- Browse All Articles
- Newsletter Sign-Up
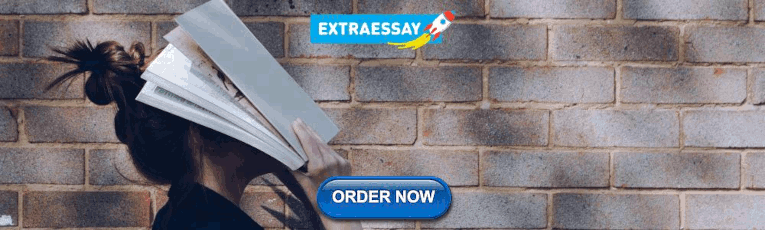
No results found in Working Knowledge
- Were any results found in one of the other content buckets on the left?
- Try removing some search filters.
- Use different search filters.
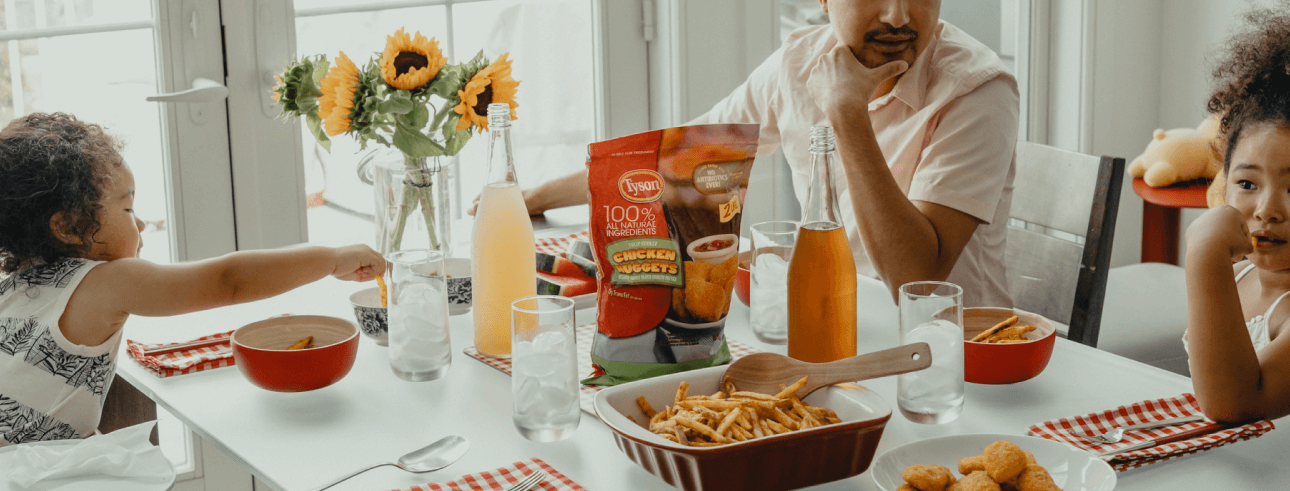
Industry Expertise
Market research for the food and beverage industry, understand the changing landscape.
The food and beverage industry moves at a break-neck pace. For consumers, every weekly trip to the grocery store is an opportunity to try something new, develop new brand or product loyalties, and even change their identity.
No brand can rest on its laurels in a landscape where unique shopping challenges, health recommendations and flavor trends are constantly changing.
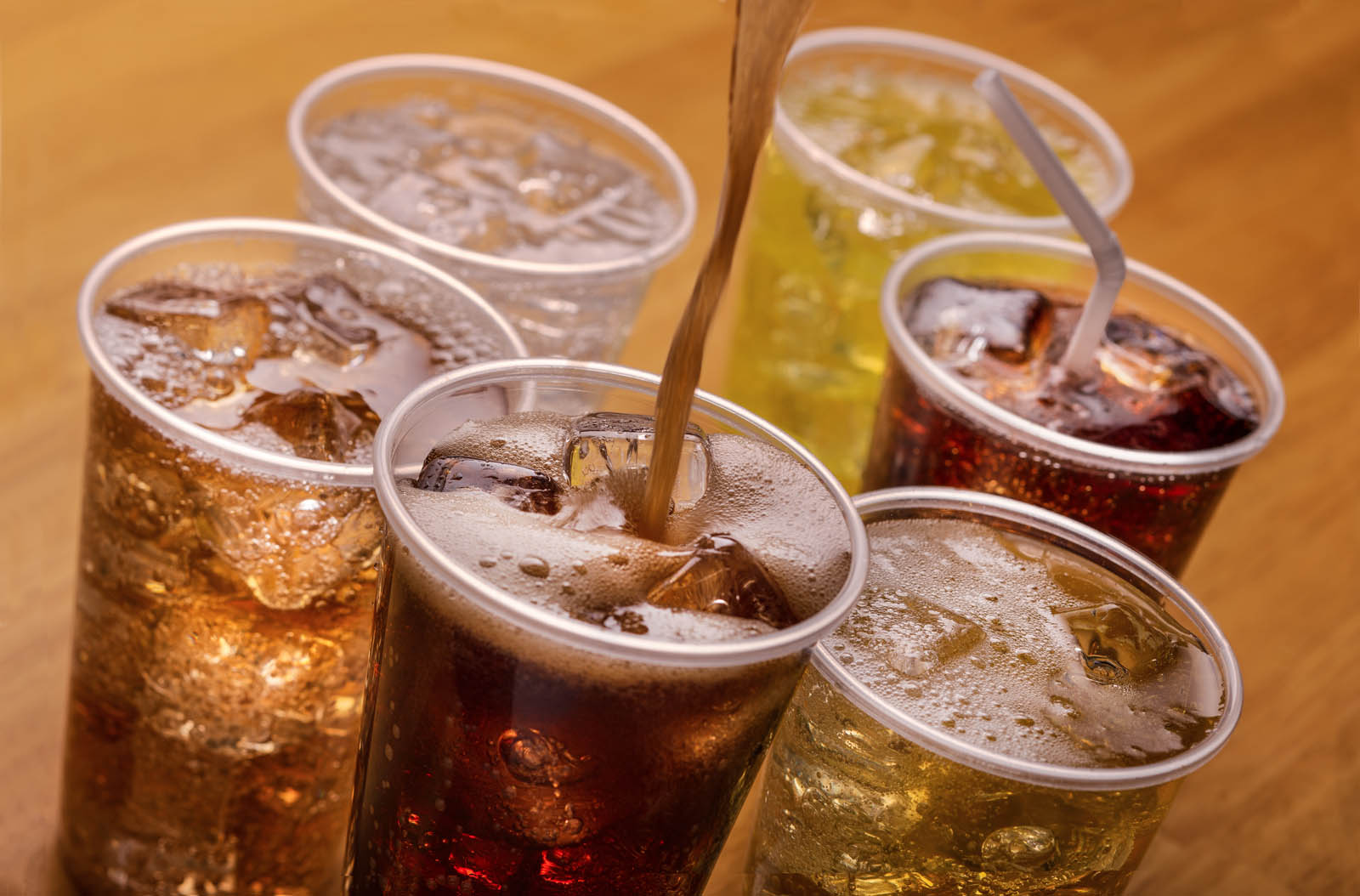
food & beverage
Key challenges we address:.
- how do we stay relevant in the world of fast-moving consumer goods?
- how do we adapt products and marketing for post-pandemic life?
- How do I retain the new customers I have attracted during the pandemic?
- how do we shift marketing strategies to reach consumers who are shopping both brick and mortar and online?
- how do we get customers to try something new?
- how do we optimize products that aren’t quite ready for market?
fresh, actionable insights
To stay relevant in the market, you need the latest data and the most accurate insights. And you need them quickly so you can launch new products and marketing approaches with confidence before the market shifts again.
C+R Research is one of the most established and trusted food and beverage market research companies in the country. For decades, we’ve been helping brands grow by applying a combination of tried-and-true methods and exploratory approaches that get fresh, immediately actionable insights.
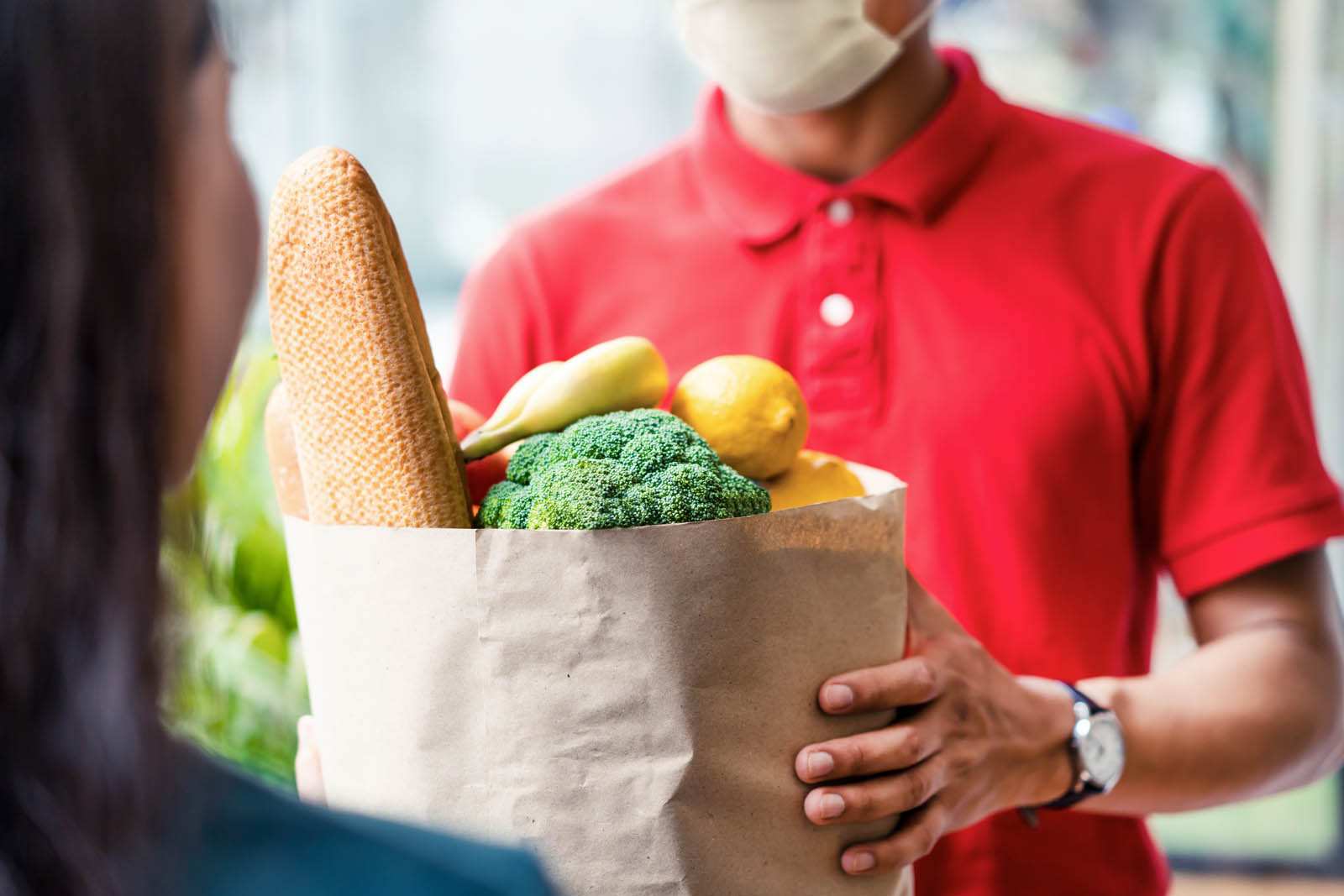
custom market research solutions
Competitive landscape, concept testing, consumer empathy, focus groups, package testing, product testing, shop-alongs, we position you for long-term success.
Whether you’re seeking tactical or exploratory research; qualitative or quantitative methods; optimizing an existing product or launching something brand new, C+R Research is your ideal partner for food and beverage industry research.
We immerse you in your consumers’ world so that you can gain a deep understanding of their tastes, perspectives and desires. Our job isn’t finished when we deliver your report. We develop long-term relationships that position you for success now and with every future shift in the market.
In an industry where agility and innovation are essential, C+R Research has the Food and Beverage insights you need to stay ahead of the curve.
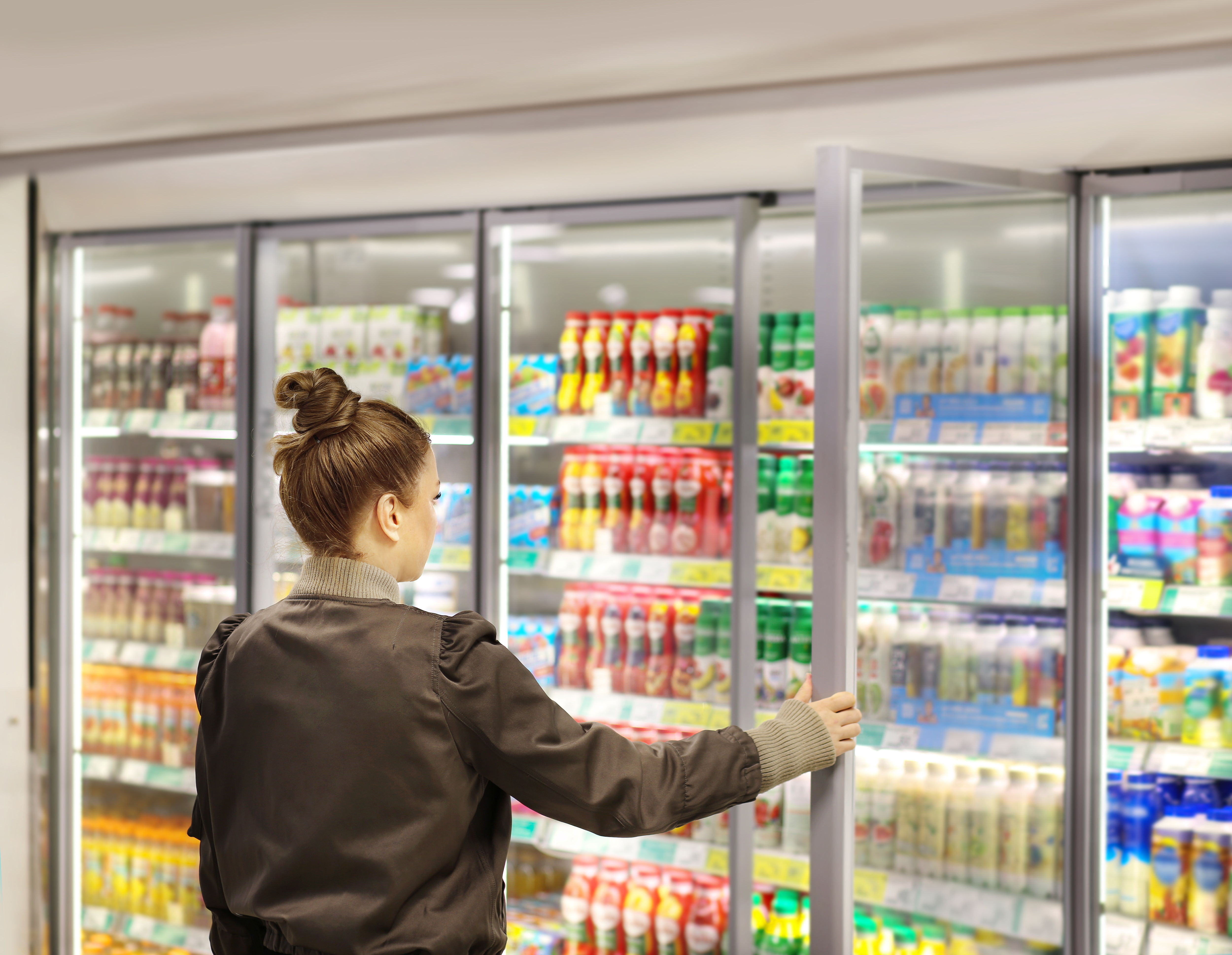
Proven Experience
Featured food & beverage case studies, assessing the physical and digital shopper journey to…, online community: a hub for innovation and connection…, identifying beverage shoppers’ pain points and…, finding the perfect brand story with qualitative…, using livehive to develop and optimize positioning, getting quick insights on consumers’ perceptions of….
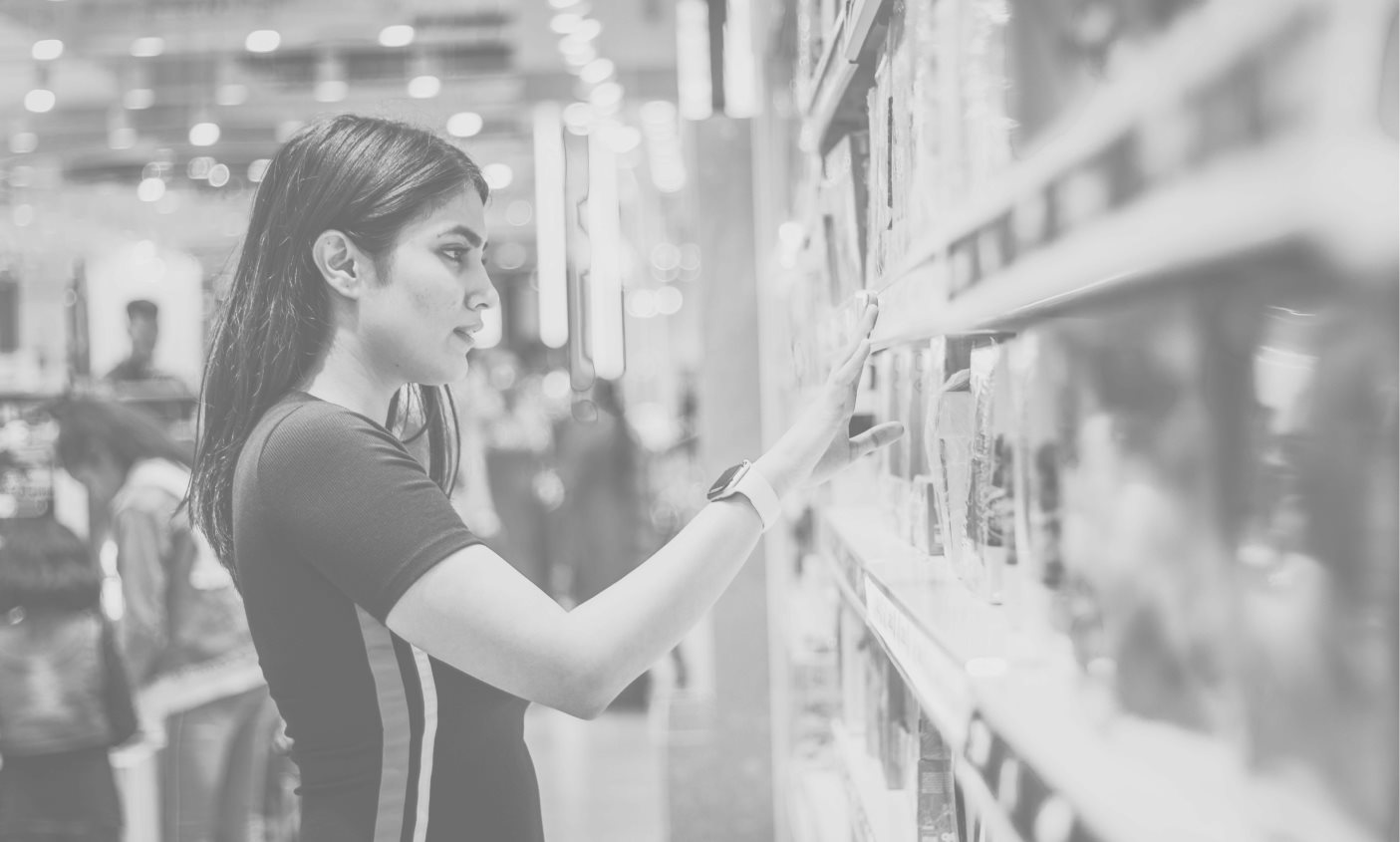
Browse our food & beverage related resources
Tasting history: celebrating the diverse culinary…, navigating the 2024 grocery landscape: insights from…, how glp-1 prescriptions are reshaping food and beverage…, ai dilemmas in the grocery sector: opportunities for…, millennial and gen z omnishoppers: it’s about…, the future of grocery shopping: a hyper-personalized….
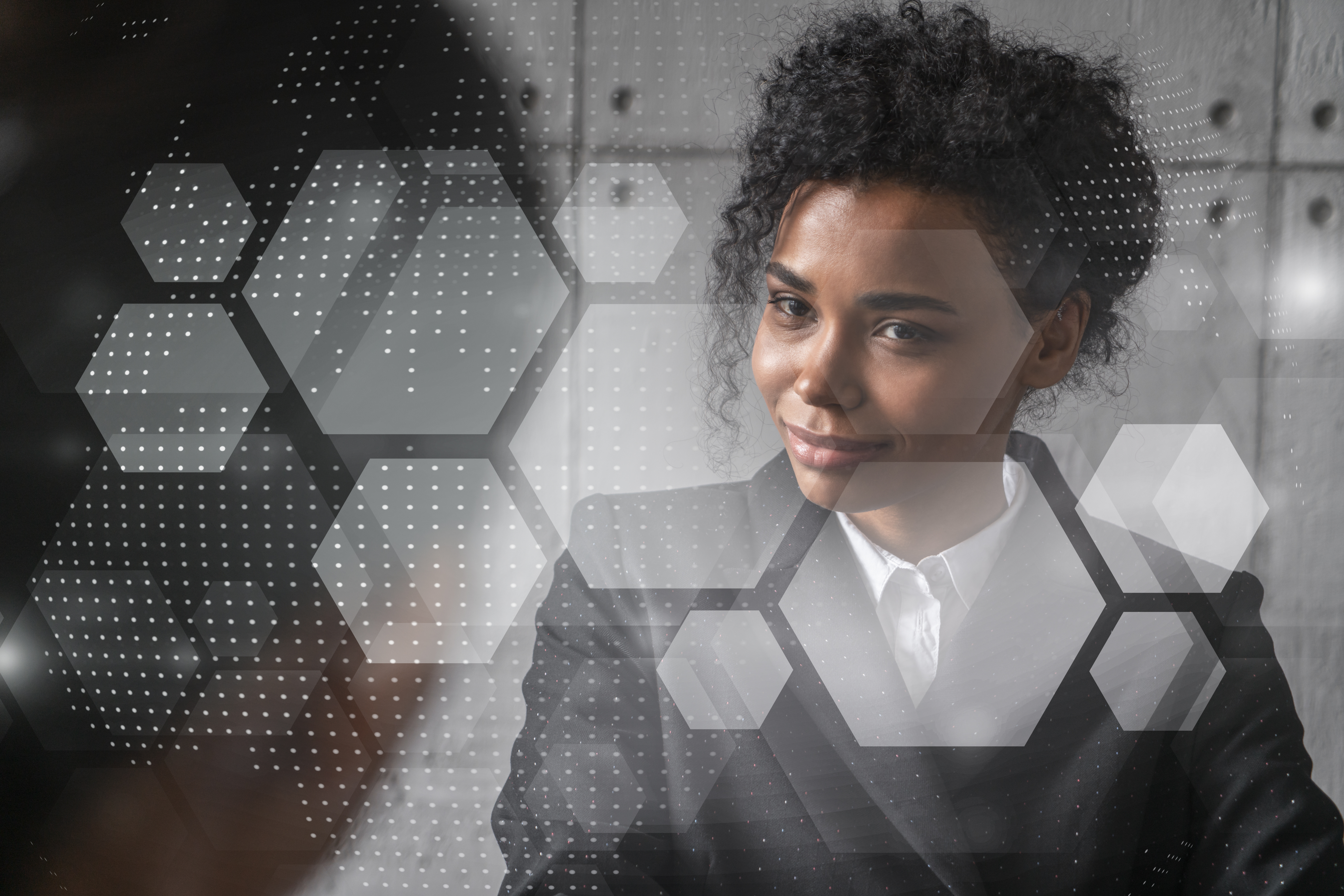
take the next step
Let’s discuss your upcoming project, i do want to say that this project again demonstrates why i have come to rely on c+r and recommend you so highly. when i get a report from you it’s complete, well-thought, and always client-ready. i really appreciate the partnership i have with you., it was always a pleasure working with the team at c+r. i really appreciated your expertise and true partnership on every project., truly appreciate the strength of the partnership we have created with you. thank you for being a consistent and reliable resource., the report goes beyond answering the questions ; it shows deep understanding of the needs of the client while ensuring the integrity of the design . thank you, thanks for one of the best reports i’ve received …. the quality of insights + deck writing > my expectations . the quality of voiceover/share out and process throughout has been my best experience to date . c+r shows up as a team-always on time, supportive of one another, and provides quality insights vs basic stuff., …we appreciate our open partnership and the constructive way we are building our relationship – we are enthusiastic when we think about future projects together , you offer your knowledge in a way that never making anyone feel like they should have known “that” about the audience, treat it as an opportunity to educate in a friendly way ., i’m really proud of this team and so appreciate the partnership we’ve created. you’ve both done a fantastic job helping us start off this journey and i look forward to continuing to build our learning with you all , search this site.
- Food Safety
- Upcoming Webinars & Online Events
- On-Demand Webinar Library
- Industry Reports
- Case Studies
- Press Releases
- Associations
- Trade Shows
- Download Our Media Kit
- Submit an Article for Publication on Food Industry Executive
- View Products
- List Your Products
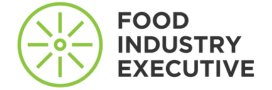
- Financial News & Capital Spending
Strong Food and Beverage Performance for Planned Industrial Projects Grew 32% in January 2023
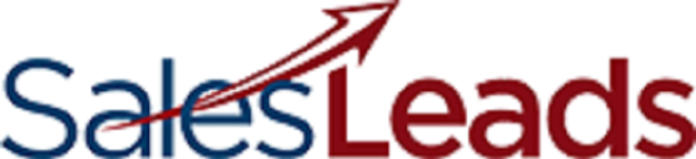
IMI SalesLeads announced today the January 2023 results for the new planned capital project spending report for the Food and Beverage industry. The Firm tracks North American planned industrial capital project activity; including facility expansions, new plant construction and significant equipment modernization projects. Research confirms 91 new projects in January as compared to 69 in December 2022 the Food and Beverage sector.
The following are selected highlights on new Food and Beverage industry construction news .
Food and Beverage Project Type
Processing Facilities – 72 New Projects
Distribution and Industrial Warehouse – 20 New Projects
Food and Beverage Project Scope/Activity
New Construction – 35 New Projects
Expansion – 20 New Projects
Renovations/Equipment Upgrades – 45 New Projects
Plant Closing – 2 New Projects
Food and Beverage Project Location (Top 10 States)
Florida – 7
Pennsylvania – 7
California – 5
Michigan – 5
Ontario – 5
Virginia – 5
Kentucky – 4
South Carolina – 4
Wisconsin – 4
Ohio – 3
Opportunities – By Equipment Need
Air Emissions Control Equipment – 66
Compressed Air Systems – 82
Control Systems and Instrumentation – 66
Conveyors – 76
Floor Coatings – 48
Material Handling/Storage Equipment – 88
Mechanical Construction – 76
Packaging Equipment – 61
Process Equipment – 59
Tanks/Vessels – 35
Largest Planned Project
During the month of January, our research team identified 7 new Food and Beverage facility construction projects with an estimated value of $100 million or more.
The largest project is owned by SK Food Group, who is planning to invest $205 million for the construction of a 525,000 sf processing facility in CLEVELAND, TN. They are currently seeking approval for the project. Construction is expected to start in late 2023, with completion slated for 2025.
Top 10 Tracked Food and Beverage Projects
Company Name: West Liberty Foods LLC
Food processing company is planning to invest $200 million for the construction of a 327,000 sf processing facility in KANSAS CITY, MO. They are currently seeking approval for the project.
Anticipated Investment: $200,000,000
Reason Behind Expansion: Establish a new processing facility
Tax Incentives: Undisclosed
Project Contacts:
Brandon Achen – President and CEO
Ed Claussen – Dir. Engineering and Maintenance
Chad Schnepper – Operations Mgr.
Equipment OppAlerts:
- Air Emissions Control Equipment
- Building Construction
- Compressed Air Systems
- Control Systems and Instrumentation
- Cranes and Hoists
- Fire Protection Equipment
- Floor Coatings
- Heat Exchangers
- HVAC Equipment
- Lift Trucks
- Loading Dock Equipment
- Material Handling/Storage Equipment
- Mechanical Construction
- Networking/Security Equipment
- Packaging Equipment
- Process Equipment
- Tank/Vessels – All
- Tanks/Vessels – Stainless
Company Name: Niagara Bottling LLC
Beverage company is planning to invest $160 million for the construction of a 500,000 sf processing facility in HAMMOND, LA. They have recently received approval for the project. Construction is expected to start in Spring 2023.
Anticipated Investment: $160,000,000
Tax Incentives: $750,000
Bill Hall – EVP, Manufacturing and Engineering
Bhaskar Tatke – VP, Supply Chain Planning
Todd Uhlick – VP, Expansion and Real Estate
Company Name: Upper Iowa Beef
Meat processing company is planning to invest $48 million for the expansion and equipment upgrades on their processing facility in LIME SPRINGS, IA. They have recently received approval for the project.
Anticipated Investment: $48,000,000
Reason Behind Expansion: Modernize equipment and expansion of their processing facility
Ed Greiman – General Mgr.
