Open Access is an initiative that aims to make scientific research freely available to all. To date our community has made over 100 million downloads. It’s based on principles of collaboration, unobstructed discovery, and, most importantly, scientific progression. As PhD students, we found it difficult to access the research we needed, so we decided to create a new Open Access publisher that levels the playing field for scientists across the world. How? By making research easy to access, and puts the academic needs of the researchers before the business interests of publishers.
We are a community of more than 103,000 authors and editors from 3,291 institutions spanning 160 countries, including Nobel Prize winners and some of the world’s most-cited researchers. Publishing on IntechOpen allows authors to earn citations and find new collaborators, meaning more people see your work not only from your own field of study, but from other related fields too.
Brief introduction to this section that descibes Open Access especially from an IntechOpen perspective
Want to get in touch? Contact our London head office or media team here
Our team is growing all the time, so we’re always on the lookout for smart people who want to help us reshape the world of scientific publishing.
Home > Books > Linked Open Data - Applications, Trends and Future Developments
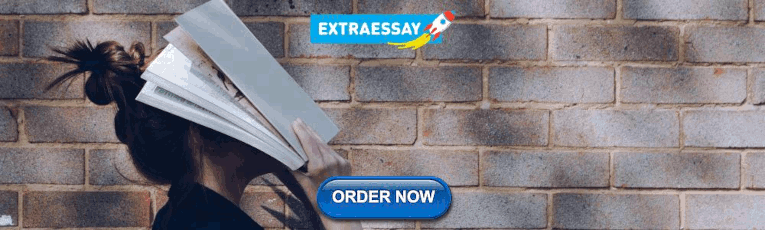
Analysis of Effective Load Balancing Techniques in Distributed Environment
Submitted: 06 December 2019 Reviewed: 01 February 2020 Published: 02 July 2020
DOI: 10.5772/intechopen.91460
Cite this chapter
There are two ways to cite this chapter:
From the Edited Volume
Linked Open Data - Applications, Trends and Future Developments
Edited by Kingsley Okoye
To purchase hard copies of this book, please contact the representative in India: CBS Publishers & Distributors Pvt. Ltd. www.cbspd.com | [email protected]
Chapter metrics overview
1,027 Chapter Downloads
Impact of this chapter
Total Chapter Downloads on intechopen.com

Total Chapter Views on intechopen.com
Computational approaches contribute a significance role in various fields such as medical applications, astronomy, and weather science, to perform complex calculations in speedy manner. Today, personal computers are very powerful but underutilized. Most of the computer resources are idle; 75% of the time and server are often unproductive. This brings the sense of distributed computing, in which the idea is to use the geographically distributed resources to meet the demand of high-performance computing. The Internet facilitates users to access heterogeneous services and run applications over a distributed environment. Due to openness and heterogeneous nature of distributed computing, the developer must deal with several issues like load balancing, interoperability, fault occurrence, resource selection, and task scheduling. Load balancing is the mechanism to distribute the load among resources optimally. The objective of this chapter is to discuss need and issues of load balancing that evolves the research scope. Various load balancing algorithms and scheduling methods are analyzed that are used for performance optimization of web resources. A systematic literature with their solutions and limitations has been presented. The chapter provides a concise narrative of the problems encountered and dimensions for future extension.
- load balancing
- resource management
- resource scheduling
- load measurement
- fault tolerance
Author Information
Anju shukla.
- Department of Computer Science and Engineering, Jaypee University of Engineering and Technology, Guna, MP, India
Shishir Kumar *
Harikesh singh.
*Address all correspondence to: [email protected]
1. Introduction
Distributes incoming traffic across multiple computational resources
Determines resource availability and reliability for task execution
Improves resource utilization
Increases client satisfaction
Provides fault tolerance and flexible framework by adding or subtracting resources as demand occurs
The load balancing algorithm (LBA) exists based on current state of the system as shown in Figure 1 . Static algorithms require prior information about the system characteristics such as processing capability, memory, number of active connections etc., while DLB algorithms use current status of the system to make scheduling decisions.

Classification based on System state.
Load balancing significantly improves global system performance in terms of throughput and resource utilization. The several reasons to use LBAs are as follows: cost optimization, fault tolerance ability, system adaptability and extensibility, decreased response time, idle time of resources, increased throughput, reliability, and prevents starvation [ 3 , 4 ].
To incorporate these benefits, it is important to select the suitable load balancing algorithm (LBA) for web resources in distributed environment [ 5 , 6 ]. Based on a process characteristic, LBAs can be categorized in three categories as shown in Figure 2 . Both sender-initiated and receiver-initiated algorithms use different transfer and location policies for implementing load balancing. Symmetric-initiated algorithms eliminate the preemption condition of receiver-initiated algorithm and offer two algorithms: above-average algorithm and adaptive algorithm. Above-average algorithm uses an acceptable range for deciding whether a node is sender-initiated or receiver-initiated.

Classification based on Process Origination.
1.1 Static load balancing
Stateless method
State-based method

Static load balancing.
In stateless method, selection of a processing element (PE) is done without having any awareness of the system environment, while in a state-based method, selecting a PE requires information of the system condition [ 7 ]. Stateless methods are simple to implement, but it provides one-to-one interaction between the client and server at a time as shown in Figure 4 .

Stateless static load balancing.
Figure 5 represents the stateful load balancing method; the load balancer keeps track for all the sessions, and decisions are taken based on server load. Various stateless techniques exist for selecting the processing element such as RR-LBA, weighted round robin (WRR)-LBA, and random allocation algorithm [ 7 ]. However, these algorithms have limited scope due to the dynamic nature of distributed environment.

Stateful static load balancing.
1.2 Dynamic load balancing
It varies from the SLB algorithms in which clients’ requests are distributed among available resources at run time. The LB assigns the request based on the dynamic information collected from all the resources as shown in Figure 6 .

Dynamic load balancing.
DLB algorithms can be classified in two categories—distributed and non-distributed. In distributed DLB, all computing resources are equally responsible for balancing the load. The responsibility of load balancing is shared among all the resources. But in non-distributed algorithms, each resource performs independently to accomplish the common goal. Generally, distributed DLB algorithms generated more message overhead than non-distributed DLB due to its interaction with all the resources.
Distributed algorithms perform better in fault conditions as it degrades only the sectional of the system instead of global system performance. Non-distributed algorithms are further classified into two categories—centralized and semi-centralized. In centralized algorithm, a central server is responsible for executing load balancing algorithm. In semi-centralized, servers are arranged in clusters, and load balancing within the cluster is managed centrally.
2. Challenges of load balancing
The load balancer implements several load balancing algorithms to determine the suitable resource. However, it faces several issues while distributing the load across available resources. Several major issues with their respective solutions are presented in the next section.
2.1 Increased web traffic
Over the last few years, the web traffic is increased very rapidly due to numerous registered websites and online transactions. As the numbers of requests are increased, the response of server becomes slow due to the limited number of open connections. The requests are added to the overall processing capability of resources. When incoming requests go beyond the capability of the resource, a resource crashes or fault occurs. Several authors analyzed and suggested the solution to resolve the issue. The first solution is the server upgradation in which requests are handled by a more powerful server for a while. But, scalability, interruption, and maintenance issues are associated with this solution. Another solution is the outsourcing in which requests are sent to another suitable server for speedy response. But this approach is costly and has limited control over the QoS issues. Chen et al. [ 8 ] suggested that the web page size and number of users both affect the system response time.
The most favorable solution is to use the multiple servers with an efficient load balancer which balances the load among servers. The performances of these servers are analyzed through queueing models or waiting line models. Broadly, two types of load balancing models are used to analyze the web server performance. Each approach has its benefits, applications, and limitations.
2.1.1 Centralized queueing model for load balancing
Random policy
Shortest queue policy

Centralized queueing model.
Zhang and Fan [ 9 ] compared these policies in terms of rejection rate and system response time. They analyzed that these algorithms perform well when traffic is light. But when web traffic becomes high, shortest queue policy performs better than random and RR policy. The number of rejections in RR and random policy is increased as the traffic increases. Singh and Kumar [ 10 ] presented a queueing algorithm for measuring the overloading and serving capacity of server in distributed load balancing environment. The algorithm performs better in both homogeneous and heterogeneous environment than the remaining capacity (RC) and server content based queue (QSC) algorithms.
