106 Artificial Intelligence Essay Topics & Samples
In a research paper or any other assignment about AI, there are many topics and questions to consider. To help you out, our experts have provided a list of 76 titles , along with artificial intelligence essay examples, for your consideration.
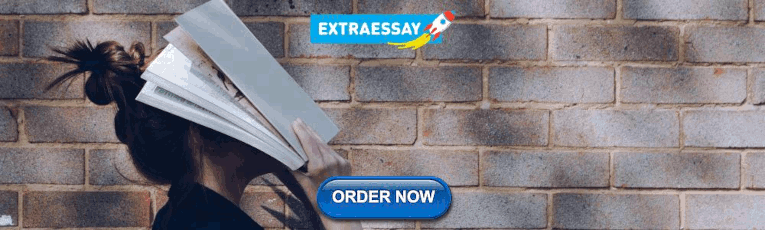
💾 Top 10 Artificial Intelligence Essay Topics
🏆 best essay topics on artificial intelligence, 🖱️ interesting artificial intelligence topics for essays, 🖥️ good ai essay titles, ❓ artificial intelligence research questions.
- AI and Human Intelligence.
- Computer Vision.
- Future of AI Technology.
- Machine Learning.
- AI in Daily Life.
- Impact of Deep Learning.
- Natural Language Processing.
- Threats in Robotics.
- Reinforcement Learning.
- Ethics of Artificial Intelligence.
- The Problem of Artificial Intelligence The introduction of new approaches to work and rest triggered the reconsideration of traditional values and promoted the growth of a certain style of life characterized by the mass use of innovations and their integration […]
- Artificial Intelligence: The Helper or the Threat? To conclude, artificial intelligence development is a problem that leaves nobody indifferent as it is closely associated with the future of the humanity.
- Artificial Intelligence: Positive or Negative Innovation? He argues that while humans will still be in charge of a few aspects of life in the near future, their control will be reduced due to the development of artificial intelligence.
- Artificial Intelligence Managing Human Life Although the above examples explain how humans can use AI to perform a wide range of tasks, it is necessary for stakeholders to control and manage the replication of human intelligence.
- Artificial Intelligence and Related Social Threats It may be expressed in a variety of ways, from peaceful attempts to attract attention to the issue to violent and criminal activities.
- Artificial Intelligence and Humans Co-Existence Some strategies to address these challenges exist; however, the strict maintenance of key areas under human control is the only valid solution to ensure people’s safety.
- Artificial Intelligence Reducing Costs in Hospitality Industry One of the factors that contribute to increased costs in the hospitality industry is the inability of management to cope with changing consumer demands.
- Application of Artificial Intelligence in Business The connection of AI and the business strategy of an organization is displayed through the ability to use its algorithm for achieving competitive advantage and maintaining it.
- Artificial Intelligence and Future of Sales It is assumed that one of the major factors that currently affect and will be affecting sales in the future is the artificial intelligence.
- Artificial Intelligence: Pros and Cons Artificial intelligence, or robots, one of the most scandalous and brilliant inventions of the XX century, causing people’s concern for the world safety, has become one of the leading branches of the modern science, which […]
- Artificial Intelligence and People-Focused Cities The aim of this research is to examine the relationship between the application of effective AI technologies to enhance urban planning approaches and the development of modern smart and people focused cities.
- What Progress Has Been Made With Artificial Intelligence? According to Dunjko and Briegel, AI contains a variety of fields and concepts, including the necessity to understand human capacities, abstract all the aspects of work, and realize similar aptitudes in machines.
- Artificial Intelligence: A Systems Approach That is to say, limitations on innovations should be applied to the degree to which robots and machine intelligence can be autonomous.
- Turing Test: Real and Artificial Intelligence The answers provided by the computer is consistent with that of human and the assessor can hardly guess whether the answer is from the machine or human.
- Saudi Arabia Information Technology: Artificial Intelligence The systems could therefore not fulfill the expectations of people who first thought that they would relieve managers and professionals of the need to make certain types of decisions.
- Artificial Intelligence and Video Games Development Therefore, in contrast to settings that have been designed for agents only, StarCraft and Blizzard can offer DeepMind an enormous amount of data gathered from playing time which teaches the AI to perform a set […]
- Artificial Intelligence System for Smart Energy Consumption The proposed energy consumption saver is an innovative technology that aims to increase the efficiency of energy consumption in residential buildings, production and commercial facilities, and other types of structures.
- Artificial Intelligence in Healthcare Delivery and Control Side Effects This report presents the status of AI in healthcare delivery and the motivations of deploying the technology in human services, information types analysed by AI frameworks, components that empower clinical outcomes and disease types.
- Artificial Intelligence for Diabetes: Project Experiences At the end of this reflective practice report, I plan to recognize my strengths and weaknesses in terms of team-working on the project about AI in diabetic retinopathy detection and want to determine my future […]
- Artificial Intelligence Company’s Economic Indicators On the other hand, it is vital to mention that if an artificial intelligence company has come of age and it is generally at the level of a large corporation, it can swiftly maneuver the […]
- Apple’s Company Announcement on Artificial Intelligence This development in Apple’s software is a reflection of the social construction of technology theory based on how the needs of the user impact how technological development is oriented.
- Artificial Intelligence Threat to Human Activities Despite the fictional and speculative nature of the majority of implications connected to the supposed threat that the artificial intelligence poses to mankind and the resulting low credibility ascribed to all such suggestions, at least […]
- Artificial Intelligence and the Associated Threats Artificial Intelligence, commonly referred to as AI refers to a branch of computer science that deals with the establishment of computer software and programs aimed at the change of the way many people carry out […]
- Artificial Intelligence Advantages and Disadvantages In the early years of the field, AI scientists sort to fully duplicate the human capacities of thought and language on the digital computer.
- Artificial Intelligence in the Documentary “Transcendent Man” The artificial intelligence is becoming a threat to the existence of humanity since these machines are slowly but steadily replacing the roles of mankind in all spheres of life.
- Non Experts: Artificial Intelligence Regardless of speed and the complexity of mathematical problems that they can solve, all that they do is to accept some input and generate desired output. This system is akin to that found in a […]
- Autonomous Controller Robotics: The Future of Robots The middle level is the Coordination level which interfaces the actions of the top and lower level s in the architecture.
- Exploring the Impact of Artificial Intelligence: Prediction versus Judgment
- Maintaining Project Networks in Automated Artificial Intelligence Planning
- The Effects Artificial Intelligence Has Had On Society And On Business
- What Role Will Artificial Intelligence Actually Play in Human Affairs in the Next Few Decades?
- How Artificial Intelligence and Machine Learning Can Impact Market Design
- The Use of Artificial Intelligence in Today’s Technological Devices
- The Correlation of Artificial Intelligence and the Invention of Modern Day Computers and Programming Languages
- How Artificial Intelligence Will Affect Social Media Monitoring
- Artificial Intelligence and Neural Network: The Future of Computing and Computer Programming
- The Foundations and History of Artificial Intelligence
- Comment on Prediction, Judgment, and Complexity: A Theory of Decision Making and Artificial Intelligence
- Artificial Intelligence And Law: A Review Of The Role Of Correctness In The General Data Protection Regulation Framework
- Artificial Intelligence: Compared To The Human Mind’s Capacity For Reasoning And Learning
- A Comparison Between Two Predictive Models of Artificial Intelligence
- Artificial Intelligence as a Positive and Negative Factor in Global Risk
- Search Applications, Java, and Complexity of Symbolic Artificial Intelligence
- Integrating Ethical Values and Economic Value to Steer Progress in Artificial Intelligence
- Computational Modeling of an Economy Using Elements of Artificial Intelligence
- The growth of Artificial Intelligence and its relevance to The Matrix
- The Impact of Artificial Intelligence on Innovation
- The Potential Negative Impact of Artificial Intelligence in the Future
- An Overview of the Principles of Artificial Intelligence and the Views of Noam Chomsky
- How Artificial Intelligence Technology can be Used to Treat Diabetes
- Artificial Intelligence and the UK Labour Market: Questions, Methods and a Call for a Systematic Approach to Information Gathering
- An Overview of Artificial Intelligence and Its Future Disadvantage to Our Modern Society
- Artificial Intelligence and Machine Learning Applications in Smart Production: Progress, Trends, and Directions
- Comparing the Different Views of John Searle and Alan Turing on the Debate on Artificial Intelligence (AI)
- A Comparison of Cognitive Ability and Information Processing in Artificial Intelligence
- Improvisation Of Unmanned Aerial Vehicles Using Artificial Intelligence
- Artificial Intelligence and Its Implications for Income Distribution and Unemployment
- The Application of Artificial Intelligence in Real-Time Strategy Games
- Advancement in Technology Can Someday Bring Artificial Intelligence to Reality
- Artificial Intelligence Based Congestion Control Mechanism Via Bayesian Networks Under Opportunistic
- Artificial Intelligence Is Lost in the Woods a Conscious Mind Will Never Be Built Out of Software
- An Analysis of the Concept of Artificial Intelligence in Relation to Business
- The Different Issues Concerning the Creation of Artificial Intelligence
- Traditional Philosophical Problems Surrounding Induction Relating to Artificial Intelligence
- The Importance of Singularity and Artificial Intelligence to People
- Man Machine Collaboration And The Rise Of Artificial Intelligence
- What Are the Ethical Challenges for Companies Working In Artificial Intelligence?
- Will Artificial Intelligence Have a Progressive or Retrogressive Impact on Our Society?
- Why Won’t Artificial Intelligence Dominate the Future?
- Will Artificial Intelligence Overpower Human Beings?
- How Does Artificial Intelligence Affect the Retail Industry?
- What Can Artificial Intelligence Offer Coral Reef Managers?
- Will Artificial Intelligence Replace Computational Economists Any Time Soon?
- How Can Artificial Intelligence and Machine Learning Impact Market Design?
- Can Artificial Intelligence Lead to a More Sustainable Society?
- Will Artificial Intelligence Replace Humans at Job?
- How Can Artificial Intelligence Help Us?
- How Will Artificial Intelligence Affect the Job Industry in the Future?
- Can Artificial Intelligence Become Smarter Than Humans?
- How Would You Define Artificial Intelligence?
- Should Artificial Intelligence Have Human Rights?
- How Do Artificial Intelligence and Siri Operate in Regards to Language?
- What Are the Impacts of Artificial Intelligence on the Creative Industries?
- How Can Artificial Intelligence Help Us Understand Human Creativity?
- When Will Artificial Intelligence Defeat Human Intelligence?
- How Can Artificial Intelligence Technology Be Used to Treat Diabetes?
- Will Artificial Intelligence Replace Mankind?
- How Will Artificial Intelligence Affect Social Media Monitoring?
- Can Artificial Intelligence Change the Way in Which Companies Recruit, Train, Develop, and Manage Human Resources in Workplace?
- How Does Mary Shelley’s Depiction Show the Threats of Artificial Intelligence?
- Why Must Artificial Intelligence Be Regulated?
- Will Artificial Intelligence Devices Become Human’s Best Friend?
- Does Artificial Intelligence Exist?
- Can Artificial Intelligence Be Dangerous?
- Why Do We Need Artificial Intelligence?
- Chicago (A-D)
- Chicago (N-B)
IvyPanda. (2023, November 8). 106 Artificial Intelligence Essay Topics & Samples. https://ivypanda.com/essays/topic/artificial-intelligence-essay-examples/
"106 Artificial Intelligence Essay Topics & Samples." IvyPanda , 8 Nov. 2023, ivypanda.com/essays/topic/artificial-intelligence-essay-examples/.
IvyPanda . (2023) '106 Artificial Intelligence Essay Topics & Samples'. 8 November.
IvyPanda . 2023. "106 Artificial Intelligence Essay Topics & Samples." November 8, 2023. https://ivypanda.com/essays/topic/artificial-intelligence-essay-examples/.
1. IvyPanda . "106 Artificial Intelligence Essay Topics & Samples." November 8, 2023. https://ivypanda.com/essays/topic/artificial-intelligence-essay-examples/.
Bibliography
IvyPanda . "106 Artificial Intelligence Essay Topics & Samples." November 8, 2023. https://ivypanda.com/essays/topic/artificial-intelligence-essay-examples/.
- Intelligence Essay Ideas
- Machine Learning Ideas
- Computers Essay Ideas
- Robots Questions
- Innovation Titles
- Technology Essay Ideas
- Business Intelligence Research Topics
- Agile Project Management Research Topics
- Data Management Essay Ideas
- Software Engineering Topics
- Digital Transformation Topics
- Functionalism Titles
- Cognitive Development Essay Ideas
- Information Management Paper Topics
The present and future of AI
Finale doshi-velez on how ai is shaping our lives and how we can shape ai.
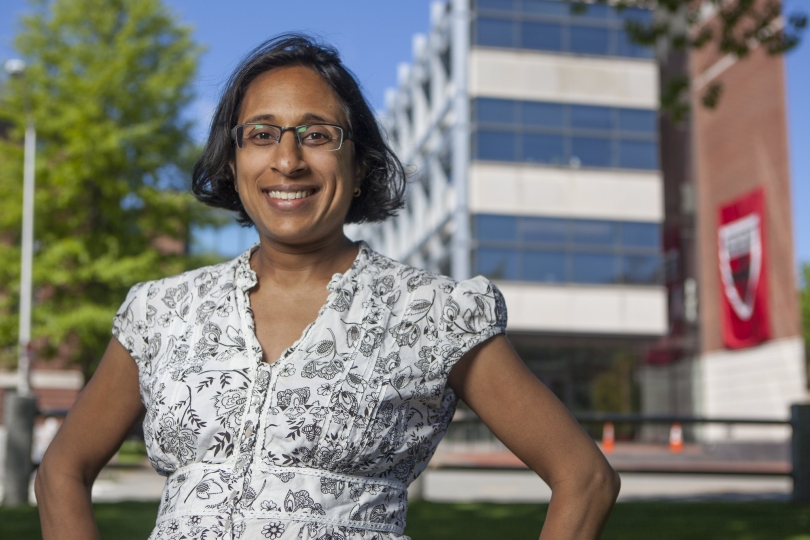
Finale Doshi-Velez, the John L. Loeb Professor of Engineering and Applied Sciences. (Photo courtesy of Eliza Grinnell/Harvard SEAS)
How has artificial intelligence changed and shaped our world over the last five years? How will AI continue to impact our lives in the coming years? Those were the questions addressed in the most recent report from the One Hundred Year Study on Artificial Intelligence (AI100), an ongoing project hosted at Stanford University, that will study the status of AI technology and its impacts on the world over the next 100 years.
The 2021 report is the second in a series that will be released every five years until 2116. Titled “Gathering Strength, Gathering Storms,” the report explores the various ways AI is increasingly touching people’s lives in settings that range from movie recommendations and voice assistants to autonomous driving and automated medical diagnoses .
Barbara Grosz , the Higgins Research Professor of Natural Sciences at the Harvard John A. Paulson School of Engineering and Applied Sciences (SEAS) is a member of the standing committee overseeing the AI100 project and Finale Doshi-Velez , Gordon McKay Professor of Computer Science, is part of the panel of interdisciplinary researchers who wrote this year’s report.
We spoke with Doshi-Velez about the report, what it says about the role AI is currently playing in our lives, and how it will change in the future.
Q: Let's start with a snapshot: What is the current state of AI and its potential?
Doshi-Velez: Some of the biggest changes in the last five years have been how well AIs now perform in large data regimes on specific types of tasks. We've seen [DeepMind’s] AlphaZero become the best Go player entirely through self-play, and everyday uses of AI such as grammar checks and autocomplete, automatic personal photo organization and search, and speech recognition become commonplace for large numbers of people.
In terms of potential, I'm most excited about AIs that might augment and assist people. They can be used to drive insights in drug discovery, help with decision making such as identifying a menu of likely treatment options for patients, and provide basic assistance, such as lane keeping while driving or text-to-speech based on images from a phone for the visually impaired. In many situations, people and AIs have complementary strengths. I think we're getting closer to unlocking the potential of people and AI teams.
There's a much greater recognition that we should not be waiting for AI tools to become mainstream before making sure they are ethical.
Q: Over the course of 100 years, these reports will tell the story of AI and its evolving role in society. Even though there have only been two reports, what's the story so far?
There's actually a lot of change even in five years. The first report is fairly rosy. For example, it mentions how algorithmic risk assessments may mitigate the human biases of judges. The second has a much more mixed view. I think this comes from the fact that as AI tools have come into the mainstream — both in higher stakes and everyday settings — we are appropriately much less willing to tolerate flaws, especially discriminatory ones. There's also been questions of information and disinformation control as people get their news, social media, and entertainment via searches and rankings personalized to them. So, there's a much greater recognition that we should not be waiting for AI tools to become mainstream before making sure they are ethical.
Q: What is the responsibility of institutes of higher education in preparing students and the next generation of computer scientists for the future of AI and its impact on society?
First, I'll say that the need to understand the basics of AI and data science starts much earlier than higher education! Children are being exposed to AIs as soon as they click on videos on YouTube or browse photo albums. They need to understand aspects of AI such as how their actions affect future recommendations.
But for computer science students in college, I think a key thing that future engineers need to realize is when to demand input and how to talk across disciplinary boundaries to get at often difficult-to-quantify notions of safety, equity, fairness, etc. I'm really excited that Harvard has the Embedded EthiCS program to provide some of this education. Of course, this is an addition to standard good engineering practices like building robust models, validating them, and so forth, which is all a bit harder with AI.
I think a key thing that future engineers need to realize is when to demand input and how to talk across disciplinary boundaries to get at often difficult-to-quantify notions of safety, equity, fairness, etc.
Q: Your work focuses on machine learning with applications to healthcare, which is also an area of focus of this report. What is the state of AI in healthcare?
A lot of AI in healthcare has been on the business end, used for optimizing billing, scheduling surgeries, that sort of thing. When it comes to AI for better patient care, which is what we usually think about, there are few legal, regulatory, and financial incentives to do so, and many disincentives. Still, there's been slow but steady integration of AI-based tools, often in the form of risk scoring and alert systems.
In the near future, two applications that I'm really excited about are triage in low-resource settings — having AIs do initial reads of pathology slides, for example, if there are not enough pathologists, or get an initial check of whether a mole looks suspicious — and ways in which AIs can help identify promising treatment options for discussion with a clinician team and patient.
Q: Any predictions for the next report?
I'll be keen to see where currently nascent AI regulation initiatives have gotten to. Accountability is such a difficult question in AI, it's tricky to nurture both innovation and basic protections. Perhaps the most important innovation will be in approaches for AI accountability.
Topics: AI / Machine Learning , Computer Science
Cutting-edge science delivered direct to your inbox.
Join the Harvard SEAS mailing list.
Scientist Profiles
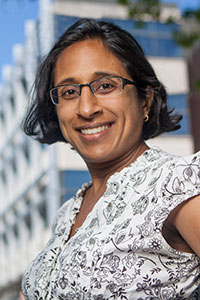
Finale Doshi-Velez
Herchel Smith Professor of Computer Science
Press Contact
Leah Burrows | 617-496-1351 | [email protected]
Related News
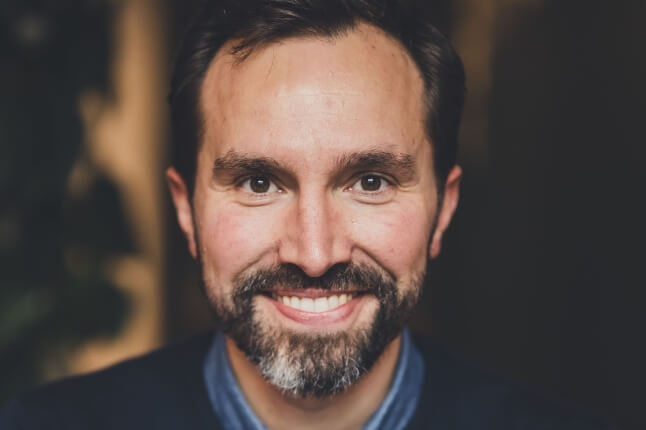
Alumni profile: Jacomo Corbo, Ph.D. '08
Racing into the future of machine learning
AI / Machine Learning , Computer Science
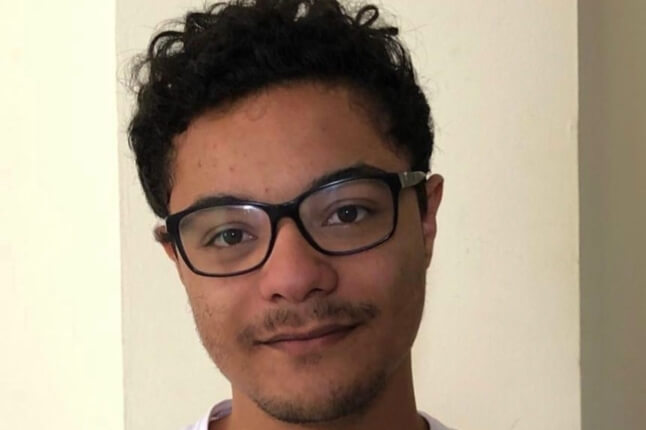
Ph.D. student Monteiro Paes named Apple Scholar in AI/ML
Monteiro Paes studies fairness and arbitrariness in machine learning models
AI / Machine Learning , Applied Mathematics , Awards , Graduate Student Profile
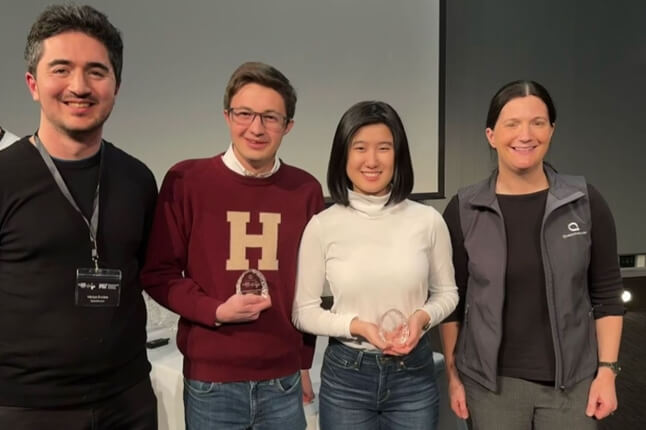
A new phase for Harvard Quantum Computing Club
SEAS students place second at MIT quantum hackathon
Computer Science , Quantum Engineering , Undergraduate Student Profile

- AI vs. Machine Learning
- AI in FinTech
- AI in Health Care
- AI in Manufacturing
- AI in Robotics
- AI in Video Games
- Faculty and Leadership
- Apply External link: open_in_new
Home / Artificial Intelligence (AI) vs. Machine Learning
Artificial Intelligence (AI) vs. Machine Learning Artificial Intelligence (AI) vs. Machine Learning Artificial Intelligence (AI) vs. Machine Learning
Artificial intelligence (AI) and machine learning are often used interchangeably, but machine learning is a subset of the broader category of AI.
Put in context, artificial intelligence refers to the general ability of computers to emulate human thought and perform tasks in real-world environments, while machine learning refers to the technologies and algorithms that enable systems to identify patterns, make decisions, and improve themselves through experience and data.
Computer programmers and software developers enable computers to analyze data and solve problems — essentially, they create artificial intelligence systems — by applying tools such as:
- machine learning
- deep learning
- neural networks
- computer vision
- natural language processing
Below is a breakdown of the differences between artificial intelligence and machine learning as well as how they are being applied in organizations large and small today.
What Is Artificial Intelligence?
Artificial Intelligence is the field of developing computers and robots that are capable of behaving in ways that both mimic and go beyond human capabilities. AI-enabled programs can analyze and contextualize data to provide information or automatically trigger actions without human interference.
Today, artificial intelligence is at the heart of many technologies we use, including smart devices and voice assistants such as Siri on Apple devices. Companies are incorporating techniques such as natural language processing and computer vision — the ability for computers to use human language and interpret images — to automate tasks, accelerate decision making, and enable customer conversations with chatbots.
What Is Machine Learning?
Machine learning is a pathway to artificial intelligence. This subcategory of AI uses algorithms to automatically learn insights and recognize patterns from data, applying that learning to make increasingly better decisions.
By studying and experimenting with machine learning, programmers test the limits of how much they can improve the perception, cognition, and action of a computer system.
Deep learning, an advanced method of machine learning, goes a step further. Deep learning models use large neural networks — networks that function like a human brain to logically analyze data — to learn complex patterns and make predictions independent of human input.
How Companies Use AI and Machine Learning
To be successful in nearly any industry, organizations must be able to transform their data into actionable insight. Artificial Intelligence and machine learning give organizations the advantage of automating a variety of manual processes involving data and decision making.
By incorporating AI and machine learning into their systems and strategic plans, leaders can understand and act on data-driven insights with greater speed and efficiency.

AI in the Manufacturing Industry
Efficiency is key to the success of an organization in the manufacturing industry. Artificial intelligence can help manufacturing leaders automate their business processes by applying data analytics and machine learning to applications such as the following:
- Identifying equipment errors before malfunctions occur, using the internet of things (IoT), analytics, and machine learning
- Using an AI application on a device, located within a factory, that monitors a production machine and predicts when to perform maintenance, so it doesn’t fail mid-shift
- Studying HVAC energy consumption patterns and using machine learning to adjust to optimal energy saving and comfort level

AI and Machine Learning in Banking
Data privacy and security are especially critical within the banking industry. Financial services leaders can keep customer data secure while increasing efficiencies using AI and machine learning in several ways:
- Using machine learning to detect and prevent fraud and cybersecurity attacks
- Integrating biometrics and computer vision to quickly authenticate user identities and process documents
- Incorporating smart technologies such as chatbots and voice assistants to automate basic customer service functions