Dan Reynolds – Plant Mgr.
Michelle Ruggeberg – Purchasing Lead
SOUTH CAROLINA:
Company Name: Highland Baking Company
Bakery is planning to invest $35 million for the expansion and equipment upgrades on their processing facility in SPARTANBURG, SC. They have recently received approval for the project. Completion is slated for late 2023.
Anticipated Investment: $35,000,000
Ronnie Williams – Dir. Engineering
Ricky Lewis – Production Mgr.
Jimmy White – Asst. Maintenance Mgr.
SOUTH DAKOTA:
Company Name: CHS Inc.
Agribusiness company is planning for the construction of a 1.1 million sf grain house and shuttle complex in SIOUX FALLS, SD. They are currently seeking approval for the project. Construction is expected to start in Spring 2023.
Anticipated Investment: Undisclosed
Kevin Hall – VP, Supply Chain and Continuous Improvement Agriculture
Scott Pearson – Dir. Operations, EHS, and Energy Equipment
Roger Tschida – Dir. Real Estate
Company Name: Montana Specialty Mills LLC
Oilseed mill is planning to invest $15 million for the expansion and equipment upgrades on their processing facility in GREAT FALLS, MT. They are currently seeking approval for the project. Completion is slated for early 2024.
Anticipated Investment: $15,000,000
Dave Loboy – President
Todd Toney – Plant Mgr.
Christopher Knight – Dir. Operations
Company Name: IMG Citrus
Citrus grower is planning for the renovation and equipment upgrades on a recently acquired 185,000 sf processing, warehouse, and cold storage facility in VERO BEACH, FL.
Reason Behind Expansion: Modernize equipment and renovation of a processing, warehouse, and cold storage facility
Melanie Ressler – Co-CEO
Timothee Sallin – Co-CEO
Chloe Gentry – Co-CEO
- Building Renovation
CALIFORNIA:
Company Name: Costco Wholesale Corporation
Global food and retail chain is planning for the construction of a 161,000 sf warehouse at 3881 E. Commerce Way in SACRAMENTO, CA. They are currently seeking approval for the project.
Reason Behind Expansion: Establish a new warehouse facility
Mark Mattis – VP Supply Chain
Tim Anderson – AVP Construction
Seth Katz – AVP Real Estate
Company Name: International Food Solutions
Frozen food processing company is planning to invest $9.6 million for the renovation and equipment upgrades on a recently acquired 29,000 sf processing facility in CLEVELAND, OH.
Anticipated Investment: $9,600,000
Reason Behind Expansion: Modernize equipment and renovation of a processing facility
Lincoln Yee – Co-President
Allan Lam – Co-President
Aahtyn Hilger – VP Operations
Company Name: Marquez Brothers International, Inc.
Cheese mfr. is planning for a 55,000 sf expansion, renovation, and equipment upgrades on their processing facility in HANFORD, CA. They are currently seeking approval for the project. Construction will occur in 4 phases.
Reason Behind Expansion: Modernize equipment, expansion, and renovation of their processing facility
Victor Flores – EHS Dir.
Jason Firkus – Project and Infrastructure Manager
Mike Quezada – Production Mgr.
About IMI SalesLeads, Inc.
Since 1959, IMI SalesLeads, based in Jacksonville, FL is a leader in delivering industrial capital project intelligence and prospecting services for sales and marketing teams to ensure a predictable and scalable pipeline. Our Industrial Market Intelligence, IMI identifies timely insights on companies planning significant capital investments such as new construction, expansion, relocation, equipment modernization and plant closings in industrial facilities. The Outsourced Prospecting Services, an extension to your sales team, is designed to drive growth with qualified meetings and appointments for your internal sales team. Visit us at salesleadsinc.com.
Each month, our team provides hundreds of industrial reports within a variety of industries, including:
- Industrial Manufacturing
- Food and Beverage
- Power Generation
- Pulp Paper and Wood
- Oil and Gas
- Mining and Aggregates
- Research and Development
- Distribution and Supply Chain
- Pharmaceutical
- Industrial Buildings
- Waste Water Treatment
- Data Centers
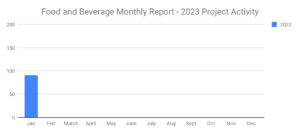
RELATED ARTICLES MORE FROM AUTHOR
Somic packaging has options for cheeseexpo audience, milk specialties global joins vanguard renewables’ farm powered strategic alliance, zag technical services announces new service offering, [report] trends & insights: optimizing food and beverage manufacturing facilities, [ebook] tales of triumph from the factory floor, vol. 1, [whitepaper] food and beverage 2024 trends and outlook for north america, [on-demand webinar] recipe for success: the importance of recipe management in your erp solution, [on-demand webinar] tech talk: it’s time to embrace erp, upcoming events, the future of snack production: leveraging technology to address macrotrends, green hydrogen plants for enhanced sustainability, and economic viability, driving sustainability goals through third-party certifications, put our food to work, not waste | sustainability in the food industry, shaping the future of poultry welfare: a multistakeholder approach, industry insights, top 5 regulatory developments in the food industry in 2024, how to unlock change in a manufacturing environment, understand top trends to drive pet food brand growth, savoring the flavors of change in the food & beverage industry, from strategy to success: mastering erp implementation challenges.
- Submit an Article
- Privacy Policy
Starting to Think Sustainably: Addressing Big Issues Is Best Accomplished With...

An official website of the United States government
The .gov means it’s official. Federal government websites often end in .gov or .mil. Before sharing sensitive information, make sure you’re on a federal government site.
The site is secure. The https:// ensures that you are connecting to the official website and that any information you provide is encrypted and transmitted securely.
- Publications
- Account settings
Preview improvements coming to the PMC website in October 2024. Learn More or Try it out now .
- Advanced Search
- Journal List
An Updated Framework for Industry Funding of Food and Nutrition Research: Managing Financial Conflicts and Scientific Integrity
Brienna m larrick.
Institute for the Advancement of Food and Nutrition Sciences, Washington, DC, USA
Johanna T Dwyer
Jean Mayer USDA Human Nutrition Research Center on Aging, Tufts University, Boston, MA, USA
Tufts Medical Center, Boston, MA, USA
John W Erdman, Jr
Department of Food Science and Human Nutrition, University of Illinois at Urbana-Champaign, Urbana, IL, USA
Richard F D'Aloisio
Richard D'Aloisio Regulatory Consulting LLC, Oradell, NJ, USA
Wendelyn Jones
Associated data.
Although the food and beverage industry plays a critical role in advancing food and nutrition science, industry-funded research is subject to intense scrutiny as a result of various perceived and real biases related to funding sources. To address this, the Institute for the Advancement of Food and Nutrition Sciences (IAFNS) Assembly on Scientific Integrity has updated its Guiding Principles for Funding Food Science and Nutrition Research to provide a modernized framework for minimizing bias and promoting integrity in industry-funded research. Existing best practices for managing conflicts and maintaining trust in science, as well as coverage related to conflicts in industry-funded research, were reviewed to inform the development of the updated Guiding Principles. The updated Guiding Principles continue to provide conflict-of-interest guidelines to protect the integrity and credibility of the scientific record. These updates provide clarification, strengthen the guardrails that separate the funding from the science, and reflect the shift within the scientific community toward increased transparency and open science. If the principles are followed as intended, there should be little reason to dispute the results of industry-funded studies, other than to debate the science itself. This article issues a challenge to the research community to strive for just that.
Conflicts of Interest and Bias in Nutrition Research
Despite its prevalence in the food and nutrition sciences—including in highly regarded peer-reviewed journals—there is widespread skepticism about the credibility and transparency of industry-funded nutrition research ( 1–3 ). In recent years this conversation has had heightened visibility stemming from a variety of factors, including adoption within the scientific community of more robust standards for transparency and disclosure, discussions about trust in science reaching broader and more general audiences in the mass and social media, and exposés of partnerships with industry gone wrong ( 4 ). Moreover, this skepticism is not limited to the research itself; by extension, the credibility of investigators having research and advisory relationships with the food industry has been called into question, along with their suitability for serving on public panels ( 5 ). The underlying concern is one of trust: Does industry funding make research less valid and credible than that funded by other sources? For food and nutrition science in particular, can the results of studies funded by industry be trusted?
In evaluating whether a conflict of interest undermines a study's credibility or not, it is important to remember that interests, in and of themselves, are not necessarily determinants of bias. The definition of “interest” offered by the US Department of Health & Human Services Office of Research Integrity (ORI) is “a commitment, goal, or value held by an individual or an institution” ( 6 ). Interests can be financial, such as a food manufacturer's interest in turning a profit, or nonfinancial, such as an academic investigator's interest in building a compelling resume for tenure. When ≥2 interests that relate to an activity are contradictory, the conflict lies in the situation rather than in any behavior (or lack thereof) by the individual or entity. A conflict of interest, therefore, is not intrinsically a bad thing. As a hypothetical example, consider an investigator who has built a research career investigating the health benefits of a specific dietary pattern, and who also profits financially by authoring books about the healthfulness of that same dietary pattern. When that investigator applies for funding to advance that line of research, the potential for financial gain from book sales—particularly if the research is supportive of the dietary pattern—represents a financial conflict of interest. It is inherent to the situation, but it neither represents wrongdoing nor guarantees impartiality or bias on the investigator's part.
Bias, on the other hand, is what introduces error into the scientific process and requires active management by all participants to preserve the integrity of research, by identifying potential sources and taking measures to prevent its occurrence. In its simplest form, bias is a systematic and measurable deviation from the truth. There are many forms of bias, and the University of Oxford's Catalogue of Bias Collaboration has identified ≥49 types that can occur throughout various stages of the research life cycle and influence the magnitude and direction of research results ( 7 ). During study conceptualization, confirmation bias, for example, may lead an investigator to search for and use information that supports their own ideas or beliefs. In the selection of study participants, ascertainment bias may lead to systematic differences between the study sample and the target population. Recall bias (when accuracy and volume of memories are influenced by subsequent events and experiences) and the Hawthorne effect (when individuals modify their behavior in response to their awareness of being observed) are examples of biases that can occur during the conduct of research. In the reporting of research, positive results bias (the tendency to submit and publish positive rather than nonsignificant or negative results) and spin bias (distortions in the interpretation of research results) are just 2 examples that can surface. These biases and others can be introduced by poor study design and reporting practices, improper use of statistical and scientific methods, or from intentional misconduct—independently of funding source.
Although some researchers have pointed out what actually is or appears to be bias in industry-related research ( 8 ), bias can also exist in publications in opposition to industry interests. “White hat” bias, leading to distortion of information by the researcher in the service of what may be perceived to be righteous ends, has also been documented with both quantitative and anecdotal evidence ( 9 , 10 ). For example, in a meta-analysis of 88 studies, investigators examined the association between soft drink consumption and nutrition and health outcomes. A positive association was found between soft drink intake and increased energy intake and body weight, with studies funded by the food industry reporting significantly smaller effect sizes than non-industry-funded studies ( 11 ). Although action by the food industry to bias study results in an attempt to minimize any association between soft drink intake and body weight is one conceivable explanation for this, independent investigators found a different underlying factor. An examination of the meta-analysis data file revealed that a standard test of publication bias, which determines whether the probability of a study being published is dependent on its outcome, was significant ( 9 ). This indicates that investigators were more likely to publish positive statistically significant findings than null findings. Interestingly, this bias was present only for non-industry-funded research, suggesting non-industry-funded scientists tended to not publish their studies if they did not show a significant positive association between soft drink intake and increased energy intake or body weight. These findings reinforce that all investigators—regardless of funding source—must be active and vigilant in minimizing bias.
As a final example, confirmation bias, or the tendency to interpret new evidence in support of existing theories or beliefs, can skew findings in favor of any interest. Revisiting the previous example of the dietary pattern investigator/book author, this person's research would be considered biased if the study was designed to favor a particular hypothesis, if it excluded relevant data that were in opposition to the investigator's hypothesis, or if the investigator published only the subset of results that supported the healthfulness of the diet while not mentioning negative or null findings. Ultimately, bias can present itself in a multitude of ways, with no person, entity, or sector free from its grasp.
Existing Checks on Bias
The scientific community has a system of checks and balances in place to detect and flag improper research practices, bias, scientific misconduct, and fraud—instances of which happen within all sectors. Pressure from peers and others serves as a check on such behaviors, with scrutiny coming from meetings, conferences, and other professional engagements; from tenure and promotion decisions within universities and research institutions; in commentaries and letters to the editor in peer-reviewed journals; and, importantly, from the process of peer review during research proposal evaluation and manuscript publication. Imperfect as it may be ( 12 ), the peer review process in particular serves as an important gatekeeper of scientific integrity, helping to ensure that research entering the public domain is designed to appropriately answer the question at hand, and that conclusions of the authors are substantiated by the evidence presented. Innovative publication formats such as the Center for Open Science's Registered Reports take the review process one step further, allowing peers to review and provide feedback on the research design itself before study initiation ( 13 ), rather than initiating peer review on the report/publication of what has already happened as in the traditional peer review system. This innovative workflow can help eliminate questionable research practices, including publication bias. Finally, for work that has not yet undergone peer review, preprint servers offer the opportunity for the wider scientific community to review and comment on work in advance of publication.
The government also implements checks against bias and improper research practices in grants and contracts. The US National Library of Medicine's database clinicaltrials.gov serves as an important check against publication bias, as well as in differentiating confirmatory from exploratory research ( 14 ). Moreover, there is government oversight within the granting agencies and federal research establishment itself, such as the US Department of Health and Human Services ORI ( 15 ) and similar bodies in other federal agencies. These offices develop policies, procedures, and regulations related to the prevention of research misconduct, executing misconduct investigations, and implementing programs and activities to promote responsible conduct of research. In addition, the National Academies of Sciences, Engineering, and Medicine has announced the creation of a new body called the Strategic Council for Research Excellence, Integrity, and Trust ( 16 ). The new Strategic Council is charged with “advancing the overall health, quality, and effectiveness of the research enterprise across all domains that fund, execute, disseminate, and apply scientific work in the public interest.” Conflicts of interest, assessment of researchers, and retractions represent just a few of the potential topics for the Strategic Council to address.
Finally, the mass media and consumers of science may also exert pressures that serve to mitigate bias and research impropriety. Through mass and social media, broad audiences are able to participate in dialogue on research methods and findings. These channels can, however, present a double-edged sword; although they facilitate communication among peers, participants in the public discourse often have diverse levels of understanding of the nuances of specific areas of research, with bots and clone accounts playing a significant role in the science communications landscape on social media ( 17 ). Further, there is no agreed-upon process for setting the record straight, as is the case with retractions and corrections in the peer-reviewed literature, should actors in these discussions be biased themselves.
Although these systems serve to bolster confidence in published works, they do not guarantee research quality or the absence of bias. So then, how does one evaluate a study in the face of real, potential, or perceived biases? Most scientists would agree that what matters most when considering the credibility of research is the rigor, quality, and transparency of the underlying science. The following set of questions can be used to assess these qualities ( 18 ):
- Is the research question important?
- Does the study design address the question?
- Were steps taken to ensure objectivity?
- Was the study well performed?
- Were the proper statistical analyses used?
- Are the conclusions drawn supported by the evidence presented?
- Is enough information reported for the reader to answer these questions?
If the answers to the foregoing questions are “yes,” disputes that arise over the results of such a study are likely to be motivated by factors other than the science itself. When pursuing research relationships and partnerships within and across sectors, having an established set of guidelines in place and enforced for protecting the integrity of these qualities and minimizing bias allows for greater scientific advancement than does avoiding any relationship in which a potential conflict of interest exists.
Need for an Updated Framework
In 2009, a set of 8 Guiding Principles were developed by the International Life Sciences Institute (ILSI) North America [now an independent organization, the Institute for the Advancement of Food and Nutrition Sciences (IAFNS) evolved from ILSI North America] to serve as a checklist for minimizing funding-related bias in research results from industry-funded activities. They were the first of their kind, appearing simultaneously in full or in excerpt form in 6 peer-reviewed journals ( 19–24 ).
In the years since the Guiding Principles were first published, there have been shifts in how research is conducted and communicated, and where people turn to get information. The last decade has seen the introduction and rise in popularity of mechanisms that provide immediate access to reviewed and unreviewed research. Some journals post full-text copies of accepted manuscripts before copyediting, typesetting, and review of the final proof by the authors. Online preprint servers such as bioRxiv ( 25 ) offer early public access to unpublished research before it undergoes peer review. Platforms such as the Center for Open Science's Open Science Framework enable investigators to share their research throughout the entire project cycle, from experimental design preregistration to data collection to report publication, even offering viewers suggested citation formats to reference active, ongoing work ( 26 ). As a result, research enters the public domain early and often, having undergone varying levels of review by peers.
A second reason for updating the Guiding Principles is greater attention to issues of conflicts of interest and bias in scientific publications. Although conflicts of interest do not necessarily introduce bias into the research process, it is nonetheless essential that mechanisms are in place to safeguard the science against potential bias, and that these mechanisms reflect the current research environment. The Global Research Report on Research Integrity developed by the Institute for Scientific Information highlights key points along the research and publication cycle that are susceptible to violations of research integrity ( 27 ):
- Research problem, literature review, hypothesis and plan
- Research, experiment, and data collection
- Data analysis, hypothesis testing, data preservation
- Manuscript preparation
- Choice of publication venue and submission
- Editorial and peer review process, including revisions
- Publication
- Use of the publication record
Actions that have undue influence on the design, conduct, and reporting of research include egregious forms of misconduct such as falsification, fabrication, and plagiarism. More subtle actions include questionable research practices, misrepresentation, and other actions that may be intentional or the result of unintentional or unrecognized biases. It is imperative that investigators—and all others involved in the research process, regardless of sector—have measures in place to safeguard against these violations of integrity.
In the years since the original Guiding Principles were developed ( 19 ), other sets of guidelines and principles for ensuring integrity in research and trust in science have also been published ( 12 , 28–31 ), but these offer investigator-specific guidance for the conduct of research itself and do not offer specific guidance for funders and investigators to address potential sources of bias in industry-funded food and nutrition research. Recognizing that the food industry does and will continue to play a significant role in supporting nutrition research, the IAFNS Assembly on Scientific Integrity has updated its Guiding Principles for Funding Food Science and Nutrition Research to provide a modernized framework for avoiding bias in industry-supported research. These updates provide clarity, reflect a shift toward increased transparency and open science, and strengthen the guardrails that separate the funding from the science.
To inform the development of the updated Guiding Principles, existing best practices for managing conflicts and maintaining trust in research ( 12 , 19 , 28–35 ) and coverage related to conflicts in industry-funded research ( 1 , 2 , 4 ) were reviewed. The revised Guiding Principles were then reviewed by an external set of stakeholders, including those from nutrition and food safety professional societies. Although these guidelines are written for providing information relevant to the context of public–private research relationships, they are applicable to research relationships between and within any sector.
Guiding Principles: An Updated Framework for Industry Funding of Food and Nutrition Research
In the conduct of public–private research relationships, all relevant parties shall:
- Conduct or sponsor research that is factual, transparent, and designed objectively; according to accepted principles of scientific inquiry, the research design will investigate an appropriately phrased hypothesis and/or question, rather than favor a particular outcome;
- Require control of the study design, the research itself, and the interpretation of findings to remain with scientific investigators;
- Neither offer nor accept remuneration geared to the outcome of a research project;
- Before the commencement of studies, ensure that there is a written agreement that the investigative team has an obligation to attempt to publish the findings within some specified timeframe and the freedom to choose the journal to which the work will be submitted;
- Require, in publications and conference presentations, full written or oral disclosure, as appropriate, of all relevant relationships (financial and nonfinancial);
- Not participate in undisclosed authorship arrangements in publications or presentations;
- Guarantee accessibility to all data and control of statistical analysis by investigators and appropriate auditors/peer reviewers; when possible, encourage the practice of open science, including depositing data and methodology on a public repository;
- Require that academic researchers, when they work in contract research organizations or act as contract researchers, make clear statements of all their affiliations; require that such researchers publish under the auspices of the contract research organization;
- Require, in publications and conference presentations, disclosure of whether the funder advised on the study design, conduct of research, and/or the development of the manuscript.
Notes: Guideline 3 does not prohibit the sponsoring agency from awarding funds using a phased approach, in which decisions to proceed to subsequent phases of a project are dependent upon the results of the preceding phase. However, in this case the decision criteria for each phase must be identified in advance in the written Agreement, before the first phase begins. It also does not preclude deciding which, if any, new work is to be undertaken based on results of a prior study. Guideline 4: The investigator's obligation to attempt to publish the research findings should persist independently of whether the results are expected or unexpected. Guideline 7 is intended to apply to peer reviewers not associated with the funding entity and appropriate scientific auditors. For those practicing open science, the Center for Open Science's Open Science Framework is an example of a suitable option for making data, analyses, methodology, and code available to the public, and is available to researchers at no cost. Guideline 8: Such academic researchers who are working in a contract research organization are encouraged to disclose their academic affiliation as well when publishing under the auspices of the contract research organization.
Like its predecessor, this set of revised Guiding Principles is intended to be dynamic, and to prompt ongoing discussion and refinement in an effort to foster a culture of integrity in industry-supported research. Supplemental Table 1 provides a side-by-side comparison of the original and the updated Guiding Principles. Many of the updates to the Guiding Principles (and to their notes) are meant to simplify language and provide improved clarity. However, several substantive updates were also made to make explicit certain items related to scope that were previously only implied. For example, Guiding Principle 2 has been updated to specify that in addition to maintaining control of the study design and the research itself, investigators also maintain control of the interpretation of data generated as part of the study. Whereas discussion of research findings—ideally with a diverse group of peers—remains an important part of scientific advancement, compulsory adherence to “interpretive guidance” from the funder has no place in public–private research relationships.
Updates have been made to the guidelines to address actions and processes that occur pre- and post-publication. The Guiding Principles now specify that not only does the investigative team have the freedom and obligation to publish, but they also retain the freedom to choose the most appropriate journal to which to submit their work. The Guiding Principles continue to highlight the importance of attempting to publish all research, even if the results are unanticipated or null, and even if it takes multiple submissions to find an appropriate peer-reviewed journal. Guiding Principle 6, which prohibits undisclosed authorship in scientific publications and presentations, has been expanded to include all types of arrangements—paid or otherwise—and made independent of which sector or entity is the sponsor. The purpose of the seventh Guiding Principle is to enable independent investigations of submitted work during and after the peer review process. This update to the Guiding Principles clarifies that this is meant to apply to peer reviewers and independent investigators; it is not meant to guarantee accessibility of the data to the entire public. However, it is recognized that the practice of open science has many benefits, including promoting actions that can increase the quality and reliability of scientific work ( 36 ). Open science therefore is now encouraged where possible, and the Center for Open Science's Open Science Framework ( 26 ) is referenced as an example of one suitable platform for exercising open science. Finally, the modification to the eighth Guiding Principle was made to recognize that many academic scientists hold joint appointments at private consulting firms or other establishments, and in these cases all relevant affiliations should be disclosed.
On the topic of disclosures, updates have been made to the requirements outlined in Guiding Principle 5. The language was updated from requiring “full signed disclosure” of interests to “full written or oral disclosure, as appropriate,” because signed disclosure is not an applicable or effective means of disclosure in the context of conferences or other presentations. In addition, the phrase “financial interests” has been replaced with “relevant relationships,” in recognition that not all interests pertaining to the conduct of research are financial.
Finally, in this update a new Guiding Principle 9 was established with the aim of providing greater transparency of relevant interactions between the funder and the investigator in public–private research relationships. This new Guiding Principle requires disclosure of whether the funder advised on the study design, conduct of research, and/or the development of the manuscript. It is recognized that whether and how the funder is involved in the development, conduct, and interpretation of research may significantly affect what and how results are presented in the peer-reviewed literature. Although requests for improved clarity and refined scope are a routine part of the proposal review and grants administration process—including for federally funded research—disclosure of engagement above and beyond this will help investigators avoid the perception of concealing information relevant to the study at hand and provide readers with context about how the study's findings were derived. This Guiding Principle is intended to achieve more robust disclosures of funder–investigator interactions; however, it should not be interpreted as granting funders of the work the authority to direct or approve the research results, discussion, or conclusions. These types of interactions are what the updated Guiding Principles are intended to prevent.
With the limited availability of funds from US federal agencies, nongovernmental sources of funding, including industry, play a critical role in offering new opportunities for advancement and innovation and relieving pressure on government agendas and budgets to respond to all research needs. In the United States, diet-related diseases such as obesity, diabetes, cardiovascular disease, and cancers cause half a million deaths each year. Further, it is estimated that 85% of national health care spending is due to management of diet-related chronic diseases; US government expenditures on direct medical care for diabetes alone are estimated at $160 billion/y ( 37 ). Compared with the magnitude of these burdens, resources available for research on the health effects of foods and nutrients are limited. In 2020, the NIH—the nation's largest funder of nutrition research—spent an estimated 4.5% ($1.9 billion) of its budget on nutrition research ( 38 ). The second-largest funder of nutrition research in the United States, the USDA, spent a fraction of that, with the Agricultural Research Service (ARS) allocating $45 million to nutrition-related research. An additional $500 million was spent by the USDA's National Institute of Food and Agriculture (NIFA) on its Agriculture and Food Research Initiative (AFRI); this program largely supports agriculture-related research but does include some related to food safety, nutrition, and health ( 39 ).
With the relevant scientific expertise and the resources to support scientific research, the food industry is well-equipped to engage in the pursuit of advancing food and nutrition science. The industry's interest in maintaining profitability does not negate its inherent interest in not harming its consumer base, nor that it is legally responsible for demonstrating the safety of its products and ensuring that any nutrient content and/or health claims it makes about them are substantiated ( 40–43 ). The food industry has specialized scientists with backgrounds in chemistry, biology, toxicology, nutrition, food science, and epidemiology—with skills that are honed in applied nutrition and food safety—and an intimate knowledge of the wants and needs of consumers. Moreover, it has unique expertise that does not typically exist in other food and nutrition research settings, including knowledge of food and dietary supplement supply chains, commercial-scale ingredient substitutions, and testing methods for various food matrices, all of which are needed to realize shifts in product nutrient composition for the betterment of public health.
Industry scientists are not a niche group; in a trend of increasing private sector employment of scientists—particularly in the life and health sciences—educational institutions now employ fewer than half of PhD scientists in the United States ( 44 ). For nutrition specifically, the 2019 Survey of Doctorate Recipients found that the private sector—including private for-profit, private not-for-profit, and self-employed business owners—employed 39% of PhD nutrition scientists in the United States, with 54% and 7% employed by educational institutions and government, respectively ( 45 ). As might be expected, food scientists are employed by the private sector at a higher rate, with ∼57% of PhD food scientists in the United States employed by the private sector, and 33% and 9% employed by educational institutions and government, respectively ( 45 ).
In addition to offering investigators and their institutions opportunities for scientific advancement and innovation, multisector collaborations may also have the added benefit of improving research quality. A retrospective cross-sectional study evaluated the quality of studies used in systematic reviews extracted from 3 databases: the Academy of Nutrition and Dietetics’ Evidence Analysis Library, the Agency for Healthcare Research and Quality (AHRQ) Evidence-Based Practice Center Reports, and the USDA Nutrition Evidence Library ( 46 ) (this study was supported by ILSI North America). In this study, 5 risk-of-bias domains—selection bias, performance bias, detection bias, attrition bias, and reporting bias—were evaluated in conjunction with other variables to quantify the research quality of >5600 nutrition-related studies. The investigators found that studies with “combined” funding sources—typically the work of public–private research partnerships—were higher in quality, by and large, than studies with single-source funding. Moreover, the review found that industry-funded studies were generally not lower in quality than those funded solely by government; publication year and study design were more consistent predictors of research quality than study funding source. As evidenced by emphasis on team science, cross-disciplinary and interdepartmental collaborations in research institutions, and federal calls for proposals, it is widely recognized that there is a benefit for research collaboration as experts bring different perspectives to the table.
The Guiding Principles provide conflict-of-interest guidelines to protect the integrity and credibility of the scientific record, and the updates presented herein strengthen the guardrails that separate the funding from the science and reflect the shift within the scientific community toward increased transparency and open science. Although the updated Guiding Principles more accurately represent the spirit of “full disclosure” in today's research environment, they by no means solve the dilemma that exists with disclosures of interests and relevant relationships. There is no consensus on what constitutes disclosure-worthy information, particularly as it relates to nutrition research ( 47 , 48 ). Further, scientific journals—including those related to food and nutrition science—have inconsistent guidelines and requirements for author disclosures, making a process that already requires a great deal of subjectivity even more cumbersome. What the investigator considers to be “relevant” to the work at hand may differ from what peers or readers consider to be relevant. Should past relationships be declared in addition to current ones? What about future commitments? Are relationships of the investigator's immediate family members “relevant”? In research on dietary patterns, at what point do the investigator's own food preferences or religious/philosophical beliefs become “relevant”? Inevitably, investigators are occasionally called out for failing to disclose certain relationships, and they commonly respond, “I didn't think I needed to declare that.”