2.1.2 Distributed queueing model for load balancing
These mechanisms address the network latency issue also, which avoids network congestion. The queueing models follow certain arrival and distribution rules to distribute the requests. Zhang and Fan [ 9 ] suggested that distributed queueing models perform well in heavy traffic conditions. Routing decisions are taken on the basis of queue length differences of web servers. The collected information is used in traffic distribution for improving the performance of web servers. Singh and Kumar [ 11 ] suggested that task completion time directly affects the queue length of the web server. They presented a model based on the ratio factor of the task’s average completion time. The model is compared with the model presented by Birdwell et al. [ 12 ], and it performs better for two performance metrics: average queue length and average waiting time of web servers.
Li et al. [ 13 ] analyzed network delay and presented a delay controlled load balancing approach for improving network performance. However, the approach has limited applicability and is suitable for stable path states.
Kamali et al. [ 14 ] used queueing theory to monitor the network traffic and its simulation is performed in a homogeneous as well as heterogeneous network environment. The traffic monitoring is required for the calculation of confidence and efficiency parameters from steady operations of the network. Based on queuing theory and little’s law network congestion rate is balanced.
2.2 Resource selection and task allocation
Many researchers have addressed the problem of resource selection and task allocation for the fair perspective of load balancing. It is the responsibility of load balancer to map resource and task before actual execution as shown in Figure 8 .

Task and resource allocation model.
The resource management consists of two major functions: resource provisioning and resource scheduling. In resource provisioning, the user submits task to the broker with various predefined QoS constraints. The broker is responsible to find the suitable resource for task execution. The resource scheduling is all about mapping and execution of task on the appropriate resource . It comprises of three major functions: resource mapping, resource execution and resource monitoring as shown in Figure 9 . Various types of resources that need to be managed are shown in Figure 10 .

Resource management classifications.

Types of resources for Management.
Hao et al. [ 15 ] categorized the resource in three categories—underloaded, normal loaded, and overloaded. The scheduler assigns the task to underloaded or normal-loaded resources only. Chang et al. [ 16 ] categorized the resources into L discrete levels for selecting the fittest resource for task execution. Arabnejad and Barbosa [ 17 ] presented a budget-based task scheduling and calculated the worthiness of all the resources for resource selection.
Naik et al. [ 18 ] presented a value function to select a resource for task execution. A value function is calculated using completion ratio and historic information of a resource. For minimizing the data transfer between the resources, Cheng et al. [ 19 ] used a hypergraph which identifies task and data dependency. Tasks that use similar data are assigned to the same resource to decrease the cost indirectly. AbdElrouf et al. [ 20 ] used a genetic algorithm for producing chromosomes. A fitness function is used for generating chromosomes. Individuals who have higher fitness value will only proceed for further chromosome reproduction.
Murugesan and Chellappan [ 21 ] suggested deadline and budget-based resource selection method for divisible workloads. The method assigns the appropriate resource in terms of cost from the list of available resources. Shah et al. [ 22 ] also claimed a linear programming-based resource allocation method for divisible workloads. The job is categorized in appropriate sizes to allocate on available resources. Singh and Kumar [ 23 ] improved the resource selection method presented by Singhal et al. [ 24 ] by determining the task workload and resource availability, respectively. Ang et al. [ 25 ] introduced a resource allocation mechanism by considering the requirement of user as well as service provider.
Various researchers suggested numerous techniques for heterogeneous task allocation. Raman et al. [ 26 ] provide improvements of traditional round robin (RR) task scheduling which performs well when all the resources have equal serving capacity. In heterogeneous environment, it does not provide prominent results. Therefore, a weight is assigned to each server which represents the priority of selecting a server. The algorithm performs well in distributing the load more efficiently then RR scheduling algorithm.
Pham and Huh [ 27 ] analyzed the cloud-fog environment and presented a task scheduling algorithm. The suggestion behind the presented algorithm is the association between fog nodes and cloud nodes to decrease the makespan and price of cloud resources. If the computation is not feasible on fog node, then tasks are executed on cloud node. Several constraints like deadline and budget can enhance the algorithm efficiency and applicability.
Wu [ 28 ] presented a task scheduling for embedded systems to enhance the performance of real applications in CloudIoT paradigm. These approaches are used in real-time networks where time constraints are strictly followed. The algorithm increases the scheduling success rate of real-time task on heterogeneous web servers. Moschakis and Karatza [ 29 ] analyzed the workload generated by IoT devices and scheduled them on multi cloud-based system. The least loaded server is selected by global dispatcher for scheduling IoT jobs.
Grandinetti et al. [ 30 ] presented an offline mathematical formula to improve task scheduling and average waiting time. Xu et al. [ 31 ] presented a task scheduling algorithm which determines crossover and mutation operations for mapping between tasks and resources. Kamalinia and Ghaffari [ 32 ] addressed the task scheduling as an NP complete problem. They also used a genetic algorithm to design task scheduling problem to improve makespan and resource efficiency. The presented scheduling algorithm reduces the communication cost among processors by using meta-heuristic methods.
2.3 Load measurement
The load measurement is very important and crucial activity in distributed environment. Various load balancing algorithms determine resource load condition before real implementation of task. Various performance metrics like fault tolerance, waiting time, response time, etc. can effectively be optimized by measuring the current load of a resource. Many authors addressed this issue and presented various resource provisioning techniques for effective distribution of incoming load.
Patel and Tripathy [ 33 ] categorized the resources in three categories: underloaded, normal-loaded, and overloaded to manage the load of available resources. Before assigning a task to a resource, the scheduler checks the current load of each resource and selects the underloaded or normal-loaded resource for task execution. Task length, processing element capacity, and deadline constraints are the factors that are considered to determine the current load of each resource. If a resource becomes overloaded, the unfinished tasks are shifted to another suitable resource for completing their execution. Checkpoint mechanism is used to save and resume the task state which greatly decreases the average response time and task resubmission time and improves the system throughput.
Liu et al. [ 34 ] advised that resource provisioning techniques may balance the resource load effectively. They presented peer load balance provision compares the demand and resource capacity by considering requirement of both customer and service provider. The presented mechanism reduced the cost and average response time than other existing methods.
Rathore and Chana [ 35 ] determined a dynamic threshold value based on standard deviation for load balancing and job migration. For job migration, the resources are categorized and the average load of each cluster is compared with processing element’s threshold value. For load balancing, tasks are selected randomly either from underloaded or overloaded resource collection.
Kaushik and Vidyarthi [ 36 ] consider various parameters for effective job scheduling and resource allocation. The presented model selects the best cluster in terms of increased system reliability and reduced energy consumption and balances the system load efficiently. The customer can prioritize their choices to select the suitable cluster for task execution. An effective approach for determining job migration overhead can increase the model adaptability in real scenarios.
2.4 Cost optimization
Load balancing algorithm maps task to various heterogeneous resources based on predefined objectives. The major objective of load balancer is to optimize task completion time, resource cost, and its utilization. Several authors addressed the cost issue and provide possible solutions for its optimization.
Garg and Singh [ 37 ] suggested an adaptive workflow scheduling (AWS) by considering resource cost and communication cost between task and resources. Due to heterogeneous nature of resources, final cost is calculated periodically. Arabnejad and Barbosa [ 17 ] presented a task scheduling algorithm which works in two phases - task selection phase and processor selection phase. For selecting the task, priority is assigned by computing the rank. For processor selection, worthiness of all processors is calculated and selects the processor with highest worthiness value.
Resource reservation
Resource expanding
Resource on demand
In the first stage, the cloud broker arranged the resources in advance without experiencing the customer requirement. In the second phase, the customer requirement and resource cost are comprehended, and the resource overutilization or underutilization is identified. If customer requirement is greater than reserved resources, the broker could request for additional resources on pay-per-use basis. Here, the on demand phase started. In on demand phase, the customer must know the appropriate future requirement which is difficult to estimate in cloud environment.
Singh and Kumar [ 39 ] presented a cost optimization method based on process activity. Processing cost and waiting time are determined by using activity time, resource utilization, and variability factor to check the method efficiency. Bittencourt and Madeira [ 40 ] presented a cost optimization method for hybrid cloud. The clouds can be categorized in three categories based on resource availability: public cloud, private cloud, and hybrid cloud. A user can use the services of public cloud by using pay-per-use method. Private clouds belong to individuals and offer free variety of services. In hybrid cloud, resources from public cloud are aggregated as per requirement. Bittencourt and Madeira [ 40 ] identified the method for appropriate resource.