AI Applications in Health Care
The health care field uses huge amounts of data and increasingly relies on informatics and analytics to provide accurate, efficient health services. AI tools can help improve patient outcomes, save time, and even help providers avoid burnout by:
- Analyzing data from users’ electronic health records through machine learning to provide clinical decision support and automated insights
- Integrating an AI system that predicts the outcomes of hospital visits to prevent readmissions and shorten the time patients are kept in hospitals
- Capturing and recording provider-patient interactions in exams or telehealth appointments using natural-language understanding
Learn more about how AI is changing the world of health care.
Integrate AI and Machine Learning into Your Company
The online Artificial Intelligence executive certificate program, offered through the Fu Foundation School of Engineering and Applied Science at Columbia University, prepares you with the skills and insights to drive AI strategy and adoption across your organization.
With courses that address algorithms, machine learning, data privacy, robotics, and other AI topics, this non-credit program is designed for forward-thinking team leaders and technically proficient professionals who want to gain a deeper understanding of the applications of AI. You can complete the program in 18 months while continuing to work.
Request Information
- Español – América Latina
- Português – Brasil
Artificial intelligence (AI) vs. machine learning (ML)
You might hear people use artificial intelligence (AI) and machine learning (ML) interchangeably, especially when discussing big data, predictive analytics, and other digital transformation topics. The confusion is understandable as artificial intelligence and machine learning are closely related. However, these trending technologies differ in several ways, including scope, applications, and more.
Increasingly AI and ML products have proliferated as businesses use them to process and analyze immense volumes of data, drive better decision-making, generate recommendations and insights in real time, and create accurate forecasts and predictions.
So, what exactly is the difference when it comes to ML vs. AI, how are ML and AI connected, and what do these terms mean in practice for organizations today?
We’ll break down AI vs. ML and explore how these two innovative concepts are related and what makes them different from each other.
What is artificial intelligence?
Artificial intelligence is a broad field, which refers to the use of technologies to build machines and computers that have the ability to mimic cognitive functions associated with human intelligence, such as being able to see, understand, and respond to spoken or written language, analyze data, make recommendations, and more.
Although artificial intelligence is often thought of as a system in itself, it is a set of technologies implemented in a system to enable it to reason, learn, and act to solve a complex problem.
What is machine learning?
Machine learning is a subset of artificial intelligence that automatically enables a machine or system to learn and improve from experience. Instead of explicit programming, machine learning uses algorithms to analyze large amounts of data, learn from the insights, and then make informed decisions.
Machine learning algorithms improve performance over time as they are trained—exposed to more data. Machine learning models are the output, or what the program learns from running an algorithm on training data. The more data used, the better the model will get.
How are AI and ML connected?
While AI and ML are not quite the same thing, they are closely connected. The simplest way to understand how AI and ML relate to each other is:
- AI is the broader concept of enabling a machine or system to sense, reason, act, or adapt like a human
- ML is an application of AI that allows machines to extract knowledge from data and learn from it autonomously
One helpful way to remember the difference between machine learning and artificial intelligence is to imagine them as umbrella categories. Artificial intelligence is the overarching term that covers a wide variety of specific approaches and algorithms. Machine learning sits under that umbrella, but so do other major subfields, such as deep learning, robotics, expert systems, and natural language processing .
Differences between AI and ML
Now that you understand how they are connected, what is the main difference between ai and ml .
While artificial intelligence encompasses the idea of a machine that can mimic human intelligence, machine learning does not. Machine learning aims to teach a machine how to perform a specific task and provide accurate results by identifying patterns.
Let’s say you ask your Google Nest device, “How long is my commute today?” In this case, you ask a machine a question and receive an answer about the estimated time it will take you to drive to your office. Here, the overall goal is for the device to perform a task successfully—a task that you would generally have to do yourself in a real-world environment (for example, research your commute time).
In the context of this example, the goal of using ML in the overall system is not to enable it to perform a task. For instance, you might train algorithms to analyze live transit and traffic data to forecast the volume and density of traffic flow. However, the scope is limited to identifying patterns, how accurate the prediction was, and learning from the data to maximize performance for that specific task.
Artificial intelligence
- AI allows a machine to simulate human intelligence to solve problems
- The goal is to develop an intelligent system that can perform complex tasks
- We build systems that can solve complex tasks like a human
- AI has a wide scope of applications
- AI uses technologies in a system so that it mimics human decision-making
- AI works with all types of data: structured, semi-structured, and unstructured
- AI systems use logic and decision trees to learn, reason, and self-correct
Machine learning
- ML allows a machine to learn autonomously from past data
- The goal is to build machines that can learn from data to increase the accuracy of the output
- We train machines with data to perform specific tasks and deliver accurate results
- Machine learning has a limited scope of applications
- ML uses self-learning algorithms to produce predictive models
- ML can only use structured and semi-structured data
- ML systems rely on statistical models to learn and can self-correct when provided with new data
Benefits of using AI and ML together
AI and ML bring powerful benefits to organizations of all shapes and sizes, with new possibilities constantly emerging. In particular, as the amount of data grows in size and complexity, automated and intelligent systems are becoming vital to helping companies automate tasks, unlock value, and generate actionable insights to achieve better outcomes.
Here are some of the business benefits of using artificial intelligence and machine learning:
Wider data ranges
Analyzing and activating a wider range of unstructured and structured data sources.
Faster decision-making
Improving data integrity, accelerating data processing, and reducing human error for more informed, faster decision-making.
Increasing operational efficiency and reducing costs.
Analytic integration
Empowering employees by integrating predictive analytics and insights into business reporting and applications.
Applications of AI and ML
Artificial intelligence and machine learning can be applied in many ways, allowing organizations to automate repetitive or manual processes that help drive informed decision-making..
Companies across industries are using AI and ML in various ways to transform how they work and do business. Incorporating AI and ML capabilities into their strategies and systems helps organizations rethink how they use their data and available resources, drive productivity and efficiency, enhance data-driven decision-making through predictive analytics, and improve customer and employee experiences.
Here are some of the most common applications of AI and ML:
Healthcare and life sciences
Patient health record analysis and insights, outcome forecasting and modeling, accelerated drug development, augmented diagnostics, patient monitoring, and information extraction from clinical notes.
Manufacturing
Production machine monitoring, predictive maintenance, IoT analytics, and operational efficiency.
Ecommerce and retail
I nventory and supply chain optimization, demand forecasting, visual search, personalized offers and experiences, and recommendation engines.
Financial services
Risk assessment and analysis, fraud detection, automated trading, and service processing optimization.
Telecommunications
Intelligent networks and network optimization, predictive maintenance, business process automation, upgrade planning, and capacity forecasting.
Solve your business challenges with Google Cloud
Related products and services.
Google Cloud offers a wide range of AI and ML tools to help your teams focus on the valuable work that matters most. Designed and built with the best of Google’s research and technology, our products and services are helping organizations transform and solve their most challenging real-world problems.
Take the next step
Start building on Google Cloud with $300 in free credits and 20+ always free products.
Start your next project, explore interactive tutorials, and manage your account.
- Need help getting started? Contact sales
- Work with a trusted partner Find a partner
- Continue browsing See all products
- Get tips & best practices See tutorials
Advertisement
Machine Learning: Algorithms, Real-World Applications and Research Directions
- Review Article
- Published: 22 March 2021
- Volume 2 , article number 160 , ( 2021 )
Cite this article
- Iqbal H. Sarker ORCID: orcid.org/0000-0003-1740-5517 1 , 2
458k Accesses
1293 Citations
21 Altmetric
Explore all metrics
In the current age of the Fourth Industrial Revolution (4 IR or Industry 4.0), the digital world has a wealth of data, such as Internet of Things (IoT) data, cybersecurity data, mobile data, business data, social media data, health data, etc. To intelligently analyze these data and develop the corresponding smart and automated applications, the knowledge of artificial intelligence (AI), particularly, machine learning (ML) is the key. Various types of machine learning algorithms such as supervised, unsupervised, semi-supervised, and reinforcement learning exist in the area. Besides, the deep learning , which is part of a broader family of machine learning methods, can intelligently analyze the data on a large scale. In this paper, we present a comprehensive view on these machine learning algorithms that can be applied to enhance the intelligence and the capabilities of an application. Thus, this study’s key contribution is explaining the principles of different machine learning techniques and their applicability in various real-world application domains, such as cybersecurity systems, smart cities, healthcare, e-commerce, agriculture, and many more. We also highlight the challenges and potential research directions based on our study. Overall, this paper aims to serve as a reference point for both academia and industry professionals as well as for decision-makers in various real-world situations and application areas, particularly from the technical point of view.
Similar content being viewed by others
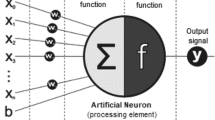
Deep Learning: A Comprehensive Overview on Techniques, Taxonomy, Applications and Research Directions
Iqbal H. Sarker
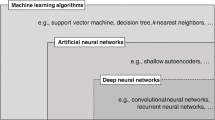
Machine learning and deep learning
Christian Janiesch, Patrick Zschech & Kai Heinrich
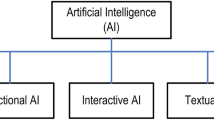
AI-Based Modeling: Techniques, Applications and Research Issues Towards Automation, Intelligent and Smart Systems
Avoid common mistakes on your manuscript.
Introduction
We live in the age of data, where everything around us is connected to a data source, and everything in our lives is digitally recorded [ 21 , 103 ]. For instance, the current electronic world has a wealth of various kinds of data, such as the Internet of Things (IoT) data, cybersecurity data, smart city data, business data, smartphone data, social media data, health data, COVID-19 data, and many more. The data can be structured, semi-structured, or unstructured, discussed briefly in Sect. “ Types of Real-World Data and Machine Learning Techniques ”, which is increasing day-by-day. Extracting insights from these data can be used to build various intelligent applications in the relevant domains. For instance, to build a data-driven automated and intelligent cybersecurity system, the relevant cybersecurity data can be used [ 105 ]; to build personalized context-aware smart mobile applications, the relevant mobile data can be used [ 103 ], and so on. Thus, the data management tools and techniques having the capability of extracting insights or useful knowledge from the data in a timely and intelligent way is urgently needed, on which the real-world applications are based.
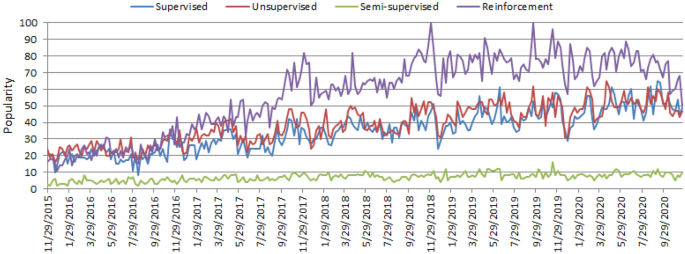
The worldwide popularity score of various types of ML algorithms (supervised, unsupervised, semi-supervised, and reinforcement) in a range of 0 (min) to 100 (max) over time where x-axis represents the timestamp information and y-axis represents the corresponding score
Artificial intelligence (AI), particularly, machine learning (ML) have grown rapidly in recent years in the context of data analysis and computing that typically allows the applications to function in an intelligent manner [ 95 ]. ML usually provides systems with the ability to learn and enhance from experience automatically without being specifically programmed and is generally referred to as the most popular latest technologies in the fourth industrial revolution (4 IR or Industry 4.0) [ 103 , 105 ]. “Industry 4.0” [ 114 ] is typically the ongoing automation of conventional manufacturing and industrial practices, including exploratory data processing, using new smart technologies such as machine learning automation. Thus, to intelligently analyze these data and to develop the corresponding real-world applications, machine learning algorithms is the key. The learning algorithms can be categorized into four major types, such as supervised, unsupervised, semi-supervised, and reinforcement learning in the area [ 75 ], discussed briefly in Sect. “ Types of Real-World Data and Machine Learning Techniques ”. The popularity of these approaches to learning is increasing day-by-day, which is shown in Fig. 1 , based on data collected from Google Trends [ 4 ] over the last five years. The x - axis of the figure indicates the specific dates and the corresponding popularity score within the range of \(0 \; (minimum)\) to \(100 \; (maximum)\) has been shown in y - axis . According to Fig. 1 , the popularity indication values for these learning types are low in 2015 and are increasing day by day. These statistics motivate us to study on machine learning in this paper, which can play an important role in the real-world through Industry 4.0 automation.
In general, the effectiveness and the efficiency of a machine learning solution depend on the nature and characteristics of data and the performance of the learning algorithms . In the area of machine learning algorithms, classification analysis, regression, data clustering, feature engineering and dimensionality reduction, association rule learning, or reinforcement learning techniques exist to effectively build data-driven systems [ 41 , 125 ]. Besides, deep learning originated from the artificial neural network that can be used to intelligently analyze data, which is known as part of a wider family of machine learning approaches [ 96 ]. Thus, selecting a proper learning algorithm that is suitable for the target application in a particular domain is challenging. The reason is that the purpose of different learning algorithms is different, even the outcome of different learning algorithms in a similar category may vary depending on the data characteristics [ 106 ]. Thus, it is important to understand the principles of various machine learning algorithms and their applicability to apply in various real-world application areas, such as IoT systems, cybersecurity services, business and recommendation systems, smart cities, healthcare and COVID-19, context-aware systems, sustainable agriculture, and many more that are explained briefly in Sect. “ Applications of Machine Learning ”.
Based on the importance and potentiality of “Machine Learning” to analyze the data mentioned above, in this paper, we provide a comprehensive view on various types of machine learning algorithms that can be applied to enhance the intelligence and the capabilities of an application. Thus, the key contribution of this study is explaining the principles and potentiality of different machine learning techniques, and their applicability in various real-world application areas mentioned earlier. The purpose of this paper is, therefore, to provide a basic guide for those academia and industry people who want to study, research, and develop data-driven automated and intelligent systems in the relevant areas based on machine learning techniques.
The key contributions of this paper are listed as follows:
To define the scope of our study by taking into account the nature and characteristics of various types of real-world data and the capabilities of various learning techniques.
To provide a comprehensive view on machine learning algorithms that can be applied to enhance the intelligence and capabilities of a data-driven application.
To discuss the applicability of machine learning-based solutions in various real-world application domains.
To highlight and summarize the potential research directions within the scope of our study for intelligent data analysis and services.
The rest of the paper is organized as follows. The next section presents the types of data and machine learning algorithms in a broader sense and defines the scope of our study. We briefly discuss and explain different machine learning algorithms in the subsequent section followed by which various real-world application areas based on machine learning algorithms are discussed and summarized. In the penultimate section, we highlight several research issues and potential future directions, and the final section concludes this paper.
Types of Real-World Data and Machine Learning Techniques
Machine learning algorithms typically consume and process data to learn the related patterns about individuals, business processes, transactions, events, and so on. In the following, we discuss various types of real-world data as well as categories of machine learning algorithms.
Types of Real-World Data
Usually, the availability of data is considered as the key to construct a machine learning model or data-driven real-world systems [ 103 , 105 ]. Data can be of various forms, such as structured, semi-structured, or unstructured [ 41 , 72 ]. Besides, the “metadata” is another type that typically represents data about the data. In the following, we briefly discuss these types of data.
Structured: It has a well-defined structure, conforms to a data model following a standard order, which is highly organized and easily accessed, and used by an entity or a computer program. In well-defined schemes, such as relational databases, structured data are typically stored, i.e., in a tabular format. For instance, names, dates, addresses, credit card numbers, stock information, geolocation, etc. are examples of structured data.
Unstructured: On the other hand, there is no pre-defined format or organization for unstructured data, making it much more difficult to capture, process, and analyze, mostly containing text and multimedia material. For example, sensor data, emails, blog entries, wikis, and word processing documents, PDF files, audio files, videos, images, presentations, web pages, and many other types of business documents can be considered as unstructured data.
Semi-structured: Semi-structured data are not stored in a relational database like the structured data mentioned above, but it does have certain organizational properties that make it easier to analyze. HTML, XML, JSON documents, NoSQL databases, etc., are some examples of semi-structured data.
Metadata: It is not the normal form of data, but “data about data”. The primary difference between “data” and “metadata” is that data are simply the material that can classify, measure, or even document something relative to an organization’s data properties. On the other hand, metadata describes the relevant data information, giving it more significance for data users. A basic example of a document’s metadata might be the author, file size, date generated by the document, keywords to define the document, etc.
In the area of machine learning and data science, researchers use various widely used datasets for different purposes. These are, for example, cybersecurity datasets such as NSL-KDD [ 119 ], UNSW-NB15 [ 76 ], ISCX’12 [ 1 ], CIC-DDoS2019 [ 2 ], Bot-IoT [ 59 ], etc., smartphone datasets such as phone call logs [ 84 , 101 ], SMS Log [ 29 ], mobile application usages logs [ 137 ] [ 117 ], mobile phone notification logs [ 73 ] etc., IoT data [ 16 , 57 , 62 ], agriculture and e-commerce data [ 120 , 138 ], health data such as heart disease [ 92 ], diabetes mellitus [ 83 , 134 ], COVID-19 [ 43 , 74 ], etc., and many more in various application domains. The data can be in different types discussed above, which may vary from application to application in the real world. To analyze such data in a particular problem domain, and to extract the insights or useful knowledge from the data for building the real-world intelligent applications, different types of machine learning techniques can be used according to their learning capabilities, which is discussed in the following.
Types of Machine Learning Techniques
Machine Learning algorithms are mainly divided into four categories: Supervised learning, Unsupervised learning, Semi-supervised learning, and Reinforcement learning [ 75 ], as shown in Fig. 2 . In the following, we briefly discuss each type of learning technique with the scope of their applicability to solve real-world problems.
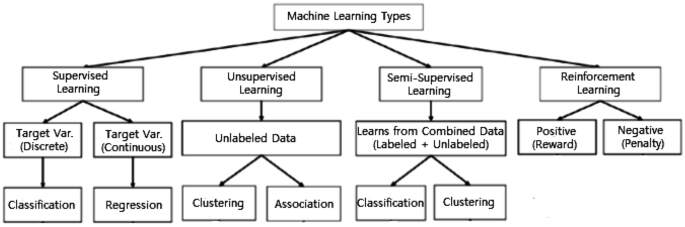
Various types of machine learning techniques
Supervised: Supervised learning is typically the task of machine learning to learn a function that maps an input to an output based on sample input-output pairs [ 41 ]. It uses labeled training data and a collection of training examples to infer a function. Supervised learning is carried out when certain goals are identified to be accomplished from a certain set of inputs [ 105 ], i.e., a task-driven approach . The most common supervised tasks are “classification” that separates the data, and “regression” that fits the data. For instance, predicting the class label or sentiment of a piece of text, like a tweet or a product review, i.e., text classification, is an example of supervised learning.
Unsupervised: Unsupervised learning analyzes unlabeled datasets without the need for human interference, i.e., a data-driven process [ 41 ]. This is widely used for extracting generative features, identifying meaningful trends and structures, groupings in results, and exploratory purposes. The most common unsupervised learning tasks are clustering, density estimation, feature learning, dimensionality reduction, finding association rules, anomaly detection, etc.
Semi-supervised: Semi-supervised learning can be defined as a hybridization of the above-mentioned supervised and unsupervised methods, as it operates on both labeled and unlabeled data [ 41 , 105 ]. Thus, it falls between learning “without supervision” and learning “with supervision”. In the real world, labeled data could be rare in several contexts, and unlabeled data are numerous, where semi-supervised learning is useful [ 75 ]. The ultimate goal of a semi-supervised learning model is to provide a better outcome for prediction than that produced using the labeled data alone from the model. Some application areas where semi-supervised learning is used include machine translation, fraud detection, labeling data and text classification.
Reinforcement: Reinforcement learning is a type of machine learning algorithm that enables software agents and machines to automatically evaluate the optimal behavior in a particular context or environment to improve its efficiency [ 52 ], i.e., an environment-driven approach . This type of learning is based on reward or penalty, and its ultimate goal is to use insights obtained from environmental activists to take action to increase the reward or minimize the risk [ 75 ]. It is a powerful tool for training AI models that can help increase automation or optimize the operational efficiency of sophisticated systems such as robotics, autonomous driving tasks, manufacturing and supply chain logistics, however, not preferable to use it for solving the basic or straightforward problems.
Thus, to build effective models in various application areas different types of machine learning techniques can play a significant role according to their learning capabilities, depending on the nature of the data discussed earlier, and the target outcome. In Table 1 , we summarize various types of machine learning techniques with examples. In the following, we provide a comprehensive view of machine learning algorithms that can be applied to enhance the intelligence and capabilities of a data-driven application.
Machine Learning Tasks and Algorithms
In this section, we discuss various machine learning algorithms that include classification analysis, regression analysis, data clustering, association rule learning, feature engineering for dimensionality reduction, as well as deep learning methods. A general structure of a machine learning-based predictive model has been shown in Fig. 3 , where the model is trained from historical data in phase 1 and the outcome is generated in phase 2 for the new test data.
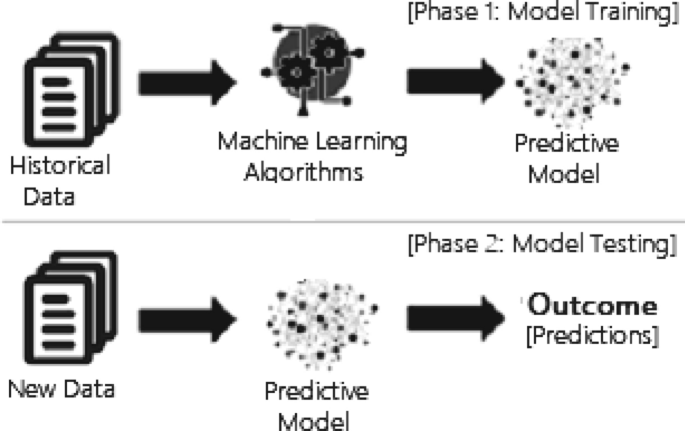
A general structure of a machine learning based predictive model considering both the training and testing phase
Classification Analysis
Classification is regarded as a supervised learning method in machine learning, referring to a problem of predictive modeling as well, where a class label is predicted for a given example [ 41 ]. Mathematically, it maps a function ( f ) from input variables ( X ) to output variables ( Y ) as target, label or categories. To predict the class of given data points, it can be carried out on structured or unstructured data. For example, spam detection such as “spam” and “not spam” in email service providers can be a classification problem. In the following, we summarize the common classification problems.
Binary classification: It refers to the classification tasks having two class labels such as “true and false” or “yes and no” [ 41 ]. In such binary classification tasks, one class could be the normal state, while the abnormal state could be another class. For instance, “cancer not detected” is the normal state of a task that involves a medical test, and “cancer detected” could be considered as the abnormal state. Similarly, “spam” and “not spam” in the above example of email service providers are considered as binary classification.
Multiclass classification: Traditionally, this refers to those classification tasks having more than two class labels [ 41 ]. The multiclass classification does not have the principle of normal and abnormal outcomes, unlike binary classification tasks. Instead, within a range of specified classes, examples are classified as belonging to one. For example, it can be a multiclass classification task to classify various types of network attacks in the NSL-KDD [ 119 ] dataset, where the attack categories are classified into four class labels, such as DoS (Denial of Service Attack), U2R (User to Root Attack), R2L (Root to Local Attack), and Probing Attack.
Multi-label classification: In machine learning, multi-label classification is an important consideration where an example is associated with several classes or labels. Thus, it is a generalization of multiclass classification, where the classes involved in the problem are hierarchically structured, and each example may simultaneously belong to more than one class in each hierarchical level, e.g., multi-level text classification. For instance, Google news can be presented under the categories of a “city name”, “technology”, or “latest news”, etc. Multi-label classification includes advanced machine learning algorithms that support predicting various mutually non-exclusive classes or labels, unlike traditional classification tasks where class labels are mutually exclusive [ 82 ].
Many classification algorithms have been proposed in the machine learning and data science literature [ 41 , 125 ]. In the following, we summarize the most common and popular methods that are used widely in various application areas.
Naive Bayes (NB): The naive Bayes algorithm is based on the Bayes’ theorem with the assumption of independence between each pair of features [ 51 ]. It works well and can be used for both binary and multi-class categories in many real-world situations, such as document or text classification, spam filtering, etc. To effectively classify the noisy instances in the data and to construct a robust prediction model, the NB classifier can be used [ 94 ]. The key benefit is that, compared to more sophisticated approaches, it needs a small amount of training data to estimate the necessary parameters and quickly [ 82 ]. However, its performance may affect due to its strong assumptions on features independence. Gaussian, Multinomial, Complement, Bernoulli, and Categorical are the common variants of NB classifier [ 82 ].
Linear Discriminant Analysis (LDA): Linear Discriminant Analysis (LDA) is a linear decision boundary classifier created by fitting class conditional densities to data and applying Bayes’ rule [ 51 , 82 ]. This method is also known as a generalization of Fisher’s linear discriminant, which projects a given dataset into a lower-dimensional space, i.e., a reduction of dimensionality that minimizes the complexity of the model or reduces the resulting model’s computational costs. The standard LDA model usually suits each class with a Gaussian density, assuming that all classes share the same covariance matrix [ 82 ]. LDA is closely related to ANOVA (analysis of variance) and regression analysis, which seek to express one dependent variable as a linear combination of other features or measurements.
Logistic regression (LR): Another common probabilistic based statistical model used to solve classification issues in machine learning is Logistic Regression (LR) [ 64 ]. Logistic regression typically uses a logistic function to estimate the probabilities, which is also referred to as the mathematically defined sigmoid function in Eq. 1 . It can overfit high-dimensional datasets and works well when the dataset can be separated linearly. The regularization (L1 and L2) techniques [ 82 ] can be used to avoid over-fitting in such scenarios. The assumption of linearity between the dependent and independent variables is considered as a major drawback of Logistic Regression. It can be used for both classification and regression problems, but it is more commonly used for classification.
K-nearest neighbors (KNN): K-Nearest Neighbors (KNN) [ 9 ] is an “instance-based learning” or non-generalizing learning, also known as a “lazy learning” algorithm. It does not focus on constructing a general internal model; instead, it stores all instances corresponding to training data in n -dimensional space. KNN uses data and classifies new data points based on similarity measures (e.g., Euclidean distance function) [ 82 ]. Classification is computed from a simple majority vote of the k nearest neighbors of each point. It is quite robust to noisy training data, and accuracy depends on the data quality. The biggest issue with KNN is to choose the optimal number of neighbors to be considered. KNN can be used both for classification as well as regression.
Support vector machine (SVM): In machine learning, another common technique that can be used for classification, regression, or other tasks is a support vector machine (SVM) [ 56 ]. In high- or infinite-dimensional space, a support vector machine constructs a hyper-plane or set of hyper-planes. Intuitively, the hyper-plane, which has the greatest distance from the nearest training data points in any class, achieves a strong separation since, in general, the greater the margin, the lower the classifier’s generalization error. It is effective in high-dimensional spaces and can behave differently based on different mathematical functions known as the kernel. Linear, polynomial, radial basis function (RBF), sigmoid, etc., are the popular kernel functions used in SVM classifier [ 82 ]. However, when the data set contains more noise, such as overlapping target classes, SVM does not perform well.
Decision tree (DT): Decision tree (DT) [ 88 ] is a well-known non-parametric supervised learning method. DT learning methods are used for both the classification and regression tasks [ 82 ]. ID3 [ 87 ], C4.5 [ 88 ], and CART [ 20 ] are well known for DT algorithms. Moreover, recently proposed BehavDT [ 100 ], and IntrudTree [ 97 ] by Sarker et al. are effective in the relevant application domains, such as user behavior analytics and cybersecurity analytics, respectively. By sorting down the tree from the root to some leaf nodes, as shown in Fig. 4 , DT classifies the instances. Instances are classified by checking the attribute defined by that node, starting at the root node of the tree, and then moving down the tree branch corresponding to the attribute value. For splitting, the most popular criteria are “gini” for the Gini impurity and “entropy” for the information gain that can be expressed mathematically as [ 82 ].
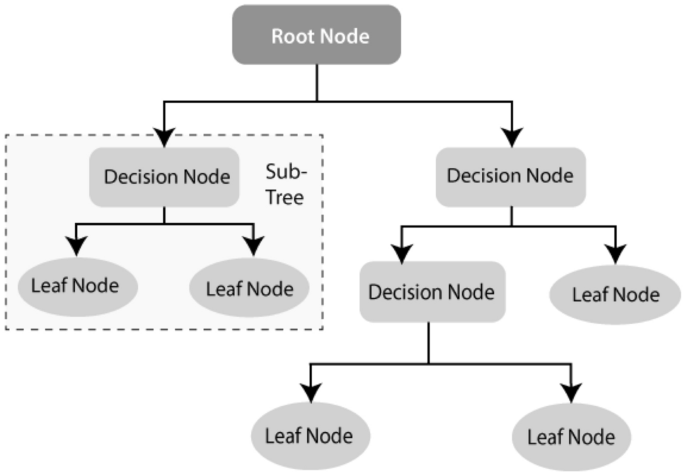
An example of a decision tree structure
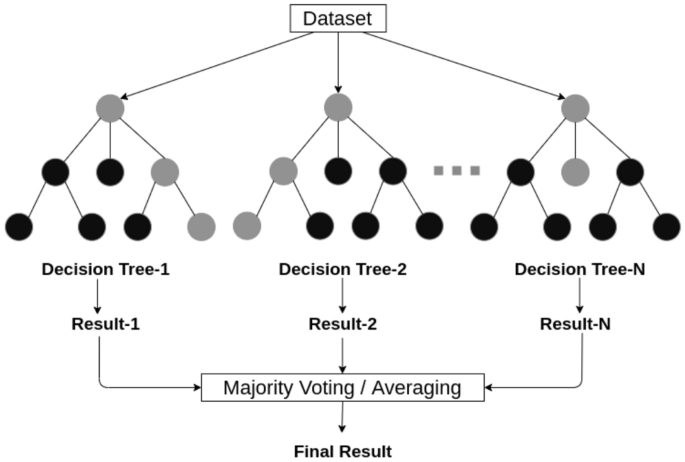
An example of a random forest structure considering multiple decision trees
Random forest (RF): A random forest classifier [ 19 ] is well known as an ensemble classification technique that is used in the field of machine learning and data science in various application areas. This method uses “parallel ensembling” which fits several decision tree classifiers in parallel, as shown in Fig. 5 , on different data set sub-samples and uses majority voting or averages for the outcome or final result. It thus minimizes the over-fitting problem and increases the prediction accuracy and control [ 82 ]. Therefore, the RF learning model with multiple decision trees is typically more accurate than a single decision tree based model [ 106 ]. To build a series of decision trees with controlled variation, it combines bootstrap aggregation (bagging) [ 18 ] and random feature selection [ 11 ]. It is adaptable to both classification and regression problems and fits well for both categorical and continuous values.
Adaptive Boosting (AdaBoost): Adaptive Boosting (AdaBoost) is an ensemble learning process that employs an iterative approach to improve poor classifiers by learning from their errors. This is developed by Yoav Freund et al. [ 35 ] and also known as “meta-learning”. Unlike the random forest that uses parallel ensembling, Adaboost uses “sequential ensembling”. It creates a powerful classifier by combining many poorly performing classifiers to obtain a good classifier of high accuracy. In that sense, AdaBoost is called an adaptive classifier by significantly improving the efficiency of the classifier, but in some instances, it can trigger overfits. AdaBoost is best used to boost the performance of decision trees, base estimator [ 82 ], on binary classification problems, however, is sensitive to noisy data and outliers.
Extreme gradient boosting (XGBoost): Gradient Boosting, like Random Forests [ 19 ] above, is an ensemble learning algorithm that generates a final model based on a series of individual models, typically decision trees. The gradient is used to minimize the loss function, similar to how neural networks [ 41 ] use gradient descent to optimize weights. Extreme Gradient Boosting (XGBoost) is a form of gradient boosting that takes more detailed approximations into account when determining the best model [ 82 ]. It computes second-order gradients of the loss function to minimize loss and advanced regularization (L1 and L2) [ 82 ], which reduces over-fitting, and improves model generalization and performance. XGBoost is fast to interpret and can handle large-sized datasets well.
Stochastic gradient descent (SGD): Stochastic gradient descent (SGD) [ 41 ] is an iterative method for optimizing an objective function with appropriate smoothness properties, where the word ‘stochastic’ refers to random probability. This reduces the computational burden, particularly in high-dimensional optimization problems, allowing for faster iterations in exchange for a lower convergence rate. A gradient is the slope of a function that calculates a variable’s degree of change in response to another variable’s changes. Mathematically, the Gradient Descent is a convex function whose output is a partial derivative of a set of its input parameters. Let, \(\alpha\) is the learning rate, and \(J_i\) is the training example cost of \(i \mathrm{th}\) , then Eq. ( 4 ) represents the stochastic gradient descent weight update method at the \(j^\mathrm{th}\) iteration. In large-scale and sparse machine learning, SGD has been successfully applied to problems often encountered in text classification and natural language processing [ 82 ]. However, SGD is sensitive to feature scaling and needs a range of hyperparameters, such as the regularization parameter and the number of iterations.
Rule-based classification : The term rule-based classification can be used to refer to any classification scheme that makes use of IF-THEN rules for class prediction. Several classification algorithms such as Zero-R [ 125 ], One-R [ 47 ], decision trees [ 87 , 88 ], DTNB [ 110 ], Ripple Down Rule learner (RIDOR) [ 125 ], Repeated Incremental Pruning to Produce Error Reduction (RIPPER) [ 126 ] exist with the ability of rule generation. The decision tree is one of the most common rule-based classification algorithms among these techniques because it has several advantages, such as being easier to interpret; the ability to handle high-dimensional data; simplicity and speed; good accuracy; and the capability to produce rules for human clear and understandable classification [ 127 ] [ 128 ]. The decision tree-based rules also provide significant accuracy in a prediction model for unseen test cases [ 106 ]. Since the rules are easily interpretable, these rule-based classifiers are often used to produce descriptive models that can describe a system including the entities and their relationships.
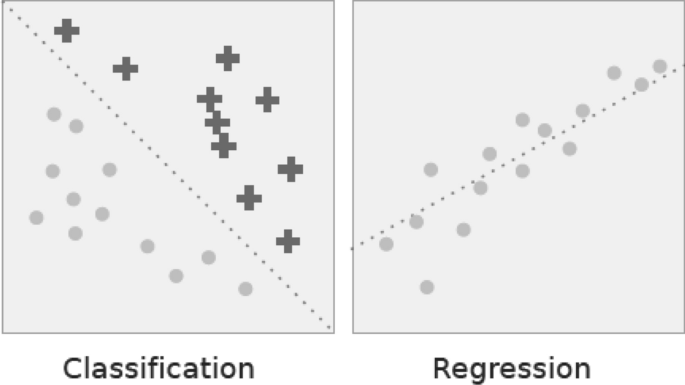
Classification vs. regression. In classification the dotted line represents a linear boundary that separates the two classes; in regression, the dotted line models the linear relationship between the two variables
Regression Analysis
Regression analysis includes several methods of machine learning that allow to predict a continuous ( y ) result variable based on the value of one or more ( x ) predictor variables [ 41 ]. The most significant distinction between classification and regression is that classification predicts distinct class labels, while regression facilitates the prediction of a continuous quantity. Figure 6 shows an example of how classification is different with regression models. Some overlaps are often found between the two types of machine learning algorithms. Regression models are now widely used in a variety of fields, including financial forecasting or prediction, cost estimation, trend analysis, marketing, time series estimation, drug response modeling, and many more. Some of the familiar types of regression algorithms are linear, polynomial, lasso and ridge regression, etc., which are explained briefly in the following.
Simple and multiple linear regression: This is one of the most popular ML modeling techniques as well as a well-known regression technique. In this technique, the dependent variable is continuous, the independent variable(s) can be continuous or discrete, and the form of the regression line is linear. Linear regression creates a relationship between the dependent variable ( Y ) and one or more independent variables ( X ) (also known as regression line) using the best fit straight line [ 41 ]. It is defined by the following equations:
where a is the intercept, b is the slope of the line, and e is the error term. This equation can be used to predict the value of the target variable based on the given predictor variable(s). Multiple linear regression is an extension of simple linear regression that allows two or more predictor variables to model a response variable, y, as a linear function [ 41 ] defined in Eq. 6 , whereas simple linear regression has only 1 independent variable, defined in Eq. 5 .
Polynomial regression: Polynomial regression is a form of regression analysis in which the relationship between the independent variable x and the dependent variable y is not linear, but is the polynomial degree of \(n^\mathrm{th}\) in x [ 82 ]. The equation for polynomial regression is also derived from linear regression (polynomial regression of degree 1) equation, which is defined as below:
Here, y is the predicted/target output, \(b_0, b_1,... b_n\) are the regression coefficients, x is an independent/ input variable. In simple words, we can say that if data are not distributed linearly, instead it is \(n^\mathrm{th}\) degree of polynomial then we use polynomial regression to get desired output.
LASSO and ridge regression: LASSO and Ridge regression are well known as powerful techniques which are typically used for building learning models in presence of a large number of features, due to their capability to preventing over-fitting and reducing the complexity of the model. The LASSO (least absolute shrinkage and selection operator) regression model uses L 1 regularization technique [ 82 ] that uses shrinkage, which penalizes “absolute value of magnitude of coefficients” ( L 1 penalty). As a result, LASSO appears to render coefficients to absolute zero. Thus, LASSO regression aims to find the subset of predictors that minimizes the prediction error for a quantitative response variable. On the other hand, ridge regression uses L 2 regularization [ 82 ], which is the “squared magnitude of coefficients” ( L 2 penalty). Thus, ridge regression forces the weights to be small but never sets the coefficient value to zero, and does a non-sparse solution. Overall, LASSO regression is useful to obtain a subset of predictors by eliminating less important features, and ridge regression is useful when a data set has “multicollinearity” which refers to the predictors that are correlated with other predictors.
Cluster Analysis
Cluster analysis, also known as clustering, is an unsupervised machine learning technique for identifying and grouping related data points in large datasets without concern for the specific outcome. It does grouping a collection of objects in such a way that objects in the same category, called a cluster, are in some sense more similar to each other than objects in other groups [ 41 ]. It is often used as a data analysis technique to discover interesting trends or patterns in data, e.g., groups of consumers based on their behavior. In a broad range of application areas, such as cybersecurity, e-commerce, mobile data processing, health analytics, user modeling and behavioral analytics, clustering can be used. In the following, we briefly discuss and summarize various types of clustering methods.
Partitioning methods: Based on the features and similarities in the data, this clustering approach categorizes the data into multiple groups or clusters. The data scientists or analysts typically determine the number of clusters either dynamically or statically depending on the nature of the target applications, to produce for the methods of clustering. The most common clustering algorithms based on partitioning methods are K-means [ 69 ], K-Mediods [ 80 ], CLARA [ 55 ] etc.
Density-based methods: To identify distinct groups or clusters, it uses the concept that a cluster in the data space is a contiguous region of high point density isolated from other such clusters by contiguous regions of low point density. Points that are not part of a cluster are considered as noise. The typical clustering algorithms based on density are DBSCAN [ 32 ], OPTICS [ 12 ] etc. The density-based methods typically struggle with clusters of similar density and high dimensionality data.
Hierarchical-based methods: Hierarchical clustering typically seeks to construct a hierarchy of clusters, i.e., the tree structure. Strategies for hierarchical clustering generally fall into two types: (i) Agglomerative—a “bottom-up” approach in which each observation begins in its cluster and pairs of clusters are combined as one, moves up the hierarchy, and (ii) Divisive—a “top-down” approach in which all observations begin in one cluster and splits are performed recursively, moves down the hierarchy, as shown in Fig 7 . Our earlier proposed BOTS technique, Sarker et al. [ 102 ] is an example of a hierarchical, particularly, bottom-up clustering algorithm.
Grid-based methods: To deal with massive datasets, grid-based clustering is especially suitable. To obtain clusters, the principle is first to summarize the dataset with a grid representation and then to combine grid cells. STING [ 122 ], CLIQUE [ 6 ], etc. are the standard algorithms of grid-based clustering.
Model-based methods: There are mainly two types of model-based clustering algorithms: one that uses statistical learning, and the other based on a method of neural network learning [ 130 ]. For instance, GMM [ 89 ] is an example of a statistical learning method, and SOM [ 22 ] [ 96 ] is an example of a neural network learning method.
Constraint-based methods: Constrained-based clustering is a semi-supervised approach to data clustering that uses constraints to incorporate domain knowledge. Application or user-oriented constraints are incorporated to perform the clustering. The typical algorithms of this kind of clustering are COP K-means [ 121 ], CMWK-Means [ 27 ], etc.
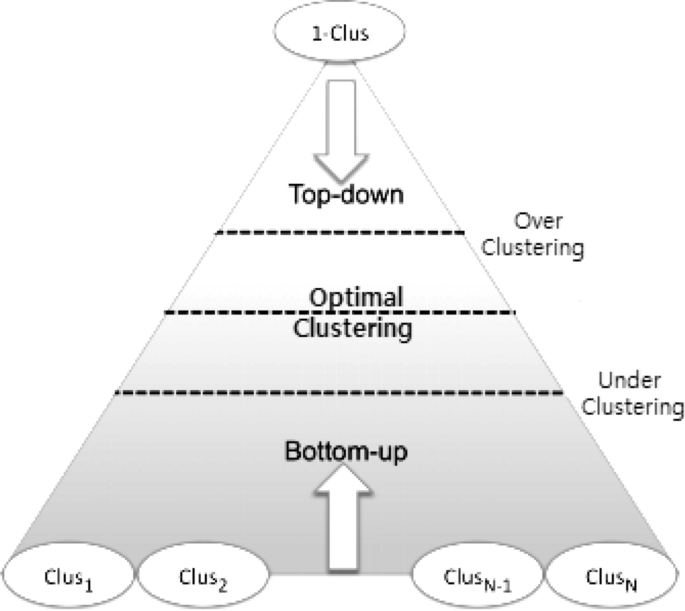
A graphical interpretation of the widely-used hierarchical clustering (Bottom-up and top-down) technique
Many clustering algorithms have been proposed with the ability to grouping data in machine learning and data science literature [ 41 , 125 ]. In the following, we summarize the popular methods that are used widely in various application areas.
K-means clustering: K-means clustering [ 69 ] is a fast, robust, and simple algorithm that provides reliable results when data sets are well-separated from each other. The data points are allocated to a cluster in this algorithm in such a way that the amount of the squared distance between the data points and the centroid is as small as possible. In other words, the K-means algorithm identifies the k number of centroids and then assigns each data point to the nearest cluster while keeping the centroids as small as possible. Since it begins with a random selection of cluster centers, the results can be inconsistent. Since extreme values can easily affect a mean, the K-means clustering algorithm is sensitive to outliers. K-medoids clustering [ 91 ] is a variant of K-means that is more robust to noises and outliers.
Mean-shift clustering: Mean-shift clustering [ 37 ] is a nonparametric clustering technique that does not require prior knowledge of the number of clusters or constraints on cluster shape. Mean-shift clustering aims to discover “blobs” in a smooth distribution or density of samples [ 82 ]. It is a centroid-based algorithm that works by updating centroid candidates to be the mean of the points in a given region. To form the final set of centroids, these candidates are filtered in a post-processing stage to remove near-duplicates. Cluster analysis in computer vision and image processing are examples of application domains. Mean Shift has the disadvantage of being computationally expensive. Moreover, in cases of high dimension, where the number of clusters shifts abruptly, the mean-shift algorithm does not work well.
DBSCAN: Density-based spatial clustering of applications with noise (DBSCAN) [ 32 ] is a base algorithm for density-based clustering which is widely used in data mining and machine learning. This is known as a non-parametric density-based clustering technique for separating high-density clusters from low-density clusters that are used in model building. DBSCAN’s main idea is that a point belongs to a cluster if it is close to many points from that cluster. It can find clusters of various shapes and sizes in a vast volume of data that is noisy and contains outliers. DBSCAN, unlike k-means, does not require a priori specification of the number of clusters in the data and can find arbitrarily shaped clusters. Although k-means is much faster than DBSCAN, it is efficient at finding high-density regions and outliers, i.e., is robust to outliers.
GMM clustering: Gaussian mixture models (GMMs) are often used for data clustering, which is a distribution-based clustering algorithm. A Gaussian mixture model is a probabilistic model in which all the data points are produced by a mixture of a finite number of Gaussian distributions with unknown parameters [ 82 ]. To find the Gaussian parameters for each cluster, an optimization algorithm called expectation-maximization (EM) [ 82 ] can be used. EM is an iterative method that uses a statistical model to estimate the parameters. In contrast to k-means, Gaussian mixture models account for uncertainty and return the likelihood that a data point belongs to one of the k clusters. GMM clustering is more robust than k-means and works well even with non-linear data distributions.
Agglomerative hierarchical clustering: The most common method of hierarchical clustering used to group objects in clusters based on their similarity is agglomerative clustering. This technique uses a bottom-up approach, where each object is first treated as a singleton cluster by the algorithm. Following that, pairs of clusters are merged one by one until all clusters have been merged into a single large cluster containing all objects. The result is a dendrogram, which is a tree-based representation of the elements. Single linkage [ 115 ], Complete linkage [ 116 ], BOTS [ 102 ] etc. are some examples of such techniques. The main advantage of agglomerative hierarchical clustering over k-means is that the tree-structure hierarchy generated by agglomerative clustering is more informative than the unstructured collection of flat clusters returned by k-means, which can help to make better decisions in the relevant application areas.
Dimensionality Reduction and Feature Learning
In machine learning and data science, high-dimensional data processing is a challenging task for both researchers and application developers. Thus, dimensionality reduction which is an unsupervised learning technique, is important because it leads to better human interpretations, lower computational costs, and avoids overfitting and redundancy by simplifying models. Both the process of feature selection and feature extraction can be used for dimensionality reduction. The primary distinction between the selection and extraction of features is that the “feature selection” keeps a subset of the original features [ 97 ], while “feature extraction” creates brand new ones [ 98 ]. In the following, we briefly discuss these techniques.
Feature selection: The selection of features, also known as the selection of variables or attributes in the data, is the process of choosing a subset of unique features (variables, predictors) to use in building machine learning and data science model. It decreases a model’s complexity by eliminating the irrelevant or less important features and allows for faster training of machine learning algorithms. A right and optimal subset of the selected features in a problem domain is capable to minimize the overfitting problem through simplifying and generalizing the model as well as increases the model’s accuracy [ 97 ]. Thus, “feature selection” [ 66 , 99 ] is considered as one of the primary concepts in machine learning that greatly affects the effectiveness and efficiency of the target machine learning model. Chi-squared test, Analysis of variance (ANOVA) test, Pearson’s correlation coefficient, recursive feature elimination, are some popular techniques that can be used for feature selection.
Feature extraction: In a machine learning-based model or system, feature extraction techniques usually provide a better understanding of the data, a way to improve prediction accuracy, and to reduce computational cost or training time. The aim of “feature extraction” [ 66 , 99 ] is to reduce the number of features in a dataset by generating new ones from the existing ones and then discarding the original features. The majority of the information found in the original set of features can then be summarized using this new reduced set of features. For instance, principal components analysis (PCA) is often used as a dimensionality-reduction technique to extract a lower-dimensional space creating new brand components from the existing features in a dataset [ 98 ].
Many algorithms have been proposed to reduce data dimensions in the machine learning and data science literature [ 41 , 125 ]. In the following, we summarize the popular methods that are used widely in various application areas.
Variance threshold: A simple basic approach to feature selection is the variance threshold [ 82 ]. This excludes all features of low variance, i.e., all features whose variance does not exceed the threshold. It eliminates all zero-variance characteristics by default, i.e., characteristics that have the same value in all samples. This feature selection algorithm looks only at the ( X ) features, not the ( y ) outputs needed, and can, therefore, be used for unsupervised learning.
Pearson correlation: Pearson’s correlation is another method to understand a feature’s relation to the response variable and can be used for feature selection [ 99 ]. This method is also used for finding the association between the features in a dataset. The resulting value is \([-1, 1]\) , where \(-1\) means perfect negative correlation, \(+1\) means perfect positive correlation, and 0 means that the two variables do not have a linear correlation. If two random variables represent X and Y , then the correlation coefficient between X and Y is defined as [ 41 ]
ANOVA: Analysis of variance (ANOVA) is a statistical tool used to verify the mean values of two or more groups that differ significantly from each other. ANOVA assumes a linear relationship between the variables and the target and the variables’ normal distribution. To statistically test the equality of means, the ANOVA method utilizes F tests. For feature selection, the results ‘ANOVA F value’ [ 82 ] of this test can be used where certain features independent of the goal variable can be omitted.
Chi square: The chi-square \({\chi }^2\) [ 82 ] statistic is an estimate of the difference between the effects of a series of events or variables observed and expected frequencies. The magnitude of the difference between the real and observed values, the degrees of freedom, and the sample size depends on \({\chi }^2\) . The chi-square \({\chi }^2\) is commonly used for testing relationships between categorical variables. If \(O_i\) represents observed value and \(E_i\) represents expected value, then
Recursive feature elimination (RFE): Recursive Feature Elimination (RFE) is a brute force approach to feature selection. RFE [ 82 ] fits the model and removes the weakest feature before it meets the specified number of features. Features are ranked by the coefficients or feature significance of the model. RFE aims to remove dependencies and collinearity in the model by recursively removing a small number of features per iteration.
Model-based selection: To reduce the dimensionality of the data, linear models penalized with the L 1 regularization can be used. Least absolute shrinkage and selection operator (Lasso) regression is a type of linear regression that has the property of shrinking some of the coefficients to zero [ 82 ]. Therefore, that feature can be removed from the model. Thus, the penalized lasso regression method, often used in machine learning to select the subset of variables. Extra Trees Classifier [ 82 ] is an example of a tree-based estimator that can be used to compute impurity-based function importance, which can then be used to discard irrelevant features.
Principal component analysis (PCA): Principal component analysis (PCA) is a well-known unsupervised learning approach in the field of machine learning and data science. PCA is a mathematical technique that transforms a set of correlated variables into a set of uncorrelated variables known as principal components [ 48 , 81 ]. Figure 8 shows an example of the effect of PCA on various dimensions space, where Fig. 8 a shows the original features in 3D space, and Fig. 8 b shows the created principal components PC1 and PC2 onto a 2D plane, and 1D line with the principal component PC1 respectively. Thus, PCA can be used as a feature extraction technique that reduces the dimensionality of the datasets, and to build an effective machine learning model [ 98 ]. Technically, PCA identifies the completely transformed with the highest eigenvalues of a covariance matrix and then uses those to project the data into a new subspace of equal or fewer dimensions [ 82 ].
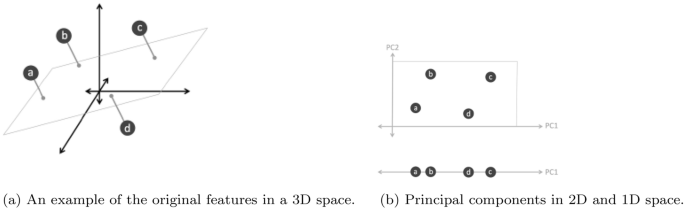
An example of a principal component analysis (PCA) and created principal components PC1 and PC2 in different dimension space
Association Rule Learning
Association rule learning is a rule-based machine learning approach to discover interesting relationships, “IF-THEN” statements, in large datasets between variables [ 7 ]. One example is that “if a customer buys a computer or laptop (an item), s/he is likely to also buy anti-virus software (another item) at the same time”. Association rules are employed today in many application areas, including IoT services, medical diagnosis, usage behavior analytics, web usage mining, smartphone applications, cybersecurity applications, and bioinformatics. In comparison to sequence mining, association rule learning does not usually take into account the order of things within or across transactions. A common way of measuring the usefulness of association rules is to use its parameter, the ‘support’ and ‘confidence’, which is introduced in [ 7 ].
In the data mining literature, many association rule learning methods have been proposed, such as logic dependent [ 34 ], frequent pattern based [ 8 , 49 , 68 ], and tree-based [ 42 ]. The most popular association rule learning algorithms are summarized below.
AIS and SETM: AIS is the first algorithm proposed by Agrawal et al. [ 7 ] for association rule mining. The AIS algorithm’s main downside is that too many candidate itemsets are generated, requiring more space and wasting a lot of effort. This algorithm calls for too many passes over the entire dataset to produce the rules. Another approach SETM [ 49 ] exhibits good performance and stable behavior with execution time; however, it suffers from the same flaw as the AIS algorithm.
Apriori: For generating association rules for a given dataset, Agrawal et al. [ 8 ] proposed the Apriori, Apriori-TID, and Apriori-Hybrid algorithms. These later algorithms outperform the AIS and SETM mentioned above due to the Apriori property of frequent itemset [ 8 ]. The term ‘Apriori’ usually refers to having prior knowledge of frequent itemset properties. Apriori uses a “bottom-up” approach, where it generates the candidate itemsets. To reduce the search space, Apriori uses the property “all subsets of a frequent itemset must be frequent; and if an itemset is infrequent, then all its supersets must also be infrequent”. Another approach predictive Apriori [ 108 ] can also generate rules; however, it receives unexpected results as it combines both the support and confidence. The Apriori [ 8 ] is the widely applicable techniques in mining association rules.
ECLAT: This technique was proposed by Zaki et al. [ 131 ] and stands for Equivalence Class Clustering and bottom-up Lattice Traversal. ECLAT uses a depth-first search to find frequent itemsets. In contrast to the Apriori [ 8 ] algorithm, which represents data in a horizontal pattern, it represents data vertically. Hence, the ECLAT algorithm is more efficient and scalable in the area of association rule learning. This algorithm is better suited for small and medium datasets whereas the Apriori algorithm is used for large datasets.
FP-Growth: Another common association rule learning technique based on the frequent-pattern tree (FP-tree) proposed by Han et al. [ 42 ] is Frequent Pattern Growth, known as FP-Growth. The key difference with Apriori is that while generating rules, the Apriori algorithm [ 8 ] generates frequent candidate itemsets; on the other hand, the FP-growth algorithm [ 42 ] prevents candidate generation and thus produces a tree by the successful strategy of ‘divide and conquer’ approach. Due to its sophistication, however, FP-Tree is challenging to use in an interactive mining environment [ 133 ]. Thus, the FP-Tree would not fit into memory for massive data sets, making it challenging to process big data as well. Another solution is RARM (Rapid Association Rule Mining) proposed by Das et al. [ 26 ] but faces a related FP-tree issue [ 133 ].
ABC-RuleMiner: A rule-based machine learning method, recently proposed in our earlier paper, by Sarker et al. [ 104 ], to discover the interesting non-redundant rules to provide real-world intelligent services. This algorithm effectively identifies the redundancy in associations by taking into account the impact or precedence of the related contextual features and discovers a set of non-redundant association rules. This algorithm first constructs an association generation tree (AGT), a top-down approach, and then extracts the association rules through traversing the tree. Thus, ABC-RuleMiner is more potent than traditional rule-based methods in terms of both non-redundant rule generation and intelligent decision-making, particularly in a context-aware smart computing environment, where human or user preferences are involved.
Among the association rule learning techniques discussed above, Apriori [ 8 ] is the most widely used algorithm for discovering association rules from a given dataset [ 133 ]. The main strength of the association learning technique is its comprehensiveness, as it generates all associations that satisfy the user-specified constraints, such as minimum support and confidence value. The ABC-RuleMiner approach [ 104 ] discussed earlier could give significant results in terms of non-redundant rule generation and intelligent decision-making for the relevant application areas in the real world.
Reinforcement Learning
Reinforcement learning (RL) is a machine learning technique that allows an agent to learn by trial and error in an interactive environment using input from its actions and experiences. Unlike supervised learning, which is based on given sample data or examples, the RL method is based on interacting with the environment. The problem to be solved in reinforcement learning (RL) is defined as a Markov Decision Process (MDP) [ 86 ], i.e., all about sequentially making decisions. An RL problem typically includes four elements such as Agent, Environment, Rewards, and Policy.
RL can be split roughly into Model-based and Model-free techniques. Model-based RL is the process of inferring optimal behavior from a model of the environment by performing actions and observing the results, which include the next state and the immediate reward [ 85 ]. AlphaZero, AlphaGo [ 113 ] are examples of the model-based approaches. On the other hand, a model-free approach does not use the distribution of the transition probability and the reward function associated with MDP. Q-learning, Deep Q Network, Monte Carlo Control, SARSA (State–Action–Reward–State–Action), etc. are some examples of model-free algorithms [ 52 ]. The policy network, which is required for model-based RL but not for model-free, is the key difference between model-free and model-based learning. In the following, we discuss the popular RL algorithms.
Monte Carlo methods: Monte Carlo techniques, or Monte Carlo experiments, are a wide category of computational algorithms that rely on repeated random sampling to obtain numerical results [ 52 ]. The underlying concept is to use randomness to solve problems that are deterministic in principle. Optimization, numerical integration, and making drawings from the probability distribution are the three problem classes where Monte Carlo techniques are most commonly used.
Q-learning: Q-learning is a model-free reinforcement learning algorithm for learning the quality of behaviors that tell an agent what action to take under what conditions [ 52 ]. It does not need a model of the environment (hence the term “model-free”), and it can deal with stochastic transitions and rewards without the need for adaptations. The ‘Q’ in Q-learning usually stands for quality, as the algorithm calculates the maximum expected rewards for a given behavior in a given state.
Deep Q-learning: The basic working step in Deep Q-Learning [ 52 ] is that the initial state is fed into the neural network, which returns the Q-value of all possible actions as an output. Still, when we have a reasonably simple setting to overcome, Q-learning works well. However, when the number of states and actions becomes more complicated, deep learning can be used as a function approximator.
Reinforcement learning, along with supervised and unsupervised learning, is one of the basic machine learning paradigms. RL can be used to solve numerous real-world problems in various fields, such as game theory, control theory, operations analysis, information theory, simulation-based optimization, manufacturing, supply chain logistics, multi-agent systems, swarm intelligence, aircraft control, robot motion control, and many more.
Artificial Neural Network and Deep Learning
Deep learning is part of a wider family of artificial neural networks (ANN)-based machine learning approaches with representation learning. Deep learning provides a computational architecture by combining several processing layers, such as input, hidden, and output layers, to learn from data [ 41 ]. The main advantage of deep learning over traditional machine learning methods is its better performance in several cases, particularly learning from large datasets [ 105 , 129 ]. Figure 9 shows a general performance of deep learning over machine learning considering the increasing amount of data. However, it may vary depending on the data characteristics and experimental set up.
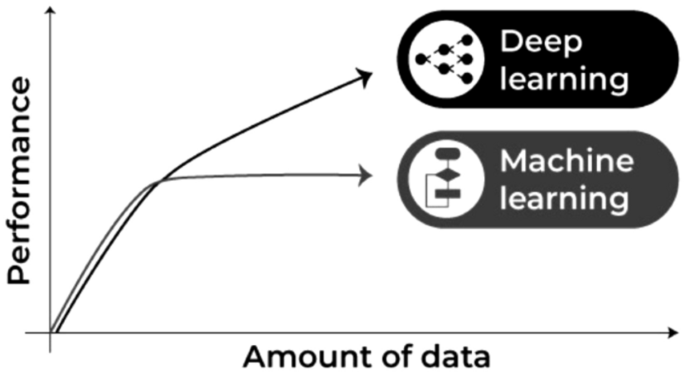
Machine learning and deep learning performance in general with the amount of data
The most common deep learning algorithms are: Multi-layer Perceptron (MLP), Convolutional Neural Network (CNN, or ConvNet), Long Short-Term Memory Recurrent Neural Network (LSTM-RNN) [ 96 ]. In the following, we discuss various types of deep learning methods that can be used to build effective data-driven models for various purposes.
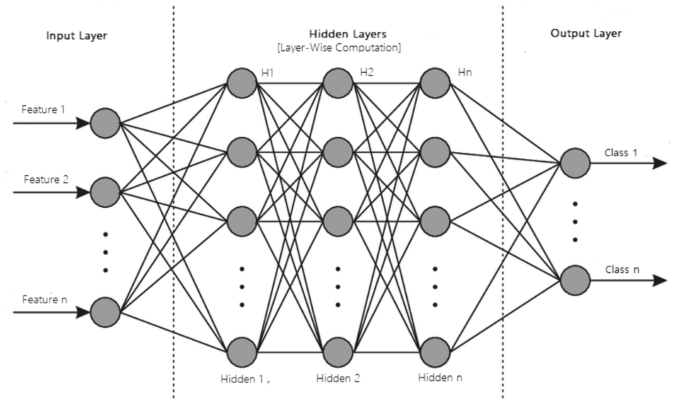
A structure of an artificial neural network modeling with multiple processing layers
MLP: The base architecture of deep learning, which is also known as the feed-forward artificial neural network, is called a multilayer perceptron (MLP) [ 82 ]. A typical MLP is a fully connected network consisting of an input layer, one or more hidden layers, and an output layer, as shown in Fig. 10 . Each node in one layer connects to each node in the following layer at a certain weight. MLP utilizes the “Backpropagation” technique [ 41 ], the most “fundamental building block” in a neural network, to adjust the weight values internally while building the model. MLP is sensitive to scaling features and allows a variety of hyperparameters to be tuned, such as the number of hidden layers, neurons, and iterations, which can result in a computationally costly model.
CNN or ConvNet: The convolution neural network (CNN) [ 65 ] enhances the design of the standard ANN, consisting of convolutional layers, pooling layers, as well as fully connected layers, as shown in Fig. 11 . As it takes the advantage of the two-dimensional (2D) structure of the input data, it is typically broadly used in several areas such as image and video recognition, image processing and classification, medical image analysis, natural language processing, etc. While CNN has a greater computational burden, without any manual intervention, it has the advantage of automatically detecting the important features, and hence CNN is considered to be more powerful than conventional ANN. A number of advanced deep learning models based on CNN can be used in the field, such as AlexNet [ 60 ], Xception [ 24 ], Inception [ 118 ], Visual Geometry Group (VGG) [ 44 ], ResNet [ 45 ], etc.
LSTM-RNN: Long short-term memory (LSTM) is an artificial recurrent neural network (RNN) architecture used in the area of deep learning [ 38 ]. LSTM has feedback links, unlike normal feed-forward neural networks. LSTM networks are well-suited for analyzing and learning sequential data, such as classifying, processing, and predicting data based on time series data, which differentiates it from other conventional networks. Thus, LSTM can be used when the data are in a sequential format, such as time, sentence, etc., and commonly applied in the area of time-series analysis, natural language processing, speech recognition, etc.
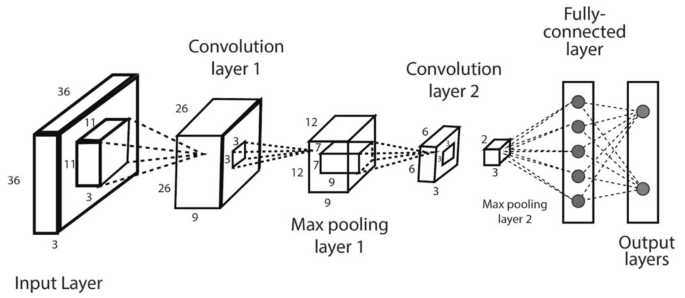
An example of a convolutional neural network (CNN or ConvNet) including multiple convolution and pooling layers
In addition to these most common deep learning methods discussed above, several other deep learning approaches [ 96 ] exist in the area for various purposes. For instance, the self-organizing map (SOM) [ 58 ] uses unsupervised learning to represent the high-dimensional data by a 2D grid map, thus achieving dimensionality reduction. The autoencoder (AE) [ 15 ] is another learning technique that is widely used for dimensionality reduction as well and feature extraction in unsupervised learning tasks. Restricted Boltzmann machines (RBM) [ 46 ] can be used for dimensionality reduction, classification, regression, collaborative filtering, feature learning, and topic modeling. A deep belief network (DBN) is typically composed of simple, unsupervised networks such as restricted Boltzmann machines (RBMs) or autoencoders, and a backpropagation neural network (BPNN) [ 123 ]. A generative adversarial network (GAN) [ 39 ] is a form of the network for deep learning that can generate data with characteristics close to the actual data input. Transfer learning is currently very common because it can train deep neural networks with comparatively low data, which is typically the re-use of a new problem with a pre-trained model [ 124 ]. A brief discussion of these artificial neural networks (ANN) and deep learning (DL) models are summarized in our earlier paper Sarker et al. [ 96 ].
Overall, based on the learning techniques discussed above, we can conclude that various types of machine learning techniques, such as classification analysis, regression, data clustering, feature selection and extraction, and dimensionality reduction, association rule learning, reinforcement learning, or deep learning techniques, can play a significant role for various purposes according to their capabilities. In the following section, we discuss several application areas based on machine learning algorithms.