Some organizations have already made efforts to standardize disclosure guidelines. For example, the International Committee of Medical Journal Editors (ICMJE) has created a uniform form ( 49 , 50 ) for disclosure of potential conflicts of interest which is utilized by all ICMJE journals ( 51 ). As recommended in the Blue Ribbon Panel's report “Best Practices in Nutrition Science to Earn and Keep the Public's Trust” ( 28 ), the ASN has created a Model Disclosure Form that covers financial and other conflict of interest sources to serve as a model for its members, stakeholders, and staff. A possible strategy cited by the National Academies of Sciences, Engineering, and Medicine Strategic Council for this issue is the extension of publication lists on Open Researcher and Contributor ID (ORCID) to include lists of current and prior employment, activities, commitments, and financial holdings ( 16 ). This information would be publicly available for readers to view and decide which relationships might be relevant. Although there is much discussion and debate around disclosures in nutrition research, there is general agreement that transparency in this area is necessary to encourage public trust in science.
Regarding the implementation of the Guiding Principles presented herein, organizations wishing to adopt these guidelines must develop a plan that outlines how they will be implemented, monitored, and enforced. An essential component of such a plan is ensuring transparency and understanding of the Guiding Principles by all relevant parties, including individuals within the funding organization as well as those at the research-performing organization. This could be accomplished, as IAFNS itself does, by including the Guiding Principles—and the expectation that they will be adhered to by all parties in resulting research partnerships—on public-facing websites, in calls for research proposals, and in formal research agreements. Communications between the funder and investigator should foster a culture of integrity and transparency. Funding organizations may find internal audits and/or other tracking systems to be helpful in monitoring whether the Guiding Principles are being implemented as intended.
In conclusion, the updated Guiding Principles presented here provide a modernized framework for minimizing funding-related bias in research results from industry-funded activities. This updated framework takes into account shifts within the scientific community toward open science and more robust disclosures of relevant relationships, and it strengthens the guardrails that separate the funding sources from the science. We hope these Guiding Principles will serve as a guide for those engaging in public–private research relationships, and that all involved parties will advocate for their vigorous implementation and enforcement. If the Guiding Principles are followed as intended, there should be little reason to dispute a resulting study, other than to debate the science itself. Let this be a challenge to the research community to strive for just that.
Supplementary Material
Nxac106_supplemental_file, acknowledgements.
We thank the public and private sector members of IAFNS's Assembly on Scientific Integrity, and others, who reviewed and provided comments on the initial proposed updates to the Guiding Principles. The authors’ responsibilities were as follows—BML: wrote the manuscript; and all authors: contributed to the development of the updated Guiding Principles, critically reviewed, commented on, and edited manuscript drafts, and read and approved the final manuscript.
Supported by the Institute for the Advancement of Food and Nutrition Sciences (IAFNS) Assembly on Scientific Integrity. IAFNS is a nonprofit science organization that pools funding from industry collaborators and advances science through the in-kind and financial contributions from public and private sector participants.
Author disclosures: JTD serves as editor of Nutrition Today ; contractor at ICF International Inc. at the NIH Office of Dietary Supplements; Scientific Advisory Board member for the Mushroom Council, McCormick Science Institute, and Bay State Milling; advisor to the IAFNS Low- and No-Calorie Sweeteners and Bioactives Committees; Governance Committee member of International Life Sciences Institute (ILSI) US and Canada; served as a one-time consultant for Nestlé in 2020; and was formerly an ILSI North America Board member (until 2015). JWE has received grants from NIH and Abbott Nutrition and a USDA National Institute of Food and Agriculture Graduate Fellowship; consulting fees from Mars, Inc.; honoraria from DSM; is a Board member of IAFNS and the Soy Nutrition Institute—Global; and a former Board member of ILSI North America. RFD was formerly employed by Mondelez International (Mondelez is a member of IAFNS) and has received consulting fees from Clif Bar & Company and support for meeting travel from Q1 Productions. WJ was formerly employed by Corteva Agrisciences. BML reports no conflicts of interest.
JWE is on the Editorial Board of The Journal of Nutrition .
Supplemental Table 1 is available from the “Supplementary data” link in the online posting of the article and from the same link in the online table of contents at https://academic.oup.com/jn/ .
Abbreviations used: IAFNS, Institute for the Advancement of Food and Nutrition Sciences; ICMJE, International Committee of Medical Journal Editors; ILSI, International Life Sciences Institute; ORI, Office of Research Integrity.
Contributor Information
Brienna M Larrick, Institute for the Advancement of Food and Nutrition Sciences, Washington, DC, USA.
Johanna T Dwyer, Jean Mayer USDA Human Nutrition Research Center on Aging, Tufts University, Boston, MA, USA. Tufts Medical Center, Boston, MA, USA.
John W Erdman, Jr, Department of Food Science and Human Nutrition, University of Illinois at Urbana-Champaign, Urbana, IL, USA.
Richard F D'Aloisio, Richard D'Aloisio Regulatory Consulting LLC, Oradell, NJ, USA.
Wendelyn Jones, Institute for the Advancement of Food and Nutrition Sciences, Washington, DC, USA.
Thank you for visiting nature.com. You are using a browser version with limited support for CSS. To obtain the best experience, we recommend you use a more up to date browser (or turn off compatibility mode in Internet Explorer). In the meantime, to ensure continued support, we are displaying the site without styles and JavaScript.
- View all journals
- My Account Login
- Explore content
- About the journal
- Publish with us
- Sign up for alerts
- Open access
- Published: 26 March 2024
Predicting and improving complex beer flavor through machine learning
- Michiel Schreurs ORCID: orcid.org/0000-0002-9449-5619 1 , 2 , 3 na1 ,
- Supinya Piampongsant 1 , 2 , 3 na1 ,
- Miguel Roncoroni ORCID: orcid.org/0000-0001-7461-1427 1 , 2 , 3 na1 ,
- Lloyd Cool ORCID: orcid.org/0000-0001-9936-3124 1 , 2 , 3 , 4 ,
- Beatriz Herrera-Malaver ORCID: orcid.org/0000-0002-5096-9974 1 , 2 , 3 ,
- Christophe Vanderaa ORCID: orcid.org/0000-0001-7443-5427 4 ,
- Florian A. Theßeling 1 , 2 , 3 ,
- Łukasz Kreft ORCID: orcid.org/0000-0001-7620-4657 5 ,
- Alexander Botzki ORCID: orcid.org/0000-0001-6691-4233 5 ,
- Philippe Malcorps 6 ,
- Luk Daenen 6 ,
- Tom Wenseleers ORCID: orcid.org/0000-0002-1434-861X 4 &
- Kevin J. Verstrepen ORCID: orcid.org/0000-0002-3077-6219 1 , 2 , 3
Nature Communications volume 15 , Article number: 2368 ( 2024 ) Cite this article
51k Accesses
856 Altmetric
Metrics details
- Chemical engineering
- Gas chromatography
- Machine learning
- Metabolomics
- Taste receptors
The perception and appreciation of food flavor depends on many interacting chemical compounds and external factors, and therefore proves challenging to understand and predict. Here, we combine extensive chemical and sensory analyses of 250 different beers to train machine learning models that allow predicting flavor and consumer appreciation. For each beer, we measure over 200 chemical properties, perform quantitative descriptive sensory analysis with a trained tasting panel and map data from over 180,000 consumer reviews to train 10 different machine learning models. The best-performing algorithm, Gradient Boosting, yields models that significantly outperform predictions based on conventional statistics and accurately predict complex food features and consumer appreciation from chemical profiles. Model dissection allows identifying specific and unexpected compounds as drivers of beer flavor and appreciation. Adding these compounds results in variants of commercial alcoholic and non-alcoholic beers with improved consumer appreciation. Together, our study reveals how big data and machine learning uncover complex links between food chemistry, flavor and consumer perception, and lays the foundation to develop novel, tailored foods with superior flavors.
Similar content being viewed by others
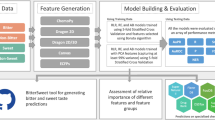
BitterSweet: Building machine learning models for predicting the bitter and sweet taste of small molecules
Rudraksh Tuwani, Somin Wadhwa & Ganesh Bagler
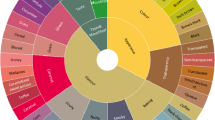
Sensory lexicon and aroma volatiles analysis of brewing malt
Xiaoxia Su, Miao Yu, … Tianyi Du
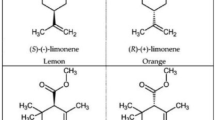
Predicting odor from molecular structure: a multi-label classification approach
Kushagra Saini & Venkatnarayan Ramanathan
Introduction
Predicting and understanding food perception and appreciation is one of the major challenges in food science. Accurate modeling of food flavor and appreciation could yield important opportunities for both producers and consumers, including quality control, product fingerprinting, counterfeit detection, spoilage detection, and the development of new products and product combinations (food pairing) 1 , 2 , 3 , 4 , 5 , 6 . Accurate models for flavor and consumer appreciation would contribute greatly to our scientific understanding of how humans perceive and appreciate flavor. Moreover, accurate predictive models would also facilitate and standardize existing food assessment methods and could supplement or replace assessments by trained and consumer tasting panels, which are variable, expensive and time-consuming 7 , 8 , 9 . Lastly, apart from providing objective, quantitative, accurate and contextual information that can help producers, models can also guide consumers in understanding their personal preferences 10 .
Despite the myriad of applications, predicting food flavor and appreciation from its chemical properties remains a largely elusive goal in sensory science, especially for complex food and beverages 11 , 12 . A key obstacle is the immense number of flavor-active chemicals underlying food flavor. Flavor compounds can vary widely in chemical structure and concentration, making them technically challenging and labor-intensive to quantify, even in the face of innovations in metabolomics, such as non-targeted metabolic fingerprinting 13 , 14 . Moreover, sensory analysis is perhaps even more complicated. Flavor perception is highly complex, resulting from hundreds of different molecules interacting at the physiochemical and sensorial level. Sensory perception is often non-linear, characterized by complex and concentration-dependent synergistic and antagonistic effects 15 , 16 , 17 , 18 , 19 , 20 , 21 that are further convoluted by the genetics, environment, culture and psychology of consumers 22 , 23 , 24 . Perceived flavor is therefore difficult to measure, with problems of sensitivity, accuracy, and reproducibility that can only be resolved by gathering sufficiently large datasets 25 . Trained tasting panels are considered the prime source of quality sensory data, but require meticulous training, are low throughput and high cost. Public databases containing consumer reviews of food products could provide a valuable alternative, especially for studying appreciation scores, which do not require formal training 25 . Public databases offer the advantage of amassing large amounts of data, increasing the statistical power to identify potential drivers of appreciation. However, public datasets suffer from biases, including a bias in the volunteers that contribute to the database, as well as confounding factors such as price, cult status and psychological conformity towards previous ratings of the product.
Classical multivariate statistics and machine learning methods have been used to predict flavor of specific compounds by, for example, linking structural properties of a compound to its potential biological activities or linking concentrations of specific compounds to sensory profiles 1 , 26 . Importantly, most previous studies focused on predicting organoleptic properties of single compounds (often based on their chemical structure) 27 , 28 , 29 , 30 , 31 , 32 , 33 , thus ignoring the fact that these compounds are present in a complex matrix in food or beverages and excluding complex interactions between compounds. Moreover, the classical statistics commonly used in sensory science 34 , 35 , 36 , 37 , 38 , 39 require a large sample size and sufficient variance amongst predictors to create accurate models. They are not fit for studying an extensive set of hundreds of interacting flavor compounds, since they are sensitive to outliers, have a high tendency to overfit and are less suited for non-linear and discontinuous relationships 40 .
In this study, we combine extensive chemical analyses and sensory data of a set of different commercial beers with machine learning approaches to develop models that predict taste, smell, mouthfeel and appreciation from compound concentrations. Beer is particularly suited to model the relationship between chemistry, flavor and appreciation. First, beer is a complex product, consisting of thousands of flavor compounds that partake in complex sensory interactions 41 , 42 , 43 . This chemical diversity arises from the raw materials (malt, yeast, hops, water and spices) and biochemical conversions during the brewing process (kilning, mashing, boiling, fermentation, maturation and aging) 44 , 45 . Second, the advent of the internet saw beer consumers embrace online review platforms, such as RateBeer (ZX Ventures, Anheuser-Busch InBev SA/NV) and BeerAdvocate (Next Glass, inc.). In this way, the beer community provides massive data sets of beer flavor and appreciation scores, creating extraordinarily large sensory databases to complement the analyses of our professional sensory panel. Specifically, we characterize over 200 chemical properties of 250 commercial beers, spread across 22 beer styles, and link these to the descriptive sensory profiling data of a 16-person in-house trained tasting panel and data acquired from over 180,000 public consumer reviews. These unique and extensive datasets enable us to train a suite of machine learning models to predict flavor and appreciation from a beer’s chemical profile. Dissection of the best-performing models allows us to pinpoint specific compounds as potential drivers of beer flavor and appreciation. Follow-up experiments confirm the importance of these compounds and ultimately allow us to significantly improve the flavor and appreciation of selected commercial beers. Together, our study represents a significant step towards understanding complex flavors and reinforces the value of machine learning to develop and refine complex foods. In this way, it represents a stepping stone for further computer-aided food engineering applications 46 .
To generate a comprehensive dataset on beer flavor, we selected 250 commercial Belgian beers across 22 different beer styles (Supplementary Fig. S1 ). Beers with ≤ 4.2% alcohol by volume (ABV) were classified as non-alcoholic and low-alcoholic. Blonds and Tripels constitute a significant portion of the dataset (12.4% and 11.2%, respectively) reflecting their presence on the Belgian beer market and the heterogeneity of beers within these styles. By contrast, lager beers are less diverse and dominated by a handful of brands. Rare styles such as Brut or Faro make up only a small fraction of the dataset (2% and 1%, respectively) because fewer of these beers are produced and because they are dominated by distinct characteristics in terms of flavor and chemical composition.
Extensive analysis identifies relationships between chemical compounds in beer
For each beer, we measured 226 different chemical properties, including common brewing parameters such as alcohol content, iso-alpha acids, pH, sugar concentration 47 , and over 200 flavor compounds (Methods, Supplementary Table S1 ). A large portion (37.2%) are terpenoids arising from hopping, responsible for herbal and fruity flavors 16 , 48 . A second major category are yeast metabolites, such as esters and alcohols, that result in fruity and solvent notes 48 , 49 , 50 . Other measured compounds are primarily derived from malt, or other microbes such as non- Saccharomyces yeasts and bacteria (‘wild flora’). Compounds that arise from spices or staling are labeled under ‘Others’. Five attributes (caloric value, total acids and total ester, hop aroma and sulfur compounds) are calculated from multiple individually measured compounds.
As a first step in identifying relationships between chemical properties, we determined correlations between the concentrations of the compounds (Fig. 1 , upper panel, Supplementary Data 1 and 2 , and Supplementary Fig. S2 . For the sake of clarity, only a subset of the measured compounds is shown in Fig. 1 ). Compounds of the same origin typically show a positive correlation, while absence of correlation hints at parameters varying independently. For example, the hop aroma compounds citronellol, and alpha-terpineol show moderate correlations with each other (Spearman’s rho=0.39 and 0.57), but not with the bittering hop component iso-alpha acids (Spearman’s rho=0.16 and −0.07). This illustrates how brewers can independently modify hop aroma and bitterness by selecting hop varieties and dosage time. If hops are added early in the boiling phase, chemical conversions increase bitterness while aromas evaporate, conversely, late addition of hops preserves aroma but limits bitterness 51 . Similarly, hop-derived iso-alpha acids show a strong anti-correlation with lactic acid and acetic acid, likely reflecting growth inhibition of lactic acid and acetic acid bacteria, or the consequent use of fewer hops in sour beer styles, such as West Flanders ales and Fruit beers, that rely on these bacteria for their distinct flavors 52 . Finally, yeast-derived esters (ethyl acetate, ethyl decanoate, ethyl hexanoate, ethyl octanoate) and alcohols (ethanol, isoamyl alcohol, isobutanol, and glycerol), correlate with Spearman coefficients above 0.5, suggesting that these secondary metabolites are correlated with the yeast genetic background and/or fermentation parameters and may be difficult to influence individually, although the choice of yeast strain may offer some control 53 .
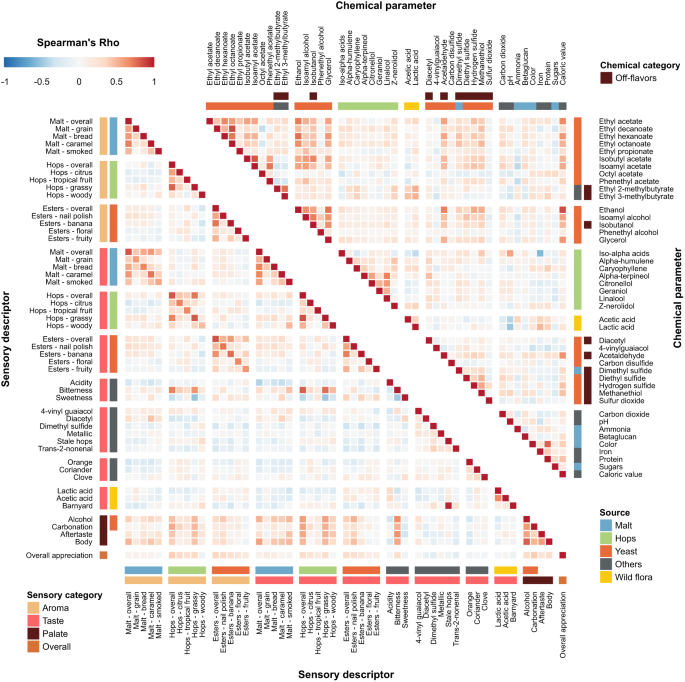
Spearman rank correlations are shown. Descriptors are grouped according to their origin (malt (blue), hops (green), yeast (red), wild flora (yellow), Others (black)), and sensory aspect (aroma, taste, palate, and overall appreciation). Please note that for the chemical compounds, for the sake of clarity, only a subset of the total number of measured compounds is shown, with an emphasis on the key compounds for each source. For more details, see the main text and Methods section. Chemical data can be found in Supplementary Data 1 , correlations between all chemical compounds are depicted in Supplementary Fig. S2 and correlation values can be found in Supplementary Data 2 . See Supplementary Data 4 for sensory panel assessments and Supplementary Data 5 for correlation values between all sensory descriptors.
Interestingly, different beer styles show distinct patterns for some flavor compounds (Supplementary Fig. S3 ). These observations agree with expectations for key beer styles, and serve as a control for our measurements. For instance, Stouts generally show high values for color (darker), while hoppy beers contain elevated levels of iso-alpha acids, compounds associated with bitter hop taste. Acetic and lactic acid are not prevalent in most beers, with notable exceptions such as Kriek, Lambic, Faro, West Flanders ales and Flanders Old Brown, which use acid-producing bacteria ( Lactobacillus and Pediococcus ) or unconventional yeast ( Brettanomyces ) 54 , 55 . Glycerol, ethanol and esters show similar distributions across all beer styles, reflecting their common origin as products of yeast metabolism during fermentation 45 , 53 . Finally, low/no-alcohol beers contain low concentrations of glycerol and esters. This is in line with the production process for most of the low/no-alcohol beers in our dataset, which are produced through limiting fermentation or by stripping away alcohol via evaporation or dialysis, with both methods having the unintended side-effect of reducing the amount of flavor compounds in the final beer 56 , 57 .
Besides expected associations, our data also reveals less trivial associations between beer styles and specific parameters. For example, geraniol and citronellol, two monoterpenoids responsible for citrus, floral and rose flavors and characteristic of Citra hops, are found in relatively high amounts in Christmas, Saison, and Brett/co-fermented beers, where they may originate from terpenoid-rich spices such as coriander seeds instead of hops 58 .
Tasting panel assessments reveal sensorial relationships in beer
To assess the sensory profile of each beer, a trained tasting panel evaluated each of the 250 beers for 50 sensory attributes, including different hop, malt and yeast flavors, off-flavors and spices. Panelists used a tasting sheet (Supplementary Data 3 ) to score the different attributes. Panel consistency was evaluated by repeating 12 samples across different sessions and performing ANOVA. In 95% of cases no significant difference was found across sessions ( p > 0.05), indicating good panel consistency (Supplementary Table S2 ).
Aroma and taste perception reported by the trained panel are often linked (Fig. 1 , bottom left panel and Supplementary Data 4 and 5 ), with high correlations between hops aroma and taste (Spearman’s rho=0.83). Bitter taste was found to correlate with hop aroma and taste in general (Spearman’s rho=0.80 and 0.69), and particularly with “grassy” noble hops (Spearman’s rho=0.75). Barnyard flavor, most often associated with sour beers, is identified together with stale hops (Spearman’s rho=0.97) that are used in these beers. Lactic and acetic acid, which often co-occur, are correlated (Spearman’s rho=0.66). Interestingly, sweetness and bitterness are anti-correlated (Spearman’s rho = −0.48), confirming the hypothesis that they mask each other 59 , 60 . Beer body is highly correlated with alcohol (Spearman’s rho = 0.79), and overall appreciation is found to correlate with multiple aspects that describe beer mouthfeel (alcohol, carbonation; Spearman’s rho= 0.32, 0.39), as well as with hop and ester aroma intensity (Spearman’s rho=0.39 and 0.35).
Similar to the chemical analyses, sensorial analyses confirmed typical features of specific beer styles (Supplementary Fig. S4 ). For example, sour beers (Faro, Flanders Old Brown, Fruit beer, Kriek, Lambic, West Flanders ale) were rated acidic, with flavors of both acetic and lactic acid. Hoppy beers were found to be bitter and showed hop-associated aromas like citrus and tropical fruit. Malt taste is most detected among scotch, stout/porters, and strong ales, while low/no-alcohol beers, which often have a reputation for being ‘worty’ (reminiscent of unfermented, sweet malt extract) appear in the middle. Unsurprisingly, hop aromas are most strongly detected among hoppy beers. Like its chemical counterpart (Supplementary Fig. S3 ), acidity shows a right-skewed distribution, with the most acidic beers being Krieks, Lambics, and West Flanders ales.
Tasting panel assessments of specific flavors correlate with chemical composition
We find that the concentrations of several chemical compounds strongly correlate with specific aroma or taste, as evaluated by the tasting panel (Fig. 2 , Supplementary Fig. S5 , Supplementary Data 6 ). In some cases, these correlations confirm expectations and serve as a useful control for data quality. For example, iso-alpha acids, the bittering compounds in hops, strongly correlate with bitterness (Spearman’s rho=0.68), while ethanol and glycerol correlate with tasters’ perceptions of alcohol and body, the mouthfeel sensation of fullness (Spearman’s rho=0.82/0.62 and 0.72/0.57 respectively) and darker color from roasted malts is a good indication of malt perception (Spearman’s rho=0.54).
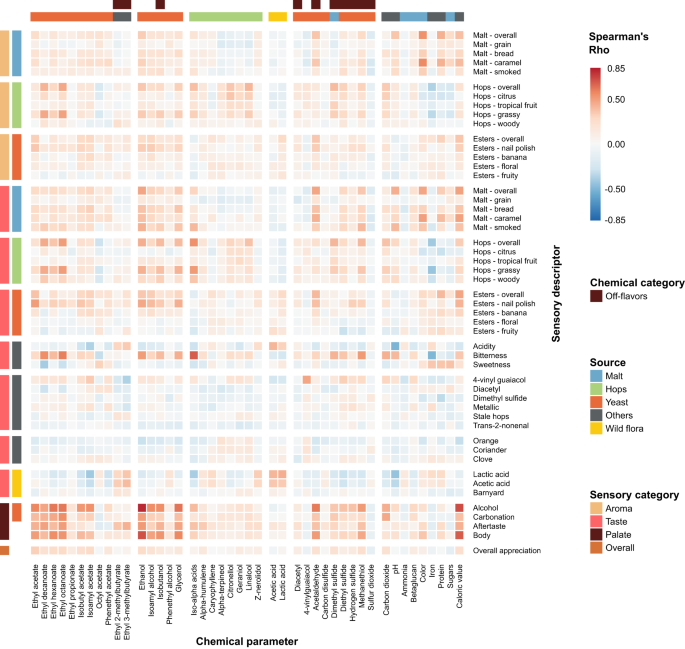
Heatmap colors indicate Spearman’s Rho. Axes are organized according to sensory categories (aroma, taste, mouthfeel, overall), chemical categories and chemical sources in beer (malt (blue), hops (green), yeast (red), wild flora (yellow), Others (black)). See Supplementary Data 6 for all correlation values.
Interestingly, for some relationships between chemical compounds and perceived flavor, correlations are weaker than expected. For example, the rose-smelling phenethyl acetate only weakly correlates with floral aroma. This hints at more complex relationships and interactions between compounds and suggests a need for a more complex model than simple correlations. Lastly, we uncovered unexpected correlations. For instance, the esters ethyl decanoate and ethyl octanoate appear to correlate slightly with hop perception and bitterness, possibly due to their fruity flavor. Iron is anti-correlated with hop aromas and bitterness, most likely because it is also anti-correlated with iso-alpha acids. This could be a sign of metal chelation of hop acids 61 , given that our analyses measure unbound hop acids and total iron content, or could result from the higher iron content in dark and Fruit beers, which typically have less hoppy and bitter flavors 62 .
Public consumer reviews complement expert panel data
To complement and expand the sensory data of our trained tasting panel, we collected 180,000 reviews of our 250 beers from the online consumer review platform RateBeer. This provided numerical scores for beer appearance, aroma, taste, palate, overall quality as well as the average overall score.
Public datasets are known to suffer from biases, such as price, cult status and psychological conformity towards previous ratings of a product. For example, prices correlate with appreciation scores for these online consumer reviews (rho=0.49, Supplementary Fig. S6 ), but not for our trained tasting panel (rho=0.19). This suggests that prices affect consumer appreciation, which has been reported in wine 63 , while blind tastings are unaffected. Moreover, we observe that some beer styles, like lagers and non-alcoholic beers, generally receive lower scores, reflecting that online reviewers are mostly beer aficionados with a preference for specialty beers over lager beers. In general, we find a modest correlation between our trained panel’s overall appreciation score and the online consumer appreciation scores (Fig. 3 , rho=0.29). Apart from the aforementioned biases in the online datasets, serving temperature, sample freshness and surroundings, which are all tightly controlled during the tasting panel sessions, can vary tremendously across online consumers and can further contribute to (among others, appreciation) differences between the two categories of tasters. Importantly, in contrast to the overall appreciation scores, for many sensory aspects the results from the professional panel correlated well with results obtained from RateBeer reviews. Correlations were highest for features that are relatively easy to recognize even for untrained tasters, like bitterness, sweetness, alcohol and malt aroma (Fig. 3 and below).
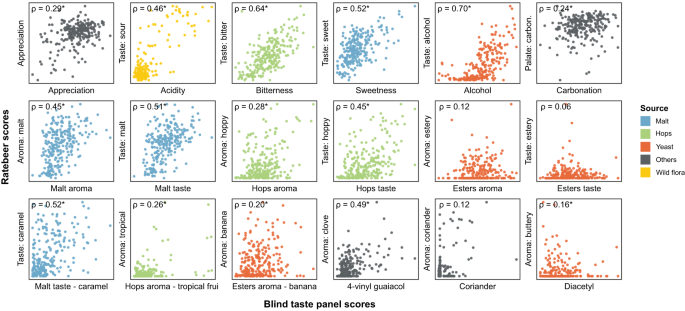
RateBeer text mining results can be found in Supplementary Data 7 . Rho values shown are Spearman correlation values, with asterisks indicating significant correlations ( p < 0.05, two-sided). All p values were smaller than 0.001, except for Esters aroma (0.0553), Esters taste (0.3275), Esters aroma—banana (0.0019), Coriander (0.0508) and Diacetyl (0.0134).
Besides collecting consumer appreciation from these online reviews, we developed automated text analysis tools to gather additional data from review texts (Supplementary Data 7 ). Processing review texts on the RateBeer database yielded comparable results to the scores given by the trained panel for many common sensory aspects, including acidity, bitterness, sweetness, alcohol, malt, and hop tastes (Fig. 3 ). This is in line with what would be expected, since these attributes require less training for accurate assessment and are less influenced by environmental factors such as temperature, serving glass and odors in the environment. Consumer reviews also correlate well with our trained panel for 4-vinyl guaiacol, a compound associated with a very characteristic aroma. By contrast, correlations for more specific aromas like ester, coriander or diacetyl are underrepresented in the online reviews, underscoring the importance of using a trained tasting panel and standardized tasting sheets with explicit factors to be scored for evaluating specific aspects of a beer. Taken together, our results suggest that public reviews are trustworthy for some, but not all, flavor features and can complement or substitute taste panel data for these sensory aspects.
Models can predict beer sensory profiles from chemical data
The rich datasets of chemical analyses, tasting panel assessments and public reviews gathered in the first part of this study provided us with a unique opportunity to develop predictive models that link chemical data to sensorial features. Given the complexity of beer flavor, basic statistical tools such as correlations or linear regression may not always be the most suitable for making accurate predictions. Instead, we applied different machine learning models that can model both simple linear and complex interactive relationships. Specifically, we constructed a set of regression models to predict (a) trained panel scores for beer flavor and quality and (b) public reviews’ appreciation scores from beer chemical profiles. We trained and tested 10 different models (Methods), 3 linear regression-based models (simple linear regression with first-order interactions (LR), lasso regression with first-order interactions (Lasso), partial least squares regressor (PLSR)), 5 decision tree models (AdaBoost regressor (ABR), extra trees (ET), gradient boosting regressor (GBR), random forest (RF) and XGBoost regressor (XGBR)), 1 support vector regression (SVR), and 1 artificial neural network (ANN) model.