Cao et al. [ 41 ] analyzed that each task is different from each other in cloud environment. They suggested an activity-based task scheduling approach for task reduction. The presented algorithm performs well than traditional task assignment approaches in terms of cost reduction.
Efficient resource provisioning plays a vital role in reducing the cost of task execution. Suresh and Varatharajan [ 42 ] presented a particle swarm optimization (PSO)-based resource provisioning algorithm. PSO is adopted to select the appropriate resource for cost optimization. Three performance metrics task execution time, memory usage, and cost are evaluated and compared with other existing methods. The simulation result shows that the presented PSO-based algorithm provides minimum execution time and memory usage with least cost than other state-of-the-art methods.
Salehan et al. [ 43 ] suggested auction-based resource allocation to meet the requirement of the customer and service provider. At the time of scheduling, resources are assigned to users that have highest bids. The algorithm provides highest profit and satisfies both the customers and service providers for multiple criteria than other existing methods. Nezarat and Dastghaibyfard [ 44 ] map the resource allocation mechanism to economic-based supply and demand problem which provides better functionality with 17% profit with other existing methods.
Netjinda et al. [ 45 ] suggested a task scheduling for workflow applications. These workflow applications consist of dependent task with deadline constraints. The aim is to select the least cost cloud resource through PSO for workflow-based task execution. The effective task scheduling decreases the execution time which directly affects the final cost. By considering communication overhead, the model effectiveness and applicability can be increased in real cloud environment.
Chunlin and Layuan [ 46 ] presented a resource provisioning method for mobile clients. The mobile devices greatly depend on cloud resources for accessing data and performing operations. The aim is to select the optimal resource at least cost. The service provider executes the tasks on appropriate resources to get the maximum profit.
2.5 Fault tolerance
Fault tolerance is a mechanism that provides the estimated quality results even in the presence of faults. A system with its components and services can consider reliable only if it has fault tolerance capability. Therefore, fault tolerance issue has got a noticeable attention by the research community over the last decades [ 47 ].
Fault tolerance techniques can be categorized into two: proactive and reactive. Proactive techniques are prevention techniques that determine the controlled state for fault tolerance before they occur. The systems are continuously monitored for fault estimation. Proactive fault tolerance can be implemented in three ways: self-healing, preemption migration, and system rejuvenation. In self-healing, fault recovery procedures are periodically applied for autonomous recovery. In preemptive migration, the tasks are shifted from fault probable resource to another resource. System rejuvenation is the mechanism in which periodic backups are taken for cleaning and removing errors from the system.
Another category is the reactive approaches that deal with faults after their occurrence. Reactive fault tolerance can also be implemented in three ways: job replication, job migration, and checkpoint. In job replication, several instances or copies of the same task make available on different resources. If one instance fails, task is executed on another instance. In job migration, tasks are migrated to another suitable resource for completing its execution. In checkpoint, task states are periodically saved and restarted from the last saved state instead of from the very beginning [ 47 ]. Several authors suggested fault tolerance mechanism and recovery solutions to resolve the issue.
Patel et al. [ 48 ] addressed resource failure issues and presented a checkpoint based recovery mechanism for task execution. If task does not complete its execution within deadline, then another suitable resource is selected for completing its execution. Before transferring it to another suitable resource, task state is saved and resumed for further execution through checkpoint. This results in reduced execution time, response time, and improved throughput than other existing methods.
Generally checkpoint increases the execution time that directly affects the execution cost. Egwutuoha et al. [ 49 ] use the process of redundant technique to reduce the task execution time. The presented technique is pretty good and reduces up to 40% checkpoint overhead. Choi et al. [ 50 ] identify the malicious users to provide fault tolerance scheduling in cloud environment. Any user which only use cloud services and reject other requests is treated as malicious user. The reputation is calculated to determine the malicious users. The work can be implemented to improve network reliability and task execution time in cloud paradigm.
Mei et al. [ 51 ] suggested that replication-based fault tolerance approaches waste lots of resources and also compromise with makespan. To resolve the issue, Mei et al. [ 51 ] presented fault tolerance scheduling mechanism that ensures successful completion of task execution. The limitation of replication is avoided by rescheduling the task for further execution. If scheduler identifies the failure, it reassigns task to another suitable resource and saves the wastage of resources. This mechanism reduces resource consumption and task execution time. However, costs are presumed for implementing scheduling, which limits the model applicability in real scenario.
Nazir et al. [ 52 ] use fault index for maintaining the history of resources. Fault index is determined based on successful and unsuccessful task completion on particular resource. Based on fault index value, grid broker replicates the task that can be used when fault occurs. Budget and time constraints are also considered at the time of task scheduling. The presented mechanism satisfies various QoS requirement, increases the reliability, and performs consistent in the existence of fault also.
Qureshi et al. [ 53 ] combined two fault tolerance techniques to inherit the favorable aspects. They perform hybridization of alternate task with retry and checkpoint mechanism and evaluate various performance metrics. The simulation result shows that alternate task with checkpoint mechanism performs better and improves system throughput than other existing methods.
Cloud facilitates the storage and access heterogeneous data in a distributed remote network. Due to dynamicity, network congestion and system faults are key factors for fault occurrence. Preventing the network from congestion and selection of suitable servers can avoid the fault conditions. Tamilvizhi and Parvathavarthini [ 54 ] suggested the concept of square matrix multiplication to manage the network traffic and avoid network congestion. The resource monitor predicts the fault conditions and uses migration policies to avoid system failure. The presented fault tolerance mechanism provides reduced cost with less energy consumption.
Garg and Singh [ 55 ] observed various fault conditions and suggested a fault tolerance-based task scheduling algorithm in grid environment. A genetic algorithm is used to determine the resource capacity for task scheduling. The presented approach increased system reliability and reduced task execution time in grid environment.
2.6 Interoperability issue
Distributed computing is a collection of various heterogeneous components that are located at remote locations, which coordinate with each other by message passing. Each component or processor has its own memory. It is a kind of parallel computing in which a task is split into subtasks to run on multiple components simultaneously.
Grid computing is a network of computer resources that are connected to solve a complex problem. Each resource is loosely linked and runs independent task to achieve a common goal. Grid computing may be classified on the basis of scale and functionality. On the basis of scale, grid computing may be classified into two categories ( Table 1 ), i.e., cluster grid and enterprise grid. Cluster means a group of similar kind of entities. So cluster grid provides services to the group or departmental level. Another type is enterprise grid that provides to share resources within the enterprise.
Cloud computing provides on demand computer resources such as storage or computational resources without direct involvement of users. It has effective data management and computing framework for executing task in parallel to improve various QoS metrics.
Fog computing is the extension of cloud computing which consists of multiple fog nodes that are directly connected to the physical devices. The difference between both technologies is that cloud is a centralized system while fog is distributed but decentralized system.
CloudIoT is an innovative trend which connects and manages millions of devices in very cost-effective manners that are dispersed globally. Cloud can profit by IoT to deal with real-world things by sharing the pool of highly computational resources rather than having local servers or personal devices to handle applications [ 56 , 57 ].
Classification of grid.
Various authors analyzed the interoperability issues that are briefly presented with their respective solutions. Aazam et al. [ 58 ] focused on analyzed two complementary technologies: cloud computing and IoT. Various challenges and integration issues of CloudIoT framework are discussed. Data analysis, service provisioning, and storage are the future dimensions to improve the performance of CloudIoT model.
Botta et al. [ 59 ] also analyzed the integration issues of cloud and IoT. Both the technologies are analyzed separately based on applications, technology, issues, and challenges. The details of existing platforms and projects are presented that are currently implementing CloudIoT. Standardization, address resolution, multi-networking, and developments of APIs are some future directions to provide full potential to CloudIoT framework. Khodkari et al. [ 60 ] present the significance and requirement of CloudIoT paradigm. They presented complementary aspects of cloud computing and IoT and assure the QoS by evaluating the integrity requirement of both the technologies.
Bonomi et al. [ 61 ] analyzed characteristics, services, and applications of fog computing. They determined the importance of collaboration of fog and cloud and address that some applications need both cloud globalization and fog localization like big data and analytics.