Applications of Machine Learning
In the current age of the Fourth Industrial Revolution (4IR), machine learning becomes popular in various application areas, because of its learning capabilities from the past and making intelligent decisions. In the following, we summarize and discuss ten popular application areas of machine learning technology.
Predictive analytics and intelligent decision-making: A major application field of machine learning is intelligent decision-making by data-driven predictive analytics [ 21 , 70 ]. The basis of predictive analytics is capturing and exploiting relationships between explanatory variables and predicted variables from previous events to predict the unknown outcome [ 41 ]. For instance, identifying suspects or criminals after a crime has been committed, or detecting credit card fraud as it happens. Another application, where machine learning algorithms can assist retailers in better understanding consumer preferences and behavior, better manage inventory, avoiding out-of-stock situations, and optimizing logistics and warehousing in e-commerce. Various machine learning algorithms such as decision trees, support vector machines, artificial neural networks, etc. [ 106 , 125 ] are commonly used in the area. Since accurate predictions provide insight into the unknown, they can improve the decisions of industries, businesses, and almost any organization, including government agencies, e-commerce, telecommunications, banking and financial services, healthcare, sales and marketing, transportation, social networking, and many others.
Cybersecurity and threat intelligence: Cybersecurity is one of the most essential areas of Industry 4.0. [ 114 ], which is typically the practice of protecting networks, systems, hardware, and data from digital attacks [ 114 ]. Machine learning has become a crucial cybersecurity technology that constantly learns by analyzing data to identify patterns, better detect malware in encrypted traffic, find insider threats, predict where bad neighborhoods are online, keep people safe while browsing, or secure data in the cloud by uncovering suspicious activity. For instance, clustering techniques can be used to identify cyber-anomalies, policy violations, etc. To detect various types of cyber-attacks or intrusions machine learning classification models by taking into account the impact of security features are useful [ 97 ]. Various deep learning-based security models can also be used on the large scale of security datasets [ 96 , 129 ]. Moreover, security policy rules generated by association rule learning techniques can play a significant role to build a rule-based security system [ 105 ]. Thus, we can say that various learning techniques discussed in Sect. Machine Learning Tasks and Algorithms , can enable cybersecurity professionals to be more proactive inefficiently preventing threats and cyber-attacks.
Internet of things (IoT) and smart cities: Internet of Things (IoT) is another essential area of Industry 4.0. [ 114 ], which turns everyday objects into smart objects by allowing them to transmit data and automate tasks without the need for human interaction. IoT is, therefore, considered to be the big frontier that can enhance almost all activities in our lives, such as smart governance, smart home, education, communication, transportation, retail, agriculture, health care, business, and many more [ 70 ]. Smart city is one of IoT’s core fields of application, using technologies to enhance city services and residents’ living experiences [ 132 , 135 ]. As machine learning utilizes experience to recognize trends and create models that help predict future behavior and events, it has become a crucial technology for IoT applications [ 103 ]. For example, to predict traffic in smart cities, parking availability prediction, estimate the total usage of energy of the citizens for a particular period, make context-aware and timely decisions for the people, etc. are some tasks that can be solved using machine learning techniques according to the current needs of the people.
Traffic prediction and transportation: Transportation systems have become a crucial component of every country’s economic development. Nonetheless, several cities around the world are experiencing an excessive rise in traffic volume, resulting in serious issues such as delays, traffic congestion, higher fuel prices, increased CO \(_2\) pollution, accidents, emergencies, and a decline in modern society’s quality of life [ 40 ]. Thus, an intelligent transportation system through predicting future traffic is important, which is an indispensable part of a smart city. Accurate traffic prediction based on machine and deep learning modeling can help to minimize the issues [ 17 , 30 , 31 ]. For example, based on the travel history and trend of traveling through various routes, machine learning can assist transportation companies in predicting possible issues that may occur on specific routes and recommending their customers to take a different path. Ultimately, these learning-based data-driven models help improve traffic flow, increase the usage and efficiency of sustainable modes of transportation, and limit real-world disruption by modeling and visualizing future changes.
Healthcare and COVID-19 pandemic: Machine learning can help to solve diagnostic and prognostic problems in a variety of medical domains, such as disease prediction, medical knowledge extraction, detecting regularities in data, patient management, etc. [ 33 , 77 , 112 ]. Coronavirus disease (COVID-19) is an infectious disease caused by a newly discovered coronavirus, according to the World Health Organization (WHO) [ 3 ]. Recently, the learning techniques have become popular in the battle against COVID-19 [ 61 , 63 ]. For the COVID-19 pandemic, the learning techniques are used to classify patients at high risk, their mortality rate, and other anomalies [ 61 ]. It can also be used to better understand the virus’s origin, COVID-19 outbreak prediction, as well as for disease diagnosis and treatment [ 14 , 50 ]. With the help of machine learning, researchers can forecast where and when, the COVID-19 is likely to spread, and notify those regions to match the required arrangements. Deep learning also provides exciting solutions to the problems of medical image processing and is seen as a crucial technique for potential applications, particularly for COVID-19 pandemic [ 10 , 78 , 111 ]. Overall, machine and deep learning techniques can help to fight the COVID-19 virus and the pandemic as well as intelligent clinical decisions making in the domain of healthcare.
E-commerce and product recommendations: Product recommendation is one of the most well known and widely used applications of machine learning, and it is one of the most prominent features of almost any e-commerce website today. Machine learning technology can assist businesses in analyzing their consumers’ purchasing histories and making customized product suggestions for their next purchase based on their behavior and preferences. E-commerce companies, for example, can easily position product suggestions and offers by analyzing browsing trends and click-through rates of specific items. Using predictive modeling based on machine learning techniques, many online retailers, such as Amazon [ 71 ], can better manage inventory, prevent out-of-stock situations, and optimize logistics and warehousing. The future of sales and marketing is the ability to capture, evaluate, and use consumer data to provide a customized shopping experience. Furthermore, machine learning techniques enable companies to create packages and content that are tailored to the needs of their customers, allowing them to maintain existing customers while attracting new ones.
NLP and sentiment analysis: Natural language processing (NLP) involves the reading and understanding of spoken or written language through the medium of a computer [ 79 , 103 ]. Thus, NLP helps computers, for instance, to read a text, hear speech, interpret it, analyze sentiment, and decide which aspects are significant, where machine learning techniques can be used. Virtual personal assistant, chatbot, speech recognition, document description, language or machine translation, etc. are some examples of NLP-related tasks. Sentiment Analysis [ 90 ] (also referred to as opinion mining or emotion AI) is an NLP sub-field that seeks to identify and extract public mood and views within a given text through blogs, reviews, social media, forums, news, etc. For instance, businesses and brands use sentiment analysis to understand the social sentiment of their brand, product, or service through social media platforms or the web as a whole. Overall, sentiment analysis is considered as a machine learning task that analyzes texts for polarity, such as “positive”, “negative”, or “neutral” along with more intense emotions like very happy, happy, sad, very sad, angry, have interest, or not interested etc.
Image, speech and pattern recognition: Image recognition [ 36 ] is a well-known and widespread example of machine learning in the real world, which can identify an object as a digital image. For instance, to label an x-ray as cancerous or not, character recognition, or face detection in an image, tagging suggestions on social media, e.g., Facebook, are common examples of image recognition. Speech recognition [ 23 ] is also very popular that typically uses sound and linguistic models, e.g., Google Assistant, Cortana, Siri, Alexa, etc. [ 67 ], where machine learning methods are used. Pattern recognition [ 13 ] is defined as the automated recognition of patterns and regularities in data, e.g., image analysis. Several machine learning techniques such as classification, feature selection, clustering, or sequence labeling methods are used in the area.
Sustainable agriculture: Agriculture is essential to the survival of all human activities [ 109 ]. Sustainable agriculture practices help to improve agricultural productivity while also reducing negative impacts on the environment [ 5 , 25 , 109 ]. The sustainable agriculture supply chains are knowledge-intensive and based on information, skills, technologies, etc., where knowledge transfer encourages farmers to enhance their decisions to adopt sustainable agriculture practices utilizing the increasing amount of data captured by emerging technologies, e.g., the Internet of Things (IoT), mobile technologies and devices, etc. [ 5 , 53 , 54 ]. Machine learning can be applied in various phases of sustainable agriculture, such as in the pre-production phase - for the prediction of crop yield, soil properties, irrigation requirements, etc.; in the production phase—for weather prediction, disease detection, weed detection, soil nutrient management, livestock management, etc.; in processing phase—for demand estimation, production planning, etc. and in the distribution phase - the inventory management, consumer analysis, etc.
User behavior analytics and context-aware smartphone applications: Context-awareness is a system’s ability to capture knowledge about its surroundings at any moment and modify behaviors accordingly [ 28 , 93 ]. Context-aware computing uses software and hardware to automatically collect and interpret data for direct responses. The mobile app development environment has been changed greatly with the power of AI, particularly, machine learning techniques through their learning capabilities from contextual data [ 103 , 136 ]. Thus, the developers of mobile apps can rely on machine learning to create smart apps that can understand human behavior, support, and entertain users [ 107 , 137 , 140 ]. To build various personalized data-driven context-aware systems, such as smart interruption management, smart mobile recommendation, context-aware smart searching, decision-making that intelligently assist end mobile phone users in a pervasive computing environment, machine learning techniques are applicable. For example, context-aware association rules can be used to build an intelligent phone call application [ 104 ]. Clustering approaches are useful in capturing users’ diverse behavioral activities by taking into account data in time series [ 102 ]. To predict the future events in various contexts, the classification methods can be used [ 106 , 139 ]. Thus, various learning techniques discussed in Sect. “ Machine Learning Tasks and Algorithms ” can help to build context-aware adaptive and smart applications according to the preferences of the mobile phone users.
In addition to these application areas, machine learning-based models can also apply to several other domains such as bioinformatics, cheminformatics, computer networks, DNA sequence classification, economics and banking, robotics, advanced engineering, and many more.
Challenges and Research Directions
Our study on machine learning algorithms for intelligent data analysis and applications opens several research issues in the area. Thus, in this section, we summarize and discuss the challenges faced and the potential research opportunities and future directions.
In general, the effectiveness and the efficiency of a machine learning-based solution depend on the nature and characteristics of the data, and the performance of the learning algorithms. To collect the data in the relevant domain, such as cybersecurity, IoT, healthcare and agriculture discussed in Sect. “ Applications of Machine Learning ” is not straightforward, although the current cyberspace enables the production of a huge amount of data with very high frequency. Thus, collecting useful data for the target machine learning-based applications, e.g., smart city applications, and their management is important to further analysis. Therefore, a more in-depth investigation of data collection methods is needed while working on the real-world data. Moreover, the historical data may contain many ambiguous values, missing values, outliers, and meaningless data. The machine learning algorithms, discussed in Sect “ Machine Learning Tasks and Algorithms ” highly impact on data quality, and availability for training, and consequently on the resultant model. Thus, to accurately clean and pre-process the diverse data collected from diverse sources is a challenging task. Therefore, effectively modifying or enhance existing pre-processing methods, or proposing new data preparation techniques are required to effectively use the learning algorithms in the associated application domain.
To analyze the data and extract insights, there exist many machine learning algorithms, summarized in Sect. “ Machine Learning Tasks and Algorithms ”. Thus, selecting a proper learning algorithm that is suitable for the target application is challenging. The reason is that the outcome of different learning algorithms may vary depending on the data characteristics [ 106 ]. Selecting a wrong learning algorithm would result in producing unexpected outcomes that may lead to loss of effort, as well as the model’s effectiveness and accuracy. In terms of model building, the techniques discussed in Sect. “ Machine Learning Tasks and Algorithms ” can directly be used to solve many real-world issues in diverse domains, such as cybersecurity, smart cities and healthcare summarized in Sect. “ Applications of Machine Learning ”. However, the hybrid learning model, e.g., the ensemble of methods, modifying or enhancement of the existing learning techniques, or designing new learning methods, could be a potential future work in the area.
Thus, the ultimate success of a machine learning-based solution and corresponding applications mainly depends on both the data and the learning algorithms. If the data are bad to learn, such as non-representative, poor-quality, irrelevant features, or insufficient quantity for training, then the machine learning models may become useless or will produce lower accuracy. Therefore, effectively processing the data and handling the diverse learning algorithms are important, for a machine learning-based solution and eventually building intelligent applications.
In this paper, we have conducted a comprehensive overview of machine learning algorithms for intelligent data analysis and applications. According to our goal, we have briefly discussed how various types of machine learning methods can be used for making solutions to various real-world issues. A successful machine learning model depends on both the data and the performance of the learning algorithms. The sophisticated learning algorithms then need to be trained through the collected real-world data and knowledge related to the target application before the system can assist with intelligent decision-making. We also discussed several popular application areas based on machine learning techniques to highlight their applicability in various real-world issues. Finally, we have summarized and discussed the challenges faced and the potential research opportunities and future directions in the area. Therefore, the challenges that are identified create promising research opportunities in the field which must be addressed with effective solutions in various application areas. Overall, we believe that our study on machine learning-based solutions opens up a promising direction and can be used as a reference guide for potential research and applications for both academia and industry professionals as well as for decision-makers, from a technical point of view.
Canadian institute of cybersecurity, university of new brunswick, iscx dataset, http://www.unb.ca/cic/datasets/index.html/ (Accessed on 20 October 2019).
Cic-ddos2019 [online]. available: https://www.unb.ca/cic/datasets/ddos-2019.html/ (Accessed on 28 March 2020).
World health organization: WHO. http://www.who.int/ .
Google trends. In https://trends.google.com/trends/ , 2019.
Adnan N, Nordin Shahrina Md, Rahman I, Noor A. The effects of knowledge transfer on farmers decision making toward sustainable agriculture practices. World J Sci Technol Sustain Dev. 2018.
Agrawal R, Gehrke J, Gunopulos D, Raghavan P. Automatic subspace clustering of high dimensional data for data mining applications. In: Proceedings of the 1998 ACM SIGMOD international conference on Management of data. 1998; 94–105
Agrawal R, Imieliński T, Swami A. Mining association rules between sets of items in large databases. In: ACM SIGMOD Record. ACM. 1993;22: 207–216
Agrawal R, Gehrke J, Gunopulos D, Raghavan P. Fast algorithms for mining association rules. In: Proceedings of the International Joint Conference on Very Large Data Bases, Santiago Chile. 1994; 1215: 487–499.
Aha DW, Kibler D, Albert M. Instance-based learning algorithms. Mach Learn. 1991;6(1):37–66.
Article Google Scholar
Alakus TB, Turkoglu I. Comparison of deep learning approaches to predict covid-19 infection. Chaos Solit Fract. 2020;140:
Amit Y, Geman D. Shape quantization and recognition with randomized trees. Neural Comput. 1997;9(7):1545–88.
Ankerst M, Breunig MM, Kriegel H-P, Sander J. Optics: ordering points to identify the clustering structure. ACM Sigmod Record. 1999;28(2):49–60.
Anzai Y. Pattern recognition and machine learning. Elsevier; 2012.
MATH Google Scholar
Ardabili SF, Mosavi A, Ghamisi P, Ferdinand F, Varkonyi-Koczy AR, Reuter U, Rabczuk T, Atkinson PM. Covid-19 outbreak prediction with machine learning. Algorithms. 2020;13(10):249.
Article MathSciNet Google Scholar
Baldi P. Autoencoders, unsupervised learning, and deep architectures. In: Proceedings of ICML workshop on unsupervised and transfer learning, 2012; 37–49 .
Balducci F, Impedovo D, Pirlo G. Machine learning applications on agricultural datasets for smart farm enhancement. Machines. 2018;6(3):38.
Boukerche A, Wang J. Machine learning-based traffic prediction models for intelligent transportation systems. Comput Netw. 2020;181
Breiman L. Bagging predictors. Mach Learn. 1996;24(2):123–40.
Article MATH Google Scholar
Breiman L. Random forests. Mach Learn. 2001;45(1):5–32.
Breiman L, Friedman J, Stone CJ, Olshen RA. Classification and regression trees. CRC Press; 1984.
Cao L. Data science: a comprehensive overview. ACM Comput Surv (CSUR). 2017;50(3):43.
Google Scholar
Carpenter GA, Grossberg S. A massively parallel architecture for a self-organizing neural pattern recognition machine. Comput Vis Graph Image Process. 1987;37(1):54–115.
Chiu C-C, Sainath TN, Wu Y, Prabhavalkar R, Nguyen P, Chen Z, Kannan A, Weiss RJ, Rao K, Gonina E, et al. State-of-the-art speech recognition with sequence-to-sequence models. In: 2018 IEEE International Conference on Acoustics, Speech and Signal Processing (ICASSP), 2018 pages 4774–4778. IEEE .
Chollet F. Xception: deep learning with depthwise separable convolutions. In: Proceedings of the IEEE conference on computer vision and pattern recognition, pages 1251–1258, 2017.
Cobuloglu H, Büyüktahtakın IE. A stochastic multi-criteria decision analysis for sustainable biomass crop selection. Expert Syst Appl. 2015;42(15–16):6065–74.
Das A, Ng W-K, Woon Y-K. Rapid association rule mining. In: Proceedings of the tenth international conference on Information and knowledge management, pages 474–481. ACM, 2001.
de Amorim RC. Constrained clustering with minkowski weighted k-means. In: 2012 IEEE 13th International Symposium on Computational Intelligence and Informatics (CINTI), pages 13–17. IEEE, 2012.
Dey AK. Understanding and using context. Person Ubiquit Comput. 2001;5(1):4–7.
Eagle N, Pentland AS. Reality mining: sensing complex social systems. Person Ubiquit Comput. 2006;10(4):255–68.
Essien A, Petrounias I, Sampaio P, Sampaio S. Improving urban traffic speed prediction using data source fusion and deep learning. In: 2019 IEEE International Conference on Big Data and Smart Computing (BigComp). IEEE. 2019: 1–8. .
Essien A, Petrounias I, Sampaio P, Sampaio S. A deep-learning model for urban traffic flow prediction with traffic events mined from twitter. In: World Wide Web, 2020: 1–24 .
Ester M, Kriegel H-P, Sander J, Xiaowei X, et al. A density-based algorithm for discovering clusters in large spatial databases with noise. Kdd. 1996;96:226–31.
Fatima M, Pasha M, et al. Survey of machine learning algorithms for disease diagnostic. J Intell Learn Syst Appl. 2017;9(01):1.
Flach PA, Lachiche N. Confirmation-guided discovery of first-order rules with tertius. Mach Learn. 2001;42(1–2):61–95.
Freund Y, Schapire RE, et al. Experiments with a new boosting algorithm. In: Icml, Citeseer. 1996; 96: 148–156
Fujiyoshi H, Hirakawa T, Yamashita T. Deep learning-based image recognition for autonomous driving. IATSS Res. 2019;43(4):244–52.
Fukunaga K, Hostetler L. The estimation of the gradient of a density function, with applications in pattern recognition. IEEE Trans Inform Theory. 1975;21(1):32–40.
Article MathSciNet MATH Google Scholar
Goodfellow I, Bengio Y, Courville A, Bengio Y. Deep learning. Cambridge: MIT Press; 2016.
Goodfellow I, Pouget-Abadie J, Mirza M, Xu B, Warde-Farley D, Ozair S, Courville A, Bengio Y. Generative adversarial nets. In: Advances in neural information processing systems. 2014: 2672–2680.
Guerrero-Ibáñez J, Zeadally S, Contreras-Castillo J. Sensor technologies for intelligent transportation systems. Sensors. 2018;18(4):1212.
Han J, Pei J, Kamber M. Data mining: concepts and techniques. Amsterdam: Elsevier; 2011.
Han J, Pei J, Yin Y. Mining frequent patterns without candidate generation. In: ACM Sigmod Record, ACM. 2000;29: 1–12.
Harmon SA, Sanford TH, Sheng X, Turkbey EB, Roth H, Ziyue X, Yang D, Myronenko A, Anderson V, Amalou A, et al. Artificial intelligence for the detection of covid-19 pneumonia on chest ct using multinational datasets. Nat Commun. 2020;11(1):1–7.
He K, Zhang X, Ren S, Sun J. Spatial pyramid pooling in deep convolutional networks for visual recognition. IEEE Trans Pattern Anal Mach Intell. 2015;37(9):1904–16.
He K, Zhang X, Ren S, Sun J. Deep residual learning for image recognition. In: Proceedings of the IEEE conference on computer vision and pattern recognition, 2016: 770–778.
Hinton GE. A practical guide to training restricted boltzmann machines. In: Neural networks: Tricks of the trade. Springer. 2012; 599-619
Holte RC. Very simple classification rules perform well on most commonly used datasets. Mach Learn. 1993;11(1):63–90.
Hotelling H. Analysis of a complex of statistical variables into principal components. J Edu Psychol. 1933;24(6):417.
Houtsma M, Swami A. Set-oriented mining for association rules in relational databases. In: Data Engineering, 1995. Proceedings of the Eleventh International Conference on, IEEE.1995:25–33.
Jamshidi M, Lalbakhsh A, Talla J, Peroutka Z, Hadjilooei F, Lalbakhsh P, Jamshidi M, La Spada L, Mirmozafari M, Dehghani M, et al. Artificial intelligence and covid-19: deep learning approaches for diagnosis and treatment. IEEE Access. 2020;8:109581–95.
John GH, Langley P. Estimating continuous distributions in bayesian classifiers. In: Proceedings of the Eleventh conference on Uncertainty in artificial intelligence, Morgan Kaufmann Publishers Inc. 1995; 338–345
Kaelbling LP, Littman ML, Moore AW. Reinforcement learning: a survey. J Artif Intell Res. 1996;4:237–85.
Kamble SS, Gunasekaran A, Gawankar SA. Sustainable industry 4.0 framework: a systematic literature review identifying the current trends and future perspectives. Process Saf Environ Protect. 2018;117:408–25.
Kamble SS, Gunasekaran A, Gawankar SA. Achieving sustainable performance in a data-driven agriculture supply chain: a review for research and applications. Int J Prod Econ. 2020;219:179–94.
Kaufman L, Rousseeuw PJ. Finding groups in data: an introduction to cluster analysis, vol. 344. John Wiley & Sons; 2009.
Keerthi SS, Shevade SK, Bhattacharyya C, Radha Krishna MK. Improvements to platt’s smo algorithm for svm classifier design. Neural Comput. 2001;13(3):637–49.
Khadse V, Mahalle PN, Biraris SV. An empirical comparison of supervised machine learning algorithms for internet of things data. In: 2018 Fourth International Conference on Computing Communication Control and Automation (ICCUBEA), IEEE. 2018; 1–6
Kohonen T. The self-organizing map. Proc IEEE. 1990;78(9):1464–80.
Koroniotis N, Moustafa N, Sitnikova E, Turnbull B. Towards the development of realistic botnet dataset in the internet of things for network forensic analytics: bot-iot dataset. Fut Gen Comput Syst. 2019;100:779–96.
Krizhevsky A, Sutskever I, Hinton GE. Imagenet classification with deep convolutional neural networks. In: Advances in neural information processing systems, 2012: 1097–1105
Kushwaha S, Bahl S, Bagha AK, Parmar KS, Javaid M, Haleem A, Singh RP. Significant applications of machine learning for covid-19 pandemic. J Ind Integr Manag. 2020;5(4).
Lade P, Ghosh R, Srinivasan S. Manufacturing analytics and industrial internet of things. IEEE Intell Syst. 2017;32(3):74–9.
Lalmuanawma S, Hussain J, Chhakchhuak L. Applications of machine learning and artificial intelligence for covid-19 (sars-cov-2) pandemic: a review. Chaos Sol Fract. 2020:110059 .
LeCessie S, Van Houwelingen JC. Ridge estimators in logistic regression. J R Stat Soc Ser C (Appl Stat). 1992;41(1):191–201.
LeCun Y, Bottou L, Bengio Y, Haffner P. Gradient-based learning applied to document recognition. Proc IEEE. 1998;86(11):2278–324.
Liu H, Motoda H. Feature extraction, construction and selection: A data mining perspective, vol. 453. Springer Science & Business Media; 1998.
López G, Quesada L, Guerrero LA. Alexa vs. siri vs. cortana vs. google assistant: a comparison of speech-based natural user interfaces. In: International Conference on Applied Human Factors and Ergonomics, Springer. 2017; 241–250.
Liu B, HsuW, Ma Y. Integrating classification and association rule mining. In: Proceedings of the fourth international conference on knowledge discovery and data mining, 1998.
MacQueen J, et al. Some methods for classification and analysis of multivariate observations. In: Proceedings of the fifth Berkeley symposium on mathematical statistics and probability, 1967;volume 1, pages 281–297. Oakland, CA, USA.
Mahdavinejad MS, Rezvan M, Barekatain M, Adibi P, Barnaghi P, Sheth AP. Machine learning for internet of things data analysis: a survey. Digit Commun Netw. 2018;4(3):161–75.
Marchand A, Marx P. Automated product recommendations with preference-based explanations. J Retail. 2020;96(3):328–43.
McCallum A. Information extraction: distilling structured data from unstructured text. Queue. 2005;3(9):48–57.
Mehrotra A, Hendley R, Musolesi M. Prefminer: mining user’s preferences for intelligent mobile notification management. In: Proceedings of the International Joint Conference on Pervasive and Ubiquitous Computing, Heidelberg, Germany, 12–16 September, 2016; pp. 1223–1234. ACM, New York, USA. .
Mohamadou Y, Halidou A, Kapen PT. A review of mathematical modeling, artificial intelligence and datasets used in the study, prediction and management of covid-19. Appl Intell. 2020;50(11):3913–25.
Mohammed M, Khan MB, Bashier Mohammed BE. Machine learning: algorithms and applications. CRC Press; 2016.
Book Google Scholar
Moustafa N, Slay J. Unsw-nb15: a comprehensive data set for network intrusion detection systems (unsw-nb15 network data set). In: 2015 military communications and information systems conference (MilCIS), 2015;pages 1–6. IEEE .
Nilashi M, Ibrahim OB, Ahmadi H, Shahmoradi L. An analytical method for diseases prediction using machine learning techniques. Comput Chem Eng. 2017;106:212–23.
Yujin O, Park S, Ye JC. Deep learning covid-19 features on cxr using limited training data sets. IEEE Trans Med Imaging. 2020;39(8):2688–700.
Otter DW, Medina JR , Kalita JK. A survey of the usages of deep learning for natural language processing. IEEE Trans Neural Netw Learn Syst. 2020.
Park H-S, Jun C-H. A simple and fast algorithm for k-medoids clustering. Expert Syst Appl. 2009;36(2):3336–41.
Liii Pearson K. on lines and planes of closest fit to systems of points in space. Lond Edinb Dublin Philos Mag J Sci. 1901;2(11):559–72.
Pedregosa F, Varoquaux G, Gramfort A, Michel V, Thirion B, Grisel O, Blondel M, Prettenhofer P, Weiss R, Dubourg V, et al. Scikit-learn: machine learning in python. J Mach Learn Res. 2011;12:2825–30.
MathSciNet MATH Google Scholar
Perveen S, Shahbaz M, Keshavjee K, Guergachi A. Metabolic syndrome and development of diabetes mellitus: predictive modeling based on machine learning techniques. IEEE Access. 2018;7:1365–75.
Santi P, Ram D, Rob C, Nathan E. Behavior-based adaptive call predictor. ACM Trans Auton Adapt Syst. 2011;6(3):21:1–21:28.
Polydoros AS, Nalpantidis L. Survey of model-based reinforcement learning: applications on robotics. J Intell Robot Syst. 2017;86(2):153–73.
Puterman ML. Markov decision processes: discrete stochastic dynamic programming. John Wiley & Sons; 2014.
Quinlan JR. Induction of decision trees. Mach Learn. 1986;1:81–106.
Quinlan JR. C4.5: programs for machine learning. Mach Learn. 1993.
Rasmussen C. The infinite gaussian mixture model. Adv Neural Inform Process Syst. 1999;12:554–60.
Ravi K, Ravi V. A survey on opinion mining and sentiment analysis: tasks, approaches and applications. Knowl Syst. 2015;89:14–46.
Rokach L. A survey of clustering algorithms. In: Data mining and knowledge discovery handbook, pages 269–298. Springer, 2010.
Safdar S, Zafar S, Zafar N, Khan NF. Machine learning based decision support systems (dss) for heart disease diagnosis: a review. Artif Intell Rev. 2018;50(4):597–623.
Sarker IH. Context-aware rule learning from smartphone data: survey, challenges and future directions. J Big Data. 2019;6(1):1–25.
Sarker IH. A machine learning based robust prediction model for real-life mobile phone data. Internet Things. 2019;5:180–93.
Sarker IH. Ai-driven cybersecurity: an overview, security intelligence modeling and research directions. SN Comput Sci. 2021.
Sarker IH. Deep cybersecurity: a comprehensive overview from neural network and deep learning perspective. SN Comput Sci. 2021.
Sarker IH, Abushark YB, Alsolami F, Khan A. Intrudtree: a machine learning based cyber security intrusion detection model. Symmetry. 2020;12(5):754.
Sarker IH, Abushark YB, Khan A. Contextpca: predicting context-aware smartphone apps usage based on machine learning techniques. Symmetry. 2020;12(4):499.
Sarker IH, Alqahtani H, Alsolami F, Khan A, Abushark YB, Siddiqui MK. Context pre-modeling: an empirical analysis for classification based user-centric context-aware predictive modeling. J Big Data. 2020;7(1):1–23.
Sarker IH, Alan C, Jun H, Khan AI, Abushark YB, Khaled S. Behavdt: a behavioral decision tree learning to build user-centric context-aware predictive model. Mob Netw Appl. 2019; 1–11.
Sarker IH, Colman A, Kabir MA, Han J. Phone call log as a context source to modeling individual user behavior. In: Proceedings of the 2016 ACM International Joint Conference on Pervasive and Ubiquitous Computing (Ubicomp): Adjunct, Germany, pages 630–634. ACM, 2016.
Sarker IH, Colman A, Kabir MA, Han J. Individualized time-series segmentation for mining mobile phone user behavior. Comput J Oxf Univ UK. 2018;61(3):349–68.
Sarker IH, Hoque MM, MdK Uddin, Tawfeeq A. Mobile data science and intelligent apps: concepts, ai-based modeling and research directions. Mob Netw Appl, pages 1–19, 2020.
Sarker IH, Kayes ASM. Abc-ruleminer: user behavioral rule-based machine learning method for context-aware intelligent services. J Netw Comput Appl. 2020; page 102762
Sarker IH, Kayes ASM, Badsha S, Alqahtani H, Watters P, Ng A. Cybersecurity data science: an overview from machine learning perspective. J Big Data. 2020;7(1):1–29.
Sarker IH, Watters P, Kayes ASM. Effectiveness analysis of machine learning classification models for predicting personalized context-aware smartphone usage. J Big Data. 2019;6(1):1–28.
Sarker IH, Salah K. Appspred: predicting context-aware smartphone apps using random forest learning. Internet Things. 2019;8:
Scheffer T. Finding association rules that trade support optimally against confidence. Intell Data Anal. 2005;9(4):381–95.
Sharma R, Kamble SS, Gunasekaran A, Kumar V, Kumar A. A systematic literature review on machine learning applications for sustainable agriculture supply chain performance. Comput Oper Res. 2020;119:
Shengli S, Ling CX. Hybrid cost-sensitive decision tree, knowledge discovery in databases. In: PKDD 2005, Proceedings of 9th European Conference on Principles and Practice of Knowledge Discovery in Databases. Lecture Notes in Computer Science, volume 3721, 2005.
Shorten C, Khoshgoftaar TM, Furht B. Deep learning applications for covid-19. J Big Data. 2021;8(1):1–54.
Gökhan S, Nevin Y. Data analysis in health and big data: a machine learning medical diagnosis model based on patients’ complaints. Commun Stat Theory Methods. 2019;1–10
Silver D, Huang A, Maddison CJ, Guez A, Sifre L, Van Den Driessche G, Schrittwieser J, Antonoglou I, Panneershelvam V, Lanctot M, et al. Mastering the game of go with deep neural networks and tree search. nature. 2016;529(7587):484–9.
Ślusarczyk B. Industry 4.0: Are we ready? Polish J Manag Stud. 17, 2018.
Sneath Peter HA. The application of computers to taxonomy. J Gen Microbiol. 1957;17(1).
Sorensen T. Method of establishing groups of equal amplitude in plant sociology based on similarity of species. Biol Skr. 1948; 5.
Srinivasan V, Moghaddam S, Mukherji A. Mobileminer: mining your frequent patterns on your phone. In: Proceedings of the International Joint Conference on Pervasive and Ubiquitous Computing, Seattle, WA, USA, 13-17 September, pp. 389–400. ACM, New York, USA. 2014.
Szegedy C, Liu W, Jia Y, Sermanet P, Reed S, Anguelov D, Erhan D, Vanhoucke V, Rabinovich A. Going deeper with convolutions. In: Proceedings of the IEEE conference on computer vision and pattern recognition. 2015; pages 1–9.
Tavallaee M, Bagheri E, Lu W, Ghorbani AA. A detailed analysis of the kdd cup 99 data set. In. IEEE symposium on computational intelligence for security and defense applications. IEEE. 2009;2009:1–6.
Tsagkias M. Tracy HK, Surya K, Vanessa M, de Rijke M. Challenges and research opportunities in ecommerce search and recommendations. In: ACM SIGIR Forum. volume 54. NY, USA: ACM New York; 2021. p. 1–23.
Wagstaff K, Cardie C, Rogers S, Schrödl S, et al. Constrained k-means clustering with background knowledge. Icml. 2001;1:577–84.
Wang W, Yang J, Muntz R, et al. Sting: a statistical information grid approach to spatial data mining. VLDB. 1997;97:186–95.
Wei P, Li Y, Zhang Z, Tao H, Li Z, Liu D. An optimization method for intrusion detection classification model based on deep belief network. IEEE Access. 2019;7:87593–605.
Weiss K, Khoshgoftaar TM, Wang DD. A survey of transfer learning. J Big data. 2016;3(1):9.
Witten IH, Frank E. Data Mining: Practical machine learning tools and techniques. Morgan Kaufmann; 2005.
Witten IH, Frank E, Trigg LE, Hall MA, Holmes G, Cunningham SJ. Weka: practical machine learning tools and techniques with java implementations. 1999.
Wu C-C, Yen-Liang C, Yi-Hung L, Xiang-Yu Y. Decision tree induction with a constrained number of leaf nodes. Appl Intell. 2016;45(3):673–85.
Wu X, Kumar V, Quinlan JR, Ghosh J, Yang Q, Motoda H, McLachlan GJ, Ng A, Liu B, Philip SY, et al. Top 10 algorithms in data mining. Knowl Inform Syst. 2008;14(1):1–37.
Xin Y, Kong L, Liu Z, Chen Y, Li Y, Zhu H, Gao M, Hou H, Wang C. Machine learning and deep learning methods for cybersecurity. IEEE Access. 2018;6:35365–81.
Xu D, Yingjie T. A comprehensive survey of clustering algorithms. Ann Data Sci. 2015;2(2):165–93.
Zaki MJ. Scalable algorithms for association mining. IEEE Trans Knowl Data Eng. 2000;12(3):372–90.
Zanella A, Bui N, Castellani A, Vangelista L, Zorzi M. Internet of things for smart cities. IEEE Internet Things J. 2014;1(1):22–32.
Zhao Q, Bhowmick SS. Association rule mining: a survey. Singapore: Nanyang Technological University; 2003.
Zheng T, Xie W, Xu L, He X, Zhang Y, You M, Yang G, Chen Y. A machine learning-based framework to identify type 2 diabetes through electronic health records. Int J Med Inform. 2017;97:120–7.
Zheng Y, Rajasegarar S, Leckie C. Parking availability prediction for sensor-enabled car parks in smart cities. In: Intelligent Sensors, Sensor Networks and Information Processing (ISSNIP), 2015 IEEE Tenth International Conference on. IEEE, 2015; pages 1–6.
Zhu H, Cao H, Chen E, Xiong H, Tian J. Exploiting enriched contextual information for mobile app classification. In: Proceedings of the 21st ACM international conference on Information and knowledge management. ACM, 2012; pages 1617–1621
Zhu H, Chen E, Xiong H, Kuifei Y, Cao H, Tian J. Mining mobile user preferences for personalized context-aware recommendation. ACM Trans Intell Syst Technol (TIST). 2014;5(4):58.
Zikang H, Yong Y, Guofeng Y, Xinyu Z. Sentiment analysis of agricultural product ecommerce review data based on deep learning. In: 2020 International Conference on Internet of Things and Intelligent Applications (ITIA), IEEE, 2020; pages 1–7
Zulkernain S, Madiraju P, Ahamed SI. A context aware interruption management system for mobile devices. In: Mobile Wireless Middleware, Operating Systems, and Applications. Springer. 2010; pages 221–234
Zulkernain S, Madiraju P, Ahamed S, Stamm K. A mobile intelligent interruption management system. J UCS. 2010;16(15):2060–80.
Download references
Author information
Authors and affiliations.
Swinburne University of Technology, Melbourne, VIC, 3122, Australia
Department of Computer Science and Engineering, Chittagong University of Engineering & Technology, 4349, Chattogram, Bangladesh
You can also search for this author in PubMed Google Scholar
Corresponding author
Correspondence to Iqbal H. Sarker .
Ethics declarations
Conflict of interest.
The author declares no conflict of interest.
Additional information
Publisher's note.
Springer Nature remains neutral with regard to jurisdictional claims in published maps and institutional affiliations.
This article is part of the topical collection “Advances in Computational Approaches for Artificial Intelligence, Image Processing, IoT and Cloud Applications” guest edited by Bhanu Prakash K N and M. Shivakumar.
Rights and permissions
Reprints and permissions
About this article
Sarker, I.H. Machine Learning: Algorithms, Real-World Applications and Research Directions. SN COMPUT. SCI. 2 , 160 (2021). https://doi.org/10.1007/s42979-021-00592-x
Download citation
Received : 27 January 2021
Accepted : 12 March 2021
Published : 22 March 2021
DOI : https://doi.org/10.1007/s42979-021-00592-x
Share this article
Anyone you share the following link with will be able to read this content:
Sorry, a shareable link is not currently available for this article.
Provided by the Springer Nature SharedIt content-sharing initiative
- Machine learning
- Deep learning
- Artificial intelligence
- Data science
- Data-driven decision-making
- Predictive analytics
- Intelligent applications
- Find a journal
- Publish with us
- Track your research
- Trending Now
- Foundational Courses
- Data Science
- Practice Problem
- Machine Learning
- System Design
- DevOps Tutorial
- ML | Active Learning
- Difference between Supervised and Unsupervised Learning
- Overview of ROBERTa model
- What is Saliency Map?
- Intuition behind Adagrad Optimizer
- Differentiate between Support Vector Machine and Logistic Regression
- Logistic Regression using Python
- Cost function in Logistic Regression in Machine Learning
- CNN | Introduction to Padding
- GrowNet: Gradient Boosting Neural Networks
- Orthogonal Projections
- Multiclass classification using scikit-learn
- Getting started with Dialogflow
- Rule-Based Classifier - Machine Learning
- Introduction to Speech Separation Based On Fast ICA
- Python | Create Test DataSets using Sklearn
- Early Stopping for Regularisation in Deep Learning
- ALBERT - A Light BERT for Supervised Learning
Machine Learning and Artificial Intelligence
Introduction :
Machine learning and artificial intelligence are two closely related fields that are revolutionizing the way we interact with technology. Machine learning refers to the process of teaching computers to learn from data, without being explicitly programmed to do so. This involves using algorithms and statistical models to find patterns in data, and then using these patterns to make predictions or decisions.
Artificial intelligence, on the other hand, is a broader field that encompasses machine learning as well as other approaches to building intelligent systems. Artificial intelligence is concerned with creating machines that can perform tasks that would normally require human intelligence, such as recognizing speech, understanding natural language, and making decisions based on complex data.
The goal of both machine learning and artificial intelligence is to create machines that can learn and adapt to new situations, without the need for explicit programming. By enabling computers to learn from data and make decisions based on that data, we can create systems that are more accurate, more efficient, and more effective at performing a wide range of tasks.
Machine learning and artificial intelligence are being used in a wide variety of applications, from self-driving cars and virtual assistants to medical diagnosis and fraud detection. As the technology continues to advance, we can expect to see even more innovative applications of machine learning and artificial intelligence in the future.
Machine Learning and Artificial Intelligence are creating a huge buzz worldwide. The plethora of applications in Artificial Intelligence has changed the face of technology. The terms Machine Learning and Artificial Intelligence are often used interchangeably. However, there is a stark difference between the two that is still unknown to industry professionals.
Let’s start by taking an example of Virtual Personal Assistants which have been familiar to most of us for quite some time now.
Machine learning and artificial intelligence (AI) are related but distinct fields.
Machine learning is a subset of AI that involves the development of algorithms and statistical models that enable computers to learn and make predictions or decisions without being explicitly programmed. Machine learning algorithms can be trained on data to identify patterns and make predictions about future events.
Artificial intelligence, on the other hand, is a broader field that encompasses machine learning as well as other techniques for creating intelligent systems. AI involves the development of computer systems that can perform tasks that typically require human intelligence, such as understanding natural language, recognizing images, and making decisions.
There are several types of machine learning, including:
Supervised learning: The algorithm is trained on a labeled dataset, where the desired output is already known. Unsupervised learning: The algorithm is not given any labeled data, it must find the underlying structure in the data on its own. Reinforcement learning: The algorithm learns from the feedback it receives from its actions in an environment. There are also several types of AI, including:
Strong AI: Capable of performing any intellectual task that a human can. Weak AI: Specialized for a specific task.
Working of Virtual Personal Assistants:
Siri (part of Apple Inc.’s iOS, watchOS, macOS, and tvOS operating systems), Google Now (a feature of Google Search offering predictive cards with information and daily updates in the Google app for Android and iOS.), Cortana (Cortana is a virtual assistant created by Microsoft for Windows 10) are intelligent digital personal assistants on the platforms like iOS, Android and Windows respectively. To put it plainly, they help to find relevant information when requested using voice. For instance, for answering queries like ‘What’s the temperature today?’ or ‘What is the way to the nearest supermarket’ etc. and the assistant will react by searching for information, transferring that information from the phone, or sending commands to various other applications.
AI is critical in these applications, as they gather data on the user’s request and utilize that data to perceive speech in a better manner and serve the user with answers that are customized to his inclination. Microsoft says that Cortana “consistently finds out about its user” and that it will in the end build up the capacity to anticipate users’ needs and cater to them. Virtual assistants process a tremendous measure of information from an assortment of sources to find out about users and be more compelling in helping them arrange and track their data. Machine learning is a vital part of these personal assistants as they gather and refine the data based on users’ past participation with them. Thereon, this arrangement of information is used to render results that are custom-made to users’ inclinations.
Roughly speaking, Artificial Intelligence (AI) is when a computer algorithm does intelligent work. On the other hand, Machine Learning is a part of AI that learns from the data that also involves the information gathered from previous experiences and allows the computer program to change its behavior accordingly. Artificial Intelligence is the superset of Machine Learning i.e. all Machine Learning is Artificial Intelligence but not all AI is Machine Learning.
Future Scope:
- Artificial Intelligence is here to stay and is going nowhere. It digs out the facts from algorithms for a meaningful execution of various decisions and goals predetermined by a firm.
- Artificial Intelligence and Machine Learning are likely to replace the current model of technology that we see these days, for example, traditional programming packages like ERP and CRM are certainly losing their charm.
- Firms like Facebook, and Google are investing a hefty amount in AI to get the desired outcome at a relatively lower computational time.
- Artificial Intelligence is something that is going to redefine the world of software and IT in the near future.
Advantages :
The advantages of machine learning and artificial intelligence are many, and include:
- Efficiency: Machine learning and artificial intelligence can automate complex processes and make them more efficient. This can save time and resources, and allow businesses to focus on more strategic tasks.
- Accuracy: Machine learning algorithms can analyze data and make predictions with a high degree of accuracy. This can lead to better decision-making and more accurate results.
- Personalization: Machine learning and artificial intelligence can be used to personalize products and services to individual users, based on their preferences and behavior.
- Scalability: Machine learning and artificial intelligence algorithms can be applied to large amounts of data, allowing organizations to scale their operations and handle larger volumes of information.
- Innovation: Machine learning and artificial intelligence can be used to identify new opportunities and create innovative solutions to complex problems.
- Cost savings: By automating processes and increasing efficiency, machine learning and artificial intelligence can help organizations save money and reduce costs.
Dis-advantages :
- Complexity: Machine learning and artificial intelligence systems can be complex and difficult to implement, requiring specialized expertise and resources.
- Bias: Machine learning algorithms can sometimes produce biased results, depending on the data that is used to train them. This can lead to unfair or discriminatory outcomes.
- Lack of transparency: Some machine learning and artificial intelligence systems are considered “black boxes,” meaning that it can be difficult to understand how they arrived at a particular decision or prediction.
- Security concerns: Machine learning and artificial intelligence systems can be vulnerable to attacks and hacking attempts, which could compromise sensitive data and systems.
- Job displacement: As automation becomes more prevalent, there may be concerns about job displacement and the impact on the workforce.
- Data quality: Machine learning and artificial intelligence systems rely on high-quality data to function effectively. Poor quality data can lead to inaccurate predictions and decisions.
Please Login to comment...
- How to Delete Whatsapp Business Account?
- Discord vs Zoom: Select The Efficienct One for Virtual Meetings?
- Otter AI vs Dragon Speech Recognition: Which is the best AI Transcription Tool?
- Google Messages To Let You Send Multiple Photos
- 30 OOPs Interview Questions and Answers (2024)
Improve your Coding Skills with Practice
What kind of Experience do you want to share?
Smart. Open. Grounded. Inventive. Read our Ideas Made to Matter.
Which program is right for you?
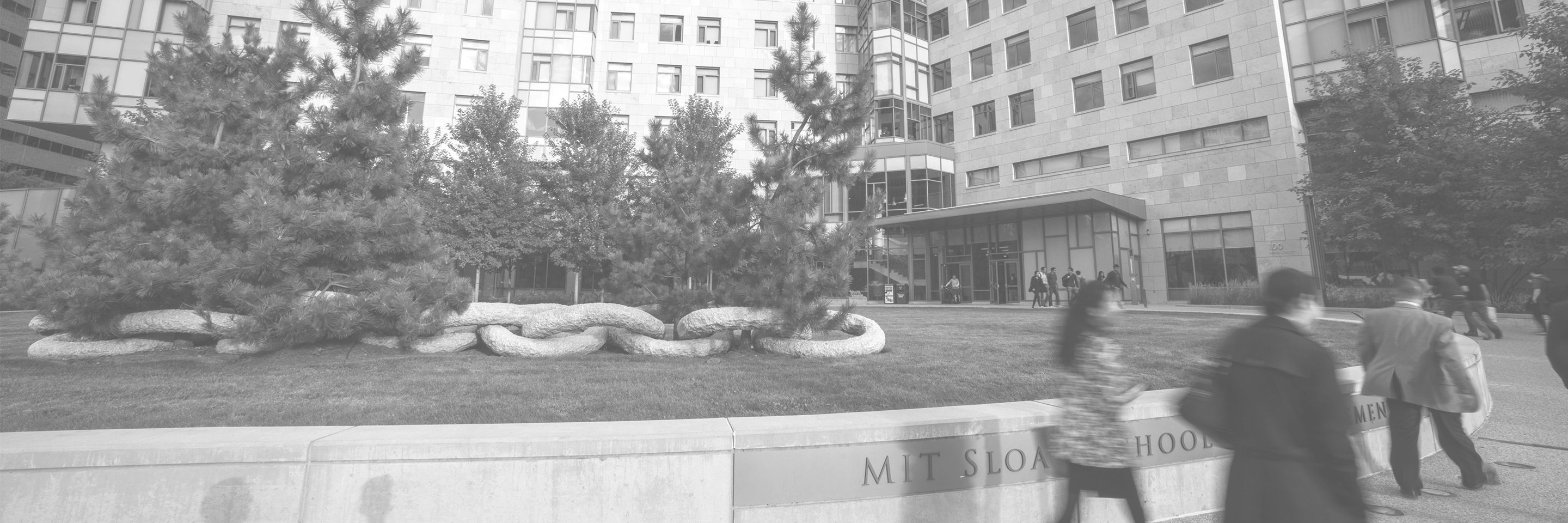
Through intellectual rigor and experiential learning, this full-time, two-year MBA program develops leaders who make a difference in the world.
A rigorous, hands-on program that prepares adaptive problem solvers for premier finance careers.
A 12-month program focused on applying the tools of modern data science, optimization and machine learning to solve real-world business problems.
Earn your MBA and SM in engineering with this transformative two-year program.
Combine an international MBA with a deep dive into management science. A special opportunity for partner and affiliate schools only.
A doctoral program that produces outstanding scholars who are leading in their fields of research.
Bring a business perspective to your technical and quantitative expertise with a bachelor’s degree in management, business analytics, or finance.
A joint program for mid-career professionals that integrates engineering and systems thinking. Earn your master’s degree in engineering and management.
An interdisciplinary program that combines engineering, management, and design, leading to a master’s degree in engineering and management.
Executive Programs
A full-time MBA program for mid-career leaders eager to dedicate one year of discovery for a lifetime of impact.
This 20-month MBA program equips experienced executives to enhance their impact on their organizations and the world.
Non-degree programs for senior executives and high-potential managers.
A non-degree, customizable program for mid-career professionals.
Job responsibilities of generative AI leaders
Cybersecurity plans should center on resilience
5 predictions for fintech in 2024
Credit: Andriy Onufriyenko / Getty Images
Ideas Made to Matter
Artificial Intelligence
Machine learning, explained
Apr 21, 2021
Machine learning is behind chatbots and predictive text, language translation apps, the shows Netflix suggests to you, and how your social media feeds are presented. It powers autonomous vehicles and machines that can diagnose medical conditions based on images.
When companies today deploy artificial intelligence programs, they are most likely using machine learning — so much so that the terms are often used interchangeably, and sometimes ambiguously. Machine learning is a subfield of artificial intelligence that gives computers the ability to learn without explicitly being programmed.
“In just the last five or 10 years, machine learning has become a critical way, arguably the most important way, most parts of AI are done,” said MIT Sloan professor Thomas W. Malone, the founding director of the MIT Center for Collective Intelligence . “So that's why some people use the terms AI and machine learning almost as synonymous … most of the current advances in AI have involved machine learning.”
With the growing ubiquity of machine learning, everyone in business is likely to encounter it and will need some working knowledge about this field. A 2020 Deloitte survey found that 67% of companies are using machine learning, and 97% are using or planning to use it in the next year.
From manufacturing to retail and banking to bakeries, even legacy companies are using machine learning to unlock new value or boost efficiency. “Machine learning is changing, or will change, every industry, and leaders need to understand the basic principles, the potential, and the limitations,” said MIT computer science professor Aleksander Madry , director of the MIT Center for Deployable Machine Learning .
While not everyone needs to know the technical details, they should understand what the technology does and what it can and cannot do, Madry added. “I don’t think anyone can afford not to be aware of what’s happening.”
That includes being aware of the social, societal, and ethical implications of machine learning. “It's important to engage and begin to understand these tools, and then think about how you're going to use them well. We have to use these [tools] for the good of everybody,” said Dr. Joan LaRovere , MBA ’16, a pediatric cardiac intensive care physician and co-founder of the nonprofit The Virtue Foundation. “AI has so much potential to do good, and we need to really keep that in our lenses as we're thinking about this. How do we use this to do good and better the world?”
What is machine learning?
Machine learning is a subfield of artificial intelligence, which is broadly defined as the capability of a machine to imitate intelligent human behavior. Artificial intelligence systems are used to perform complex tasks in a way that is similar to how humans solve problems.
The goal of AI is to create computer models that exhibit “intelligent behaviors” like humans, according to Boris Katz , a principal research scientist and head of the InfoLab Group at CSAIL. This means machines that can recognize a visual scene, understand a text written in natural language, or perform an action in the physical world.
Machine learning is one way to use AI. It was defined in the 1950s by AI pioneer Arthur Samuel as “the field of study that gives computers the ability to learn without explicitly being programmed.”
The definition holds true, according to Mikey Shulman, a lecturer at MIT Sloan and head of machine learning at Kensho , which specializes in artificial intelligence for the finance and U.S. intelligence communities. He compared the traditional way of programming computers, or “software 1.0,” to baking, where a recipe calls for precise amounts of ingredients and tells the baker to mix for an exact amount of time. Traditional programming similarly requires creating detailed instructions for the computer to follow.
But in some cases, writing a program for the machine to follow is time-consuming or impossible, such as training a computer to recognize pictures of different people. While humans can do this task easily, it’s difficult to tell a computer how to do it. Machine learning takes the approach of letting computers learn to program themselves through experience.
Machine learning starts with data — numbers, photos, or text, like bank transactions, pictures of people or even bakery items , repair records, time series data from sensors, or sales reports. The data is gathered and prepared to be used as training data, or the information the machine learning model will be trained on. The more data, the better the program.
From there, programmers choose a machine learning model to use, supply the data, and let the computer model train itself to find patterns or make predictions. Over time the human programmer can also tweak the model, including changing its parameters, to help push it toward more accurate results. (Research scientist Janelle Shane’s website AI Weirdness is an entertaining look at how machine learning algorithms learn and how they can get things wrong — as happened when an algorithm tried to generate recipes and created Chocolate Chicken Chicken Cake.)
Some data is held out from the training data to be used as evaluation data, which tests how accurate the machine learning model is when it is shown new data. The result is a model that can be used in the future with different sets of data.
Successful machine learning algorithms can do different things, Malone wrote in a recent research brief about AI and the future of work that was co-authored by MIT professor and CSAIL director Daniela Rus and Robert Laubacher, the associate director of the MIT Center for Collective Intelligence.
“The function of a machine learning system can be descriptive , meaning that the system uses the data to explain what happened; predictive , meaning the system uses the data to predict what will happen; or prescriptive , meaning the system will use the data to make suggestions about what action to take,” the researchers wrote.
There are three subcategories of machine learning:
Supervised machine learning models are trained with labeled data sets, which allow the models to learn and grow more accurate over time. For example, an algorithm would be trained with pictures of dogs and other things, all labeled by humans, and the machine would learn ways to identify pictures of dogs on its own. Supervised machine learning is the most common type used today.
In unsupervised machine learning, a program looks for patterns in unlabeled data. Unsupervised machine learning can find patterns or trends that people aren’t explicitly looking for. For example, an unsupervised machine learning program could look through online sales data and identify different types of clients making purchases.
Reinforcement machine learning trains machines through trial and error to take the best action by establishing a reward system. Reinforcement learning can train models to play games or train autonomous vehicles to drive by telling the machine when it made the right decisions, which helps it learn over time what actions it should take.
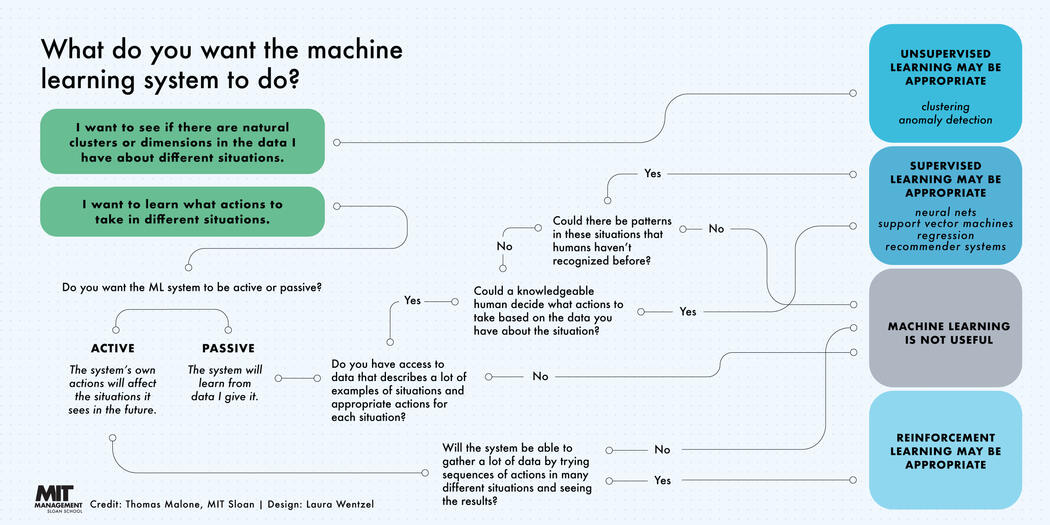
Source: Thomas Malone | MIT Sloan. See: https://bit.ly/3gvRho2, Figure 2.
In the Work of the Future brief, Malone noted that machine learning is best suited for situations with lots of data — thousands or millions of examples, like recordings from previous conversations with customers, sensor logs from machines, or ATM transactions. For example, Google Translate was possible because it “trained” on the vast amount of information on the web, in different languages.
In some cases, machine learning can gain insight or automate decision-making in cases where humans would not be able to, Madry said. “It may not only be more efficient and less costly to have an algorithm do this, but sometimes humans just literally are not able to do it,” he said.
Google search is an example of something that humans can do, but never at the scale and speed at which the Google models are able to show potential answers every time a person types in a query, Malone said. “That’s not an example of computers putting people out of work. It's an example of computers doing things that would not have been remotely economically feasible if they had to be done by humans.”
Machine learning is also associated with several other artificial intelligence subfields:
Natural language processing
Natural language processing is a field of machine learning in which machines learn to understand natural language as spoken and written by humans, instead of the data and numbers normally used to program computers. This allows machines to recognize language, understand it, and respond to it, as well as create new text and translate between languages. Natural language processing enables familiar technology like chatbots and digital assistants like Siri or Alexa.
Neural networks
Neural networks are a commonly used, specific class of machine learning algorithms. Artificial neural networks are modeled on the human brain, in which thousands or millions of processing nodes are interconnected and organized into layers.
In an artificial neural network, cells, or nodes, are connected, with each cell processing inputs and producing an output that is sent to other neurons. Labeled data moves through the nodes, or cells, with each cell performing a different function. In a neural network trained to identify whether a picture contains a cat or not, the different nodes would assess the information and arrive at an output that indicates whether a picture features a cat.
Deep learning
Deep learning networks are neural networks with many layers. The layered network can process extensive amounts of data and determine the “weight” of each link in the network — for example, in an image recognition system, some layers of the neural network might detect individual features of a face, like eyes, nose, or mouth, while another layer would be able to tell whether those features appear in a way that indicates a face.
Like neural networks, deep learning is modeled on the way the human brain works and powers many machine learning uses, like autonomous vehicles, chatbots, and medical diagnostics.
“The more layers you have, the more potential you have for doing complex things well,” Malone said.
Deep learning requires a great deal of computing power, which raises concerns about its economic and environmental sustainability.
How businesses are using machine learning
Machine learning is the core of some companies’ business models, like in the case of Netflix’s suggestions algorithm or Google’s search engine . Other companies are engaging deeply with machine learning, though it’s not their main business proposition.
67% of companies are using machine learning, according to a recent survey.
Others are still trying to determine how to use machine learning in a beneficial way. “In my opinion, one of the hardest problems in machine learning is figuring out what problems I can solve with machine learning,” Shulman said. “There’s still a gap in the understanding.”
In a 2018 paper , researchers from the MIT Initiative on the Digital Economy outlined a 21-question rubric to determine whether a task is suitable for machine learning. The researchers found that no occupation will be untouched by machine learning, but no occupation is likely to be completely taken over by it. The way to unleash machine learning success, the researchers found, was to reorganize jobs into discrete tasks, some which can be done by machine learning, and others that require a human.
Companies are already using machine learning in several ways, including:
Recommendation algorithms. The recommendation engines behind Netflix and YouTube suggestions, what information appears on your Facebook feed, and product recommendations are fueled by machine learning. “[The algorithms] are trying to learn our preferences,” Madry said. “They want to learn, like on Twitter, what tweets we want them to show us, on Facebook, what ads to display, what posts or liked content to share with us.”
Image analysis and object detection. Machine learning can analyze images for different information, like learning to identify people and tell them apart — though facial recognition algorithms are controversial. Business uses for this vary. Shulman noted that hedge funds famously use machine learning to analyze the number of cars in parking lots, which helps them learn how companies are performing and make good bets.
Fraud detection . Machines can analyze patterns, like how someone normally spends or where they normally shop, to identify potentially fraudulent credit card transactions , log-in attempts, or spam emails.
Automatic helplines or chatbots. Many companies are deploying online chatbots, in which customers or clients don’t speak to humans, but instead interact with a machine. These algorithms use machine learning and natural language processing, with the bots learning from records of past conversations to come up with appropriate responses.
Self-driving cars. Much of the technology behind self-driving cars is based on machine learning, deep learning in particular .
Medical imaging and diagnostics. Machine learning programs can be trained to examine medical images or other information and look for certain markers of illness, like a tool that can predict cancer risk based on a mammogram.
Read report: Artificial Intelligence and the Future of Work
How machine learning works: promises and challenges
While machine learning is fueling technology that can help workers or open new possibilities for businesses, there are several things business leaders should know about machine learning and its limits.
Explainability
One area of concern is what some experts call explainability, or the ability to be clear about what the machine learning models are doing and how they make decisions. “Understanding why a model does what it does is actually a very difficult question, and you always have to ask yourself that,” Madry said. “You should never treat this as a black box, that just comes as an oracle … yes, you should use it, but then try to get a feeling of what are the rules of thumb that it came up with? And then validate them.”
Related Articles
This is especially important because systems can be fooled and undermined, or just fail on certain tasks, even those humans can perform easily. For example, adjusting the metadata in images can confuse computers — with a few adjustments, a machine identifies a picture of a dog as an ostrich .
Madry pointed out another example in which a machine learning algorithm examining X-rays seemed to outperform physicians. But it turned out the algorithm was correlating results with the machines that took the image, not necessarily the image itself. Tuberculosis is more common in developing countries, which tend to have older machines. The machine learning program learned that if the X-ray was taken on an older machine, the patient was more likely to have tuberculosis. It completed the task, but not in the way the programmers intended or would find useful.
The importance of explaining how a model is working — and its accuracy — can vary depending on how it’s being used, Shulman said. While most well-posed problems can be solved through machine learning, he said, people should assume right now that the models only perform to about 95% of human accuracy. It might be okay with the programmer and the viewer if an algorithm recommending movies is 95% accurate, but that level of accuracy wouldn’t be enough for a self-driving vehicle or a program designed to find serious flaws in machinery.
Bias and unintended outcomes
Machines are trained by humans, and human biases can be incorporated into algorithms — if biased information, or data that reflects existing inequities, is fed to a machine learning program, the program will learn to replicate it and perpetuate forms of discrimination. Chatbots trained on how people converse on Twitter can pick up on offensive and racist language , for example.
In some cases, machine learning models create or exacerbate social problems. For example, Facebook has used machine learning as a tool to show users ads and content that will interest and engage them — which has led to models showing people extreme content that leads to polarization and the spread of conspiracy theories when people are shown incendiary, partisan, or inaccurate content.
Ways to fight against bias in machine learning including carefully vetting training data and putting organizational support behind ethical artificial intelligence efforts, like making sure your organization embraces human-centered AI , the practice of seeking input from people of different backgrounds, experiences, and lifestyles when designing AI systems. Initiatives working on this issue include the Algorithmic Justice League and The Moral Machine project.
Putting machine learning to work
Shulman said executives tend to struggle with understanding where machine learning can actually add value to their company. What’s gimmicky for one company is core to another, and businesses should avoid trends and find business use cases that work for them.
The way machine learning works for Amazon is probably not going to translate at a car company, Shulman said — while Amazon has found success with voice assistants and voice-operated speakers, that doesn’t mean car companies should prioritize adding speakers to cars. More likely, he said, the car company might find a way to use machine learning on the factory line that saves or makes a great deal of money.
“The field is moving so quickly, and that's awesome, but it makes it hard for executives to make decisions about it and to decide how much resourcing to pour into it,” Shulman said.
It’s also best to avoid looking at machine learning as a solution in search of a problem, Shulman said. Some companies might end up trying to backport machine learning into a business use. Instead of starting with a focus on technology, businesses should start with a focus on a business problem or customer need that could be met with machine learning.
A basic understanding of machine learning is important, LaRovere said, but finding the right machine learning use ultimately rests on people with different expertise working together. “I'm not a data scientist. I'm not doing the actual data engineering work — all the data acquisition, processing, and wrangling to enable machine learning applications — but I understand it well enough to be able to work with those teams to get the answers we need and have the impact we need,” she said. “You really have to work in a team.”
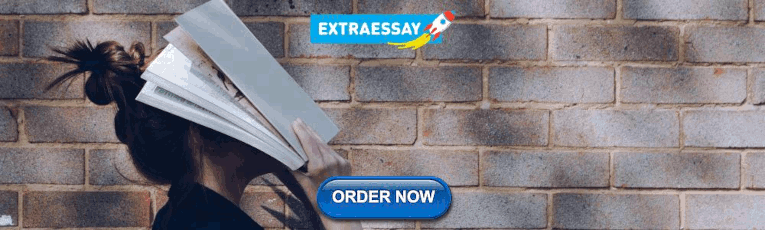
Learn more:
Sign-up for a Machine Learning in Business Course .
Watch an Introduction to Machine Learning through MIT OpenCourseWare .
Read about how an AI pioneer thinks companies can use machine learning to transform .
Watch a discussion with two AI experts about machine learning strides and limitations .
Take a look at the seven steps of machine learning .
Read next: 7 lessons for successful machine learning projects
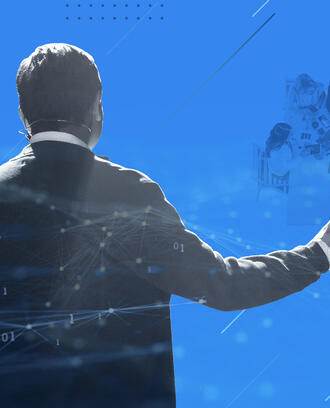
Artificial Intelligence And Machine Learning
Ieee account.
- Change Username/Password
- Update Address
Purchase Details
- Payment Options
- Order History
- View Purchased Documents
Profile Information
- Communications Preferences
- Profession and Education
- Technical Interests
- US & Canada: +1 800 678 4333
- Worldwide: +1 732 981 0060
- Contact & Support
- About IEEE Xplore
- Accessibility
- Terms of Use
- Nondiscrimination Policy
- Privacy & Opting Out of Cookies
A not-for-profit organization, IEEE is the world's largest technical professional organization dedicated to advancing technology for the benefit of humanity. © Copyright 2024 IEEE - All rights reserved. Use of this web site signifies your agreement to the terms and conditions.