To compare the performance of our machine learning models, the dataset was randomly split into a training and test set, stratified by beer style. After a model was trained on data in the training set, its performance was evaluated on its ability to predict the test dataset obtained from multi-output models (based on the coefficient of determination, see Methods). Additionally, individual-attribute models were ranked per descriptor and the average rank was calculated, as proposed by Korneva et al. 64 . Importantly, both ways of evaluating the models’ performance agreed in general. Performance of the different models varied (Table 1 ). It should be noted that all models perform better at predicting RateBeer results than results from our trained tasting panel. One reason could be that sensory data is inherently variable, and this variability is averaged out with the large number of public reviews from RateBeer. Additionally, all tree-based models perform better at predicting taste than aroma. Linear models (LR) performed particularly poorly, with negative R 2 values, due to severe overfitting (training set R 2 = 1). Overfitting is a common issue in linear models with many parameters and limited samples, especially with interaction terms further amplifying the number of parameters. L1 regularization (Lasso) successfully overcomes this overfitting, out-competing multiple tree-based models on the RateBeer dataset. Similarly, the dimensionality reduction of PLSR avoids overfitting and improves performance, to some extent. Still, tree-based models (ABR, ET, GBR, RF and XGBR) show the best performance, out-competing the linear models (LR, Lasso, PLSR) commonly used in sensory science 65 .
GBR models showed the best overall performance in predicting sensory responses from chemical information, with R 2 values up to 0.75 depending on the predicted sensory feature (Supplementary Table S4 ). The GBR models predict consumer appreciation (RateBeer) better than our trained panel’s appreciation (R 2 value of 0.67 compared to R 2 value of 0.09) (Supplementary Table S3 and Supplementary Table S4 ). ANN models showed intermediate performance, likely because neural networks typically perform best with larger datasets 66 . The SVR shows intermediate performance, mostly due to the weak predictions of specific attributes that lower the overall performance (Supplementary Table S4 ).
Model dissection identifies specific, unexpected compounds as drivers of consumer appreciation
Next, we leveraged our models to infer important contributors to sensory perception and consumer appreciation. Consumer preference is a crucial sensory aspects, because a product that shows low consumer appreciation scores often does not succeed commercially 25 . Additionally, the requirement for a large number of representative evaluators makes consumer trials one of the more costly and time-consuming aspects of product development. Hence, a model for predicting chemical drivers of overall appreciation would be a welcome addition to the available toolbox for food development and optimization.
Since GBR models on our RateBeer dataset showed the best overall performance, we focused on these models. Specifically, we used two approaches to identify important contributors. First, rankings of the most important predictors for each sensorial trait in the GBR models were obtained based on impurity-based feature importance (mean decrease in impurity). High-ranked parameters were hypothesized to be either the true causal chemical properties underlying the trait, to correlate with the actual causal properties, or to take part in sensory interactions affecting the trait 67 (Fig. 4A ). In a second approach, we used SHAP 68 to determine which parameters contributed most to the model for making predictions of consumer appreciation (Fig. 4B ). SHAP calculates parameter contributions to model predictions on a per-sample basis, which can be aggregated into an importance score.
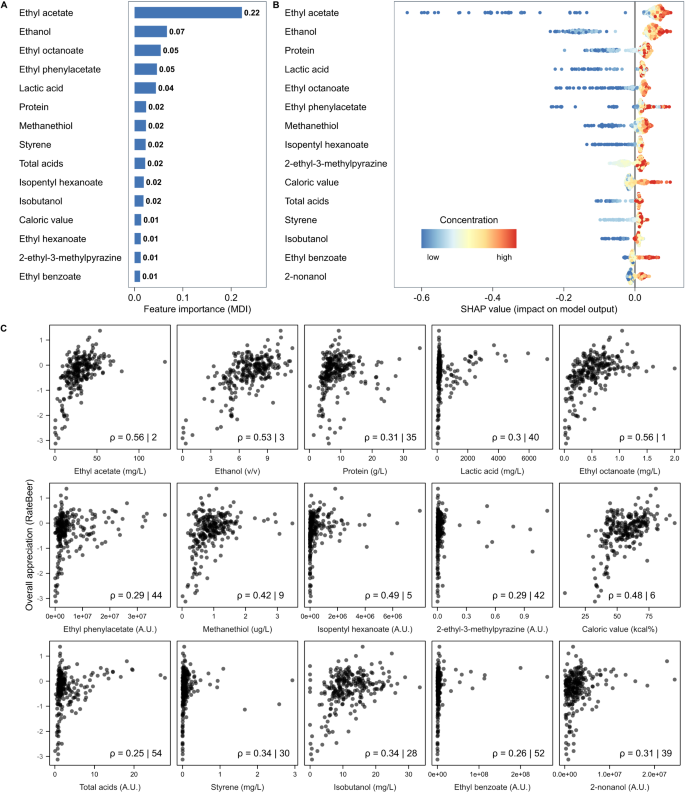
A The impurity-based feature importance (mean deviance in impurity, MDI) calculated from the Gradient Boosting Regression (GBR) model predicting RateBeer appreciation scores. The top 15 highest ranked chemical properties are shown. B SHAP summary plot for the top 15 parameters contributing to our GBR model. Each point on the graph represents a sample from our dataset. The color represents the concentration of that parameter, with bluer colors representing low values and redder colors representing higher values. Greater absolute values on the horizontal axis indicate a higher impact of the parameter on the prediction of the model. C Spearman correlations between the 15 most important chemical properties and consumer overall appreciation. Numbers indicate the Spearman Rho correlation coefficient, and the rank of this correlation compared to all other correlations. The top 15 important compounds were determined using SHAP (panel B).
Both approaches identified ethyl acetate as the most predictive parameter for beer appreciation (Fig. 4 ). Ethyl acetate is the most abundant ester in beer with a typical ‘fruity’, ‘solvent’ and ‘alcoholic’ flavor, but is often considered less important than other esters like isoamyl acetate. The second most important parameter identified by SHAP is ethanol, the most abundant beer compound after water. Apart from directly contributing to beer flavor and mouthfeel, ethanol drastically influences the physical properties of beer, dictating how easily volatile compounds escape the beer matrix to contribute to beer aroma 69 . Importantly, it should also be noted that the importance of ethanol for appreciation is likely inflated by the very low appreciation scores of non-alcoholic beers (Supplementary Fig. S4 ). Despite not often being considered a driver of beer appreciation, protein level also ranks highly in both approaches, possibly due to its effect on mouthfeel and body 70 . Lactic acid, which contributes to the tart taste of sour beers, is the fourth most important parameter identified by SHAP, possibly due to the generally high appreciation of sour beers in our dataset.
Interestingly, some of the most important predictive parameters for our model are not well-established as beer flavors or are even commonly regarded as being negative for beer quality. For example, our models identify methanethiol and ethyl phenyl acetate, an ester commonly linked to beer staling 71 , as a key factor contributing to beer appreciation. Although there is no doubt that high concentrations of these compounds are considered unpleasant, the positive effects of modest concentrations are not yet known 72 , 73 .
To compare our approach to conventional statistics, we evaluated how well the 15 most important SHAP-derived parameters correlate with consumer appreciation (Fig. 4C ). Interestingly, only 6 of the properties derived by SHAP rank amongst the top 15 most correlated parameters. For some chemical compounds, the correlations are so low that they would have likely been considered unimportant. For example, lactic acid, the fourth most important parameter, shows a bimodal distribution for appreciation, with sour beers forming a separate cluster, that is missed entirely by the Spearman correlation. Additionally, the correlation plots reveal outliers, emphasizing the need for robust analysis tools. Together, this highlights the need for alternative models, like the Gradient Boosting model, that better grasp the complexity of (beer) flavor.
Finally, to observe the relationships between these chemical properties and their predicted targets, partial dependence plots were constructed for the six most important predictors of consumer appreciation 74 , 75 , 76 (Supplementary Fig. S7 ). One-way partial dependence plots show how a change in concentration affects the predicted appreciation. These plots reveal an important limitation of our models: appreciation predictions remain constant at ever-increasing concentrations. This implies that once a threshold concentration is reached, further increasing the concentration does not affect appreciation. This is false, as it is well-documented that certain compounds become unpleasant at high concentrations, including ethyl acetate (‘nail polish’) 77 and methanethiol (‘sulfury’ and ‘rotten cabbage’) 78 . The inability of our models to grasp that flavor compounds have optimal levels, above which they become negative, is a consequence of working with commercial beer brands where (off-)flavors are rarely too high to negatively impact the product. The two-way partial dependence plots show how changing the concentration of two compounds influences predicted appreciation, visualizing their interactions (Supplementary Fig. S7 ). In our case, the top 5 parameters are dominated by additive or synergistic interactions, with high concentrations for both compounds resulting in the highest predicted appreciation.
To assess the robustness of our best-performing models and model predictions, we performed 100 iterations of the GBR, RF and ET models. In general, all iterations of the models yielded similar performance (Supplementary Fig. S8 ). Moreover, the main predictors (including the top predictors ethanol and ethyl acetate) remained virtually the same, especially for GBR and RF. For the iterations of the ET model, we did observe more variation in the top predictors, which is likely a consequence of the model’s inherent random architecture in combination with co-correlations between certain predictors. However, even in this case, several of the top predictors (ethanol and ethyl acetate) remain unchanged, although their rank in importance changes (Supplementary Fig. S8 ).
Next, we investigated if a combination of RateBeer and trained panel data into one consolidated dataset would lead to stronger models, under the hypothesis that such a model would suffer less from bias in the datasets. A GBR model was trained to predict appreciation on the combined dataset. This model underperformed compared to the RateBeer model, both in the native case and when including a dataset identifier (R 2 = 0.67, 0.26 and 0.42 respectively). For the latter, the dataset identifier is the most important feature (Supplementary Fig. S9 ), while most of the feature importance remains unchanged, with ethyl acetate and ethanol ranking highest, like in the original model trained only on RateBeer data. It seems that the large variation in the panel dataset introduces noise, weakening the models’ performances and reliability. In addition, it seems reasonable to assume that both datasets are fundamentally different, with the panel dataset obtained by blind tastings by a trained professional panel.
Lastly, we evaluated whether beer style identifiers would further enhance the model’s performance. A GBR model was trained with parameters that explicitly encoded the styles of the samples. This did not improve model performance (R2 = 0.66 with style information vs R2 = 0.67). The most important chemical features are consistent with the model trained without style information (eg. ethanol and ethyl acetate), and with the exception of the most preferred (strong ale) and least preferred (low/no-alcohol) styles, none of the styles were among the most important features (Supplementary Fig. S9 , Supplementary Table S5 and S6 ). This is likely due to a combination of style-specific chemical signatures, such as iso-alpha acids and lactic acid, that implicitly convey style information to the original models, as well as the low number of samples belonging to some styles, making it difficult for the model to learn style-specific patterns. Moreover, beer styles are not rigorously defined, with some styles overlapping in features and some beers being misattributed to a specific style, all of which leads to more noise in models that use style parameters.
Model validation
To test if our predictive models give insight into beer appreciation, we set up experiments aimed at improving existing commercial beers. We specifically selected overall appreciation as the trait to be examined because of its complexity and commercial relevance. Beer flavor comprises a complex bouquet rather than single aromas and tastes 53 . Hence, adding a single compound to the extent that a difference is noticeable may lead to an unbalanced, artificial flavor. Therefore, we evaluated the effect of combinations of compounds. Because Blond beers represent the most extensive style in our dataset, we selected a beer from this style as the starting material for these experiments (Beer 64 in Supplementary Data 1 ).
In the first set of experiments, we adjusted the concentrations of compounds that made up the most important predictors of overall appreciation (ethyl acetate, ethanol, lactic acid, ethyl phenyl acetate) together with correlated compounds (ethyl hexanoate, isoamyl acetate, glycerol), bringing them up to 95 th percentile ethanol-normalized concentrations (Methods) within the Blond group (‘Spiked’ concentration in Fig. 5A ). Compared to controls, the spiked beers were found to have significantly improved overall appreciation among trained panelists, with panelist noting increased intensity of ester flavors, sweetness, alcohol, and body fullness (Fig. 5B ). To disentangle the contribution of ethanol to these results, a second experiment was performed without the addition of ethanol. This resulted in a similar outcome, including increased perception of alcohol and overall appreciation.
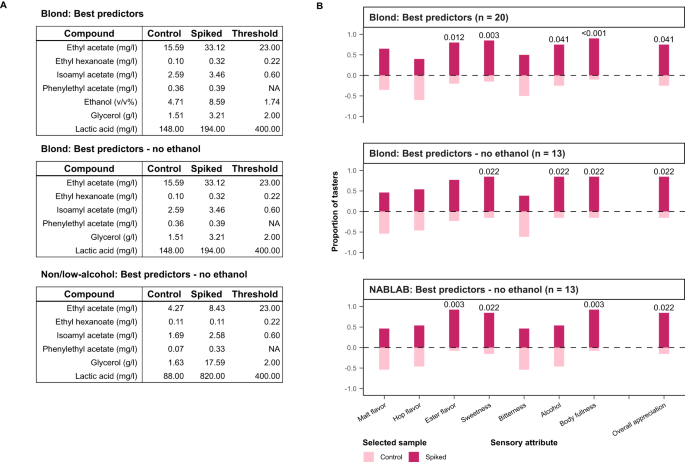
Adding the top chemical compounds, identified as best predictors of appreciation by our model, into poorly appreciated beers results in increased appreciation from our trained panel. Results of sensory tests between base beers and those spiked with compounds identified as the best predictors by the model. A Blond and Non/Low-alcohol (0.0% ABV) base beers were brought up to 95th-percentile ethanol-normalized concentrations within each style. B For each sensory attribute, tasters indicated the more intense sample and selected the sample they preferred. The numbers above the bars correspond to the p values that indicate significant changes in perceived flavor (two-sided binomial test: alpha 0.05, n = 20 or 13).
In a last experiment, we tested whether using the model’s predictions can boost the appreciation of a non-alcoholic beer (beer 223 in Supplementary Data 1 ). Again, the addition of a mixture of predicted compounds (omitting ethanol, in this case) resulted in a significant increase in appreciation, body, ester flavor and sweetness.
Predicting flavor and consumer appreciation from chemical composition is one of the ultimate goals of sensory science. A reliable, systematic and unbiased way to link chemical profiles to flavor and food appreciation would be a significant asset to the food and beverage industry. Such tools would substantially aid in quality control and recipe development, offer an efficient and cost-effective alternative to pilot studies and consumer trials and would ultimately allow food manufacturers to produce superior, tailor-made products that better meet the demands of specific consumer groups more efficiently.
A limited set of studies have previously tried, to varying degrees of success, to predict beer flavor and beer popularity based on (a limited set of) chemical compounds and flavors 79 , 80 . Current sensitive, high-throughput technologies allow measuring an unprecedented number of chemical compounds and properties in a large set of samples, yielding a dataset that can train models that help close the gaps between chemistry and flavor, even for a complex natural product like beer. To our knowledge, no previous research gathered data at this scale (250 samples, 226 chemical parameters, 50 sensory attributes and 5 consumer scores) to disentangle and validate the chemical aspects driving beer preference using various machine-learning techniques. We find that modern machine learning models outperform conventional statistical tools, such as correlations and linear models, and can successfully predict flavor appreciation from chemical composition. This could be attributed to the natural incorporation of interactions and non-linear or discontinuous effects in machine learning models, which are not easily grasped by the linear model architecture. While linear models and partial least squares regression represent the most widespread statistical approaches in sensory science, in part because they allow interpretation 65 , 81 , 82 , modern machine learning methods allow for building better predictive models while preserving the possibility to dissect and exploit the underlying patterns. Of the 10 different models we trained, tree-based models, such as our best performing GBR, showed the best overall performance in predicting sensory responses from chemical information, outcompeting artificial neural networks. This agrees with previous reports for models trained on tabular data 83 . Our results are in line with the findings of Colantonio et al. who also identified the gradient boosting architecture as performing best at predicting appreciation and flavor (of tomatoes and blueberries, in their specific study) 26 . Importantly, besides our larger experimental scale, we were able to directly confirm our models’ predictions in vivo.
Our study confirms that flavor compound concentration does not always correlate with perception, suggesting complex interactions that are often missed by more conventional statistics and simple models. Specifically, we find that tree-based algorithms may perform best in developing models that link complex food chemistry with aroma. Furthermore, we show that massive datasets of untrained consumer reviews provide a valuable source of data, that can complement or even replace trained tasting panels, especially for appreciation and basic flavors, such as sweetness and bitterness. This holds despite biases that are known to occur in such datasets, such as price or conformity bias. Moreover, GBR models predict taste better than aroma. This is likely because taste (e.g. bitterness) often directly relates to the corresponding chemical measurements (e.g., iso-alpha acids), whereas such a link is less clear for aromas, which often result from the interplay between multiple volatile compounds. We also find that our models are best at predicting acidity and alcohol, likely because there is a direct relation between the measured chemical compounds (acids and ethanol) and the corresponding perceived sensorial attribute (acidity and alcohol), and because even untrained consumers are generally able to recognize these flavors and aromas.
The predictions of our final models, trained on review data, hold even for blind tastings with small groups of trained tasters, as demonstrated by our ability to validate specific compounds as drivers of beer flavor and appreciation. Since adding a single compound to the extent of a noticeable difference may result in an unbalanced flavor profile, we specifically tested our identified key drivers as a combination of compounds. While this approach does not allow us to validate if a particular single compound would affect flavor and/or appreciation, our experiments do show that this combination of compounds increases consumer appreciation.
It is important to stress that, while it represents an important step forward, our approach still has several major limitations. A key weakness of the GBR model architecture is that amongst co-correlating variables, the largest main effect is consistently preferred for model building. As a result, co-correlating variables often have artificially low importance scores, both for impurity and SHAP-based methods, like we observed in the comparison to the more randomized Extra Trees models. This implies that chemicals identified as key drivers of a specific sensory feature by GBR might not be the true causative compounds, but rather co-correlate with the actual causative chemical. For example, the high importance of ethyl acetate could be (partially) attributed to the total ester content, ethanol or ethyl hexanoate (rho=0.77, rho=0.72 and rho=0.68), while ethyl phenylacetate could hide the importance of prenyl isobutyrate and ethyl benzoate (rho=0.77 and rho=0.76). Expanding our GBR model to include beer style as a parameter did not yield additional power or insight. This is likely due to style-specific chemical signatures, such as iso-alpha acids and lactic acid, that implicitly convey style information to the original model, as well as the smaller sample size per style, limiting the power to uncover style-specific patterns. This can be partly attributed to the curse of dimensionality, where the high number of parameters results in the models mainly incorporating single parameter effects, rather than complex interactions such as style-dependent effects 67 . A larger number of samples may overcome some of these limitations and offer more insight into style-specific effects. On the other hand, beer style is not a rigid scientific classification, and beers within one style often differ a lot, which further complicates the analysis of style as a model factor.
Our study is limited to beers from Belgian breweries. Although these beers cover a large portion of the beer styles available globally, some beer styles and consumer patterns may be missing, while other features might be overrepresented. For example, many Belgian ales exhibit yeast-driven flavor profiles, which is reflected in the chemical drivers of appreciation discovered by this study. In future work, expanding the scope to include diverse markets and beer styles could lead to the identification of even more drivers of appreciation and better models for special niche products that were not present in our beer set.
In addition to inherent limitations of GBR models, there are also some limitations associated with studying food aroma. Even if our chemical analyses measured most of the known aroma compounds, the total number of flavor compounds in complex foods like beer is still larger than the subset we were able to measure in this study. For example, hop-derived thiols, that influence flavor at very low concentrations, are notoriously difficult to measure in a high-throughput experiment. Moreover, consumer perception remains subjective and prone to biases that are difficult to avoid. It is also important to stress that the models are still immature and that more extensive datasets will be crucial for developing more complete models in the future. Besides more samples and parameters, our dataset does not include any demographic information about the tasters. Including such data could lead to better models that grasp external factors like age and culture. Another limitation is that our set of beers consists of high-quality end-products and lacks beers that are unfit for sale, which limits the current model in accurately predicting products that are appreciated very badly. Finally, while models could be readily applied in quality control, their use in sensory science and product development is restrained by their inability to discern causal relationships. Given that the models cannot distinguish compounds that genuinely drive consumer perception from those that merely correlate, validation experiments are essential to identify true causative compounds.
Despite the inherent limitations, dissection of our models enabled us to pinpoint specific molecules as potential drivers of beer aroma and consumer appreciation, including compounds that were unexpected and would not have been identified using standard approaches. Important drivers of beer appreciation uncovered by our models include protein levels, ethyl acetate, ethyl phenyl acetate and lactic acid. Currently, many brewers already use lactic acid to acidify their brewing water and ensure optimal pH for enzymatic activity during the mashing process. Our results suggest that adding lactic acid can also improve beer appreciation, although its individual effect remains to be tested. Interestingly, ethanol appears to be unnecessary to improve beer appreciation, both for blond beer and alcohol-free beer. Given the growing consumer interest in alcohol-free beer, with a predicted annual market growth of >7% 84 , it is relevant for brewers to know what compounds can further increase consumer appreciation of these beers. Hence, our model may readily provide avenues to further improve the flavor and consumer appreciation of both alcoholic and non-alcoholic beers, which is generally considered one of the key challenges for future beer production.
Whereas we see a direct implementation of our results for the development of superior alcohol-free beverages and other food products, our study can also serve as a stepping stone for the development of novel alcohol-containing beverages. We want to echo the growing body of scientific evidence for the negative effects of alcohol consumption, both on the individual level by the mutagenic, teratogenic and carcinogenic effects of ethanol 85 , 86 , as well as the burden on society caused by alcohol abuse and addiction. We encourage the use of our results for the production of healthier, tastier products, including novel and improved beverages with lower alcohol contents. Furthermore, we strongly discourage the use of these technologies to improve the appreciation or addictive properties of harmful substances.
The present work demonstrates that despite some important remaining hurdles, combining the latest developments in chemical analyses, sensory analysis and modern machine learning methods offers exciting avenues for food chemistry and engineering. Soon, these tools may provide solutions in quality control and recipe development, as well as new approaches to sensory science and flavor research.
Beer selection
250 commercial Belgian beers were selected to cover the broad diversity of beer styles and corresponding diversity in chemical composition and aroma. See Supplementary Fig. S1 .
Chemical dataset
Sample preparation.
Beers within their expiration date were purchased from commercial retailers. Samples were prepared in biological duplicates at room temperature, unless explicitly stated otherwise. Bottle pressure was measured with a manual pressure device (Steinfurth Mess-Systeme GmbH) and used to calculate CO 2 concentration. The beer was poured through two filter papers (Macherey-Nagel, 500713032 MN 713 ¼) to remove carbon dioxide and prevent spontaneous foaming. Samples were then prepared for measurements by targeted Headspace-Gas Chromatography-Flame Ionization Detector/Flame Photometric Detector (HS-GC-FID/FPD), Headspace-Solid Phase Microextraction-Gas Chromatography-Mass Spectrometry (HS-SPME-GC-MS), colorimetric analysis, enzymatic analysis, Near-Infrared (NIR) analysis, as described in the sections below. The mean values of biological duplicates are reported for each compound.
HS-GC-FID/FPD
HS-GC-FID/FPD (Shimadzu GC 2010 Plus) was used to measure higher alcohols, acetaldehyde, esters, 4-vinyl guaicol, and sulfur compounds. Each measurement comprised 5 ml of sample pipetted into a 20 ml glass vial containing 1.75 g NaCl (VWR, 27810.295). 100 µl of 2-heptanol (Sigma-Aldrich, H3003) (internal standard) solution in ethanol (Fisher Chemical, E/0650DF/C17) was added for a final concentration of 2.44 mg/L. Samples were flushed with nitrogen for 10 s, sealed with a silicone septum, stored at −80 °C and analyzed in batches of 20.
The GC was equipped with a DB-WAXetr column (length, 30 m; internal diameter, 0.32 mm; layer thickness, 0.50 µm; Agilent Technologies, Santa Clara, CA, USA) to the FID and an HP-5 column (length, 30 m; internal diameter, 0.25 mm; layer thickness, 0.25 µm; Agilent Technologies, Santa Clara, CA, USA) to the FPD. N 2 was used as the carrier gas. Samples were incubated for 20 min at 70 °C in the headspace autosampler (Flow rate, 35 cm/s; Injection volume, 1000 µL; Injection mode, split; Combi PAL autosampler, CTC analytics, Switzerland). The injector, FID and FPD temperatures were kept at 250 °C. The GC oven temperature was first held at 50 °C for 5 min and then allowed to rise to 80 °C at a rate of 5 °C/min, followed by a second ramp of 4 °C/min until 200 °C kept for 3 min and a final ramp of (4 °C/min) until 230 °C for 1 min. Results were analyzed with the GCSolution software version 2.4 (Shimadzu, Kyoto, Japan). The GC was calibrated with a 5% EtOH solution (VWR International) containing the volatiles under study (Supplementary Table S7 ).
HS-SPME-GC-MS
HS-SPME-GC-MS (Shimadzu GCMS-QP-2010 Ultra) was used to measure additional volatile compounds, mainly comprising terpenoids and esters. Samples were analyzed by HS-SPME using a triphase DVB/Carboxen/PDMS 50/30 μm SPME fiber (Supelco Co., Bellefonte, PA, USA) followed by gas chromatography (Thermo Fisher Scientific Trace 1300 series, USA) coupled to a mass spectrometer (Thermo Fisher Scientific ISQ series MS) equipped with a TriPlus RSH autosampler. 5 ml of degassed beer sample was placed in 20 ml vials containing 1.75 g NaCl (VWR, 27810.295). 5 µl internal standard mix was added, containing 2-heptanol (1 g/L) (Sigma-Aldrich, H3003), 4-fluorobenzaldehyde (1 g/L) (Sigma-Aldrich, 128376), 2,3-hexanedione (1 g/L) (Sigma-Aldrich, 144169) and guaiacol (1 g/L) (Sigma-Aldrich, W253200) in ethanol (Fisher Chemical, E/0650DF/C17). Each sample was incubated at 60 °C in the autosampler oven with constant agitation. After 5 min equilibration, the SPME fiber was exposed to the sample headspace for 30 min. The compounds trapped on the fiber were thermally desorbed in the injection port of the chromatograph by heating the fiber for 15 min at 270 °C.
The GC-MS was equipped with a low polarity RXi-5Sil MS column (length, 20 m; internal diameter, 0.18 mm; layer thickness, 0.18 µm; Restek, Bellefonte, PA, USA). Injection was performed in splitless mode at 320 °C, a split flow of 9 ml/min, a purge flow of 5 ml/min and an open valve time of 3 min. To obtain a pulsed injection, a programmed gas flow was used whereby the helium gas flow was set at 2.7 mL/min for 0.1 min, followed by a decrease in flow of 20 ml/min to the normal 0.9 mL/min. The temperature was first held at 30 °C for 3 min and then allowed to rise to 80 °C at a rate of 7 °C/min, followed by a second ramp of 2 °C/min till 125 °C and a final ramp of 8 °C/min with a final temperature of 270 °C.
Mass acquisition range was 33 to 550 amu at a scan rate of 5 scans/s. Electron impact ionization energy was 70 eV. The interface and ion source were kept at 275 °C and 250 °C, respectively. A mix of linear n-alkanes (from C7 to C40, Supelco Co.) was injected into the GC-MS under identical conditions to serve as external retention index markers. Identification and quantification of the compounds were performed using an in-house developed R script as described in Goelen et al. and Reher et al. 87 , 88 (for package information, see Supplementary Table S8 ). Briefly, chromatograms were analyzed using AMDIS (v2.71) 89 to separate overlapping peaks and obtain pure compound spectra. The NIST MS Search software (v2.0 g) in combination with the NIST2017, FFNSC3 and Adams4 libraries were used to manually identify the empirical spectra, taking into account the expected retention time. After background subtraction and correcting for retention time shifts between samples run on different days based on alkane ladders, compound elution profiles were extracted and integrated using a file with 284 target compounds of interest, which were either recovered in our identified AMDIS list of spectra or were known to occur in beer. Compound elution profiles were estimated for every peak in every chromatogram over a time-restricted window using weighted non-negative least square analysis after which peak areas were integrated 87 , 88 . Batch effect correction was performed by normalizing against the most stable internal standard compound, 4-fluorobenzaldehyde. Out of all 284 target compounds that were analyzed, 167 were visually judged to have reliable elution profiles and were used for final analysis.
Discrete photometric and enzymatic analysis
Discrete photometric and enzymatic analysis (Thermo Scientific TM Gallery TM Plus Beermaster Discrete Analyzer) was used to measure acetic acid, ammonia, beta-glucan, iso-alpha acids, color, sugars, glycerol, iron, pH, protein, and sulfite. 2 ml of sample volume was used for the analyses. Information regarding the reagents and standard solutions used for analyses and calibrations is included in Supplementary Table S7 and Supplementary Table S9 .
NIR analyses
NIR analysis (Anton Paar Alcolyzer Beer ME System) was used to measure ethanol. Measurements comprised 50 ml of sample, and a 10% EtOH solution was used for calibration.
Correlation calculations
Pairwise Spearman Rank correlations were calculated between all chemical properties.
Sensory dataset
Trained panel.