The linked open data (LOD) provides a new dimension for various heterogeneous interoperability issues based on Web server architectures. These issues require attention to support heterogeneous description principles that is necessary to deal with different data from web resources. The LOD interoperability follows bottom up approach to establish the strong relationships among datasets. Various researchers addressed LOD interoperability issues and presents respective solutions to meet with the users demand [ 1 , 2 ].
2.7 QoS issues
The user submits the tasks with various QoS constraints (cost execution time, energy consumption, delay, etc.) to improve the performance in distributed environment. Researchers addressed several QoS issues and provide the solutions for meeting the objective. Aron and Chana [ 62 ] observed various QoS issues and identified four issues, i.e., cost, reliability, security, and time, for resource provisioning in grid environment. Service-level agreement (SLA) reduced the complexity of resource provisioning by maintaining up-to-date information of all the resources. The presented approach performs better in terms of resource utilization, cost, and customer satisfaction.
Popularity of cloud computing increased burden on distributed data centers. These data centers consumes excessive amount of energy to provide services and fulfill consumer satisfaction. Horri et al. [ 63 ] identified overloaded and underloaded servers and shift load from overloaded to under loaded resources. This makes a trade-off between energy consumption and SLA. Hoseiny Farahabady et al. [ 64 ] suggested an objective function to reduce cost and performance improvement for resource allocation mechanism. Two test cases are considered: tasks with known running time and tasks with unknown running time. They listed Monte Carlo method to determine the task’s unknown values ( Table 2 ).
Existing load balancing techniques and future scope.
3. Conclusion
Development of a resource allocation model which considers resource as well as task characteristics to optimize various QoS metrics
Development of a fault tolerance load balancing model for partial executed tasks due to resource failure and construction of a resource selection policy for task execution
Analysis of contextual relationship among CloudIoT issues and optimization through effective scheduling
Development of an execution time prediction model for efficient resource provisioning, selection, and scheduling.
- 1. Bermes E. Convergence and interoperability: A linked data perspective. In: IFLA World Library and Information Congress. Vol. 77. 2011. pp. 1-12
- 2. Hidalgo-Delgado Y, Xu B, Marino-Molerio AJ, Febles-Rodriguez JP, Leiva-Mederos AA. A linked data-based semantic interoperability framework for digital libraries. RevistaCubana de CienciasInformáticas. 2019; 13 (1):14-30
- 3. Alakeel AM. A guide to dynamic load balancing in distributed computer systems. International Journal of Computer Science and Information Security. 2010; 10 (6):153-160
- 4. Khan RZ, Ali MF. An efficient diffusion load balancing algorithm in distributed system. International Journal of Information Technology and Computer Science. 2014; 6 (8):65-71
- 5. Khatchadourian S, Consens MP. ExpLOD: Summary-based exploration of interlinking and RDF usage in the linked open data cloud. In: Extended Semantic Web Conference; May 2010. pp. 272-287
- 6. Schwarte A, Haase P, Hose K, Schenkel R, Schmidt M. Fedx: Optimization techniques for federated query processing on linked data. In: International Semantic Web Conference. Berlin, Heidelberg: Springer; October 2011. pp. 601-616
- 7. Kumar B, Richhariya V. Load Balancing of Web Server System Using Service Queue Length. M.tech Scholar (CSE) Bhopal. Vol. 5(5). 2014. Available from: http://www.ijetae.Com/files/Volume4Issue5/IJETAE_0514_14.pdf
- 8. Chen C, Bai Y, Chung C, Peng H. Performance measurement and queueing analysis of web servers with a variation of webpage size. In: Proceedings of the International Conference on Computer Applications and Network Security. 2011. pp. 170-174
- 9. Zhang Z, Fan W. Web server load balancing: A queueing analysis. European Journal of Operational Research. 2008; 186 (2):681-693
- 10. Singh H, Kumar S. WSQ: Web server queueing algorithm for dynamic load balancing. Wireless Personal Communications. 2015a; 80 (1):229-245
- 11. Singh H, Kumar S. Analysis & minimization of the effect of delay on load balancing for efficient web server queueing model. International Journal of System Dynamics Applications. 2014; 3 (4):1-16
- 12. Birdwell JD, Chiasson J, Tang Z, Abdallah C, Hayat MM, Wang T. Dynamic time delay models for load balancing. Part I: Deterministic models. In: Advances in Time-Delay Systems. Berlin, Heidelberg: Springer; 2004. pp. 355-370
- 13. Li M, Nishiyama H, Kato N, Mizutani K, Akashi O, Takahara A. On the fast-convergence of delay-based load balancing over multipaths for dynamic traffic environments. In: International Conference on Wireless Communications and Signal Processing. October 2013. pp. 1-6
- 14. Kamali SH, Hedayati M, Izadi AS, Hoseiny HR. The monitoring of the network traffic based on queuing theory and simulation in heterogeneous network environment. In: International Conference on Computer Technology and Development; November 2009. pp. 322-326
- 15. Hao Y, Liu G, Wen N. An enhanced load balancing mechanism based on deadline control on GridSim. Future Generation Computer Systems. 2012; 28 (4):657-665
- 16. Chang RS, Lin CF, Chen JJ. Selecting the most fitting resource for task execution. Future Generation Computer Systems. 2011; 27 (2):227-231
- 17. Arabnejad H, Barbosa JG. A budget constrained scheduling algorithm for workflow applications. Journal of Grid Computing. 2014; 12 (4):665-679
- 18. Naik KJ, Jagan A, Narayana NS. A novel algorithm for fault tolerant job scheduling and load balancing in grid computing environment. In: International Conference on Green Computing and Internet of Things. 2015. pp. 1113-1118
- 19. Cheng B, Guan X, Wu H. A hypergraph based task scheduling strategy for massive parallel spatial data processing on master-slave platforms. In: 23rd International Conference on Geoinformatics. 2015. pp. 1-5
- 20. AbdElrouf W, Yousif A, Bashir MB. High exploitation genetic algorithm for job scheduling on grid computing. International Journal of Grid and Distributed Computing. 2016; 9 (3):221-228
- 21. Murugesan G, Chellappan C. An economic allocation of resources for divisible workloads in grid computing paradigm. European Journal of Scientific Research. 2011; 65 (3):434-443
- 22. Shah SNM, Mahmood AKB, Oxley A. Modified least cost method for grid resource allocation. In: International Conference on Cyber-Enabled Distributed Computing and Knowledge Discovery. 2010. pp. 218-225
- 23. Singh H, Kumar S. Optimized resource allocation mechanism for web server grid. In: IEEE UP Section Conference on Electrical Computer and Electronics. 2015b. pp. 1-6
- 24. Singhal S, Kumar M, Kant K. An economic allocation of resources in grid environment. In: International Conference on Information Systems and Computer Networks. March 2013. pp. 185-190
- 25. Ang TF, Por LY, Liew CS. Dynamic pricing scheme for resource allocation in multi-cloud environment. Malaysian Journal of Computer Science. 2017; 30 (1):1-17
- 26. Raman K, Subramanyam A, Rao AA. Comparative analysis of distributed web server system load balancing algorithms using qualitative parameters. VSRD International Journal of Computer Science and Information Technology. 2011; 8 :592-600
- 27. Pham XQ , Huh EN. Towards task scheduling in a cloud-fog computing system. In: 18th Asia-Pacific Network Operations and Management Symposium. October 2016. pp. 1-4
- 28. Wu DH. Task optimization scheduling algorithm in embedded system based on internet of things. Applied Mechanics and Materials. 2014; 513 :2398-2402
- 29. Moschakis IA, Karatza HD. Towards scheduling for Internet of Things applications on clouds: A simulated annealing approach. Concurrency and Computation: Practice and Experience. 2015; 27 (8):1886-1899
- 30. Grandinetti L, Pisacane O, Sheikhalishahi M. An approximate—Constraint method for a multi-objective job scheduling in the cloud. Future Generation Computer Systems. 2013; 29 (8):1901-1908