An official website of the United States government
The .gov means it’s official. Federal government websites often end in .gov or .mil. Before sharing sensitive information, make sure you’re on a federal government site.
The site is secure. The https:// ensures that you are connecting to the official website and that any information you provide is encrypted and transmitted securely.
- Publications
- Account settings
Preview improvements coming to the PMC website in October 2024. Learn More or Try it out now .
- Advanced Search
- Journal List
- Future Healthc J
- v.8(2); 2021 Jul

Artificial intelligence in healthcare: transforming the practice of medicine
Junaid bajwa.
A Microsoft Research, Cambridge, UK
Usman Munir
B Microsoft Research, Cambridge, UK
Aditya Nori
C Microsoft Research, Cambridge, UK
Bryan Williams
D University College London, London, UK and director, NIHR UCLH Biomedical Research Centre, London, UK
Artificial intelligence (AI) is a powerful and disruptive area of computer science, with the potential to fundamentally transform the practice of medicine and the delivery of healthcare. In this review article, we outline recent breakthroughs in the application of AI in healthcare, describe a roadmap to building effective, reliable and safe AI systems, and discuss the possible future direction of AI augmented healthcare systems.
Introduction
Healthcare systems around the world face significant challenges in achieving the ‘quadruple aim’ for healthcare: improve population health, improve the patient's experience of care, enhance caregiver experience and reduce the rising cost of care. 1–3 Ageing populations, growing burden of chronic diseases and rising costs of healthcare globally are challenging governments, payers, regulators and providers to innovate and transform models of healthcare delivery. Moreover, against a backdrop now catalysed by the global pandemic, healthcare systems find themselves challenged to ‘perform’ (deliver effective, high-quality care) and ‘transform’ care at scale by leveraging real-world data driven insights directly into patient care. The pandemic has also highlighted the shortages in healthcare workforce and inequities in the access to care, previously articulated by The King's Fund and the World Health Organization (Box (Box1 1 ). 4,5
Workforce challenges in the next decade
The application of technology and artificial intelligence (AI) in healthcare has the potential to address some of these supply-and-demand challenges. The increasing availability of multi-modal data (genomics, economic, demographic, clinical and phenotypic) coupled with technology innovations in mobile, internet of things (IoT), computing power and data security herald a moment of convergence between healthcare and technology to fundamentally transform models of healthcare delivery through AI-augmented healthcare systems.
In particular, cloud computing is enabling the transition of effective and safe AI systems into mainstream healthcare delivery. Cloud computing is providing the computing capacity for the analysis of considerably large amounts of data, at higher speeds and lower costs compared with historic ‘on premises’ infrastructure of healthcare organisations. Indeed, we observe that many technology providers are increasingly seeking to partner with healthcare organisations to drive AI-driven medical innovation enabled by cloud computing and technology-related transformation (Box (Box2 2 ). 6–8
Quotes from technology leaders
Here, we summarise recent breakthroughs in the application of AI in healthcare, describe a roadmap to building effective AI systems and discuss the possible future direction of AI augmented healthcare systems.
What is artificial intelligence?
Simply put, AI refers to the science and engineering of making intelligent machines, through algorithms or a set of rules, which the machine follows to mimic human cognitive functions, such as learning and problem solving. 9 AI systems have the potential to anticipate problems or deal with issues as they come up and, as such, operate in an intentional, intelligent and adaptive manner. 10 AI's strength is in its ability to learn and recognise patterns and relationships from large multidimensional and multimodal datasets; for example, AI systems could translate a patient's entire medical record into a single number that represents a likely diagnosis. 11,12 Moreover, AI systems are dynamic and autonomous, learning and adapting as more data become available. 13
AI is not one ubiquitous, universal technology, rather, it represents several subfields (such as machine learning and deep learning) that, individually or in combination, add intelligence to applications. Machine learning (ML) refers to the study of algorithms that allow computer programs to automatically improve through experience. 14 ML itself may be categorised as ‘supervised’, ‘unsupervised’ and ‘reinforcement learning’ (RL), and there is ongoing research in various sub-fields including ‘semi-supervised’, ‘self-supervised’ and ‘multi-instance’ ML.
- Supervised learning leverages labelled data (annotated information); for example, using labelled X-ray images of known tumours to detect tumours in new images. 15
- ‘Unsupervised learning’ attempts to extract information from data without labels; for example, categorising groups of patients with similar symptoms to identify a common cause. 16
- In RL, computational agents learn by trial and error, or by expert demonstration. The algorithm learns by developing a strategy to maximise rewards. Of note, major breakthroughs in AI in recent years have been based on RL.
- Deep learning (DL) is a class of algorithms that learns by using a large, many-layered collection of connected processes and exposing these processors to a vast set of examples. DL has emerged as the predominant method in AI today driving improvements in areas such as image and speech recognition. 17,18
How to build effective and trusted AI-augmented healthcare systems?
Despite more than a decade of significant focus, the use and adoption of AI in clinical practice remains limited, with many AI products for healthcare still at the design and develop stage. 19–22 While there are different ways to build AI systems for healthcare, far too often there are attempts to force square pegs into round holes ie find healthcare problems to apply AI solutions to without due consideration to local context (such as clinical workflows, user needs, trust, safety and ethical implications).
We hold the view that AI amplifies and augments, rather than replaces, human intelligence. Hence, when building AI systems in healthcare, it is key to not replace the important elements of the human interaction in medicine but to focus it, and improve the efficiency and effectiveness of that interaction. Moreover, AI innovations in healthcare will come through an in-depth, human-centred understanding of the complexity of patient journeys and care pathways.
In Fig Fig1, 1 , we describe a problem-driven, human-centred approach, adapted from frameworks by Wiens et al , Care and Sendak to building effective and reliable AI-augmented healthcare systems. 23–25