Our trained tasting panel consisted of volunteers who gave prior verbal informed consent. All compounds used for the validation experiment were of food-grade quality. The tasting sessions were approved by the Social and Societal Ethics Committee of the KU Leuven (G-2022-5677-R2(MAR)). All online reviewers agreed to the Terms and Conditions of the RateBeer website.
Sensory analysis was performed according to the American Society of Brewing Chemists (ASBC) Sensory Analysis Methods 90 . 30 volunteers were screened through a series of triangle tests. The sixteen most sensitive and consistent tasters were retained as taste panel members. The resulting panel was diverse in age [22–42, mean: 29], sex [56% male] and nationality [7 different countries]. The panel developed a consensus vocabulary to describe beer aroma, taste and mouthfeel. Panelists were trained to identify and score 50 different attributes, using a 7-point scale to rate attributes’ intensity. The scoring sheet is included as Supplementary Data 3 . Sensory assessments took place between 10–12 a.m. The beers were served in black-colored glasses. Per session, between 5 and 12 beers of the same style were tasted at 12 °C to 16 °C. Two reference beers were added to each set and indicated as ‘Reference 1 & 2’, allowing panel members to calibrate their ratings. Not all panelists were present at every tasting. Scores were scaled by standard deviation and mean-centered per taster. Values are represented as z-scores and clustered by Euclidean distance. Pairwise Spearman correlations were calculated between taste and aroma sensory attributes. Panel consistency was evaluated by repeating samples on different sessions and performing ANOVA to identify differences, using the ‘stats’ package (v4.2.2) in R (for package information, see Supplementary Table S8 ).
Online reviews from a public database
The ‘scrapy’ package in Python (v3.6) (for package information, see Supplementary Table S8 ). was used to collect 232,288 online reviews (mean=922, min=6, max=5343) from RateBeer, an online beer review database. Each review entry comprised 5 numerical scores (appearance, aroma, taste, palate and overall quality) and an optional review text. The total number of reviews per reviewer was collected separately. Numerical scores were scaled and centered per rater, and mean scores were calculated per beer.
For the review texts, the language was estimated using the packages ‘langdetect’ and ‘langid’ in Python. Reviews that were classified as English by both packages were kept. Reviewers with fewer than 100 entries overall were discarded. 181,025 reviews from >6000 reviewers from >40 countries remained. Text processing was done using the ‘nltk’ package in Python. Texts were corrected for slang and misspellings; proper nouns and rare words that are relevant to the beer context were specified and kept as-is (‘Chimay’,’Lambic’, etc.). A dictionary of semantically similar sensorial terms, for example ‘floral’ and ‘flower’, was created and collapsed together into one term. Words were stemmed and lemmatized to avoid identifying words such as ‘acid’ and ‘acidity’ as separate terms. Numbers and punctuation were removed.
Sentences from up to 50 randomly chosen reviews per beer were manually categorized according to the aspect of beer they describe (appearance, aroma, taste, palate, overall quality—not to be confused with the 5 numerical scores described above) or flagged as irrelevant if they contained no useful information. If a beer contained fewer than 50 reviews, all reviews were manually classified. This labeled data set was used to train a model that classified the rest of the sentences for all beers 91 . Sentences describing taste and aroma were extracted, and term frequency–inverse document frequency (TFIDF) was implemented to calculate enrichment scores for sensorial words per beer.
The sex of the tasting subject was not considered when building our sensory database. Instead, results from different panelists were averaged, both for our trained panel (56% male, 44% female) and the RateBeer reviews (70% male, 30% female for RateBeer as a whole).
Beer price collection and processing
Beer prices were collected from the following stores: Colruyt, Delhaize, Total Wine, BeerHawk, The Belgian Beer Shop, The Belgian Shop, and Beer of Belgium. Where applicable, prices were converted to Euros and normalized per liter. Spearman correlations were calculated between these prices and mean overall appreciation scores from RateBeer and the taste panel, respectively.
Pairwise Spearman Rank correlations were calculated between all sensory properties.
Machine learning models
Predictive modeling of sensory profiles from chemical data.
Regression models were constructed to predict (a) trained panel scores for beer flavors and quality from beer chemical profiles and (b) public reviews’ appreciation scores from beer chemical profiles. Z-scores were used to represent sensory attributes in both data sets. Chemical properties with log-normal distributions (Shapiro-Wilk test, p < 0.05 ) were log-transformed. Missing chemical measurements (0.1% of all data) were replaced with mean values per attribute. Observations from 250 beers were randomly separated into a training set (70%, 175 beers) and a test set (30%, 75 beers), stratified per beer style. Chemical measurements (p = 231) were normalized based on the training set average and standard deviation. In total, three linear regression-based models: linear regression with first-order interaction terms (LR), lasso regression with first-order interaction terms (Lasso) and partial least squares regression (PLSR); five decision tree models, Adaboost regressor (ABR), Extra Trees (ET), Gradient Boosting regressor (GBR), Random Forest (RF) and XGBoost regressor (XGBR); one support vector machine model (SVR) and one artificial neural network model (ANN) were trained. The models were implemented using the ‘scikit-learn’ package (v1.2.2) and ‘xgboost’ package (v1.7.3) in Python (v3.9.16). Models were trained, and hyperparameters optimized, using five-fold cross-validated grid search with the coefficient of determination (R 2 ) as the evaluation metric. The ANN (scikit-learn’s MLPRegressor) was optimized using Bayesian Tree-Structured Parzen Estimator optimization with the ‘Optuna’ Python package (v3.2.0). Individual models were trained per attribute, and a multi-output model was trained on all attributes simultaneously.
Model dissection
GBR was found to outperform other methods, resulting in models with the highest average R 2 values in both trained panel and public review data sets. Impurity-based rankings of the most important predictors for each predicted sensorial trait were obtained using the ‘scikit-learn’ package. To observe the relationships between these chemical properties and their predicted targets, partial dependence plots (PDP) were constructed for the six most important predictors of consumer appreciation 74 , 75 .
The ‘SHAP’ package in Python (v0.41.0) was implemented to provide an alternative ranking of predictor importance and to visualize the predictors’ effects as a function of their concentration 68 .
Validation of causal chemical properties
To validate the effects of the most important model features on predicted sensory attributes, beers were spiked with the chemical compounds identified by the models and descriptive sensory analyses were carried out according to the American Society of Brewing Chemists (ASBC) protocol 90 .
Compound spiking was done 30 min before tasting. Compounds were spiked into fresh beer bottles, that were immediately resealed and inverted three times. Fresh bottles of beer were opened for the same duration, resealed, and inverted thrice, to serve as controls. Pairs of spiked samples and controls were served simultaneously, chilled and in dark glasses as outlined in the Trained panel section above. Tasters were instructed to select the glass with the higher flavor intensity for each attribute (directional difference test 92 ) and to select the glass they prefer.
The final concentration after spiking was equal to the within-style average, after normalizing by ethanol concentration. This was done to ensure balanced flavor profiles in the final spiked beer. The same methods were applied to improve a non-alcoholic beer. Compounds were the following: ethyl acetate (Merck KGaA, W241415), ethyl hexanoate (Merck KGaA, W243906), isoamyl acetate (Merck KGaA, W205508), phenethyl acetate (Merck KGaA, W285706), ethanol (96%, Colruyt), glycerol (Merck KGaA, W252506), lactic acid (Merck KGaA, 261106).
Significant differences in preference or perceived intensity were determined by performing the two-sided binomial test on each attribute.
Reporting summary
Further information on research design is available in the Nature Portfolio Reporting Summary linked to this article.
Data availability
The data that support the findings of this work are available in the Supplementary Data files and have been deposited to Zenodo under accession code 10653704 93 . The RateBeer scores data are under restricted access, they are not publicly available as they are property of RateBeer (ZX Ventures, USA). Access can be obtained from the authors upon reasonable request and with permission of RateBeer (ZX Ventures, USA). Source data are provided with this paper.
Code availability
The code for training the machine learning models, analyzing the models, and generating the figures has been deposited to Zenodo under accession code 10653704 93 .
Tieman, D. et al. A chemical genetic roadmap to improved tomato flavor. Science 355 , 391–394 (2017).
Article ADS CAS PubMed Google Scholar
Plutowska, B. & Wardencki, W. Application of gas chromatography–olfactometry (GC–O) in analysis and quality assessment of alcoholic beverages – A review. Food Chem. 107 , 449–463 (2008).
Article CAS Google Scholar
Legin, A., Rudnitskaya, A., Seleznev, B. & Vlasov, Y. Electronic tongue for quality assessment of ethanol, vodka and eau-de-vie. Anal. Chim. Acta 534 , 129–135 (2005).
Loutfi, A., Coradeschi, S., Mani, G. K., Shankar, P. & Rayappan, J. B. B. Electronic noses for food quality: A review. J. Food Eng. 144 , 103–111 (2015).
Ahn, Y.-Y., Ahnert, S. E., Bagrow, J. P. & Barabási, A.-L. Flavor network and the principles of food pairing. Sci. Rep. 1 , 196 (2011).
Article CAS PubMed PubMed Central Google Scholar
Bartoshuk, L. M. & Klee, H. J. Better fruits and vegetables through sensory analysis. Curr. Biol. 23 , R374–R378 (2013).
Article CAS PubMed Google Scholar
Piggott, J. R. Design questions in sensory and consumer science. Food Qual. Prefer. 3293 , 217–220 (1995).
Article Google Scholar
Kermit, M. & Lengard, V. Assessing the performance of a sensory panel-panellist monitoring and tracking. J. Chemom. 19 , 154–161 (2005).
Cook, D. J., Hollowood, T. A., Linforth, R. S. T. & Taylor, A. J. Correlating instrumental measurements of texture and flavour release with human perception. Int. J. Food Sci. Technol. 40 , 631–641 (2005).
Chinchanachokchai, S., Thontirawong, P. & Chinchanachokchai, P. A tale of two recommender systems: The moderating role of consumer expertise on artificial intelligence based product recommendations. J. Retail. Consum. Serv. 61 , 1–12 (2021).
Ross, C. F. Sensory science at the human-machine interface. Trends Food Sci. Technol. 20 , 63–72 (2009).
Chambers, E. IV & Koppel, K. Associations of volatile compounds with sensory aroma and flavor: The complex nature of flavor. Molecules 18 , 4887–4905 (2013).
Pinu, F. R. Metabolomics—The new frontier in food safety and quality research. Food Res. Int. 72 , 80–81 (2015).
Danezis, G. P., Tsagkaris, A. S., Brusic, V. & Georgiou, C. A. Food authentication: state of the art and prospects. Curr. Opin. Food Sci. 10 , 22–31 (2016).
Shepherd, G. M. Smell images and the flavour system in the human brain. Nature 444 , 316–321 (2006).
Meilgaard, M. C. Prediction of flavor differences between beers from their chemical composition. J. Agric. Food Chem. 30 , 1009–1017 (1982).
Xu, L. et al. Widespread receptor-driven modulation in peripheral olfactory coding. Science 368 , eaaz5390 (2020).
Kupferschmidt, K. Following the flavor. Science 340 , 808–809 (2013).
Billesbølle, C. B. et al. Structural basis of odorant recognition by a human odorant receptor. Nature 615 , 742–749 (2023).
Article ADS PubMed PubMed Central Google Scholar
Smith, B. Perspective: Complexities of flavour. Nature 486 , S6–S6 (2012).
Pfister, P. et al. Odorant receptor inhibition is fundamental to odor encoding. Curr. Biol. 30 , 2574–2587 (2020).
Moskowitz, H. W., Kumaraiah, V., Sharma, K. N., Jacobs, H. L. & Sharma, S. D. Cross-cultural differences in simple taste preferences. Science 190 , 1217–1218 (1975).
Eriksson, N. et al. A genetic variant near olfactory receptor genes influences cilantro preference. Flavour 1 , 22 (2012).
Ferdenzi, C. et al. Variability of affective responses to odors: Culture, gender, and olfactory knowledge. Chem. Senses 38 , 175–186 (2013).
Article PubMed Google Scholar
Lawless, H. T. & Heymann, H. Sensory evaluation of food: Principles and practices. (Springer, New York, NY). https://doi.org/10.1007/978-1-4419-6488-5 (2010).
Colantonio, V. et al. Metabolomic selection for enhanced fruit flavor. Proc. Natl. Acad. Sci. 119 , e2115865119 (2022).
Fritz, F., Preissner, R. & Banerjee, P. VirtualTaste: a web server for the prediction of organoleptic properties of chemical compounds. Nucleic Acids Res 49 , W679–W684 (2021).
Tuwani, R., Wadhwa, S. & Bagler, G. BitterSweet: Building machine learning models for predicting the bitter and sweet taste of small molecules. Sci. Rep. 9 , 1–13 (2019).
Dagan-Wiener, A. et al. Bitter or not? BitterPredict, a tool for predicting taste from chemical structure. Sci. Rep. 7 , 1–13 (2017).
Pallante, L. et al. Toward a general and interpretable umami taste predictor using a multi-objective machine learning approach. Sci. Rep. 12 , 1–11 (2022).
Malavolta, M. et al. A survey on computational taste predictors. Eur. Food Res. Technol. 248 , 2215–2235 (2022).
Lee, B. K. et al. A principal odor map unifies diverse tasks in olfactory perception. Science 381 , 999–1006 (2023).
Mayhew, E. J. et al. Transport features predict if a molecule is odorous. Proc. Natl. Acad. Sci. 119 , e2116576119 (2022).
Niu, Y. et al. Sensory evaluation of the synergism among ester odorants in light aroma-type liquor by odor threshold, aroma intensity and flash GC electronic nose. Food Res. Int. 113 , 102–114 (2018).
Yu, P., Low, M. Y. & Zhou, W. Design of experiments and regression modelling in food flavour and sensory analysis: A review. Trends Food Sci. Technol. 71 , 202–215 (2018).
Oladokun, O. et al. The impact of hop bitter acid and polyphenol profiles on the perceived bitterness of beer. Food Chem. 205 , 212–220 (2016).
Linforth, R., Cabannes, M., Hewson, L., Yang, N. & Taylor, A. Effect of fat content on flavor delivery during consumption: An in vivo model. J. Agric. Food Chem. 58 , 6905–6911 (2010).
Guo, S., Na Jom, K. & Ge, Y. Influence of roasting condition on flavor profile of sunflower seeds: A flavoromics approach. Sci. Rep. 9 , 11295 (2019).
Ren, Q. et al. The changes of microbial community and flavor compound in the fermentation process of Chinese rice wine using Fagopyrum tataricum grain as feedstock. Sci. Rep. 9 , 3365 (2019).
Hastie, T., Friedman, J. & Tibshirani, R. The Elements of Statistical Learning. (Springer, New York, NY). https://doi.org/10.1007/978-0-387-21606-5 (2001).
Dietz, C., Cook, D., Huismann, M., Wilson, C. & Ford, R. The multisensory perception of hop essential oil: a review. J. Inst. Brew. 126 , 320–342 (2020).
CAS Google Scholar
Roncoroni, Miguel & Verstrepen, Kevin Joan. Belgian Beer: Tested and Tasted. (Lannoo, 2018).
Meilgaard, M. Flavor chemistry of beer: Part II: Flavor and threshold of 239 aroma volatiles. in (1975).
Bokulich, N. A. & Bamforth, C. W. The microbiology of malting and brewing. Microbiol. Mol. Biol. Rev. MMBR 77 , 157–172 (2013).
Dzialo, M. C., Park, R., Steensels, J., Lievens, B. & Verstrepen, K. J. Physiology, ecology and industrial applications of aroma formation in yeast. FEMS Microbiol. Rev. 41 , S95–S128 (2017).
Article PubMed PubMed Central Google Scholar
Datta, A. et al. Computer-aided food engineering. Nat. Food 3 , 894–904 (2022).
American Society of Brewing Chemists. Beer Methods. (American Society of Brewing Chemists, St. Paul, MN, U.S.A.).
Olaniran, A. O., Hiralal, L., Mokoena, M. P. & Pillay, B. Flavour-active volatile compounds in beer: production, regulation and control. J. Inst. Brew. 123 , 13–23 (2017).
Verstrepen, K. J. et al. Flavor-active esters: Adding fruitiness to beer. J. Biosci. Bioeng. 96 , 110–118 (2003).
Meilgaard, M. C. Flavour chemistry of beer. part I: flavour interaction between principal volatiles. Master Brew. Assoc. Am. Tech. Q 12 , 107–117 (1975).
Briggs, D. E., Boulton, C. A., Brookes, P. A. & Stevens, R. Brewing 227–254. (Woodhead Publishing). https://doi.org/10.1533/9781855739062.227 (2004).
Bossaert, S., Crauwels, S., De Rouck, G. & Lievens, B. The power of sour - A review: Old traditions, new opportunities. BrewingScience 72 , 78–88 (2019).
Google Scholar
Verstrepen, K. J. et al. Flavor active esters: Adding fruitiness to beer. J. Biosci. Bioeng. 96 , 110–118 (2003).
Snauwaert, I. et al. Microbial diversity and metabolite composition of Belgian red-brown acidic ales. Int. J. Food Microbiol. 221 , 1–11 (2016).
Spitaels, F. et al. The microbial diversity of traditional spontaneously fermented lambic beer. PLoS ONE 9 , e95384 (2014).
Blanco, C. A., Andrés-Iglesias, C. & Montero, O. Low-alcohol Beers: Flavor Compounds, Defects, and Improvement Strategies. Crit. Rev. Food Sci. Nutr. 56 , 1379–1388 (2016).
Jackowski, M. & Trusek, A. Non-Alcohol. beer Prod. – Overv. 20 , 32–38 (2018).
Takoi, K. et al. The contribution of geraniol metabolism to the citrus flavour of beer: Synergy of geraniol and β-citronellol under coexistence with excess linalool. J. Inst. Brew. 116 , 251–260 (2010).
Kroeze, J. H. & Bartoshuk, L. M. Bitterness suppression as revealed by split-tongue taste stimulation in humans. Physiol. Behav. 35 , 779–783 (1985).
Mennella, J. A. et al. A spoonful of sugar helps the medicine go down”: Bitter masking bysucrose among children and adults. Chem. Senses 40 , 17–25 (2015).
Wietstock, P., Kunz, T., Perreira, F. & Methner, F.-J. Metal chelation behavior of hop acids in buffered model systems. BrewingScience 69 , 56–63 (2016).
Sancho, D., Blanco, C. A., Caballero, I. & Pascual, A. Free iron in pale, dark and alcohol-free commercial lager beers. J. Sci. Food Agric. 91 , 1142–1147 (2011).
Rodrigues, H. & Parr, W. V. Contribution of cross-cultural studies to understanding wine appreciation: A review. Food Res. Int. 115 , 251–258 (2019).
Korneva, E. & Blockeel, H. Towards better evaluation of multi-target regression models. in ECML PKDD 2020 Workshops (eds. Koprinska, I. et al.) 353–362 (Springer International Publishing, Cham, 2020). https://doi.org/10.1007/978-3-030-65965-3_23 .
Gastón Ares. Mathematical and Statistical Methods in Food Science and Technology. (Wiley, 2013).
Grinsztajn, L., Oyallon, E. & Varoquaux, G. Why do tree-based models still outperform deep learning on tabular data? Preprint at http://arxiv.org/abs/2207.08815 (2022).
Gries, S. T. Statistics for Linguistics with R: A Practical Introduction. in Statistics for Linguistics with R (De Gruyter Mouton, 2021). https://doi.org/10.1515/9783110718256 .
Lundberg, S. M. et al. From local explanations to global understanding with explainable AI for trees. Nat. Mach. Intell. 2 , 56–67 (2020).
Ickes, C. M. & Cadwallader, K. R. Effects of ethanol on flavor perception in alcoholic beverages. Chemosens. Percept. 10 , 119–134 (2017).
Kato, M. et al. Influence of high molecular weight polypeptides on the mouthfeel of commercial beer. J. Inst. Brew. 127 , 27–40 (2021).
Wauters, R. et al. Novel Saccharomyces cerevisiae variants slow down the accumulation of staling aldehydes and improve beer shelf-life. Food Chem. 398 , 1–11 (2023).
Li, H., Jia, S. & Zhang, W. Rapid determination of low-level sulfur compounds in beer by headspace gas chromatography with a pulsed flame photometric detector. J. Am. Soc. Brew. Chem. 66 , 188–191 (2008).
Dercksen, A., Laurens, J., Torline, P., Axcell, B. C. & Rohwer, E. Quantitative analysis of volatile sulfur compounds in beer using a membrane extraction interface. J. Am. Soc. Brew. Chem. 54 , 228–233 (1996).
Molnar, C. Interpretable Machine Learning: A Guide for Making Black-Box Models Interpretable. (2020).
Zhao, Q. & Hastie, T. Causal interpretations of black-box models. J. Bus. Econ. Stat. Publ. Am. Stat. Assoc. 39 , 272–281 (2019).
Article MathSciNet Google Scholar
Hastie, T., Tibshirani, R. & Friedman, J. The Elements of Statistical Learning. (Springer, 2019).
Labrado, D. et al. Identification by NMR of key compounds present in beer distillates and residual phases after dealcoholization by vacuum distillation. J. Sci. Food Agric. 100 , 3971–3978 (2020).
Lusk, L. T., Kay, S. B., Porubcan, A. & Ryder, D. S. Key olfactory cues for beer oxidation. J. Am. Soc. Brew. Chem. 70 , 257–261 (2012).
Gonzalez Viejo, C., Torrico, D. D., Dunshea, F. R. & Fuentes, S. Development of artificial neural network models to assess beer acceptability based on sensory properties using a robotic pourer: A comparative model approach to achieve an artificial intelligence system. Beverages 5 , 33 (2019).
Gonzalez Viejo, C., Fuentes, S., Torrico, D. D., Godbole, A. & Dunshea, F. R. Chemical characterization of aromas in beer and their effect on consumers liking. Food Chem. 293 , 479–485 (2019).
Gilbert, J. L. et al. Identifying breeding priorities for blueberry flavor using biochemical, sensory, and genotype by environment analyses. PLOS ONE 10 , 1–21 (2015).
Goulet, C. et al. Role of an esterase in flavor volatile variation within the tomato clade. Proc. Natl. Acad. Sci. 109 , 19009–19014 (2012).
Article ADS CAS PubMed PubMed Central Google Scholar
Borisov, V. et al. Deep Neural Networks and Tabular Data: A Survey. IEEE Trans. Neural Netw. Learn. Syst. 1–21 https://doi.org/10.1109/TNNLS.2022.3229161 (2022).
Statista. Statista Consumer Market Outlook: Beer - Worldwide.
Seitz, H. K. & Stickel, F. Molecular mechanisms of alcoholmediated carcinogenesis. Nat. Rev. Cancer 7 , 599–612 (2007).
Voordeckers, K. et al. Ethanol exposure increases mutation rate through error-prone polymerases. Nat. Commun. 11 , 3664 (2020).
Goelen, T. et al. Bacterial phylogeny predicts volatile organic compound composition and olfactory response of an aphid parasitoid. Oikos 129 , 1415–1428 (2020).
Article ADS Google Scholar
Reher, T. et al. Evaluation of hop (Humulus lupulus) as a repellent for the management of Drosophila suzukii. Crop Prot. 124 , 104839 (2019).
Stein, S. E. An integrated method for spectrum extraction and compound identification from gas chromatography/mass spectrometry data. J. Am. Soc. Mass Spectrom. 10 , 770–781 (1999).
American Society of Brewing Chemists. Sensory Analysis Methods. (American Society of Brewing Chemists, St. Paul, MN, U.S.A., 1992).
McAuley, J., Leskovec, J. & Jurafsky, D. Learning Attitudes and Attributes from Multi-Aspect Reviews. Preprint at https://doi.org/10.48550/arXiv.1210.3926 (2012).
Meilgaard, M. C., Carr, B. T. & Carr, B. T. Sensory Evaluation Techniques. (CRC Press, Boca Raton). https://doi.org/10.1201/b16452 (2014).
Schreurs, M. et al. Data from: Predicting and improving complex beer flavor through machine learning. Zenodo https://doi.org/10.5281/zenodo.10653704 (2024).
Download references
Acknowledgements
We thank all lab members for their discussions and thank all tasting panel members for their contributions. Special thanks go out to Dr. Karin Voordeckers for her tremendous help in proofreading and improving the manuscript. M.S. was supported by a Baillet-Latour fellowship, L.C. acknowledges financial support from KU Leuven (C16/17/006), F.A.T. was supported by a PhD fellowship from FWO (1S08821N). Research in the lab of K.J.V. is supported by KU Leuven, FWO, VIB, VLAIO and the Brewing Science Serves Health Fund. Research in the lab of T.W. is supported by FWO (G.0A51.15) and KU Leuven (C16/17/006).
Author information
These authors contributed equally: Michiel Schreurs, Supinya Piampongsant, Miguel Roncoroni.
Authors and Affiliations
VIB—KU Leuven Center for Microbiology, Gaston Geenslaan 1, B-3001, Leuven, Belgium
Michiel Schreurs, Supinya Piampongsant, Miguel Roncoroni, Lloyd Cool, Beatriz Herrera-Malaver, Florian A. Theßeling & Kevin J. Verstrepen
CMPG Laboratory of Genetics and Genomics, KU Leuven, Gaston Geenslaan 1, B-3001, Leuven, Belgium
Leuven Institute for Beer Research (LIBR), Gaston Geenslaan 1, B-3001, Leuven, Belgium
Laboratory of Socioecology and Social Evolution, KU Leuven, Naamsestraat 59, B-3000, Leuven, Belgium
Lloyd Cool, Christophe Vanderaa & Tom Wenseleers
VIB Bioinformatics Core, VIB, Rijvisschestraat 120, B-9052, Ghent, Belgium
Łukasz Kreft & Alexander Botzki
AB InBev SA/NV, Brouwerijplein 1, B-3000, Leuven, Belgium
Philippe Malcorps & Luk Daenen
You can also search for this author in PubMed Google Scholar
Contributions
S.P., M.S. and K.J.V. conceived the experiments. S.P., M.S. and K.J.V. designed the experiments. S.P., M.S., M.R., B.H. and F.A.T. performed the experiments. S.P., M.S., L.C., C.V., L.K., A.B., P.M., L.D., T.W. and K.J.V. contributed analysis ideas. S.P., M.S., L.C., C.V., T.W. and K.J.V. analyzed the data. All authors contributed to writing the manuscript.
Corresponding author
Correspondence to Kevin J. Verstrepen .
Ethics declarations
Competing interests.
K.J.V. is affiliated with bar.on. The other authors declare no competing interests.
Peer review
Peer review information.
Nature Communications thanks Florian Bauer, Andrew John Macintosh and the other, anonymous, reviewer(s) for their contribution to the peer review of this work. A peer review file is available.
Additional information
Publisher’s note Springer Nature remains neutral with regard to jurisdictional claims in published maps and institutional affiliations.
Supplementary information
Supplementary information, peer review file, description of additional supplementary files, supplementary data 1, supplementary data 2, supplementary data 3, supplementary data 4, supplementary data 5, supplementary data 6, supplementary data 7, reporting summary, source data, source data, rights and permissions.
Open Access This article is licensed under a Creative Commons Attribution 4.0 International License, which permits use, sharing, adaptation, distribution and reproduction in any medium or format, as long as you give appropriate credit to the original author(s) and the source, provide a link to the Creative Commons licence, and indicate if changes were made. The images or other third party material in this article are included in the article’s Creative Commons licence, unless indicated otherwise in a credit line to the material. If material is not included in the article’s Creative Commons licence and your intended use is not permitted by statutory regulation or exceeds the permitted use, you will need to obtain permission directly from the copyright holder. To view a copy of this licence, visit http://creativecommons.org/licenses/by/4.0/ .
Reprints and permissions
About this article
Cite this article.
Schreurs, M., Piampongsant, S., Roncoroni, M. et al. Predicting and improving complex beer flavor through machine learning. Nat Commun 15 , 2368 (2024). https://doi.org/10.1038/s41467-024-46346-0
Download citation
Received : 30 October 2023
Accepted : 21 February 2024
Published : 26 March 2024
DOI : https://doi.org/10.1038/s41467-024-46346-0
Share this article
Anyone you share the following link with will be able to read this content:
Sorry, a shareable link is not currently available for this article.
Provided by the Springer Nature SharedIt content-sharing initiative
By submitting a comment you agree to abide by our Terms and Community Guidelines . If you find something abusive or that does not comply with our terms or guidelines please flag it as inappropriate.
Quick links
- Explore articles by subject
- Guide to authors
- Editorial policies
Sign up for the Nature Briefing: Translational Research newsletter — top stories in biotechnology, drug discovery and pharma.

Academia.edu no longer supports Internet Explorer.
To browse Academia.edu and the wider internet faster and more securely, please take a few seconds to upgrade your browser .
- We're Hiring!
- Help Center
Food and Beverage
- Most Cited Papers
- Most Downloaded Papers
- Newest Papers
- Save to Library
- Last »
- Clinical Pharmacy Practice Follow Following
- Pharmaceutical Formulation Technology Follow Following
- Pharmaceuticals Follow Following
- Primary Care Follow Following
- Colorectal cancer Follow Following
- Stem Cell Research Follow Following
- DOCTOR OF PHARMACY Follow Following
- Urology Follow Following
- Food and Beverage Management Follow Following
- FOOD AND BEVERAGES Follow Following
Enter the email address you signed up with and we'll email you a reset link.
- Academia.edu Publishing
- We're Hiring!