- 31. Xu Y, Li K, Hu J, Li K. A genetic algorithm for task scheduling on heterogeneous computing systems using multiple priority queues. Information Sciences. 2014; 270 :255-287
- 32. Kamalinia A, Ghaffari A. Hybrid task scheduling method for cloud computing by genetic and DE algorithms. Wireless Personal Communications. 2017; 97 (4):6301-6323
- 33. Patel DK, Tripathy C. An improved approach for load balancing among heterogeneous resources in computational grids. Engineering with Computers. 2015; 31 (4):825-839
- 34. Liu L, Mei H, Xie B. Towards a multi-QoS human-centric cloud computing load balance resource allocation method. The Journal of Supercomputing. 2016; 72 (7):2488-2501
- 35. Rathore N, Chana I. Variable threshold-based hierarchical load balancing technique in grid. Engineering with Computers. 2015; 31 (3):597-615
- 36. Kaushik A, Vidyarthi DP. An energy-efficient reliable grid scheduling model using NSGA-II. Engineering with Computers. 2016; 32 (3):355-376
- 37. Garg R, Singh AK. Adaptive workflow scheduling in grid computing based on dynamic resource availability. Engineering Science and Technology an International Journal. 2015; 18 (2):256-269
- 38. Chaisiri S, Lee BS, Niyato D. Optimization of resource provisioning cost in cloud computing. IEEE Transactions on Services Computing. 2012; 5 (2):164-177
- 39. Singh H, Kumar S. Resource cost optimization for dynamic load balancing on web server system. International Journal of Distributed and Cloud Computing. 2014; 2 (1):7-18
- 40. Bittencourt LF, Madeira ERM. HCOC: A cost optimization algorithm for workflow scheduling in hybrid clouds. Journal of Internet Services and Applications. 2011; 2 (3):207-227
- 41. Cao Q , Wei ZB, Gong WM. An optimized algorithm for task scheduling based on activity based costing in cloud computing. In: 3rd International Conference on Bioinformatics and Biomedical Engineering. June 2009. pp. 1-3
- 42. Suresh A, Varatharajan R. Competent resource provisioning and distribution techniques for cloud computing environment. Cluster Computing. 2017:1-8
- 43. Salehan A, Deldari H, Abrishami S. An online valuation-based sealed winner-bid auction game for resource allocation and pricing in clouds. The Journal of Supercomputing. 2017; 73 (11):4868-4905
- 44. Nezarat A, Dastghaibyfard G. A game theoretical model for profit maximization resource allocation in cloud environment with budget and deadline constraints. The Journal of Supercomputing. 2016; 72 (12):4737-4770
- 45. Netjinda N, Sirinaovakul B, Achalakul T. Cost optimal scheduling in IaaS for dependent workload with particle swarm optimization. The Journal of Supercomputing. 2014; 68 (3):1579-1603
- 46. Chunlin L, Layuan L. Cost and energy aware service provisioning for mobile client in cloud computing environment. The Journal of Supercomputing. 2015; 71 (4):1196-1223
- 47. Hasan M, Goraya MS. Fault tolerance in cloud computing environment: A systematic survey. Computers in Industry. 2018; 99 :156-172
- 48. Patel DK, Tripathy D, Tripathy C. An improved load-balancing mechanism based on deadline failure recovery on GridSim. Engineering with Computers. 2016; 32 (2):173-188
- 49. Egwutuoha IP, Chen S, Levy D, Selic B. A fault tolerance framework for high performance computing in cloud. In: 12th IEEE/ACM International Symposium on Cluster, Cloud and Grid Computing. May 2012. pp. 709-710
- 50. Choi S, Chung K, Yu H. Fault tolerance and QoS scheduling using CAN in mobile social cloud computing. Cluster Computing. 2014; 17 (3):911-926
- 51. Mei J, Li K, Zhou X, Li K. Fault-tolerant dynamic rescheduling for heterogeneous computing systems. Journal of Grid Computing. 2015; 13 (4):507-525
- 52. Nazir B, Qureshi K, Manuel P. Replication based fault tolerant job scheduling strategy for economy driven grid. The Journal of Supercomputing. 2015; 62 (2):855-873
- 53. Qureshi K, Khan FG, Manuel P, Nazir B. A hybrid fault tolerance technique in grid computing system. The Journal of Supercomputing. 2011; 56 (1):106-128
- 54. Tamilvizhi T, Parvathavarthini B. A novel method for adaptive fault tolerance during load balancing in cloud computing. Cluster Computing. 2017:1-14
- 55. Garg R, Singh AK. Fault tolerant task scheduling on computational grid using checkpointing under transient faults. Arabian Journal for Science and Engineering. 2014; 39 (12):8775-8791
- 56. Singh S, Chana I. A survey on resource scheduling in cloud computing: Issues and challenges. Journal of Grid Computing. 2016; 14 (2):217-264
- 57. Yassa S, Chelouah R, Kadima H, Granado B. Multi-objective approach for energy-aware workflow scheduling in cloud computing environments. The Scientific World Journal. 2013:1-13
- 58. Aazam M, Khan I, Alsaffar AA, Huh EN. Cloud of things: Integrating Internet of Things and cloud computing and the issues involved. In: Proceedings of 2014 11th International Bhurban Conference on Applied Sciences & Technology (IBCAST) Islamabad, Pakistan. 2014. pp. 414-419
- 59. Botta A, De Donato W, Persico V, Pescapé A. Integration of cloud computing and internet of things: A survey. Future Generation Computer Systems. 2016; 56 :684-700
- 60. Khodkari H, Maghrebi SG, Branch R. Necessity of the integration Internet of Things and cloud services with quality of service assurance approach. Bulletin de la Société Royale des Sciences de Liège. 2016; 85 (1):434-445
- 61. Bonomi F, Milito R, Zhu J, Addepalli S. Fog computing and its role in the internet of things. In: Proceedings of the First Edition of the MCC Workshop on Mobile Cloud Computing. August 2012. pp. 13-16
- 62. Aron R, Chana I. Formal QoS policy based grid resource provisioning framework. Journal of Grid Computing. 2012; 10 (2):249-264
- 63. Horri A, Mozafari MS, Dastghaibyfard G. Novel resource allocation algorithms to performance and energy efficiency in cloud computing. The Journal of Supercomputing. 2014; 69 (3):1445-1461
- 64. HoseinyFarahabady M, Lee YC, Zomaya AY. Randomized approximation scheme for resource allocation in hybrid-cloud environment. The Journal of Supercomputing. 2014; 69 (2):576-592
© 2020 The Author(s). Licensee IntechOpen. This chapter is distributed under the terms of the Creative Commons Attribution 3.0 License , which permits unrestricted use, distribution, and reproduction in any medium, provided the original work is properly cited.
Continue reading from the same book
Linked open data.
Published: 19 November 2020
By Kuo-Chi Chang, Kai-Chun Chu, Hsiao-Chuan Wang, Yuh...
581 downloads
By Monday Osagie Adenomon
809 downloads
By Jung-Ran Park, Andrew Brenza and Lori Richards
1051 downloads
Applications of Parallel Computers
Load balancing.
Prof David Bindel
Please click the play button below.
Inefficiencies in parallel code
Poor single processor performance
- Typically in the memory system
- Saw this in matrix multiply assignment
Overhead for parallelism
- Thread creation, synchronization, communication
- Saw this in shallow water assignment
Load imbalance
- Different amounts of work across processors
- Different speeds / available resources
- Insufficient parallel work
- All this can change over phases
Where does the time go?
- Load balance looks like large sync cost
- ... maybe so does ordinary sync overhead!
- And spin-locks may make sync look like useful work
- And ordinary time sharing can confuse things more
- Can get some help from profiling tools
Many independent tasks
- What if task costs are inhomogeneous?
- Worse: all expensive tasks on one thread?
- Many small tasks, randomly assigned
- Dynamic task assignment
- Issue: what about scheduling overhead?
Variations on a theme
How to avoid overhead? Chunks! (Think OpenMP loops)
- Small chunks: good balance, large overhead
- Large chunks: poor balance, low overhead
- Fixed chunk size (requires good cost estimates)
- Guided self-scheduling (take \(\lceil (\mbox{tasks left})/p \rceil\) work)
- Tapering (size chunks based on variance)
- Weighted factoring (GSS with heterogeneity)
Static dependency
- Graph \(G = (V,E)\) with vertex and edge weights
- Goal: even partition, small cut (comm volume)
- Optimal partitioning is NP complete – use heuristics
- Tradeoff quality vs speed
- Good software exists (e.g. METIS)
The limits of graph partitioning
- We don’t know task costs?
- We don’t know the comm/dependency pattern?