Multi-step, iterative approach to build effective and reliable AI-augmented systems in healthcare.
Design and develop
The first stage is to design and develop AI solutions for the right problems using a human-centred AI and experimentation approach and engaging appropriate stakeholders, especially the healthcare users themselves.
Stakeholder engagement and co-creation
Build a multidisciplinary team including computer and social scientists, operational and research leadership, and clinical stakeholders (physician, caregivers and patients) and subject experts (eg for biomedical scientists) that would include authorisers, motivators, financiers, conveners, connectors, implementers and champions. 26 A multi-stakeholder team brings the technical, strategic, operational expertise to define problems, goals, success metrics and intermediate milestones.
Human-centred AI
A human-centred AI approach combines an ethnographic understanding of health systems, with AI. Through user-designed research, first understand the key problems (we suggest using a qualitative study design to understand ‘what is the problem’, ‘why is it a problem’, ‘to whom does it matter’, ‘why has it not been addressed before’ and ‘why is it not getting attention’) including the needs, constraints and workflows in healthcare organisations, and the facilitators and barriers to the integration of AI within the clinical context. After defining key problems, the next step is to identify which problems are appropriate for AI to solve, whether there is availability of applicable datasets to build and later evaluate AI. By contextualising algorithms in an existing workflow, AI systems would operate within existing norms and practices to ensure adoption, providing appropriate solutions to existing problems for the end user.
Experimentation
The focus should be on piloting of new stepwise experiments to build AI tools, using tight feedback loops from stakeholders to facilitate rapid experiential learning and incremental changes. 27 The experiments would allow the trying out of new ideas simultaneously, exploring to see which one works, learn what works and what doesn't, and why. 28 Experimentation and feedback will help to elucidate the purpose and intended uses for the AI system: the likely end users and the potential harm and ethical implications of AI system to them (for instance, data privacy, security, equity and safety).
Evaluate and validate
Next, we must iteratively evaluate and validate the predictions made by the AI tool to test how well it is functioning. This is critical, and evaluation is based on three dimensions: statistical validity, clinical utility and economic utility.
- Statistical validity is understanding the performance of AI on metrics of accuracy, reliability, robustness, stability and calibration. High model performance on retrospective, in silico settings is not sufficient to demonstrate clinical utility or impact.
- To determine clinical utility, evaluate the algorithm in a real-time environment on a hold-out and temporal validation set (eg longitudinal and external geographic datasets) to demonstrate clinical effectiveness and generalisability. 25
- Economic utility quantifies the net benefit relative to the cost from the investment in the AI system.
Scale and diffuse
Many AI systems are initially designed to solve a problem at one healthcare system based on the patient population specific to that location and context. Scale up of AI systems requires special attention to deployment modalities, model updates, the regulatory system, variation between systems and reimbursement environment.
Monitor and maintain
Even after an AI system has been deployed clinically, it must be continually monitored and maintained to monitor for risks and adverse events using effective post-market surveillance. Healthcare organisations, regulatory bodies and AI developers should cooperate to collate and analyse the relevant datasets for AI performance, clinical and safety-related risks, and adverse events. 29
What are the current and future use cases of AI in healthcare?
AI can enable healthcare systems to achieve their ‘quadruple aim’ by democratising and standardising a future of connected and AI augmented care, precision diagnostics, precision therapeutics and, ultimately, precision medicine (Table (Table1 1 ). 30 Research in the application of AI healthcare continues to accelerate rapidly, with potential use cases being demonstrated across the healthcare sector (both physical and mental health) including drug discovery, virtual clinical consultation, disease diagnosis, prognosis, medication management and health monitoring.
Widescale adoption and application of artificial intelligence in healthcare
Timings are illustrative to widescale adoption of the proposed innovation taking into account challenges / regulatory environment / use at scale.
We describe a non-exhaustive suite of AI applications in healthcare in the near term, medium term and longer term, for the potential capabilities of AI to augment, automate and transform medicine.
AI today (and in the near future)
Currently, AI systems are not reasoning engines ie cannot reason the same way as human physicians, who can draw upon ‘common sense’ or ‘clinical intuition and experience’. 12 Instead, AI resembles a signal translator, translating patterns from datasets. AI systems today are beginning to be adopted by healthcare organisations to automate time consuming, high volume repetitive tasks. Moreover, there is considerable progress in demonstrating the use of AI in precision diagnostics (eg diabetic retinopathy and radiotherapy planning).
AI in the medium term (the next 5–10 years)
In the medium term, we propose that there will be significant progress in the development of powerful algorithms that are efficient (eg require less data to train), able to use unlabelled data, and can combine disparate structured and unstructured data including imaging, electronic health data, multi-omic, behavioural and pharmacological data. In addition, healthcare organisations and medical practices will evolve from being adopters of AI platforms, to becoming co-innovators with technology partners in the development of novel AI systems for precision therapeutics.
AI in the long term (>10 years)
In the long term, AI systems will become more intelligent , enabling AI healthcare systems achieve a state of precision medicine through AI-augmented healthcare and connected care. Healthcare will shift from the traditional one-size-fits-all form of medicine to a preventative, personalised, data-driven disease management model that achieves improved patient outcomes (improved patient and clinical experiences of care) in a more cost-effective delivery system.
Connected/augmented care
AI could significantly reduce inefficiency in healthcare, improve patient flow and experience, and enhance caregiver experience and patient safety through the care pathway; for example, AI could be applied to the remote monitoring of patients (eg intelligent telehealth through wearables/sensors) to identify and provide timely care of patients at risk of deterioration.
In the long term, we expect that healthcare clinics, hospitals, social care services, patients and caregivers to be all connected to a single, interoperable digital infrastructure using passive sensors in combination with ambient intelligence. 31 Following are two AI applications in connected care.
Virtual assistants and AI chatbots
AI chatbots (such as those used in Babylon ( www.babylonhealth.com ) and Ada ( https://ada.com )) are being used by patients to identify symptoms and recommend further actions in community and primary care settings. AI chatbots can be integrated with wearable devices such as smartwatches to provide insights to both patients and caregivers in improving their behaviour, sleep and general wellness.
Ambient and intelligent care
We also note the emergence of ambient sensing without the need for any peripherals.
- Emerald ( www.emeraldinno.com ): a wireless, touchless sensor and machine learning platform for remote monitoring of sleep, breathing and behaviour, founded by Massachusetts Institute of Technology faculty and researchers.
- Google nest: claiming to monitor sleep (including sleep disturbances like cough) using motion and sound sensors. 32
- A recently published article exploring the ability to use smart speakers to contactlessly monitor heart rhythms. 33
- Automation and ambient clinical intelligence: AI systems leveraging natural language processing (NLP) technology have the potential to automate administrative tasks such as documenting patient visits in electronic health records, optimising clinical workflow and enabling clinicians to focus more time on caring for patients (eg Nuance Dragon Ambient eXperience ( www.nuance.com/healthcare/ambient-clinical-intelligence.html )).
Precision diagnostics
Diagnostic imaging.
The automated classification of medical images is the leading AI application today. A recent review of AI/ML-based medical devices approved in the USA and Europe from 2015–2020 found that more than half (129 (58%) devices in the USA and 126 (53%) devices in Europe) were approved or CE marked for radiological use. 34 Studies have demonstrated AI's ability to meet or exceed the performance of human experts in image-based diagnoses from several medical specialties including pneumonia in radiology (a convolutional neural network trained with labelled frontal chest X-ray images outperformed radiologists in detecting pneumonia), dermatology (a convolutional neural network was trained with clinical images and was found to classify skin lesions accurately), pathology (one study trained AI algorithms with whole-slide pathology images to detect lymph node metastases of breast cancer and compared the results with those of pathologists) and cardiology (a deep learning algorithm diagnosed heart attack with a performance comparable with that of cardiologists). 35–38
We recognise that there are some exemplars in this area in the NHS (eg University of Leeds Virtual Pathology Project and the National Pathology Imaging Co-operative) and expect widescale adoption and scaleup of AI-based diagnostic imaging in the medium term. 39 We provide two use cases of such technologies.
Diabetic retinopathy screening
Key to reducing preventable, diabetes-related vision loss worldwide is screening individuals for detection and the prompt treatment of diabetic retinopathy. However, screening is costly given the substantial number of diabetes patients and limited manpower for eye care worldwide. 40 Research studies on automated AI algorithms for diabetic retinopathy in the USA, Singapore, Thailand and India have demonstrated robust diagnostic performance and cost effectiveness. 41–44 Moreover, Centers for Medicare & Medicaid Services approved Medicare reimbursement for the use of Food and Drug Administration approved AI algorithm ‘IDx-DR’, which demonstrated 87% sensitivity and 90% specificity for detecting more-than-mild diabetic retinopathy. 45
Improving the precision and reducing waiting timings for radiotherapy planning
An important AI application is to assist clinicians for image preparation and planning tasks for radiotherapy cancer treatment. Currently, segmentation of the images is time consuming and laborious task, performed manually by an oncologist using specially designed software to draw contours around the regions of interest. The AI-based InnerEye open-source technology can cut this preparation time for head and neck, and prostate cancer by up to 90%, meaning that waiting times for starting potentially life-saving radiotherapy treatment can be dramatically reduced (Fig (Fig2 2 ). 46,47

