faculty members of universities should be aware of these barriers and requirements as a way to improve the teaching quality.
Effective teaching also requires structural changes that can only be brought about by academic leaders. These changes include hiring practices reward structures that recognize the importance of teaching expertise, quality assurance approaches that measure learning processes, outcomes in a much more sophisticated way than routine methods, and changing the way of attaining university accreditation.
The nationally and locally recognized professors are good leaders in providing ideas, insight, and the best strategies to educators who are passionate for effective teaching in the higher education. Finally, it is supposed that there is an important role for nationally and locally recognized professors in higher education to become more involved in the regulation of teaching rules. This will help other university teachers to be familiar with effective teaching and learning procedures. Therefore, curriculum planners and faculty members can improve their teaching methods.
Acknowledgement
The authors would like to thank all research participants of Isfahan University of Technology (faculties) who contributed to this study and spent their time to share their experiences through interviews.
Conflict of Interest: None declared.
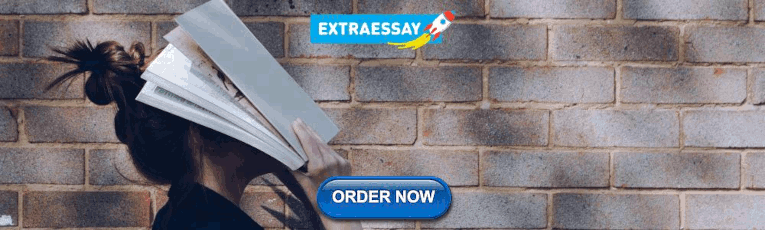
IMAGES
VIDEO
COMMENTS
URGENT NEED FOR NEW DIRECTIONS IN EDUCATION-HEALTH RESEARCH. Americans have worse health than people in other high-income countries, and have been falling further behind in recent decades ().This is partially due to the large health inequalities and poor health of adults with low education ().Understanding the health benefits of education is thus integral to reducing health disparities and ...
Education and health and wellbeing are intrinsically linked. The evidence behind the importance of education as a determinant of health is amongst the most compelling. Education is strongly associated with life expectancy, morbidity, health behaviours, and educational attainment plays an important role in health by shaping opportunities, employment, and income. In this issue of The Lancet ...
Additionally, Perdima et al. [12] analysed journal articles in the Scopus database related to educational technology in physical education. However, none of these reviews addressed studies on educational technology at the primary or elementary education level.
The American Educational Research Journal (AERJ) is the flagship journal of AERA, with articles that advance the empirical, theoretical, and methodological understanding of education and learning. It publishes original peer-reviewed analyses spanning the field of education research across all subfields and disciplines and all levels of analysis, all levels of education throughout the life span ...
A clear understanding of the macro-level contexts in which education impacts health is integral to improving national health administration and policy. In this research, we use a visual analytic approach to explore the association between education and health over a 20-year period for countries around the world. Using empirical data from the OECD and the World Bank for 26 OECD countries for ...
Education is a process and a product.From a societal perspective, the process of education (from the Latin, ducere, "to lead," and e, "out from," yield education, "a leading out") intentionally engages the receptive capacities of children and others to imbue them with knowledge, skills of reasoning, values, socio-emotional awareness and control, and social interaction, so they can ...
In Denmark, no specific education group dominated the mortality decline. The highest contribution originated from the low education level (33.4% for the total population; 34.6% for men; 26.4% for women), but the contribution of the medium education level was not much smaller (27.1% for the total population; 28.3% for men; 23.1% for women).
The two articles in this issue of Science of Learning touch on aspects of this discussion rarely included in the educational research literature. ... the highest level of education of the student ...
Level of education is a characteristic of individuals and populations that plays a key role in determining a number of aspects of well-being, including health, income and empowerment 1,2,3 ...
Level of education can impact access to healthcare and health outcomes. Increasing rates of depression are a major public health concern, and vulnerability to depression is compounded for individuals with a lower level of education. ... Mini-Mental State exam scores vary with education in Blacks and Whites. Journal of the American Geriatrics ...