- These things change over time?
May want dynamic load balancing?
Even in regular case: not every problem looks like an undirected graph!
Dependency graphs
So far: Graphs for dependencies between unknowns .
For dependency between tasks or computations:
- Arrow from \(A\) to \(B\) means that \(B\) depends on \(A\)
- Result is a directed acyclic graph (DAG)
Longest Common Substring
Goal: Longest sequence of (not necessarily contiguous) characters common to strings \(S\) and \(T\) .
Recursive formulation: \[\begin{aligned} & \mathrm{LCS}[i,j] = \\ & \begin{cases} \max(\mathrm{LCS}[i-1,j], \mathrm{LCS}[j,i-1]), & S[i] \neq T[j] \\ 1 + \mathrm{LCS}[i-1,j-1], & S[i] = T[j] \end{cases} \end{aligned}\] Dynamic programming: Form a table of \(\mathrm{LCS}[i,j]\)
Process in any order consistent with dependencies. Limits to available parallel work early on or late!
Partition into coarser-grain tasks for locality?
Dependence between coarse tasks limits parallelism.
Alternate perspective
Two approaches to LCS:
- Solve subproblems from bottom up
- Solve top down, memoize common subproblems
Parallel question: shared memoization (and synchronize) or independent memoization (and redundant computation)?
Load balancing and task-based parallelism
- Task DAG captures data dependencies
- May be known at outset or dynamically generated
- Topological sort reveals parallelism opportunities
Basic parameters
- Do all tasks have equal costs?
- Known statically, at creation, at completion?
- Can tasks be run in any order?
- If not, when are dependencies known?
- Tasks co-located to reduce communication?
- When is this information known?
Dependencies
Locality/communication.
When do you communicate?
- Easy: Only at start/end (embarrassingly parallel)
- Harder: In a predictable pattern (PDE solver)
- Hardest: Unpredictable (discrete event simulation)
A spectrum of solutions
Depending on cost, dependency, locality:
Static scheduling
Semi-static scheduling, dynamic scheduling.
- Everything known in advance
- Can schedule offline (e.g. graph partitioning)
- Example: Shallow water solver
- Everything known at start of step (for example)
- Use offline ideas (e.g. Kernighan-Lin refinement)
- Example: Particle-based methods
- Don’t know what we’re doing until we’ve started
- Have to use online algorithms
- Example: most search problems
Search problems
- Different set of strategies from physics sims!
- Usually require dynamic load balance
- Optimal VLSI layout
- Robot motion planning
- Game playing
- Speech processing
- Reconstructing phylogeny
Example: Tree search
- Tree unfolds dynamically during search
- Common problems on different paths (graph)?
- Graph may or may not be explicit in advance
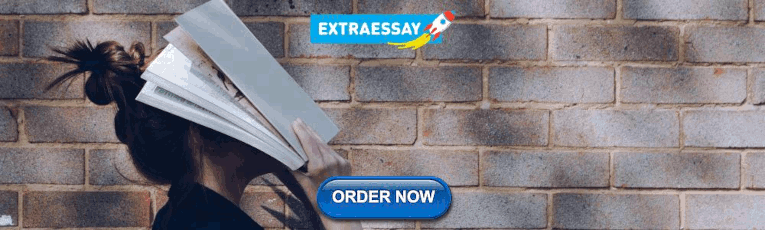
Search algorithms
Generic search:
- Put root in stack/queue
- remove node \(n\) from queue
- if \(n\) satisfies goal, return
- mark \(n\) as searched
- queue viable unsearched children (Can branch-and-bound)
DFS (stack), BFS (queue), A \(^*\) (priority queue), ...
Simple parallel search
Static load balancing:
- Each new task on a proc until all have a subtree
- Ineffective without work estimates for subtrees!
- How can we do better?
Centralized scheduling
Idea: obvious parallelization of standard search
- Locks on shared data structure (stack, queue, etc)
- Or might be a manager task
Teaser: What could go wrong with this parallel BFS?
- obtain queue lock
- release queue lock
- process \(n\) , mark as searched
- enqueue unsearched children
- remove node \(n\) from queue; workers active ++
- enqueue unsearched children; workers active –
Centralized task queue
- Tasks might be range of loop indices
- Assume independent iterations
- Loop body has unpredictable time (or do it statically)
- Pro: dynamic, online scheduling
- Con: centralized, so doesn’t scale
- Con: high overhead if tasks are small
Beyond centralized task queue
Basic distributed task queue idea:
- Each processor works on part of a tree
- When done, get work from a peer
- Or if busy, push work to a peer
- Asynch communication useful
Also goes by work stealing, work crews...
Picking a donor
- Asynchronous round-robin
- Global round-robin (current donor ptr at P0)
- Randomized – optimal with high probability!
Diffusion-based balancing
- But not all connections are equal
- Idea: prefer to poll more local neighbors
- Average out load with neighbors \(\implies\) diffusion!
Mixed parallelism
- Today: mostly coarse-grain task parallelism
- Other times: fine-grain data parallelism
- Why not do both? Switched parallelism.
- Lots of ideas, not one size fits all!
- Axes: task size, task dependence, communication
- Dynamic tree search is a particularly hard case!
- Overdecompose (load balance) vs keep tasks big (overhead, locality)
- Steal work globally (balance) vs steal from neighbors (comm. overhead)
- Sometimes hard to know when code should stop!
- Trending Now
- Foundational Courses
- Data Science
- Practice Problem
- Machine Learning
- System Design
- DevOps Tutorial
- Distributed Systems Tutorial
Introduction to Distributed System
- What is a Distributed System?
- Features of Distributed Operating System
- Evolution of Distributed Computing Systems
- Types of Transparency in Distributed System
- What is Scalable System in Distributed System?
- Role of Middleware in Distributed System
- Difference between Hardware and Middleware
- What is Groupware in Distributed System?
- Difference between Parallel Computing and Distributed Computing
- Difference between Loosely Coupled and Tightly Coupled Multiprocessor System
- Design Issues of Distributed System
- Introduction to Distributed Computing Environment (DCE)
- Limitation of Distributed System
- Various Failures in Distributed System
- Types of Operating Systems
- Types of Distributed System
- Comparison - Centralized, Decentralized and Distributed Systems
- Three-Tier Client Server Architecture in Distributed System
Communication in Distributed Systems
- Features of Good Message Passing in Distributed System
- Issues in IPC By Message Passing in Distributed System
- What is Message Buffering?
- Multidatagram Messages in Distributed System
- Group Communication in distributed Systems
Remote Procedure Calls in Distributed System
- What is RPC Mechanism in Distributed System?
- Distributed System - Transparency of RPC
- Stub Generation in Distributed System
- Marshalling in Distributed System
- Server Management in Distributed System
- Distributed System - Parameter Passing Semantics in RPC
- Distributed System - Call Semantics in RPC
- Communication Protocols For RPCs
- Client-Server Model
- Lightweight Remote Procedure Call in Distributed System
- Difference Between RMI and DCOM
- Difference between RPC and RMI
Synchronization in Distributed System
- Synchronization in Distributed Systems
- Logical Clock in Distributed System
- Lamport's Algorithm for Mutual Exclusion in Distributed System
- Vector Clocks in Distributed Systems
- Event Ordering in Distributed System
- Mutual exclusion in distributed system
- Performance Metrics For Mutual Exclusion Algorithm
- Cristian's Algorithm
- Berkeley's Algorithm
- Difference between Token based and Non-Token based Algorithms in Distributed System
- Ricart–Agrawala Algorithm in Mutual Exclusion in Distributed System
- Suzuki–Kasami Algorithm for Mutual Exclusion in Distributed System
Source Management and Process Management
- Features of Global Scheduling Algorithm in Distributed System
- What is Task Assignment Approach in Distributed System?
- Load Balancing Approach in Distributed System
Load-Sharing Approach in Distributed System
- Difference Between Load Balancing and Load Sharing in Distributed System
- Process Migration in Distributed System
Distributed File System and Distributed shared memory
- What is DFS (Distributed File System)?
- Andrew File System
- File Service Architecture in Distributed System
- File Models in Distributed System
- File Accessing Models in Distributed System
- File Caching in Distributed File Systems
- What is Replication in Distributed System?