- Help Center
- Find new research papers in:
- Health Sciences
- Earth Sciences
- Cognitive Science
- Mathematics
- Computer Science
- Academia ©2024
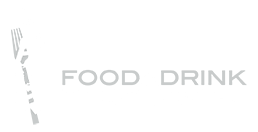
Consumer Research and Insights
Simplify culinary consumer research. At the FDR Research & Innovation Center, our market research moderators and development chefs handle all aspects of a food/beverage and restaurant consumer research project.
Market Research For The Food And Beverage Industry
Food & Drink Resources (FDR) hosts and facilitates qualitative and quantitative research projects for restaurants, food and beverage manufacturers, and other consumer packaged goods companies. Whatever your consumer research needs, you will find it's possible at FDR.
- Moderating service led by experienced facilitators
- Central location testing (including a quantitative/qualitative approach)
- Comparison testing (paired, triangle, duo-trio)
- Focus groups (two focus group rooms with two-way mirrors and audio/video recording)
- Online surveys (concept testing/sorts, communications, menu satisfaction/TURF)
- Ideation/innovation sessions
- Immersion tours
- In-depth interviews/ethnographies/diaries
- Industry trends
- Mystery shopping/competitive shopping
- Sensory/taste tests (20 booths each with iPads)
- Usability studies (appliances, packaging, product preparation)
- Our very own 3C Development Process™
- Restaurant market research
- Food/beverage market research
- CPG market research
With a large consumer test kitchen and commercial test kitchen with bar, you will get on-the-spot adjustments to your recipe to perfect the product and expedite the commercialization process. This turns into significant savings for you.
Why Choose Our Denver Market Research Company?
Historically, marketing research projects require you to work with one vendor for a location, a second for a facilitator, a third for respondent recruiting, and a fourth for data analysis. Food market research doesn’t have to be a disconnected process anymore.
At FDR, we offer both turnkey and single-service market research support. We are happy to work with your current vendors but please know that we can also provide all you need for a less expensive and more fluid consumer research experience.
To inquire about culinary or any other consumer research at our FDR's Denver Research & Innovation Center, please request a bid . You may also find more information on our Consumer Research FAQs page .

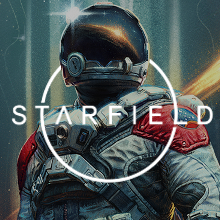
Starfield Walkthrough & Guides Wiki
- Main Quests
- Side Quests
- Beginner's Guide
- New Game Plus
Research Projects
List of All Food and Drink Research Projects
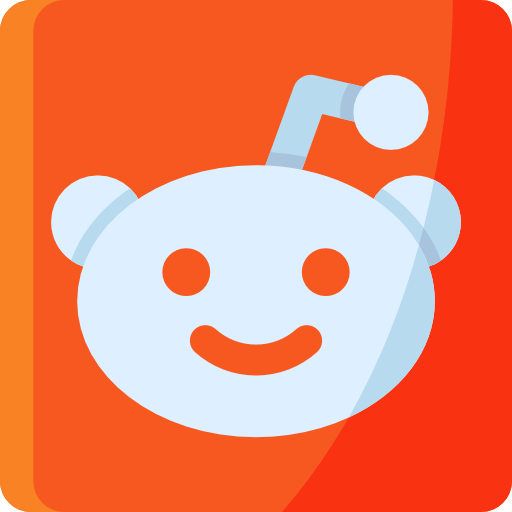
★ Best Guides: Best Weapons | Best Skills ☆ All Ship Parts and Ship Part Level Unlocks ★ Advanced Outpost Guide ☆ Maps: New Atlantis | Cydonia | Akila City | Neon ★ Ship Designs : Rocinante | TARDIS | Millennium Falcon
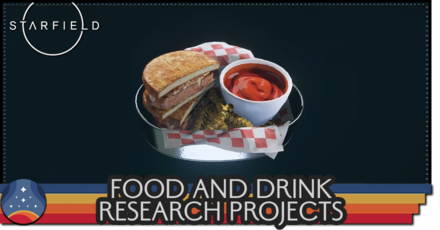
Food and Drink Research Projects can be completed at a Research Lab in Starfield. Read on to learn the different Food and Drink Research Projects, how to complete and unlock them, the Resources and Skills needed to complete them, as well as what each project unlocks.
List of Contents
All Food and Drink Research Projects
Old earth cuisine, beverage development.
- How to Complete
- How to Unlock
What Are Food and Drink Research Projects?
- Related Guides
Exotic Recipes
How to complete food and drink research projects, complete at a research lab.
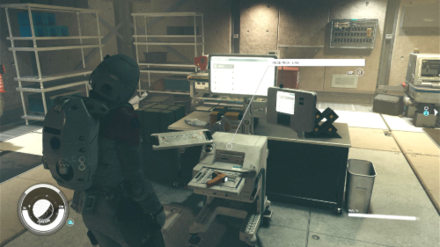
You can research Food and Drink Research Projects at a Research Lab. These can be found in your ship, outposts, and major settlements in the game like New Atlantis .
Once you're at the Research Lab, you can do the following.
- Access the Research Lab terminal and select the " Food and Drink " category.
- Next, choose a research project that you want to work on. Each project will have a list of requirements, such as the types of resources you need and the skills needed to complete it.
- Gather the resources and unlock the skills needed to complete the project, then go back to the Research lab to complete it.
- You can contribute resources to the project at any time, and you can also speed up the research by assigning crew members to the Research Lab. Once the project is complete, you will be able to craft recipes that provide healing and stat boosts at a Cooking Station.
How to Unlock Food and Drink Research Projects
Complete more research projects.
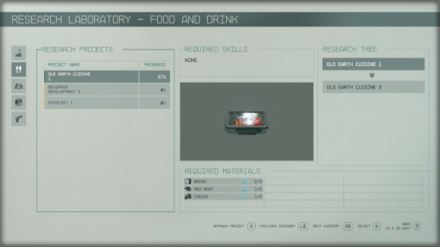
There are four Food and Drink Research Project trees in the game. The first tier of each tree is already unlocked the first time you access the Research Lab terminal.
You need to complete the pre-requisite Research Projects in a tree in order to unlock all succeeding Research Projects in that tree.
Unlock Food and Drink Recipes
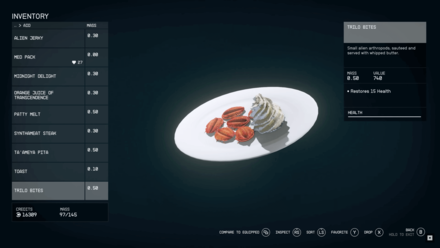
Food and drink research projects unlock the crafting recipes of consumable items that provide healing and stat boosts.
List of All Food
Starfield Related Guides
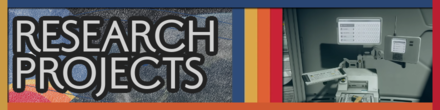
- List of All Research Projects
All Research Project Types
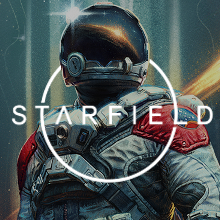
We at Game8 thank you for your support.
In order for us to make the best articles possible, share your corrections, opinions, and thoughts about 「List of All Food and Drink Research Projects | Starfield」 with us!
When reporting a problem, please be as specific as possible in providing details such as what conditions the problem occurred under and what kind of effects it had.
Do you want to send this information?
Walkthrough Menu
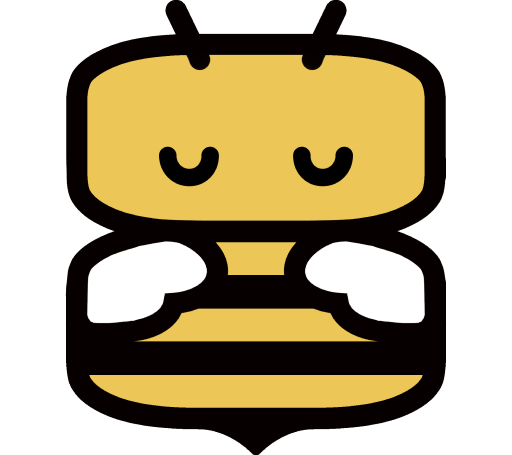
- Starfield Wiki Top
- List of All Main Quests
- One Small Step
- The Old Neighborhood
- The Empty Nest
- Back to Vectera
- Into the Unknown
- All That Money Can Buy
- Further Into the Unknown
- Short Sighted
- No Sudden Moves
- High Price to Pay
- In Their Footsteps
- Final Glimpses
- Missed Beyond Measure
- One Giant Leap
- Among the Stars
- Foreknowledge
Joinable Factions
- List of All Factions
- Constellation
- Crimson Fleet
- UC Vanguard
- Freestar Collective
- Ryujin industries
- Best Faction to Join
- House Va'ruun
- Sanctum Universum
- Enlightened
- List of All Side Quests
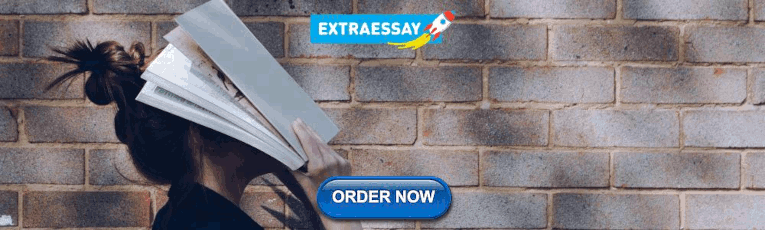
All Side Quests
- In Memoriam
- Divided Loyalties
- A Tree Grows in New Atlantis
- Left Behind
- Alternating Currents
- Beautiful Secrets
- Tapping the Grid
- Out on a Limb
- Distilling Confidence
- Missed the Mark
- Missed Connections
- First Contact
- A Break at Dawn
- Search and Seizure
- Defensive Measures
- Groundpounder
- False Positives
- Red Tape Blues
- Red Tape Runaround
- Red Tape Reclamation
- Juno's Gambit
- Failure to Communicate
- Overdesigned
- Saburo's Solution
- Tourists Go Home
- Lost and Found
- Operation Starseed
- Secret Lives
- Power From Beyond
- Charity of the Wolf
- The Audition
- Media Sponge
- Fishy Business
- Last Will and Testament
- Managing Assets
- Supply Line
- Breach of Contract
- Heart of Mars
- Late Bloomer
- Display of Power
- Two Tales Two Cities
- Mob Mentality
- The Showdown
- Special Sauce
- Burden of Proof
- Rough Landings
- Space Frog From Outer Space
- Special Delivery
- Due in Full
- Taste of Home
- Pirate Activity
- Preventive Action
- Primary Sources
- Apex Predator
- A Light in the Darkness
- All For One
- The Kindness of Strangers
- Start-up Stopped
- Infinity's End
- The Great Laredo Caper
- Dirty Laundry
- Suspicious Activities
- Starsap Tours
- Captain's Bounty
- Gem Jealousy
- The Akila Run
- Freight Fright
- Spreading the News
- Top of the L.I.S.T.
- Matters of the Hart
- Re-Re-Application
- Unjustified
- Winning Hearts and Minds
- Proper Tea Theft
- Liquid Assets
- An Invitation
- A Shipment for Salinas
- Olive Branch
- UC Wants You!
- Worlds Apart
- Terror Brew
- A Parting Gift
- Reliable Care
- Refurbished Goods
- The Bounty That Got Away
- Companion's Belongings
- Last Resort
- Debt Forgiveness
- Drinks on the House
- Family Reunion
- Wanted Guest
- Escape from the Endless Voyage
- Fueling Greatness
- My North Star
- One Riot, One Ranger
- Drydock Blues
Other Side Quest Guides
- Best Side Quests
Mission Board
- Mission Board Quests
- How to Accept Bounty Missions
Tips and Tricks
- Beginner's Guide to Starfield
Starter Guides
- All UI and Menu Icons Explained
- Periodic Table Explained
- All Stats Explained
- Reputation System Explained
- All Religions Explained
- Level Scaling Explained
- How to Remove Bounties
- Digipick Guide
- Persuasion Guide
- Stealing Guide
- Durability Guide
- All Crimes You Can Commit
- Zero G Effects Explained
- How to Cure Infections
- Space Piracy Explained
- What to Do First
- Oxygen System Explained
- Environmental Damage Guide
- How to Avoid Getting Encumbered
- How to Cure All Status Afflictions
- How to Cure Broken Bones
- How to Cure Burns
- How to Cure Addiction
- All Damage Types Explained
- Crew Explained
- Red and Yellow Item Icons Explained
- How to Use the Quest Marker
- Health Bar Explained
- Weapon Tiers and Rarity Explained
Combat Guides
- Combat Tips
- Aiming Guide
- How to Stealth Kill Enemies
- How to Throw Grenades
- How to Block Attacks
- How to Mantle and Climb Ledges
- How to Remove Weapon Mods
- How to Combat Slide
- Critical Hit Chance and Rate Guide
- How to Stun and Stagger Enemies
- How to Disarm Enemies
- How to Detect and Disarm Landmines
Ship Guides
- Space Battles Combat Guide
- How to Increase Jump Range
- Ship Customization Guide
- How to Get Fuel
- How to Dock Your Ship
- How to Upgrade Ship
- How to Target a Ship's Engine
- How to Get Shielded Cargo Holds
- How to Power Up the Grav Drive
- How to Fix Unattached Modules
- How to Repair Ships
- How to Get and Fire Turrets
- How to Sell Ships
- How to Hijack Ships
- How to Rename Ships
- How to Change Home Ships
- What is GRV?
- Missing Weapon Assignment Error
- Ship Classes Explained
Farming Guides
- How to Level Up Fast
- How to Farm Credits
- How to Farm Legendary Weapons
- How to Get More Digipicks
- Infinite Money Glitch
- Infinite Weapons Exploit
General Tips and Tricks
- All Missables Guide
- All Names Companions Can Say
- Best Settings for PC and Console
- Character Creation Options
- All Romance Options
- Where to Sell Items
- All Planetary Traits
- Difficulty Differences
- Where to Store Items
- Crafting Guide: How to Craft
- Companion Affinity Guide
- How to Access Computers
- How to Pay Off Mortgage and Bank Loans
- Industrial Workbench Locations
- Weapon Workbench Locations
- How to Install Mods
- Spacesuit Workbench Locations
- How to Get Free Ships
- Endings Guide
- Nova Galactic Staryard Skip
- How to Restock Vendors
- Keybinds for PC and Xbox
- Best Mods List
- List of All Unlockables
- List of Easter Eggs and Secrets
- How to Show Damage Numbers
- Language Options and How to Change Language
- List of Exploits
- How to Turn on the Flashlight
- List of Error Prompts
- List of All Random Encounters
- New Game Plus Explained
- All Universe Variations Explained
- Where to Find Cooking Stations
- Where to Find Pharmaceutical Labs
- Rescue Barrett Skip
- How to Speedrun New Game Plus
Quest Objective Guides
- Vanguard Simulation Guide
Equipment Guides
- Hunter Armor and Weapon Location
- Emissary Armor and Weapon Location
Exploration Guides
- How to Get Cold Resistance
- How to Get Radiation Resistance
- How to Get 100% Survey Score
- How to Mine Faster
- What to Do on Gas Giant Planets
- How to Go to the Ocean Biome
- All Unexplored Ecological Features and How to Scan
Location Guides
- Sanctum Universum Location
- Locked Door in the Well
- How to Access the Dev Room
- Almagest Casino Jackpot Location
- How to Hide Spacesuit and Helmet
- How to Change Background
- How to Change FOV
- How to Fast Travel
- What Happens When You Die?
- How to Remove Traits
- How to Wait and Skip Time
- How to Skip Dialogue and Cutscenes
- How to Holster Your Weapon
- How to Scrap Junk
- Can You Change Crosshairs?
- How to Increase Health
- How to Use Photo Mode
- How to Intimidate People
- How to Use Jetpack
- Can You Kill NPCs?
- How to Avoid Jail
- Are Mechs Usable?
- What is the Max Level?
- Can You Get Pets?
- How Does Free Roam Work?
- Is There Underwater Exploration?
- Are There Land or Water Vehicles?
- Is There Fishing?
- Are There Black Holes?
- Are There Cybernetic Enhancements?
- Can You Build a Fleet?
- How to Pickpocket Successfully
- How to Sneak And Avoid Detection
- How to Increase Carrying Capacity
- Is There Fall Damage?
- How to Increase Jump Height
- Can You Adopt Kids?
- How to Use the Hand Scanner
- How to Bribe NPCs
- Cannot Play Early Access Fix
- Can You Change Pronouns?
- How to Change POV
- How to Turn Off XP Pop Up
- Should You Do the Main Quest?
- How to Claim Pre-Order Bonuses
- How to Access Ship Storage
- How to Use Quick Slots and Switch Weapons
- Is there a Survival Mode?
- Unlock Master Lock in The Lodge
- Change Companions and Crew
- How to Change Your Name
- Which First Follower to Choose
- How to Display FPS Counter
- What to Do If You Get Stuck
- What to Buy First
- Where to Sell Survey Data
- Cannot Fast Travel Fix Guide
- Can You Dismantle Weapons?
- How to Save
- How to Remove Stolen Label on Items
- Can You Craft Ammo?
- Can You Add More Saves?
- How to Put Out Fires
- How Does Procedural Generation Work
- Are There Radio Stations?
- How Do Tiles Work?
- How to Reenable Achievements
- Can You Steal the Fortuna?
- Best Builds
- Beast Hunter Build
- Bouncer Build
- Bounty Hunter Build
- Combat Medic Build
- Cyber Runner Build
- Cyberneticist Build
- Diplomat Build
- Explorer Build
- File Not Found Build
- Gangster Build
- Homesteader Build
- Industrialist Build
- Long Hauler Build
- Pilgrim Build
- Professor Build
- Ronin Build
- Sculptor Build
- Soldier Build
- Space Scoundrel Build
- Xenobiologist Build
- Stealth Sniper Build
- Space Pirate Build
- Space Cowboy Build
- Unarmed Build
- Pacifist Build
Best Weapons
- Best Weapons: Weapon Tier List
- Best Weapon Combinations
- Best Weapon Modifiers
- Best Melee Weapons
- Best Shotguns
- Best Rifles
- Best Scoped Weapons
- Best Pistols
- Best Laser Weapons
- Best Laser Rifles
- Best Particle Beam Weapons
- Best Heavy Weapons
- List of All Skills
- Best Skills
- Physical Skills
- Social Skills
- Combat Skills
- Science Skills
- Tech Skills
- List of All Traits
- Best Traits
- Freestar Collective Settler
- Hero Worshipped
- Neon Street Rat
- Raised Enlightened
- Raised Universal
- Serpent's Embrace
- Terra Firma
- United Colonies Native
Starborn Powers
- List of All Starborn Powers
- Best Starborn Powers
- Alien Reanimation
- Anti-Gravity Field
- Create Vacuum
- Creators' Peace
- Elemental Pull
- Eternal Harvest
- Gravity Wave
- Gravity Well
- Inner Demon
- Life Forced
- Parallel Self
- Particle Beam
- Personal Atmosphere
- Phased Time
- Precognition
- Reactive Shield
- Sense Star Stuff
- Solar Flare
- Sunless Space
- List of All Companions
- Best Companions
- Sarah Morgan
- Marika Boros
- Adoring Fan
- Simeon Bankowski
- Gideon Aker
- Moara Otero
- Andromeda Kepler
- Omari Hassan
- Rafael Aguerro
- Rosie Tannehill
- Mickey Caviar
- Jessamine Griffin
- Sophia Grace
- Dani Garcia
- Walter Stroud
- Matteo Khatri
- Vladimir Sall
- Amelia Earhart
- Lyle Brewer
- Betty Howser
- Erick Von Price
- Mathis Castillo
- Major Hadrian Sanon
- Autumn MacMillan
- Alternate Self
- List of All Planets
Star Systems
- List of All Star Systems
Cities and POIs
All cities and pois.
- All Cities and Points of Interests
- New Atlantis
- New Homestead
Points Of Interest
- Deimos Staryard
- Nova Galactic Staryard
- Starstation UCN-48
- The Colander
- Starstation RE-939
- Vulture's Roost
- Deimos Armored Transport
- ECS Constant
- Eleos Retreat
- The Clinic Space Station
- List of All Shops and Space Ports
- List of All Bars
- Trade Authority
- Jemison Mercantile
- The Dream House
- The Well Apartment
- Mercury Tower Penthouse
- The Stretch Apartment
- Core Residence
- List of All Weapons
- All Unique Weapons
- All EM (Non-Lethal) Weapons
- All Pistols
- All Shotguns
- All Heavy Weapons
- All Melee Weapons
- All Ballistic Weapons
- All Ballistic Rifles
- All Ballistic Pistols
- All Laser Weapons
- All Laser Rifles
- All Laser Pistols
- All Particle Beam Weapons
- All Particle Beam Rifles
- All Particle Beam Pistols
- All Old Earth Weapons
Weapon Mods
- List of All Weapon Mods
- Grips & Stocks
- Magazines & Batteries
- List of All Armor and Gear
- All Unique Armor
- All Helmets
- All Spacesuits
- All Apparel
- All Armor Sets
- Best Armor Sets
- How to Get All Starborn Armors
- List of All Armor Mods
- Helmet Mods
- Spacesuit Mods
Resources and Materials
- List of All Resources
- List of All Ships
- Legendary Ships
Ship Designs
- Best Ship Designs
- Event Horizon
- Millennium Falcon
- Star Destroyer
- List of All Ship Parts
- Best Ship Parts
- All Ship Part Level Unlocks
- Cargo Holds
- Grav Drives
- Landing Bays
- Ship Weapons
- Landing Gears
- Shield Generators
Ship Manufacturers
- List of All Ship Manufacturers
- Collectibles
- Skill Magazines
- All CombaTech Catalogs
- All Constellation Guides
- All Cyber Runner's Ciphers
- All Freestar Captain's Logs
- All GRUNT Issues
- All Gunslinger's Guides
- All Kryx's Journal Entries
- All Mining Monthly Issues
- All Neon Nights
- All New Atlantians
- All Nova Galactic Manuals
- All Peak Performances
- All Solomon's Adventures
- All Tracker's Primers
- All UC Defense Manuals
- All Vanguard Space Tactics
- All Va'ruun Scriptures
- Quantum Essences
- Snow Globes
- Dragonstar Force Books
- Dialogue Choices and Consequences
- Should You Have Sarah Morgan Be Your Follower?
- Should You Kill Brogan?
- Should You Defend the Lodge or Go to The Eye?
- Should You Give Evidence to Louisa or Zoe?
- How to Persuade Emilio Hadek
- Should You Kill Petrov?
- Should You Kill Austin Rake?
- What is the Vanguard Motto?
- Should You Accept Maldonaldo's Offer?
- Should Betray Delgado?
- Should You Skip the Final Boss Fight?
- Should You Walk into the Light at the Unity?
- Should You Destroy Governor Hurst's Ship?
- Should You Side with Mathis and Kill Delgado?
- Should You Kill Tomisar?
- Should You Kill Marco Graziani?
- Should You Kill Shaw?
- Should You Kill Maya?
- Should You Kill Matsura?
- Should You Kill Ron Hope?
- Should You Kill Livvey?
- Should You Betray Huan?
- Should You Kill Tomo?
- Should You Give Naeva the ES Award?
- Should You Turn In Vae Victis?
- Should You Kill Imogene?
- Should You Kill Larry Dumbrosky?
- Should You Buy a Grav Drive or Take the Settlement Deal?
- Should You Kill Rusty?
- Pharmacology
- Food and Drink
- Outpost Development
Backgrounds
- List of Backgrounds
- Best Backgrounds Tier List
- List of All Items
- Food and Aid
- All Outpost Modules and How to Build
- Best Outpost Locations
Outpost Building FAQs
- All Outpost Extractors
- All Outpost Power Modules
- All Outpost Structures
- All Outpost Storage
- All Outpost Furniture
- All Outpost Robots
- All Outpost Decorations
- All Outpost Defenses
- All Outpost Displays
- All Outpost Miscellaneous Modules
- All Outpost Crafting Modules
- How to Delete Outposts
- How to Add Doors to Outpost Habs
- How to Delete Outpost Links
- Transfer Container Storage Guide
- How to Raise Flora
- How to Domesticate Fauna
- How to Craft a Greenhouse
- Animal Husbandry Facility Guide
- Best Houses and How to Get All Houses
- List of All Enemies
- List of All Flora and Plants
- List of All Fauna and Alien Creatures
Achievements
- List of All Achievements
- All Puzzle Solutions
- Mantis Puzzle Solution
- Glyph Puzzle Solution
- Generator Power Puzzle Solution
- Temple Eta Puzzle
Console Commands
- Console Commands List
- All Item IDs
- Weapon Item IDs
- Armor Item IDs
- Mod Item IDs
- Ammo Item IDs
- Background IDs
- Faction IDs
- Resource and Material Item IDs
- Unique and Legendary Weapon IDs
- Unique and Legendary Armor IDs
- Weapon Modifier IDs
- Armor Modifier IDs
- Aid Item IDs
- Food Item IDs
- Location IDs
- Skill Magazine IDs
- Starborn Power IDs
- Apparel IDs
- Armor and Weapon Quality Level IDs
- NoClip Command
- Increase Carry Weight Console Command
Bugs and Errors
- All Bugs, Glitches, and Known Issues
- Low FPS Performance Issue
- Failed to Create Save Game Error
- Headless Character Bug
- Puddle Glitch Explained
News and Updates
Updates and patch notes.
- Patch Notes and Updates
- Day One Patch
- Update 1.7.29 Patch Notes
- Update 1.7.33 Patch Notes
- Update 1.7.36 Patch Notes
- Latest News
- Preload Guide
- Release Time and Countdown
- Early Access Date and Times
- PC System Requirements
- Available Platforms
- Upcoming DLC
- Pre-Order Bonus Guide
- Xbox Game Pass
- Plot Summary and Lore Explained
- How Long to Beat
- How to Get Controller and Headset
- Is There Multiplayer?
- All Available Editions
- Accessibility Features
- File Download Size
- Keyboard and Mouse Support
- Age Rating and Parental Guide
- Trailers and Gameplay Videos
- Cast and List of Voice Actors
- Joystick or HOTAS Support
- Is Starfield an MMO?
- Is Starfield Compatible on Steam Deck?
- Game Pass Release Time
- 1 Beta Ternion III Planet Location...
- 2 Beta Ternion II Planet Location ...
- 3 Beta Ternion Star System Locatio...
- 4 Space Trucker Haul Wrap - How to...
- 5 List of All Ship Parts
- We could not find the message board you were looking for.
Gaming News
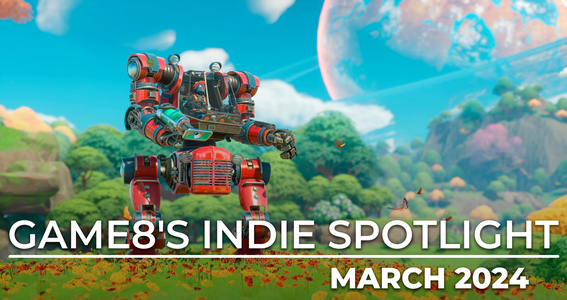
Game8's Indie Spotlight
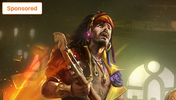
RAID Shadow Legends Promo Codes Give $100 Worth of Bonuse...