Potential applications for the InnerEye deep learning toolkit include quantitative radiology for monitoring tumour progression, planning for surgery and radiotherapy planning. 47
Precision therapeutics
To make progress towards precision therapeutics, we need to considerably improve our understanding of disease. Researchers globally are exploring the cellular and molecular basis of disease, collecting a range of multimodal datasets that can lead to digital and biological biomarkers for diagnosis, severity and progression. Two important future AI applications include immunomics / synthetic biology and drug discovery.
Immunomics and synthetic biology
Through the application of AI tools on multimodal datasets in the future, we may be able to better understand the cellular basis of disease and the clustering of diseases and patient populations to provide more targeted preventive strategies, for example, using immunomics to diagnose and better predict care and treatment options. This will be revolutionary for multiple standards of care, with particular impact in the cancer, neurological and rare disease space, personalising the experience of care for the individual.
AI-driven drug discovery
AI will drive significant improvement in clinical trial design and optimisation of drug manufacturing processes, and, in general, any combinatorial optimisation process in healthcare could be replaced by AI. We have already seen the beginnings of this with the recent announcements by DeepMind and AlphaFold, which now sets the stage for better understanding disease processes, predicting protein structures and developing more targeted therapeutics (for both rare and more common diseases; Fig Fig3 3 ). 48,49

An overview of the main neural network model architecture for AlphaFold. 49 MSA = multiple sequence alignment.
Precision medicine
New curative therapies.
Over the past decade, synthetic biology has produced developments like CRISPR gene editing and some personalised cancer therapies. However, the life cycle for developing such advanced therapies is still extremely inefficient and expensive.
In future, with better access to data (genomic, proteomic, glycomic, metabolomic and bioinformatic), AI will allow us to handle far more systematic complexity and, in turn, help us transform the way we understand, discover and affect biology. This will improve the efficiency of the drug discovery process by helping better predict early which agents are more likely to be effective and also better anticipate adverse drug effects, which have often thwarted the further development of otherwise effective drugs at a costly late stage in the development process. This, in turn will democratise access to novel advanced therapies at a lower cost.
AI empowered healthcare professionals
In the longer term, healthcare professionals will leverage AI in augmenting the care they provide, allowing them to provide safer, standardised and more effective care at the top of their licence; for example, clinicians could use an ‘AI digital consult’ to examine ‘digital twin’ models of their patients (a truly ‘digital and biomedical’ version of a patient), allowing them to ‘test’ the effectiveness, safety and experience of an intervention (such as a cancer drug) in the digital environment prior to delivering the intervention to the patient in the real world.
We recognise that there are significant challenges related to the wider adoption and deployment of AI into healthcare systems. These challenges include, but are not limited to, data quality and access, technical infrastructure, organisational capacity, and ethical and responsible practices in addition to aspects related to safety and regulation. Some of these issues have been covered, but others go beyond the scope of this current article.
Conclusion and key recommendations
Advances in AI have the potential to transform many aspects of healthcare, enabling a future that is more personalised, precise, predictive and portable. It is unclear if we will see an incremental adoption of new technologies or radical adoption of these technological innovations, but the impact of such technologies and the digital renaissance they bring requires health systems to consider how best they will adapt to the changing landscape. For the NHS, the application of such technologies truly has the potential to release time for care back to healthcare professionals, enabling them to focus on what matters to their patients and, in the future, leveraging a globally democratised set of data assets comprising the ‘highest levels of human knowledge’ to ‘work at the limits of science’ to deliver a common high standard of care, wherever and whenever it is delivered, and by whoever. 50 Globally, AI could become a key tool for improving health equity around the world.
As much as the last 10 years have been about the roll out of digitisation of health records for the purposes of efficiency (and in some healthcare systems, billing/reimbursement), the next 10 years will be about the insight and value society can gain from these digital assets, and how these can be translated into driving better clinical outcomes with the assistance of AI, and the subsequent creation of novel data assets and tools. It is clear that we are at an turning point as it relates to the convergence of the practice of medicine and the application of technology, and although there are multiple opportunities, there are formidable challenges that need to be overcome as it relates to the real world and the scale of implementation of such innovation. A key to delivering this vision will be an expansion of translational research in the field of healthcare applications of artificial intelligence. Alongside this, we need investment into the upskilling of a healthcare workforce and future leaders that are digitally enabled, and to understand and embrace, rather than being intimidated by, the potential of an AI-augmented healthcare system.
Healthcare leaders should consider (as a minimum) these issues when planning to leverage AI for health:
- processes for ethical and responsible access to data: healthcare data is highly sensitive, inconsistent, siloed and not optimised for the purposes of machine learning development, evaluation, implementation and adoption
- access to domain expertise / prior knowledge to make sense and create some of the rules which need to be applied to the datasets (to generate the necessary insight)
- access to sufficient computing power to generate decisions in real time, which is being transformed exponentially with the advent of cloud computing
- research into implementation: critically, we must consider, explore and research issues which arise when you take the algorithm and put it in the real world, building ‘trusted’ AI algorithms embedded into appropriate workflows.
Subscribe to the PwC Newsletter
Join the community, trending research, aniportrait: audio-driven synthesis of photorealistic portrait animation.

In this study, we propose AniPortrait, a novel framework for generating high-quality animation driven by audio and a reference portrait image.

Mini-Gemini: Mining the Potential of Multi-modality Vision Language Models
We try to narrow the gap by mining the potential of VLMs for better performance and any-to-any workflow from three aspects, i. e., high-resolution visual tokens, high-quality data, and VLM-guided generation.

Long-form factuality in large language models
google-deepmind/long-form-factuality • 27 Mar 2024
Empirically, we demonstrate that LLM agents can achieve superhuman rating performance - on a set of ~16k individual facts, SAFE agrees with crowdsourced human annotators 72% of the time, and on a random subset of 100 disagreement cases, SAFE wins 76% of the time.
T-Rex2: Towards Generic Object Detection via Text-Visual Prompt Synergy
idea-research/t-rex • 21 Mar 2024
Recognizing the complementary strengths and weaknesses of both text and visual prompts, we introduce T-Rex2 that synergizes both prompts within a single model through contrastive learning.

AIOS: LLM Agent Operating System
agiresearch/aios • 25 Mar 2024
Inspired by these challenges, this paper presents AIOS, an LLM agent operating system, which embeds large language model into operating systems (OS) as the brain of the OS, enabling an operating system "with soul" -- an important step towards AGI.

VoiceCraft: Zero-Shot Speech Editing and Text-to-Speech in the Wild
We introduce VoiceCraft, a token infilling neural codec language model, that achieves state-of-the-art performance on both speech editing and zero-shot text-to-speech (TTS) on audiobooks, internet videos, and podcasts.
Mora: Enabling Generalist Video Generation via A Multi-Agent Framework
lichao-sun/mora • 20 Mar 2024
Sora is the first large-scale generalist video generation model that garnered significant attention across society.
SDXS: Real-Time One-Step Latent Diffusion Models with Image Conditions
IDKiro/sdxs • 25 Mar 2024
Recent advancements in diffusion models have positioned them at the forefront of image generation.

StreamingT2V: Consistent, Dynamic, and Extendable Long Video Generation from Text
picsart-ai-research/streamingt2v • 21 Mar 2024
To overcome these limitations, we introduce StreamingT2V, an autoregressive approach for long video generation of 80, 240, 600, 1200 or more frames with smooth transitions.
General Object Foundation Model for Images and Videos at Scale
We present GLEE in this work, an object-level foundation model for locating and identifying objects in images and videos.

Artificial Intelligence Essay for Students and Children
500+ words essay on artificial intelligence.
Artificial Intelligence refers to the intelligence of machines. This is in contrast to the natural intelligence of humans and animals. With Artificial Intelligence, machines perform functions such as learning, planning, reasoning and problem-solving. Most noteworthy, Artificial Intelligence is the simulation of human intelligence by machines. It is probably the fastest-growing development in the World of technology and innovation . Furthermore, many experts believe AI could solve major challenges and crisis situations.

Types of Artificial Intelligence
First of all, the categorization of Artificial Intelligence is into four types. Arend Hintze came up with this categorization. The categories are as follows:
Type 1: Reactive machines – These machines can react to situations. A famous example can be Deep Blue, the IBM chess program. Most noteworthy, the chess program won against Garry Kasparov , the popular chess legend. Furthermore, such machines lack memory. These machines certainly cannot use past experiences to inform future ones. It analyses all possible alternatives and chooses the best one.
Type 2: Limited memory – These AI systems are capable of using past experiences to inform future ones. A good example can be self-driving cars. Such cars have decision making systems . The car makes actions like changing lanes. Most noteworthy, these actions come from observations. There is no permanent storage of these observations.
Type 3: Theory of mind – This refers to understand others. Above all, this means to understand that others have their beliefs, intentions, desires, and opinions. However, this type of AI does not exist yet.
Type 4: Self-awareness – This is the highest and most sophisticated level of Artificial Intelligence. Such systems have a sense of self. Furthermore, they have awareness, consciousness, and emotions. Obviously, such type of technology does not yet exist. This technology would certainly be a revolution .
Get the huge list of more than 500 Essay Topics and Ideas
Applications of Artificial Intelligence
First of all, AI has significant use in healthcare. Companies are trying to develop technologies for quick diagnosis. Artificial Intelligence would efficiently operate on patients without human supervision. Such technological surgeries are already taking place. Another excellent healthcare technology is IBM Watson.
Artificial Intelligence in business would significantly save time and effort. There is an application of robotic automation to human business tasks. Furthermore, Machine learning algorithms help in better serving customers. Chatbots provide immediate response and service to customers.

AI can greatly increase the rate of work in manufacturing. Manufacture of a huge number of products can take place with AI. Furthermore, the entire production process can take place without human intervention. Hence, a lot of time and effort is saved.
Artificial Intelligence has applications in various other fields. These fields can be military , law , video games , government, finance, automotive, audit, art, etc. Hence, it’s clear that AI has a massive amount of different applications.
To sum it up, Artificial Intelligence looks all set to be the future of the World. Experts believe AI would certainly become a part and parcel of human life soon. AI would completely change the way we view our World. With Artificial Intelligence, the future seems intriguing and exciting.
{ “@context”: “https://schema.org”, “@type”: “FAQPage”, “mainEntity”: [{ “@type”: “Question”, “name”: “Give an example of AI reactive machines?”, “acceptedAnswer”: { “@type”: “Answer”, “text”: “Reactive machines react to situations. An example of it is the Deep Blue, the IBM chess program, This program defeated the popular chess player Garry Kasparov.” } }, { “@type”: “Question”, “name”: “How do chatbots help in business?”, “acceptedAnswer”: { “@type”: “Answer”, “text”:”Chatbots help in business by assisting customers. Above all, they do this by providing immediate response and service to customers.”} }] }
Customize your course in 30 seconds
Which class are you in.