- Atomic Commit Protocol in Distributed System
- Design Principles of Distributed File System
- What is Distributed shared memory and its advantages
- Architecture of Distributed Shared Memory(DSM)
- Difference between Uniform Memory Access (UMA) and Non-uniform Memory Access (NUMA)
- Algorithm for implementing Distributed Shared Memory
- Consistency Model in Distributed System
- Distributed System - Thrashing in Distributed Shared Memory
Distributed Scheduling and Deadlock
- Scheduling and Load Balancing in Distributed System
- Issues Related to Load Balancing in Distributed System
- Components of Load Distributing Algorithm | Distributed Systems
- Distributed System - Types of Distributed Deadlock
- Deadlock Detection in Distributed Systems
- Conditions for Deadlock in Distributed System
- Deadlock Handling Strategies in Distributed System
- Deadlock Prevention Policies in Distributed System
- Chandy-Misra-Haas's Distributed Deadlock Detection Algorithm
- Security in Distributed System
- Types of Cyber Attacks
- Cryptography and its Types
- Implementation of Access Matrix in Distributed OS
- Digital Signatures and Certificates
- Design Principles of Security in Distributed System
Distributed Multimedia and Database System
- Distributed Database System
- Functions of Distributed Database System
- Multimedia Database
Distributed Algorithm
- Deadlock-Free Packet Switching
- Wave and Traversal Algorithm in Distributed System
- Election algorithm and distributed processing
- Introduction to Common Object Request Broker Architecture (CORBA) - Client-Server Software Development
- Difference between CORBA and DCOM
- Difference between COM and DCOM
- Life cycle of Component Object Model (COM) Object
- Distributed Component Object Model (DCOM)
Distributed Transactions
- Flat & Nested Distributed Transactions
- Transaction Recovery in Distributed System
- Mechanism for building Distributed file system
- Two Phase Commit Protocol (Distributed Transaction Management)
Load sharing basically denotes the process of forwarding a router to share the forwarding of traffic, in case of multiple paths if available in the routing table. In case there are equal paths then the forwarding process will follow the load-sharing algorithm. In load sharing systems, all nodes share the overall workload, and the failure of some nodes increases the pressure of the rest of the nodes. The load sharing approach ensures that no node is kept idle so that each node can share the load.
For example, suppose there are two connections of servers of different bandwidths of 500Mbps and another 250Mbps. Let, there are 2 packets. Instead of sending the 2 packets to the same connection i.e. 500Mbps, 1 packet will be forwarded to the 500Mbps and another to the 250Mbps connection. Here the goal is not to use the same amount of bandwidth in two connections but to share the load so that each connection can sensibly deal with it without any traffic.
Why use Load Sharing?
There are several issues in designing Load Balancing Algorithms. To overcome these issues we use the load-sharing algorithm. The issues are:
- Load assessment: It decides how to evaluate the workload of a node in a distributed framework.
- Process transfer: It concludes whether the process can be executed locally or from a distance.
- Static information exchange: It decides how the framework loads information that can be exchanged among the nodes.
- Location policy: It decides the determination of an objective hub during process migration.
- Priority assignment: It decides the priority of execution of a bunch of nearby and remote processes on a specific node.
- Migration restricting policy: It decides the absolute number of times a process can move starting with one hub then onto the next.
Load Sharing algorithm includes policies like location policy, process transfer policy, state information exchange policy, load estimation policy, priority assignment policy, and migration limiting policy.
1. Location Policies: The location policy concludes the sender node or the receiver node of a process that will be moved inside the framework for load sharing. Depending upon the sort of node that steps up and searches globally for a reasonable node for the process, the location strategies are of the accompanying kinds:
- Sender-inaugurated policy: Here the sender node of the process has the priority to choose where the process has to be sent. The actively loaded nodes search for lightly loaded nodes where the workload has to be transferred to balance the pressure of traffic. Whenever a node’s load turns out to be more than the threshold esteem, it either communicates a message or arbitrarily tests different nodes individually to observe a lightly loaded node that can acknowledge at least one of its processes. In the event that a reasonable receiver node isn’t found, the node on which the process began should execute that process.
- Receiver-inaugurated policy: Here the receiver node of the process has the priority to choose where to receive the process. In this policy, lightly loaded nodes search for actively loaded nodes from which the execution of the process can be accepted. Whenever the load on a node falls under threshold esteem, it communicates a text message to all nodes or tests nodes individually to search for the actively loaded nodes. Some vigorously loaded node might move one of its processes if such a transfer does not reduce its load underneath the normal threshold.
2. Process transfer Policy: All or nothing approach is used in this policy. The threshold value of all the nodes is allotted as 1. A node turns into a receiver node if there is no process and on the other side a node becomes a sender node if it has more than 1 process. If the nodes turn idle then they can’t accept a new process immediately and thus it misuses the processing power To overcome this problem, transfer the process in such a node that is expected to be idle in the future. Sometimes to ignore the processing power on the nodes, the load-sharing algorithm turns the threshold value from 1 to 2.
3. State Information exchange Policy: In load-sharing calculation, it is not required for the nodes to regularly exchange information, however, have to know the condition of different nodes when it is either underloaded or overloaded. Thus two sub-policies are used here:
- Broadcast when the state changes: The nodes will broadcast the state information request only when there is a change in state. In the sender-inaugurated location policy, the state information request is only broadcasted by the node when a node is overloaded. In the receiver-inaugurated location policy, the state information request is only broadcasted by the node when a node is underloaded.
- Poll when the state changes: In a large network the polling operation is performed. It arbitrarily asks different nodes for state information till it gets an appropriate one or it reaches the test limit.
4. Load Estimation Policy: Load-sharing algorithms aim to keep away from nodes from being idle yet it is adequate to know whether a node is occupied or idle. Consequently, these algorithms typically utilize the least complex load estimation policy of counting the absolute number of processes on a node.
5. Priority Assignment Policy: It uses some rules to determine the priority of a particular node. The rules are:
- Selfish: Higher priority is provided to the local process than the remote process. Thus, it has the worst response time performance for the remote process and the best response time performance for the local process.
- Altruistic: Higher priority is provided to the remote process than the local process. It has the best response time performance.
- Intermediate: The number of local and remote processes on a node decides the priority. At the point when the quantity of local processes is more or equivalent to the number of remote processes then local processes are given higher priority otherwise remote processes are given higher priority than local processes.
6. Migration limiting policy: This policy decides the absolute number of times a process can move. One of the accompanying two strategies might be utilized.
- Uncontrolled: On arrival of a remote process at a node is handled similarly as a process emerging at a node because of which any number of times a process can migrate.
- Controlled: A migration count parameter is used to fix the limit of the migration of a process. Thus, a process can migrate a fixed number of times here. This removes the instability of uncontrolled strategy.
Please Login to comment...
Similar reads.
- Geeks-Premier-League-2022
- Distributed System
- Geeks Premier League
Improve your Coding Skills with Practice
What kind of Experience do you want to share?
Deterministic and Randomized Algorithms for Distributed On-Line Task Assignment and Load Balancing without Load Status Information
- Published: 05 September 2007
- Volume 34 , pages 453–469, ( 2001 )
Cite this article
71 Accesses
Explore all metrics
We find two interesting thresholds for the number of tasks generated under a new model of distributed on-line task assignment and load balancing environment, in which tasks are assigned without any information of current load status and load balancing is achieved together with task assignment. It is shown that balanced load distribution can be obtained deterministically when the number of tasks exceeds the first threshold. Otherwise, a simple randomized strategy can achieve balanced load distribution with high probability if the number of tasks exceeds the second threshold.
This is a preview of subscription content, log in via an institution to check access.
Access this article
Price includes VAT (Russian Federation)
Instant access to the full article PDF.
Rent this article via DeepDyve
Institutional subscriptions
Similar content being viewed by others
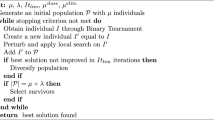
Population-based iterated local search for batch scheduling on parallel machines with incompatible job families, release dates, and tardiness penalties
Simulated Annealing: From Basics to Applications
Genetic Algorithms and Their Applications
Author information, authors and affiliations.
Department of Computer Science, State University of New York, [email protected], New Paltz, NY 12561, USA, US
You can also search for this author in PubMed Google Scholar
Additional information
Received October 30, 2000, and in revised form May 10, 2001. Online publication October 30, 2001.