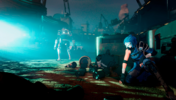
Ereban Review
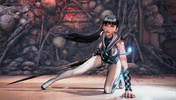
Stellar Blade Was Inspired by Self-Driving Cars
More Gaming News
Popular Games
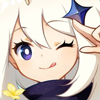
Genshin Impact Walkthrough & Guides Wiki
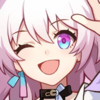
Honkai: Star Rail Walkthrough & Guides Wiki
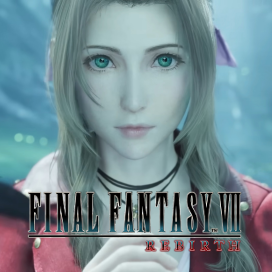
FF7 Rebirth Walkthrough & Guides Wiki
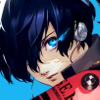
Persona 3 Reload Walkthrough & Guides Wiki
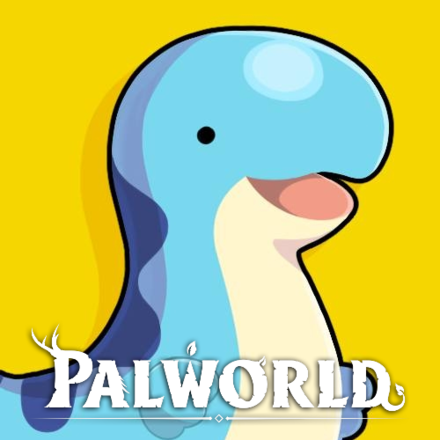
Palworld Walkthrough & Guides Wiki
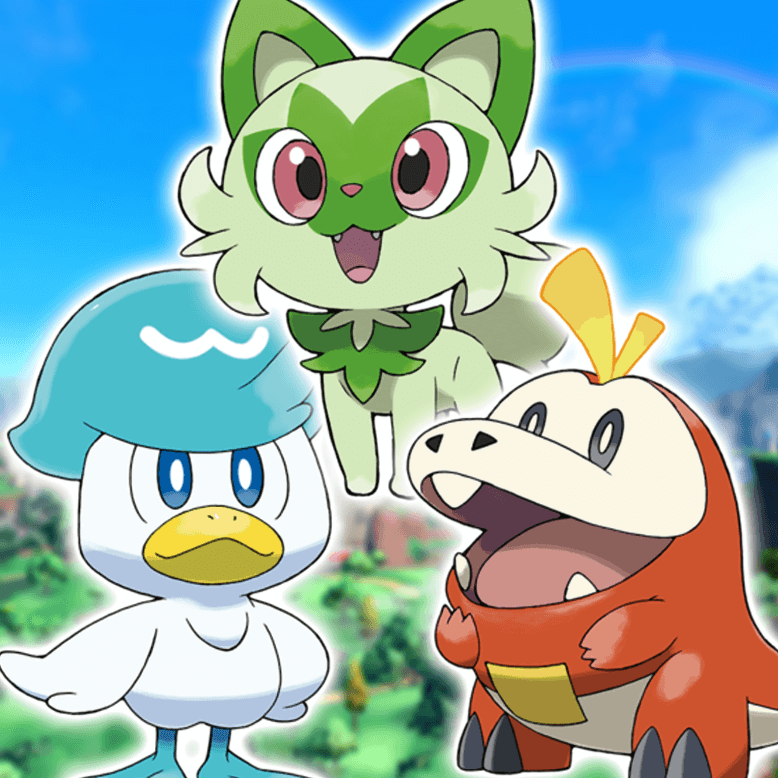
Pokemon Scarlet and Violet (SV) Walkthrough & Guides Wiki
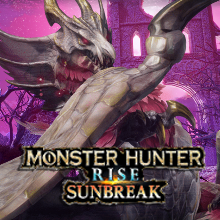
Monster Hunter Rise: Sunbreak Walkthrough & Guides Wiki
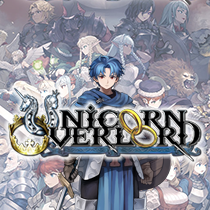
Unicorn Overlord Walkthrough & Guides Wiki
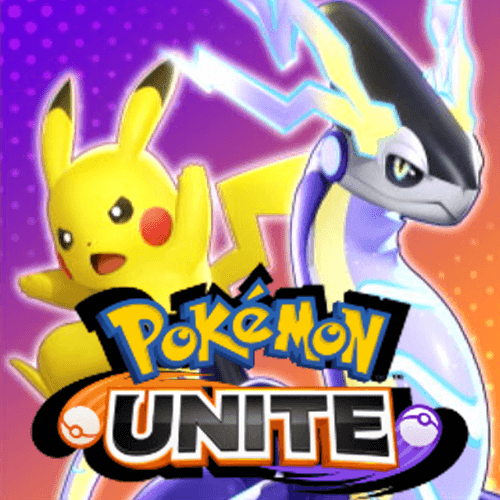
Pokemon UNITE Walkthrough & Guides Wiki
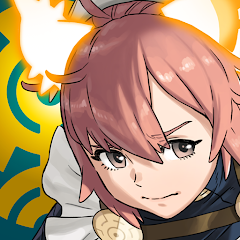
Fire Emblem Heroes (FEH) Walkthrough & Guides Wiki
Recommended Games
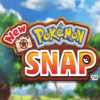
New Pokemon Snap Walkthrough & Guides Wiki
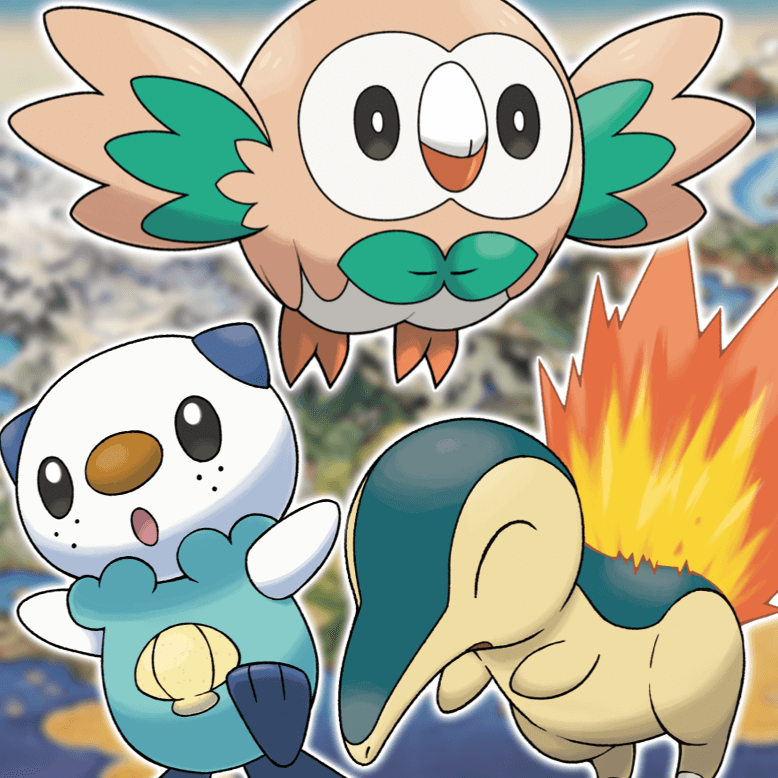
Pokemon Legends: Arceus Walkthrough & Guides Wiki
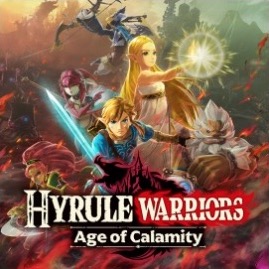
Hyrule Warriors: Age of Calamity Walkthrough & Guides Wiki
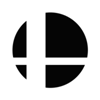
Super Smash Bros. Ultimate Walkthrough & Guides Wiki
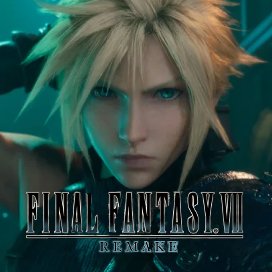
FF7 Remake Walkthrough & Guides Wiki
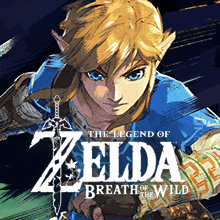
The Legend of Zelda: Breath of the Wild Walkthrough & Guides Wiki
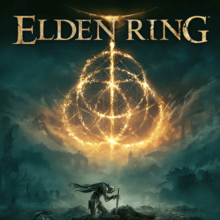
Elden Ring Walkthrough & Guides Wiki
All rights reserved
© 2023 Bethesda Softworks LLC, a ZeniMax Media company. Trademarks belong to their respective owners. All Rights Reserved. The copyrights of videos of games used in our content and other intellectual property rights belong to the provider of the game. The contents we provide on this site were created personally by members of the Game8 editorial department. We refuse the right to reuse or repost content taken without our permission such as data or images to other sites.
- Popular Articles

Michigan Sustainable Agriculture Research and Education Program
Dig into sustainable farm innovations with a search tool and map of sare grant projects.
Katie Brandt <[email protected]> , Michigan State University Extension - April 09, 2024
Find hundreds of SARE grants funded in Michigan and thousands nationally.
Search tool for successful SARE grants and project reports
Searching through projects funded by Sustainable Agriculture Research and Education Program (SARE) grants is simple and allows you to see ideas and results directly from sustainable farm innovators. Examples of grants that have been funded by SARE can help you to write a successful SARE grant and improve sustainability on your farm.
To search the SARE database, go to Search SARE Funded Projects as shown below:
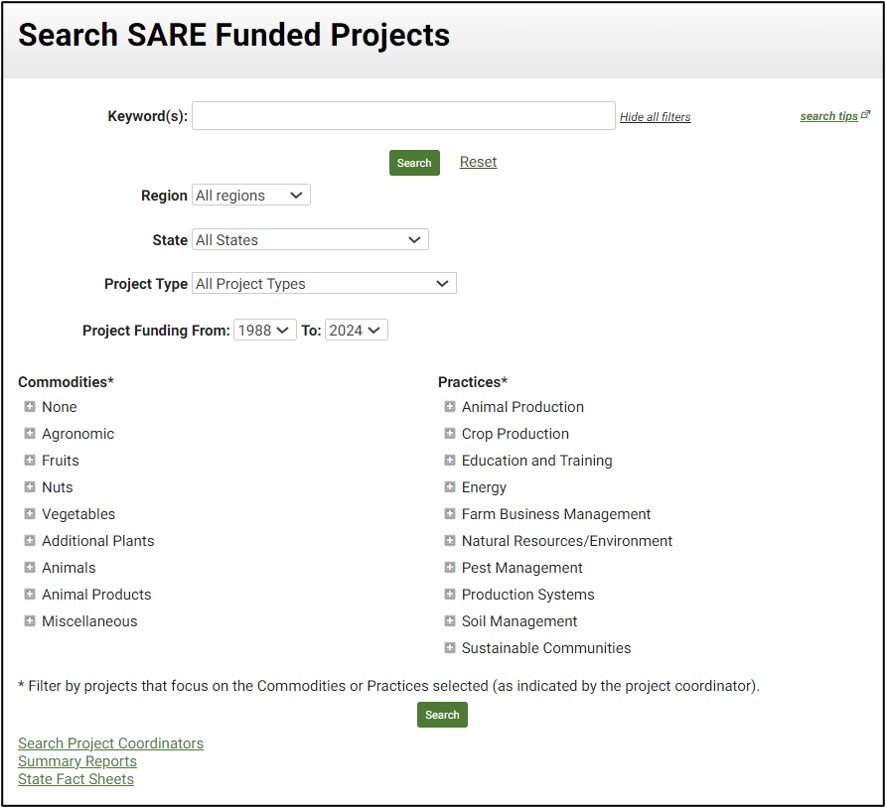
For example, I might be curious about using clover as a cover crop in blueberries and could run the following search:
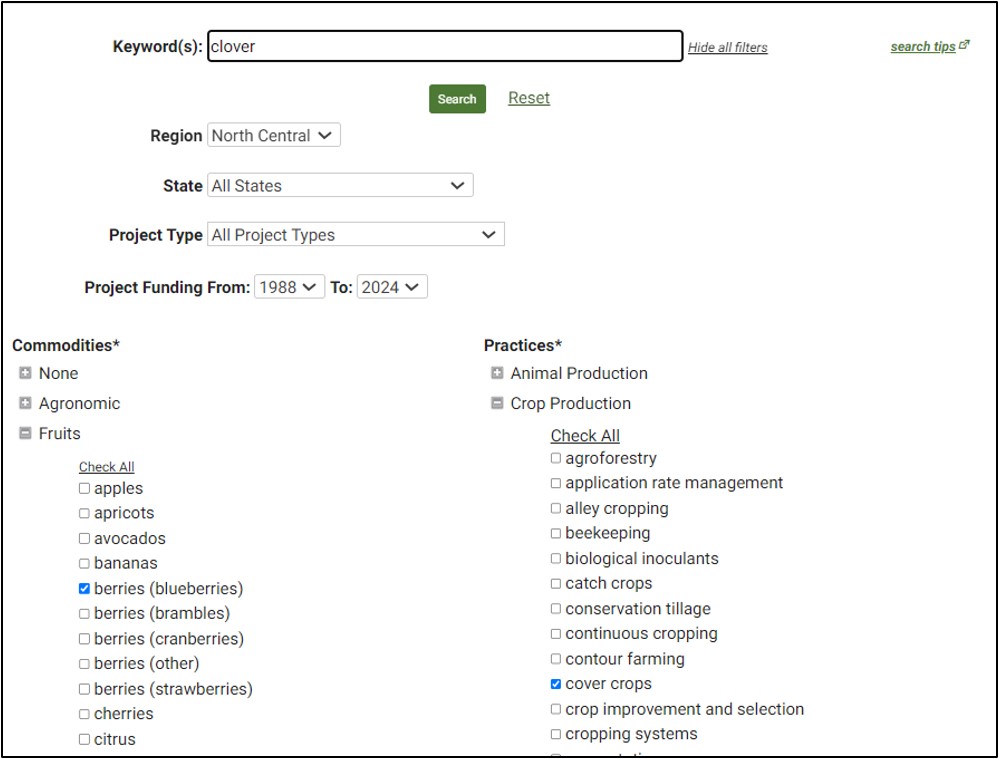
Although that is a very specific search, we found four results:
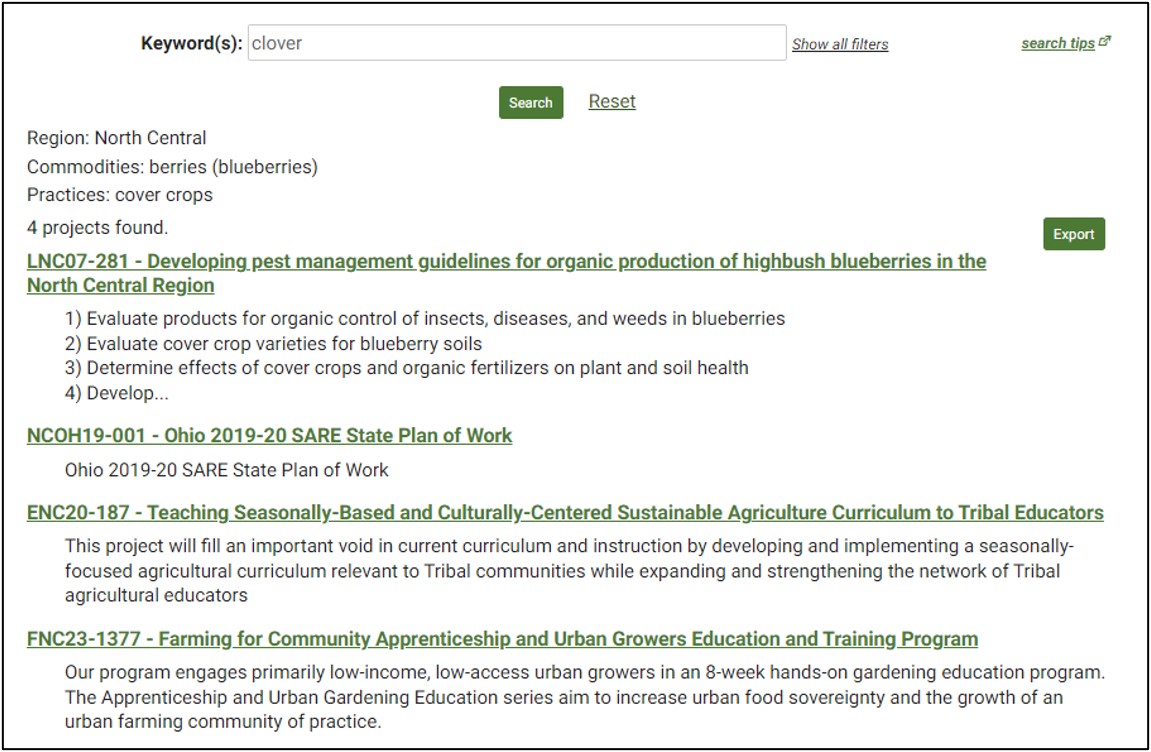
Clicking on any of the proposals will show you the description of the project. If the project is complete, you will also be able to see the final report and possibly also videos, curriculum, slide sets, data or other information products.
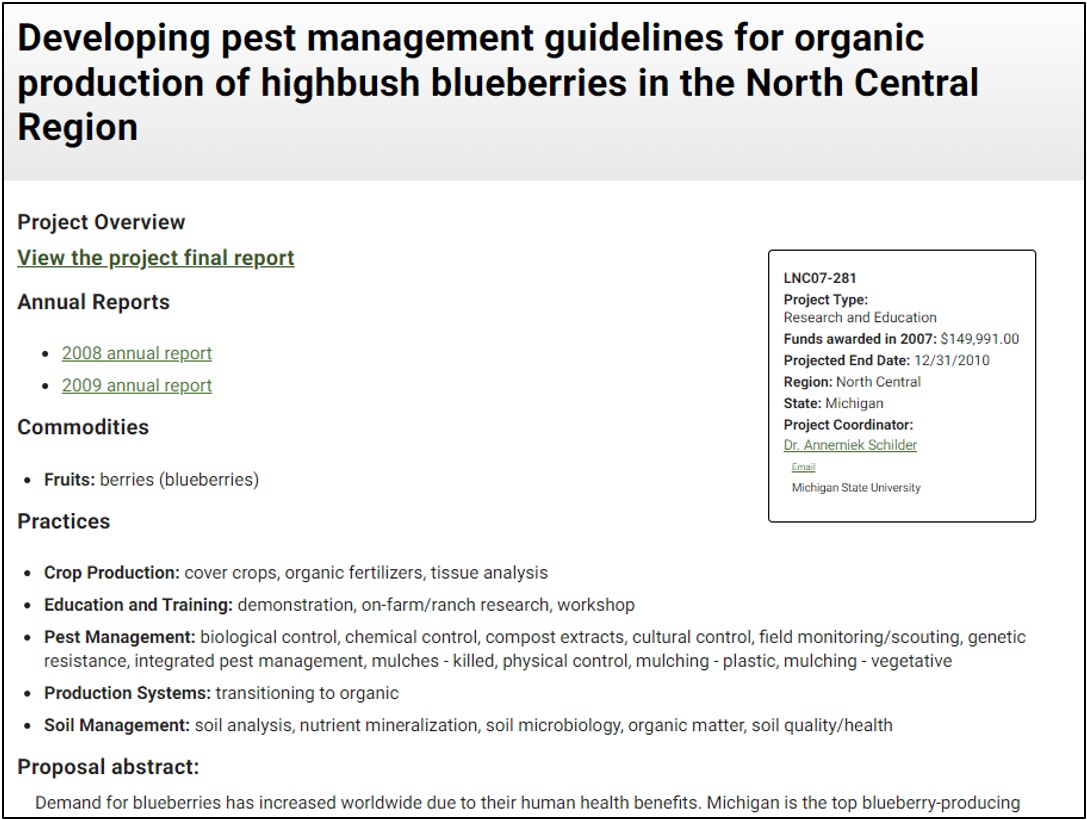
Or maybe you’re considering building a pizza oven on your farm and try a simple keyword search for “pizza” as shown below:
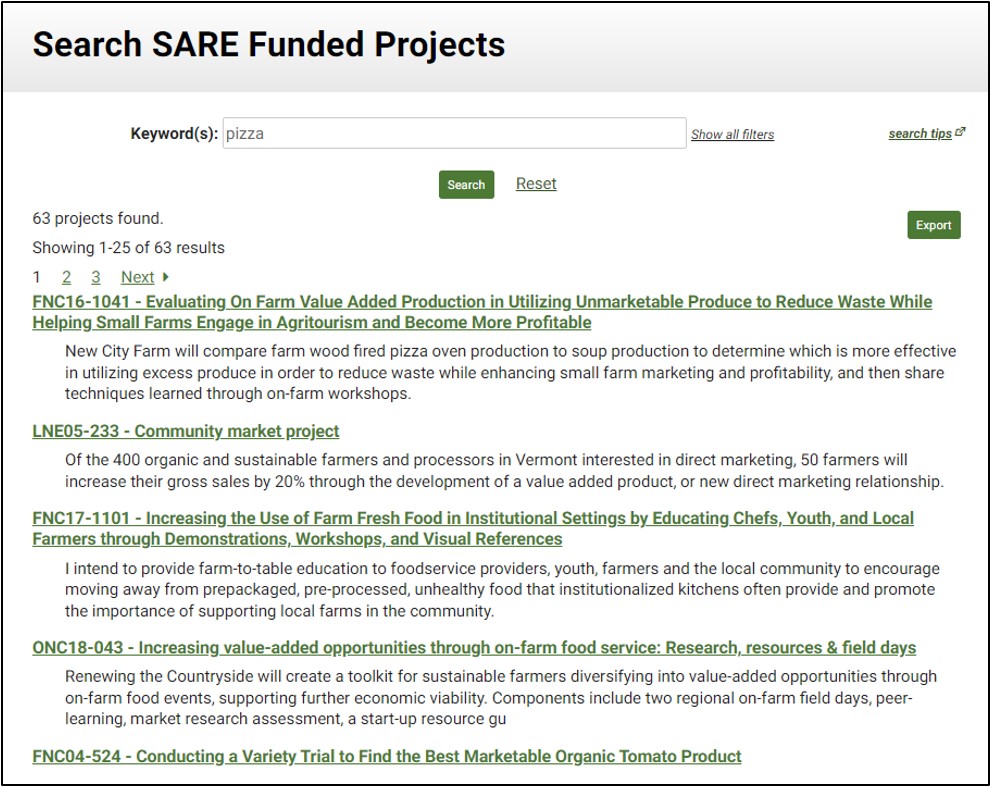
Clicking on the first result takes us to a project in Grand Rapids, Michigan, where you can watch a video or read the final report:
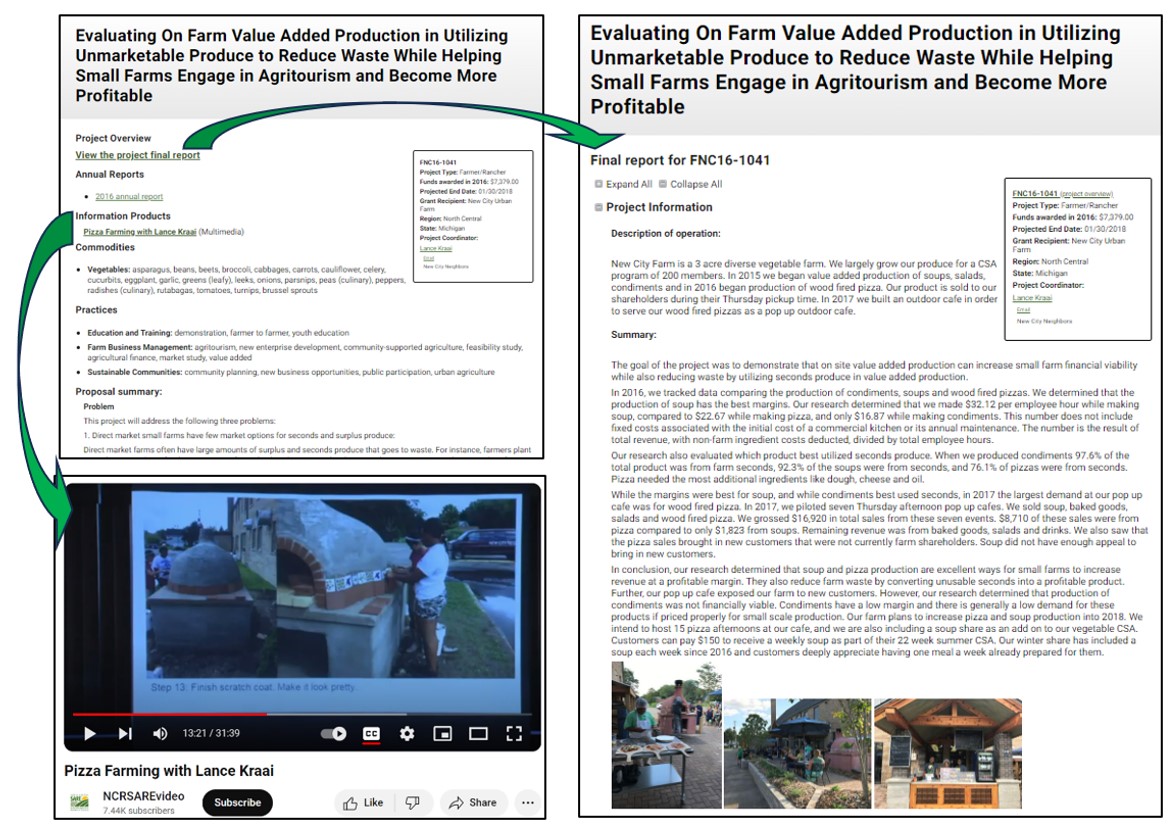
Or you may want to download a page of up to 25 results as a spreadsheet. Just click on the “Export” button at the top right of your search result to see, analyze and sort many similar projects with one click.
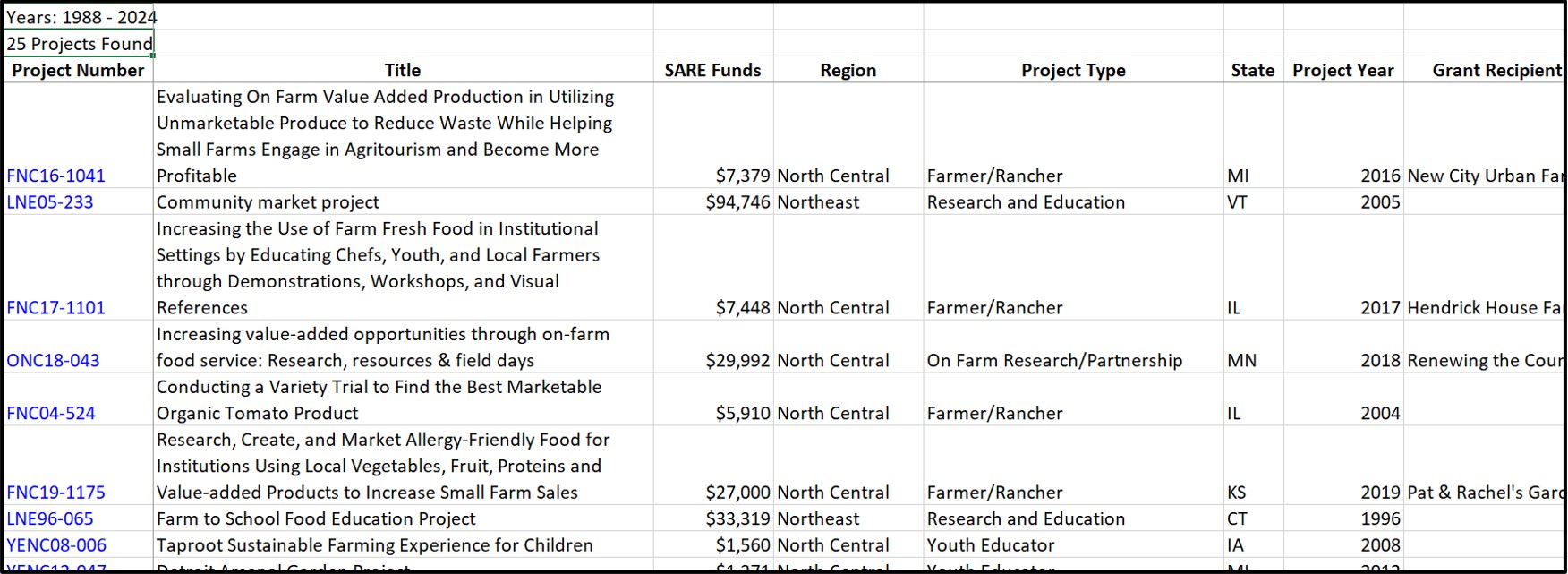
New map features Michigan’s SARE sustainable farming innovators
A new option for searching Michigan’s SARE projects is the Map of Michigan North Central SARE Grants . This map shows over 100 sustainable agriculture projects funded by SARE since 2016 and allows unique search options:
- Click on any icon or grant title in the side bar to see:
- The project title
- A link to the project summary and reports
- Funding amount (e.g. $6,000)
- North Central SARE grant type (e.g. Farmer Rancher, Youth Educator or Graduate Student)
- Zoom into a region and click on the icons to see who and what has been funded in your area.
- The icon color and sidebar show what year each grant was funded. You can click the checkboxes by year to see or hide layers if you just want to see grants from a certain time period.
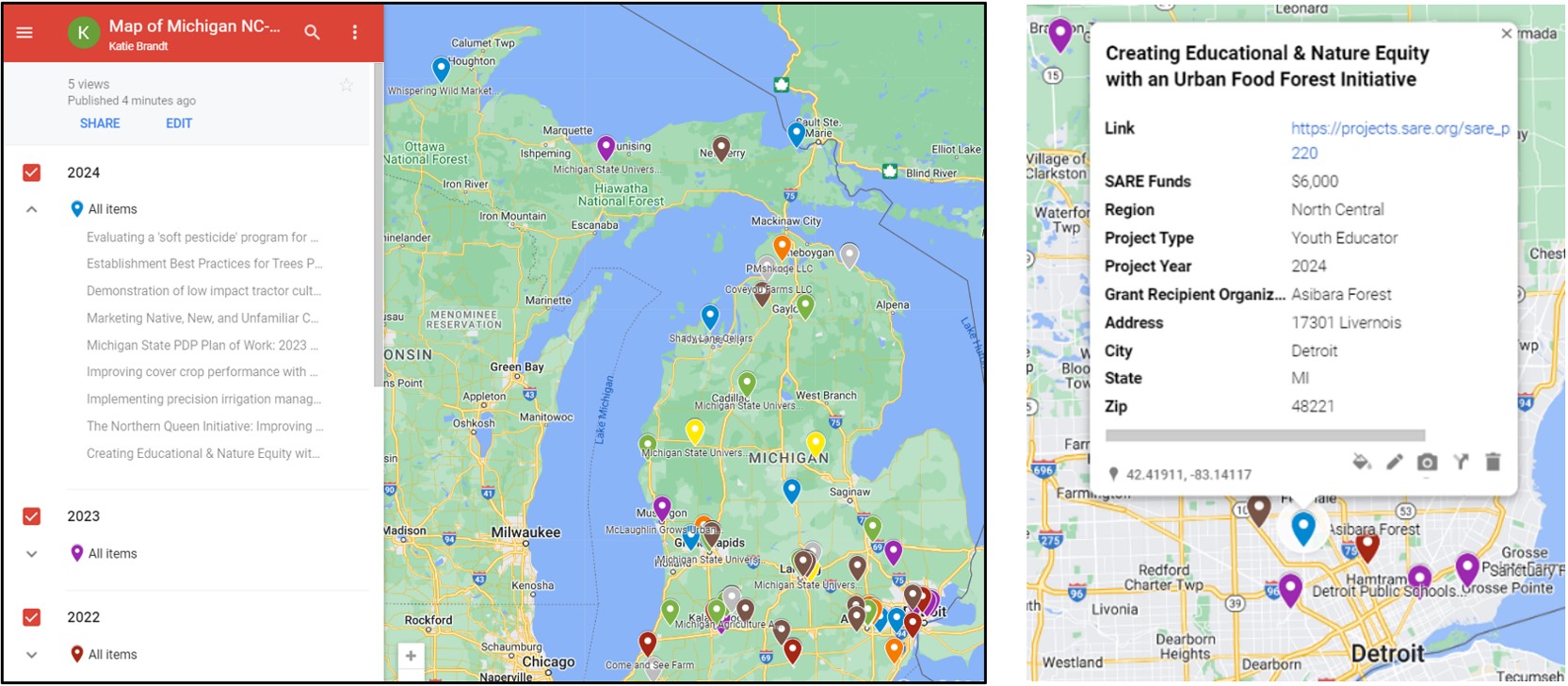
Please let us know if you have any questions or suggestions about the map, SARE grants or an innovative idea you would like to propose for SARE funding. You can contact state SARE coordinator Sarah Fronczak at [email protected] or the map designer Katie Brandt at [email protected] .