- Travelling Essay
- Picnic Essay
- Our Country Essay
- My Parents Essay
- Essay on Favourite Personality
- Essay on Memorable Day of My Life
- Essay on Knowledge is Power
- Essay on Gurpurab
- Essay on My Favourite Season
- Essay on Types of Sports
Leave a Reply Cancel reply
Your email address will not be published. Required fields are marked *
Download the App

Thank you for visiting nature.com. You are using a browser version with limited support for CSS. To obtain the best experience, we recommend you use a more up to date browser (or turn off compatibility mode in Internet Explorer). In the meantime, to ensure continued support, we are displaying the site without styles and JavaScript.
- View all journals
- Explore content
- About the journal
- Publish with us
- Sign up for alerts
- Perspective
- Published: 22 March 2024
A collective AI via lifelong learning and sharing at the edge
- Andrea Soltoggio ORCID: orcid.org/0000-0002-9750-8358 1 ,
- Eseoghene Ben-Iwhiwhu ORCID: orcid.org/0000-0002-1176-866X 1 ,
- Vladimir Braverman 2 ,
- Eric Eaton 3 ,
- Benjamin Epstein 4 ,
- Yunhao Ge 5 ,
- Lucy Halperin 6 ,
- Jonathan How 6 ,
- Laurent Itti 5 ,
- Michael A. Jacobs ORCID: orcid.org/0000-0002-1125-1644 2 , 7 , 8 ,
- Pavan Kantharaju 9 ,
- Long Le ORCID: orcid.org/0000-0002-8581-6601 3 ,
- Steven Lee 10 ,
- Xinran Liu 11 ,
- Sildomar T. Monteiro ORCID: orcid.org/0000-0001-7694-9536 10 , 12 ,
- David Musliner 9 ,
- Saptarshi Nath ORCID: orcid.org/0009-0000-9023-5345 1 ,
- Priyadarshini Panda 13 ,
- Christos Peridis 1 ,
- Hamed Pirsiavash 14 ,
- Vishwa Parekh 15 ,
- Kaushik Roy ORCID: orcid.org/0000-0002-0735-9695 16 ,
- Shahaf Shperberg 17 ,
- Hava T. Siegelmann ORCID: orcid.org/0000-0003-4938-8723 18 ,
- Peter Stone ORCID: orcid.org/0000-0002-6795-420X 19 , 20 ,
- Kyle Vedder 3 ,
- Jingfeng Wu ORCID: orcid.org/0009-0009-3414-4487 21 ,
- Lin Yang 22 ,
- Guangyao Zheng 2 &
- Soheil Kolouri 11
Nature Machine Intelligence volume 6 , pages 251–264 ( 2024 ) Cite this article
995 Accesses
1259 Altmetric
Metrics details
- Computational science
- Computer science
One vision of a future artificial intelligence (AI) is where many separate units can learn independently over a lifetime and share their knowledge with each other. The synergy between lifelong learning and sharing has the potential to create a society of AI systems, as each individual unit can contribute to and benefit from the collective knowledge. Essential to this vision are the abilities to learn multiple skills incrementally during a lifetime, to exchange knowledge among units via a common language, to use both local data and communication to learn, and to rely on edge devices to host the necessary decentralized computation and data. The result is a network of agents that can quickly respond to and learn new tasks, that collectively hold more knowledge than a single agent and that can extend current knowledge in more diverse ways than a single agent. Open research questions include when and what knowledge should be shared to maximize both the rate of learning and the long-term learning performance. Here we review recent machine learning advances converging towards creating a collective machine-learned intelligence. We propose that the convergence of such scientific and technological advances will lead to the emergence of new types of scalable, resilient and sustainable AI systems.
This is a preview of subscription content, access via your institution
Access options
Access Nature and 54 other Nature Portfolio journals
Get Nature+, our best-value online-access subscription
24,99 € / 30 days
cancel any time
Subscribe to this journal
Receive 12 digital issues and online access to articles
111,21 € per year
only 9,27 € per issue
Rent or buy this article
Prices vary by article type
Prices may be subject to local taxes which are calculated during checkout
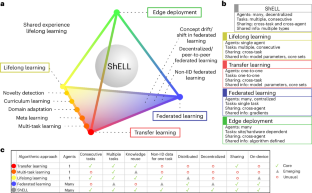
Similar content being viewed by others
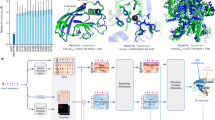
Highly accurate protein structure prediction with AlphaFold
John Jumper, Richard Evans, … Demis Hassabis
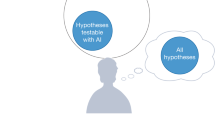
Artificial intelligence and illusions of understanding in scientific research
Lisa Messeri & M. J. Crockett
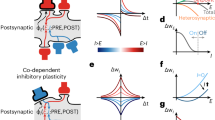
Co-dependent excitatory and inhibitory plasticity accounts for quick, stable and long-lasting memories in biological networks
Everton J. Agnes & Tim P. Vogels
Fagan, M. Collective scientific knowledge. Philos. Compass 7 , 821–831 (2012).
Article Google Scholar
Csibra, G. & Gergely, G. Natural pedagogy as evolutionary adaptation. Phil. Trans. R. Soc. B 366 , 1149–1157 (2011).
Article PubMed PubMed Central Google Scholar
Wooldridge, M. & Jennings, N. R. Intelligent agents: theory and practice. Knowl. Eng. Rev. 10 , 115–152 (1995).
Ferber, J. & Weiss, G. Multi-Agent Systems: An Introduction to Distributed Artificial Intelligence Vol. 1 (Addison-Wesley, 1999).
Stone, P. & Veloso, M. Multiagent systems: a survey from a machine learning perspective. Auton. Rob. 8 , 345–383 (2000).
Conitzer, V. & Oesterheld, C. Foundations of cooperative AI. In Proc. AAAI Conference on Artificial Intelligence Vol. 37, 15359–15367 (AAAI, 2022).
Semsar-Kazerooni, E. & Khorasani, K. Multi-agent team cooperation: a game theory approach. Automatica 45 , 2205–2213 (2009).
Article MathSciNet Google Scholar
Thrun, S. Is learning the n -th thing any easier than learning the first? In Advances in Neural Information Processing Systems Vol. 8 (1995).
Thrun, S. Lifelong learning algorithms. Learning to Learn 8 , 181–209 (1998).
Chen, Z. & Liu, B. Lifelong Machine Learning Vol. 1 (Springer, 2018).
Kudithipudi, D. et al. Biological underpinnings for lifelong learning machines. Nat. Mach. Intell. 4 , 196–210 (2022).
Mundt, M., Hong, Y., Pliushch, I. & Ramesh, V. A wholistic view of continual learning with deep neural networks: forgotten lessons and the bridge to active and open world learning. Neural Networks 160 , 306–336 (2023).
Article PubMed Google Scholar
Khetarpal, K., Riemer, M., Rish, I. & Precup, D. Towards continual reinforcement learning: a review and perspectives. J. Artif. Intell. Res. 75 , 1401–1476 (2022).
Mendez, J. A., van Seijen, H. & Eaton, E. Modular lifelong reinforcement learning via neural composition. In International Conference on Learning Representations (2022).
Li, T., Sahu, A. K., Talwalkar, A. & Smith, V. Federated learning: challenges, methods, and future directions. IEEE Signal Process. Mag. 37 , 50–60 (2020).
CAS Google Scholar
Dorri, A., Kanhere, S. S. & Jurdak, R. Multi-agent systems: a survey. IEEE Access 6 , 28573–28593 (2018).
Shi, W., Cao, J., Zhang, Q., Li, Y. & Xu, L. Edge computing: vision and challenges. IEEE Internet Things J. 3 , 637–646 (2016).
Cai, H. et al. Enable deep learning on mobile devices: methods, systems, and applications. ACM Trans. Des. Autom. Electron. Syst. 27 , 20 (2022).
Shared-Experience Lifelong Learning (ShELL). Opportunity DARPA-PA-20-02-11. SAM.gov https://sam.gov/opp/1afbf600f2e04b26941fad352c08d1f1/view (accessed 10 October 2023).
Smith, P. et al. Network resilience: a systematic approach. IEEE Commun. Mag. 49 , 88–97 (2011).
Zhang, J., Cheung, B., Finn, C., Levine, S. & Jayaraman, D. Cautious adaptation for reinforcement learning in safety-critical settings. In International Conference on Machine Learning 11055–11065 (PMLR, 2020).
McMahan, B., Moore, E., Ramage, D., Hampson, S. & Arcas, B. A. Y. Communication-efficient learning of deep networks from decentralized data. In Artificial Intelligence and Statistics 1273–1282, (PMLR, 2017).
Liu, J. et al. From distributed machine learning to federated learning: a survey. Knowl. Inf. Syst. 64 , 885–917 (2022).
Verbraeken, J. et al. A survey on distributed machine learning. ACM Comput. Surv. 53 , 30 (2020).
Google Scholar
Henderson, P. et al. Towards the systematic reporting of the energy and carbon footprints of machine learning. J. Mach. Learn. Res. 21 , 10039–10081 (2020).
MathSciNet Google Scholar
de Vries, A. The growing energy footprint of artificial intelligence. Joule 7 , 2191–2194 (2023).
Silver, D. L., Yang, Q. & Li, L. Lifelong machine learning systems: beyond learning algorithms. In 2013 AAAI Spring Symposium Series (AAAI, 2013).
Hadsell, R., Rao, D., Rusu, A. A. & Pascanu, R. Embracing change: continual learning in deep neural networks. Trends Cognit. Sci. 24 , 1028–1040 (2020).
French, R. M. Catastrophic forgetting in connectionist networks. Trends Cognit. Sci. 3 , 128–135 (1999).
Article CAS Google Scholar
Kirkpatrick, J. et al. Overcoming catastrophic forgetting in neural networks. Proc. Natl Acad. Sci. USA 114 , 3521–3526 (2017).
Article ADS MathSciNet CAS PubMed PubMed Central Google Scholar
Parisi, G. I., Kemker, R., Part, J. L., Kanan, C. & Wermter, S. Continual lifelong learning with neural networks: a review. Neural Networks 113 , 54–71 (2018).
Lange, M. D. et al. A continual learning survey: defying forgetting in classification tasks. IEEE Trans. Pattern Anal. Mach. Intell. 44 , 3366–3385 (2022).
PubMed Google Scholar
van de Ven, G. M., Tuytelaars, T. & Tolias, A. S. Three types of incremental learning. Nat. Mach. Intell. 4 , 1185–1197 (2022).
Soltoggio, A., Stanley, K. O. & Risi, S. Born to learn: the inspiration, progress, and future of evolved plastic artificial neural networks. Neural Networks 108 , 48–67 (2018).
Lifelong learning machines (L2M). DARPA https://www.darpa.mil/news-events/2017-03-16 (accessed 10 October 2023).
New, A., Baker, M., Nguyen, E. & Vallabha, G. Lifelong learning metrics. Preprint at https://doi.org/10.48550/arXiv.2201.08278 (2022).
Baker, M. M. et al. A domain-agnostic approach for characterization of lifelong learning systems. Neural Networks 160 , 274–296 (2023).
Mendez, J. A. & Eaton, E. Lifelong learning of compositional structures. In International Conference on Learning Representations (2021).
Xie, A. & Finn, C. Lifelong robotic reinforcement learning by retaining experiences. In Conference on Lifelong Learning Agents 838–855 (PMLR, 2022).
Ben-Iwhiwhu, E., Nath, S., Pilly, P. K., Kolouri, S. & Soltoggio, A. Lifelong reinforcement learning with modulating masks. In Transactions on Machine Learning Research (2023).
Tasse, G. N., James, S. & Rosman, B. Generalisation in lifelong reinforcement learning through logical composition. In International Conference on Learning Representations (2022).
Merenda, M., Porcaro, C. & Iero, D. Edge machine learning for AI-enabled IoT devices: a review. Sensors 20 , 2533 (2020).
Article ADS PubMed PubMed Central Google Scholar
Sipola, T., Alatalo, J., Kokkonen, T. & Rantonen, M. Artificial intelligence in the IoT era: a review of edge AI hardware and software. In 2022 31st Conference of Open Innovations Association (FRUCT) 320–331 (IEEE, 2022).
Prabhu, A. et al. Computationally budgeted continual learning: What does matter? In Proc. IEEE/CVF Conference on Computer Vision and Pattern Recognition 3698–3707 (2023).
Díaz-Rodríguez, N., Lomonaco, V., Filliat, D. & Maltoni, D. Don’t forget, there is more than forgetting: new metrics for continual learning. Preprint at https://doi.org/10.48550/arXiv.1810.13166 (2018).
De Lange, M., van de Ven, G. & Tuytelaars, T. Continual evaluation for lifelong learning: identifying the stability gap. In 11th International Conference on Learning Representations https://openreview.net/forum?id=Zy350cRstc6 (ICLR, 2023).
Ghunaim, Y. et al. Real-time evaluation in online continual learning: a new hope. In Proc. IEEE/CVF Conference on Computer Vision and Pattern Recognition 11888–11897 (2023).
Sarker, I. H. Machine learning: algorithms, real-world applications and research directions. SN Comput. Sci. 2 , 160 (2021).
Tsuda, B., Tye, K. M., Siegelmann, H. T. & Sejnowski, T. J. A modeling framework for adaptive lifelong learning with transfer and savings through gating in the prefrontal cortex. Proc. Natl Acad. Sci. USA 117 , 29872–29882 (2020).
Article ADS CAS PubMed PubMed Central Google Scholar
Kairouz, P. et al. Advances and open problems in federated learning. Found. Trends Mach. Learn. 14 , 1–210 (2021).
Zhu, H., Xu, J., Liu, S. & Jin, Y. Federated learning on non-IID data: a survey. Neuropcomputing 465 , 371–390 (2021).
Nguyen, D. C. et al. Federated learning for internet of things: a comprehensive survey. IEEE Commun. Surv. Tutorials 23 , 1622–1658 (2021).
Abreha, H. G., Hayajneh, M. & Serhani, M. A. Federated learning in edge computing: a systematic survey. Sensors 22 , 450 (2022).
Guo, Y., Lin, T. & Tang, X. Towards federated learning on time-evolving heterogeneous data. Preprint at https://doi.org/10.48550/arXiv.2112.13246 (2021).
Criado, M. F., Casado, F. E., Iglesias, R., Regueiro, C. V. & Barro, S. Non-IID data and continual learning processes in federated learning: a long road ahead. Inf. Fusion 88 , 263–280 (2022).
Gao, L. et al. FedDC: federated learning with non-IID data via local drift decoupling and correction. In Proc. IEEE/CVF Conference on Computer Vision and Pattern Recognition (CVPR) 10112–10121 (2022).
Yoon, J., Jeong, W., Lee, G., Yang, E. & Hwang, S. J. Federated continual learning with weighted inter-client transfer. In International Conference on Machine Learning 12073–12086 (PMLR, 2021).
Pellegrini, L., Lomonaco, V., Graffieti, G. & Maltoni, D. Continual learning at the edge: real-time training on smartphone devices. In Proc. European Symposium on Artificial Neural Networks https://doi.org/10.14428/esann/2021.ES2021-136 (2021).
Gao, D. et al. Rethinking pruning for accelerating deep inference at the edge. In Proc. 26th ACM SIGKDD International Conference on Knowledge Discovery and Data Mining 155–164 (2020).
Huang, W., Ye, M. & Du, B. Learn from others and be yourself in heterogeneous federated learning. In Proc. IEEE/CVF Conference on Computer Vision and Pattern Recognition (CVPR) 10143–10153 (2022).
Sun, T., Li, D. & Wang, B. Decentralized federated averaging. IEEE Trans. Pattern Anal. Mach. Intell. 45 , 4289–4301 (2023).
Taylor, M. E. & Stone, P. Transfer learning for reinforcement learning domains: a survey. J. Mach. Learn. Res. 10 , 1633–1685 (2009).
Zamir, A. R. et al. Taskonomy: disentangling task transfer learning. In Proc. IEEE Conference on Computer Vision and Pattern Recognition (2018).
Zhuang, F. et al. A comprehensive survey on transfer learning. Proc. IEEE 109 , 43–76 (2020).
Ding, N. et al. Parameter-efficient fine-tuning of large-scale pre-trained language models. Nat. Mach. Intell. 5 , 220–235 (2023).
Koohpayegani, S. A., Navaneet, K., Nooralinejad, P., Kolouri, S. & Pirsiavash, H. NOLA: networks as linear combination of low rank random basis. In International Conference on Learning Representations (ICLR, 2024).
Wang, M. & Deng, W. Deep visual domain adaptation: a survey. Neurocomputing 312 , 135–153 (2018).
Wilson, G. & Cook, D. J. A survey of unsupervised deep domain adaptation. ACM Trans. Intell. Syst. Technol. 11 , 51 (2020).
Farahani, A., Voghoei, S., Rasheed, K. & Arabnia, H. R. A brief review of domain adaptation. In Advances in Data Science and Information Engineering: Proceedings from ICDATA 2020 and IKE 2020 877–894 (2021).
Kim, Y., Cho, D., Han, K., Panda, P. & Hong, S. Domain adaptation without source data. IEEE Trans. Artif. Intell. 2 , 508–518 (2021).
Caruana, R. Multitask learning. Mach. Learn. 28 , 41–75 (1997).
Luong, M.-T., Le, Q. V., Sutskever, I., Vinyals, O. & Kaiser, L. Multi-task sequence to sequence learning. Preprint at https://doi.org/10.48550/arXiv.1511.06114 (2015).
Ruder, S. An overview of multi-task learning in deep neural networks. Preprint at https://doi.org/10.48550/arXiv.1706.05098 (2017).
Liu, X., He, P., Chen, W. & Gao, J. Multi-task deep neural networks for natural language understanding. In Proc. 57th Annual Meeting of the Association for Computational Linguistics (2019).
Hospedales, T., Antoniou, A., Micaelli, P. & Storkey, A. Meta-learning in neural networks: a survey. IEEE Trans. Pattern Anal. Mach. Intell. 44 , 5149–5169 (2021).
Kayaalp, M., Vlaski, S. & Sayed, A. H. Dif-MAML: decentralized multi-agent meta-learning. IEEE Open J. Signal Process. 3 , 71–93 (2022).
Riemer, M. et al. Learning to learn without forgetting by maximizing transfer and minimizing interference. In 7th International Conference on Learning Representations, ICLR 2019 (OpenReview, 2019).
Bengio, Y., Louradour, J., Collobert, R. & Weston, J. Curriculum learning. In Proc. 26th Annual International Conference on Machine Learning 41–48 (2009).
Narvekar, S. et al. Curriculum learning for reinforcement learning domains: a framework and survey. J. Mach. Learn. Res. 21 , 7382–7431 (2020).
Wang, W., Zheng, V. W., Yu, H. & Miao, C. A survey of zero-shot learning: settings, methods, and applications. ACM Trans. Intell. Syst. Technol. 10 , 13 (2019).
Rostami, M., Isele, D. & Eaton, E. Using task descriptions in lifelong machine learning for improved performance and zero-shot transfer. J. Artif. Intell. Res. 67 , 673–704 (2020).
Chen, J. et al. Knowledge-aware zero-shot learning: survey and perspective. In Proc. 30th International Joint Conference on Artificial Intelligence (IJCAI-21) (2021).
Xie, G.-S., Zhang, Z., Xiong, H., Shao, L. & Li, X. Towards zero-shot learning: a brief review and an attention-based embedding network. IEEE Trans. Circuits Syst. Video Technol. 33 , 1181–1197 (2022).
Cao, W. et al. A review on multimodal zero-shot learning. Wiley Interdiscip. Rev. Data Min. Knowl. Discovery 13 , e1488 (2023).
Jones, A. M. et al. USC-DCT: a collection of diverse classification tasks. Data 8 , 153 (2023).
Liu, X., Bai, Y., Lu, Y., Soltoggio, A. & Kolouri, S. Wasserstein task embedding for measuring task similarities. Preprint at https://doi.org/10.48550/arXiv.2208.11726 (2022).
Yang, J., Zhou, K., Li, Y. & Liu, Z. Generalized out-of-distribution detection: a survey. Preprint at https://doi.org/10.48550/arXiv.2110.11334 (2021).
Abdar, M. et al. A review of uncertainty quantification in deep learning: techniques, applications and challenges. Inf. Fusion 76 , 243–297 (2021).
Musliner, D. J. et al. OpenMIND: planning and adapting in domains with novelty. In Proc. 9th Conference on Advances in Cognitive Systems (2021).
Rios, A. & Itti, L. Lifelong learning without a task oracle. In 2020 IEEE 32nd International Conference on Tools with Artificial Intelligence 255–263 (IEEE, 2020).
Carvalho, D. V., Pereira, E. M. & Cardoso, J. S. Machine learning interpretability: a survey on methods and metrics. Electronics 8 , 832 (2019).
Masana, M. et al. Class-incremental learning: survey and performance evaluation on image classification. IEEE Trans. Pattern Anal. Mach. Intell. 45 , 5513–5533 (2022).
Isele, D. & Cosgun, A. Selective experience replay for lifelong learning. In Proc. AAAI Conference on Artificial Intelligence Vol. 32 (2018).
Nath, S. et al. Sharing lifelong reinforcement learning knowledge via modulating masks. In Proc. of Machine Learning Research Vol. 232 (2023).
Pimentel, M. A., Clifton, D. A., Clifton, L. & Tarassenko, L. A review of novelty detection. Signal Process. 99 , 215–249 (2014).
Da Silva, B. C., Basso, E. W., Bazzan, A. L. & Engel, P. M. Dealing with non-stationary environments using context detection. In Proc. 23rd International Conference on Machine Learning 217–224 (2006).
Niv, Y. Learning task-state representations. Nat. Neurosci. 22 , 1544–1553 (2019).
Article CAS PubMed PubMed Central Google Scholar
Mendez, J. & Eaton, E. How to reuse and compose knowledge for a lifetime of tasks: a survey on continual learning and functional composition. In Transactions on Machine Learning Research (2023).
Hu, E. J. et al. LoRA: low-rank adaptation of large language models. International Conference on Learning Representations (ICLR) (2021).
Nooralinejad, P. et al. PRANC: pseudo random networks for compacting deep models. In Proc. IEEE/CVF International Conference on Computer Vision 17021–17031 (2023).
Lester, B., Al-Rfou, R. & Constant, N. The power of scale for parameter-efficient prompt tuning. In Proc. 2021 Conference on Empirical Methods in Natural Language Processing (2021).
Ge, Y. et al. Lightweight learner for shared knowledge lifelong learning. In Transactions on Machine Learning Research (2023).
Ge, Y. et al. CLR: Channel-wise lightweight reprogramming for continual learning. In Proc. IEEE/CVF International Conference on Computer Vision 18798–18808 (2023).
Sarker, M. K., Zhou, L., Eberhart, A. & Hitzler, P. Neuro-symbolic artificial intelligence. AI Commun. 34 , 197–209 (2021).
Zoph, B. & Le, Q. Neural architecture search with reinforcement learning. In International Conference on Learning Representations (2017).
Ren, P. et al. A comprehensive survey of neural architecture search: challenges and solutions. ACM Comput. Surv. 54 , 76 (2021).
Zhang, C., Patras, P. & Haddadi, H. Deep learning in mobile and wireless networking: a survey. IEEE Commun. Surv. Tutorials 21 , 2224–2287 (2019).
Deng, S. et al. Edge intelligence: the confluence of edge computing and artificial intelligence. IEEE Internet Things J. 7 , 7457–7469 (2020).
Murshed, M. S. et al. Machine learning at the network edge: a survey. ACM Comput. Surv. 54 , 170 (2021).
Ajani, T. S., Imoize, A. L. & Atayero, A. A. An overview of machine learning within embedded and mobile devices–optimizations and applications. Sensors 21 , 4412 (2021).
Dhar, S. et al. A survey of on-device machine learning: an algorithms and learning theory perspective. ACM Trans. Internet Things 2 , 15 (2021).
Singh, R. & Gill, S. S. Edge AI: a survey. Internet Things Cyber-Phys. Syst. 3 , 71–92 (2023).
Mao, Y., You, C., Zhang, J., Huang, K. & Letaief, K. B. A survey on mobile edge computing: the communication perspective. IEEE Commun. Surv. Tutorials 19 , 2322–2358 (2017).
Xu, D. et al. Edge intelligence: architectures, challenges, and applications. Preprint at https://doi.org/10.48550/arXiv.2003.12172 (2020).
Li, E., Zeng, L., Zhou, Z. & Chen, X. Edge AI: on-demand accelerating deep neural network inference via edge computing. IEEE Trans. Wireless Commun. 19 , 447–457 (2019).
Mehlin, V., Schacht, S. & Lanquillon, C. Towards energy-efficient deep learning: an overview of energy-efficient approaches along the deep learning lifecycle. Preprint at https://doi.org/10.48550/arXiv.2303.01980 (2023).
Lin, J. et al. On-device training under 256kb memory. 36th Conference on Neural Information Processing Systems (NeurIPS)(2022).
Yang, Y., Li, G. & Marculescu, R. Efficient on-device training via gradient filtering. In Proc. IEEE/CVF Conference on Computer Vision and Pattern Recognition 3811–3820 (2023).
Hayes, T. L. & Kanan, C. Online continual learning for embedded devices. In Proc. First Conference on Lifelong Learning Agents (eds Chandar, S. et al.) 744–766 (PMLR, 2022).
Wang, Z. et al. SparCL: sparse continual learning on the edge. In 36th Conference on Neural Information Processing Systems (2022).
Harun, M. Y., Gallardo, J., Hayes, T. L. & Kanan, C. How efficient are today’s continual learning algorithms? In Proc. IEEE/CVF Conference on Computer Vision and Pattern Recognition 2430–2435 (2023).
Yang, J. et al. Quantization networks. In Proc. IEEE/CVF Conference on Computer Vision and Pattern Recognition 7308–7316 (2019).
Cai, Z., He, X., Sun, J. & Vasconcelos, N. Deep learning with low precision by half-wave gaussian quantization. In Proc. IEEE Conference on Computer Vision and Pattern Recognition 5918–5926 (2017).
Jain, A., Bhattacharya, S., Masuda, M., Sharma, V. & Wang, Y. Efficient execution of quantized deep learning models: a compiler approach. Preprint at https://doi.org/10.48550/arXiv.2006.10226 (2020).
Goel, A., Tung, C., Lu, Y.-H. & Thiruvathukal, G. K. A survey of methods for low-power deep learning and computer vision. In 2020 IEEE 6th World Forum on Internet of Things (IEEE, 2020).
Ma, X. et al. Cost-effective on-device continual learning over memory hierarchy with Miro. In Proc. 29th Annual International Conference on Mobile Computing and Networking 83, 1–15 (ACM, 2023).
Kudithipudi, D. et al. Design principles for lifelong learning AI accelerators. Nat. Electron. 6 , 807–822 (2023).
Machupalli, R., Hossain, M. & Mandal, M. Review of ASIC accelerators for deep neural network. Microprocess. Microsyst. 89 , 104441 (2022).
Jouppi, P. N. et al. In-datacenter performance analysis of a tensor processing unit. In Proc. 44th Annual International Symposium on Computer Architecture (2017).
Sebastian, A., Le Gallo, M., Khaddam-Aljameh, R. & Eleftheriou, E. Memory devices and applications for in-memory computing. Nat. Nanotechnol. 15 , 529–544 (2020).
Article ADS CAS PubMed Google Scholar
Tang, K.-T. et al. Considerations of integrating computing-in-memory and processing-in-sensor into convolutional neural network accelerators for low-power edge devices. In 2019 Symposium on VLSI Circuits T166–T167 (IEEE, 2019).
Roy, K., Jaiswal, A. & Panda, P. Towards spike-based machine intelligence with neuromorphic computing. Nature 575 , 607–617 (2019).
Chakraborty, I., Jaiswal, A., Saha, A., Gupta, S. & Roy, K. Pathways to efficient neuromorphic computing with non-volatile memory technologies. Appl. Phys. Rev. 7 , 021308 (2020).
Article ADS CAS Google Scholar
Christensen, D. V. et al. 2022 roadmap on neuromorphic computing and engineering. Neuromorph. Comput. Eng. 2 , 022501 (2022).
Rathi, N. et al. Exploring neuromorphic computing based on spiking neural networks: algorithms to hardware. ACM Comput. Surv. 55 , 243 (2023).
Zhang, W. et al. Neuro-inspired computing chips. Nat. Electron. 3 , 371–382 (2020).
Article ADS Google Scholar
Feldmann, J. et al. Parallel convolutional processing using an integrated photonic tensor core. Nature 589 , 52–58 (2021).
Peserico, N., Shastri, B. J. & Sorger, V. J. Integrated photonic tensor processing unit for a matrix multiply: a review. J. Lightwave Technol. 41 , 3704–3716 (2023).
Shastri, B. J. et al. Photonics for artificial intelligence and neuromorphic computing. Nat. Photonics 15 , 102–114 (2021).
Toczé, K. & Nadjm-Tehrani, S. A taxonomy for management and optimization of multiple resources in edge computing. Wireless Commun. Mobile Comput. 2018 , 7476201 (2018).
Bhattacharjee, A., Venkatesha, Y., Moitra, A. & Panda, P. MIME: adapting a single neural network for multi-task inference with memory-efficient dynamic pruning. In Proc. 59th ACM/IEEE Design Automation Conference 499–504 (2022).
Extreme Computing BAA. DARPA https://sam.gov/opp/211b1819bd5f46eba20d4a466358d8bb/view (accessed 10 October 2023).
Rostami, M., Kolouri, S., Kim, K. & Eaton, E. Multi-agent distributed lifelong learning for collective knowledge acquisition. In Proc. 17th International Conference on Autonomous Agents and Multiagent Systems 2018 (2017).
Boyd, S. et al. Distributed optimization and statistical learning via the alternating direction method of multipliers. Found. Trends Mach. Learn. 3 , 1–122 (2011).
Mohammadi, J. & Kolouri, S. Collaborative learning through shared collective knowledge and local expertise. In 2019 IEEE 29th International Workshop on Machine Learning for Signal Processing (2019).
Wortsman, M. et al. Supermasks in superposition. Adv. Neural Inf. Process. Syst. 33 , 15173–15184 (2020).
Koster, N., Grothe, O. & Rettinger, A. Signing the supermask: keep, hide, invert. In International Conference on Learning Representations https://openreview.net/forum?id=e0jtGTfPihs (2022).
Wen, S., Rios, A., Ge, Y. & Itti, L. Beneficial perturbation network for designing general adaptive artificial intelligence systems. IEEE Trans. Neural Networks Learn. Syst. 33 , 3778–3791 (2021).
Saha, G., Garg, I. & Roy, K. Gradient projection memory for continual learning. In International Conference on Learning Representations (2021).
Choudhary, S., Aketi, S. A., Saha, G. & Roy, K. CoDeC: communication-efficient decentralized continual learning. Preprint at https://doi.org/10.48550/arXiv.2303.15378 (2023).
Singh, P., Verma, V. K., Mazumder, P., Carin, L. & Rai, P. Calibrating CNNs for lifelong learning. Adv. Neural Inf. Process. Syst. 33 , 15579–15590 (2020).
Verma, V. K., Liang, K. J., Mehta, N., Rai, P. & Carin, L. Efficient feature transformations for discriminative and generative continual learning. In Proc. IEEE/CVF Conference on Computer Vision and Pattern Recognition 13865–13875 (2021).
Ma, Z., Lu, Y., Li, W. & Cui, S. EFL: elastic federated learning on non-IID data. In Conference on Lifelong Learning Agents 92–115 (PMLR, 2022).
Shenaj, D., Toldo, M., Rigon, A. & Zanuttigh, P. Asynchronous federated continual learning. IEEE/CVF Conference on Computer Vision and Pattern Recognition Workshops (CVPRW) https://ieeexplore.ieee.org/stamp/stamp.jsp?arnumber=10208460 (2023).
Venkatesha, Y., Kim, Y., Park, H. & Panda, P. Divide-and-conquer the NAS puzzle in resource constrained federated learning systems. Neural Networks 168 , 569–579 (2023).
Usmanova, A., Portet, F., Lalanda, P. & Vega, G. A distillation-based approach integrating continual learning and federated learning for pervasive services. 3rd Workshop on Continual and Multimodal Learning for Internet of Things – Co-located with IJCAI 2021, Aug 2021, Montreal, Canada https://doi.org/10.48550/arXiv.2109.04197 (2021).
Wang, T., Zhu, J.-Y., Torralba, A. & Efros, A. A. Dataset distillation. Preprint at https://doi.org/10.48550/arXiv.1811.10959 (2018).
Cazenavette, G., Wang, T., Torralba, A., Efros, A. A. & Zhu, J.-Y. Dataset distillation by matching training trajectories. In Proc. IEEE/CVF Conference on Computer Vision and Pattern Recognition 4750–4759 (2022).
Baradad Jurjo, M., Wulff, J., Wang, T., Isola, P. & Torralba, A. Learning to see by looking at noise. Adv. Neural Inf. Process. Syst. 34 , 2556–2569 (2021).
Carta, A., Cossu, A., Lomonaco, V., Bacciu, D. & van de Weijer, J. Projected latent distillation for data-agnostic consolidation in distributed continual learning. Preprint at https://doi.org/10.48550/arXiv.2303.15888 (2023).
Teh, Y. et al. Distral: robust multitask reinforcement learning. In Advances in Neural Information Processing Systems Vol. 30 (2017).
Zheng, G., Jacobs, M. A., Braverman, V. & Parekh, V. S. Asynchronous decentralized federated lifelong learning for landmark localization in medical imaging. In International Workshop on Federated Learning for Distributed Data Minin g (2023).
Zheng, G., Lai, S., Braverman, V., Jacobs, M. A. & Parekh, V. S. A framework for dynamically training and adapting deep reinforcement learning models to different, low-compute, and continuously changing radiology deployment environments. Preprint at https://doi.org/10.48550/arXiv.2306.05310 (2023).
Zheng, G., Lai, S., Braverman, V., Jacobs, M. A. & Parekh, V. S. Multi-environment lifelong deep reinforcement learning for medical imaging. Preprint at https://doi.org/10.48550/arXiv.2306.00188 (2023).
Zheng, G., Zhou, S., Braverman, V., Jacobs, M. A. & Parekh, V. S. Selective experience replay compression using coresets for lifelong deep reinforcement learning in medical imaging. In Proc. Machine Learning Research 227, 1751–1764 (2024).
Shperberg, S. S., Liu, B. & Stone, P. Learning a shield from catastrophic action effects: never repeat the same mistake. Preprint at https://doi.org/10.48550/arXiv.2202.09516 (2022).
Shperberg, S. S., Liu, B., Allievi, A. & Stone, P. A rule-based shield: Accumulating safety rules from catastrophic action effects. In Conference on Lifelong Learning Agents 231–242 (PMLR, 2022).
Alshiekh, M. et al. Safe reinforcement learning via shielding. In Proc. 32nd AAAI Conference on Artificial Intelligence and Thirtieth Innovative Applications of Artificial Intelligence Conference and Eighth AAAI Symposium on Educational Advances in Artificial Intelligence (AAAI, 2018).
Garcıa, J. & Fernández, F. A comprehensive survey on safe reinforcement learning. J. Mach. Learn. Res. 16 , 1437–1480 (2015).
Jang, D., Yoo, J., Son, C. Y., Kim, D. & Kim, H. J. Multi-robot active sensing and environmental model learning with distributed gaussian process. IEEE Robot. Autom. Lett. 5 , 5905–5912 (2020).
Igoe, C., Ghods, R. & Schneider, J. Multi-agent active search: a reinforcement learning approach. IEEE Rob. Autom. Lett. 7 , 754–761 (2021).
Raja, G., Baskar, Y., Dhanasekaran, P., Nawaz, R. & Yu, K. An efficient formation control mechanism for multi-UAV navigation in remote surveillance. In 2021 IEEE Globecom Workshops (IEEE, 2021).
Sitzmann, V., Martel, J., Bergman, A., Lindell, D. & Wetzstein, G. Implicit neural representations with periodic activation functions. Adv. Neural Inf. Process. Syst. 33 , 7462–7473 (2020).
Yu, A., Ye, V., Tancik, M. & Kanazawa, A. pixelNeRF: neural radiance fields from one or few images. In Proc. IEEE/CVF Conference on Computer Vision and Pattern Recognition 4578–4587 (2021).
Zhang, K., Riegler, G., Snavely, N. & Koltun, V. NeRF++: analyzing and improving neural radiance fields. Preprint at https://doi.org/10.48550/arXiv.2010.07492 (2020).
Bylow, E., Sturm, J., Kerl, C., Kahl, F. & Cremers, D. Real-time camera tracking and 3D reconstruction using signed distance functions. Rob. Sci. Syst. 2 , 2 (2013).
Park, J. J., Florence, P., Straub, J., Newcombe, R. & Lovegrove, S. DeepSDF: learning continuous signed distance functions for shape representation. In Proc. IEEE/CVF Conference on Computer Vision and Pattern Recognition 165–174 (2019).
Kolouri, S., Abbasi, A., Koohpayegani, S. A., Nooralinejad, P. & Pirsiavash, H. Multi-agent lifelong implicit neural learning. IEEE Signal Process. Lett. 30 , 1812–1816 (2023).
Bortnik, J. & Camporeale, E. Ten ways to apply machine learning in the earth and space sciences. In AGU Fall Meeting Abstracts IN12A-06 (2021).
Zhang, Y., Bai, Y., Wang, M. & Hu, J. Cooperative adaptive cruise control with robustness against communication delay: an approach in the space domain. IEEE Trans. Intell. Transport. Syst. 22 , 5496–5507 (2020).
Gao, Y. & Chien, S. Review on space robotics: toward top-level science through space exploration. Sci. Rob. 2 , eaan5074 (2017).
Bornstein, B. J. et al. Autonomous exploration for gathering increased science. NASA Tech Briefs 34 (9), 10 (2010).
Swan, R. M. et al. AI4MARS: a dataset for terrain-aware autonomous driving on Mars. In Proc. IEEE/CVF Conference on Computer Vision and Pattern Recognition 1982–1991 (2021).
Bayer, T. Planning for the un-plannable: redundancy, fault protection, contingency planning and anomaly response for the mars reconnaissance oribiter mission. In AIAA SPACE 2007 Conference and Exposition 6109 (2007).
Rieke, N. et al. The future of digital health with federated learning. NPJ Dig. Med. 3 , 119 (2020).
Sheller, M. J. et al. Federated learning in medicine: facilitating multi-institutional collaborations without sharing patient data. Sci. Rep. 10 , 12598 (2020).
Moor, M. et al. Foundation models for generalist medical artificial intelligence. Nature 616 , 259–265 (2023).
Bécue, A., Praça, I. & Gama, J. Artificial intelligence, cyber-threats and industry 4.0: challenges and opportunities. Artif. Intell. Rev. 54 , 3849–3886 (2021).
Buczak, A. L. & Guven, E. A survey of data mining and machine learning methods for cyber security intrusion detection. IEEE Commun. Surv. Tutorials 18 , 1153–1176 (2015).
Shaukat, K., Luo, S., Varadharajan, V., Hameed, I. A. & Xu, M. A survey on machine learning techniques for cyber security in the last decade. IEEE Access 8 , 222310–222354 (2020).
Berman, D. S., Buczak, A. L., Chavis, J. S. & Corbett, C. L. A survey of deep learning methods for cyber security. Information 10 , 122 (2019).
Kozik, R., Choras, M. & Keller, J. Balanced efficient lifelong learning (B-ELLA) for cyber attack detection. J. Univers. Comput. Sci. 25 , 2–15 (2019).
Bernstein, D. S., Givan, R., Immerman, N. & Zilberstein, S. The complexity of decentralized control of Markov decision processes. Math. Oper. Res. 27 , 819–840 (2002).
Goldman, C. V. & Zilberstein, S. Decentralized control of cooperative systems: categorization and complexity analysis. J. Artif. Intell. Res. 22 , 143–174 (2004).
Melo, F. S., Spaan, M. T. J. & Witwicki, S. J. In Multi-Agent Systems (eds Cossentino, M. et al.) 189–204 (Springer, 2012).
Vaswani, A. et al. Attention is all you need. In Advances in Neural Information Processing Systems Vol. 30 (2017).
Khan, S. et al. Transformers in vision: a survey. ACM Comput. Surv. 54 , 200 (2022).
Bommasani, R. et al. On the opportunities and risks of foundation models. Preprint at https://doi.org/10.48550/arXiv.2108.07258 (2021).
Yang, S. et al. Foundation models for decision making: problems, methods, and opportunities. Preprint at https://doi.org/10.48550/arXiv.2303.04129 (2023).
Knight, W. OpenAI’s CEO says the age of giant AI models is already over. Wired https://www.wired.com/story/openai-ceo-sam-altman-the-age-of-giant-ai-models-is-already-over/ (17 April 2023).
Rahwan, I. et al. Machine behaviour. Nature 568 , 477–486 (2019).
Cath, C. Governing artificial intelligence: ethical, legal and technical opportunities and challenges. Phil. Trans. R. Soc. A 376 , 20180080 (2018).
Cao, Y. & Yang, J. Towards making systems forget with machine unlearning. In 2015 IEEE Symposium on Security and Privacy 463–480 (IEEE, 2015).
Nick, B. Superintelligence: Paths, Dangers, Strategies . (Oxford Univ. Press, 2014).
Marr, B. The 15 biggest risks of artificial intelligence. Forbes https://www.forbes.com/sites/bernardmarr/2023/06/02/the-15-biggest-risks-of-artificial-intelligence/?sh=309f29002706 (2 June 2023).
Bengio, Y. et al. Managing AI risks in an era of rapid progress. Preprint at https://doi.org/10.48550/arXiv.2310.17688 (2023).
Wu, C.-J. et al. Sustainable AI: environmental implications, challenges and opportunities. Proc. Mach. Learn. Syst. 4 , 795–813 (2022).
Download references
Acknowledgements
This material is based on work supported by DARPA under contracts HR00112190132, HR00112190133, HR00112190134, HR00112190135, HR00112190130 and HR00112190136. Any opinions, findings, conclusions or recommendations expressed in this material are those of the authors and do not necessarily reflect the views of DARPA. The authors would like to thank B. Bertoldson, A. Carta, B. Clipp, N. Jennings, K. Stanley, C. Ekanadham, N. Ketz, M. Paravia, M. Petrescu, T. Senator and J. Steil for constructive discussions and comments on early versions of the manuscript.
Author information
Authors and affiliations.
Computer Science Department, Loughborough University, Loughborough, UK
Andrea Soltoggio, Eseoghene Ben-Iwhiwhu, Saptarshi Nath & Christos Peridis
Computer Science Department, Rice University, Houston, TX, USA
Vladimir Braverman, Michael A. Jacobs & Guangyao Zheng
University of Pennsylvania, Philadelphia, PA, USA
Eric Eaton, Long Le & Kyle Vedder
ECS Federal, Arlington, VA, USA
Benjamin Epstein
Thomas Lord Department of Computer Science, Viterbi School of Engineering, University of Southern California, Los Angeles, CA, USA
Yunhao Ge & Laurent Itti
Department of Aeronautics and Astronautics, Massachusetts Institute of Technology, Cambridge, MA, USA
Lucy Halperin & Jonathan How
Department of Diagnostic and Interventional Imaging, The University of Texas McGovern Medical School at Houston, Houston, TX, USA
Michael A. Jacobs
The Department of Radiology and Oncology, The Johns Hopkins University School of Medicine, Baltimore, MD, USA
Smart Information Flow Technologies, Minneapolis, MN, USA
Pavan Kantharaju & David Musliner
Aurora Flight Sciences, Cambridge, MA, USA
Steven Lee & Sildomar T. Monteiro
Department of Computer Science, Vanderbilt University, Nashville, TN, USA
Xinran Liu & Soheil Kolouri
Massachusetts Institute of Technology, Cambridge, MA, USA
Sildomar T. Monteiro
Department of Electrical Engineering, Yale University, New Haven, CT, USA
Priyadarshini Panda
Department of Computer Science, University of California, Davis, Davis, CA, USA
Hamed Pirsiavash
Department of Diagnostic Radiology and Nuclear Medicine, University of Maryland School of Medicine, Baltimore, MD, USA
Vishwa Parekh
Department of Electrical and Computer Engineering, Purdue University, West Lafayette, IN, USA
Kaushik Roy
Department of Software and Information System Engineering, Ben-Gurion University, Beer Sheva, Israel
Shahaf Shperberg
University of Massachusetts, Amherst, Amherst, MA, USA
Hava T. Siegelmann
Department of Computer Science, The University of Texas at Austin, Austin, TX, USA
Peter Stone
Sony AI America, Sony AI, Austin, TX, USA
Simons Institute, University of California, Berkeley, Berkeley, CA, USA
Jingfeng Wu
Department of Electrical and Computer Engineering, University of California, Los Angeles, Los Angeles, CA, USA
You can also search for this author in PubMed Google Scholar
Contributions
All authors contributed with insights during brainstorming, ideas and writing the paper. A.S. conceived the main idea and led the integration of all contributions.
Corresponding author
Correspondence to Andrea Soltoggio .
Ethics declarations
Competing interests.
P.S. serves as the executive director of Sony AI America and receives financial compensation for this work. The terms of this arrangement have been reviewed and approved by the University of Texas at Austin in accordance with its policy on objectivity in research. All other authors declare no competing interests.
Peer review
Peer review information.
Nature Machine Intelligence thanks Senen Barro, Vincenzo Lomonaco, Xiaoying Tang, Gido van de Ven and the other, anonymous, reviewer(s) for their contribution to the peer review of this work.
Additional information
Publisher’s note Springer Nature remains neutral with regard to jurisdictional claims in published maps and institutional affiliations.
Supplementary information
Supplementary information.
Supplementary Section 1: ShELL algorithms and their implementations. Supplementary Section 2: additional technical details are provided on application scenarios and performance metrics.
Rights and permissions
Springer Nature or its licensor (e.g. a society or other partner) holds exclusive rights to this article under a publishing agreement with the author(s) or other rightsholder(s); author self-archiving of the accepted manuscript version of this article is solely governed by the terms of such publishing agreement and applicable law.
Reprints and permissions
About this article
Cite this article.
Soltoggio, A., Ben-Iwhiwhu, E., Braverman, V. et al. A collective AI via lifelong learning and sharing at the edge. Nat Mach Intell 6 , 251–264 (2024). https://doi.org/10.1038/s42256-024-00800-2
Download citation
Received : 30 April 2023
Accepted : 24 January 2024
Published : 22 March 2024
Issue Date : March 2024
DOI : https://doi.org/10.1038/s42256-024-00800-2
Share this article
Anyone you share the following link with will be able to read this content:
Sorry, a shareable link is not currently available for this article.
Provided by the Springer Nature SharedIt content-sharing initiative
Quick links
- Explore articles by subject
- Guide to authors
- Editorial policies
Sign up for the Nature Briefing newsletter — what matters in science, free to your inbox daily.