Rights and permissions
Reprints and permissions
About this article
Li, K. Deterministic and Randomized Algorithms for Distributed On-Line Task Assignment and Load Balancing without Load Status Information . Theory Comput. Systems 34 , 453–469 (2001). https://doi.org/10.1007/s00224-001-1022-6
Download citation
Published : 05 September 2007
Issue Date : October 2001
DOI : https://doi.org/10.1007/s00224-001-1022-6
Share this article
Anyone you share the following link with will be able to read this content:
Sorry, a shareable link is not currently available for this article.
Provided by the Springer Nature SharedIt content-sharing initiative
- Load Balance
- Task Assignment
- Competitive Ratio
- Load Generation
- Load Balance Algorithm
- Find a journal
- Publish with us
- Track your research
Latency aware mobile task assignment and load balancing for edge cloudlets
Ieee account.
- Change Username/Password
- Update Address
Purchase Details
- Payment Options
- Order History
- View Purchased Documents
Profile Information
- Communications Preferences
- Profession and Education
- Technical Interests
- US & Canada: +1 800 678 4333
- Worldwide: +1 732 981 0060
- Contact & Support
- About IEEE Xplore
- Accessibility
- Terms of Use
- Nondiscrimination Policy
- Privacy & Opting Out of Cookies
A not-for-profit organization, IEEE is the world's largest technical professional organization dedicated to advancing technology for the benefit of humanity. © Copyright 2024 IEEE - All rights reserved. Use of this web site signifies your agreement to the terms and conditions.
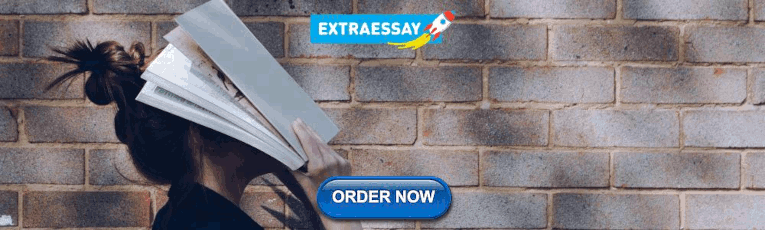
IMAGES
VIDEO
COMMENTS
Task Assignment Approach: In the Task Assignment Approach, the user-submitted process is composed of multiple related tasks which are scheduled to appropriate nodes in a system to improve the performance of a system as a whole. ... Distributed: In Distributed Load Balancing Algorithms, the decision task of assigning processes is distributed ...
Load balancing plays a vital role in the operation of distributed and parallel computing. It partitioned the incoming workload into smaller tasks that are assigned to computational resources for concurrent execution. The load may be CPU capacity, memory size, network load, delay, etc.
A practical task assignment is one of the core issues of a multi-robot system. In this paper, a multi-robot task assignment strategy based on load balancing is proposed to effectively balance and plan out the execution cost of each robot when it has a large number of working task points. Considering the variability of the execution task cost in practical situations with different task point ...
Abstract: A graph-theoretic approach for determining an optimal task (or routing) assignment of p autonomous vehicles (AV's) among m workstations in a flexible manufacturing system which both minimizes the assignment completion time and balances the load among the AV's is presented. This task assignment problem is equivalent to an optimal routing assignmenl of destinating the m workstations to ...
Load balancing is the process of distributing customer tasks among multiple computing resources, such as virtual machines (VMs), servers and networks. It is a major concern in cloud computing as the number of customers demanding the service is growing exponentially. An efficient load balancing approach can monitor the load of the VMs proactively and assigns the customer tasks to the VMs ...
Abstract: This paper presents a graph-theoretic approach to determining an optimal task (or routing) assignment of p autonomous vehicles (AVs) among m workstations in a flexible manufacturing system (FMS) to minimize the assignment completion time, as well as to achieve load balancing among the AVs. The task assignment problem is equivalent to an optimal routing assignment of destinating the m ...
1997. Abstract We consider the problem of task assignment in a distributed system (such as a distributed Web server) in which task sizes are drawn from a heavy-tailed distribution. Many task assignment algorithms are based on the heuristic that balancing the load at the server hosts will result in optimal performance.
To perform a set of tasks in a robotic network cloud system as fast as possible, it is recommended to use a scheduling approach that minimizes the makespan. The makespan is defined as the time between the start of the first scheduled task and the completion of all scheduled tasks. Load balancing is a technique to distribute incoming tasks across processing units in a way that the resource ...
This technique also considers task load when mapping tasks. They argue that their solution influences communication performance and load balancing in parallel architectures because EagerMap helps to evenly distribute load among clusters and grids. ... Adewojo A, Bass J. A novel weight-assignment load balancing algorithm for cloud applications ...
effectively solve the uneven execution cost of each robot in the task assignment process and improve the efficiency of the system in completing tasks. Keywords: multi-robot; task assignment; k-means; load balancing; MTSP problem 1. Introduction The multi-robot system (MRS) is one of the most extensive research areas in robotics,
Deterministic and randomized algorithms for distributed on-line task assignment and load balancing without load status information. Pages 613-622. Previous Chapter Next Chapter. References 1. M. Adler, S. Chakrabarti, M. Mitzenmacher, and L. Rasmussen, "Parallel randomized load balancing,'' Proc. of A CM Symposium on Theory of Computing, pp ...
Latency aware mobile task assignment and load balancing for edge cloudlets. This paper addresses the issue of task assignment in a scenario (i.e. which cloudlet serves which mobile device) aimed towards improving the quality of service experienced by the mobile devices in terms of minimizing the latency and demonstrates the performance gains of ...
This paper has proposed a hybrid technique for solving task assignment problem in cloud platform by using variants of Particle Swarm Optimization which gives better result than PSO and other heuristics for load balancing in cloud computing environment. Load balancing is one of the major issue in cloud computing. Load balancing helps in achieving maximum resource utilization and user satisfaction.
Task Assignment Approach. Load-Balancing Approach. Load-Sharing Approach. Summary. This chapter contains sections titled: Exercises. Bibliography. Pointers to Bibliographies on the Internet. Article #: ISBN Information: Print ISBN: 9780780311190 Online ISBN: 9780470544419
The simplest approach is to statically partition the work into fixed size chunks, but this requires good cost estimates a priori. The other extreme, sometimes called self-scheduling, involves querying a work queue for every new task; this is good for load balance under uncertain costs, but the overhead is pretty high.
Abstract. Load Balancing is fundamental for effective activities in conveyed conditions. As Cloud Computing is developing quickly with customers be requesting extra services and superior outcomes, load balancing in favor of the Cloud have turned into an extremely intriguing and imperative research region.
The load balancing is dynamic load balancing, where the load distribution evolves over time. One example is scientific simulations, where weather simulations change over time. The change is dependending on each use case though. - The third step is to decide what information is needed for load balancing. - The fourth step is to design a load ...
A graph-theoretic approach for determining an optimal task (or routing) assignment of p autonomous vehicles (AV's) among m workstations in a flexible manufacturing system which both minimizes the assignment completion time and balances the load among the AV's is presented. This task assignment problem is equivalent to an optimal routing assignmenl of destinating the m workstations to the p ...
Why use Load Sharing? There are several issues in designing Load Balancing Algorithms. To overcome these issues we use the load-sharing algorithm. The issues are: Load assessment: It decides how to evaluate the workload of a node in a distributed framework. Process transfer: It concludes whether the process can be executed locally or from a ...
Load balancing algorithms: A typical load balancing algorithm has input of amount of work assigned to each process Output is new assignment to different processes to balance them Main goals are to bring the metric closer to 1 by bringing maximum load closer to average load and minimize amount of data migration
We find two interesting thresholds for the number of tasks generated under a new model of distributed on-line task assignment and load balancing environment, in which tasks are assigned without any information of current load status and load balancing is achieved together with task assignment. It is shown that balanced load distribution can be obtained deterministically when the number of ...
Latency aware mobile task assignment and load balancing for edge cloudlets Abstract: With the various technological advances, mobile devices are not just being used as a means to make voice calls; but are being used to accomplish a variety of tasks. Mobile devices are being envisioned to practically accomplish any task which could be done on a ...
A total of 18 task assignment state features for the load balancing-oriented TALBP environment have been considered, which ensure that the agent can obtain more useful information from the environment and perform optimal selection as completely as possible, and different reward functions according to objectives and their implicit information ...