Sustainable Agriculture Research and Education (SARE)
SARE is a United States Department of Agriculture program that shares numerous resources for farmers and agriculture educators including books, bulletins, videos and more. For more information about SARE grants and resources, visit Michigan SARE or SARE .
North Central Region (NCR) SARE grants include Farmer Rancher Grants , Research and Education Grants , Youth Educator Grants other grant programs. You can find them all at NCR-SARE Grants . Michigan SARE grants include the Michigan SARE Travel Scholarship and two funding opportunities for people organizing sustainable agriculture workshops and conferences.
Michigan SARE Coordinator Sarah Fronczak, Tribal SARE Coordinator Emily Proctor and Katie Brandt from the Michigan State University Organic Farmer Training Program are glad to advise grant applicants, review proposals and support farmers, educators and students applying for NCR-SARE grants. Grant-writing support is available free of charge for all six North Central SARE grants and for Michigan SARE mini-grants. Email [email protected] or [email protected] with questions, to have a proposal reviewed or to set up a time to discuss your proposal idea. Tribal members and communities are encouraged to reach out to Emily Proctor at [email protected] for assistance with SARE proposals.
The program aims to support an inclusive mix of farmers and does not discriminate on the basis of race, color, national origin, religion, sex, gender identity (including gender expression), sexual orientation, disability, age, marital status, family/parental status, income derived from a public assistance program or political beliefs.
This article was published by Michigan State University Extension . For more information, visit https://extension.msu.edu . To have a digest of information delivered straight to your email inbox, visit https://extension.msu.edu/newsletters . To contact an expert in your area, visit https://extension.msu.edu/experts , or call 888-MSUE4MI (888-678-3464).
Did you find this article useful?
Haul manure? Check out the Michigan Manure Hauler Certification Program!
Check out the MSU Viticulture Program!
new - method size: 3 - Random key: 0, method: tagSpecific - key: 0
You Might Also Be Interested In
MSU researcher awarded five-year, $2.5 million grant to develop risk assessment training program
Published on October 13, 2020
MSU Product Center helps Michigan food entrepreneurs survive and thrive throughout pandemic
Published on August 31, 2021
Protecting Michigan’s environment and wildlife through the Conservation Reserve Enhancement Program
Published on September 1, 2021
MSU Extension to undertake three-year, $7 million vaccination education effort
Published on August 17, 2021
MSU to study precision livestock farming adoption trends in U.S. swine industry
Published on March 15, 2021
MSU research team receives USDA grant to evaluate effectiveness, cost of new blueberry pest management strategies
Published on February 19, 2021
- agriculture
- agriculture and environment
- beginning farmer
- christmas trees
- community food systems
- community gardening
- farm & farmers markets
- farm management
- field crops
- floriculture
- food policy
- fruit & nuts
- master gardener
- msu extension
- organic agriculture
- tribal extension
- tribal sare
- agriculture,
- agriculture and environment,
- beginning farmer,
- christmas trees,
- community food systems,
- community gardening,
- farm & farmers markets,
- farm management,
- field crops,
- floriculture,
- food policy,
- fruit & nuts,
- master gardener,
- msu extension,
- organic agriculture,
- tribal extension,
- tribal sare,
- vegetables,
- Restaurants
- Development
- The Way It Was
- Film & TV
- Things to Do
- Fitness & Wellness
- Hour Media Events
- Partner Events
- Photography Request Form
- Best of Detroit
- Excellence in Care
- Faces of Detroit
- Top Docs Event
- Give Detroit
- Great Places
- Canna-Business
- Women Who Move Detroit
- Parents’ Guide to College
- Professionals
- Digital Edition
- Purchase Single Issues
- Newsletters
- Where to Find
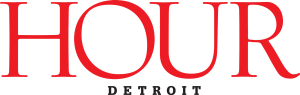
Metro Detroit’s Top Food and Beverage News for April 2024

Jackson Pollock-inspired restaurant coming to The Village of Rochester Hills
In the space once occupied by Noodles & Co. arrives a brand-new restaurant — The Jackson — from hospitality veteran Michael Mauro and award-winning chef Justin Vaiciunas, who has worked in notable kitchens such as those at the Detroit Athletic Club and Birmingham’s 220 Merrill.
The Jackson will feature a bar and dining area inspired by the paintings of pioneering abstract expressionist Jackson Pollock. The interior will be designed by Pophouse , a Detroit-based design firm owned by Jennifer Gilbert, the wife of multibillionaire Dan Gilbert.
The menu will feature local, seasonal ingredients. Visit thejacksonrestaurant.com for more.
Lincoln Yard now open in Birmingham
This long-awaited new restaurant from Union Joints opened this past March in Birmingham’s Rail District.
It features cocktails and serves up comforting foods like rotisserie-roasted and smoked meats as well as wood-fired pizza and vegetarian options. Union Joints is the restaurant group behind the Clarkston Union , Union Woodshop (also in Clarkston), and Vinsetta Garage in Berkley, among many more local eateries.
It’s located at 2159 E. Lincoln St. in a repurposed garage on the former site of the Birmingham Public Schools bus yard, across the street from tennis courts, the Birmingham Racquet Club, Lincoln Skate Park, and Birmingham Ice Sports Arena.
“It’s lively and has the ethos of being able to have kids come over after a practice across the street and grab a pizza,” says Ann Stevenson, who co-owns Union Joints with her husband, Curt Catallo. “And I’m stoked about the cocktail list that we have. I like the plurality of who we’re serving.”
Find more information at eatlincolnyard.com .
Sexy Steak opens in Detroit’s Grand Army of the Republic Building

A new restaurant serving steaks, Italian fare, and seafood opened in the historic Grand Army of the Republic Building , located at Cass and Grand River avenues in downtown Detroit.
The concept features an oyster bar and two levels of dining with more than 200 seats. There’s also the option to pick out your own cut from a display case (lined with Himalayan salt) before it’s cooked.
Plus, above the restaurant, there are another two levels of spaces, dubbed Castle Hall, that can be rented out for events.
The castlelike structure is on the National Register of Historic Places . Many of the original elements were kept intact, and the renovated building features a memorial space with historical artifacts from the building’s history, which dates back over 120 years.
For more, visit sexysteakdetroit.com .
Albena’s chef-owner moves to The Dixboro Project
Starting April 1, Garrett Lipar joins Ann Arbor’s Dixboro Project as partner and executive chef.
In February, Garrett and his wife/business partner, Tiffany Lipar, announced that Albena , their high-end tasting menu concept in Detroit’s Siren Hotel , would close in March after six years of operation. Garrett Lipar was previously head chef at Torino in Ferndale.
Dixboro Project, which opened in 2021, is run by Ann Arbor restaurateur Sava Farah of The Pulpo Group ( Sava’s , Aventura ).
Housed inside a reclaimed barn, the restaurant has changed chefs more than once since its inception — it initially opened with Michelin-starred chef Louis Maldonado and, more recently, was helmed by chef Joseph VanWagner.
Learn more at thedixboroproject.com .
James Beard Foundation to announce finalists
Thirteen metro Detroit restaurants are semifinalists in the 2024 James Beard Awards, known as the “Oscars of food.” There are a couple of fresh faces: Alpino (best new restaurant) and John Yelinek of Ladder 4 Wine Bar (best chef: Great Lakes). The 2024 nominees were announced on Wednesday, April 3 .
The final James Beard Restaurant and Chef Awards Ceremony takes place Monday, June 10, at the Lyric Opera of Chicago. For updates, visit jamesbeard.org .
This story originally appeared in the April 2024 issue of Hour Detroit magazine. To read more, pick up a copy of Hour Detroit at a local retail outlet. Our digital edition will be available on April 5.
RELATED ARTICLES MORE FROM AUTHOR
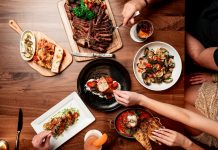
Fabio Viviani’s Adelina to Open near Campus Martius

James Beard Winner Alex Young Joins Lakeview Hills Country Club as Executive Chef

Buddy’s Pizza is Set to Open 23rd Location in Canton
Newsletters.
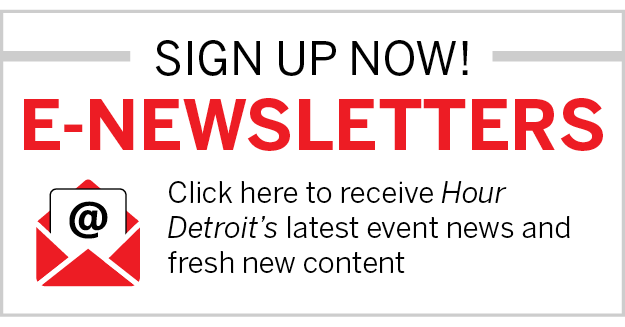
FROM OUR PARTNERS
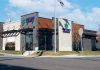
Unveiling Ferndale’s Premier Cannabis Haven: Nature’s Remedy
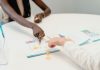
5 Things To Know About Brazilian Laser Hair Removal
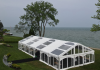
Transforming Ordinary Spaces into Extraordinary Venues
- Terms & Conditions
- Privacy & Cookies Policy

Moscow Sheremetyevo Airport SVO
Explore Today's Deals
Moscow Sheremetyevo Airport (SVO) Guide
Discover Moscow Sheremetyevo Airport (SVO), Russia's busiest hub, known for its striking Terminal D, inspired by the shapes of seagulls. This guide simplifies your travel through SVO, offering essential information for both newcomers and seasoned travelers. From flight details, terminal maps, to dining and shopping options, we ensure a seamless journey. Navigate SVO with confidence and make the most of your visit. For more insights into major airports, explore our Comprehensive Airport Guide .
Helpful Tips & Resources
Is Chashnikovo - Moskow Airport (SVO) airport a bit unfamiliar to you? Look no further than iFly.com for insightful tips and advice. Read on!
- Upon passing through security, go to the map of SVO's airport to navigate through the terminals, and view the restaurant, bars and shopping options as you head to your gate.
- Check here for info on lounges or clubs where you might be able to access via a day pass if you're not a member or flying in first class.
- At times, Chashnikovo - Moskow airport has changes to its airlines. Review the airlines that have scheduled flights from Moscow Sheremetyevo Airport SVO here.
- Waiting to pick up an arriving passenger? To see where the plane is, when its slated to land, and which terminal it will deplane, use the live flight tracker .
- Want to find the cheapest flight fare from SVO airport ? Compare multiple providers, check directly with airlines, and look into alternative airports.
Navigating SVO's Terminals
Moscow Sheremetyevo Airport has four main terminals: Terminal C, Terminal D, Terminal E, and Terminal F. Terminal D is the largest terminal with 22 gates, followed by Terminal E with 17 gates, Terminal F with 15 gates, and Terminal C with 9 gates. Major airlines such as Aeroflot, Delta, Air France, and KLM operate out of Terminal D, while Terminal E is mainly used by international airlines such as Emirates, Qatar Airways, and Turkish Airlines. Terminal C is used by low-cost carriers such as Pobeda and Terminal F is used for domestic flights.
When navigating the airport, it is important to pay attention to the signs and announcements as they will guide you to the correct terminal and gate. There are also shuttle buses that connect the terminals, which can be useful if you have a connecting flight in a different terminal.
Use our interactive airport map to locate food, services, and airline departure gates.
Connecting Between Terminals
Moscow Airport has four main terminals: Terminal C, Terminal D, Terminal E, and Terminal F. The terminals are connected by a free shuttle bus service that runs every 20 minutes, 24 hours a day. The shuttle bus stops are located outside each terminal and are clearly marked. The bus ride between terminals takes approximately 10-15 minutes, depending on traffic.
If you prefer to walk, there are pedestrian walkways connecting all terminals. The walkways are well-lit and clearly marked, and it takes approximately 10-15 minutes to walk between the terminals. However, if you have a lot of luggage or are in a hurry, taking the shuttle bus is recommended.
Picking Up and Dropping Off Passengers
At the airport, passengers can be picked up and dropped off at designated curbside areas outside of each terminal. However, there are strict restrictions on curbside parking, and vehicles cannot be left unattended. Drivers are advised to use the airport's short-term parking facilities if they need to leave their vehicle for an extended period of time.
For those waiting to pick up passengers, the airport provides cell-phone waiting areas near each terminal. These areas allow drivers to wait in their vehicles until their passengers are ready to be picked up, without having to circle the airport or park in a curbside area. It is important to note that vehicles cannot be left unattended in these areas, and drivers must remain in their vehicles at all times.
Overall, Moscow Sheremetyevo Airport provides convenient options for picking up and dropping off passengers, while ensuring the safety and efficiency of airport operations.
Parking Facilities & Options
If you're flying out of Moscow Airport, you'll find a variety of parking options available to you. The airport offers both short-term and long-term parking in garages and lots located near each of the airport's four terminals: Terminal C, Terminal D, Terminal E, and Terminal F.
If you're waiting to pick up arriving passengers, there are cell phone waiting areas available at the airport. These areas are free to use and allow you to wait in your car until your party has arrived and is ready to be picked up.
Handicapped parking is available at all of the airport's parking facilities, with designated spaces located near the entrances of each garage and lot. If you require assistance getting to or from your car, you can contact the airport's assistance service for help.
If you're looking for a more economical parking option, off-airport parking lots are available near the airport. These lots offer shuttle service to and from the airport, and can be a great way to save money on parking fees.
Get details about SVO parking options and rates, along with more economical off-airport parking here .
Transportation Options
SVO Airport offers a variety of ground transportation options for travelers. If you prefer to drive yourself, car rental facilities are available at the airport. Major providers include Avis, Europcar, Hertz, and Sixt. You can find rental car counters in Terminal D.
If you prefer to take a taxi, there are several options available. Taxis are located outside the arrivals area of each terminal. Fares are calculated based on distance and time, and can vary depending on traffic conditions. It is recommended to negotiate the fare with the driver before starting the trip.
Shared ride services such as Uber and Yandex.Taxi are also available at the airport. Pickup areas are located outside the arrivals area of each terminal. However, it is important to note that ride sharing services are not allowed to pick up passengers from the airport without a special permit.
Airport shuttles are available for passengers who need to travel between terminals or to nearby hotels. The shuttle service is free of charge and runs 24/7. Pickup areas are located outside the arrivals area of each terminal.
If you prefer to use public transportation , Aeroexpress trains provide direct service between the airport and Moscow city center. Trains run every 30 minutes and the journey takes approximately 35 minutes. Tickets can be purchased at the airport or online.
Airport Hotels
Traveling can be exhausting, but finding the perfect place to rest shouldn't be. Whether you're catching a flight early in the morning or arriving late at night, our selection of airport hotels ensures you're never far from the terminal. From budget-friendly options to luxury suites, there's something for every traveler. Check out our Sheremetyevo Airport Hotels for a comfortable and convenient stay.
Dining, Shopping & Enjoying the Airport
SVO offers a wide variety of dining and shopping options for travelers to enjoy. Terminal D is the main hub for food and beverage options, with popular choices such as Starbucks, Burger King, and Subway. For a taste of traditional Russian cuisine, travelers can head to the Russian Standard Restaurant located in Terminal D, which offers a range of dishes including borscht and beef stroganoff. Terminal E also offers a range of dining options, including a sushi bar and a pub-style restaurant.
When it comes to shopping, Terminal D and Terminal E offer the most options. Terminal D features a variety of duty-free shops selling everything from luxury clothing brands to traditional Russian souvenirs. Terminal E offers a range of shops selling electronics, books, and accessories. For those looking for a last-minute gift, the Sheremetyevo Airport Gift Shop in Terminal D is a great option.
The locations of all food, shops, and services at SVO Airport can be found using iFly's interactive map. For a complete list of restaurants, bars, shops, and news stands, see your options here .
Passenger Services
Moscow Sheremetyevo Airport is a modern airport that offers a wide range of passenger services to make your travel experience as comfortable as possible. The airport has four terminals: Terminal C, Terminal D, Terminal E, and Terminal F. Here is some helpful information about the passenger services at Moscow Airport:
For passengers who need to stay connected, the airport offers free Wi-Fi throughout all terminals. Lost and found services are available in each terminal, so if you misplace something during your travels, you can easily find it again.
If you are traveling with children, there are designated children's areas in each terminal where kids can play and burn off some energy before their flight. Additionally, special-needs services are available to assist passengers with disabilities or mobility issues. These services include wheelchair assistance, accessible restrooms, and designated parking spaces.
For your banking needs, there are ATMs and currency exchange offices located throughout the airport. If you require medical assistance, there is a medical center located in Terminal D that is staffed by trained medical professionals. The medical center is equipped to handle a variety of medical issues, from minor injuries to more serious medical emergencies.
Flight Status Info
As an air traveler, it's important to stay informed about the status of your flight at all times. At SVO, there are several ways to check your flight status and stay up-to-date on any changes.
One of the easiest ways to check your flight status is by using the in-airport flight displays (FIDS). These displays are located throughout the airport and provide real-time information on flight departures, delays, and gate information. Simply find the display that corresponds to your flight and check the status.
Another convenient way to stay informed is by using your airline's mobile phone app. Many airlines offer apps that allow you to receive alerts about departure delays, cancelations, or gate changes directly to your phone. You can also check your flight status and make changes to your reservation through the app.
In addition to mobile apps, airlines may also send updates via text, email, or push notifications. Make sure to check with your airline to see what options are available to you.
Passengers can check the status of their flight departure at SVO Airport by visiting the flight departures page on ifly.com, which provides real-time updates on flight departures, delays, and gate information.
Helpful Tips
When to arrive.
When traveling through Moscow Airport, it is recommended to arrive at least 2-3 hours before your scheduled departure time. This allows enough time for check-in, security screening, and navigating the airport. The busiest times to avoid are typically during peak travel seasons and holidays, as well as early morning and late evening flights.
Getting to the Airport
SVO is located approximately 29 kilometers northwest of Moscow city center. The best ways to get to the airport include taking a taxi, using public transportation such as the Aeroexpress train or bus, or driving and parking in one of the airport's parking lots. It is important to allow enough time for traffic and potential delays when choosing your mode of transportation.
Getting Oriented
When arriving at SVO Airport, it is recommended to use iFly.com's interactive airport maps to get oriented and navigate the airport. The maps provide detailed information on the airport's terminals, gates, restaurants, shops, and other amenities. Additionally, there are information desks located throughout the airport where you can ask for assistance and directions.
Avoiding Delays
To avoid delays at the airport, it is important to arrive early, have all necessary documents and identification ready, and follow all security protocols. Additionally, it is recommended to check the status of your flight before arriving at the airport and to stay updated on any potential delays or cancellations. You can also download the airport's mobile app for real-time flight information and updates.
Airport Facts & Stats
The airport is one of the busiest airports in Russia, serving millions of passengers annually. The airport has four terminals: Terminal C, Terminal D, Terminal E, and Terminal F.
On average, Moscow Sheremetyevo Airport handles around 40,000 passengers daily and operates approximately 500 daily flights. The airport serves more than 200 destinations worldwide, making it a popular hub for both domestic and international flights.
Some of the top airlines with the most daily flights at Moscow Airport include Aeroflot, Rossiya Airlines, and Nordwind Airlines. These airlines offer flights to various destinations across the globe.
The top five destinations served by SVO are Saint Petersburg, Simferopol, Sochi, Novosibirsk, and Yekaterinburg. These destinations are popular among both business and leisure travelers.
Several air carriers use SVO Airport as a hub, including Aeroflot, Rossiya Airlines, and Nordwind Airlines. These airlines offer connecting flights to various destinations across Russia and beyond.
Airport History
The airport, also known as Sheremetyevo International Airport, is one of the largest airports in Russia and serves as a hub for several major airlines. Here is a brief history of the airport:
- 1957 - Sheremetyevo Airport opens for civilian use
- 1960s - The airport undergoes major expansion and modernization
- 1970s - Sheremetyevo becomes the main international airport for Moscow
- 1980s - A new terminal is built to handle increased passenger traffic
- 1990s - The airport experiences a decline in passenger traffic due to economic instability in Russia
- 2000s - Sheremetyevo undergoes a major renovation and expansion, including the construction of a new terminal and runway
- 2010s - The airport continues to expand and modernize, with plans for a new terminal and additional runways in the works
Today, Sheremetyevo Airport is a modern and efficient airport that serves millions of passengers each year.
Airport Contact Information
For more information about Moscow Sheremetyevo Airport, please refer to the following contact details:
- IATA Code: SVO
- Airport Website
- Phone: +7 495 578-65-65
- Email: [email protected]
- Mailing Address: Moscow Sheremetyevo International Airport, Khimki, Moscow Oblast, 141400, Russian Federation
Note that the contact information provided here is subject to change by the airport operator(s).
Last updated: March 8, 2024
Find more help here for your journey through the airport
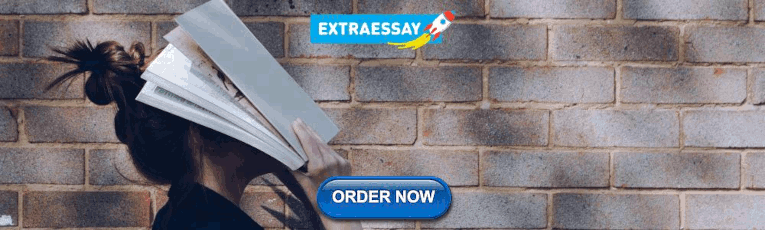
IMAGES
COMMENTS
Inside the Epic Challenge of Cannabis-Infused Drinks. by Jay Fitzgerald. The market for cannabis products has exploded as more states legalize marijuana. But the path to success is rife with complexity as a case study about the beverage company Cann by Ayelet Israeli illustrates. 15 Nov 2022.
During the month of March, our research team identified 4 new Food and Beverage facility construction projects with an estimated value of $100 million or more. The largest project is owned by PepsiCo Beverages, who is planning to invest $260 million for a 260,000 sf expansion and equipment upgrade at their processing facility in STONE MOUNTAIN, GA.
During the month of February, our research team identified 4 new Food and Beverage facility construction projects with an estimated value of $100 million or more. The largest project is owned by Great Lakes Cheese, who is planning to invest $500 million for the construction of a 486,000 sf processing facility in BELVIDERE, NY.
Whether you're seeking tactical or exploratory research; qualitative or quantitative methods; optimizing an existing product or launching something brand new, C+R Research is your ideal partner for food and beverage industry research. We immerse you in your consumers' world so that you can gain a deep understanding of their tastes ...
Abstract. This paper is woven with dynamic trends, pioneering innovations, and formidable challenges, explored in the study of food and beverage management: trends, innovations, and challenges ...
The Firm tracks North American planned industrial capital project activity; including facility expansions, new plant construction and significant equipment modernization projects. Research confirms 61 new projects in the Food and Beverage sector. The following are selected highlights on new Food and Beverage industry construction news.
Largest Planned Project. During the month of May, our research team identified 5 new Food and Beverage facility construction projects with an estimated value of $100 million or more. The largest project is owned by Hilmar Cheese Company, Inc., who is planning to invest $460 million for the construction of a processing facility in DODGE CITY, KS.
During the month of May, our research team identified 3 new Food and Beverage facility construction projects with an estimated value of $100 million or more. The largest project is owned by The Coca-Cola Company, who is planning to invest $650 million for the construction of a 745,000 sf processing facility on Tebor Rd. in WEBSTER, NY.
Journal of Hospitality and Tourism Management. The Future of Food and Beverage Management Research. This article offers an overview of the current state of food and beverage management research and some recommendations for the future development of the field. It begins from the premise that establishing such an overview requires an appreciation ...
During the month of January, our research team identified 7 new Food and Beverage facility construction projects with an estimated value of $100 million or more. The largest project is owned by SK Food Group, who is planning to invest $205 million for the construction of a 525,000 sf processing facility in CLEVELAND, TN.
Although the food and beverage industry plays a critical role in advancing food and nutrition science, industry-funded research is subject to intense scrutiny as a result of various perceived and real biases related to funding sources. ... Platforms such as the Center for Open Science's Open Science Framework enable investigators to share their ...
43. Contribute to research on food donation processes 44. Explore use of nanotechnology and other novel materials in food waste prevention, and potential public health benefits and risks 45. More broadly, CLF is interested in mentoring other student projects related to wasted food, so if you have an idea, feel free to reach out.
Predicting and understanding food perception and appreciation is one of the major challenges in food science. Accurate modeling of food flavor and appreciation could yield important opportunities ...
The Food Label Consumer Research is a three-phase project with both qualitative and quantitative phases. The overall project objectives are to: 1. Understand how consumers make food and beverage purchasing decisions using the NFP in the context of the entire package as well as other food and nutrition information sources and 2.
Food and beverage packaging using naturally occurring things up to modern technologies and its impact. June 2021; ... European Food Research and . Technology,2005:220(3-4):331-40. 33.
New Beverage development in the food industry can be achieved by introducing new strategies in the field of market. 1.Identify the target customers 2.Seasonal beverages 3.Originate a brand 4.Wrapping a product 5.Blogging a beverage... more. Download. by food research. 4.
Largest Planned Project. In March, the research team identified five new food and beverage facility construction projects with an estimated value of a minimum of $100 million. The largest project is by McCain Foods, who is planning to invest $600 million for the expansion and equipment upgrades on their processing facility in Coaldale, AB.
The food industry comprises a complex network of activities related to the. supply, consumption, and catering of food products and services. It plays a. significant role in the economic ...
Food & Drink Resources (FDR) hosts and facilitates qualitative and quantitative research projects for restaurants, food and beverage manufacturers, and other consumer packaged goods companies. Whatever your consumer research needs, you will find it's possible at FDR. With a large consumer test kitchen and commercial test kitchen with bar, you ...
Complete at a Research Lab. You can research Food and Drink Research Projects at a Research Lab. These can be found in your ship, outposts, and major settlements in the game like New Atlantis. Once you're at the Research Lab, you can do the following. Access the Research Lab terminal and select the " Food and Drink " category.
Search tool for successful SARE grants and project reports. Searching through projects funded by Sustainable Agriculture Research and Education Program (SARE) grants is simple and allows you to see ideas and results directly from sustainable farm innovators. Examples of grants that have been funded by SARE can help you to write a successful SARE grant and improve sustainability on your farm.
James Beard Foundation to announce finalists. Thirteen metro Detroit restaurants are semifinalists in the 2024 James Beard Awards, known as the "Oscars of food.". There are a couple of fresh faces: Alpino (best new restaurant) and John Yelinek of Ladder 4 Wine Bar (best chef: Great Lakes). The 2024 nominees were announced on Wednesday, April 3.
The Research Institute for Future Food (RiFood) at the Hong Kong Polytechnic University (PolyU) has developed a groundbreaking fat replacer called AkkMore™. This innovative formula has been used in the production of low-fat food products, including low-fat ice cream with a fat content of 3%. AkkMore™ is a fungus and plant-based supplement targeting obesity or prediabetes.
The analysis here is particularly based on the case of Khimki — a former Soviet off-limits 'satellite city' of Moscow and more recently a fast-growing area featuring many new retail, office ...
Find company research, competitor information, contact details & financial data for SFERA, OOO of Khimki, Moscow region. Get the latest business insights from Dun & Bradstreet. ... Specialty Food Retailers Grocery and Convenience Retailers Food and Beverage Retailers Retail Trade. Printer Friendly View Address: str. 6A etazh 2 kom. 72, ul ...
Find company research, competitor information, contact details & financial data for BEST PRICE LLC» of Khimki, Moscow region. Get the latest business insights from Dun & Bradstreet.
Terminal D is the main hub for food and beverage options, with popular choices such as Starbucks, Burger King, and Subway. For a taste of traditional Russian cuisine, travelers can head to the Russian Standard Restaurant located in Terminal D, which offers a range of dishes including borscht and beef stroganoff. Terminal E also offers a range ...