The first-stage coefficient indicates that one additional year of compulsory schooling increases the education level by 0.16 years, which is of a similar size as in previous studies with a comparable European multi-country setup (e.g ... Journal of Common Market Studies, 38 (3) (2000), pp. 427-448, 10.1111/1468-5965.00229. View in Scopus Google ...
About Journal of Economic Geography Editorial Board Advertising and Corporate Services ... Education level mother (categorical) 361,625: 9.49: 3.45: 2.00: 14.00 Education level father (categorical) 340,446: 9.10: 3.55: 2.00: 14.00: Secondary school enrollment and graduation variables End of primary school test score:
Introduction. Girls' education and gender inequalities associated with education were areas of major policy attention before the COVID-19 pandemic, and remain central to the agendas of governments, multilateral organisations and international NGOs in thinking about agendas to build back better, more equal or to build forward (Save the Children Citation 2020; UN Women Citation 2021; UNESCO ...
The effect of popularising higher education on economic growth. The population segment with higher education is regarded as an important contributor to economic growth, generating scientific ...
Research has demonstrated that nurses' professional values are cultivated during prelicensure academic education. Aim: The aim of this systematic review was to determine how level of education affects professional nursing values of clinical practising nurses. Methods: A systematic search of quantitative research published through December 2015 ...
The interplay among economic freedom, education level, and gender inequality was analyzed by Westerlund and Edgerton (2007) using an LM (Lagrange Multiplier) bootstrap cointegration test and Dumitrescu and Hurlin (2012) causality test because a significant heterogeneity and cross-sectional dependence among three variables are unveiled. The causality analysis is useful to see the mutual ...
To limit the scope of this article, we focus on linking classroom-level mechanisms to study achievement outcomes and do not consider more distal social-emotional outcomes. In this vein, we are interested in answering two fairly broad research questions: ... Journal of Research in Childhood Education, 27, 283-301. Crossref. Google Scholar.
The 21st-century skillset is generally understood to encompass a range of competencies, including critical thinking, problem solving, creativity, meta-cognition, communication, digital and technological literacy, civic responsibility, and global awareness (for a review of frameworks, see Dede, 2010).And nowhere is the development of such competencies more important than in developing country ...
4 issues/year. 0195-6744. 1549-6511. 2.5. Ranked #123 out of 269 "Education & Educational Research" journals. 3.3. Ranked #414 out of 1,469 "Education" journals. The American Journal of Education seeks to bridge and integrate the intellectual, methodological, and substantive diversity of educational scholarship and to encourage a ...
The analysis of the field and suggestions for future research in this positional article is primarily based on (a) a review of influential articles in inclusive education research (Nilholm and Göransson Citation 2017); (b) an overview (Nilholm Citation submitted) of influential reviews in five leading special needs journals (European Journal ...
Parental involvement in school has been demonstrated to play a crucial role in children's academic achievement. This article examines the relation of adolescent's academic achievement to parental involvement and parent's education level, separately, grounded on a quantitative approach. All participants were middle-class dual-earner parents from eight cities across the United States.
International Journal of Information Security - During the pandemic, the prevailing online learning has brought tremendous benefits to the education field. ... used students' year level as a proxy for the impact of education level, and used work exposure for the influence of social education level, to compare CSA among undergraduates ...
Journal of Education. The oldest educational publication in the country, the Journal of Education's mission is to disseminate knowledge that informs practice in PK-12, higher, and professional education. A refereed publication, the Journal offers … | View full journal description. This journal is a member of the Committee on Publication ...
Monica Adya, Ph.D., dean, Rutgers School of Business-Camden: The last three years have probably been some of the most transformative years for higher education. While we can consider multiple ...
Klug and colleagues in an article entitled "teaching and learning in education" raise some questions and want the faculty members to ask themselves these questions regularly. 1- How to increase the students' motivation. 2- How to help students feel confident in solving problems. 3- How to teach students to plan their learning activities.
Entry-level doesn't mean "no education or experience required" — In fact, many entry-level jobs require both. Here's what the misunderstood term actually means in the modern job market and how ...