Scientists create AI models that can talk to each other and pass on skills with limited human input
Scientists modeled human-like communication skills and the transfer of knowledge between AIs — so they can teach each other to perform tasks without a huge amount of training data.
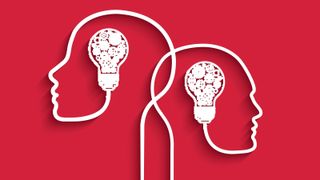
The next evolution in artificial intelligence (AI) could lie in agents that can communicate directly and teach each other to perform tasks, research shows.
Scientists have modeled an AI network capable of learning and carrying out tasks solely on the basis of written instructions. This AI then described what it learned to a “sister” AI, which performed the same task despite having no prior training or experience in doing it.
The first AI communicated to its sister using natural language processing (NLP), the scientists said in their paper published March 18 in the journal Nature .
NLP is a subfield of AI that seeks to recreate human language in computers — so machines can understand and reproduce written text or speech naturally. These are built on neural networks, which are collections of machine learning algorithms modeled to replicate the arrangement of neurons in the brain.
‘‘Once these tasks had been learned, the network was able to describe them to a second network — a copy of the first — so that it could reproduce them. To our knowledge, this is the first time that two AIs have been able to talk to each other in a purely linguistic way,’’ said lead author of the paper Alexandre Pouget , leader of the Geneva University Neurocenter, in a statement .
The scientists achieved this transfer of knowledge by starting with an NLP model called "S-Bert," which was pre-trained to understand human language. They connected S-Bert to a smaller neural network centered around interpreting sensory inputs and simulating motor actions in response.
Related: AI-powered humanoid robot can serve you food, stack the dishes — and have a conversation with you
Sign up for the Live Science daily newsletter now
Get the world’s most fascinating discoveries delivered straight to your inbox.
This composite AI — a "sensorimotor-recurrent neural network (RNN)" — was then trained on a set of 50 psychophysical tasks. These centered on responding to a stimulus — like reacting to a light — through instructions fed via the S-Bert language model.
Through the embedded language model, the RNN understood full written sentences. This let it perform tasks from natural language instructions, getting them 83% correct on average, despite having never seen any training footage or performed the tasks before.
That understanding was then inverted so the RNN could communicate the results of its sensorimotor learning using linguistic instructions to an identical sibling AI, which carried out the tasks in turn — also having never performed them before.
Do as we humans do
The inspiration for this research came from the way humans learn by following verbal or written instructions to perform tasks — even if we’ve never performed such actions before. This cognitive function separates humans from animals; for example, you need to show a dog something before you can train it to respond to verbal instructions.
While AI-powered chatbots can interpret linguistic instructions to generate an image or text, they can’t translate written or verbal instructions into physical actions, let alone explain the instructions to another AI.
However, by simulating the areas of the human brain responsible for language perception, interpretation and instructions-based actions, the researchers created an AI with human-like learning and communication skills.
— This video of a robot making coffee could signal a huge step in the future of AI robotics. Why?
— Human-like robot tricks people into thinking it has a mind of its own
— Robot hand exceptionally 'human-like' thanks to new 3D printing technique
This won't alone lead to the rise of artificial general intelligence (AGI) — where an AI agent can reason just as well as a human and perform tasks in multiple areas. But the researchers noted that AI models like the one they created can help our understanding of how human brains work.
There’s also scope for robots with embedded AI to communicate with each other to learn and carry out tasks. If only one robot received initial instructions, it could be really effective in manufacturing and training other automated industries.
‘‘The network we have developed is very small,” the researchers explained in the statement. “Nothing now stands in the way of developing, on this basis, much more complex networks that would be integrated into humanoid robots capable of understanding us but also of understanding each other.’’
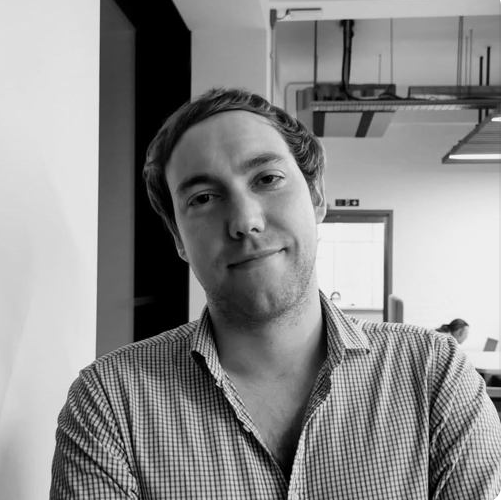
Roland Moore-Colyer is a freelance writer for Live Science and managing editor at consumer tech publication TechRadar, running the Mobile Computing vertical. At TechRadar, one of the U.K. and U.S.’ largest consumer technology websites, he focuses on smartphones and tablets. But beyond that, he taps into more than a decade of writing experience to bring people stories that cover electric vehicles (EVs), the evolution and practical use of artificial intelligence (AI), mixed reality products and use cases, and the evolution of computing both on a macro level and from a consumer angle.
MIT scientists have just figured out how to make the most popular AI image generators 30 times faster
Researchers gave AI an 'inner monologue' and it massively improved its performance
Where does the solar system end?
Most Popular
By Keumars Afifi-Sabet March 29, 2024
By Carys Matthews March 29, 2024
By Jamie Carter March 29, 2024
By Nicoletta Lanese March 28, 2024
By Kristina Killgrove March 28, 2024
By Jennifer Nalewicki March 28, 2024
By Joe Rao March 28, 2024
By Sascha Pare March 28, 2024
By Lobato Felizola March 28, 2024
- 2 James Webb telescope confirms there is something seriously wrong with our understanding of the universe
- 3 'You could almost see and smell their world': Remnants of 'Britain's Pompeii' reveal details of life in Bronze Age village
- 4 Hair-straightening cream tied to woman's repeated kidney damage
- 5 Future quantum computers will be no match for 'space encryption' that uses light to beam data around — with the 1st satellite launching in 2025
- 2 9,000-year-old rock art discovered among dinosaur footprints in Brazil
- 3 The 7 most powerful supercomputers in the world right now
- 4 Single enormous object left 2 billion craters on Mars, scientists discover
Help | Advanced Search
Computer Science > Machine Learning
Title: accelerating scientific discovery with generative knowledge extraction, graph-based representation, and multimodal intelligent graph reasoning.
Abstract: Leveraging generative Artificial Intelligence (AI), we have transformed a dataset comprising 1,000 scientific papers into an ontological knowledge graph. Through an in-depth structural analysis, we have calculated node degrees, identified communities and connectivities, and evaluated clustering coefficients and betweenness centrality of pivotal nodes, uncovering fascinating knowledge architectures. The graph has an inherently scale-free nature, is highly connected, and can be used for graph reasoning by taking advantage of transitive and isomorphic properties that reveal unprecedented interdisciplinary relationships that can be used to answer queries, identify gaps in knowledge, propose never-before-seen material designs, and predict material behaviors. We compute deep node embeddings for combinatorial node similarity ranking for use in a path sampling strategy links dissimilar concepts that have previously not been related. One comparison revealed structural parallels between biological materials and Beethoven's 9th Symphony, highlighting shared patterns of complexity through isomorphic mapping. In another example, the algorithm proposed a hierarchical mycelium-based composite based on integrating path sampling with principles extracted from Kandinsky's 'Composition VII' painting. The resulting material integrates an innovative set of concepts that include a balance of chaos/order, adjustable porosity, mechanical strength, and complex patterned chemical functionalization. We uncover other isomorphisms across science, technology and art, revealing a nuanced ontology of immanence that reveal a context-dependent heterarchical interplay of constituents. Graph-based generative AI achieves a far higher degree of novelty, explorative capacity, and technical detail, than conventional approaches and establishes a widely useful framework for innovation by revealing hidden connections.
Submission history
Access paper:.
- Other Formats

References & Citations
- Google Scholar
- Semantic Scholar
BibTeX formatted citation

Bibliographic and Citation Tools
Code, data and media associated with this article, recommenders and search tools.
- Institution
arXivLabs: experimental projects with community collaborators
arXivLabs is a framework that allows collaborators to develop and share new arXiv features directly on our website.
Both individuals and organizations that work with arXivLabs have embraced and accepted our values of openness, community, excellence, and user data privacy. arXiv is committed to these values and only works with partners that adhere to them.
Have an idea for a project that will add value for arXiv's community? Learn more about arXivLabs .
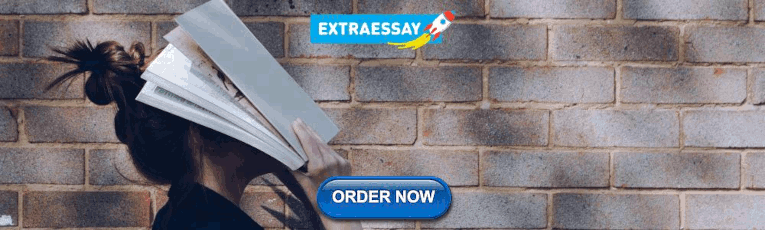
IMAGES
VIDEO
COMMENTS
Large amounts of adequately structured data are produced by customer service, for example, when consumers ask queries and support teams respond. According to F33 (2021), the harsh truth is that, despite the fact that artificial intelligence (AI) and machine learning (ML) are currently very trendy terms and that almost every tech company's ...
Difference between AI and ML: The goal of Artificial Intelligence is to create a machine that can mimic a human mind, and it needs learning capabilities as well. However, it is more than just ...
106 Artificial Intelligence Essay Topics & Samples. Updated: Nov 8th, 2023. 7 min. In a research paper or any other assignment about AI, there are many topics and questions to consider. To help you out, our experts have provided a list of 76 titles, along with artificial intelligence essay examples, for your consideration.
Considering that Machine Learning (ML) and AI are apt to reach unforeseen levels of accuracy and efficiency, this special issue sought to promote research on AI and ML seen as functions of data-driven innovation and digital transformation. ... A variety of innovative topics are included in the agenda of the published papers in this special ...
The 2021 report is the second in a series that will be released every five years until 2116. Titled "Gathering Strength, Gathering Storms," the report explores the various ways AI is increasingly touching people's lives in settings that range from movie recommendations and voice assistants to autonomous driving and automated medical ...
Artificial intelligence (AI) and machine learning are often used interchangeably, but machine learning is a subset of the broader category of AI. Put in context, artificial intelligence refers to the general ability of computers to emulate human thought and perform tasks in real-world environments, while machine learning refers to the ...
However, recent technological rapid developments and new approaches to computing have set education and learning in a completely new context. The panel of 22 invited experts in learning sciences, education, and computing (Roschelle et al., 2020, p.8) assessed the future and new designs of AI in learning and education: "These design concepts expand beyond familiar ideas of technology ...
The simplest way to understand how AI and ML relate to each other is: AI is the broader concept of enabling a machine or system to sense, reason, act, or adapt like a human. ML is an application of AI that allows machines to extract knowledge from data and learn from it autonomously. One helpful way to remember the difference between machine ...
In simplest terms, AI is computer software that mimics the ways that humans think in order to perform complex tasks, such as analyzing, reasoning, and learning. Machine learning, meanwhile, is a subset of AI that uses algorithms trained on data to produce models that can perform such complex tasks. Most AI is performed using machine learning ...
Artificial intelligence (AI), particularly, machine learning (ML) have grown rapidly in recent years in the context of data analysis and computing that typically allows the applications to function in an intelligent manner [].ML usually provides systems with the ability to learn and enhance from experience automatically without being specifically programmed and is generally referred to as the ...
Decision-making on numerous aspects of our daily lives is being outsourced to machine-learning (ML) algorithms and artificial intelligence (AI), motivated by speed and efficiency in the decision ...
Higher education perceptions of artificial intelligence. Studies have explored the diverse functionalities of these AI tools and their impact on writing productivity, quality, and students' learning experiences. The integration of Artificial Intelligence (AI) in writing academic essays has become a significant area of interest in higher education.
Objective is to maximize accuracy. Artificial intelligence uses logic and decision tree. Machine learning uses statistical models. AI is concerned with knowledge dissemination and conscious Machine actions. ML is concerned with knowledge accumulation. Focuses on giving machines cognitive and intellectual capabilities similar to thone of humans.
Machine learning is a subfield of artificial intelligence that gives computers the ability to learn without explicitly being programmed. "In just the last five or 10 years, machine learning has become a critical way, arguably the most important way, most parts of AI are done," said MIT Sloan professor.
Introduction. Originally coined in the 1950s, the term "artificial intelligence" initially began as the simple theory of human intelligence being exhibited by machines [1•].In 1976, Jerrold S. Maxmen foretold that artificial intelligence (AI) would bring about the "post-physician era" in the twenty-first century [2, 3].In today's era of rapid technological advancement and ...
In the evolution of artificial Intelligence (AI) and machine learning (ML), reasoning, knowledge representation, planning, learning, natural language processing, perception, and the ability to move and manipulate objects have been widely used. These features enable the creation of intelligent mechanisms for decision support to overcome the limits of human knowledge processing. In addition, ML ...
MACHINE LEARNING. Machine learning (ML) is a sub-set of artificial intelligence (AI) and is generally understood as the ability of the system to make predictions or draw conclusions, based on the analysis of a large historical data set. At its most basic level, machine learning refers to any type of computer program that can "learn" by ...
Simply put, AI refers to the science and engineering of making intelligent machines, through algorithms or a set of rules, which the machine follows to mimic human cognitive functions, such as learning and problem solving. 9 AI systems have the potential to anticipate problems or deal with issues as they come up and, as such, operate in an ...
1. Introduction. Artificial intelligence (AI), machine learning (ML), and deep learning (DL) are all important technologies in the field of robotics [1].The term artificial intelligence (AI) describes a machine's capacity to carry out operations that ordinarily require human intellect, such as speech recognition, understanding of natural language, and decision-making.
Diffusion models currently dominate the field of data-driven image synthesis with their unparalleled scaling to large datasets. Ranked #1 on Image Generation on ImageNet 512x512. Image Generation Philosophy. 214. 1.30 stars / hour. Paper. Code.
Machine learning is the ability of a machine to improve its performance based on previous results. Machine learning methods enable computers to learn without being explicitly programmed and have ...
500+ Words Essay on Artificial Intelligence. Artificial Intelligence refers to the intelligence of machines. This is in contrast to the natural intelligence of humans and animals. With Artificial Intelligence, machines perform functions such as learning, planning, reasoning and problem-solving.
that by 2022, AI and ML will create 133 million new jobs while displac ing 75 million (WEF, 2020). Artificial. intelligence (AI) and machine learning (ML) are rapidly changing the way businesses ...
The perspective of such a collective AI is becoming more realistic thanks to recent advances in fields such as lifelong learning (LL) 8,9,10,11,12, lifelong reinforcement learning 13,14, federated ...
NLP is a subfield of AI that seeks to recreate human language in computers — so machines can understand and reproduce written text or speech naturally.
Download PDF Abstract: Using generative Artificial Intelligence (AI), we transformed a set of 1,000 scientific papers in the area of biological materials into detailed ontological knowledge graphs, revealing their inherently scale-free nature. Using graph traversal path detection between dissimilar concepts based on combinatorial ranking of node similarity and betweenness centrality, we reveal ...
To address these challenges, we (1) fitted machine learning models to a large longitudinal dataset with the goal of predicting individual electrode thresholds and deactivation as a function of stimulus, electrode, and clinical parameters ('predictors') and (2) leveraged explainable artificial intelligence (XAI) to reveal which of these ...
With machine learning, IT teams can automate, detect, invest, and organize the incident analysis response process. The process works by using AI to ingest company data from multiple sources and ...
Essay; Drone Swarms Are About to Change the Balance of Military Power On today's battlefields, drones are a manageable threat. When hundreds of them can be harnessed to AI technology, they will ...